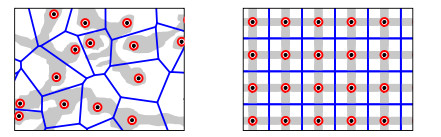
In this first part of a series of 3 papers, we set up a framework to study the existence of uniformly bounded extension and trace operators for W1,p-functions on randomly perforated domains, where the geometry is assumed to be stationary ergodic. We drop the classical assumption of minimally smoothness and study stationary geometries which have no global John regularity. For such geometries, uniform extension operators can be defined only from W1,p to W1,r with the strict inequality r<p. In particular, we estimate the Lr-norm of the extended gradient in terms of the Lp-norm of the original gradient. Similar relations hold for the symmetric gradients (for Rd-valued functions) and for traces on the boundary. As a byproduct we obtain some Poincaré and Korn inequalities of the same spirit.
Such extension and trace operators are important for compactness in stochastic homogenization. In contrast to former approaches and results, we use very weak assumptions: local (δ,M)-regularity to quantify statistically the local Lipschitz regularity and isotropic cone mixing to quantify the density of the geometry and the mesoscopic properties. These two properties are sufficient to reduce the problem of extension operators to the connectivity of the geometry. In contrast to former approaches we do not require a minimal distance between the inclusions and we allow for globally unbounded Lipschitz constants and percolating holes. We will illustrate our method by applying it to the Boolean model based on a Poisson point process and to a Delaunay pipe process, for which we can explicitly estimate the connectivity terms.
Citation: Martin Heida. Stochastic homogenization on perforated domains Ⅰ – Extension Operators[J]. Networks and Heterogeneous Media, 2023, 18(4): 1820-1897. doi: 10.3934/nhm.2023079
[1] | Martin Heida, Benedikt Jahnel, Anh Duc Vu . Regularized homogenization on irregularly perforated domains. Networks and Heterogeneous Media, 2025, 20(1): 165-212. doi: 10.3934/nhm.2025010 |
[2] | Hakima Bessaih, Yalchin Efendiev, Florin Maris . Homogenization of the evolution Stokes equation in a perforated domain with a stochastic Fourier boundary condition. Networks and Heterogeneous Media, 2015, 10(2): 343-367. doi: 10.3934/nhm.2015.10.343 |
[3] | Martin Heida . Stochastic homogenization on perforated domains III–General estimates for stationary ergodic random connected Lipschitz domains. Networks and Heterogeneous Media, 2023, 18(4): 1410-1433. doi: 10.3934/nhm.2023062 |
[4] | Nils Svanstedt . Multiscale stochastic homogenization of monotone operators. Networks and Heterogeneous Media, 2007, 2(1): 181-192. doi: 10.3934/nhm.2007.2.181 |
[5] | Patrick Henning . Convergence of MsFEM approximations for elliptic, non-periodic homogenization problems. Networks and Heterogeneous Media, 2012, 7(3): 503-524. doi: 10.3934/nhm.2012.7.503 |
[6] | Mogtaba Mohammed, Mamadou Sango . Homogenization of nonlinear hyperbolic stochastic partial differential equations with nonlinear damping and forcing. Networks and Heterogeneous Media, 2019, 14(2): 341-369. doi: 10.3934/nhm.2019014 |
[7] | Valeria Chiado Piat, Sergey S. Nazarov, Andrey Piatnitski . Steklov problems in perforated domains with a coefficient of indefinite sign. Networks and Heterogeneous Media, 2012, 7(1): 151-178. doi: 10.3934/nhm.2012.7.151 |
[8] | Luca Lussardi, Stefano Marini, Marco Veneroni . Stochastic homogenization of maximal monotone relations and applications. Networks and Heterogeneous Media, 2018, 13(1): 27-45. doi: 10.3934/nhm.2018002 |
[9] | Brahim Amaziane, Leonid Pankratov, Andrey Piatnitski . Homogenization of variational functionals with nonstandard growth in perforated domains. Networks and Heterogeneous Media, 2010, 5(2): 189-215. doi: 10.3934/nhm.2010.5.189 |
[10] | Luis Caffarelli, Antoine Mellet . Random homogenization of fractional obstacle problems. Networks and Heterogeneous Media, 2008, 3(3): 523-554. doi: 10.3934/nhm.2008.3.523 |
In this first part of a series of 3 papers, we set up a framework to study the existence of uniformly bounded extension and trace operators for W1,p-functions on randomly perforated domains, where the geometry is assumed to be stationary ergodic. We drop the classical assumption of minimally smoothness and study stationary geometries which have no global John regularity. For such geometries, uniform extension operators can be defined only from W1,p to W1,r with the strict inequality r<p. In particular, we estimate the Lr-norm of the extended gradient in terms of the Lp-norm of the original gradient. Similar relations hold for the symmetric gradients (for Rd-valued functions) and for traces on the boundary. As a byproduct we obtain some Poincaré and Korn inequalities of the same spirit.
Such extension and trace operators are important for compactness in stochastic homogenization. In contrast to former approaches and results, we use very weak assumptions: local (δ,M)-regularity to quantify statistically the local Lipschitz regularity and isotropic cone mixing to quantify the density of the geometry and the mesoscopic properties. These two properties are sufficient to reduce the problem of extension operators to the connectivity of the geometry. In contrast to former approaches we do not require a minimal distance between the inclusions and we allow for globally unbounded Lipschitz constants and percolating holes. We will illustrate our method by applying it to the Boolean model based on a Poisson point process and to a Delaunay pipe process, for which we can explicitly estimate the connectivity terms.
In 1979 Papanicolaou and Varadhan [22] and Kozlov [15] for the first time independently introduced concepts for the averaging of random elliptic operators. At that time, the periodic homogenization theory had already advanced to some extend (as can be seen in the book [23] that had appeared one year before) dealing also with non-uniformly elliptic operators [17] and domains with periodic holes [3]. The most recent and most complete work for extension operators on periodically perforated domains is [11].
In contrast, the homogenization on randomly perforated domains is still open to a large extend. Recent results focus on minimally smooth domains [9,24] or on decreasing size of the perforations when the smallness parameter tends to zero [8] (and references therein). The main issue in homogenization on perforated domains compared to classical homogenization problems is compactness. For elasticity, this is completely open.
The results presented below are meant for application in quenched homogenization, i.e. when one special but also typical realization of the random geometry is picked as a working model for the perforated domain. The presented estimates for the extension and trace operators strongly depend on this given realization of the geometry - thus on the random variable ω. However, in order to have a law of large numbers, which allows us to consider ever larger domains (or arbitrary scaling ε>0), we will assume that the geometry is stationary. This implies that even though the constant depends on the given realization, still such an estimate can be achieved for almost every ω.
In order to illustrate the issues in stochastic homogenization on perforated domains, we introduce the following example.
Let P(ω)⊂Rd be a stationary random open set and let ε>0 be the smallness parameter and let ˜P(ω) be an infinitely connected component (i.e., an unbounded connected domain) of P(ω). For a bounded open domain Q, we consider Qε˜P(ω):=Q∩ε˜P(ω) and Γε(ω):=Q∩ε∂˜P(ω) with outer normal νΓε(ω). For a sufficiently regular and Rd-valued function u we denote ∇su:=12(∇u+(∇u)⊤) the symmetric part of ∇u. A typical homogenization problem then is the following:
−div(|∇suε|p−2∇suε)=g(uε)on Qε˜P(ω),u=0on ∂Q∩(εP),|∇suε|p−2∇uε⋅νΓε(ω)=f(uε)on Γε(ω). | (1.1) |
Note that for simplicity of illustration, the only randomness that we consider in this problem is due to P(ω).
One way to prove homogenization of Eq (1.1) is to prove Γ-convergence of
Eε,ω(u)=∫Qε˜P(ω)(1p|∇su|p−G(u))+∫Γε(ω)F(u), |
in a suitably chosen space where G′=g and F′=f. Conceptually, this implies convergence of the minimizers uε to a minimizer of a limit functional but if G or F are non-monotone, we need compactness. However, the minimizers are elements of W1,p(Qε˜P):=W1,p(Qε˜P;Rd) and since this space changes with ε, there is apriori no compactness of uε, even though we have uniform apriori estimates on the symmetric gradients.
One of the canonical paths to circumvent this issue in periodic homogenization–i.e., when P is a periodic set–is via uniformly bounded extension operators Uε:W1,p(Qε˜P)→W1,p(Q) that share the property that for some C>0 independent from ε it holds for all u∈W1,p(Qε˜P) with u|Rd∖Q≡0
‖∇Uεu‖Lp(Q)≤C‖∇u‖Lp(Qε˜P),‖Uεu‖Lp(Q)≤C‖u‖Lp(Qε˜P), | (1.2) |
see [11,12], combined with uniformly bounded trace operators, see [7,9]. Such operators have also been provided for elasticity problems [11,21,30,31], i.e.,
‖∇sUεu‖Lp(Q)≤C‖∇su‖Lp(Qε˜P). |
The last estimate then allows to use Korn's inequality combined with Sobolev's embedding theorem to find Uεuε⇀u0 weakly in W1,p(Q).
What is the classical strategy? The existing results on extension and trace operators for random domains are focused on a.s. minimally smooth domains: A connected domain P⊂Rd is minimally smooth [26] if there exist (δ,M) such that for every x∈∂P the set ∂P∩Bδ(x) is the graph of a Lipschitz continuous function with Lipschitz constant less than M. It is further assumed that the complement Rd∖P consists of uniformly bounded sets. This concept leads to almost sure construction of uniformly bounded extension operators W1,ploc(P)→W1,p(Rd) [9] in the sense that for every bounded domain Q and every u∈W1,p(Rd∩P) with u|Rd∖Q≡0 holds
‖∇Uu‖Lp(Q)≤C‖∇u‖Lp(Q∩P),‖Uu‖Lp(Q)≤C‖u‖Lp(Q∩P), | (1.3) |
with C independent from Q. Similarly, one obtains for the trace T that [24]
‖Tu‖Lp(Q∩∂P)≤C(‖u‖Lp(Q∩P)+‖∇u‖Lp(Q∩P)). |
Using a scaling argument to obtain e.g., Eq (1.2), such extension and trace operators are typically used in order to deal with nonlinearities in homogenization problems.
Why does this work? The theory cited above is directly connected to the theory of Jones [13] and Duran and Muschietti [5] on so-called John domains. These are precisely the bounded domains P that admit extension operators W1,p(P)→W1,p(Rd) satisfying
‖Uu‖W1,p(Rd)≤C‖u‖W1,p(Q∩P). |
Definition (John domains). A bounded domain P⊂Rd is a John domain (a.k.a (ε,δ)-domain) if there exists ε,δ>0 such that for every x,y∈P with |x−y|<δ there exists a rectifiable path γ:[0,1]→P from x to y such that
lengthγ≤1ε|x−y|and∀t∈(0,1):infz∈Rd∖P|γ(t)−z|≥ε|x−γ(t)||γ(t)−y||x−y|. |
Because of the locality implied by δ, it is possible to glue together local extension operators on John domains such as done in [11] for periodic or [9] for minimally smooth domains. In the stochastic case [9] one benefits a lot from the uniform boundedness of the components of Rd∖P, which allows to split the extension problem into independent extension problems on uniformly John-regular domains.
Why this is not enough for general random domains? As one could guess from the emphasis that is put on the above explanations, random geometries are merely minimally smooth or even John. On an unbounded random domain P, the constant M can locally become very large in points x∈∂P, while simultaneously, δ can become very small in the very same x. In fact, they are not even "uniformly John" as the following, yet deterministic example illustrates.
Example 1.1. Considering
P:={(x1,x2)∈R2:∃n∈N:x1−(2n+1)∈(−1,1],x2<max{1,n|x1−(2n+1)|}} |
the Lipschitz constant on (2n,2n+2) is n and it is easy to figure out that this non-uniformly Lipschitz domain violates the John condition due to the cusps. Hence, a uniform estimate of the form Eq (1.3) cannot exist.
Therefore, an alternative concept to measure the large scale regularity of a random geometry is needed. Since the classical results do not exclude the existence of an estimate
1|Q|∫Q|∇Uu|r≤(C|Q|∫Q∩Br(P)|∇u|p)rp,1|Q|∫Q|Uu|r≤(C|Q|∫Q∩Br(P)|u|p)rp, | (1.4) |
or
1|Q|∫Q|∇sUu|r≤(C|Q|∫Q∩Br(P)|∇su|p)rp,1|Q|∫Q|Uu|r≤(C|Q|∫Q∩Br(P)|u|p)rp, | (1.5) |
where 1≤r<p and C is independent from Q, such inequalities will be our goal.
Our results in a nutshell We will provide inequalities of the form Eqs (1.4) and (1.5) for a Voronoi-pipe model and for a Boolean model. On the way, we will provide several concepts and intermediate results that can be reused in further examples and general considerations such as in Part Ⅲ of this series. Scaled versions (replacing ε=m−1 in Theorems 1.15 and 1.17) of Eqs (1.4) and (1.5) can be formulated for functions
u∈W1,p0,∂Q(εP∩Q):={u∈W1,p(Q∩εP):u|(εP)∩∂Q≡0}, |
and will be of the form
1|Q|∫Rd|∇Uεu|r≤C(1|Q|∫Q∩εP|∇u|p)rp,1|Q|∫Rd|Uεu|r≤C(1|Q|∫Q∩εP|u|p)rp, |
resp.
1|Q|∫Rd|∇sUεu|r≤C(1|Q|∫Q∩εP|∇su|p)rp,1|Q|∫Rd|Uεu|r≤C(1|Q|∫Q∩εP|u|p)rp. |
We will further show that there exists β∈(0,1) such that the support of Uεu lies within Bεβ(Q) for ε small enough. This is a compensation for the property of periodic geometries, where the extended function has support within Bε(Q)⊂Bεβ(Q) (for 0<ε,β<1).
On the way to our final results, we will face the following problems that have to be overcome:
1. Quantifying local and global properties of the random geometry that are involved in the extension operator: These quantities are related to the local Lipschitz regularity, the mesoscopic distribution of mass and the connectivity of the perforated domain.
2. The "local" norm of the extension operator, i.e., when restricted to a given bounded domain, will be related to a polynomial expression on the above mentioned quantities. In order to find an upper bound for the local norms, i.e., one constant to bound the extension operator on all bounded domains, we need a law of large numbers for the involved geometric quantities. This law of large numbers will be provided by stationarity and ergodicity of the geometry.
3. In order to use the stationarity of the geometry, we are requested to show that they are Borel measurable. This allows one to consider them as stationary random variables and to apply the ergodic theorem.
While the author considers the first topic as the essential contribution of this article, the other two points are technically necessary to make the theory work. Many of the background provided below may sound very familiar to many readers, even if some details in the formulation are new. However, the author strongly suggest that the reader may have a detailed look at the following parts:
● Sections 2.8, 2.11 and Lemma 2.60
● Lemmas 2.60–3.9 and 3.17–3.18
● Lemmas 4.7 and 4.10
● This may be enough to develop a basic understanding how the concepts interact in the final proofs of Section 4.10.
As a replacement for periodicity, we introduce the concept of mesoscopic regularity of a stationary random open set:
Definition 1.2 (Mesoscopic regularity). Let P be a stationary ergodic random open set, let ˜f be a positive, monotonically decreasing function ˜f with ˜f(R)→0 as R→∞ and let r>0 such that
P(∃x∈BR(0):B4√dr(x)⊂BR(0)∩P)≥1−˜f(R). | (1.6) |
Then P is called (r,˜f)-mesoscopic regular. P is called polynomially (exponentially) regular if 1/˜f grows polynomially (exponentially).
As a consequence of Lemmas 3.14, 3.16 and 3.17 we obtain the following.
Corollary 1.3 (Stationary ergodic random open sets are mesoscopic regular, see Figure 1).
Let P(ω) be a stationary ergodic random open set. Then there exists r>0 and a monotonically decreasing function with ˜f(R)→0 as R→∞ such that P is (r,˜f)-mesoscopic regular.
Furthermore, there exists a jointly stationary random point process Xr(ω)=(xa)a∈N and for every a∈N it holds Br2(xa)⊂P and for all a,b∈N, a≠b, it holds |xa−xb|>2r.
Construct from Xr a Voronoi tessellation of cells Ga with diameter da=d(xa). Then for some constant C>0 and some monotone decreasing f:(0,∞)→R and C>0 with f(R)≤C˜f(C−1R) it holds
P(d(xa)>D)<f(D). |
The objects r, Xr and f from Corollary 1.3 will play a central role in the analysis: They are connected to the stationarity of the geometry and will hence serve to construct a substitute for the periodic unit cell. We summarize some of their properties in the following.
Assumption 1.4. Let P be a Lipschitz domain and assume there exists a set of points Xr=(xa)a∈N having mutual distance |xa−xb|>2r if a≠b and with Br2(xa)⊂P for every a∈N (e.g., Xr(P), see Eq (2.53)).
The second important concept to quantify in a stochastic manner is that of local Lipschitz regularity.
Definition 1.5 (Local (δ,M)-Regularity). Let P⊂Rd be an open set. P is called (δ,M)-regular in p0∈∂P if there exists an open set U⊂Rd−1 and a Lipschitz continuous function ϕ:U→R with Lipschitz constant greater or equal to M such that ∂P∩Bδ(p0) is subset of the graph of the function φ:U→Rd,˜x↦(˜x,ϕ(˜x)) in some suitable coordinate system.
Every Lipschitz domain P is locally (δ,M)-regular in every p0∈∂P. In what follows, we bound δ from above by r only for practical reasons in the proofs. The following quantities can be derived from local (δ,M)-regularity.
Definition 1.6. For a Lipschitz domain P⊂Rd and for every p∈∂P and n∈N∪{0}
δΔ(p):=supδ<r{∃M>0:P is (δ,M)-regular in p}, | (1.7) |
∀r>0,Mr(p):=infη>rinf{M:P is (η,M)-regular in p}, | (1.8) |
ρn(p):=supr<δ(p)r(4Mr(p)2+2)−n2, | (1.9) |
If no confusion occurs, we write δ=δΔ. Furthermore, for c∈(0,1] let η(p)=cδΔ(p) or η(p)=cρn(p), n∈N and r∈C0,1(∂P) and define
η[r],Rd(x):=inf{η(˜x):˜x∈∂Ps.t. x∈Br(˜x)(˜x)}, | (1.10) |
M[r,η],Rd(x):=sup{Mr(˜x)(˜x):˜x∈∂Ps.t. x∈¯Bη(˜x)(˜x)}, | (1.11) |
where inf∅=sup∅:=0 for notational convenience. We also write M[η],Rd(x):=M[η,η],Rd(x) and ηRd(x):=η[η],Rd(x). Of course, we can also consider M[r],∂P:p↦Mr(p)(p) as a function on ∂P, and we will do this once in Lemma 3.9.
When it comes to application of the abstract results found below, it is important to have in mind that η and Mr are quantities on ∂P, while η[r],Rd and M[r,η],Rd are quantities on Rd. Hence, while trivially
P(η[r],Rd∈(η1,η2))=limn→∞n−d|Q|−1|{x∈nQ:η[r],Rd∈(η1,η2)}| |
(and similarly for M[r,η],Rd) for every convex bounded open Q, we have in mind
P(η∈(η1,η2))=(limn→∞Hd−1(∂P∩nQ))−1Hd−1({x∈(nQ)∩∂P:η∈(η1,η2)}). |
We will prove measurability of η[r],Rd and M[r,η],Rd in Lemma 3.11 and see how the weighted expectations of η[r],Rd and M[r,η],Rd can be estimated by weighted expectations of M and η in Lemma 3.12.
The first important result is the boundedness of the trace operator.
Theorem 1.7. Let P⊂Rd be a Lipschitz domain, 18>r>0 and let Q⊂Rd be a bounded open set and let 1≤r<p0<p. Then the trace operator T satisfies for every u∈W1,ploc(P)
1|Q|∫Q∩∂P|Tu|r≤C(1|Q|∫B14(Q)∩P|u|p+|∇u|p)rp |
where for some constant C0 depending only on p0, p and r and d and for ˜ρ=2−5ρ1 one has
C=C0(1|Q|∫B14r(Q)∩∂P˜ρ−1p0−rRd)p0−rp0(1|Q|∫B14r(Q)∩P(1+˜M[132δ],Rd)p(p−10+1+ˆd)p−p0)p−p0p0p, | (1.12) |
C=C0(1|Q|∫B14r(Q)∩∂P(˜ρRd(1+˜M[132δ],Rd))−1p−r)p−rp. | (1.13) |
Proof. This is proved in Section 4.6.
In view of Corollary 3.8 and recalling Eq (1.9), for every n=1 or n=2 there exist a complete covering of ∂P by balls B˜ρn(pni)(pni), (pni)i∈N, where ˜ρn(p):=2−5ρn(p). We write ˜ρn,i:=˜ρn(pni). The theory we develop is further using the following two quantities.
Definition 1.8 (Microscopic regularity (see also Figure 2)). The inner microscopic regularity α is
α:=inf{˜α≥0:∀p∈∂P∃y∈P:B˜ρ(p)/32(1+M˜ρ(p)(p)˜α)(y)⊂B˜ρ(p)/8(p)}. |
In Lemma 3.1 we will see that indeed α≤1.
Definition 1.9 (Extension order (see also Figure 2)). The geometry is of extension order n∈N∪{0} if there exists C>0 such that for almost every p∈∂P there exists a local extension operator
U:W1,p(B18δ(p)(p)∩P)→W1,p(B18ρn(p)(p)),‖∇Uu‖Lp(B18ρn(p)(p))≤C(1+M18δ(p)(p))‖∇u‖Lp(B18δ(p)(p)). | (1.14) |
The geometry is of symmetric extension order n∈N∪{0} if there exists C>0 such that for almost every p∈∂P there exists a local extension operator
U:W1,p(B18δ(p)(p)∩P)→W1,p(B18ρn(p)(p)),‖∇sUu‖Lp(B18ρn(p)(p))≤C(1+M18δ(p)(p))2‖∇su‖Lp(B18δ(p)(p)). | (1.15) |
Corollary 3.7 shows that every locally Lipschitz geometry is of extension order n=1 and every locally Lipschitz geometry is of symmetric extension order n=2. However, for particular geometries better results for n are possible, as we will see below.
Let X=(xa)a∈N be a jointly stationary point process with P such that Br(X)⊂P. In this work, we will often assume that |xa−xb|>2r for all a≠b for simplicity in Sections 5 and 6. The existence of such a process is always guarantied by Lemmas 3.14 and 3.16. Its choice in a concrete example is, however, delicate. Worth mentioning, most of the theory developed until the end of Section 4 (Except for Lemmas 3.17 and 3.18 which are not used before Section 5), is completely independent from this mutual minimal distance assumption.
From X we construct a Voronoi tessellation with cells (Ga)a∈N and we chose for each xa a radius ra≤r with Bra(xa)⊂Ga∩P. Again, using Corollary 1.3, we assume that ra=r is constant for simplicity.
Notation. Given an extension order n∈{0,1} and microscopic regularity α∈[0,1] we chose
rn,α,i:=˜ρn,i/32(1+M˜ρn,i(pn,i)α) | (1.16) |
and some yn,α,i such that
Bn,α,i:=Brn,α,i(yn,α,i)⊂P∩B18˜ρn,i(pn,i). | (1.17) |
For every i and a, we define
τn,α,iu:=−∫Bn,α,iu,Mau:=−∫Bra16(xa)u, |
local averages close to ∂P and in "bulk points" xa. We say that xa∼∼xb if Ga∩Br(Gb)≠∅ and we say xa∈Xr(Q) if Br(Ga)∩Q≠∅. Based on the explicit extension operator U given in (4.14) below we will obtain the following result.
Theorem 1.10. Let r>0 and let P⊂Rd be a stationary ergodic random Lipschitz domain such that Assumption 1.4 holds for X=(xa)a∈N and P has microscopic regularity α with extension order n. Let Q⊂Rd be a bounded open set with B14(0)⊂Q and let 1≤r<p. Furthermore, let
E(((1+M[3δ8,δ8],Rd)nd(1+M[18δ],Rd)r(1+M[˜ρn],Rd)α(d−1))pp−r)<∞ |
then there exist C>0 depending only on d, r and p such that for a.e. ω there exists an extension operator Uω:W1,ploc(P(ω))→W1,ploc(Rd) and Cω>0 such that for every m≥1 and every u∈W1,p(P(ω)) with u|P(ω)∖mQ≡0 it holds
1|mQ|∫mQ|∇(Uωu)|r≤Cω(1md∫P∩Br(mQ)|∇u|p)rp+C1md∫P∩Br(mQ)∑i≠0∑a˜ρ−rPχBr2(Ga)χB˜ρn,i(pn,i)|τn,α,iu−Mau|r+C|1md∫P∩mQ∑a∑a∼∼bχBr(Ga)|Mau−Mbu||r,1|mQ|∫mQ|Uωu|r≤Cω(1md∫P∩Br(mQ)|u|p)rp. |
Proof. This is a consequence of Lemma 4.7.
Imposing less restrictions on ˜ρ and M leads to a weaker estimate on the extension operator.
Theorem 1.11. Under the assumptions of Theorem 1.10 let additionally
E(˜ρ−rpp−rP)<∞ |
then there exists an extension operator Uω:W1,ploc(P(ω))→W1,ploc(Rd) such that for every m≥1 and every u∈W1,p(P(ω)) with u|P(ω)∖mQ≡0 it holds
1|mQ|∫mQ(|∇(Uωu)|r+|Uωu|r)≤Cω(1md∫P∩Br(mQ)(|∇u|p+|u|p))rp. |
Proof. This is a consequence of the proof of Lemma 4.7, replacing Mau in the definition of Uu by 0.
The terms depending on |τn,α,iu−Mau| or |Mau−Mau| appearing on the right-hand side in Theorem 1.10 need to be replaced by an integral over |∇u|p. Here, the pathwise topology of the geometry comes into play. By this we mean that we have to integrate the gradient of u over a path connecting e.g., pi and xa. Here, the mesoscopic properties of the geometry will play a role. In particular, we need pathwise connectedness of the random domain, a phenomenon which is known as percolation in the theory of random sets. We will discuss two different examples to see that these terms can indeed be handled in application, but shift a general discussion of arbitrary geometries to a later publication.
We now turn to the situation that u is a Rd-valued function and that the given PDE system yields only estimates for ∇su=12(∇u+(∇u)T). We introduce the following quantities:
Definition 1.12. Given n∈{0,1,2} and α∈[0,1] such that (1.17) holds for ri=rn,α,i for every i let for i,a
ˉ∇⊥n,α,iu:=−∫Brn,α,i(yn,α,i)(∇u−∇su),[τsn,α,iu](x):=ˉ∇⊥n,α,iu(x−y2,i)+−∫Brn,α,i(yn,α,i)u,ˉ∇⊥au:=−∫Bra16(xa)(∇u−∇su),[Msau](x):=ˉ∇⊥au(x−xa)+−∫Bra16(xa)u. |
Using above introduced notation and the symbol W to denote Rd-valued Sobolev spaces, we find the following.
Theorem 1.13. Let r>0 and let P⊂Rd be a stationary ergodic random Lipschitz domain such that Assumption 1.4 holds for X=(xa)a∈N and P has microscopic regularity α with symmetric extension order n≤2. Let Q⊂Rd be a bounded open set with B14(0)⊂Q and let 1≤r<p0<p. Furthermore, let
E(((1+M[3δ8,δ8],Rd)nd(1+M[18δ],Rd)2r(1+M[˜ρn],Rd)α(d−1))pp−r)<∞ |
Then there exist C>0 depending only on d, r, s and p such that for a.e. ω there exists an extension operator Uω:W1,ploc(P(ω))→W1,ploc(Rd) and Cω>0 such that for every m≥1 and every u∈W1,p(P(ω)) with u|P(ω)∖Q≡0 it holds
1|Q|∫Q|∇s(Uωu)|r≤Cω(1|Q|∫Br(Q)∩P|∇su|p)rp+C1|Q|∫Q∖P∑a∑i≠0ρ−r1,iχA1,iχA1,a|τsn,α,iu−Msau|r+1|Q|∫Q|d∑l=1∑a:∂lΦa>0∑b:∂lΦb<0∂lΦa|∂lΦb|DΦl+(Msau−Msbu)|r,1|Q|∫Q|Uωu|r≤Cω(1|Q|∫Br(Q)∩P|u|p)rp, |
Proof. This is a consequence of Lemma 4.10.
Theorem 1.14. Under the assumptions of Theorem 1.13 let additionally
E(˜ρ−rpp−rP)<∞. |
Then there exists an extension operator Uω:W1,ploc(P(ω))→W1,ploc(Rd) such that for every m≥1 and every u∈W1,p(P(ω)) with u|P(ω)∖mQ≡0 it holds
1|mQ|∫mQ(|∇(Uωu)|r+|Uωu|r)≤Cω(1md∫P∩Br(mQ)(|∇u|p+|u|p))rp. |
Proof. This is a consequence of the proof of Lemma 4.7, replacing Msau in the definition of Uu by 0.
In Section 6 we discuss two standard models from the theory of stochastic geometries. The first one is a system of random pipes: Starting from a Poisson point process and deleting all points with nearest neighbor closer than 2r and introducing the Delaunay neighboring condition on the points, every two neighbors are connected through a pipe of random thickness 2δ, where δ is distributed i.i.d among the pipes and we complete the geometry by adding a ball of radius r2 around each point. Defining for bounded open domains Q⊂Rd and n∈N
u∈W1,p0,∂(nQ)(P∩nQ):={u∈W1,p(P∩nQ):u|∂(nQ)≡0}, |
and using again W instead of W for Rd-valued functions, we find our first result:
Theorem 1.15. In the pipe model of Section 6.1 let P(δ(x,y)<δ0)≤Cδδβ0 and let 1≤r<s<p be such that max{p(s+d)p−s,p(2d−s−1)p−s}≤β and srs−r≤β+d−1. Then α=n=0 both for extension and symmetric extension order and there almost surely exists an extension operator U:W1,ploc(P)→W1,ploc(Rd) and constants C,R>1 such that for all m∈N and every u∈W1,p0,∂(mQ)(P∩mQ) it holds
1|mQ|∫Rd|∇(Uu)|r≤C(1md∫P∩mQ|∇u|p)rp. |
Furthermore there almost surely exists an extension operator U:W1,ploc(P)→W1,ploc(Rd) and a constant C>0 such that for all m∈N and every u∈W1,p0,∂(mQ)(P∩mQ)
1|mQ|∫Rd|∇s(Uu)|r≤C(1md∫P∩mQ|∇su|p)rp. |
In both cases for every β∈(0,1) the following holds: for some m0>1 depending on ω and every m>m0 the support of Uu lies within Bm1−β(mQ).
Proof. The proof is given at the very end of Section 6.1.
Corollary 1.16. If P(δ(x,y)<δ0)≤Cδe−γδ−10 then the last theorem holds for every 1≤r<p.
In Section 6.2 we study the Boolean model based on a Poisson point process in the percolation case. Introduced in Example 2.49 we will consider a Poisson point process Xpois(ω)=(xi(ω))i∈N with intensity λ (recall Example 2.49). To each point xi a random ball Bi=B1(xi) is assigned and the family B:=(Bi)i∈N is called the Poisson ball process. We say that xi∼xj if |xi−xj|<2. In case λ>λc, where λc>0 is the percolation threshold, the union of these balls has a unique infinite connected component (that means we have percolation) and we denote Xpois,∞ the selection of all points that contribute to the infinite component and P∞(ω):=⋃i∈Xpois,∞Bi this infinite open set and seek for a corresponding uniform extension operator. The connectedness of P∞ is hereby essential as we use results from percolation theory that otherwise would not hold.
Here we can show that the micro- and mesoscopic assumptions are fulfilled, at least in case P is given as the union of balls. If we choose P as the complement of the balls, the situation becomes more involved. On one hand, Theorem 6.8 shows that α and n change in an unfortunate way. Furthermore, the connectivity estimate remains open. However, some of these problems might be overcome in the future using a Matern modification of the Poisson process. For the moment, we state the following.
Theorem 1.17. In the Boolean model of Section 6.2 it holds α=0 in case P=P∞ and both the extension order and the symmetric extension order are n=0. If d<p and
prp−r<2,r<d+2 |
Then there almost surely exists an extension operator U:W1,ploc(P)→W1,ploc(Rd) and a constant C>0 such that for all m∈N and every u∈W1,p0,∂Q(P∩mQ)
1|mQ|∫mQ|∇(Uu)|r≤C(1md∫P∩mQ|∇u|p)rp. |
If furthermore
r<d+22 |
then there almost surely exists an extension operator U:W1,ploc(P)→W1,ploc(Rd) and a constant C>0 such that for all m∈N and every u∈W1,p0,∂Q(P∩mQ)
1|mQ|∫mQ|∇s(Uu)|r≤C(1md∫P∩mQ|∇su|p)rp. |
In both cases for every β∈(0,1) the following holds: for some m0>1 depending on ω and every m>m0 the support of Uu lies within Bm1−β(mQ).
Proof. The proof is given at the very end of Section 6.2.
We close the introduction by providing an overview over the article and its main contributions. In Section 2 we collect some basic concepts and inequalities from the theory of Sobolev spaces, random geometries and discrete and continuous ergodic theory. We furthermore establish local regularity properties for what we call η-regular sets, as well as a related covering theorem in Section 2.8. In Section 2.13 we will demonstrate that stationary ergodic random open sets induce stationary processes on Zd, a fact which is used later in the construction of the mesoscopic Voronoi tessellation in Section 3.2.
In Section 3 we introduce the regularity concepts of this work. More precisely, in Section 3.1 we introduce the concept of local (δ,M)-regularity and use the theory of Section 2.8 in order to establish a local covering result for ∂P, which will allow us to infer most of our extension and trace results. In Section 3.2 we show how isotropic cone mixing geometries allow us to construct a stationary Voronoi tessellation of Rd such that all related quantities like "diameter" of the cells are stationary variables whose expectation can be expressed in terms of the isotropic cone mixing function f. Moreover we prove the important integration Lemma 3.18.
In Sections 4–5 we finally provide the aforementioned extension operators and prove estimates for these extension operators and for the trace operator. In Section 6 we study the sample geometries from Theorems 1.15 and 1.17.
This article uses concepts from partial differential equations, measure theory, probability theory and random geometry. Additionally, we introduce concepts which we believe have not been introduced before. This makes it difficult to introduce readable self contained notation (the most important aspect being symbols used with different meaning in different disciplines) and enforces the use of various different mathematical fonts. Therefore, we provide an index of notation at the end of this work. As a rough orientation, the reader may keep the following in mind:
We use the standard notation N, Q, R, Z for natural (>0), rational, real and integer numbers. P denotes a probability measure, E the expectation. Furthermore, we use special notation for some geometrical objects, i.e., Td=[0,1)d for the torus (T equipped with the topology of the torus), Id=(0,1)d the open interval as a subset of Rd (we often omit the index d), B a ball, C a cone and X a set of points. In the context of finite sets A, we write #A for the number of elements.
Bold large symbols (U, Q, P, …) refer to open subsets of Rd or to closed subsets with ∂P=∂˚P. The Greek letter Γ refers to a d−1 dimensional manifold.
Calligraphic symbols (A, U, …) usually refer to operators and large Gothic symbols (B,C,…) indicate topological spaces, except for A.
This work is the first part of a trilogy. In part Ⅱ, we will see how to apply the extension and trace operators introduced above.
In part Ⅲ we will discuss general quantifiable properties of the geometry that are eventually accessible also to computer algorithms that will allow to predict homogenization behavior of random geometries.
We first collect some notation and mathematical concepts which will be frequently used throughout this paper. We first start with the standard geometric objects, which will be labeled by bold letters.
Throughout this work, we use (ei)i=1,…d for the Euclidean basis of Rd. By C>0 we denote any constant that depends on p and d but no further dependencies unless explicitly mentioned. Such mentioning may be expressed in some cases through the notation C(a,b,…). Once the dependencies are made clear we may sometimes drop them for shortness of presentation. Furthermore, we use the following notation.
Unit cube The torus T=[0,1)d is equipped with the topology of the metric d(x,y)=minz∈Zd|x−y+z|. In contrast, the open interval Id:=(0,1)d is considered as a subset of Rd. We often omit the index d if this does not provoke confusion.
Balls Given a metric space (M,d) we denote Br(x) the open ball around x∈M with radius r>0. The surface of the unit ball in Rd is Sd−1. Furthermore, we denote for every A⊂Rd by Br(A):=⋃x∈ABr(x).
Points A sequence of points will be labeled by X:=(xi)i∈N.
A cone in Rd is usually labeled by C. In particular, we define for a vector ν of unit length, 0<α<π2 and R>0 the cone
Cν,α,R(x):={z∈BR(x):z⋅ν>|z|cosα}andCν,α(x):=Cν,α,∞(x). | (2.1) |
Inner and outer hull We use balls of radius r>0 to define for a closed set P⊂Rd the sets
Pr:=¯Br(P):={x∈Rd:dist(x,P)≤r},P−r:=Rd∖[Br(Rd∖P)]:={x∈Rd:dist(x,Rd∖P)≥r}. | (2.2) |
One can consider these sets as inner and outer hulls of P. The last definition resembles a concept of "negative distance" of x∈P to ∂P and "positive distance" of x∉P to ∂P. For A⊂Rd we denote conv(A) the closed convex hull of A.
The natural geometric measures we use in this work are the Lebesgue measure on Rd, written |A| for A⊂Rd, and the k-dimensional Hausdorff measure, denoted by Hk on k-dimensional sub-manifolds of Rd (for k≤d).
In the following, we formulate some extension and trace results. Although it is well known how such results are proved and the proofs are standard, we include them for completeness since we are interested in the dependence of the operator norm on the local Lipschitz regularity of the boundary.
The following is well known:
Lemma 2.1. For every 1≤p≤∞ there exists Cp>0 such that for every R>0 there exists an extension operator U:W1,p(BR(0))→W1,p(B2R(0)) such that
‖∇Uu‖Lp(B2R(0))≤Cp‖∇u‖Lp(BR(0)). |
Let P⊂Rd be an open set and let p∈∂P and δ>0 be a constant such that Bδ(p)∩∂P is graph of a Lipschitz function. We denote
M(p,δ):=inf{M:∃ϕ:U⊂Rd−1→R :=infϕLipschitz, with constant M s.t. Bδ(p)∩∂P is graph of ϕ}. | (2.3) |
Remark 2.2. For every p, the function M(p,⋅) is monotone increasing in δ.
Lemma 2.3 (Uniform Extension for Balls). Let P⊂Rd be an open set, 0∈∂P and assume there exists δ>0, M>0 and an open domain U⊂Bδ(0)⊂Rd−1 such that ∂P∩Bδ(0) is graph of a Lipschitz function φ:U⊂Rd−1→Rd of the form φ(˜x)=(˜x,ϕ(˜x)) in Bδ(0) with Lipschitz constant M and φ(0)=0. Writing x=(˜x,xd) and defining ρ=δ√4M2+2−1 there exist an extension operator
(Uu)(x)={u(x)ifxd<ϕ(˜x)u(˜x,−xd+2ϕ(˜x))ifxd>ϕ(˜x), | (2.4) |
such that for
A(0,P,ρ):={(˜x,−xd+2ϕ(˜x)):(˜x,xd)∈Bρ(0)∖P}⊂Bδ(0), | (2.5) |
and for every p∈[1,∞] the operator
U:W1,p(A(0,P,ρ))→W1,p(Bρ(0)), |
is continuous with
‖Uu‖Lp(Bρ(0)∖P)≤‖u‖Lp(A(0,P,ρ)),‖∇Uu‖Lp(Bρ(0)∖P)≤2M‖∇u‖Lp(A(0,P,ρ)). | (2.6) |
Remark 2.4. In case ϕ(˜x)≥0 we find A(0,P,ρ)⊂Bρ(0).
Proof of Lemma 2.3. In case ϕ(˜x)≡0 we consider the extension operator U+:W1,p(Rd−1×(−∞,0))→W1,p(Rd) having the form (compare also [6, chapter 5], [1])
(U+u)(x)={u(x) if xd<0u(˜x,−xd) if xd>0. |
The general case follows from transformation.
Lemma 2.5. Let P⊂Rd be an open set, 0∈∂P and assume there exists δ>0, M>0 and an open domain U⊂Bδ(0)⊂Rd−1 such that ∂P∩Bδ(0) is graph of a Lipschitz function φ:U⊂Rd−1→Rd of the form φ(˜x)=(˜x,ϕ(˜x)) in Bδ(0) with Lipschitz constant M and φ(0)=0 and define ρ=δ√4M2+2−1. Writing x=(˜x,xd) we consider the trace operator T:C1(P∩Bδ(0))→C(∂P∩Bρ(0)). For every p∈[1,∞] and every r≥1 with 1−dp>1−dr the operator T can be continuously extended to
T:W1,p(P∩Bδ(0))→Lr(∂P∩Bρ(0)), |
such that
‖Tu‖Lr(∂P∩Bρ(0))≤Cr,pρd(p−r)rp−1r√4M2+21r+1‖u‖W1,p(P∩Bδ(0)). | (2.7) |
Proof. We proceed similar to the proof of Lemma 2.3.
Step 1: Writing Br=Br(0) together with B−r={x∈Br:xd<0} and Σr:={x∈Br:xd=0} we recall the standard estimate
(∫Σ1|u|r)1r≤Cr,p((∫B−1|∇u|p)1p+(∫B−1|u|p)1p), |
which leads to
(∫Σρ|u|r)1r≤Cr,pρd(p−r)rp−1r(ρ(∫B−ρ|∇u|p)1p+(∫B−ρ|u|p)1p). |
Step 2: Using the transformation rule and the fact that 1≤|detDφ|≤√4M2+2 we infer Eq (2.7) in the following way:
(∫∂P∩Bρ(0)|u|r)1r≤√4M2+21r(∫Σρ|u∘φ|r)1r≤Cr,pρd(p−r)rp−1r√4M2+21r(ρ(∫B−ρ|∇(u∘φ)|p)1p+(∫B−ρ|u∘φ|p)1p)≤Cr,pρd(p−r)rp−1r√4M2+21r+1⋅⋅(ρ(∫B−ρ|(∇u)∘φ|pdetDφ)1p+(∫B−ρ|u∘φ|pdetDφ)1p) |
and from this we conclude the Lemma with φ−1(B−ρ)⊂Bδ(0).
We recall that we will use bold letters for Rd-valued function spaces. In particular, we introduce for 1≤p≤∞
Lp(Q):=Lp(u;Rd),W1,p(Q):={u∈Lp(Q):∇u∈Lp(Q;Rd×d)}. |
From [5] we know that on general Lipschitz domains an estimate like the following holds:
Lemma 2.6. For every 1≤p≤∞ there exists a constant C>0 depending only on the dimension d≥2 such that the following holds: For every radius R>0 there exists an extension operator UR:W1,p(BR(0))→W1,p(B2R(0)) such that
‖∇s(URu)‖W1,p(B2R(0))≤CN‖∇su‖W1,p(BR(0)). |
Again, we will need a refined estimate on extensions on Lipschitz domains which explicitly accounts for the local Lipschitz constant.
Lemma 2.7 (Uniform Nitsche-Extension for Balls). For every d≥2 there exists a constant CN depending only on the dimension d such that the following holds: Let P⊂Rd be an open set, 0∈∂P and assume there exists δ>0, M>0 and an open domain U⊂Bδ(0)⊂Rd−1 such that ∂P∩Bδ(0) is graph of a Lipschitz function φ:U⊂Rd−1→Rd of the form φ(˜x)=(˜x,ϕ(˜x)) in Bδ(0) with Lipschitz constant M and φ(0)=0. Writing x=(˜x,xd) we define ρ=δ√4M2+2−1 and
A(0,P,ρ):={(˜x,xd)∈P:|˜x|<ρ,xd≤CN(1+M2)}. | (2.8) |
Then for every p∈[1,∞] there exists a continuous operator
U:W1,p(A(0,P,ρ))→W1,p(Bρ(0)), |
such that for some constant C independent from (δ,M) and P it holds
‖∇sUu‖Lp(Bρ(0)∖P)≤C(1+M)2‖∇su‖Lp(A(0,P,ρ)). | (2.9) |
Remark 2.8. In case ϕ(˜x)≥0 the proof reveals A(0,P,ρ)⊂Bcρ(0) for some c depending only on the dimension d.
In order to prove such a result we need the following lemma.
Lemma 2.9 ([26] Chapter 6 Section 1 Theorem 2). There exist constants c1,c2,c3>0 such that for every open set P⊂Rd with local Lipschitz boundary there exists a function dP:Rd∖¯P∁→R with
c1dP(x)≤dist(x,P)≤c2dP(x),∀i∈{1,…,d}|∂idP(x)|≤c3,∀i,k∈{1,…,d}|∂i∂kdP(x)|≤c3|dP(x)|−1. |
From the theory presented by Stein [26] we will not get an explicit form of CN but only an upper bound that grows exponentially with dimension d.
Proof of Lemma 2.7. We use an idea by Nitsche [20], which we transfer from p=2 to the general case, thereby explicitly quantifying the influence of M. For simplicity we write Pδ:=P∩Bδ(0) and P∁δ:=Bδ(0)∖P and assume that x∈Pδ iff x∈Bδ(0) and xd<ϕ(˜x).
As observed by Nitsche, it holds
∀x∈P∁δ:0<(1+M2)−12(xd−ϕ(˜x))≤dist(x,∂P)≤xd−ϕ(˜x), |
and together with Lemma 2.9, we can define dP,M(x):=2c2(1+M2)12dP(x) and find for c>max{2c2c1,4c2c3} that
2(xd−ϕ(˜x))≤dP,M(x)≤c(1+M2)12(xd−ϕ(˜x)),∀i∈{1,…,d}:|∂idP,M(x)|≤c(1+M2)12,∀i,k∈{1,…,d}:|∂i∂kdP,M(x)|≤c(1+M2)|dP,M(x)|−1. |
Using ψ∈C([1,2]) satisfying
∫21ψ(t)dt=1,∫21tψ(t)dt=0, | (2.10) |
Nitsche introduced xλ:=(˜x,xd−λdP,M(x)) and proposed the following extension on x∈P∁δ:
ui(x):=∫21ψ(λ)(ui(xλ)+λud(xλ)∂idP,M(x))dλ. |
One can quickly verify that this maps C(¯Pδ) onto C(¯Bρ(0)). In what follows, we write ε[u](x):=∇su(x) and particularly εij[u](x):=12(∂iuj+∂jui) as well as ελij[u](x)=εij[u](xλ) for x∈P∁δ. Then for x∈P∁δ∩Bρ(0)
εij[u](x)=∫21ψ(λ)(ελij(x)+λ∂idP,M(x)ελjd(x)+λ∂jdP,M(x)ελid(x) | (2.11) |
+λ2∂idP,M(x)∂jdP,M(x)ελdd(x)+λ∂i∂jdP,M(x)ud(xλ)) | (2.12) |
From the fundamental theorem of calculus we find
ud(xλ)=ud(x1)+δ(˜x)∫λ1∂dud(xt)dt, |
which leads by Eq (2.10) to
∫21ψ(λ)λ∂i∂jdP,M(x)ud(xλ)dλ=∂i∂jdP,M(x)dP,M(x)∫21εdd[u](xt)dt∫2μψ(λ)λdλ. |
We may now apply |⋅|p on both sides of Eq (2.11), integrate over P∁δ∩Bρ(0) and use the integral transformation theorem for each λ to find
‖ε[u]‖Lp(P∁δ∩Bρ(0))≤C(1+M2)‖ε[u]‖Lp(Pδ). |
We denote for bounded open A⊂Rd
W1,p(0),r(A):={u∈W1,p(A):∃x:Br(x)⊂A∨−∫Br(x)u=0}. |
Note that this is not a linear vector space.
Lemma 2.10. For every p∈[1,∞) there exists Cp>0 such that the following holds: Let 0<r<R and x∈BR(0) such that Br(x)⊂BR(0) then for every u∈W1,p(BR(0))
‖u‖pLp(BR(0))≤Cp(RpRd−1rd−1‖∇u‖pLp(BR(0))+Rdrd‖u‖pLp(Br(x))), | (2.13) |
and for every u∈W1,p(0),r((BR(0)) it holds
‖u‖pLp(BR(0))≤CpRp(rR)1−d(1+(rR)p−1)‖∇u‖pLp(BR(0)). | (2.14) |
Remark 2.11 In case p≥d we find that (2.14) holds iff u(x)=0 for some x∈B1(0).
Proof. In a first step, we assume x=0 and R=1. The underlying idea of the proof is to compare every u(y), y∈B1(0)∖Br(0) with u(ry). In particular, we obtain for y∈B1(0)∖Br(0) that
u(y)=u(ry)+∫10∇u(ry+t(1−r)y)⋅(1−r)ydt |
and hence by Jensen's inequality
|u(y)|p≤C(∫10|∇u(ry+t(1−r)y)|p(1−r)p|y|pdt+|u(ry)|p). |
We integrate the last expression over B1(0)∖Br(0) and find
∫B1(0)∖Br(0)|u(y)|pdy≤∫B1(0)∖Br(0)|u(ry)|pdy+∫Sd−1∫1rC(∫10|∇u(rsν+t(1−r)sν)|p(1−r)pspdt)sd−1dsdν≤∫Sd−1∫1rC(∫srs|∇u(tν)|p(1−r)p−1sp−1dt)sd−1ds+∫B1(0)∖Br(0)|u(ry)|pdy≤C∫1rdssd−11(rs)d−1∫srsdttd−1∫Sd−1|∇u(tν)|p(1−r)p−1sp−1+∫B1(0)∖Br(0)|u(ry)|pdy≤C1rd−1‖∇u‖pLp(B1(0))+1rd‖u‖pLp(Br(0)). |
For general x∈B1(0), use the extension operator U:W1,p(B1(0))→W1,p(B4(0)) such that ‖Uu‖W1,p(B4(0))≤C‖u‖W1,p(B1(0)) and
‖∇Uu‖W1,p(B4(0))≤C‖∇u‖W1,p(B1(0)). |
Since B1(0)⊂B2(x)⊂B4(0) we infer
‖u‖pLp(B1(0))≤‖Uu‖pLp(B2(x))≤C(1rd−1‖∇Uu‖pLp(B2(x))+1rd‖Uu‖pLp(Br(x))). |
and hence Eq (2.13). Furthermore, since there holds ‖u‖pLp(B1(0))≤C‖∇u‖pLp(B1(0)) for every u∈W1,p(0)(B1(0)), a scaling argument shows ‖u‖pLp(Br(0))≤Crp‖∇u‖pLp(Br(0)) for every u∈W1,p(0),r(B1(0)) and hence Eq (2.14). For general R>0 use a scaling argument.
A similar argument leads to the following, where we remark that the difference in the appearing of 1r is due to the fact, that integrating the cylinder needs no surface element rd−1.
Corollary 2.12. For every p∈[1,∞) and r>0 there exists Cp>0 such that the following holds: Let r<L, PL,r:=B#1#2(0)×(0,L) and x∈PL,r such that Br(x)⊂PL,r then for every u∈W1,p(PL,r)
‖u‖pLp(PL,r)≤Cp(Lp‖∇u‖pLp(PL,r)+Lr‖u‖pLp(Br(x))), | (2.15) |
and if additionally −∫Br(x)u=0 then
‖u‖pLp(PL,r)≤Cp(Lp‖∇u‖pLp(PL,r)+Lrp−1‖∇u‖pLp(Br(x))), | (2.16) |
Let y∈PL,r such that Br(y)⊂PL,r then for every u∈W1,p(PL,r)
|−∫Br(y)u−−∫Br(x)u|p≤Cp(Lp−1r1−d‖∇u‖pLp(PL,r)). | (2.17) |
We introduce on open sets A⊂Rd the Sobolev space
W1,p∇⊥(0)(A):={u∈W1,p(A):∀i,j:∫A∂iuj−∂jui=0}. |
To the authors best knowledge, the following is the most general Korn inequality in literature and it is formulated for John domains.
Theorem 2.13 ([5] Theorem 2.7 and Corollary 2.8). Let A be a John domain with constants ε,δ. Let 1≤p≤∞ and ˜δ>0 such that δ/diamA≥˜δ. Then there exists a constant Cp>0 depending only on d, p, ε and ˜δ but not on A such that it holds
∀u∈W1,p∇⊥(0)(A):‖∇u‖Lp(A)≤Cp‖∇su‖Lp(A). | (2.18) |
Remark 2.14. In the original work the claimed dependence of Cp was on d, p, ε, δ and A with the observation that Eq (2.18) is invariant under scaling of A. However, this scale invariance results in the dependence on d, p, ε and δ/diamA since ε, p and d are not sensitive to scaling of A.
Corollary 2.15. For every 1≤p≤∞ there exists Cp depending only on d and p such that for every bounded open convex set A⊂Rd the estimate (2.18) holds.
We furthermore introduce the set
W1,p∇⊥(0),r(A):={u∈W1,p(A):∃x:Br(x)⊂A∨∀i,j∫Br(x)∂iuj−∂jui=0} |
which is not a vector space.
Lemma 2.16 (Mixed Korn inequality). Let 1≤p≤∞ and ε,δ∈(0,1). Then there exists a constant ˜Cp>0 depending only on d, p, ε and δ such that for every (ε,δ)-John domain A⊂B1(0), for every r∈(0,1) and every x∈A with Br(x)⊂A it holds
∀u∈W1,p(A):‖∇u‖Lp(A)≤˜Cp(|A|rd)1p(‖∇su‖Lp(A)+‖∇u‖Lp(Br(x))). | (2.19) |
Furthermore,
∀u∈W1,p∇⊥(0),r(A):‖∇u‖Lp(A)≤2˜Cp(|A||Sd−1|rd)1p(‖∇su‖Lp(A)). | (2.20) |
The difference to Theorem 2.13 is that ∫Br(x)∂iuj−∂jui=0 only holds on a subset of A. Unfortunately, we do not have a reference for a comparable Lemma in the literature except for [25] in case p=2. The author strongly supposes a proof must exist somewhere, however, we provide it for completeness.
Proof. Let Cp be the constant from Theorem 2.13 for domains with a diameter less than 2 and suppose Eq (2.19) was wrong. Then there exists a sequence of (ε,δ)-John domains An⊂B1(0) with xn∈An, rn∈(0,1) with Brn(xn)⊂An and functions un∈W1,p(An) such that
1=‖∇un‖Lp(An)≥Cp(|An||Sd−1|rdn)1pn(‖∇sun‖Lp(An)+‖∇un‖Lp(Brn(xn))). |
We define ¯∇⊥n(un):=−∫An(∇un−∇sun) and un,⊥(x):=un(x)−¯∇⊥n(un)x with ∇sun,⊥=∇sun. Hence by Eq (2.18)
‖∇un−¯∇⊥n(un)‖Lp(An)≤Cp‖∇sun,⊥‖Lp(An)=Cp‖∇sun‖Lp(An). |
We directly infer with Cn:=|An||Sd−1|rdn
CpC1pn(‖∇sun‖Lp(An)+‖∇un‖Lp(Brn(xn)))+C1pn‖∇un−¯∇⊥n(un)‖Lp(An)→0. | (2.21) |
Furthermore, we find
1=‖∇un‖Lp(An)≥‖¯∇⊥n(un)‖Lp(An)−‖∇un−¯∇⊥n(un)‖Lp(An),and‖¯∇⊥n(un)‖Lp(An)≥‖∇un‖Lp(An)−‖∇un−¯∇⊥n(un)‖Lp(An), |
and hence ‖¯∇⊥n(un)‖Lp(An)→1 due to Eq (2.21). Since ¯∇⊥n(un) are constant, it holds
Cn‖¯∇⊥n(un)‖pLp(Brn(xn))=‖¯∇⊥n(un)‖pLp(An) |
and we infer from a similar calculation
C1pn(‖∇un‖Lp(Brn(xn))+‖∇un−¯∇⊥n(un)‖Lp(Brn(xn)))≥C1pn‖¯∇⊥n(un)‖Lp(Brn(xn))≥‖¯∇⊥n(un)‖Lp(An). |
This implies ‖¯∇⊥n(un)‖Lp(An)→0 by Eq (2.21), a contradiction. Hence, Eq (2.19) holds with ˜Cp=nCp for some n∈N.
Estimate Eq (2.20) now follows from Eqs (2.19) and (2.18) (the latter applied to A=Br(x) and the definition of W1,p∇⊥(0),r(BR(0)).
Generalizing the above Korn inequality to a Korn-Poincaré inequality, we define
W1,p(0),∇⊥(0),r(BR(0)):={u∈W1,p(Br(0)):∃x:Br(x)⊂BR(0)∨:=W1,p∫Br(x):={u}∫Br(x)ui=0∨∀i,j:∫Br(x)∂iuj−∂jui=0}. |
Lemma 2.17 (Mixed Korn-Poincaré inequality on balls). For every p∈[1,∞) there exists Cp>0 such that for every R>0, r∈(0,R), every x∈BR(0) with Br(x)⊂BR(0) and every u∈W1,p(0),∇⊥(0),r(BR(0)) it holds
‖∇u‖pLp(BR(0))≤Cp(Rr)d‖∇su‖pLp(BR(0)), | (2.22) |
‖u‖pLp(BR(0))≤Cp(Rr)2d−1(1+(Rr)1−p)Rp‖∇su‖pLp(BR(0)). | (2.23) |
Proof. Apply Eq (2.20) in Lemma 2.16 for R=1 and use a simple scaling argument to obtain
‖∇u‖Lp(BR(0))≤Cp(Rr)d(‖∇su‖Lp(Br(0))). |
Afterwards apply Lemma 2.10.
Lemma 2.18 (Mixed Korn-Poincaré inequality on cylinders). For every p∈[1,∞) and r>0 there exists Cp>0 such that the following holds: Let r<L, PL,r:=(0,L)×B#1#2(0) and x∈PL,r such that Br(x)⊂PL,r then for every u∈W1,p(PL,r)
‖∇u‖pLp(PL,r)≤Cp((Lr)p‖∇su‖pLp(PL,r)+Lr‖∇u‖pLp(Br(x))). | (2.24) |
Furthermore,
‖u‖pLp(PL,r)≤Cp(L2prp‖∇su‖pLp(PL,r)+Lp+1r‖∇u‖pLp(Br(x))+Lr‖u‖pLp(Br(x))), | (2.25) |
and if additionally u∈W1,p(0),∇⊥(0),r(PL,r) then
‖∇u‖pLp(PL,r)≤CpLprp‖∇su‖pLp(PL,r),‖u‖pLp(PL,r)≤CpL2prp‖∇su‖pLp(PL,r), | (2.26) |
Defining ¯∇⊥a,δu:=−∫Bδ(a)(∇u−∇su) and
[Ms,δau](x):=¯∇⊥a,δu(x−a)+−∫Bδ(a)u | (2.27) |
we find for a,b with Bδ(a),Bδ(b)⊂PL,r for every u∈W1,p(PL,r) that
|[Ms,δau](x)−[Ms,δbu](x)|p≤C|x−a|p|a−b|2pδp+d(∫conv(Bδ(a)∪Bδ(b))|∇su|p). | (2.28) |
Furthermore, for every δ<r we find
|[Ms,rau](x)−[Ms,δau](x)|p ≤C((δr)−d|x−a|p+(δr)1−d(1+(δr)p−d))rp−d‖∇su‖pLp(Br(a)). | (2.29) |
Proof. Step1: We can assume L∈N, a=12e1, b=(L−12)e1, r=12 and define
Pk:=(ke1+[0,1)×B#1#2(0)),Bk:=ke1+B12(12e1)τsku(x):=[Ms,12(k+12)e1u](x)=[−∫Bk(∇u−∇su)]x+−∫Bku. | (2.30) |
Then we find by Lemma 2.16 for some C>0 independent from u or K
‖∇u‖pLp(PK)≤C(‖∇(u−τsKu)‖pLp(PK)+‖∇τsKu‖pLp(PK))≤C(‖∇su‖pLp(PK)+‖∇τsKu‖pLp(PK)). |
Since ∇τsku are constant functions for every k, we find
‖∇τsKu‖pLp(PK)≤C‖∇τs0u‖pLp(P0)+C(K−1∑k=0‖∇(τsk+1u−τsku)‖L1(Pk+1))p. |
Furthermore, we find
τsk(u−τsk+1u)=−∫Bk(∇u−−∫Bk+1(∇u−∇su)−∇su)x+−∫Bk(u−−∫Bk+1u)=τsku−τsk+1u=τsk+1(u−τsku). |
This implies by ∇τsk+1(u−τsku)=−∫Bk+1(∇−∇s)(u−τsku) as a consequence of Eq (2.30) and Lemma 2.16 and Theorem 2.13
‖∇(τsk+1u−τsku)‖pLp(Pk+1)≤C‖∇τsk+1(u−τsku)‖pLp(Bk+1)≤C‖∇(u−τsku)‖pLp(Bk+1)2.16≤C(‖∇s(u−τsku)‖pLp(Pk+1∪Pk)+‖∇(u−τsku)‖pLp(Bk))2.13≤C‖∇su‖pLp(Pk+1∪Pk). |
Since the last inequality implies
(K−1∑k=0‖∇(τsk+1u−τsku)‖L1(Pk+1))p≤Kp−1C‖∇su‖pLp((0,K)×B#1#2(0)) |
and since ‖∇τs0u‖pLp(P0)≤C(‖∇su‖pLp(P0)+‖∇u‖pLp(B0)) by Lemma 2.16 we find in total
‖∇τsKu‖pLp(PK)≤C‖∇u‖pLp(B0)+CKp−1‖∇su‖pLp((0,K)×B#1#2(0)). |
Adding the last inequality from K=0 to K=L implies Eq (2.24) through scaling. Applying Corollary 2.12 we infer that Eqs (2.25) and (2.26).
Step 2: We observe that Step 1 also holds for PL,r being replaced by conv(Bδ(a)∪Bδ(b)). Writing ub:=u−Ms,δbu we find from the above calculations
|Ms,δau−Ms,δbu|p(x)=|Ms,δa(u−Ms,δbu)|p(x)≤C1δd(|x−a|p∫Bδ(a)|∇ub−∇sub|p+∫Bδ(a)|ub|p). |
Using that ub∈W1,p(0),∇⊥(0),r(conv(Bδ(a)∪Bδ(b))), we find Eq (2.28) with help of Eq (2.26) and Lemma 2.17.
Step 3: We assume a=0 for notational simplicity. Writing ˉu(y):=u(y)−(¯∇⊥a,δu)y with −∫Br(0)u=−∫Br(0)¯u we infer Eq (2.29) from Lemmas 2.16 and 2.10 via
|[Ms,10u](x)−[Ms,δ0u](x)|p≤C|∫B1(0)∇u−∇su−¯∇⊥a,δu|p|x|p+|−∫B1(0)¯u−−∫Bδ(0)¯u|p≤C∫B1(0)(|∇u−¯∇⊥a,δu|p+|∇su|p)|x|p+−∫B1(0)|¯u−−∫Bδ(0)¯u|p≤C(δ−d|x|p‖∇su‖pLp(B1(0))+δ1−d(1+δp−d)‖∇¯u‖pLp(B1(0))). |
Definition 2.19 (Voronoi Tessellation). Let X=(xi)i∈N be a sequence of points in Rd with xi≠xk if i≠k. For each x∈X let
G(x):={y∈Rd:∀˜x∈X∖{x}|x−y|<|˜x−y|}. |
Then (G(xi))i∈N is called the Voronoi tessellation of Rd with respect to X. For each x∈X we define d(x):=diamG(x) the diameter of G(x).
We will need the following result on Voronoi tessellation of a minimal diameter.
Lemma 2.20. Let r>0 and let X=(xi)i∈N be a sequence of points in Rd with |xi−xk|>2r if i≠k. For x∈X let I(x):={y∈X:G(y)∩Br(G(x))≠∅}. Then y∈I(x) implies |x−y|≤4d(x) and
#I(x)≤(4d(x)r)d. | (2.31) |
Proof. Let Xk={xj∈X:Hd−1(∂Gk∩∂Gj)≥0} the neighbors of xk and dk:=d(xk). Then all xj∈X satisfy |xk−xj|≤2dk. Moreover, every ˜x∈X with |˜x−xk|>4dk has the property that dist(∂G(˜x),xk)>2dk>dk+r and ˜x∉Ik. Since every Voronoi cell contains a ball of radius r, this implies that #Ik≤|B4dk(xk)|/|Br(0)|=(4dkr)d.
Definition 2.21 (Delaunay Triangulation). Let X=(xi)i∈N be a sequence of points in Rd with xi≠xk if i≠k. The Delaunay triangulation is the dual unoriented graph of the Voronoi tessellation, i.e. we say D(X):={(x,y):Hd−1(∂G(x)∩∂G(y))≠0}.
Definition 2.22 (η- regularity). For a function η:∂P→(0,r] we call P η-regular if
∀p∈∂P,ε∈(0,12),˜p∈Bεη(p)(p)∩∂P it holds η(˜p)>(1−ε)η(p). | (2.32) |
Remark 2.23. This concept and its consequences from Lemma 2.24 and Theorem 2.25 will be extensively used later to cover ∂P by a suitable family of open balls.
Lemma 2.24. Let P be a locally η-regular set for η:∂P→(0,r). Then η:P→R is locally Lipschitz continuous with Lipschitz constant 1 and for every ε∈(0,12) and ˜p∈Bεη(p)∩P it holds
1−ε1−2εη(p)>η(˜p)>η(p)−|p−˜p|>(1−ε)η(p). | (2.33) |
Furthermore,
|p−˜p|≤εmax{η(p),η(˜p)}⇒|p−˜p|≤ε1−εmin{η(p),η(˜p)} | (2.34) |
Proof. Let p,˜p such that |˜p−p|<12η(p) with εp,˜p:=inf{ε:|˜p−p|<εη(p)}. This means ε∈[εp,˜p,12) iff η(˜p)≥(1−ε)η(p) and we find
η(˜p)≥η(p)−|p−˜p|=η(p)−εp,˜pη(p)>(1−ε)η(p) | (2.35) |
which implies |˜p−p|<ε1−εη(˜p) and the local Lipschitz continuity by a symmetry argument in p, ˜p. Also this leads to |p−˜p|≥η(p)−η(˜p) and η(p)>(1−ε1−ε)η(˜p) or
η(p)=1−ε1−εη(p)<11−ε(η(p)−|p−˜p|)<11−εη(˜p)≤11−2εη(p), |
implying Eq (2.33).
In order to prove Eq (2.34), assume η(˜p)≤η(p). Then Eq (2.35)
|p−˜p|≤εη(p)≤ε1−εη(˜p). |
Theorem 2.25. Let Γ⊂Rd be a closed set and let η(⋅)∈C(Γ) be bounded and satisfy for every ε∈(0,12) and for |p−˜p|<εη(p)
1−ε1−2εη(p)>η(˜p)>η(p)−|p−˜p|>(1−ε)η(p). | (2.36) |
and define ˜η(p)=2−Kη(p), K≥2. Then for every C∈(0,1) there exists a locally finite covering of Γ with balls B˜η(pk)(pk) for a countable number of points (pk)k∈N⊂Γ such that for every i≠k with B˜η(pi)(pi)∩B˜η(pk)(pk)≠∅ it holds
2K−1−12K−1˜η(pi)≤˜η(pk)≤2K−12K−1−1˜η(pi)and2K−12K−1−1min{˜η(pi),˜η(pk)}≥|pi−pk|≥Cmax{˜η(pi),˜η(pk)} | (2.37) |
Remark 2.26. The fact that Eq (2.37) can be satisfied for any given C∈(0,1) (even having in mind that the choice of points depends on C) is surprising. In fact, η(p)−|p−˜p|>(1−ε)η(p) in Eq (2.36) seems to contradict (2.37). However, we have to keep in mind that Eq (2.37) holds for ˜η=2−Kη, K≥2. Now suppose |p−˜p|=2−Kη(p) and η(p)>η(˜p). Since Eq (2.36) holds for every ε∈(0,12) we find for ε=2−K that η(˜p)>(1−2−K)η(p) and hence
2K−12K−1−1˜η(˜p)≥|p−˜p|=˜η(p). |
So the above calculation shows that the lemma to hold for every C<1 is plausible. The major difficulty in the original proof is to provide an algorithm which provides the covering as claimed.
Proof. We chose δ>0, n∈N such that (1−1n)(1−δ)>C. Multiplying η and Γ by a factor C>0 we can assume ˜η<(1−δ). Consider ˜Q:=[0,1n]d, let q1,…,nd denote the nd elements of [0,1)d∩Qdn and let ˜Qz,i=˜Q+z+qi, z∈Zd. We set B(0):=∅, Γ1=Γ, ηk:=(1−δ)k and for k≥1 we construct the covering using inductively defined open sets B(k) and closed set Γk as follows:
1. Define Γk,1=Γk. For i=1,…,nd do the following:
(a) For every z∈Zd do
if ∃p∈(ηk˜Qz,i)∩Γk,i,˜η(p)∈(ηk,ηk−1]then set bz,i=B˜η(p)(p),Xz,i={p}otherwise set bz,i=∅,Xz,i=∅. |
(b) B(k),i:=⋃z∈Zdbz,i and Γk,i+1=Γk,i∖B(k),i and X(k),i:=⋃z∈ZdXz,i.
Observe: p1,p2∈X(k),i implies |p1−p2|>(1−1n)ηk and p3∈X(k),j, j<i implies p1∉Bηk(p3) and hence |p1−p3|>ηk. Similar, p3∈Xl, l<k, implies |p1−p3|>ηl>ηk.
2. Define Γk+1:=Γk,nd+1, Xk:=⋃iX(k),i.
The above covering of Γ is complete in the sense that every x∈Γ lies in one of the balls (by contradiction). We denote X:=⋃kXk=(pi)i∈N the family of centers of the above constructed covering of Γ and find the following properties: Let p1,p2∈X be such that B˜η(p1)(p1)∩B˜η(p2)(p2)≠∅. Assuming ˜η(p1)≥˜η(p2) the following two properties are satisfied due to Eq (2.36)
1. It holds |p1−p2|≤2˜η(p1)≤12K−1η(p1) and hence B˜η(p2)(p2)⊂B22−Kη(p1)(p1) and η(p2)≥2K−1−12K−1η(p1). Furthermore ˜η(p1)≥˜η(p2)≥2K−1−12K−1˜η(p1).
2. Let k such that ˜η(p1)∈(ηk,ηk+1]. If also ˜η(p2)∈(ηk,ηk+1] then the observation in Step 1(b) implies
|p1−p2|≥(1−1n)ηk≥(1−1n)(1−δ)˜η(p1). |
If ˜η(p2)∉[ηk,ηk+1) then ˜η(p2)<ηk and hence p2∉B˜η(p1)(p1), implying |p1−p2|>˜η(p1).
Due to our choice of n and δ, this concludes the proof.
The concept of stationarity provides us automatically with a law of large numbers (in space) when we average properties of random geometries over an ever growing large domain in space. The concept of ergodicity ensures that this law of large numbers in space holds independently from a given realization and thus it is equivalent with the law of large numbers for averaging over many samples in a given small region instead.
Assumption 2.27. Throughout this work we assume that (Ω,F,P) is a probability space with countably generated σ-algebra F.
Due to the insight in [10], shortly sketched in the next two subsections, after a measurable transformation the probability space Ω can be assumed to be metric and separable, which always ensures Assumption 2.27.
Definition 2.28 (Dynamical system). A dynamical system on Ω is a family (τx)x∈Rd of measurable bijective mappings τx:Ω↦Ω satisfying (ⅰ)–(ⅲ):
(i) τx∘τy=τx+y, τ0=id (Group property)
(ii) P(τ−xB)=P(B)∀x∈Rd,B∈F (Measure preserving)
(iii) A:Rd×Ω→Ω(x,ω)↦τxω is measurable (Measurability of evaluation)
A⊂Ω is almost invariant if for every x∈Rd holds P((A∪τxA)∖(A∩τxA))=0. The family
I={A∈F:∀x∈RdP((A∪τxA)∖(A∩τxA))=0} | (2.38) |
of almost invariant sets is a σ-algebra and
E(f|I)denotes the expectation of f:Ω→R w.r.t. I. | (2.39) |
A concept linked to dynamical systems is the concept of stationarity.
Definition 2.29 (Stationary). Let X be a measurable space and let f:Ω×Rd→X. Then f is called (weakly) stationary if f(ω,x)=f(τxω,0) for (almost) every x.
Definition 2.30. A family (An)n∈N⊂Rd is called convex averaging sequence if
(i) each An is convex
(ii) for every n∈N holds An⊂An+1
(iii) there exists a sequence rn with rn→∞ as n→∞ such that Brn(0)⊆An.
We sometimes may take the following stronger assumption.
Definition 2.31. A convex averaging sequence An is called regular if
|An|−1#{z∈Zd:(z+T)∩∂An≠∅}→0. |
The latter condition is evidently fulfilled for sequences of cones or balls. Convex averaging sequences are important in the context of ergodic theorems.
Theorem 2.32 (Ergodic Theorem [4] Theorems 10.2.Ⅱ and also [27]).
Let (An)n∈N⊂Rd be a convex averaging sequence, let (τx)x∈Rd be a dynamical system on Ω with invariant σ-algebra I and let f:Ω→R be measurable with |E(f)|<∞. Then for almost all ω∈Ω
|An|−1∫Anf(τxω)dx→E(f|I). | (2.40) |
For the calculations in this work, we will particularly focus on the case of trivial I. This is called ergodicity, as we will explain in the following.
Definition 2.33 (Ergodicity and mixing). A dynamical system (τx)x∈Rd on a probability space (Ω,F,P) is called mixing if for every measurable A,B⊂Ω it holds
lim‖x‖→∞P(A∩τxB)=P(A)P(B). | (2.41) |
A dynamical system is called ergodic if
limn→∞1(2n)d∫[−n,n]dP(A∩τxB)dx=P(A)P(B). | (2.42) |
Remark 2.34. a) Let Ω={ω0=0} with the trivial σ-algebra and τxω0=ω0. Then τ is evidently mixing. However, the realizations are constant functions fω(x)=c on Rd for some constant c.
b) A typical ergodic system is given by Ω=T=[0,1]d with the Lebesgue σ-algebra and P=Ld the Lebesgue measure in Rd. The dynamical system is given by .
c) It is known that is ergodic if and only if every almost invariant set has probability (see [4] Proposition 10.3.Ⅲ) i.e.,
(2.43) |
d) It is sufficient to show Eq (2.41) or (2.42) for and in a ring that generates the -algebra . We refer to [4], Section 10.2.
A further useful property of ergodic dynamical systems, which we will use below, is the following:
Lemma 2.35 (Ergodic times mixing is ergodic). Let and be probability spaces with dynamical systems and respectively. Let be the usual product measure space with the notation for and . If is ergodic and is mixing, then is ergodic.
Proof. Relying on Remark 2.34.c) we verify Eq (2.42) by proving it for sets and which generate . We make use of and observe that
Using ergodicity, we find that
(2.44) |
Since is mixing, we find for every some such that implies . For we find
(2.45) |
The last two limits Eqs (2.44) and (2.45) imply Eq (2.42).
Remark 2.36. The above proof heavily relies on the mixing property of . Note that for being only ergodic, the statement is wrong, as can be seen from the product of two periodic processes in (see Remark 2.34). Here, the invariant sets are given by for arbitrary measurable .
We recall some facts from random measure theory (see [4]) which will be needed for constructing below. Let denote the space of locally bounded Borel measures on (i.e., bounded on every bounded Borel-measurable set) equipped with the Vague topology, which is generated by the sets
This topology is metrizable, complete and countably generated. A random measure is a measurable mapping
which is equivalent to both of the following conditions
1. For every bounded Borel set the map is measurable
2. For every the map is measurable.
A random measure is stationary if the distribution of is invariant under translations of that is and share the same distribution. From stationarity of one concludes the existence ([10,22] and references therein) of a dynamical system on such that . By a deep theorem due to Mecke (see [4,19]) the measure
can be defined on for every positive with and with compact support. is independent from and in case we find . Furthermore, for every -measurable non negative or - integrable functions the Campbell formula
holds. The measure has finite intensity if .
We denote by
(2.46) |
For random measures we find a more general version of Theorem 2.32.
Theorem 2.37 (Ergodic Theorem [4] 12.2.Ⅷ). Let be a probability space, let be a convex averaging sequence, let be a dynamical system on with invariant -algebra and let be measurable with . Then for -almost all
(2.47) |
Given a bounded open (and convex) set , it is not hard to see that the following generalization holds:
Theorem 2.38 (General Ergodic Theorem). Let be a probability space, be a bounded open set with , let be a dynamical system on with invariant -algebra and let be measurable with . Then for -almost all it holds
(2.48) |
Sketch of proof. Chose a countable dense family of functions that spans and that have support on a ball. Use a Cantor argument and Theorem 2.37 to prove the statement for a countable dense family of . From here, we conclude by density.
Theorem 2.39 (General Ergodic Theorem for the Lebesgue measure). Let be a probability space, be a bounded open set with , let be a dynamical system on with invariant -algebra and let and , where , . Then for -almost all it holds
Proof. Let with . Then
which implies the claim.
The theory of random measures and the theory of random geometry are closely related. In what follows, we recapitulate those results that are important in the context of the theory developed below and shed some light on the relations between random sets and random measures.
Let denote the set of all closed sets in . We write
(2.49) |
(2.50) |
The Fell-topology is created by all sets and and the topological space is compact, Hausdorff and separable [18].
Remark 2.40. We find for closed sets in that if and only if [18]
1. for every there exists such that and
2. if is a subsequence, then every convergent sequence with satisfies .
If we restrict the Fell-topology to the compact sets it is equivalent with the Hausdorff topology given by the Hausdorff distance
Remark 2.41. For closed, the set
is a closed subset of . This holds since
.
Lemma 2.42. (Continuity of geometric operations). The maps and are continuous in .
Proof. We show that preimages of open sets are open. For open sets we find
The calculations for and are analogue.
Remark 2.43. The Matheron--field is the Borel--algebra of the Fell-topology and is fully characterized either by the class of .
Definition 2.44 (Random closed / open set according to Choquet (see [18] for more details)).
Let be a probability space. Then a Random Closed Set (RACS) is a measurable mapping
Let be a dynamical system on . A random closed set is called stationary if its characteristic functions are stationary, i.e. they satisfy for almost every for almost all . Two random sets are jointly stationary if they can be parameterized by the same probability space such that they are both stationary.
A random closed set is called a Random closed -Manifold if is a piece-wise -manifold for P almost every .
A measurable mapping
is called Random Open Set (RAOS) if is a RACS.
The importance of the concept of random geometries for stochastic homogenization stems from the following Lemma by Zähle. It states that every random closed set induces a random measure. Thus, every stationary RACS induces a stationary random measure.
Lemma 2.45 ([32] Theorem 2.1.3 resp. Corollary 2.1.5). Let be the space of closed m-dimensional sub manifolds of such that the corresponding Hausdorff measure is locally finite. Then, the -algebra is the smallest such that
is measurable for every measurable and bounded .
This means that
is measurable with respect to the -algebra created by the Vague topology on . Hence a random closed set always induces a random measure. Based on Lemma 2.45 and on Palm-theory, the following useful result was obtained in [10] (See Lemma 2.14 and Section 3.1 therein). We can thus assume in the following that is a separable metric space.
Theorem 2.46 (See [10]). Let be a probability space with an ergodic dynamical system . Let be a stationary random closed -dimensional -Manifold.
There exists a separable metric space with an ergodic dynamical system and a mapping such that and have the same law and such that still is stationary. Furthermore, is continuous. We identify , and .
Also the following result will be useful below.
Lemma 2.47. Let be a Radon measure on and let be a bounded open set. Let be such that , is continuous. Then
is measurable.
Proof. For we introduce through
and observe that is measurable if and only if for every the map is measurable (see Section 2.10). Hence, if we prove the latter property, the lemma is proved.
We assume and we show that the mapping is even upper continuous. In particular, let in and assume that for all . Since is compact, Remark 2.40. 2. implies that . Furthermore, since has compact support and every Borel measure on is regular, we find . On the other hand, if there exists a subsequence such that for all , then either and or and . For we obtain lower semicontinuity and for general the map is the sum of an upper and a lower semicontinuous map, hence measurable.
Definition 2.48 ((Simple) point processes). A -valued random measure is called point process. In what follows, we consider the particular case that for almost every there exist points and values in such that
The point process is called simple if almost surely for all it holds .
Example 2.49 (Poisson process). A particular example for a stationary point process is the Poisson point process with intensity . Here, the probability to find points in a Borel-set with finite measure is given by a Poisson distribution
(2.51) |
with expectation . Shift-invariance of Eq (2.51) implies that the Poisson point process is stationary.
We can use a given random point process to construct further processes.
Example 2.50 (Hard core Matern process). The hard core Matern process is constructed from a given point process by mutually erasing all points with the distance to the nearest neighbor smaller than a given constant . If the original process is stationary (ergodic), the resulting hard core process is stationary (ergodic) respectively.
Example 2.51 (Hard core Poisson–Matern process). If a Matern process is constructed from a Poisson point process, we call it a Poisson–Matern point process.
Lemma 2.52. Let be a simple point process with almost surely for all . Then is a random closed set of isolated points with no limit points. On the other hand, if is a random closed set that almost surely has no limit points then is a point process.
Proof. Let be a point process. For open and compact we will show that and are measurable. Since and generate the -algebra on , it then follows that is measurable. Now let
Then is Lipschitz with constant and is Lipschitz with constant and support in . Moreover, since is locally bounded, the number of points that lie within is bounded. In particular, we obtain
are measurable.
In order to prove the opposite direction, let be a random closed set of points. Since has almost surely no limit points the measure is locally bounded almost surely. We prove that is a random measure by showing that
For let . By Lemmas 2.42 and 2.47 we obtain that are measurable. Moreover, for almost every we find uniformly and hence is measurable.
Corollary 2.53. A random simple point process is stationary iff is stationary.
Hence we can provide the following definition based on Definition 2.44.
Definition 2.54. A point process and a random set are jointly stationary if and are jointly stationary.
Lemma 2.55. Let be a Matern point process from Example 2.50 with distance and let for be . Then is a random closed set.
Proof. This follows from Lemma 2.42: is measurable and is continuous. Hence is measurable.
Definition 2.56. Let be a probability space and . A discrete dynamical system on is a family of measurable bijective mappings satisfying (i)–(iii) of Definition 2.28 with replaced by . A set is almost invariant if for every it holds and is called ergodic with respect to if every almost invariant set has measure or .
Similar to the continuous dynamical systems, also in this discrete setting an ergodic theorem can be proved.
Theorem 2.57 (See Krengel and Tempel'man [16,27]). Let be a convex averaging sequence, let be a dynamical system on with invariant -algebra and let be measurable with . Then for almost all
(2.52) |
In the following, we restrict to for simplicity of notation.
Let . We consider an enumeration of such that and write for all . We define a metric on through
We write and . The topology of is generated by the open sets , where for some , is an open set. In case is compact, the space is compact. Further, is separable in any case since is separable (see [14]).
Lemma 2.58. Suppose for every there exists a probability measure on such that for every measurable it holds . Then defined as follows defines a probability measure on :
Proof. We consider the ring
and make the observation that is additive and positive on and . Next, let be an increasing sequence of sets in such that . Then, there exists such that and since , for every , we conclude for some . Therefore, where . We have thus proved that can be extended to a measure on the Borel--Algebra on (See [2, Theorem 6-2]).
We define for the mapping
Remark 2.59. In this paper, we consider particularly . Then is equivalent to the power set of and every is a sequence of and corresponding to a subset of . Shifting the set by corresponds to an application of to .
Now, let be a stationary ergodic random open set and let . Recalling Eq (2.2) the map is measurable due to Lemma 2.42 and we can define .
Lemma 2.60. If is a stationary ergodic random open set then the set
(2.53) |
is a stationary random point process with respect to .
Remark 2.61. Note that is in general not ergodic since is not necessarily injective.
Proof. By a simple scaling we can assume and write . Evidently, corresponds to a process on with values in writing if and if . In particular, we write . This process is stationary as the shift invariance of induces a shift-invariance of with respect to . It remains to observe that the probabilities and induce a random measure on in the way described in Remark 2.59.
Remark 2.62. If is mixing one can follow the lines of the proof of Lemma 2.35 to find that is ergodic. However, in the general case is not ergodic. This is due to the fact that by nature on has more invariant sets than. For sufficiently complex geometries the map is onto.
Definition 2.63 (Jointly stationary). We call a point process with values in to be strongly jointly stationary with a random set if the functions , are jointly stationary with respect to the dynamical system on .
Lemma 3.1. Let be a Lipschitz domain. Then for every with the following holds: For every and there exists with such that with it holds .
Proof. We can assume that is locally a cone as in Figure 4. With regard to Figure 4, for with and as in the statement we can place a right circular cone with vertex (apex) and axis and an aperture inside , where . In other words, it holds . Along the axis we may select with . Then the distance of to the cone is given through
In particular as defined above satisfies the claim.
Continuity properties of , and
Lemma 3.2 ( is -regular). Let , be a Lipschitz domain and recall Eq (1.7). Then is -regular in the sense of Definition 2.22. In particular, is locally Lipschitz continuous with Lipschitz constant and for every and it holds
(3.1) |
Remark 3.3. The latter lemma does not imply global Lipschitz regularity of .
Proof of Lemma 3.2. It is straightforward to verify that implies and we conclude with Lemma 2.24.
Corollary 3.4. For fixed the function is right continuous and monotone increasing (i.e. u.s.c.) on .
Proof. This follows because being Lipschitz constant of in implies being Lipschitz constant of in for every .
With regard to Lemma 2.3, the relevant quantity for local extension operators is related to , where is the related Lipschitz constant. While we can quantify in terms of and , this does not work for . Hence we cannot quantify in terms of its neighbors. This drawback is compensated by a variational trick in the following statement.
Lemma 3.5. Let be a Lipschitz domain and let satisfy Eq (3.1) such that is -regular. For and let be given in Eq (1.8) and define for
(3.2) |
(3.3) |
Then, is positive and locally Lipschitz continuous on with Lipschitz constant and is -regular in the sense of Definition 2.22. In particular, for it holds
(3.4) |
Furthermore, is well defined.
Remark 3.6. Like in Remark 3.3 this does not imply global Lipschitz regularity of or .
Corollary 3.7. Every Lipschitz domain has extension order 1 and symmetric extension order 2.
Proof. This follows from and Lemmas 2.3 and 2.7 applied to and .
Proof of Lemma 3.5. Let with by Lemma 3.2. For every let such that
Since and we find and hence . This implies at the same time that is -regular and that
Since was arbitrary, we conclude . Moreover, we find . And we conclude the first part with Lemma 2.24.
Second, it holds for every and that
and choosing and taking the supremum on both sides, we infer that is well defined.
Corollary 3.8. Let and let be a locally -regular open set, where we restrict by . Then there exists a countable number of points such that is completely covered by balls where for some . Writing
For two such balls with it holds
(3.5) |
Furthermore, there exists and such that
and for .
Proof. The existence of the points and Balls satisfying Eq (3.5) follows from Theorem 2.25, in particular Eq (2.37). It holds for
Lemma 3.1 yields existence of such that . By the mutual minimal distance the latter implies for .
Lemma 3.9. Let , be a locally -regular open set and let such that for every there exists , such that is -regular in . For let from Lemma 3.2 or from Lemma 3.5 and define
(3.6) |
Then, for fixed , is upper semicontinuous and on each bounded measurable set the quantity
(3.7) |
with if is well defined. The functions
are upper semicontinuous.
Remark 3.10. Note at this point that defined in Eq (1.11) is a function on and different from .
Notation. The infimum in Eq (3.6) is a for . We sometimes use the special notation
Proof of Lemma 3.9. Let with . Writing and and
we observe from -regularity of (given by Lemma 3.5) that and . Hence we find
Observing that as we find and is u.s.c.
Let . First observe that . The set is compact and hence in the Hausdorff metric as . Let such that . Since we find converges along a subsequence and . Hence
In particular, is u.s.c.
Measurability and Integrability of Extended Variables
Lemma 3.11. Let , let be a Lipschitz domain, let be continuous such that and let be - and -regular. For let from Lemma 3.2 or , , from Lemma 3.5. Then from (1.10) is measurable and from (1.11) is upper semicontinuous.
In what follows, we write for
Proof. Step 1: We write for simplicity. Let be a dense subset. If for some then also for sufficiently small, by continuity of . Hence every is upper semicontinuous and it holds . In particular, is a measurable function and hence the set is measurable. This implies is measurable.
Step 2: We show that for every the preimage is closed. Let be a sequence with and . Let be a sequence with where boundedness of implies along a subsequence. Since is continuous, it follows . On the other hand and thus .
Lemma 3.12. Under the assumptions of Lemma 3.11 let . Then there exists a constant only depending on the dimension such that for every bounded open domain and it holds
(3.8) |
(3.9) |
Finally, it holds
(3.10) |
Remark 3.13. Estimates Eqs (3.8) and (3.9) are only rough estimates and better results could be obtained via more sophisticated calculations that make use of particular features of given geometries.
Proof. Like in the previous proof we write for simplicity.
Step 1: Given with let
(3.11) |
Such exists because is locally compact and is continuous. We observe with help of the definition of , the triangle inequality and Eq (2.36)
The last line particularly implies Eq (3.10) and
Step 2: By Theorem 2.25 we can chose a countable number of points such that is completely covered by balls where . For simplicity of notation we write and . Assume with given by Eq (3.11). Since the balls cover , there exists with , implying and hence . Hence we find
Step 3: For with we can distinguish two cases:
1. : Then and hence by Eq (2.36).
2. : Then and hence by Eq (2.36).
and hence
Step 4: Let be fixed and define , . By construction, every with satisfies and hence if and we find and . This implies that
We further observe that the minimal surface of is given in case when is a cone with opening angle . The surface area of in this case is bounded by . This particularly implies up to a constant independent from :
The second integral formula follows in a similar way.
Lemma 3.14. Let be a stationary and ergodic random open set such that
Then there exists and a positive, monotonically decreasing function such that almost surely is -mesoscopic regular.
Proof. Step 1: For some and with positive probability the set contains a ball with radius : Otherwise, for every the set almost surely would not contain an open ball with radius . In particular with probability the set would not contain any ball. But then almost surely, contradicting the assumptions.
Step 2: We define
Due to Step 1 the stationary ergodic random measure has positive intensity . It holds further for that implies the existence of . We prove this using an indirect argument assuming that . In particular, there exists for every a set with for every with and
But for almost every it holds by the ergodic theorem
which implies the existence of , a contradiction.
Definition 3.15 (Isotropic cone mixing). A random set is isotropic cone mixing if there exists a jointly stationary point process in or in , , such that almost surely two points have mutual minimal distance and such that . Further there exists a function with as and such that with ( being the canonical basis of ) and with the notation given in Eq (2.1)
(3.12) |
Lemma 3.16 (A simple sufficient criterion for Eq (3.12)). Let be stationary ergodic and -regular. Then is isotropic cone mixing with
and with
(3.13) |
from Lemma 2.60. Vice versa, if is isotropic cone mixing for then satisfies Eq (1.6) with .
Proof of Lemma 3.16. Because of it holds for
The existence of implies that there exists at least one such that and we find
In particular, for and large enough we discover
The relation Eq (3.12) holds with .
The other direction is evident.
Properties of from Lemma 3.16
The formulation of Definition 3.15 is particularly useful for the following statement.
Lemma 3.17 (Size distribution of cells). Let be a stationary and ergodic random open set that is isotropic cone mixing for , , and . Then and its Voronoi tessellation have the following properties:
1. If is the open Voronoi cell of with diameter then is jointly stationary with and for some constant depending only on
(3.14) |
2. For let . Then
(3.15) |
Proof. 1. For simplicity of notation let . The first part follows from the definition of isotropic cone mixing: We take arbitrary points . Then the planes given by the respective equations define a bounded cell around , with a maximal diameter which is proportional to . The constant depends nonlinearly on with as . Estimate Eq (3.14) can now be concluded from the relation between and and from Eq (3.12).
2. This follows from Lemma 2.31.
Lemma 3.18. Let be a stationary and ergodic random point process with minimal mutual distance for and let be such that the Voronoi tessellation of has the property
Furthermore, let be measurable and i.i.d. among and let be independent from each other. Let either
be the cell enlarged by the factor or a ball of radius around , let and let
where are fixed a constant. Then is jointly stationary with and for every there exists such that
(3.16) |
where
Corollary 3.19. Under the assumptions of Lemma 3.18 let additionally , . Then
Proof of Lemma 3.18. We write , , , . Let
We observe that the mutual minimal distance implies for every
(3.17) |
which follows from the uniform boundedness of cells , and the minimal distance of . Then, writing for every it holds by stationarity and the ergodic theorem
In the last inequality we made use of the fact that every cell , , has volume smaller than . We note that for
Due to Eq (3.17) we find
and obtain for and :
For the sum to converge, it is sufficient that
for some . Hence, for such it holds
and thus Eq (3.16).
Remark 4.1. All calculations that follow in the present Section 4 equally work for arbitrarily distributed radii associated to and replacing the constant , e.g., with
However, for simplicity of presentation, we chose to work with constant from the start.
For this whole section, let be a Lipschitz domain which furthermore satisfies the following assumption.
Assumption 4.2. Let be an open (unbounded) set and let be a set of points having mutual distance if and with for every (e.g. , see Eq (2.53)). We construct from a Voronoi tessellation and denote by the Voronoi cell corresponding to with diameter with . Let be monotone decreasing with , if and for . We define on the Lipschitz functions
(4.1) |
Lemma 2.20 implies
(4.2) |
and thus Eq (4.1) yields for some depending only on , and that
(4.3) |
Definition 4.3 (Weak Neighbors). Under the Assumption 4.2, two points are called to be weakly connected (or weak neighbors), written or if . For open we say if . We then define
(4.4) |
In view of Assumption 4.2 we bound by and recall Eq (3.1). As announced in the introduction, we apply Corollary 3.8 for (we study mostly and in the following) to obtain a complete covering of by balls , , where . Recalling Eqs (3.2) and (3.3) we define with and with and define
(4.5) |
where we recall the construction of and in Eqs (1.16) and (1.17) and note that independent from .
Lemma 4.4. For , and any two balls either or and
(4.6) |
Furthermore, there exists a constant depending only on the dimension and some such that
(4.7) |
(4.8) |
(4.9) |
Finally, there exist non-negative functions and independent from such that for : , for . Further, on all and on and and there exists depending only on such that for all it holds
(4.10) |
Remark 4.5. We often can improve to at least . To see this assume is flat on the scale of . Then all points lie on a -dimensional plane and we can thus improve the argument in the following proof to .
Proof. Eq (4.6) follows from Eq (3.5). For improved readability we drop the indices and .
Let be fixed. By construction in Corollary 3.8, every with satisfies and hence if and we find and . This implies Eqs (4.7) and (4.8) for and the statement for follows analogously.
For two points , such that it holds due to the triangle inequality . Let and choose such that is maximal. Then and every satisfies . Correspondingly, for all such . In view of Eq (3.5) this lower local bound of implies a lower local bound on the mutual distance of the . Since this distance is proportional to , this implies Eq (4.9) with . This is by the same time the upper estimate on .
Let be symmetric, smooth, monotone on with and on . For each we consider a radially symmetric smooth function and an additional function . In a similar way we may modify such that for . Then we define . Note that by construction of and we find and on .
Estimate Eq (4.10) follows from Eq (4.7).
By Lemma 2.3 in case there exist local extension operator
(4.11) |
which is linear continuous with bounds
(4.12) |
(4.13) |
Of course, higher are always valid, but the result becomes worse, as we will see.
Definition 4.6. Using Notation 1.1.6 for every let
(4.14) |
Due to the definitions, we find
(4.15) |
Lemma 4.7. Let be a Lipschitz domain (i.e. locally -regular) with bounded by and let Assumption 1.8 hold and let be the constant from (4.9). Then for every bounded open with and the linear operator
is continuous and writing
the operator satisfies for some not depending on
(4.16) |
(4.17) |
where
(4.18) |
Remark 4.8. Since the covering is locally finite we find
By Lemmas 3.5 and 2.7 in case the local extension operator
(4.19) |
is linear continuous with bounds
(4.20) |
Like in Section 4.2 lower values of are possible, acknowledged by Definition 1.9 of symmetric extension order.
Definition 4.9. Using the notation of Definition 1.12 let
(4.21) |
where are the extension operators on given by the symmetric extension order of .
By definition we verify as well as
and similarly for . Furthermore, it holds
(4.22) |
Lemma 4.10. Let be a locally -regular open set with delta bounded by , let Assumption 1.8 hold and let be the constant from Eq (4.9). Then for every bounded open , the operator
is linear, well defined and with
satisfies
(4.23) |
(4.24) |
(4.25) |
where is given by Eq (4.18).
Due to Eq (4.14), even if has boundary values on , the function can have values for every , while it will be zero outside of . For periodic geometries, however, we can assume without loss of generality that , while in the stochastic case the set can be arbitrary large, even though large might be unlikely. Fortunately, as the diameter of increases, the diameter of statistically approaches that of . We can quantify this approaching via the following result.
Theorem 4.11. For and for both operators given in Eqs (4.14) and (4.21) the following holds: For every bounded open set with and let
If the mesoscopic regularity function of satisfies for some , and then there exists almost surely such that for every it holds .
Proof. We consider two balls with .
We write and for with . For we introduce
and find
On the other hand,
where depends only on the minimal mutual distance of the points, i.e. , and the shape of . Now, since we can choose and find
Since the right-hand side converges to as , we can conclude.
Lemma 4.12. Let , , , be a family of real numbers such that and let . Then
Proof.
Proof of Lemma 4.7. For improved readability, we drop the indices and in the following.
We prove Eq (4.16) of Lemma 4.7. Equation (4.7) can be derived in a similar but shorter way. Lemma 4.10 can be proved in a similar way with some inequalities used below being replaced by the "symmetrized" counterparts. We will make some comments towards this direction in Step 4 of this proof.
For shortness of notation (and by abuse of notation) we write
and similar for integrals over and . For simplicity of notation, we further drop the index in the subsequent calculations.
We introduce the quantities
and note that as well as . Writing
the integral over can be estimated via
(4.26) |
(4.27) |
Step 1: Using Eq (1.14) and as well as we conclude
After a Hölder estimate and using on , we obtain
(4.28) |
and it only remains to estimate .
Step 2: Concerning , we first observe that for each it holds
(4.29) |
We use for every together with Eqs (4.29) and (4.7) to obtain
Note that
(4.30) |
Furthermore and are defined on and respectively and on and because of Eq (4.6). Furthermore, both functions can be extended from and to and on and respectively using Lemma 2.1 such that for some independent from
Since now on and we chose such that for and it holds by the Poincaré inequality (2.14), the microscopic regularity and the estimate Eq (3.4)
We obtain with microscopic regularity , the finite covering Eq (4.8) and the proportionality (3.5) that
Next we estimate from Jensens inequality with and Eq (4.10)
Using once more Assumption 1.8 as well as
(4.31) |
and we infer from Eq (2.14)
Now we make use of the extension estimate Eq (1.14) to find
which in total implies for
Making use of Eq (4.9) we find
and it only remains to estimate .
Step 3: We observe with help of and that
and Lemma 4.12 yields
Step 4: Concerning the proof of Lemma 4.10 we follow the above lines with the following modifications.
We use the Nitsche extension operators. Hence, instead of Eq (1.14) we use Eq (1.15). The local extended functions are called
and Eq (4.30) remains valid. We find it worth mentioning that and hence
We furthermore replace Lemma 2.1 by Lemma 2.6 and the Poincaré inequality (2.14) by (2.23). Finally we observe that Eq (4.31) is replaced by
Proof. We use the covering of by and set , and write , . Due to Lemma 2.5 we find locally
(4.32) |
We thus obtain
which yields by the uniform local bound of the covering, defined in Lemma 3.12, twice the application of Eqs (3.10) and (4.32)
With Hölders inequality and replacing by , the last estimate leads to Eq (1.12). The second estimate goes analogue since the local covering by is finite.
Remark 5.1. The following Lemmas 5.2 and 5.3 also hold with and replaced by and respectively.
Lemma 5.2. Under Assumptions 1.8, 4.2 let be non-negative and have support and let .
Writing , and
for every and it holds
and
Proof. We find from Hölder's and Jensen's inequality
The second part follows accordingly.
Lemma 5.3. Under Assumptions 1.8, 4.2 for every and it holds
with the similar formula holding for replaced by .
Proof. We observe with help of Eq (4.3) and with Lemma 3.17.2)
(5.1) |
(5.2) |
We write
and find
Now we make use of Eq (5.1) and once more of Lemma 3.17.2) to obtain for the first bracket on the right-hand side an estimate of the form
which implies
where we used . From Hölder's inequality the Lemma follows.
For two points , we denote
the cylinder (or pipe) around the straight line segment connecting and with radius .
Recalling Example 2.49 we consider a Poisson point process with intensity (recall Example 2.49) and construct a hard core Matern process by deleting all points with a mutual distance smaller than for some (refer to Example 2.50). From the remaining point process we construct the Delaunay triangulation and assign to each a random number in in an i.i.d. manner from some probability distribution . We finally define
(6.1) |
the family of all pipes generated by the Delaunay grid "smoothed" by balls with the fix radius around each point of the generating Matern process.
Since the Matern process is mixing and is mixing, Lemma 2.35 yields that the whole process is still ergodic. We start with a trivial observation.
Corollary 6.1. The microscopic regularity of is (Def. 1.8) and it holds in Lemma 4.4. Furthermore both the extension order and the symmetric extension order are . In particular, it holds
(6.2) |
with independent from .
Proof. This follows from the fact that can be locally represented as a graph in the upper half space with filling the lower half space.
Lemma 6.2. For the Voronoi tessellation corresponding to holds
Proof. For the underlying Poisson point process it holds for the void probability inside a ball
The probability for a point to be removed is thus and is i.i.d distributed among points of . The total probability to not find any point of in is thus given by not finding a point of plus the probability that all points of are removed, i.e.
From here one concludes.
Remark 6.3. The family of balls can also be dropped from the model . However, this would imply and would cause technical difficulties which would not change much in the result, as the probability for the size of Voronoi cells would still decrease exponentially.
Lemma 6.4. is a point process for that satisfies Assumption 4.2 and is isotropic cone mixing for with exponentially decreasing and it holds and . Furthermore, assume there exists such that , then for some . If then for every it holds
(6.3) |
where is the expectation of the length of pipes.
Proof. Isotropic cone mixing: For the events and are mutually independent, implying
Hence the open set is isotropic cone mixing for with exponentially decaying .
Estimate on the distribution of : By definition of the Delaunay triangulation, two pipes intersect only if they share one common point .
Given three points with and , the highest local Lipschitz constant on is attained in
It is bounded by
where in the following denotes the angle between and , see Figure 7. If is the diameter of the Voronoi cell of , we show that a necessary (but not sufficient) condition that the angle can be smaller than some is given by
(6.4) |
where is a constant depending only on the dimension . Since for small we find , and since the distribution for decays sub-exponentially, also the distribution for at the junctions of two pipes decays sub-exponentially. However, inside the pipes, we find . Due to the cylindrical structure, we furthermore find essential boundedness of . This also implies inside the pipes. At the junction of Balls and pipes we find to be in the upper half of the local plane approximation and hence also here can be chosen (see also Remarks 2.4 and 2.8).
Concerning the expectation of and , we only have to account for the pipes by the above argumentation since the contribution to by the balls is exponentially distributed. In particular, we find for one single pipe that
and hence Eq (6.3) due to the mutual independence of and diameter . It thus remains to proof Eq (6.4).
Proof of Eq (6.4): Given an angle and we derive a lower bound for the diameter of such that for two neighbors of it can hold . With regard to Figure 7, we assume .
Writing the diameter of and , let , where and , . Hence we can assume and in what follows, we focus on the first two coordinates only. The boundaries between the cells and and and lie on the planes
respectively. The intersection of these planes has the first two coordinates
Using the explicit form of , the latter point has the distance
to the origin . Using and we obtain
Given , the latter expression becomes small for small, with the smallest value being . But then
and hence the distance becomes
We finally use and obtain
The latter expression now needs to be smaller than . We observe that the expression on the right-hand side decreases for fixed if increases.
On the other hand, we can resolve . From the conditions and , we then infer Eq (6.4).
Theorem 6.5. Assuming and using the notation of Lemma 5.2 from 6.1 has the property that for there almost surely exists such that for every and every
and for every
Lemma 6.6. For every bounded open set with and let
Then for fixed and there almost surely exists such that for every it holds
Proof. There exists such that and we assume without loss of generality that . We denote and observe that where . For
we observe that due to the minimal mutual distance. The probability that at least one satisfies is given by
Now let and observe while . Then the probability that there exists such that is smaller than
and the right-hand side tends uniformly to as .
Proof of Theorem 6.5. In what follows, we will mostly perform the calculations for and since these calculations are more involved and drop the index in the definition except for the last Step 5.
We first estimate the difference for two directly neighbored points of the Delaunay grid. These are connected through a cylindrical pipe
with round ends and of thickness and total length and we first introduce the new averages in the spirit of Eq (2.27)
As for Eqs (4.15) and (4.22) we obtain
For every with there exists almost surely such that and are connected in through a straight line segment (i.e. lies on the boundary of one of the pipes emerging at or in ) and
The second terms on the right-hand sides are of "mesoscopic type", while the first terms are of local type. We will study both separately in Steps 1 and 2.
Step 1: Using Eqs (2.28) and (2.29), we observe for neighbors
(6.5) |
where
(6.6) |
Step 2: For reasons that we will encounter below, we define
(6.7) |
Recalling the notations of Section 4.1 we assume . Then it holds which implies for from Lemma 5.2
(6.8) |
Hence, for making a non-zero contribution to Eq (6.8), we encounter the conditions and as well as
In particular, this is covered by the more general and symmetric condition
and with respect to Eq (6.7)
(6.9) |
Similarly
(6.10) |
Step 3: We now derive an estimate for . For pairs with let be a discrete path on the Delaunay grid of with length smaller than (this exists due to [28]) that connects and . By the minimal mutual distance of points, this particularly implies that and the path lies completely within . Because
it holds with Eq (6.5)
We make use of and and to find
In the integrals , any of the integrals has and we have an estimate of the form
With this estimate, and using
the integral can be controlled through
(6.11) |
Denoting
and using outside , we observe
(6.12) |
Step 4: We observe
and for every fixed (and using that ) using again Jensens inequality
Having this in mind and using Eq (6.2), we may sum over all to find
With the splitting and Lemmas 2.18 and 2.17 it follows with from Eq (6.6)
Restructuring the right-hand side similar to (6.11)(6.12), the right-hand side is bounded by
where
Step 5: We can replace in the above calculations by . By Lemma 6.6 we can extend to for some fixed and on we can use standard ergodic theory. Hence, the expressions in and converge to a constant as provided
(6.13) |
However, , and are stationary by definition and and or and are independent. Since and clearly have finite expectation by the exponential distribution of and Lemma 3.18, we only mention that due to the strong mixing of and its independence from the distribution of connections
and thus Eq (6.13) holds.
The work [28] which we used in the last proof also opens the door to demonstrate the following result which will be used in part Ⅲ of this series to prove regularity properties of the homogenized equation.
Theorem 6.7. For fixed and every let
with be the shortest path of points in connecting and in and having length . Then there exists
such that is invertible for every and . For let
Then there exists such that for every it holds
Proof. The function consists basically of pipes connecting with that conically become smaller within the ball before entering the pipe and vice versa in . Defining
[28] implies for all but also due to the minimal mutual distance , where depends only on and . Hence writing we can estimate for every
We close this section by proving Theorem 1.15.
Proof of Theorem 1.15. The statement on the support is provided by Theorem 4.11 and the fact that we restrict to functions with support in . Hence in the following we can apply all cited results to instead of . According to Lemmas 4.7 and 5.2, 5.3 and to Theorem 6.5 we need only need to ensure
since is distributed exponentially and the corresponding terms are bounded as long as . We note that the exponential distribution of allows us to restrict to the study of and .
According to Lemma 6.4 it is sufficient that and .
The following argumentation will be strongly based on the so called void probability. This is the probability to not find any point of the point process in a given open set and is given by Eq (2.51) i.e., . The void probability (which we recall to be the probability that no ball intersects with ) for the ball process is given accordingly by
Theorem 6.8. Let (or ). Then is almost surely locally regular and we can define
where for convenience. For every , it holds
Furthermore, it holds and in inequalities (4.9) and (4.4). Furthermore the extension order and symmetric extension order are both . If the above holds with replaced by and with extension order and symmetric extension order .
Remark 6.9. In view of the influence of and on the --ratio given by Lemmas 4.7 4.10 we observe that the union of balls has better properties than the complement.
Proof. We study only since is the complement sharing the same boundary. Hence, in case , all calculations remain basically the same. However, in the first case, it is evident that and because the geometry has only cusps and no dendrites and we refer to Remarks 2.4 and 2.8.
In what follows, we use that the distribution of balls is mutually independent. That means, given a ball around , the set is also a Poisson process. By translation invariance, we assume with . First we note that if and only if , which holds with probability . This is a fixed quantity, independent from .
Now assuming , the distance to the closest ball besides is denoted
with a conditioned probability distribution
It is important to observe that is -regular in the sense of Lemma 2.24. Another important feature in view of Lemma 3.2 is . In particular, and is -regular in case . Hence, in what follows, we will derive estimates on , which immediately imply estimates on .
Estimate on : A lower estimate for the distribution of is given by
(6.14) |
This implies that almost surely for
i.e., .
Intersecting balls: Now assume there exists , such that and for readability assume and . Then
and is at least -regular. Again, a lower estimate for the probability of is given by Eq (6.14) on the interval . Above this value, the probability is approximately given by (for small i.e., ). We introduce as a new variable and obtain from that
(6.15) |
No touching: Assume now that two balls "touch" each other, i.e., we would have in the previous step and for every we had . But
Therefore, the probability that two balls "touch" (i.e., that ) is zero. The almost sure local boundedness of now follows from the countable number of balls.
Estimate on : We again study each ball separately. Let with tangent space and normal space . Let and such that , then also and and by Lemma 2.24. Defining
we find
Studying on we can assume in Eq (3.8) and we find
Hence we find
Estimate on : For two points let and . For the fixed ball we write and obtain with from Eq (6.15). Therefore, we find
We now derive an estimate for To this aim, let . Then implies and
The only random quantity in the latter expression is . Therefore, we obtain with that
Since the point process has finite intensity, this property carries over to the whole ball process and we obtain the condition in order for the right-hand side to remain bounded.
Estimate on : We have to estimate the local maximum number of overlapping in a single point in terms of . We first recall that . Thus large discrepancy between and occurs in points where is large. This is at the intersection of at least two balls. Despite these "cusps", the set consists locally on the order of of almost flat parts. Arguing like in Lemma 4.4 resp. Remark 4.5 this yields .
It remains to verify bounded average connectivity of the Boolean set or its complement. Associated with the connected component there is a graph distance
Using this distance, we shall rely on the following concept.
Definition 6.10 (Statistical Stretch Factor). For and we denote
the statistical local stretch factor and statistical (global) stretch factor .
Lemma 6.11. There exists depending only on and such that for it holds
where are the constants from the following Theorem 6.12.
In order to prove this, we will need the following large deviation result.
Theorem 6.12. (Shape Theorem [29, Thm 2.2]). Let . Then there exist positive constants , and such that the following holds: For every
Proof of Lemma 6.11. We have for
i.e.
One quickly verifies for that and implies for all . Hence we find
While the choice of the points is clearly specified in Section 4.1, there is lots of room in the choice and construction of . In what follows, we choose in the form Eq (2.53). Then we find the following:
Theorem 6.13. Under the above assumptions on the construction of , as well as and using the notation of Lemma 5.2, for every there almost surely exists such that for every and every
and for every
We will prove Theorem 6.13 in a moment, but we first need to provide two more lemmas.
Lemma 6.14. Let be a Poisson point process with finite intensity. Generate a Voronoi tessellation from and for each let be the diameter of the corresponding Voronoi cell. Then for each the following function has finite expectation
Note that this statement is not covered by Lemma 3.18 due to the lack of a minimal distance between the points.
Proof. Given the condition we observe
Since for some , we infer
From here, we conclude with the exponentially in decreasing probability to find more than points within :
for some . Summing up over all cubes we infer
An important property of is the following.
Lemma 6.15. Let with and
Then there exists such that
and
(6.16) |
Furthermore for some fixed and for every
(6.17) |
(6.18) |
Proof. We only treat the vector valued case, the other is proved similarly using results from Section 2.4.
Without loss of generality , and let and . Furthermore, let for and with . Using (2.27), for every number let further
Then for we find from Lemma 2.18
Defining
and using local finiteness of the covering as well as
we find with
From here we conclude the first part. Inequality (6.17) follows from the fact that and the disjointedness of the balls with and Lemma 2.17 with .
Proof of Theorem 6.13. We work with the enumeration and and make use of the underlying point process : For every there exists such that for every there almost surely exists a unique such that . Due to the minimal mutual distance of points in , we can conclude the following: Since , there exists a constant depending only on and such that always
(6.19) |
Since
we find after multiplying with , with corresponding to , then summing over and and integrating over :
where we provide and estimate , and in the following. For the moment, let us observe that an estimate on implies an estimate on from Lemma 5.2 when we choose the present to coincide with the there. To simplify further notation, we will do so. Further, we will estimate a more general term than , which allows us to immediately apply Lemma 5.3.
Step 1: First, we observe there exists such that . Then with help of Eq (6.17) and as well as
Because of Lemmas 3.18 and 6.14 and the exponential decay of probabilities of the first integral on the right-hand side is always bounded.
Step 2. Note that (6.17) also implies by the boundedness of that
Again, the first integral on the right-hand side is bounded.
Step 3: Last but not least, the term
is the most tricky part. The value of the integral will only grow if we assume .
We find a path such that , such that , are neighbors. By our assumptions, for every two points with , the convex hull of lies in . Hence we iteratively replace sequences in the path by if . Hence, we obtain from Eq (6.19) and the definition of the statistical stretch factor
Therefore, for with we observe and the shortest path and with Lemma 6.15
Now all points lie within a radius of around , which implies
Now, by independence of the respective variables, the constant in front converges to
The first term in the product can be estimated with help of Lemma 3.18 and is bounded for every and by the exponential distribution of and . The second term can be estimated similarly.
Step 4: The term
can be estimated in the same way as in Step 3. Together this yields the claim.
A further important property which we will not use in this work, but which is central for part Ⅲ of this series is the following result.
Theorem 6.16. Let be a Matern reduction of the infinite component. For fixed and every let with be the shortest path of points in connecting and in and having length . Then there exists
such that is invertible for every and . For let
Then for every there exists almost surely , such that it holds
Proof. The function consists basically of pipes connecting with that conically become smaller within the ball to fit through the connection between two neighboring balls. Defining
we apply Lemma 6.11 instead of [28] implies for all but also due to the minimal mutual distance , where depends only on and . From here we follow the proof of Theorem 6.7.
We close this section and this work by proving Theorem 1.17.
Proof of Theorem 1.17. The statement on the support is provided by Theorem 4.11 and the fact that we restrict to functions with support in . Hence in the following we can apply all cited results to instead of . According to Lemmas 4.7 and 5.2–5.3 and to Theorem 6.13 we need only need to ensure as well as
where for the simple extension case and for the symmetric extension case. Since is distributed exponentially and the corresponding terms are bounded as long as , we observe that we do not have to care about the involved polynomial terms.
According to Theorem 6.8 it is sufficient that (i.e., ) and .
The authors declare they have not used Artificial Intelligence (AI) tools in the creation of this article.
The work was financed by DFG through the SPP2256 VariationalMethods for Predicting Complex Phenomena in Engineering Structuresand Materials, project HE 8716/1-1 project ID: 441154659.
The authors declare that there is no conflict of interest.
[1] | R. A Adams, J. J. F Fournier, Sobolev Spaces, Netherlands: Elsevier, 2003. |
[2] | S. K. Berberian, Measure and Integration, New York: Chelsea Pub, 1970. |
[3] |
D. Cioranescu, J. S. J. Paulin, Homogenization in open sets with holes, J Math Anal Appl, 71 (1979), 590–607. https://doi.org/10.1016/0022-247X(79)90211-7 doi: 10.1016/0022-247X(79)90211-7
![]() |
[4] | D. J. Daley, D. Vere-Jones, An Introduction to the Theory of Point Processes, New York: Springer-Verlag, 1988. |
[5] | Ricardo G Durán and Maria Amelia Muschietti. The Korn inequality for Jones domains. Electron. J. Differ. Equ., 2004 (2004), 1–10. Available from: http://ejde.math.txstate.edu |
[6] | L. C. Evans, Partial Differential Equations, Providence: American Mathematical Society, 2010. |
[7] |
M. Gahn, M. Neuss-Radu, P. Knabner, Homogenization of reaction–diffusion processes in a two-component porous medium with nonlinear flux conditions at the interface, SIAM Journal on Applied Mathematics, 76 (2016), 1819–1843. https://doi.org/10.1137/15M1018484 doi: 10.1137/15M1018484
![]() |
[8] | A. Giunti, Derivation of Darcy's law in randomly punctured domains, arXiv: 2101.01046, [preprint], (2021) [cited 2023 November 20]. Available from: https://doi.org/10.48550/arXiv.2101.01046 |
[9] |
N. Guillen, I. Kim, Quasistatic droplets in randomly perforated domains, Arch Ration Mech Anal, 215 (2015), 211–281. https://doi.org/10.1007/s00205-014-0777-2 doi: 10.1007/s00205-014-0777-2
![]() |
[10] |
M. Heida, An extension of the stochastic two-scale convergence method and application, Asymptot. Anal., 72 (2011), 1–30. https://doi.org/10.3233/ASY-2010-1022 doi: 10.3233/ASY-2010-1022
![]() |
[11] | M. Höpker, Extension Operators for Sobolev Spaces on Periodic Domains, Their Applications, and Homogenization of a Phase Field Model for Phase Transitions in Porous Media, (German), Doctoral Thesis of University Bremen, Bremen, 2016. |
[12] |
M. Höpker, M. Böhm, A note on the existence of extension operators for Sobolev spaces on periodic domains, Comptes Rendus Math., 352 (2014), 807–810. https://doi.org/10.1016/j.crma.2014.09.002 doi: 10.1016/j.crma.2014.09.002
![]() |
[13] | P. W Jones, Quasiconformal mappings and extendability of functions in Sobolev spaces, Acta Math., 147 (1981), 71–88. |
[14] | J. L. Kelley, General Topology, New York: Van Nostrand, 1955. |
[15] |
S. M. Kozlov, Averaging of random operators, Matematicheskii Sbornik, 151 (1979), 188–202. https://doi.org/10.1070/SM1980v037n02ABEH001948 doi: 10.1070/SM1980v037n02ABEH001948
![]() |
[16] | U. Krengel, A. Brunel, Ergodic theorems, Berlin: Walter de Gruyter, 1985. |
[17] |
P. Marcellini, C. Sbordone, Homogenization of non-uniformly elliptic operators, Appl Anal, 8 (1978), 101–113. https://doi.org/10.1080/00036817808839219 doi: 10.1080/00036817808839219
![]() |
[18] | G. Matheron, Random sets and integral geometry, New York: Wiley, 1975. |
[19] |
J. Mecke, Stationäre zufällige Maße auf lokalkompakten abelschen Gruppen, Probab Theory Relat Fields, 9 (1967), 36–58. https://doi.org/10.1007/BF00535466 doi: 10.1007/BF00535466
![]() |
[20] | J. A. Nitsche, On Korn's second inequality, RAIRO. Analyse numérique, 15 (1981), 237–248. |
[21] | O. A. Oleïnik, A. S Shamaev, G. A Yosifian, Mathematical problems in elasticity and homogenization, Netherlands: Elsevier Science, 2009. |
[22] | G. C. Papanicolaou, S. R. S. Varadhan, Boundary value problems with rapidly oscillating random coefficients, Colloq. Math. Soc. János Bolyai, 27 (1979), 835–873. |
[23] | G Papanicolau, A Bensoussan, J. L Lions, Asymptotic analysis for periodic structures, Netherlands: Elsevier, 1978. |
[24] |
A. Piatnitski, M. Ptashnyk, Homogenization of biomechanical models of plant tissues with randomly distributed cells, Nonlinearity, 33 (2020), 5510. https://doi.org/10.1088/1361-6544/ab95ab doi: 10.1088/1361-6544/ab95ab
![]() |
[25] | B. Schweizer, Partielle Differentialgleichungen: Eine Anwendungsorientierte Einfuhrung, Berlin: Springer, 2013. |
[26] | Elias M Stein, Singular integrals and differentiability properties of functions (PMS-30), Princeton NJ: Princeton University Press, 2016. |
[27] | A. A. Tempel'man, Ergodic theorems for general dynamical systems. Trudy Moskovskogo Matematicheskogo Obshchestva, 26 (1972), 95–132. |
[28] |
G. Xia, The stretch factor of the delaunay triangulation is less than 1.998, SIAM J Sci Comput, 42 (2013), 1620–1659. https://doi.org/10.1137/110832458 doi: 10.1137/110832458
![]() |
[29] |
C. L. Yao, G. Chen, T. D. Guo, Large deviations for the graph distance in supercritical continuum percolation, J Appl Prob, 48 (2011), 154–172. https://doi.org/10.1239/jap/1300198142 doi: 10.1239/jap/1300198142
![]() |
[30] | G. Yosifian, Homogenization of some contact problems for the system of elasticity in perforated domains, Rendiconti del Seminario Matematico della Università di Padova, 105 (2001), 37–64. |
[31] |
G. A Yosifian, Some unilateral boundary-value problems for elastic bodies with rugged boundaries, J. Math. Sci., 108 (2002), 574–607. https://doi.org/10.1023/A:1013162423511 doi: 10.1023/A:1013162423511
![]() |
[32] |
M. Zaehle, Random processes of hausdorff rectifiable closed sets, Math. Nachr., 108 (1982), 49–72. https://doi.org/10.1002/mana.19821080105 doi: 10.1002/mana.19821080105
![]() |
1. | Martin Heida, Benedikt Jahnel, Anh Duc Vu, An ergodic and isotropic zero-conductance model with arbitrarily strong local connectivity, 2024, 29, 1083-589X, 10.1214/24-ECP633 | |
2. | Martin Heida, Benedikt Jahnel, Anh Duc Vu, Regularized homogenization on irregularly perforated domains, 2025, 20, 1556-1801, 165, 10.3934/nhm.2025010 |