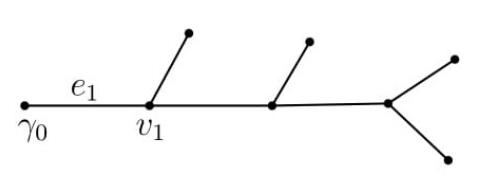
Influence maximization (IM), a central issue in optimizing information diffusion on social platforms, aims to spread posts or comments more widely, rapidly, and efficiently. Existing studies primarily focus on the positive effects of incorporating heuristic calculations in IM approaches. However, heuristic models fail to consider the potential enhancements that can be achieved through network representation learning techniques. Some recent work is keen to use representation learning to deal with IM issues. However, few in-depth studies have explored the existing challenges in IM representation learning, specifically regarding the role characteristics and role representations. This paper highlights the potential advantages of combining heuristic computing and role embedding to solve IM problems. First, the method introduces role granularity classification to effectively categorize users into three distinct roles: opinion leaders, structural holes and normal nodes. This classification enables a deeper understanding of the dynamics of users within the network. Second, a novel role-based network embedding (RbNE) algorithm is proposed. By leveraging the concept of node roles, RbNE captures the similarity between nodes, allowing for a more accurate representation of the network structure. Finally, a superior IM approach, named RbneIM, is recommended. RbneIM combines heuristic computing and role embedding to establish a fusion-enhanced IM solution, resulting in an improved influence analysis process. Exploratory outcomes on six social network datasets indicate that the proposed approach outperforms state-of-the-art seeding algorithms in terms of maximizing influence. This finding highlights the effectiveness and efficacy of the proposed method in achieving higher levels of influence within social networks. The code is available at https://github.com/baiyazi/IM2.
Citation: Xu Gu, Zhibin Wang, Xiaoliang Chen, Peng Lu, Yajun Du, Mingwei Tang. Influence maximization in social networks using role-based embedding[J]. Networks and Heterogeneous Media, 2023, 18(4): 1539-1574. doi: 10.3934/nhm.2023068
[1] | Sergei Avdonin, Julian Edward . An inverse problem for quantum trees with observations at interior vertices. Networks and Heterogeneous Media, 2021, 16(2): 317-339. doi: 10.3934/nhm.2021008 |
[2] | Travis G. Draper, Fernando Guevara Vasquez, Justin Cheuk-Lum Tse, Toren E. Wallengren, Kenneth Zheng . Matrix valued inverse problems on graphs with application to mass-spring-damper systems. Networks and Heterogeneous Media, 2020, 15(1): 1-28. doi: 10.3934/nhm.2020001 |
[3] | Robert Carlson . Dirichlet to Neumann maps for infinite quantum graphs. Networks and Heterogeneous Media, 2012, 7(3): 483-501. doi: 10.3934/nhm.2012.7.483 |
[4] | Kirill D. Cherednichenko, Alexander V. Kiselev, Luis O. Silva . Functional model for extensions of symmetric operators and applications to scattering theory. Networks and Heterogeneous Media, 2018, 13(2): 191-215. doi: 10.3934/nhm.2018009 |
[5] | Arnaud Ducrot, Michel Langlais, Pierre Magal . Multiple travelling waves for an $SI$-epidemic model. Networks and Heterogeneous Media, 2013, 8(1): 171-190. doi: 10.3934/nhm.2013.8.171 |
[6] | Franco Cardin, Alberto Lovison . Finite mechanical proxies for a class of reducible continuum systems. Networks and Heterogeneous Media, 2014, 9(3): 417-432. doi: 10.3934/nhm.2014.9.417 |
[7] | Iryna Pankratova, Andrey Piatnitski . Homogenization of convection-diffusion equation in infinite cylinder. Networks and Heterogeneous Media, 2011, 6(1): 111-126. doi: 10.3934/nhm.2011.6.111 |
[8] | Kun-Peng Jin, Can Liu . RETRACTED ARTICLE: Decay estimates for the wave equation with partial boundary memory damping. Networks and Heterogeneous Media, 2024, 19(3): 1402-1423. doi: 10.3934/nhm.2024060 |
[9] | Feiyang Peng, Yanbin Tang . Inverse problem of determining diffusion matrix between different structures for time fractional diffusion equation. Networks and Heterogeneous Media, 2024, 19(1): 291-304. doi: 10.3934/nhm.2024013 |
[10] | Hyeontae Jo, Hwijae Son, Hyung Ju Hwang, Eun Heui Kim . Deep neural network approach to forward-inverse problems. Networks and Heterogeneous Media, 2020, 15(2): 247-259. doi: 10.3934/nhm.2020011 |
Influence maximization (IM), a central issue in optimizing information diffusion on social platforms, aims to spread posts or comments more widely, rapidly, and efficiently. Existing studies primarily focus on the positive effects of incorporating heuristic calculations in IM approaches. However, heuristic models fail to consider the potential enhancements that can be achieved through network representation learning techniques. Some recent work is keen to use representation learning to deal with IM issues. However, few in-depth studies have explored the existing challenges in IM representation learning, specifically regarding the role characteristics and role representations. This paper highlights the potential advantages of combining heuristic computing and role embedding to solve IM problems. First, the method introduces role granularity classification to effectively categorize users into three distinct roles: opinion leaders, structural holes and normal nodes. This classification enables a deeper understanding of the dynamics of users within the network. Second, a novel role-based network embedding (RbNE) algorithm is proposed. By leveraging the concept of node roles, RbNE captures the similarity between nodes, allowing for a more accurate representation of the network structure. Finally, a superior IM approach, named RbneIM, is recommended. RbneIM combines heuristic computing and role embedding to establish a fusion-enhanced IM solution, resulting in an improved influence analysis process. Exploratory outcomes on six social network datasets indicate that the proposed approach outperforms state-of-the-art seeding algorithms in terms of maximizing influence. This finding highlights the effectiveness and efficacy of the proposed method in achieving higher levels of influence within social networks. The code is available at https://github.com/baiyazi/IM2.
This paper concerns inverse problems for differential equations on quantum graphs. Under quantum graphs or differential equation networks (DENs) we understand differential operators on geometric graphs coupled by certain vertex matching conditions. Network-like structures play a fundamental role in many problems of science and engineering. The range for the applications of DENs is enormous. Here is a list of a few.
–Structural Health Monitoring. {DENs, classically, arise in the study of stability, health, and oscillations of flexible structures that are made of strings, beams, cables}, and struts. Analysis of these networks involve DENs associated with heat, wave, or beam equations whose parameters inform the state of the structure, see, e.g., [44].
–Water, Electricity, Gas, and Traffic Networks. An important example of DENs is the Saint-Venant system of equations, which model hydraulic networks for water supply and irrigation, see, e.g., [33]. Other important examples of DENs include the telegrapher equation for modeling electric networks, see, e.g., [3], the isothermal Euler equations for describing the gas flow through pipelines, see, e.g., [21], and the Aw-Rascle equations for describing road traffic dynamics, see e.g., [29].
–Nanoelectronics and Quantum Computing. Mesoscopic quasi-one-dimensional structures such as quantum, atomic, and molecular wires are the subject of extensive experimental and theoretical studies, see, e.g., [37], the collection of papers in [38,39,40]. The simplest model describing conduction in quantum wires is the Schrödinger operator on a planar graph. For similar models appear in nanoelectronics, high-temperature superconductors, quantum computing, and studies of quantum chaos, see, e.g., [42,41,45].
–Material Science. DENs arise in analyzing hierarchical materials like ceramic and metallic foams, percolation networks, carbon and graphene nano-tubes, and gra-phene ribbons, see, e.g., [1,46,47].
–Biology. Challenging problems involving ordinary and partial differential equations on graphs arise in signal propagation in dendritic trees, particle dispersal in respiratory systems, species persistence, and biochemical diffusion in delta river systems, see, e.g., [7,24,48].
Quantum graph theory gives rise to numerous challenging problems related to many areas of mathematics from combinatoric graph theory to PDE and spectral theories. A number of surveys and collections of papers on quantum graphs appeared in previous years; we refer to the monograph by Berkolaiko and Kuchment, [25], for a complete reference list. The inverse theory of network-like structures is an important part of a rapidly developing area of applied mathematics---analysis on graphs. Being tremendously important for all aforementioned applications these theories have not been, however, sufficiently developed. To date, there are relatively few results related to inverse problems on graphs, and almost exclusively they concern trees, i.e. graphs without cycles.
The first question to be asked when studying inverse problems is how to establish the uniqueness result, i.e. to characterize spectral, or scattering, or dynamical data ensuring uniques solution of the inverse problem. It was shown that inverse boundary spectral and scattering problems for differential equations on graphs with cycles do not have in general a unique solution [41,34,43]. The results on stable identification are known only for trees, and only for the case of boundary inputs (controls) and observations. It was proved that a DEN is identifiable if the actuators and sensors are placed at all or all but one boundary vertices.
There are two groups of uniqueness results in this direction: for trees with a priori known topology and lengths of the edges [28,49,32] and for trees with unknown topology [22,23,11,13]. The most significant result of the last two cited papers is developing a constructive and robust procedure for the recovery tree's parameters, which became known as the leaf peeling method. This method was extended to boundary inverse problems for various types of PDEs on trees in a series of our subsequent papers [7,15,20].
The boundary control method in inverse theory demonstrates [11,23] that inverse (identification) problems for DENs are closely related to control and observation problems for PDEs on graphs. The latter problems were studied in numerous papers, see, e.g. [5,16,30,35,44,50] and references therein.
In this paper, we solve a non-standard dynamical inverse problem for the wave equation on a metric tree graph. Let
The graph
We will assume that for each internal vertex
utt−uxx+qu=0, (x,t)∈(Ω∖V)×[0,T], | (1) |
u|t=0=ut|t=0=0, x∈Ω | (2) |
ui(vk,t)−uj(vk,t)=0, i,j∈J(vk), vk∈V∖Γ, t∈[0,T], | (3) |
∑j∈J(vk)∂uj(vk,t)=Mkutt(vk,t), vk∈V∖Γ, t∈[0,T], | (4) |
u(γ0,t)=f(t), t∈[0,T], | (5) |
u(γk,t)=0, k=1,…,m, t∈[0,T]. | (6) |
Here
Inverse Problem 1. Assume an observer knows the topology of the tree, i.e. the number of boundary vertices and interior vertices, the adjacency relations for this tree, i.e. for each pair of vertices, whether or not there is an edge joining them. Assume the observer also knows the boundary condition (5), and that (6) holds at the other boundary vertices. The unknowns are the lengths
Let
(R01f)(t):=∂uf1(γ0,t). | (7) |
Physically, this corresponds to applying a Dirichlet control and placing a tension sensor, both at
Theorem 1.1. From operator
The proof of this, appearing in Section 2, is an adaptation of an argument well known for the massless case,
We now define the other measurements required for the inverse problem. For interior vertex
(Rkjf)(t):=∂ufj(vk,t), j=2,...,Υk−1. | (8) |
We remark in passing that because the control and sensors are at different places, Theorem 1.1 does not apply. We will show that it is not required to measure
Let
Theorem 1.2. Assume
Placement of internal sensors has been considered in the engineering and computer science literature, see, e.g. [26,27]. We are unaware of any mathematical works treating the inverse problem on general tree graphs with measurements at the internal vertices, except for [8] where the interior vertices are assumed to satisfy delta-prime matching conditions instead of (3), (4). Internal measurements might have advantages in situations where some boundary vertices are inaccessible. In future work, we will study inverse problems of graphs with cycles, in which case both boundary and internal observations appear to be necessary. For a discussion on inverse problems for graphs with cycles see [4] and references therein.
We briefly mention some of the ideas used in the proof of Theorem 1.2. Denote by
The iterative nature of our solution actually allows us to solve what at first glance seems to be a much harder inverse problem.
Inverse Problem 2. Assume an observer knows
To solve this inverse problem, for
Theorem 1.3. Assume
We remark that in the theorem,
In an engineering setting, Inverse Problem 2 could be solved using the following process. One begins with only the control and sensor at
We now compare our paper with the literature. All papers referred to in this paragraph assume all controls and measurements take place at boundary vertices. In [11], the authors consider trees with no masses, and assume that controls and measurements are placed at
In the present paper we develop a new version of the dynamical leaf peeling method. A special feature of our paper is that we use only one control together internal observations. This may be useful in some physical settings where some or most boundary points are inaccessible, or where use of more than one control might be difficult. The extension of dynamical leafing peeling to systems with attached masses, for which the underlying analysis is more complicated than in the mass-free setting, should also be of interest. Another potential advantage of the method presented here is that we recover all parameters of the graphs, including its topology, from the
In this section, we prove well-posedness of our IBVP for a star shape graph. We also derive representations of both the solution and the Schwartz kernel of the components of the response operator. The representations will be used in Section 3 to solve the inverse problem. We then indicate how these results can be extended from star graphs to arbitrary trees.
In what follows, it will convenient to denote
Fn={f∈Hn(R): f(t)=0 if t≤0}, |
where
dndtnHn=H; |
at times we will use
Consider a star shaped graph with edges
Recall the notation
∂2u∂t2−∂2u∂x2+qu=0, x∈ej, j=1,...,N, t∈×[0,T], | (9) |
u|t=0=ut|t=0=0, | (10) |
u(0,t)=ui(0,t)=uj(0,t), i≠j, t∈[0,T], | (11) |
N∑j=1∂uj(0,t)=M∂2u∂t2(0,t), t∈[0,T], | (12) |
u1(ℓ1,t)=f(t), t∈[0,T], | (13) |
uj(ℓj,t)=0, j=2,...,N, t∈[0,T]. | (14) |
Let
g(t)=uf(0,t). | (15) |
For (10), it is standard that the waves have unit speed of propagation on the interval, so
We will use a representation of
Define
{∂w2∂t2(x,s)−∂w2∂x2(x,s)+qj(x)w(x,s)=0, 0<x<s<∞,w(0,s)=0, w(x,x)=−12∫x0qj(η)dη, x>0. |
A proof of solvability of the Goursat problem can be found in [14].
Consider the IBVP on the interval
˜utt−˜uxx+qj(x)˜u=0, 0<x<ℓj, t∈(0,T), | (16) |
˜u(x,0)=˜ut(x,0)=0, 0<x<ℓj,˜u(0,t)=h(t),˜u(ℓj,t)=0, t>0. | (17) |
Then the solution to (16)-(17) on
˜uh(x,t)=∑n≥0: 0≤2nℓj+x≤t(h(t−2nℓj−x)+∫t2nℓj+xwj(2nℓj+x,s)h(t−s)ds) |
−∑n≥1: 0≤2nℓj−x≤t(h(t−2nℓj+x)+∫t2nℓj−xwj(2nℓj−x,s)h(t−s)ds). | (18) |
Setting
Define the "reduced response operator" on
(˜R0jh)(t)=∂˜uj(0,t), t∈[0,T], |
associated to the IBVP (16)-(17). From (18) we immediately obtain:
Lemma 2.1. For
(˜R0jh)(t)=∫t0˜R0j(s)h(t−s)ds, |
with
˜R0j(s)=−δ′(s)−2∑n≥1δ′(s−2nℓj)−2∑n≥1wj(2nℓj,2nℓj)δ(s−2nℓj)+˜r0j(s). |
and
In what follows we will refer to
It will be useful also to represent the solution of a wave equation on an interval when the control is on the right end. Thus consider the IBVP:
vtt−vxx+q1(x)v=0, 0<x<ℓ1, t>0,v(x,0)=vt(x,0)=0, 0<x<ℓ1,v(0,t)=0,v(ℓ1,t)=f(t), t>0. | (19) |
Set
{∂ω2∂t2(x,s)−∂ω2∂x2(x,s)+˜qj(x)ω(x,s)=0, 0<x<s,ω(0,s)=0, ω(x,x)=−12∫x0˜qj(η)dη, x<ℓj. |
By changing coordinates in (18), we get
vf(x,t)= f(t−ℓ1+x)+∫tℓ1−xω1(ℓ1−x,s)f(t−s)ds−f(t−ℓ1−x)−∫tℓ1+xω1(ℓ1+x,s)f(t−s)ds+f(t−3ℓ1+x)+∫t3ℓ1−xω1(3ℓ1−x,s)f(t−s)ds−f(t−3ℓ1−x)−∫t3ℓ1+xω1(3ℓ1+x,s)f(t−s)ds… | (20) |
We begin section by proving an analog of Lemma 2.1 for
Lemma 2.2. The response function for
R01(s)=r01(s)+∑n≥0(anδ′(s−2nℓ1)+bnδ(s−2nℓ1)). |
Here
a1=−2and,b1=−2ω1(2ℓ1,2ℓ1)+4/M. | (21) |
If
Proof. We see that on
uf(x,t)=vf(x,t)+˜ug(x,t). | (22) |
Thus by (18) with
(R01)f(t)=−ufx(ℓ1,t)=−vfx(ℓ1,t)−˜ugx(ℓ1,t) |
=−f′(t)−2∑n≥1f′(t−2nℓ1)−2∑n≥1ω1(2nℓ1,2nℓ1)f(t−2nℓ1)+∫t0∂ω1(0,s)f(t−s)ds |
+2∑n≥1∫t2nℓ1∂ω1(2nℓ1,s)f(t−s)ds+2∑n≥0g′(t−(2n+1)ℓ1) |
+2∑n≥0w1((2n+1)ℓ1,(2n+1)ℓ1)g(t−(2n+1)ℓ1) |
−2∑n≥0∫t(2n+1)ℓ1∂w1((2n+1)ℓ1,s)g(t−s)ds. | (23) |
Next, we study the structure of
Mg″(t)+Ng′(t)=2∑n≥0f′(t−(2n+1)ℓ1)+2∑n≥0ω1((2n+1)ℓl,(2n+1)ℓ1)f(t−(2n+1)ℓ1)−2∑n≥0∫t(2n+1)ℓ1∂ω1((2n+1)ℓ1,s)f(t−s)ds+N∑j=1∫t0∂wj(0,s)g(t−s)ds+2N∑j=1∑n≥1[−g′(t−2nℓj)−wj(2nℓj,2nℓj)g(t−2nℓj)+2∫t2nℓj∂wj(2nℓj,s)g(t−s)ds]. | (24) |
In what follows, we will assume
up(x,t)=(p∗uδ)(x,t)=∫ts=0p(t−s)uδ(x,s)ds. |
As a consequence,
For
g(t)=∑m≥0cmH(t−(2m+1)ℓ1)+tmH1(t−βm)+˜a(t−ℓ1). | (25) |
Here
We will now justify the claim. Substituting (25) into (24), and matching the
M∑m≥0cmδ′(t−(2m+1)l1)=2∑n≥0δ′(t−(2n+1)ℓ1), t∈[0,T]. | (26) |
We conclude that
M(∑m≥0tmδ(t−βm))=−Nc(∑m≥0δ(t−(2m+1)l1))−2cN∑j=1∑n≥1∑m≥0δ(t−2nℓj−(2m+1)ℓ1)+2∑n≥0ω1((2n+1)ℓ1,(2n+1)ℓ1)δ(t−(2n+1)ℓ1). | (27) |
We can solve for
{2nℓj+(2m+1)ℓ1: m≥0; n≥0; j=1,2,...,N}. |
Next, for any given
β0=ℓ1, t0=2(ω1(ℓ1,ℓ1)/M−N/M2). | (28) |
For larger
Case 1.
Case 2. For some positive integer
Case 3. For some positive integer
tm=−NcM−2LcM+2ω1((2nl+1)ℓ1,(2nl+1)ℓ1)M. |
Accounting for these cases, we thus solve for
Next, we solve for
M˜a″(t−ℓ1)+N˜a′(t−ℓ1)+2N∑j=1∑n≥1˜a′(t−ℓ1−2nℓj) |
=˜b0(t)+N∑j=1∫t0∂wj(0,s)˜a(t−s−ℓ1)ds |
+2N∑j=1∑n≥1[−wj(2nℓj,2nℓj)˜a(t−2nℓj−ℓ1)+2∫t2nℓj∂wj(2nℓj,s)˜a(t−s−ℓ1)ds], |
where
M˜a′(t−ℓ1)+N˜a(t−ℓ1)+2N∑j=1∑n≥1˜a(t−2nℓj−ℓ1) |
=˜b1(t−ℓ1)+∫ts=ℓ1(N∑j=1∫s0∂wj(0,r)˜a(s−r−ℓ1)dr |
+2N∑j=1∑n≥1−wj(2nℓj,2nℓj)˜a(s−2nℓj−ℓ1) |
+2∫s2nℓj∂wj(2nℓj,r)˜a(s−r−ℓ1)dr) ds, | (29) |
with
with
Again we solve this Volterra equation to determine
Lemma 2.3. Let
(30) |
where
(31) |
Furthermore,
Proof. Since
Proposition 1. Let
Proof. One can determine
where
Lemma 2.4. Label the central vertex
Here
Proof. the lemma follows immediately from Lemmas 2.2 and 2.3; the details are left to the reader.
We can adapt the methods of the previous subsection to the case the internal vertex is massless (also see [11] for a proof of the results below). Here we will only mention the modifications necessary. In Subsection 2.3, the argument carries through word for word until (24), which becomes a first order integral-differential equation, since
Lemma 2.5. Let
(32) |
where
(33) |
Here
Inserting
Lemma 2.6. The response function for
Here
Proposition 2. Let
The reader is referred to [11] for a proof of this.
We conclude this section with the following lemma, whose proof is similar to that of Lemma 2.4 and is left to the reader.
Lemma 2.7. Label the central vertex
Here
This lemma should be compared with Lemma 2.4. For this lemma, the lead singularity is of the form
In this subsection, we extend some of the previous results to trees. The extensions will be used in Section 3 in solving the inverse problem on trees.
We begin by discussing the wellposed of the system (1)-(6). Let
Theorem 2.8. If
The proof of the theorem is based on the analysis of the waves incoming to, transmitted through and reflected from an interior vertex, and the waves reflected from the boundary vertices. The details are left to the reader; also see [6].
Theorem 2.9. Let
a) The response function for
Here
b) from
Proof. We sketch this proof, leaving the details to the reader. The key point is the waves propagate at unit speed. Hence for
For an internal vertex
Lemma 2.10. Let
Here
Proof. The proof follows from the proof of Lemma 2.2, together with the transmission and reflection properties of waves at interior vertices, and reflection properties at boundary vertices. The details are left to the reader; see also [11]- where, however, the formula analogous to Lemma 2.2 should have the terms of the form
In this section we prove Theorem 1.2. In the first subsection, we establish some notation, and give an outline of the solution method, Steps 1-3. Then in Subsection 3.2, we present the technical heart of our argument, using the equation
and the expansions for
We begin by establishing some notation. Let
We will define an associated response operator as follows. Suppose
(34) |
(35) |
(36) |
(37) |
(38) |
and initial conditions
(39) |
Then we define an associated reduced response operator
with associated response function
We have the following important result is essentially a restatement of Theorem 2.9.
Theorem 3.1. For vertex
In this section we will present an iterative method to determine the operator
Step 1. Let
Step 2. Consider
Step 3. In Subsection 3.3, we will solve for determine
Proposition 3. The function
The rest of this subsection will be devoted to proving this proposition.
Let
Since the operator
This follows from the definition of the response operators for any
(40) |
Below, we will use (40) to determine
Since
(41) |
Here
We now must separately consider the cases
Case A.
In what follows, it will be convenient to extend
(42) |
(43) |
Here
Lemma 3.2. The sets
Proof. By (40), (41), (42), and (43), we get by matching delta functions:
(44) |
Step 1. We solve for
Step 2. We solve for
Case 1.
In this case, we must have
Case 2a.
Case 2b.
Again there are three cases:
Case 2bⅰ.
Case 2bⅱ.
Case 2bⅲ.
Repeating this procedure as necessary, say for a total of
We must have
Step
Iterating the procedure above, suppose for
Here
We can again distinguish three cases:
Case 1.
Case 2. There exists an integer
Note that all the numbers
Case 2ⅰ.
We thus solve for
Case 2ⅱ.
Repeating the reasoning in Case 2ii as often as necessary, we will eventually solve for
Hence we can solve for
Lemma 3.3. The sets
Proof. We identify the Heavyside functions in (40). By (41), (42), and (43), we get
Since the left hand side is known, we can argue as in Lemma 3.2 to solve for
Lemma 3.4. The function
Proof. We solve for
Hence by (41), (42), and (43), we calculate
We set
(45) |
Setting
Since we will be choosing finite
1) For
2) Suppose we have solved for
and identify terms in (45) that we already know. We have for
For
so
(46) |
Similarly, for
(47) |
Combining (46) and (47) with (45), we get
This is a Volterra equation of the second kind, and thus we solve for
Iterating this argument finitely many times, we will have solved for
Case B:
In this case, we must replace (42), (43) by
with piecewise continuous
Careful reading of Steps 2, 3 shows that we can choose any
The purpose of this subsection is to determine
Of course
Since
The rest of the argument here is a straightforward adaptation of the previous subsection. The details are left to the reader.
We would like to thank the referee for his many suggestions that improved the exposition in this paper.
[1] |
J. Gu, G. Li, N. D. Vo, J. J. Jung, Contextual Word2Vec model for understanding chinese out of vocabularies on online social media, Int. J. Semant. Web. Inf. Syst., 18 (2022), 1–14. https://doi.org/10.4018/ijswis.309428 doi: 10.4018/ijswis.309428
![]() |
[2] | G. Manal, Social media data for the conservation of historic urban landscapes: Prospects and challenges, in Culture and Computing. Design Thinking and Cultural Computing (eds. M. Rauterberg), Springer, (2021), 209–223. https://doi.org/10.1007/978-3-030-77431-8_13 |
[3] | J. Zhao, L. Yang, X. Yang, Maximum profit of viral marketing: An optimal control approach, in Proceedings of the 2019 4th International Conference on Mathematics and Artificial Intelligence, Association for Computing Machinery, (2019), 209–214. https://doi.org/10.1145/3325730.3325767 |
[4] | D. Pedro, R. Matt, Mining the network value of customers, in Proceedings of the seventh ACM SIGKDD international conference on Knowledge discovery and data mining, Association for Computing Machinery, (2001), 57–66. https://doi.org/10.1145/502512.502525 |
[5] | X. Song, B. L. Tseng, C. Lin, M. Sun, Personalized recommendation driven by information flow, in Proceedings of the 29th annual international ACM SIGIR conference on Research and development in information retrieval, Association for Computing Machinery, (2006), 509–516. https://doi.org/10.1145/1148170.1148258 |
[6] |
Y. Li, D. Zhang, K. Tan, Real-time targeted influence maximization for online advertisements, Proc. VLDB Endow., 8 (2015), 1070–1081. https://doi.org/10.14778/2794367.2794376 doi: 10.14778/2794367.2794376
![]() |
[7] | L. Simone, M. Diego, R. Giuseppe, M. Maurizio, Mining micro-influencers from social media posts, in Proceedings of the 35th Annual ACM Symposium on Applied Computing, Association for Computing Machinery, (2020), 867–874. https://doi.org/10.1145/3341105.3373954 |
[8] |
X. Zhou, S. Li, Z. Li, W. Li, Information diffusion across cyber-physical-social systems in smart city: a survey, Neurocomputing, 444 (2021), 203–213. https://doi.org/10.1016/j.neucom.2020.08.089 doi: 10.1016/j.neucom.2020.08.089
![]() |
[9] |
V. Soroush, M. Mostafa, R. Deb, Rumor gauge: predicting the veracity of rumors on Twitter, ACM Trans. Knowl. Discov. Data, 11 (2017), 1–36. https://doi.org/10.1145/3070644 doi: 10.1145/3070644
![]() |
[10] |
S. R. Sahoo, B. B. Gupta, Multiple features based approach for automatic fake news detection on social networks using deep learning, Appl. Soft Comput., 100 (2021), 106983. https://doi.org/10.1016/j.asoc.2020.106983 doi: 10.1016/j.asoc.2020.106983
![]() |
[11] | D. Kempe, J. Kleinberg, E. Tardos, Maximizing the spread of influence through a social network, in Proceedings of the Ninth ACM SIGKDD International Conference on Knowledge Discovery and Data Mining, Association for Computing Machinery, (2003), 137–146. https://doi.org/10.1145/956750.956769 |
[12] |
A. G. Cecilia, B. Manuel, T. M. Valentina, An agent-based social simulation for citizenship competences and conflict resolution styles, Int. J. Semant. Web Inf. Syst., 18 (2022), 1–23. https://doi.org/10.4018/IJSWIS.306749 doi: 10.4018/IJSWIS.306749
![]() |
[13] | Y. Rong, Q. Zhu, H. Cheng, A model-free approach to infer the diffusion network from event cascade, in Proceedings of the 25th ACM International on Conference on Information and Knowledge Management, Association for Computing Machinery, (2016), 1653–1662. https://doi.org/10.1145/2983323.2983718 |
[14] | S. Galhotra, A. Arora, S. Virinchi, S. Roy, Asim: A scalable algorithm for influence maximization under the independent cascade model, in Proceedings of the 24th International Conference on World Wide Web, Association for Computing Machinery, (2015), 35–36. https://doi.org/10.1145/2740908.2742725 |
[15] |
A. Cetto, M. Klier, A. Richter, J. F. Zolitschka, "Thanks for sharing"—Identifying users' roles based on knowledge contribution in Enterprise Social Networks, Comput. Net., 135 (2018), 275–288. https://doi.org/10.1016/j.comnet.2018.02.012 doi: 10.1016/j.comnet.2018.02.012
![]() |
[16] |
L. Sopjani, J. J. Stier, S. Ritzén, M. Hesselgren, P. Georén, Involving users and user roles in the transition to sustainable mobility systems: The case of light electric vehicle sharing in Sweden, Transp. Res. Part D: Transp. Environ., 71 (2019), 207–221. https://doi.org/10.1016/j.trd.2018.12.011 doi: 10.1016/j.trd.2018.12.011
![]() |
[17] |
L. B. Jeppesen, K. Laursen, The role of lead users in knowledge sharing, Res. Policy, 38 (2009), 1582–1589. https://doi.org/10.1016/j.respol.2009.09.002 doi: 10.1016/j.respol.2009.09.002
![]() |
[18] |
I. Singh, N. Kumar, S. K. G., T. Sharma, V. Kumar, S. Singhal, Database intrusion detection using role and user behavior based risk assessment, J. Inf. Secur. Appl., 55 (2020), 102654. https://doi.org/10.1016/j.jisa.2020.102654 doi: 10.1016/j.jisa.2020.102654
![]() |
[19] | D. Kempe, J. M. Kleinberg, E. Tardos, Maximizing the spread of influence through a social network, in Proceedings of the ninth ACM SIGKDD international conference on Knowledge discovery and data mining, Association for Computing Machinery, (2003), 137–146. https://doi.org/10.1145/956750.956769 |
[20] | P. Shakarian, A. Bhatnagar, A. Aleali, E. Shaabani, R. Guo, The independent cascade and linear threshold models, in Proceedings of the 2015 IEEE/ACM International Conference on Advances in Social Networks Analysis and Mining, Association for Computing Machinery, (2015), 177–184. https://doi.org/10.1007/978-3-319-23105-1_4 |
[21] | M. Richardson, P. Domingos, Mining knowledge-sharing sites for viral marketing, in Proceedings of the eighth ACM SIGKDD international conference on Knowledge discovery and data mining, Association for Computing Machinery, (2002), 61–70. https://doi.org/10.1145/775047.775057 |
[22] | D. Oriedi, C. de Runz, Z. Guessoum, A. A. Nyongesa, Influence maximization through user interaction modeling, in Proceedings of the 35th Annual ACM Symposium on Applied Computing, Association for Computing Machinery, (2020), 1888–1890. https://doi.org/10.1145/3386901.3388999 |
[23] | L. Sun, A. Chen, P. S. Yu, W. Chen, Influence maximization with spontaneous user adoption, in Proceedings of the 13th International Conference on Web Search and Data Mining, Association for Computing Machinery, (2020), 573–581. https://doi.org/10.1145/3336191.3371785 |
[24] |
J. Guo, W. Wu, Adaptive influence maximization: if influential node unwilling to be the seed, ACM Trans. Knowl. Discov. Data, 15 (2021), 1–23. https://doi.org/10.1145/3447396 doi: 10.1145/3447396
![]() |
[25] | J. Luo, X. Liu, X. Kong, Competitive opinion maximization in social networks, in Proceedings of the 2019 IEEE/ACM International Conference on Advances in Social Networks Analysis and Mining, Association for Computing Machinery, (2019), 250–257. https://doi.org/10.1145/3341161.3342899 |
[26] | Y. Zhang, Y. Zhang, Top-K influential nodes in social networks: A game perspective, in Proceedings of the 40th International ACM SIGIR Conference on Research and Development in Information Retrieval, Association for Computing Machinery, (2017), 1029–1032. https://doi.org/10.1145/3077136.3080709 |
[27] | X. Liu, X. Kong, P. S. Yu, Active ppinion maximization in social networks, Proceedings of the 24th ACM SIGKDD International Conference on Knowledge Discovery & Data Mining, Association for Computing Machinery, (2018), 1840–1849. https://doi.org/10.1145/3219819.3220061 |
[28] | P. Banerjee, W. Chen, L. V.S. Lakshmanan, Maximizing welfare in social networks under a utility driven influence diffusion model, in Proceedings of the 2019 International Conference on Management of Data, Association for Computing Machinery, (2019), 1078–1095. https://doi.org/10.1145/3299869.3319879 |
[29] |
M. M. Keikha, M. Rahgozar, M. Asadpour, M. F. Abdollahi, Influence maximization across heterogeneous interconnected networks based on deep learning, Expert Syst. Appl., 140 (2020). https://doi.org/10.1016/j.eswa.2019.112905 doi: 10.1016/j.eswa.2019.112905
![]() |
[30] |
Q. Zhan, W. Zhuo, Y. Liu, Social influence maximization for public health campaigns, IEEE Access, 7 (2019), 151252–151260. https://doi.org/10.1109/ACCESS.2019.2946391 doi: 10.1109/ACCESS.2019.2946391
![]() |
[31] |
S. Tian, S. Mo, L. Wang, Z. Peng, Deep reinforcement learning-based approach to tackle topic-aware influence maximization, Data Sci. Engineer., 5 (2020), 1–11. https://doi.org/10.1007/s41019-020-00117-1 doi: 10.1007/s41019-020-00117-1
![]() |
[32] | D. Li, J. Liu, J. Jeon, S. Hong, T. Le, D. Lee, et al., Large-scale data-rriven airline market influence maximization, in Proceedings of the 27th ACM SIGKDD Conference on Knowledge Discovery & Data Mining, Association for Computing Machinery, (2021), 914–924. https://doi.org/10.1145/3447548.3467365 |
[33] |
C. Zhang, W. Li, D. Wei, Y. Liu, Z. Li, Network dynamic GCN influence maximization algorithm with leader fake labeling mechanism, IEEE Trans. Comput. Soc. Syst., (2022), 1–9. https://doi.org/10.1109/TCSS.2022.3193583 doi: 10.1109/TCSS.2022.3193583
![]() |
[34] |
W. Li, Z. Li, A. M. Luvembe, C. Yang, Influence maximization algorithm based on Gaussian propagation model, Inf. Sci., 568 (2021), 386–402. https://doi.org/10.1016/j.ins.2021.04.061 doi: 10.1016/j.ins.2021.04.061
![]() |
[35] |
W. Li, Y. Li, W. Liu, C. Wang, An influence maximization method based on crowd emotion under an emotion-based attribute social network, Inf. Process. Manage., 59 (2022), 102818. https://doi.org/10.1016/j.ipm.2021.102818 doi: 10.1016/j.ipm.2021.102818
![]() |
[36] |
W. Li, Y. Hu, C. Jiang, S. Wu, Q. Bai, E. M. K. Lai, ABEM: an adaptive agent-based evolutionary approach for influence maximization in dynamic social networks, Appl. Soft Comput., 136 (2023), 110062. https://doi.org/10.1016/j.asoc.2023.110062 doi: 10.1016/j.asoc.2023.110062
![]() |
[37] |
H. Cai, V. W. Zheng, K. C. Chang, A comprehensive survey of graph embedding: Problems, techniques, and applications, IEEE Trans. Knowl. Data Engineer., 30 (2018), 1616–1637. https://doi.org/10.1109/TKDE.2018.2807452 doi: 10.1109/TKDE.2018.2807452
![]() |
[38] | B. Perozzi, R. Al-Rfou, S. Skiena, Deepwalk: online learning of social representations, in Proceedings of the 20th ACM SIGKDD international conference on Knowledge discovery and data mining, Association for Computing Machinery, (2014), 701–710. https://doi.org/10.1145/2623330.2623732 |
[39] | J. Tang, M. Qu, M. Wang, M. Zhang, J. Yan, Q. Mei, Line: large-scale information network embedding, in Proceedings of the 24th international conference on World Wide Web, Association for Computing Machinery, (2015), 1067–1077. https://doi.org/10.1145/2736277.2741093 |
[40] | A. Grover, J. Leskovec, node2vec: scalable feature learning for networks, in Proceedings of the 22nd ACM SIGKDD international conference on Knowledge discovery and data mining, Association for Computing Machinery, (2016), 855–864. https://doi.org/10.1145/2939672.2939754 |
[41] | C. McCormick, Word2vec tutorial-the skip-gram model, 2016. Available from: http://mccormickml.com/2016/04/19/word2vec-tutorial-the-skip-gram-model. |
[42] |
M. M. Keikha, M. Rahgozar, M. Asadpour, Community aware random walk for network embedding, Knowledge-Based Syst., 148 (2018), 47–54. https://doi.org/10.1016/j.knosys.2018.02.028 doi: 10.1016/j.knosys.2018.02.028
![]() |
[43] | T. Lou, J. Tang, Mining structural hole spanners through information diffusion in social networks, in Proceedings of the 22nd international conference on World Wide Web, Association for Computing Machinery, (2013), 825–836. https://doi.org/10.1145/2488388.2488461 |
[44] |
R. S. Burt, Structural holes and good ideas, Am. J. Soc., 110 (2004), 349–399. https://doi.org/10.1086/421787 doi: 10.1086/421787
![]() |
[45] | S. Wu, J. M. Hofman, W. A. Mason, D. J. Watts, Who says what to whom on Twitter, in Proceedings of the 20th International Conference on World Wide Web, WWW 2011, Association for Computing Machinery, (2011). https://doi.org/10.1145/1963405.1963504 |
[46] | T. Mikolov, K. Chen, G. Corrado, J. Dean, Efficient estimation of word representations in vector space, 2013. Available from: http://arXiv.org/abs/1301.3781. |
[47] |
D. Lusseau, K. Schneider, O. J. Boisseau, P. Haase, E. Slooten, S. M. Dawson, The bottlenose dolphin community of Doubtful Sound features a large proportion of long-lasting associations, Behav. Ecol. Soc., 54 (2003), 396–405. https://doi.org/10.1007/s00265-003-0651-y doi: 10.1007/s00265-003-0651-y
![]() |
[48] | A. L. Traud, P. J. Mucha, M. A. Porter, Social structure of Facebook networks, Phys. A, 391 (2012), 4165–4180. https://arXiv.org/1102.2166 |
[49] | M. J. Newman, Finding community structure in networks using the eigenvectors of matrices, Phys. Rev. E, 74 (2006), 036104. https://arXiv.org/abs/physics/0605087v3 |
[50] |
J. Leskovec, J. Kleinberg, C. Faloutsos, Graph evolution: Densification and shrinking diameters, ACM Trans. Knowl. Discovery Data, 1 (2007). https://doi.org/10.1145/1217299.1217301 doi: 10.1145/1217299.1217301
![]() |
[51] | B. Rozemberczki, R. Davies, R. Sarkar, C. Sutton, Gemsec: graph embedding with self clustering, in Proceedings of the 2019 IEEE/ACM International Conference on Advances in Social Networks Analysis and Mining 2019, Association for Computing Machinery, (2019), 65–72. https://doi.org/10.1145/3341161.3342890 |
[52] | J. Zhang, Y. Luo, Degree centrality, betweenness centrality, and closeness centrality in social network, in Proceedings of the 2017 2nd International Conference on Modelling, Simulation and Applied Mathematics (MSAM2017), 132 (2017), 300–303. https://doi.org/10.2991/msam-17.2017.68 |
[53] |
M. E. J. Newman, A measure of betweenness centrality based on random walks, Soc. Net., 27 (2005), 39–54. https://doi.org/10.1016/j.socnet.2004.11.009 doi: 10.1016/j.socnet.2004.11.009
![]() |
[54] |
D. F. Gleich, PageRank beyond the Web, SIAM Rev., 57 (2015), 321–363. https://doi.org/10.1137/140976649 doi: 10.1137/140976649
![]() |
1. | Satoru Iwasaki, Initial state estimation from limited observations of the heat equation in metric graphs, 2022, 38, 0266-5611, 035007, 10.1088/1361-6420/ac4afc | |
2. | Xiao Xuan Feng, Mahyar Mahinzaeim, Gen Qi Xu, Spectral analysis of a viscoelastic tube conveying fluid with generalized boundary conditions, 2022, 149, 0022-2526, 657, 10.1111/sapm.12516 | |
3. | S. Avdonin, J. Edward, Y. Zhao, Shape, velocity, and exact controllability for the wave equation on a graph with cycle, 2024, 35, 1061-0022, 1, 10.1090/spmj/1791 | |
4. | Z. A. Sobirov, Inverse Source Problem for the Subdiffusion Equation on a Metric Star Graph with Integral Overdetermination Condition, 2023, 44, 1995-0802, 5426, 10.1134/S1995080223120326 | |
5. | R. R. Ashurov, Z. A. Sobirov, A. A. Turemuratova, Inverse Source Problem for the Space-Time Fractional Parabolic Equation on a Metric Star Graph with an Integral Overdetermination Condition, 2024, 116, 0001-4346, 892, 10.1134/S0001434624110026 |