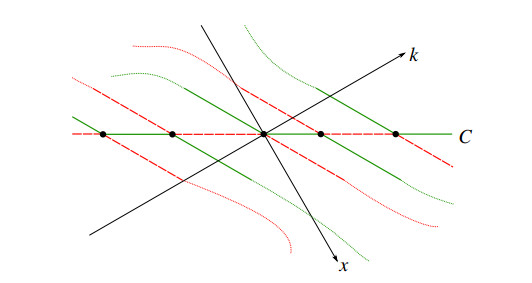
In this paper, we study a class of equations representing nonlinear diffusion on networks. A particular instance of our model could be seen as a network equivalent of the porous-medium equation. We are interested in studying perturbations of such a system and describing the consensus dynamics. The nonlinearity of the equations gives rise to potentially intricate structures of equilibria that could intersect the consensus space, creating singularities. For the unperturbed case, we characterize the sets of equilibria by exploiting the symmetries under group transformations of the nonlinear vector field. Under small perturbations, we obtain a slow-fast system. Thus, we analyze the slow-fast dynamics near the singularities on the consensus space. The analysis at this stage is carried out for complete networks, allowing a detailed characterization of the system. We provide a linear approximation of the intersecting branches of equilibria at the singular points; as a consequence, we show that, generically, the singularities on the consensus space turn out to be transcritical. We prove under local assumptions the existence of canard solutions. For generic graph structures, assuming more strict conditions on the perturbation, we prove the existence of a maximal canard, which coincides with the consensus subspace. In addition, we validate by numerical simulations the principal findings of our main theory, extending the study to non-complete graphs. Moreover, we show how the delayed loss of stability associated with the canards induces transient spatio-temporal patterns.
Citation: Riccardo Bonetto, Hildeberto Jardón Kojakhmetov. Nonlinear diffusion on networks: Perturbations and consensus dynamics[J]. Networks and Heterogeneous Media, 2024, 19(3): 1344-1380. doi: 10.3934/nhm.2024058
[1] | Yicheng Liu, Yipeng Chen, Jun Wu, Xiao Wang . Periodic consensus in network systems with general distributed processing delays. Networks and Heterogeneous Media, 2021, 16(1): 139-153. doi: 10.3934/nhm.2021002 |
[2] | Wenlian Lu, Fatihcan M. Atay, Jürgen Jost . Consensus and synchronization in discrete-time networks of multi-agents with stochastically switching topologies and time delays. Networks and Heterogeneous Media, 2011, 6(2): 329-349. doi: 10.3934/nhm.2011.6.329 |
[3] | GuanLin Li, Sebastien Motsch, Dylan Weber . Bounded confidence dynamics and graph control: Enforcing consensus. Networks and Heterogeneous Media, 2020, 15(3): 489-517. doi: 10.3934/nhm.2020028 |
[4] | Marina Dolfin, Mirosław Lachowicz . Modeling opinion dynamics: How the network enhances consensus. Networks and Heterogeneous Media, 2015, 10(4): 877-896. doi: 10.3934/nhm.2015.10.877 |
[5] | Yuntian Zhang, Xiaoliang Chen, Zexia Huang, Xianyong Li, Yajun Du . Managing consensus based on community classification in opinion dynamics. Networks and Heterogeneous Media, 2023, 18(2): 813-841. doi: 10.3934/nhm.2023035 |
[6] | Mattia Bongini, Massimo Fornasier, Oliver Junge, Benjamin Scharf . Sparse control of alignment models in high dimension. Networks and Heterogeneous Media, 2015, 10(3): 647-697. doi: 10.3934/nhm.2015.10.647 |
[7] | Chaoqun Huang, Nung Kwan Yip . Singular perturbation and bifurcation of diffuse transition layers in inhomogeneous media, part II. Networks and Heterogeneous Media, 2015, 10(4): 897-948. doi: 10.3934/nhm.2015.10.897 |
[8] | Zhuchun Li, Xiaoping Xue, Seung-Yeal Ha . A revisit to the consensus for linearized Vicsek model under joint rooted leadership via a special matrix. Networks and Heterogeneous Media, 2014, 9(2): 335-351. doi: 10.3934/nhm.2014.9.335 |
[9] | Luca Di Persio, Giacomo Ziglio . Gaussian estimates on networks with applications to optimal control. Networks and Heterogeneous Media, 2011, 6(2): 279-296. doi: 10.3934/nhm.2011.6.279 |
[10] | Juan Manuel Pastor, Javier García-Algarra, José M. Iriondo, José J. Ramasco, Javier Galeano . Dragging in mutualistic networks. Networks and Heterogeneous Media, 2015, 10(1): 37-52. doi: 10.3934/nhm.2015.10.37 |
In this paper, we study a class of equations representing nonlinear diffusion on networks. A particular instance of our model could be seen as a network equivalent of the porous-medium equation. We are interested in studying perturbations of such a system and describing the consensus dynamics. The nonlinearity of the equations gives rise to potentially intricate structures of equilibria that could intersect the consensus space, creating singularities. For the unperturbed case, we characterize the sets of equilibria by exploiting the symmetries under group transformations of the nonlinear vector field. Under small perturbations, we obtain a slow-fast system. Thus, we analyze the slow-fast dynamics near the singularities on the consensus space. The analysis at this stage is carried out for complete networks, allowing a detailed characterization of the system. We provide a linear approximation of the intersecting branches of equilibria at the singular points; as a consequence, we show that, generically, the singularities on the consensus space turn out to be transcritical. We prove under local assumptions the existence of canard solutions. For generic graph structures, assuming more strict conditions on the perturbation, we prove the existence of a maximal canard, which coincides with the consensus subspace. In addition, we validate by numerical simulations the principal findings of our main theory, extending the study to non-complete graphs. Moreover, we show how the delayed loss of stability associated with the canards induces transient spatio-temporal patterns.
Diffusion is certainly among the most renowned subjects in dynamical systems. The ordinary differential equation ˙x=−Lx, where L is the graph Laplacian, describes a (linear) diffusion process on a network. In fact, the Laplacian matrix plays the role of a discrete counterpart of the Laplacian differential operator, Δ, present for example in the heat equation, ˙u=Δu. A lot of effort has been made to precisely analyze the behavior of Laplacian systems, especially for the linear case [48,57,58]. More recently, nonlinear extensions of the Laplacian dynamics have been explored [1,3,31,32,55].
In the present paper, we aim to analyze a class of nonlinear Laplacian systems defined by
˙x=−LF(x)+ϵH(x,Λ), | (1.1) |
where x=x(t)∈Rn, F(x) is a nonlinear vector field, 0<ϵ≪1 is a small parameter, and H(x,Λ) is a perturbation with Λ∈Rp an extra set of parameters; more precise definitions follow in the next sections. Eq (1.1) describes a nonlinear diffusion process on a network. In the literature, such systems are also known as Absolute Laplacian Flows (ALFs) [3,55]. Similarities with the continuum case can be found by looking at drift-diffusion equations [11], in particular with the porous-medium equation, ˙u=Δum [56]. The porous-medium equation has several potential applications, for example: flow of gases [38,41], theoretical biology [54], and particle-based models [28] (where the power considered is m=2). A network model for the porous-medium equation has been proposed in [19]; similar systems have also been studied in [9,47]. Let us moreover notice that by considering the rewriting F(x)=:K(x)x one can interpret the matrix K(x) as a state-dependent diffusion coefficient, leading, in turn, to another interpretation in terms of the network structure. In fact, we can define a state-dependent Laplacian, LK(x):=LK(x), which can be seen either as the Laplacian of a directed graph under the definition of out-degree Laplacian [2,46], or as a particular case of the Laplacian tensor for consensus models on hypergraphs [52]. Nonlinear diffusion processes have also been considered in discrete time systems, in particular for lattice networks [45].
Within the context of Laplacian dynamics the diffusion of 'information' among the agents can lead the system to reach a common state, referred to as consensus. As such, Laplacian systems find a remarkable application in consensus problems [15,48,50], which have been extensively studied for their importance in several branches of science, e.g., synchronization, opinion dynamics, coordination of robots, rendezvous problems, among many others.
One significant challenge in studying networked dynamical systems is the high dimensionality of the problem. Furthermore, the nonlinearities inherent in such models contribute to an even greater degree of complexity. The contributions of this paper address such challenges in particular contexts where the model we consider is a nonlinear version of the well-known consensus dynamics. For complete graphs, under appropriate conditions accounting for the symmetries of the network system, we provide a reduction to a planar system, see Lemma 4. Hence, in this particular setup, a large dimensional nonlinear system can be studied with low-dimensional techniques of dynamical systems. For example, by exploiting the aforementioned reduction, we prove that, generically, the singularities along the consensus space are transcritical; see Proposition 8. Later, for nondegenerate transcritical singularities, we provide conditions for the existence of ''canard solutions'' in Proposition 10. These canards are relevant in the context of perturbation problems since they are solutions that are not expected from zeroth-order approximations. Although the previous results are for complete graphs, we also notice that, under some conditions, the canard condition can be provided for more general graph structures; see Proposition 11. Finally, the insights that we gain from our analysis are used in some numerical experiments showing some intriguing phenomena that we associate with the canards; see Section 6.2.
The paper is structured as follows: In Section 2, we define the (unperturbed) ALF and exploit some general properties. Section 3 is dedicated to understanding the role of symmetries in ALFs; for a brief introduction to the main concepts related to symmetries see Appendix 8. In Section 4, we introduce a perturbation term leading to Eq (1.1). As we will see, the perturbations, in general, give rise to a slow-fast behavior of the system. A brief summary of the main ideas of singular perturbation theory useful for the main analysis can be found in Appendix 9. The slow-fast consensus dynamics of ALFs with complete graph structure is studied in Section 5, where we also provide conditions under which systems with arbitrary graph structure admit a maximal canard. In Section 6, we explore numerically different network structures, spatio-temporal configurations, and we describe the effects of canard conditions in different setups. A brief discussion and the conclusions are drawn in Section 7.
From a mathematical point of view, an undirected network structure can be described by a graph, G={V,E}, which consists of a finite set of nodes (or vertices) V={1,...,n}, and a set of edges eij∈E, where each eij represents a connection between the node j and i [24]. Furthermore, if we assign a set of weights W to the graph G, then we obtain a weighted graph, Gw={V,E,W}. We assume (unless otherwise stated) that the weights, wij∈W, are positive reals. Such a choice is natural both for the algebraic properties that follow from it [5], and for the modeling perspective, since often the weights represent some measure of distance or strengths of the coupling [42]. In other contexts, negative weights are also considered [53], for example in models representing inhibition or antagonism; in this paper we do not consider such situations. The edges and the weights are in one-to-one correspondence, in fact, we assign to each edge eij a weight wij. Throughout this paper, we consider only undirected graphs. Therefore, we have that the weights are symmetric, that is wij=wji.
Definition 1. A simple graph is an undirected graph without self-loops or multiple edges.
Unless otherwise stated, we consider simple graphs. For weighted graphs, we assume a simple unweighted structure, i.e., Gw∖W is a simple graph. Moreover, we make no nomenclature distinction between graphs and weighted graphs with unit weights, because the algebraic structures in such cases are exactly the same.
We now recall a few well-known concepts of graph theory [7,24,42]. A path is a sequence of edges that joins a sequence of distinct vertices. A connected component of a graph is a sub-graph such that every pair of nodes is connected by a path. A relevant class of graphs is given by the complete graphs.
Definition 2. A complete graph with n nodes, denoted by Kn, is a graph such that for all pairs of nodes there is an edge connecting them, i.e., ∀i,j∈V, with i≠j, ∃ eij∈E.
Given a graph it is possible to define some algebraic structures on it. We recall here the ones that are useful for the purpose of the paper.
● The adjacency matrix, A, of a graph is the n×n matrix with components Aij=wij if eij∈E, and Aij=0 if eij∉E.
● The degree matrix, Δ, of a graph is the n×n diagonal matrix with (diagonal) components Δii=∑jwij.
● The Laplacian matrix, L, of a graph is the n×n matrix defined by L:=Δ−A.
For simple graphs, let us recall that the Laplacian matrix, L, is symmetric, degenerate and semi-positive definite; moreover, the following proposition holds [5].
Proposition 1. The algebraic multiplicity of the 0 eigenvalue of L, μa(0), is equal to the number of connected components of the graph. Furthermore, the vector 1=(1,...,1)⊺ is an eigenvector of L with eigenvalue 0, i.e., 1∈ker(L).
We now introduce the class of systems we are going to investigate in the present paper. Let us assign to each node of a graph, i∈V, a state xi∈R and a smooth function
f:R→Rxi↦f(xi), |
which we call the response function. So, given the set of nodes V, we obtain a state vector x=(x1,...,xn)⊺, and a response vector field F(x)=(f(x1),…,f(xn))⊺. An Absolute Laplacian Flow [3,55] is defined by the equation
˙x=−LF(x). | (2.1) |
Remark 1. Due to Proposition 1, the Laplacian decomposes into a direct sum L=L(1)⊕⋯⊕L(μa(0)), and, therefore, an ALF decomposes in μa(0) independent systems. So, in general, we consider connected graphs.
Note that if we choose the response vector field to be the identity, i.e., F(x)=x, then Eq (2.1) becomes the widely studied (linear) Laplacian dynamics, ˙x=−Lx, [48,57,58]. Indeed, the ALF is a direct nonlinear generalization of the linear Laplacian dynamics [1,3,31,55]. ALFs have received considerably less attention than the linear Laplacian dynamics, although they have strong relations with consensus problems. For example, ALFs have been considered as models for nonlinear communications protocols in [43,61].
An important observation is that, in contrast to linear Laplacian dynamics and depending on the response function, ALFs may exhibit bifurcations of the consensus state, see (2.3). Therefore, our objective is to characterize the dynamic behavior near consensus for such systems under small perturbations.
ALFs have some generic properties derived from the symmetric and degenerate structure of the Laplacian matrix. First, notice that since 1∈kerL, then for any h∈Cm(Rn,R), m≥3, one has that L(F(x)+h(x)1)=LF(x), meaning that ALFs are invariant under response field transformations F(x)↦F(x)+h(x)1. As a consequence, we have that the response vector field is not unique: in fact, there exists an infinite set of vector fields leading to the same dynamics. This freedom can be used in practice to choose the "simplest" response field. Such a property reminds in some way of a gauge freedom [37], where one can choose among a class of response fields F(h)(x)=F(x)+h(x)1 without affecting the evolution equation (2.1). Another important property of ALFs is the existence of a constant of motion.
Lemma 1. Given an ALF (2.1), there exists a constant of motion k,
k=⟨1,x⟩, | (2.2) |
where ⟨⋅,⋅⟩ is the usual Euclidean scalar product in Rn.
Proof. The time evolution of k is given by ˙k=⟨1,˙x⟩. Then we have ⟨1,˙x⟩=−1⊺LF(x)=−(L1)⊺F(x)=0. Thus, k is a constant (in time) value.
It is well known that the constant of motion k is related to the arithmetic mean of the states, k=n⟨x⟩, where ⟨x⟩:=⟨1,x⟩/n. Thus, we can interpret the presence of the constant of motion k as a conservation law for ⟨x⟩. More generally, every quantity of the form ck, with c a constant, is a constant of motion for an ALF.
Lemma 2. The set of equilibria of an ALF (2.1) is given by
E:={x∈Rn | f(x1)=⋯=f(xn)}. |
Proof. In order to have an equilibrium for Eq (2.1) we need F(x)∈ker(L), which means f(x1)=⋯=f(xn), implying in turn that x is an equilibrium if and only if x∈E.
Corollary 1. The set
C:={x∈Rn | x1=⋯=xn} | (2.3) |
is a subset of equilibria of system (2.1), i.e., C⊆E.
The set C is the so-called consensus space [32,48,50]. As we already mentioned, one of our goals is to characterize the behavior near the consensus space (2.3). Our characterization aims to be qualitative, in the sense of topological equivalence [27,62].
Lemma 3. The consensus space can be parametrized by the constant k, i.e.,
C={x∈Rn | x1=⋯=xn=kn, k∈R}. |
Proof. Follows from straightforward computations.
From the previous lemma, we denote a parametrized point in C by x∗k=(x∗k,…,x∗k)⊺, where
x∗k=kn. | (2.4) |
Notice that, since the system has a constant of motion, the evolution is actually restricted to a family of (n−1)-dimensional hyperplanes
Pk:={x∈Rn | ⟨1,x⟩=k}. |
It is known that for linear Laplacian dynamics, systems associated with weighted graphs are topologically equivalent to the corresponding unweighted ones [8]. An important observation is that ALFs on weighted and unweighted graphs are equivalent near consensus on Pk.
Definition 3. Two vector fields, X,Y, are said to be topologically equivalent if there exists a homeomorphism which takes orbits of X to orbits of Y, preserving directions but not necessarily time parametrisation.
Proposition 2. For any f and k fixed, in a small neighborhood of x∗k∈C and restricted to Pk, the weighted, ˙x=−LwF(x), and the unweighted, ˙x=−LF(x), ALFs are topologically equivalent.
Proof. Let Lw be the Laplacian of a weighted graph Gw. Then the Jacobian of the associated ALF restricted to the consensus space is given by −LwDF(x∗k), where DF(x∗k) is the Jacobian of F(x) evaluated on the consensus set parametrized by k. On the other hand, by setting all the weights to one on Gw we obtain the simple graph G. Thus, for the ALF with unweighted graph G the Jacobian is −LDF(x∗k), where L is the Laplacian matrix associated to G. We notice that DF(x∗k)=Id dxf(x∗k), where dxf is a short-hand notation for the derivative of f with respect to x, and Id is the n×n identity matrix. So, the two matrices to compare are −dxf(x∗k)Lw and −dxf(x∗k)L. We remark that −dxf(x∗k) is a common scalar factor and that the matrices Lw and L do not depend on x∗k.
Let us recall that the Laplacian matrix is a semi-positive definite matrix [5], which means that its eigenvalues are all greater than or equal to zero. Such a property holds for both simple graphs and positive-weighted graphs. Moreover, since in our context Lw and L are symmetric, we can transform (by a similarity transformation) the Jacobians to the diagonal forms
−dxf(x∗)diag(0,μ1,…,μn−1)and−dxf(x∗)diag(0,λ1,…,λn−1), |
where {0,μ1,…,μn−1} are the eigenvalues of Lw, and {0,λ1,…,λn−1} are the eigenvalues of L; in both cases the non-zero eigenvalues are positive. Moreover, since the 0 eigenvalue is associated to the one-dimensional set of equilibria C (Proposition 1 and Corollary 1), we can reduce the analysis to the hyperplane Pk, which leads to the (n−1)-dimensional diagonal matrices
−dxf(x∗k)diag(μ1,…,μn−1)and−dxf(x∗k)diag(λ1,…,λn−1), | (2.5) |
where x∗k is now a parameter. Whenever the common term dxf(x∗k) is nonzero, we have that both matrices have eigenvalues with the same sign. Therefore, topological equivalence follows from the Hartman-Grobman Theorem. Actually, it is possible to explicitly compute the homeomorphism between the two systems described by Eq (2.5). Since the matrices are diagonal, effectively, we compute n−1 homeomorphisms between one-dimensional systems. The flows of the i-th components are respectively
xμi(t,x0):=x0exp(−dxf(x∗k)μit)andxλi(t,x0):=x0exp(−dxf(x∗k)λit). |
Thus, the homeomorphism, z:R→R, such that z(xμi(t,x0))=xλi(t,z(x0)), is given by
z(x)={xλiμiifx≥0,−|x|λiμiifx<0. | (2.6) |
It is straightforward to check that Eq (2.6) is a homeomorphism of the real line, as it is also proven in [29]. Notice that the transformation (2.6) does not depend on the common factor of the systems (2.5), and therefore, it holds also when −dxf(x∗k)=0.
Remark 2. Having in mind Proposition 2, from now on we assume that ALFs have all weights equal to one, i.e., they are defined by a simple (unweighted) graph G.
Notice that the topological equivalence described by Proposition 2, defines a local equivalence close to the consensus space. However, in what follows the assumption of a simple graph structure is global. This is motivated by the later analysis that is dedicated to the consensus space, which is the focus of the paper. The reader should keep in mind that away of the consensus space the equivalence is not proven, and, therefore, does not apply in general. The results we stated until this point rely mainly on the algebraic properties of the Laplacian. For simple graphs, together with Proposition 2, it is particularly useful to exploit the symmetry properties induced by the graph, which in turn can provide insightful information for the ALF.
In the following sections, we are going to look at how the symmetry properties of the response function and of the graph affect the equilibria and the dynamics of ALFs. Some fundamental notions of group theory and its applications are contained in Appendix A.
Corollary 1 shows that the consensus space is always a subset of equilibria for (2.1). Now, we show that, starting from the consensus space, the group transformations leaving the response function invariant induce new equilibria.
Proposition 3. Let f be a Γ-invariant response function. Then, the group Γn:=Γ×⋯×Γ⏟n-times maps the equilibria coinciding with the consensus space, C, to equilibria, i.e.,
Γn(C)⊆E. |
Proof. Let us consider −LF(Γnx∗), where x∗∈C. We recall that by definition every component of the vector field F has the same response function f. Also, each response function is invariant under Γ, i.e., f(Γx)=f(x). So we have that F(Γnx∗)=(f(Γx∗1),…,f(Γx∗n))⊺=(f(x∗1),…,f(x∗n))⊺=F(x∗). Therefore −LF(Γnx∗)=−LF(x∗)=0.
Remark 3. From Proposition 3 it follows that any subgroup of Γn maps C to equilibria.
The invariance of the response function leads to a richer structure of the equilibria, similarly to what happens for equivariant bifurcations [12,26]. Such a richer structure can give rise, for example, to bipartite consensus [3,39,64] where the agents converge to two separate clusters of consensus. A simple exemplification of the appearance of bipartite consensus in ALFs is given by considering the response function f(x)=x2. It is clear that the symmetry group for such a function is Z2, in particular we are considering the representation {1,−1}. From Proposition 3 we have that the set of equilibria consists of all the possible combinations ±x1=±x2=⋯=±xn, and, therefore, we have bipartite consensus among the potential equilibria of the system. Let us notice that the linear set-up for bipartite consensus requires negative weights on the network structure in order to obtain such a result, while for ALFs bipartite consensus follows from nonlinearity, and symmetry properties of the response function. It is interesting to notice that the lines of bipartite consensus, ±x1=±x2=⋯=±xn, arising from generic even response functions are saddles; this property follows from straightforward computations of the Jacobian.
Since we are considering systems with a network structure, the symmetry group associated with the equivariance properties, i.e., the automorphism group of the graph, denoted by Aut(G), is a subgroup of the symmetric group over n elements, denoted by Sn. Moreover, if an ALF is Sn⊇Γ-equivariant, it follows that it is also equivariant under any subgroup Σ⊂Γ. Therefore, Fix(Σ) is also a flow-invariant set, and Fix(Γ)⊆Fix(Σ). From these arguments, if we are considering a Γ-equivariant ALF, the analysis of the dynamics can be reduced to the fixed-point spaces Fix(Σ), for all subgroups Σ of Γ. Considering that the phase space of an ALF is Rn, when acting on a state, x, we use the standard/permutation representation of Sn in Rn. An element of the standard representation is called a permutation matrix.
Definition 4. A permutation matrix σ∈Sn is an n×n matrix with components
σij={1ifσ(i)=j,0otherwise, |
where the expression σ(i)=j is the abstract action of the group element σ on an element of the vertex set V={1,…,n}, meaning that the component σij is 1 if the vertex i is permuted with the vertex j, and is zero otherwise.
From now on, if not otherwise explicitly mentioned, when we write the action of a permutation group, we implicitly consider the standard representation.
Proposition 4. G-ALFs are Aut(G)-equivariant, where Aut(G) is a subgroup of Sn in the standard representation.
Proof. Let σ∈Aut(G) be an n×n permutation matrix. We start by applying a permutation to the vector field, σLF(x). Let us notice that [L,σ]=0 for all σ∈Aut(G), where [⋅,⋅] is the commutator, as it follows from the definition of symmetry for a graph (Definition 9, Appendix A). Thus, we have that σLF(x)=LσF(x). Notice that a permutation applied to the vector field F(x)=(f(x1),…,f(xn))⊺ exchanges the order of the components, but since the functions are indistinguishable we have that σF(x)=F(σx). Therefore, σLF(x)=LF(σx), which proves the statement.
Remark 4. Note that the action of Aut(G)⊆Sn on the (nonlinear) vector field F(x) is linear.
Proposition 5. Let Aut(G) be an irreducible subgroup of Sn in the standard representation. If Ord(Aut(G))≥n then Fix(Aut(G))=C.
Proof. Let Ord(Aut(G))=k≥n. The fixed-point space is obtained by considering the equations
σ(1)x=⋯=σ(k)x, | (3.1) |
where σ(s)∈Aut(G), s=1,…,k. Suppose, by contradiction, that Eq (3.1) does not fix all the components to the same value, but m<n components are fixed to one common value and the other n−m components are fixed to a different common value, i.e., Eq (3.1) leads to
x1=⋯=xm,xm+1=⋯=xn. |
As a consequence, we have that the permutation matrices σ(s) can be written as follows:
(σ(s)11…σ(s)1m0…0⋮⋱⋮⋮⋱⋮σ(s)m1…σ(s)mm0…00…0σ(s)(m+1)(m+1)…σ(s)(m+1)n⋮⋱⋮⋮⋱⋮0…0σ(s)n(m+1)…σ(s)nn), |
which is a block form. This would imply that the representation is decomposable and therefore not irreducible, which is a contradiction. The case where the components are fixed to a number of distinct values l>2 is analogous, and would give rise to block matrices with l blocks. In turn, such block matrices would be associated to a decomposable representation, leading again to a contradiction.
We have established a link between ALFs and equivariant systems. Let us notice that, if we have a dynamical system equivariant under a finite group, such dynamical system can be seen as a cell-network [25]. A cell-network is a system of differential equations symmetric under a group(oid) associated to a network that could have directed and multiple edges. So, there is a strict correspondence between cell-networks and the ALF framework. For example, a Kn-ALF is a cell-network with complete graph structure, Kn. This alternative point of view provides an extra tool in the analysis of ALFs. Indeed, from Proposition 12 it follows that Fix(Aut(G)) is flow-invariant, and so, in turn, the consensus space is an invariant space for a Kn-ALF, or in the language of cell-networks it is a pattern of synchrony, which is already known from Corollary 1. Actually, Corollary 1 not only holds for all ALFs, but also tells us that the consensus space consists of equilibria of the system, and is not just an invariant set. However, Proposition 5 does not rely on the algebraic structure of the Laplacian, it is a general result for a class of equivariant systems. Let us notice that the results following from equivariance are, in general, more robust than the algebraic ones. Therefore, the symmetry perspective could be very useful when some transformation is applied to the system.
Let us notice that, when the order of Aut(G) is less than n, we have dimFix(Aut(G))>1, so the fixed-point space is related to clustering, and C⊂Fix(Aut(G)); we illustrate such a case in the following example. We consider the path graph Pn with n>2, which has Z2 as group of symmetry and Ord(Z2)=2. We can see that the equalities imposed by Z2x=x are
x1=xnx2=xn−1⋮xn−1=x2xn=x1, |
which do not lead to consensus in general, but to clustering {x∈Rn | x1=xn,…,xn=x1}.
In summary, in this section we outlined a different perspective on the consensus space. Let us recall that a weighted ALF, near the consensus space, is equivalent to an ALF with simple graph structure (Proposition 2). Thus, the weighted ALF acquires the property of equivariance under the automorphism group of the (simple) graph; the symmetry group is a subgroup of the symmetric group. Therefore, an ALF possesses also a cell-network structure. At this point, forgetting momentarily the specific properties of ALFs, we can study the connections between the consensus space and the symmetry properties. It turns out that the consensus space arises as an invariant space for a class of subgroups of Sn (Proposition 5).
We conclude this section by recalling a theorem highlighting the fundamental role of the graph structure in the definition of a dynamic network. Although, as we have seen, graphs and groups are intimately related, if we consider the logic flow of definitions for dynamic networks the graph structure should be defined first.
Theorem 1 (Frucht [22]). For any finite group Γ, there exists a finite graph G such that Aut(G)≅Γ.
Theorem 1 ensures that for any given finite group we can construct a graph which is invariant under the group. However, uniqueness is not provided, au contraire, in general there are infinitely many graphs with such a property [7]. For this reason, the definition of the graph structure of a dynamic network precedes the group properties.
A perturbation is a small 'change' of the differential equations governing the system. The order of magnitude can be quantified by a parameter ϵ, 0<ϵ≪1. A perturbation is called singular if the solutions of the perturbed ODEs have a different qualitative behavior with respect to the solutions of the unperturbed system [60]. On the other hand, regular perturbations are the ones that preserve the qualitative structure. For this reason, singular perturbations are the most interesting if we aim to characterize a system qualitatively. In Appendix B, we briefly review some of the standard terminology of singular perturbations.
As mentioned in the introduction, we now consider perturbations of ALFs given by
˙x=−LF(x)+ϵH(x,Λ), | (4.1) |
where 0<ϵ≪1, H(x,Λ)=(h1(x,Λ),…,hn(x,Λ))⊺, and Λ is a set of parameters. The consensus space C is always a set of equilibrium points for ϵ=0 (Corollary 1). Moreover, C is one-dimensional and hence generic perturbations are singular [60]. The system as written in (4.1) is in non-standard form. In many cases, it is useful to transform a singularly perturbed system into a standard form, one reason among all is that a large amount of results in singular perturbation theory are stated for standard forms.
Proposition 6. Let l∈V, and ˜x=(˜x1,…,˜xn−1)⊺ be an (n−1)-dimensional vector. Let ˜L be the (n−1)×(n−1) matrix obtained from the Laplacian L by removing the l-th column and row, i.e.,
˜L:=(L11…L1(l−1)L1(l+1)…L1n⋮⋱⋮⋮⋱⋮L(l−1)1…L(l−1)(l−1)L(l−1)(l+1)…L(l−1)nL(l+1)1…L(l+1)(l−1)L(l+1)(l+1)…L(l+1)n⋮⋱⋮⋮⋱⋮Ln1…Ln(l−1)Ln(l+1)…Lnn), |
and let v(l) be the (n−1)-dimensional vector defined as follows:
v(l):=(L1l,…,L(l−1)l,L(l+1)l,…,Lnl)⊺. |
The coordinate transformation
x1↦˜x1,⋮xl↦xl(k,˜x):=k−∑j˜xj,⋮xn↦˜xn−1, | (4.2) |
where k is the constant of motion (2.2), transforms system (4.1) into the standard form
˙˜xi=−∑j˜Lijf(˜xj)−v(l)if(xl(k,˜x))+ϵgi(˜x,k,Λ)˙k=ϵ∑jgj(˜x,k,Λ), | (4.3) |
where gi(˜x,k,Λ):=hi(˜x1,…,xl(k,˜x),…,˜xn,Λ).
Proof. Substituting the coordinate change Eq (4.2) into Eq (4.1) we obtain the set of equations for ˜xi. Then, the equation for k is retrieved by evaluating the scalar product ⟨1,˙x⟩.
Let us notice that the perturbation transforms the constant of motion into a slow variable of the system. Since the constant k is related to the arithmetic mean of the states, ⟨x⟩, perturbing the system, in general, means that the states' density is changing, e.g., the concentration of the system is increasing/decreasing. In other words, the perturbation acts as a drift/dissipation in the undergoing diffusive evolution. Notice that if the perturbation H is 'perpendicular' to the consensus space, ⟨1,H(x,Λ)⟩=0, then ˙k=0, which in turn means that the perturbation problem is regular.
As mentioned, we are interested in singular perturbations for two major reasons. First of all, in the setting of our problem where the unperturbed system has a set of equilibria of dimension greater than zero, generic perturbations are singular. The second reason is that singular perturbations are the ones that could lead to a different qualitative behavior of the system. In the following section, we look at the near consensus behavior under a singular perturbation, and we characterize the dynamics for a selected class of systems.
In this section, we further specialize our analysis to complete networks, Kn. We will show how this assumption allows a reduction of the equations, and a detailed description of the consensus behavior will be given.
Let us consider a perturbed Kn-ALF in the standard form (4.3). The layer problem, obtained by setting ϵ to zero, reads as
˙˜xi=−[∑j˜Lijf(˜xj)+v(l)if(xl(k,˜x))]. | (5.1) |
The system described by Eq (5.1) is (n−1)-dimensional with one parameter, k. It is worth noting that although the graph structure in (5.1) seems to be lost, some information is readily available. On the one hand, the term ∑j˜Lijf(˜xj) corresponds to a K(n−1)-ALF, i.e., a complete graph with (n−1) nodes. On the other hand, the scalar term v(l)if(xl(k,˜x)), can be interpreted as a higher-order interaction [6] naturally arising from the reduction performed.
Furthermore, and for our specific purposes, there is a more relevant property that survives the transformation: The symmetry. In fact, starting with an Sn-equivariant unperturbed system, after the transformation into the standard form we get a layer problem which is Sn−1-equivariant. This is a first example of robustness of the symmetries. Since we are interested in the consensus dynamics, we consider Eq (5.1) on the invariant space given by Fix(Sn−1). Let us notice that, in general, the invariant space Fix(Sn−1) is not globally stable. For example, in a neighborhood of an attracting branch of the consensus space it is stable. Instead, in a neighborhood of a repelling part of the consensus space it is unstable. This means that the restriction to Fix(Sn−1) provides a robust description of the system only in the basin of attraction of a stable region of Fix(Sn−1); in the unstable regions the restriction works only if the initial conditions lie exactly in the invariant space and if the perturbation preserves Fix(Sn−1). Then, restricting Eq (5.1) to Fix(Sn−1)={˜x∈Rn−1 | ˜x1=⋯=˜xn−1} we obtain
˙x=−(f(x)−f(k−(n−1)x)), | (5.2) |
where x:=˜x1=⋯=˜xn−1, and we used the properties of the Laplacian of a complete graph. So, in the invariant space the system reduces to a one dimensional system (with one parameter). The former consensus space, C=Fix(Sn), gives the equilibria of Eq (5.2). This is a consequence of the fact that the layer problem is a coordinate transformation of the unperturbed non-standard form Eq (2.1), for which we know the consensus space is a set of equilibria.
Remark 5. The parametrized consensus states (2.4) given by x∗k=k/n are equilibria of Eq (5.2).
Since the equilibria of the layer equation constitute the critical manifold for the slow-fast system, then the consensus space is a (part of the) critical manifold of the system.
Proposition 7. The stability of the consensus set (2.4) is determined by the derivative of the response function. In particular, we can decompose the consensus into three components,
Ca={x∗k∈C | dxf(x∗k)>0},Cr={x∗k∈C | dxf(x∗k)<0},Cs={x∗k∈C | dxf(x∗k)=0}, |
where Ca is attracting (stable), Cr is repelling (unstable), and Cs is singular.
Proof. By straightforward computations we obtain the Jacobian of the system evaluated at the consensus, −dxf(k/n)n, which implies the proposition.
For 0<ϵ≪1, thanks to Theorem 2 (Fenichel), Appendix B, compact submanifolds of Ca/r perturb to O(ϵ)-close locally invariant slow manifolds of the singularly perturbed system (4.3) (denoted correspondingly by Ca/rϵ). For the singularities Cs we need further analyses. We are going to consider the case of transcritical singularities which, as we will show, turn out to be generic due to the geometric properties of the problem.
We start by considering a symmetry condition on the perturbation. While restrictive, we impose it to guarantee the invariance of Fix(Sn−1) for the perturbed system (4.3).
Lemma 4. Let H(x,Λ) be a perturbation such that hi=h, ∀i≠l, and hl=˜h. Then, Fix(Sn−1) is an invariant space for the perturbed system (4.3).
Proof. Let us consider the equations in standard form (4.3) with a perturbation satisfying the conditions hi=h, ∀i≠l, and hl=˜h. So, if we restrict the equations to
{(x,k)∈R2 | x:=˜x1=⋯=˜xn−1}, | (5.3) |
we obtain
˙x=−[f(x)−f(k−(n−1)x)]+ϵg(x,k,Λ)˙k=ϵ(n−1)g(x,k,Λ)+ϵ˜g(x,k,Λ), | (5.4) |
where g(x,k,Λ):=h(x,…,k−(n−1)x,…,x,Λ), and ˜g(x,k,Λ):=˜h(x,…,k−(n−1)x,…,x,Λ). Then Fix(Sn−1) is an invariant space.
Assuming a perturbation satisfying Lemma 4, we are allowed to continue our analysis on the invariant space (5.3), which we will refer to as (x,k)-plane. We remark once again that, in a neighborhood of a stable region of Fix(Sn−1), the reduction is robust; while in unstable regions the reduction is preserved only by fine tuning of initial conditions and perturbation.
The analysis to be performed in this section is local. Thus, let us now consider that the response function can be written as
f(x)=N∑j=1ajxj+O(xN+1), | (5.5) |
where aj are real constant coefficients, N∈N, and we assume that at least one of the coefficients aj, j≠1, is different from zero. We know that the consensus space, for an ALF, does not depend on the particular choice of the response function. On the other hand, the remaining equilibrium sets, that together with C give rise to the full critical manifold, depend on the form of the response function. By detailing the local form of f, we are able to further describe the local shape of the other branches of the critical manifold crossing the consensus space. We focus our analysis on a neighborhood of the consensus space. The following proposition shows that if there is a singularity on the consensus space, implying that another branch of equilibria intersects with the consensus space, then we can derive the linear approximation of such intersecting branches of equilibria.
Proposition 8. Let the response function f be of the form (5.5) with N≥2. In the (x,k)-plane, if a branch of the critical manifold intersects the consensus space, then such a branch has the local form
κ(x)=2xsk+(n−2)x, |
where (xsk,…,xsk)∈Cs.
Proof. Let us consider the function ˜ϕ(x,y):=−[f(x)−f(y+x)] and the auxiliary system
˙x=˜ϕ(x,y)˙y=0, |
where y acts as a parameter, with y close to zero. We are going to evaluate the slope of the tangents of the curves of zeros of ˜ϕ(x,y) intersecting the line y=0. Considering the response function (5.5), we expand f(x+y) by using the binomial theorem,
f(y+x)=N∑j=1aj(y+x)j+O((x+y)N+1)=N∑j=1ajxj+N∑j=1j∑k=1(jk)ajykxj−k+O(xN+1)+yO(yuxv), |
where, for brevity, we collect in O(yuxv) all higher order terms of such order for all combinations u+v=N. So, we can rewrite ˜ϕ(x,y) as
˜ϕ(x,y)=y(N∑j=1j−1∑l=0(jl+1)ajylxj−l−1+O(yuxv)), |
where we factored out y, and set l:=k−1. We regularize ˜ϕ(x,y) by removing the line y=0 from the set of zeros, i.e. ˜ϕ(x,y)=:y˜ϕreg(x,y), where
˜ϕreg(x,y)=N∑j=1j−1∑l=0(jl+1)ajylxj−l−1+O(yuxv). |
We continue by disregarding the higher-order terms as they do not play a role in our forthcoming arguments. The regularized auxiliary system reads
˙x=˜ϕreg(x,y)˙y=0. |
Let us compute the Jacobian, J, for the regularized auxiliary system. We get
J=(∂˜ϕreg∂x∂˜ϕreg∂y00), |
where
∂˜ϕreg∂x=N∑j=1j−1∑l=0(jl+1)aj(j−l−1)ylxj−l−2,∂˜ϕreg∂y=N∑j=1j−1∑l=0(jl+1)ajlyl−1xj−l−1 |
Since we are interested in what happens at the intersection with y=0, we evaluate the Jacobian on that line, namely
J0:=J|y=0=N∑j=1j(j−1)ajxj−2(11200). |
The eigenvalues of J0 are determined by the equation det(J0−μId)=0, which in our case reads
−μ[N∑j=1j(j−1)ajxj−2−μ]=0, |
and so the eigenvalues are
μ1=0,μ2=N∑j=1j(j−1)ajxj−2. |
Let us notice that, in general, for the regularized auxiliary system the Jacobian J0 cannot be interpreted as the linearisation of the system, because y=0 is no more an equilibrium. However, there could be other equilibria on the line y=0 at the intersections with the other curves of equilibria. At such points the eigenvector with zero eigenvalue gives the direction of the tangent of the intersecting curve of zeros. The eigenvector ˜v(μ1)=(˜v(μ1)x,˜v(μ1)y)⊺ must satisfy the equation J0˜v(μ1)=0, that is
N∑j=1j(j−1)ajxj−2(˜v(μ1)x+12˜v(μ1)y)=0. |
Therefore, since for j≠1 at least one aj≠0, ˜v(μ1)x=−12˜v(μ1)y, and so ˜v(μ1)∝(1,−2)⊺, where ∝ is the proportionality symbol. Notice that ˜v(μ1) does not depend on x, which means that wherever the intersection takes place, the tangent will have the direction of ˜v(μ1). At this point, we need to obtain the equivalent vector for the system of our interest (5.2). Note that the equation y=k−nx puts in relation the auxiliary system with the layer problem (5.2). Thanks to this relation we can construct the transformation connecting the tangent vectors of the two systems, in particular we have
Π:=(∂x(x,k)∂x∂x(x,k)∂k∂y(x,k)∂x∂y(x,k)∂k)=(10−n1), |
and then the inverse transformation reads
Π−1=(10n1). |
Thus, the tangents of the critical manifold at the intersections with the consensus space, in the original system, have direction given by vμ1=Π−1˜vμ1∝(1,n−2), which proves that the slope of the tangent in the (x,k)-plane is n−2. Therefore, the tangent lines have an equation of the form κ(x)=˜κ+(n−2)x. In order to find ˜κ, we use the fact that κ(xsk)=nxsk, obtaining ˜κ=2xsk; the statement is proven. A sketch of the result of this proposition is shown in Figure 1.
Proposition 8 tells us that, up to linear approximation, all other branches of equilibria of the critical manifold crossing the consensus space are parallel to each other and are in fact transverse to the consensus space. Moreover, for n>2 such branches of the critical manifold locally are also transverse to the fast-flow. As a consequence, we have that pitchfork singularities are not possible on the consensus space for the class of ALFs under examination, this statement is also confirmed by checking the conditions for pitchfork singularities [34]. By a similar reasoning, fold singularities on the consensus space are also excluded. Finally, Hopf singularities do not appear either by the fact that the layer system has always real eigenvalues. Thus, one can conclude that for Kn-ALFs transcritical singularities are generic on the consensus space, in the sense of codimension one bifurcations; while on other branches of the critical manifold it is possible to have other singularities, see some examples in Section 6.
Remark 6. Although in this section we have only considered complete networks, we conjecture that other topologies also present, generically, transcritical singularities along the consensus space. The main argument is that, no matter the network structure, as long as the consensus space exists, it is a one dimensional linear subspace of the phase-space. Singularities of this linear subspace appear as intersections with other branches of the critical manifold induced by the nonlinear response function F. Locally, and generically, one would expect that these intersections are transversal, leading to transcritical singularities (possibly in higher dimensions). In particular, recalling the result of Proposition 3, we know that the symmetries induced by the invariance of the response function generates linear branches of the critical manifold intersecting transversely the consensus set. The great advantage of considering complete networks is the reduction to a planar problem. This reduction requires extra work for other network topologies. Nevertheless, our conjecture will be supported by numerical simulations in Section 6.
We now state the conditions for (nondegenerate) transcritical singularities.
Proposition 9. Let xsk∈Cs be a singular consensus point. Let f and hi, i=1,…,n, be Cm-functions with m≥3, and assume that Lemma 4 holds. If
d2xf(xsk)≠0,n≠2,⟨1,H(xsk,Λ)⟩≠0, |
then xsk is a nondegenerate transcritical singularity of Eq (5.4).
Proof. We check the conditions for transcritical singularities on planar systems provided by Krupa and Szmolyan [34]. Given a planar system of the form (B.1) then the origin is a transcritical singularity if
f(0,0,0)=0, | (5.6) |
∂xf(0,0,0)=0,∂yf(0,0,0)=0, | (5.7) |
|∂2xf(0,0,0)∂2xyf(0,0,0)∂2yxf(0,0,0)∂2yf(0,0,0)|<0, | (5.8) |
∂2xf(0,0,0)≠0, | (5.9) |
g0:=g(0,0,0)≠0. | (5.10) |
We now prove that these conditions applied to our system lead to the conditions of the statement. Conditions Eqs (5.6) and (5.7) are satisfied because xsk is a singular consensus point. For the non-degeneracy condition (5.8), we obtain
d2xf(xsk)≠0. |
The transversality condition (5.9) gives,
n(n−2)d2xf(xsk)≠0, |
which implies n≠2. Finally, the last condition (5.10) leads to ⟨1,H(xsk,Λ)⟩≠0.
We consider the case where all the singularities on the consensus space are nondegenerate transcritical, i.e., the conditions of Proposition 9 are met on Cs. So, in our current setting, the consensus space consists of a cascade of transcritical singularities changing the stability from attracting to repelling, and vice-versa. If at xsk the stability transition, in the direction of the slow flow, is from attracting to repelling, we call xsk a type-1 transcritical point. Type-1 transcritical points are characterized by the sign ratio
ρ:=sgn(d2xf(xsk))sgn(⟨1,H(xsk,Λ)⟩)=−1. |
If at xsk the stability transition, in the direction of the slow flow, is from repelling to attracting, we call xsk a type-2 transcritical point. Type-2 transcritical points are characterized by the sign ratio ρ=1.
Under perturbations the consensus space, being a component of the critical manifold, extends to a slow manifold as predicted by Fenichel, Theorem 2. More specifically, compact normally hyperbolic submanifolds of the consensus perturb to slow manifolds with the same stability properties. The continuation of such slow manifolds close to a singularity depends on the perturbation. Suppose we follow a trajectory starting close to an attracting section of the consensus space. When such trajectory reaches a neighborhood of a singularity its behavior depends specifically on the perturbation and on the geometry of the aforementioned slow manifolds. In particular, a trajectory reaching a neighborhood of a transcritical point could proceed in three different manners.
Exchange of stability The trajectory exchanges to another branch, which intersects the consensus space transversely, of the critical manifold. In other words, the trajectory does not follow anymore the consensus space, but another stable branch of the critical manifold that results from the nonlinear response function.
Fast escape The trajectory, once traversing a small neighborhood of the singularity, continues along the fast flow.
Bifurcation delay The trajectory continues along an unstable slow manifold that is perturbed from a normally hyperbolic repelling submanifold of the consensus space for a O(1) time.
The third characteristic behavior is called canard, and acts as a separating case between the other two [35,60]. Usually, the presence of a canard in a planar system is associated to a critical value of the parameters of the system. The description in terms of the parameters of the system has the advantage of enabling the unfolding of the singularity to be studied. Here, however, we adopt a geometric perspective on the problem. Near fold singularities a geometric perspective on canard solutions in the plane has been treated extensively in [13]. In our case of study we show that the requirement for a canard solution can be stated as an algebraic condition on the perturbation.
Proposition 10. Let xsk∈Cs be a singular consensus point satisfying the conditions of Proposition 9. If the perturbation satisfies the property
H(xsk,Λ)∝1, |
where ∝ is the proportionality sign, then for ϵ sufficiently small, system (4.3) admits canard solutions.
When a perturbation satisfies the condition of Proposition 10, we refer to it as critical perturbation, and we use the notation Hcrit(x,Λ).
Proof. We use again the results from [34] to check that our statement holds. Considering a system of the form (B.1), satisfying conditions (5.6)–(5.10), then there exists a parameter λ controlling the behavior at the transcritical singularity (which we remind to be at the origin for this benchmark system). The parameter λ is given by
λ=1|g0|√β2−γα(δα+g0β), |
where
α=12∂2xf(0,0,0),β=12∂2xyf(0,0,0),γ=12∂2yf(0,0,0),δ=∂ϵf(0,0,0). |
So, the parameter λ for the system (5.4), at a transcritical point xsk, reads
λ=−ρh(xsk,Λ)+(n−1)˜h(xsk,Λ)˜h(xsk,Λ)+(n−1)h(xsk,Λ). |
For type-1 transcritical points, we have ρ=−1, and the condition for canards is λ=λcrit:=1. So, we obtain the equation
(n−2)˜h(xsk,Λ)=(n−2)h(xsk,Λ). |
Since the case n=2 is excluded by Proposition 9, we have ˜h(xsk,Λ)=h(xsk,Λ). Therefore the critical perturbation Hcrit(x,Λ) at xsk reads
Hcrit(xsk,Λ)=(h(xsk,Λ),…,h(xsk,Λ),…,h(xsk,Λ))⊺=h(xsk,Λ)1, |
which agrees with the statement. In order to complete the proof, we show that for a type-2 transcritical point a critical perturbation admits faux-canards. Let us recall that faux-canards for planar systems are generic, and appear for λ<λcrit [34]. Using the fact that for a type-2 transcritical point ρ=1, we get λ=−1.
In Figure 2, we sketch the blow-up of the transcritical points under a critical perturbation [33,34,40]. The blow-up picture makes clear the different behavior of the type-1/2 transcritical points and illustrates how the continuation of the consensus space occurs.
Note that we can interpret the result of Proposition 10 as follows: If the perturbation is tangent to the consensus space at a singularity, then canard solutions exist. As a matter of fact, assuming a perturbation that is (everywhere) tangent to the consensus space, it is possible to prove a result that holds for every network structure.
Proposition 11. Given an ALF with arbitrary graph structure G, and H(x,Λ) a perturbation satisfying H(x∗,Λ)∝1 and H(x∗,Λ)≠0, ∀x∗∈C. Then the consensus space is a trajectory of the perturbed system.
Proof. From Corollary 1 we know that the consensus space is an equilibrium of the unperturbed system; moreover, since H(x∗,Λ)∝1, ∀x∗∈C, we have that C is an invariant space for the perturbed vector field. So, projecting Eq (4.1) onto C we obtain
˙x∗=ϵH(x∗,Λ), |
x∗∈C. Given the assumption that the perturbation is non-zero on the consensus space, which is a one-dimensional space, we obtain that C is a trajectory of the perturbed system.
In essence, Proposition 11 gives some conditions under which a perturbed equivariant ALF (4.1) exhibits a maximal canard. Let us also notice that Proposition 11 holds for all simple graphs, and it does not make any particular assumption on the singularities of the consensus space. In other words, paying the price of making more stringent assumptions on the perturbation we can cover the cases where the slow-fast theory would be difficult, or not even possible to apply.
In this section, we present several examples of ALFs, exploiting generic and canard behavior from different perspectives. The simulations we show are performed in Mathematica [63], where the precision of the numerical simulations can be easily set. We show phase portraits, time series and spatio-temporal plots highlighting the nonlinear diffusion and drift mechanism on non-complete graphs. In view of Proposition 2, our simulations are restricted to simple graphs but we emphasize that for weighted graphs one would obtain equivalent results.
We start by looking at a K3-ALF considering two nonlinear response functions, one that is even f(x)=(x−1)2(x+1)2 (Figure 3), and one odd f(x)=(x−1)(x+1)2 (Figure 4). The two response functions induce different critical manifolds, Figures 3a and 4c. Nevertheless, the typical behavior is similar, see Figures 3b and 4a.
It is worth recalling now a few issues with numerical simulations of transcritical points, see more details in [18]. Roughly speaking, canard solutions, as shown in Figure 3c, contract first towards the critical manifold, cross a singularity, and then follow the unstable branch of the critical manifold enough time to compensate the aforementioned contraction before leaving an O(ϵ)-neighborhood of the critical manifold. For the particular case of a K3-ALF with response function f(x)=(x−1)2(x+1)2, one can check that the transverse eigenvalue along the critical manifold is −49k(9−k2) implying that the contraction and expansion rate is symmetric with respect to the origin (this balance is more precisely given by the entry-exit relation [18,35]). Such a time symmetry should be visible as a spatial symmetry in correspondence with a transcritical point on the consensus manifold. As one can see in Figure 3c, the symmetry described is not very well reproduced by the numerical simulation, although the computations are performed with a working precision of 50 digits. There are several factors that induce such a problem. First, we have that the symmetry is exact only in the limit for ϵ→0, so we expect that a numerical simulation will just approximate such symmetry. The simulation displayed in Figure 3c is performed with ϵ=1/10, which is quite large to clearly see this effect. However, by lowering ϵ one would need to further increase the working precision, leading to problems with the time performance of the CPU. Since our present work has a theoretical focus, we do not dive deeper in the numerical analysis of these delicate systems, leaving the problem of highly accurate simulations for future works.
There is another detail to take into account: In Figure 3c, we have canard solutions that cross three transcritical points on the consensus space without leaving the repelling intermediate section (light blue and pink curves). Here the question is: Where should we expect the canards to leave the consensus manifold? As we just discussed, we expect some symmetry, but here we need to be more careful stating with respect to which transcritical point. We argue that, for these particular solutions, the intermediate section has a null effect, and, therefore, the solution should leave the consensus after the third transcritical point (counting from top to bottom) after a distance (approximately) equal to the distance traveled close to the attracting section before the first transcritical point. In order to prove the aforementioned statement, we use the slow-divergence integral [13,14,17]. So, let us consider the slow-divergence integral along the consensus manifold for the system under analysis. Since the perturbation is constant the divergence of the vector field in the (x,k)-plane, evaluated on the consensus space, is simply −ndxf(k/n). Therefore the slow-divergence integral becomes
−n∫dxf(k/n)dk. |
We now notice two facts. First, the intermediate section is divided into two spatially equal sections by a transcritical point. Second, on the first subsection dxf(k/n)>0, and on the second subsection we have dxf(k/n)<0. Therefore the slow-divergence integral on the intermediate section of the consensus space is null, so on the intermediate section the attracting and repelling effect compensate each other. The considerations we have done hold for the dynamics near consensus under a constant critical perturbation. It is worth mentioning that the nonlocal interaction of transcritical singularities via hysteresis processes could give rise to an enhanced delay for the canard [21,59].
In this section, we consider the spatio-temporal configurations induced by different network structures both under generic and critical perturbations, the latter giving rise to canards. The simulations are not subject to any reduction, and the evolution of the full system is computed. Given a graph we consider a planar embedding, and we assume the obtained coordinates as representative of a portion of a square. The spatio-temporal configurations are then obtained by evaluating a heat map where the colors, from blue to yellow, are in correspondence with the state's values of the system. Each frame represents a unit time step in the sampled interval, and the time flow is represented as follows:
t1tn+1⋯t2tn+2⋯⋮⋮⋮tntn+n⋯. |
We considered the following network structures: random networks (Figures 5 and 6), a complete graph with 10 nodes (Figure 7), and a lattice with 30×30 nodes (Figures 8–10). Throughout this section we adopt the response function f(x)=(x−1)2(x+1)2.
Spatio-temporal patterns are usually considered in the realm of partial differential equations [4,16,44] where a wide range of equations, e.g., reaction-diffusion systems, can give rise to complex patterns observable in nature. Here, the continuous space is replaced by a network and consequently in place of partial differential equations we have a set of ordinary differential equations. Given the analogy with diffusion and drift mechanisms that perturbed ALFs undergo it is quite natural to explore the potential spatio-temporal patterns appearing under different conditions. The most remarkable observation we can draw from the simulations is that under generic perturbations no recognizable pattern appear, while under a critical perturbation we observe transient patterns. In other words, our simulations suggest that spatio-temporal patterns are induced by canards. Such an effect is related to the delayed loss of stability produced by a canard. In fact, we generically have that the individual states, once they reach the singularity, leave consensus one after the other, with no particular order. On the other hand, under a critical perturbation a significant amount of states transition at the same time and such a configuration persists for several units of time. Such phenomenon is more clearly visible when the number of nodes is large, to see this compare the plots for a complete graph with 10 nodes (Figure 7c), a random graph with 30 nodes (Figure 6a) and a lattice with 900 nodes (Figure 10).
It is worth mentioning that spatio-temporal patterns are commonly studied and understood for reaction-diffusion equations where the two species interaction could give rise to patterns. Here, we are studying equations that resemble a drift-diffusion, and the (transient) pattern structure arises from the nonlinearity of the diffusion mechanism and the geometry of the drift.
In general, we can notice that the nonlinearity given by the response function induces a different diffusion mechanism. By looking at the generic cases, illustrated in Figures 5a, 7a, and 9, we see that the transition between the initial consensus state to the final one exhibits jumps instead of a homogeneous process. Such an effect is due to the fact that the nonlinearity induces an intricate structure of the critical manifold, as we have already seen in the previous examples. We can partially observe the homogeneous process of diffusion when the canard conditions apply, see Figures 6a and 7c (for the lattice in Figure 10 such an effect is suppressed by the image quality compression).
As a final remark, we observe that the transition time between two consensus sections is visibly shorter in the canard cases.
This paper has been dedicated to the analysis of a class of dynamical systems describing nonlinear diffusion on a simple graph structure. The analysis starts by looking at (unperturbed) ALFs on graphs with positive weights. We emphasize the importance of the fiber-wise topological equivalence proved in Proposition 2. Indeed, a weighted graph, in general, loses the symmetry properties that its own unweighted counterpart has. The fact that a positive-weighted graph induces an evolution of the system that is topologically equivalent to the one induced by the unweighted counterpart, allows us to consider unweighted simple graphs. An ALF with a simple graph structure inherits the symmetry properties of the underlying graph, enabling us to use the tools from equivariant dynamical systems theory, such as fixed-point spaces and invariant properties of the response function. The structure of the equations allows for a detailed analysis of the equilibria, that in turn led us to study the local structure of singularities on the consensus space for complete graphs (Propositions 8 and 10), and to prove the existence of a maximal canard for generic simple graphs (Proposition 11).
Several new directions arise from the results of our paper. For example, one could consider response functions f(x) depending also on "neighboring" nodes; in such a case the network structure also appears inside the response vector field, and not only through the Laplacian matrix. Moreover, one may be interested in studying the case of heterogeneous response functions, i.e., different nodes might contribute differently to the mutual interaction. Regarding the graph structure, one may take into account negative weights or directed graphs, which, even for the linear case, give rise to much richer dynamics than the positive-weights case. Hypergraphs are also becoming more and more relevant. Incorporating a hypergraph structure in our model could take different directions. The simplest choice would be to study which class of hypergraph models can be associated with an ALF. Of course, such a choice would lead unavoidably to a restricted family of hypergraphs. On the other hand, one could extend the model to hypergraphs, by replacing the graph Laplacian with the hypergraph Laplacian, and the other quantities accordingly. In this case, the system will differ dramatically from the one we studied. However, our analysis could serve as a guideline: Exploiting symmetries and algebraic properties could lead to relevant simplifications for the study. In a similar line of thought, reduction techniques similar to those presented here, but for other network topologies than complete networks, seems worth pursuing. Another possibility is to study Laplacian systems where the states have dimensions larger than one, for example, one might consider Laplacian systems where the states evolve in the real plane, or even in a two-dimensional manifold. The dynamics of planar systems entail interesting phenomena, such as periodic orbits, that could potentially give rise to new phenomena unseen in the one-dimensional framework. For similar reasons, states of dimension three might be relevant as well, since it is well-known that it is for three-dimensional systems that chaos, at the level of the nodes, may appear. In all the aforementioned cases, a natural question is to investigate, and classify the possible singularities that arise and the influence of the network on them (for example, in this paper, we showed that complete ALFs can only have transcritical singularities along the consensus manifold). Moreover, one would then be interested in the behavior under small perturbations, which, as seen here, is also closely related to the network structure. Finally, given its relevance for network dynamics, it would be interesting to further investigate which perturbations lead to persistence, or on the other hand destroy, the singular canards of Proposition 10. One possibility is already given in Proposition 11, but its generalization seems rather promising.
The principal author is RB, who conducted the research and wrote the article. HJK contributed to the research phase through active discussion and undertook some revisions during the writing process.
The authors declare they have not used Artificial Intelligence (AI) tools in the creation of this article.
The authors thank the anonymous reviewers whose thorough comments helped to improve the manuscript. All datasets are available upon request to the corresponding author.
The authors declare there is no conflict of interest.
We recall some basic notions about group theory [23,49].
Definition 5. Let Γ be a set and ⋆:Γ×Γ→Γ a binary operation. A group is a couple (Γ,⋆) satisfying the following properties:
1. Associativity: (γi⋆γj)⋆γk=γi⋆(γj⋆γk), ∀γi,γj,γk∈Γ.
2. Identity: ∃ e∈Γ such that e⋆γ=γ⋆e=γ, ∀γ∈Γ.
3. Inverse: ∀γ∈Γ, ∃ γ−1∈Γ such that γ−1⋆γ=γ⋆γ−1=e.
The operation ⋆ is called group operation. Note that, closure under the group operation follows from the definition of the operation itself. Unless strictly necessary, we refer to a group just by the set, e.g., Γ, implicitly considering it equipped with a group operation; we also omit the group operation symbol, e.g., γiγj=γi⋆γj.
Definition 6. A group is said to be finite if the set Γ has a finite number of elements. The order of a finite group, Ord(Γ), is the number of elements in the group.
Given a vector space, a representation, ψ, is a homomorphism from the group to the general linear group of the vector space. Since the states, x, of an ALF belong to Rn, we are interested in how Γ acts on Rn, therefore, we consider representations ψ:Γ→GL(Rn). To avoid new unnecessary notation, we omit to explicitly write the representation. It is implicit that when we write a group element applied to a vector we are considering the representation on the appropriate vector space, e.g., γx=ψ(γ)x.
Definition 7. Given two groups Γ(1), Γ(2), the direct product Γ(1)×Γ(2) is a group with set of elements given by the Cartesian product
Γ(1)×Γ(2)={(γ(1),γ(2)) | γ(1)∈Γ(1),γ(2)∈Γ(2)}, |
and operation defined as follows
(γ(1)i,γ(2)j)(γ(1)k,γ(2)l)=(γ(1)iγ(1)k,γ(2)jγ(2)l), |
∀γ(1)i,γ(1)k∈Γ(1) and ∀γ(2)j,γ(2)l∈Γ(2).
It follows that the direct product can be extended to an arbitrary number of groups, Γ(1)×Γ(2)×⋯×Γ(n). Another important concept is the one of fixed-point space, i.e., the set of points that are kept fixed by the action of the group.
Definition 8. Let Σ⊆Γ be a subgroup of Γ. The fixed-point space (under the action in Rn) of the group Σ is denoted by Fix(Σ) and it is given by
Fix(Σ):={x∈Rn | σx=x, ∀σ∈Σ}. |
A direct interplay between graph theory and group theory is given by the graph's automorphism group. For graphs the automorphism group is a subgroup of the (finite dimensional) symmetric group Sn [51], i.e., the group of permutations of n symbols.
Definition 9. Given a (simple) graph G, the automorphism group, Aut(G), is the group of permutations which preserves the adjacency structure of the vertices of G.
Remark 7. From an algebraic point of view, preservation of adjacency structure correspond to the fact that σ∈Aut(G) if and only if [A,σ]=0, where [⋅,⋅] represent the commutator of matrices [7]. Notice that the same statement holds also if we substitute the adjacency matrix with the Laplacian matrix.
Definition 10. Let Γ be a group*. A function f is called Γ-invariant if
*Notice that this is not related to Aut(G), we use the same symbol Γ for different groups in different context.
f∘Γ=f, |
where ∘ denotes the composition of maps.
The concept of symmetry, in dynamical systems theory, refers to the fact that there exists a group mapping solutions to solutions. When a vector field is given, such a concept can be translated in terms of equivariance of the vector field.
Definition 11. Let Γ be a group, and X a vector field. Then X is called Γ-equivariant if
X∘Γ=Γ∘X. |
An element of the group, γ∈Γ, is called a symmetry of the vector field.
Remark 8. It is straightforward to see that if a system is Γ-equivariant, then Γ maps solutions to solutions.
A solution of a dynamical system is a simple example of an invariant set under the flow. Invariant sets, in general, are extremely relevant in the study of ODEs, since they allow to reduce the analysis to potentially simpler equations. We recall here the general definition of invariant set under the flow induced by a vector field. Later we state the interplay between symmetries and invariant sets.
Definition 12. Let ϕ be the flow generated by a vector field on Rn. A set M⊂Rn is said to be invariant (respectively positively/negatively invariant) under the flow if for every x∈M, ϕt(x)∈M, ∀t∈R (respectively ∀t∈R+/R−).
In order to keep a compact notation, we call a set invariant under the flow a ϕ-invariant set. From equivariance of the vector field we can immediately infer the existence of a ϕ-invariant set.
Proposition 12. The fixed-point space, Fix(Γ), is a ϕ-invariant set for Γ-equivariant systems [26].
In general, the symbols used in this section are not related to those in the main text.
A singularly perturbed system of ODEs, in the standard form, is also called a slow-fast system, and it has the form
˙x=f(x,y,ϵ)˙y=ϵg(x,y,ϵ), | (B.1) |
where 0<ϵ≪1, x∈Rn, y∈Rm, and the time-parametrisation t is called fast-time. Eq (B.1) is said to be in the standard form because the time separation between the fast-variables, x, and the slow-variables, y, is explicit. In the non-standard form a singularly perturbed system has the form
˙z=F(z,ϵ), |
where z∈Rn+m, and the vector field F(z,0) has at least one set of equilibria of dimension greater than zero [60].
Defining the slow-time, τ:=ϵt, Eq (B.1) can be rewritten as
ϵx′=f(x,y,ϵ)y′=g(x,y,ϵ). | (B.2) |
Setting ϵ to zero in Eq (B.2), we obtain the reduced system
0=f(x,y,0)y′=g(x,y,0), | (B.3) |
which is a set of constrained differential equations. Instead, setting ϵ to zero in Eq (B.1) we obtain the layer problem
˙x=f(x,y,0)˙y=0, | (B.4) |
where the slow-variables, y, play the role of parameters.
Definition 13. The critical manifold is defined as the set
C0={(x,y)∈Rn×Rm | f(x,y,0)=0}. |
Remark 9. Regardless of the commonly accepted name, the critical manifold is not necessarily a manifold.
The critical manifold is the constraint space for the reduced system (B.3), or equivalently, it is the set of equilibria of the layer problem (B.4).
Definition 14. A point x∗∈C0 is called normally hyperbolic if the Jacobian matrix Dxf(x∗,y,0), associated to the layer problem (B.4), has no eigenvalues on the imaginary axis.
Definition 15. A submanifold of the critical manifold M0⊆C0 is called normally hyperbolic if all points in M0 are normally hyperbolic. Moreover, invariant manifolds that are normally hyperbolic are called normally hyperbolic invariant manifold (NHIM) [30].
Theorem 2 (Fenichel). For ϵ sufficiently small, compact normally hyperbolic submanifolds of the critical manifold, M0⊆C0, persists as O(ϵ)-close locally invariant slow manifolds Mϵ diffeomorphic to M0. Moreover, Mϵ is normally hyperbolic and has the same stability properties with respect to the fast variables as M0.
Remark 10. Theorem 2 is restricted to the results relevant for the present paper. An exhaustive version can be found in [20,35]. The terminology O(ϵ)-close means that, as ϵ→0, Mϵ has Hausdorff distance O(ϵ) from M0.
For nonhyperbolic points, x∗∈C0, also known as singularities, Fenichel's theorem does not provide information about the perturbed system in a neighborhood of x∗. The process of analysis of the system in a neighborhood of a singularity is called desingularisation. We briefly introduce the blow-up (desingularisation) for nilpotent singularities [33].
Definition 16. A point x∗∈C0 is a nilpotent singularity if all the eigenvalues of the Jacobian, at x∗, are zero.
When a singular point has a mixture of zero eigenvalues, and non-zero (real part) eigenvalues it is possible to reduce the problem to a nilpotent singularity via a center manifold reduction [10].
Definition 17. Let x∗ be a nilpotent singularity. The spherical blow-up is a local transformation
β:Sn+mx∗×I→Rn+m+1(ˉx,ˉy,ˉϵ,r)↦(x,y,ϵ), | (B.5) |
where Sn+mx∗ is the n+m-dimensional sphere, embedded in Rn+m+1, centred at x∗, (ˉx,ˉy,ˉϵ)∈Sn+mx∗, I⊆R≥0 is an interval such that 0∈I, and r∈I.
Let us notice that, for r>0 the transformation (B.5) is a diffeomorphism, while for r=0 it is not invertible. Roughly speaking, the blow-up maps the point x∗ into a (n+m)-dimensional sphere. The core idea of the blow-up is to analyze the induced flow on the sphere and deduct the behavior of the singularity. However, the blow-up transformation by itself does not desingularise the system. After the blow-up it is necessary to perform a conformal transformation (time reparametrization) in order to regularize the system, i.e., retrieve hyperbolicity. We described the spherical blow-up, but several other blow-up transformations are possible, e.g., quasihomogeneous, directional, or even to other manifolds, not necessarily spheres [36]. The choice of the appropriate blow-up should be done by considering the regularization, and the feasibility of the analysis. Since this work is not concerned with a direct application of the blow-up, but rather with the implementation of results involving the blow-up technique, we presented the spherical set-up which provides the most clear geometrical visualization.
[1] | C. O. Aguilar, B. Gharesifard, Necessary conditions for controllability of nonlinear networked control systems, in 2014 American Control Conference, 2014, 5379–5383. https://doi.org/10.1109/ACC.2014.6859170 |
[2] |
S. Ahmadizadeh, I. Shames, S. Martin, D. Nešić, On eigenvalues of laplacian matrix for a class of directed signed graphs, Linear Algebra Appl., 523 (2017), 281–306. https://doi.org/10.1016/j.laa.2017.02.029 doi: 10.1016/j.laa.2017.02.029
![]() |
[3] |
C. Altafini, Consensus problems on networks with antagonistic interactions, IEEE Trans. Autom. Control, 58 (2013), 935–946. https://doi.org/10.1109/TAC.2012.2224251 doi: 10.1109/TAC.2012.2224251
![]() |
[4] |
D. Avitabile, M. Desroches, R. Veltz, M. Wechselberger, Local theory for spatio-temporal canards and delayed bifurcations, SIAM J. Math. Anal., 52 (2020), 5703–5747. https://doi.org/10.1137/19M1306610 doi: 10.1137/19M1306610
![]() |
[5] | L. Beineke, R. Wilson, Topics in Algebraic Graph Theory, Encyclopedia of Mathematics and its Applications, Cambridge University Press, 2004. |
[6] |
C. Bick, E. Gross, H. Harrington, M. Schaub, What are higher-order networks, SIAM Rev, 65 (2023), 686–731. https://doi.org/10.1137/21M1414024 doi: 10.1137/21M1414024
![]() |
[7] | N. Biggs, Algebraic Graph Theory, Cambridge Mathematical Library, Cambridge University Press, 1993. |
[8] |
J. C. Bronski, L. DeVille, Spectral theory for dynamics on graphs containing attractive and repulsive interactions, SIAM J. Appl. Math., 74 (2014), 83–105. https://doi.org/10.1137/130913973 doi: 10.1137/130913973
![]() |
[9] |
T. Carletti, M. Asllani, D. Fanelli, V. Latora, Nonlinear walkers and efficient exploration of congested networks, Phys. Rev. Res., 2 (2020), 033012. https://doi.org/10.1103/PhysRevResearch.2.033012 doi: 10.1103/PhysRevResearch.2.033012
![]() |
[10] | J. Carr, Applications of Centre Manifold Theory, Lefschetz Center for Dynamical Systems, Division of Applied Mathematics, Brown University, 1979. |
[11] |
S. Chandrasekhar, Stochastic problems in physics and astronomy, Rev. Mod. Phys., 15 (1943), 1–89. https://doi.org/10.1103/RevModPhys.15.1 doi: 10.1103/RevModPhys.15.1
![]() |
[12] | P. Chossat, R. Lauterbach, Methods in Equivariant Bifurcations and Dynamical Systems, World Scientific Publishing Company, 2000. |
[13] | P. De Maesschalck, F. Dumortier, R. Roussarie, Canard Cycles, Springer Cham, 2021. https://doi.org/10.1007/978-3-030-79233-6 |
[14] |
P. De Maesschalck, R. Huzak, Slow divergence integrals in classical liénard equations near centers, J. Dyn. Differ. Equations, 27 (2015), 177–185. https://doi.org/10.1007/s10884-014-9358-1 doi: 10.1007/s10884-014-9358-1
![]() |
[15] | G. De Pasquale, M. Valcher, Tripartite and sign consensus for clustering balanced social networks, in 2021 American Control Conference (ACC), (2021), 3056–3061. |
[16] | A. Doelman, Pattern formation in reaction-diffusion systems—an explicit approach, in Complexity Science: An Introduction, World Scientific, Singapore, (2019), 129–182. |
[17] |
F. Dumortier, Slow divergence integral and balanced canard solutions, Qual. Theory Dyn. Syst., 10 (2011), 65–85. https://doi.org/10.1007/s12346-011-0038-9 doi: 10.1007/s12346-011-0038-9
![]() |
[18] |
M. Engel, H. Jardón-Kojakhmetov, Extended and symmetric loss of stability for canards in planar fast-slow maps, SIAM J. Appl. Dyn. Syst., 19 (2020), 2530–2566. https://doi.org/10.1137/20M1313611 doi: 10.1137/20M1313611
![]() |
[19] |
C. Falcó, From random walks on networks to nonlinear diffusion, Phys. Rev. E, 106 (2022), 054103. https://doi.org/10.1103/PhysRevE.106.054103 doi: 10.1103/PhysRevE.106.054103
![]() |
[20] | N. Fenichel, Geometric singular perturbation theory for ordinary differential equations, J. Differ. Equations, 31 (1979), 53–98. |
[21] |
J. P. Françoise, C. Piquet, A. Vidal, Enhanced delay to bifurcation, Bull. Belg. Math. Soc. Simon Stevin, 15 (2008), 825–831. https://doi.org/10.36045/bbms/1228486410 doi: 10.36045/bbms/1228486410
![]() |
[22] | R. Frucht, Herstellung von Graphen mit vorgegebener abstrakter Gruppe, Compos. Math., 6 (1939), 239–250. |
[23] | W. Fulton, J. Harris, Representation Theory: A First Course, Graduate Texts in Mathematics, Springer, New York, 1991. |
[24] | C. Godsil, G. Royle, Algebraic Graph Theory, Springer, New York, 2001. |
[25] |
M. Golubitsky, I. Ian Stewart, Nonlinear dynamics of networks : the groupoid formalism, Bull. Amer. Math. Soc., 43 (2006), 305–365. https://doi.org/10.1090/S0273-0979-06-01108-6 doi: 10.1090/S0273-0979-06-01108-6
![]() |
[26] | M. Golubitsky, I. Stewart, D. Schaeffer, Singularities and Groups in Bifurcation Theory: Volume II, Springer, New York, 2012. |
[27] | J. Guckenheimer, P. Holmes, Nonlinear Oscillations, Dynamical Systems, and Bifurcations of Vector Fields, Springer, New York, 1983. |
[28] |
W. S. Gurney, R. M. Nisbet, The regulation of inhomogeneous populations, J. Theor. Biol., 52 (1975), 441–457. https://doi.org/10.1016/0022-5193(75)90011-9 doi: 10.1016/0022-5193(75)90011-9
![]() |
[29] | M. W. Hirsch, S. Smale, R. L. Devaney, Differential equations, dynamical systems, and an introduction to chaos, Academic Press, Cambridge, 2013. |
[30] | M. Hirsch, C. Pugh, M. Shub, Invariant Manifolds, Springer Berlin, Heidelberg, 2006. |
[31] |
M. Homs-Dones, K. Devriendt, R. Lambiotte, Nonlinear consensus on networks: Equilibria, effective resistance, and trees of motifs, SIAM J. Appl. Dyn. Syst., 20 (2021), 1544–1570. https://doi.org/10.1137/20M1376844 doi: 10.1137/20M1376844
![]() |
[32] |
H. Jardón-Kojakhmetov, C. Kuehn, On fast–slow consensus networks with a dynamic weight, J. Nonlinear Sci., 30 (2020), 2737–2786. https://doi.org/10.1007/s00332-020-09634-9 doi: 10.1007/s00332-020-09634-9
![]() |
[33] | H. Jardón-Kojakhmetov, C. Kuehn, A survey on the blow-up method for fast-slow systems, preprint, arXiv: 1901.01402. |
[34] |
M. Krupa, P. Szmolyan, Extending slow manifolds near transcritical and pitchfork singularities, Nonlinearity, 14 (2001), 1473–1491. https://doi.org/10.1088/0951-7715/14/6/304 doi: 10.1088/0951-7715/14/6/304
![]() |
[35] | C. Kuehn, Multiple Time Scale Dynamics, Springer Cham, Switzerland, 2015. |
[36] |
C. Kuehn, A remark on geometric desingularization of a non-hyperbolic point using hyperbolic space, J. Phys. Conf. Ser., 727 (2016), 012008. https://doi.org/10.1088/1742-6596/727/1/012008 doi: 10.1088/1742-6596/727/1/012008
![]() |
[37] | L. Landau, E. Lifshitz, The Classical Theory of Fields, Elsevier Science, New York, 2013. |
[38] | L. Leibenzon, The motion of a gas in a porous medium. complete works, vol. 2, acad, Sciences URSS, 63 (1930), 8–9. |
[39] |
J. Liu, X. Chen, T. Başar, M. Belabbas, Exponential convergence of the discrete- and continuous-time altafini models, IEEE Trans. Autom. Control, 62 (2017), 6168–6182. https://doi.org/10.1109/TAC.2017.2700523 doi: 10.1109/TAC.2017.2700523
![]() |
[40] |
P. Maesschalck, Planar canards with transcritical intersections, Acta Appl. Math., 137 (2015), 159–184. https://doi.org/10.1007/s10440-014-9994-9 doi: 10.1007/s10440-014-9994-9
![]() |
[41] | M. Muskat, The flow of homogeneous fluids through porous media, Soil Sci., 46 (1938), 169. |
[42] | M. Newman, Networks, Oxford University Press, Oxford, 2018. |
[43] |
S. Nosrati, M. Shafiee, M. Menhaj, Dynamic average consensus via nonlinear protocols, Automatica, 48 (2012), 2262–2270. https://doi.org/10.1016/j.automatica.2012.06.031 doi: 10.1016/j.automatica.2012.06.031
![]() |
[44] |
K. M. Page, P. K. Maini, N. A. Monk, Complex pattern formation in reaction–diffusion systems with spatially varying parameters, Physica D, 202 (2005), 95–115. https://doi.org/10.1016/j.physd.2005.01.022 doi: 10.1016/j.physd.2005.01.022
![]() |
[45] |
M. Peng, X. Yi, R. Cheng, Rich dynamics caused by diffusion, Nonlinear Dyn, 111 (2023), 9201–9213. https://doi.org/10.1007/s11071-023-08307-y doi: 10.1007/s11071-023-08307-y
![]() |
[46] | M. Prayitno, S. Utama, S. Aminah, D. Silaban, Properties of adjacency, in-degree laplacian, and out-degree laplacian matrices of directed cyclic sun graph, in AIP Conference Proceedings, 2192 (2019), 040011. https://doi.org/10.1063/1.5139137 |
[47] |
A. Rahimabadi, H. Benali, Extended fractional-polynomial generalizations of diffusion and fisher–kpp equations on directed networks, Chaos, Soliton Fractals, 174 (2023), 113771. https://doi.org/10.1016/j.chaos.2023.113771 doi: 10.1016/j.chaos.2023.113771
![]() |
[48] | W. Ren, R. Beard, E. Atkins, A survey of consensus problems in multi-agent coordination, in Proceedings of the 2005, American Control Conference, (2005), 1859–1864. https://doi.org/10.1109/ACC.2005.1470239 |
[49] | J. Rotman, An Introduction to the Theory of Groups, Springer, New York, 1999. |
[50] | R. Saber, R. Murray, Consensus protocols for networks of dynamic agents, in Proceedings of the 2003 American Control Conference, 2 (2003), 951–956. |
[51] | B. Sagan, The Symmetric Group: Representations, Combinatorial Algorithms, and Symmetric Functions, Springer, New York, 2001. |
[52] |
R. Sahasrabuddhe, L. Neuhäuser, R. Lambiotte, Modelling non-linear consensus dynamics on hypergraphs, J. Phys. Complex, 2 (2021), 025006. https://doi.org/10.1088/2632-072X/abcea3 doi: 10.1088/2632-072X/abcea3
![]() |
[53] |
G. Shi, C. Altafini, J. Baras, Dynamics over signed networks, SIAM Rev., 61 (2019), 229–257. https://doi.org/10.1137/17M1134172 doi: 10.1137/17M1134172
![]() |
[54] |
M. J. Simpson, R. E. Baker, S. W. McCue, Models of collective cell spreading with variable cell aspect ratio: a motivation for degenerate diffusion models, Phys. Rev. E, 83 (2011), 021901. https://doi.org/10.1103/PhysRevE.83.021901 doi: 10.1103/PhysRevE.83.021901
![]() |
[55] |
V. Srivastava, J. Moehlis, F. Bullo, On bifurcations in nonlinear consensus networks, J. Nonlinear Sci., 21 (2011), 875–895. https://doi.org/10.1007/s00332-011-9103-4 doi: 10.1007/s00332-011-9103-4
![]() |
[56] | J. L. Vázquez, The porous medium equation: mathematical theory, Oxford University Press, Oxford, 2007. |
[57] |
J. Veerman, E. Kummel, Diffusion and consensus on weakly connected directed graphs, Linear Algebra Appl., 578 (2019), 184–206. https://doi.org/10.1016/j.laa.2019.05.014 doi: 10.1016/j.laa.2019.05.014
![]() |
[58] | J. Veerman, R. Lyons, A primer on laplacian dynamics in directed graphs, preprint, arXiv: 2002.02605. |
[59] |
A. Vidal, J. P. Françoise, Canards cycles in global dynamics, Int. J. Bifurcation Chaos, 22 (2012), 1250026. https://doi.org/10.1142/S0218127412500265 doi: 10.1142/S0218127412500265
![]() |
[60] | M. Wechselberger, Geometric Singular Perturbation Theory Beyond the Standard Form, Springer Cham, Cham, 2020. https://doi.org/10.1007/978-3-030-36399-4 |
[61] |
J. Wei, X. Yi, H. Sandberg, K. Johansson, Nonlinear consensus protocols with applications to quantized communication and actuation, IEEE Trans. Control Network Syst., 6 (2019), 598–608. https://doi.org/10.1109/TCNS.2018.2860461 doi: 10.1109/TCNS.2018.2860461
![]() |
[62] | S. Wiggins, Introduction to Applied Nonlinear Dynamical Systems and Chaos, Springer, New York, 2003. |
[63] | S. Wolfram, The reform of mathematical notation, Wolfram Research, 2013. Available from: https://content.wolfram.com/sites/39/2015/02/MathematicalNotation-SW.pdf. |
[64] | H. Zhang, J. Chen, Bipartite consensus of general linear multi-agent systems, in 2014 American Control Conference, (2014), 808–812. https://doi.org/10.1109/ACC.2014.6858991 |