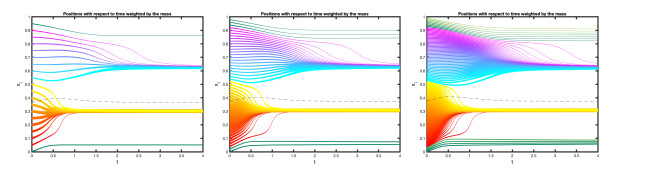
Evolution of the positions for
In this paper, we derive the mean-field limit of a collective dynamics model with time-varying weights, for weight dynamics that preserve the total mass of the system as well as indistinguishability of the agents. The limit equation is a transport equation with source, where the (non-local) transport term corresponds to the position dynamics, and the (non-local) source term comes from the weight redistribution among the agents. We show existence and uniqueness of the solution for both microscopic and macroscopic models and introduce a new empirical measure taking into account the weights. We obtain the convergence of the microscopic model to the macroscopic one by showing continuity of the macroscopic solution with respect to the initial data, in the Wasserstein and Bounded Lipschitz topologies.
Citation: Nastassia Pouradier Duteil. Mean-field limit of collective dynamics with time-varying weights[J]. Networks and Heterogeneous Media, 2022, 17(2): 129-161. doi: 10.3934/nhm.2022001
[1] | Charles Bordenave, David R. McDonald, Alexandre Proutière . A particle system in interaction with a rapidly varying environment: Mean field limits and applications. Networks and Heterogeneous Media, 2010, 5(1): 31-62. doi: 10.3934/nhm.2010.5.31 |
[2] | Michele Gianfelice, Enza Orlandi . Dynamics and kinetic limit for a system of noiseless $d$-dimensional Vicsek-type particles. Networks and Heterogeneous Media, 2014, 9(2): 269-297. doi: 10.3934/nhm.2014.9.269 |
[3] | Fabio Camilli, Italo Capuzzo Dolcetta, Maurizio Falcone . Preface. Networks and Heterogeneous Media, 2012, 7(2): i-ii. doi: 10.3934/nhm.2012.7.2i |
[4] | Peter V. Gordon, Cyrill B. Muratov . Self-similarity and long-time behavior of solutions of the diffusion equation with nonlinear absorption and a boundary source. Networks and Heterogeneous Media, 2012, 7(4): 767-780. doi: 10.3934/nhm.2012.7.767 |
[5] | Maria Teresa Chiri, Xiaoqian Gong, Benedetto Piccoli . Mean-field limit of a hybrid system for multi-lane car-truck traffic. Networks and Heterogeneous Media, 2023, 18(2): 723-752. doi: 10.3934/nhm.2023031 |
[6] | Olivier Guéant . New numerical methods for mean field games with quadratic costs. Networks and Heterogeneous Media, 2012, 7(2): 315-336. doi: 10.3934/nhm.2012.7.315 |
[7] | Michael Herty, Lorenzo Pareschi, Giuseppe Visconti . Mean field models for large data–clustering problems. Networks and Heterogeneous Media, 2020, 15(3): 463-487. doi: 10.3934/nhm.2020027 |
[8] | Michael Herty, Lorenzo Pareschi, Sonja Steffensen . Mean--field control and Riccati equations. Networks and Heterogeneous Media, 2015, 10(3): 699-715. doi: 10.3934/nhm.2015.10.699 |
[9] | Seung-Yeal Ha, Jeongho Kim, Jinyeong Park, Xiongtao Zhang . Uniform stability and mean-field limit for the augmented Kuramoto model. Networks and Heterogeneous Media, 2018, 13(2): 297-322. doi: 10.3934/nhm.2018013 |
[10] | Martino Bardi . Explicit solutions of some linear-quadratic mean field games. Networks and Heterogeneous Media, 2012, 7(2): 243-261. doi: 10.3934/nhm.2012.7.243 |
In this paper, we derive the mean-field limit of a collective dynamics model with time-varying weights, for weight dynamics that preserve the total mass of the system as well as indistinguishability of the agents. The limit equation is a transport equation with source, where the (non-local) transport term corresponds to the position dynamics, and the (non-local) source term comes from the weight redistribution among the agents. We show existence and uniqueness of the solution for both microscopic and macroscopic models and introduce a new empirical measure taking into account the weights. We obtain the convergence of the microscopic model to the macroscopic one by showing continuity of the macroscopic solution with respect to the initial data, in the Wasserstein and Bounded Lipschitz topologies.
A wide range of mathematical models fall into the category of interacting particle systems. Whether they describe the trajectories of colliding particles [7], the behavior of animal groups [1,6,13,22], the cooperation of robots [4] or the evolution of opinions [9,12,15], their common objective is to model the dynamics of a group of particles in interaction. Some of the most widely used models include the Hegselmann-Krause model for opinion dynamics [15], the Vicsek model for fish behavior [22] and the Cucker-Smale model for bird flocks [6]. Two main points of view can be adopted in the modeling process. The Lagrangian (or microscopic) approach deals with individual particles and models the trajectory of each one separately, via a system of coupled Ordinary Differential Equations (ODE). This approach's major limitation is that the dimension of the resulting system is proportional to the number of particles, which can quickly become unmanageable. To combat this effect, one can instead adopt the Eulerian (or macroscopic) approach, and track the concentration of particles at each point of the state space. The resulting equation is a Partial Differential Equation (PDE) giving the evolution of the density of particles over the state space, and whose dimension is independent of the number of particles.
The question of how microscopic properties of particles give rise to macroscopic properties of the system is fundamental in physics. A way to connect the microscopic and the macroscopic points of view is through the mean-field limit. First introduced in the context of gas dynamics, the mean-field limit, applied to systems of weakly interacting particles with a large radius of interaction, derives the macroscopic equation as the limit of the microscopic one when the number of particles tends to infinity [3,10]. The term mean-field refers to the fact that the effects of all particles located at the same position are averaged, instead of considering the individual force exerted by each particle. The mean-field limits of the Hegselmann-Krause, Vicsek and Cucker-Smale models were derived in [5,8,10,14]. More specifically, the mean-field limit of a general system of interacting particles described by
˙xi(t)=1NN∑j=1ϕ(xj(t)−xi(t)) | (1) |
is given by the non-local transport equation in the space of probability measures
∂tμt(x)+∇⋅(V[μt](x)μt(x))=0,V[μt](x)=∫Rdϕ(y−x)dμt(y), | (2) |
where
In [16,17], we introduced an augmented model for opinion dynamics with time-varying influence. In this model, each particle, or agent, is represented both by its opinion
{˙xi(t)=1MN∑j=1mj(t)ϕ(xj(t)−xi(t)),˙mi(t)=ψi((xj(t))j∈{1,⋯,N},(mj(t))j∈{1,⋯,N}), | (3) |
where the functions
As for the classical dynamics (1), we aim to address the natural question of the large population limit. To take into account the weights of the particles, we can define a modified empirical measure by
ψi(x,m):=mi1MqN∑j1=1⋯N∑jq=1mj1⋯mjqS(xi,xj1,⋯xjq). | (4) |
Given symmetry assumptions on
∂tμt(x)+∇⋅(V[μt](x)μt(x))=h[μt](x), | (5) |
in which the left-hand part of the equation, representing non-local transport, is identical to the limit PDE (2) for the system without time-varying weights. The non-local source term of the right-hand side corresponds to the weight dynamics and is given by convolution with
h[μt](x)=(∫(Rd)qS(x,y1,⋯,yq)dμt(y1)⋯dμt(yq))μt(x). |
Since we impose no restriction on the sign of
In [21], well-posedness of (5) was proven for a globally bounded source term satisfying a global Lipschitz condition with respect to the density
Thus, the aim of this paper is to give a meaning to the transport equation with source (5), to prove existence and uniqueness of its solution, and to show that it is the mean-field limit of the microscopic system (3)-(4). Denoting by
Theorem 1.1. For all
Theorem 1.2. For each
limN→∞D(μNt,μt)=0, |
where
The convergence holds in the Bounded Lipschitz and in the Wasserstein topologies, where
We begin by presenting the microscopic model, and by showing that under key assumptions on the mass dynamics, it preserves not only indistinguishability of the agents, but also positivity of the weights as well as the total weight of the system. We then recall the definition and relationship between the Wasserstein, Generalized Wasserstein and Bounded Lipschitz distances. The third section is dedicated to the proof of existence and uniqueness of the solution to the macroscopic equation, by means of an operator-splitting numerical scheme. We show continuity with respect to the initial data in the Bounded Lipschitz and Wasserstein topologies. This allows us to conclude with the key convergence result, in Section 4. Lastly, we illustrate our results with numerical simulations comparing the solutions to the microscopic and the macroscopic models, for a specific choice of weight dynamics.
In [16], a general model was introduced for opinion dynamics with time-varying influence. Given a set of
{˙xi(t)=1MN∑j=1mj(t)ϕ(xj(t)−xi(t)),˙mi(t)=ψi((xj(t))j∈{1,⋯,N},(mj(t))j∈{1,⋯,N}),i∈{1,⋯,N}, | (6) |
where
In this paper, we aim to study the mean-field limit of system (6) for a more specific choice of weight dynamics that will ensure the following properties:
● positivity of the weights:
● conservation of the total mass:
● indistinguishability of the agents.
These key properties will be used extensively to prove well-posedness of the system and convergence to the mean-field limit. We now introduce the model that will be our focus for the rest of the paper. Let
{˙xi(t)=1MN∑j=1mj(t)ϕ(xj(t)−xi(t)),xi(0)=x0i,˙mi(t)=mi(t)1MqN∑j1=1⋯N∑jq=1mj1(t)⋯mjq(t)S(xi(t),xj1(t),⋯xjq(t)),mi(0)=m0i | (7) |
where
Hypothesis 1.
Hypothesis 2.
∀y∈(Rd)q+1,|S(y)|≤ˉS. | (8) |
and
∀y∈(Rd)q+1,∀z∈(Rd)q+1,|S(y0,⋯,yq)−S(z0,⋯,zq)|≤LSq∑i=0|yi−zi|. | (9) |
Furthermore, we require that
S(y0,⋯,yi,⋯,yj,⋯,yq)=−S(y0,⋯,yj,⋯,yi,⋯,yq). | (10) |
Remark 1. The most common models encountered in the literature use an interaction function
●
●
Remark 2. The global boundedness of
In (7), the
The skew-symmetric property of
Proposition 1. Let
(i) For all
(ii) If for all
(iii) If for all
Proof.
S(y0,y1,⋯,yq)=−S(y1,y0,⋯,yq). |
Then it holds
ddtN∑i=1mi=1MqN∑j2=1⋯N∑jq=1[∑j0<j1mj0⋯mjqS(xj0,⋯xjq)+∑j0>j1mj0⋯mjqS(xj0,⋯xjq)]=1MqN∑j2=1⋯N∑jq=1[∑j0<j1mj0⋯mjqS(xj0,xj1,⋯xjq)+∑j1>j0mj0⋯mjqS(xj1,xj0,⋯xjq)]=0. |
˙mi=mi1MqN∑j1=1⋯N∑jq=1mj1⋯mjqS(xi,xj1,⋯xjq)≥−mi1MqN∑j1=1⋯N∑jq=1mj1⋯mjqˉS=−ˉSmi, |
where the last equality comes from the first part of the proposition. From Gronwall's Lemma, for all
mi(t)≥m0ie−ˉSt≥m0ie−ˉSt∗>0. |
Since
Well-posedness of the system (7) is a consequence of the boundedness of the total mass. We have the following result.
Proposition 2. For all
Proof. The proof, modeled after the proofs for the well-posedness of the Graph Limit model in [2], is provided in the Appendix.
We draw attention to the fact that System (7) also preserves indistinguishability of the agents. This property, introduced in [17] and [2], is necessary for the definition of empirical measure to make sense in this new setting.
Indeed, the empirical measure, defined by
Definition 2.1. We say that system (6) satisfies indistinguishability if for all
{x0i=y0i=x0j=y0j for all (i,j)∈J2x0i=y0i for all i∈{1,⋯,N}m0i=p0i for all i∈Jc∑i∈Jm0i=∑i∈Jp0i, |
the solutions
{xi(t)=yi(t)=xj(t)=yj(t) for all (i,j)∈J2xi(t)=yi(t) for all i∈{1,⋯,N}mi(t)=pi(t) for all i∈Jc∑i∈Jmi(t)=∑i∈Jpi(t). |
Whereas the general system (6) does not necessarily satisfy this property, one easily proves that system (7) does satisfy indistinguishability (see [2] for the detailed proof).
Let
From here onward,
‖f‖Lip:=supx,y∈E,x≠ydF(f(x)−f(y))dE(x−y). |
For all
For all
We begin by giving a brief reminder on the various distances that will be used throughout this paper. The natural distance to study the transport of the measure
Wp(μ,ν):=(infπ∈Π(μ,ν)∫Rd×Rd|x−y|pdπ(x,y))1/p, |
for all
Π(μ,ν)={π∈P(Rd×Rd);∀A,B∈B(Rd),π(A×Rd)=μ(A),π(Rd×B)=ν(B)}. |
In the particular case
W1(μ,ν)=sup{∫Rdf(x)d(μ(x)−ν(x));f∈C0,Lipc(Rd),‖f‖Lip≤1} |
for all
Wa,bp(μ,ν)=(inf˜μ,˜ν∈Mp(Rd),|˜μ|=|˜ν|ap(|μ−˜μ|+|ν−˜ν|)p+bp˜Wpp(˜μ,˜ν))1/p |
for all
Remark 3. Observe that the classical and the generalized Wasserstein distances do not generally coincide on the set of probability measures. Indeed, the Wasserstein distance between
W1,11(δx1,δx2)=inf0≤ε≤1(|δx1−εδx1|+|δx2−εδx2|+εWp(δx1,δx2))=inf0≤ε≤1(2(1−ε)+εd(x1,x2)) |
from which it holds:
More generally, if
ρ(μ,ν):=sup{∫Rdf(x)d(μ(x)−ν(x));f∈C0,Lipc(Rd),‖f‖Lip≤1,‖f‖L∞≤1}. |
In turn, this Generalized Wasserstein distance was extended in [21] to the space
∀μ,ν∈Ms1(Rd),Wa,b1(μ,ν)=Wa,b1(μ++ν−,μ−+ν+) |
where
∀μ,ν∈M1(Rd),Wa,b1(μ,ν)=Wa,b1(μ,ν). |
Again, for
∀μ,ν∈Ms1(Rd),W1,11(μ,ν)=ρ(μ,ν). |
From here onward, we will denote by
ρ(μ,ν)≤|μ|+|ν|. | (11) |
We recall other properties of the Generalized Wasserstein distance proven in [21] (Lemma 18 and Lemma 33). Although they hold for any
Proposition 3. Let
●
●
The following proposition, proven in [21], holds for any
Proposition 4. Let
ddtΦvit(x)=vi(t,Φvit(x));Φvi0(x)=x. |
Then
●
●
●
●
The notation
We end this section with a result of completeness that will prove central in the subsequent sections. As remarked in [21],
Proposition 5.
Proof. Let
In particular, note that
From the definition of the Bounded-Lipschitz distance as a particular case of the Generalized Wasserstein distance
∀μ,ν∈P(Rd),ρ(μ,ν)≤W1(μ,ν). | (12) |
As pointed out in Remark 3, the converse is not true in general. However, we can show that for measures with bounded support, one can indeed control the
Proposition 6. Let
ρ(μ,ν)≤W1(μ,ν)≤CRρ(μ,ν) |
where
Proof. Let
Let
Let
∫Rd~fbd(μ−ν)=∫B(0,R)~fbd(μ−ν)=∫B(0,R)fbd(μ−ν)−f(0)∫B(0,R)d(μ−ν)=b |
where the last equality is deduced from
Let us now show that there exists
b=‖~fb‖L∞(Rd)∫Rd~fb/‖~fb‖L∞(Rd)d(μ−ν)≤‖~fb‖L∞(Rd)a. |
Since
It is a well-known property of the Wasserstein distances that for all
Wm(μ,ν)≤Wp(μ,ν). | (13) |
The proof of this result is a simple application of the Jensen inequality [23].
The converse is false in general. However, once again, we can prove more for measures with compact support in the case
Proposition 7. Let
Wp(μ,ν)≤(2R)p−1pW1(μ,ν)1p. |
Proof. Let
∫Rd×Rdd(x,y)pdπ(x,y)=∫B(0,R)2d(x,y)pdπ(x,y)≤(2R)p−1∫B(0,R)2d(x,y)dπ(x,y) |
from which we deduce the claimed property.
In this section, we give a meaning to the non-linear and non-local transport equation with source:
∂tμt(x)+∇⋅(V[μt](x)μt(x))=h[μt](x),μt=0=μ0, | (14) |
where the non-local vector field
● Let
∀μ∈M(Rd),∀x∈Rd,V[μ](x):=∫Rdϕ(x−y)dμ(y). | (15) |
● Let
h[μ](x):=(∫(Rd)qS(x,y1,⋯,yq)dμ(y1)⋯dμ(yq))μ(x). | (16) |
The solution to (14) will be understood in the following weak sense:
Definition 4.1. A measure-valued weak solution to (14) is a measured-valued map
ddt∫Rdf(x)dμt(x)=∫RdV[μt]⋅∇f(x)dμt(x)+∫Rdf(x)dh[μt](x). | (17) |
Remark 4. This model is a modified version of the one proposed in [20]. The form of the source term (16) is slightly more general than the one of [20] (where
The first aim of this paper will be to prove Theorem 1.1, stated again for convenience:
Theorem 1. For all
Notice that we are almost in the frameworks of [18] and [21]. In [18], existence and uniqueness was proven for a transport equation with source of the form (14), for measures in
We now prove that the vector field
First, notice that the continuity of
Proposition 8. The vector field
● For all
● For all
● For all
Proof. The first and second properties are immediate from the definition of
|V[μ](x)−V[ν](x)|=∫B(0,R)ϕ(y−x)d(μ(y)−ν(y))≤(Lϕ+ϕR)supf∈C0,Lipc,‖f‖Lip≤1,‖f‖∞≤1∫Rdf(y)d(μ(y)−ν(y))≤(Lϕ+ϕR)ρ(μ,ν), |
where we used the fact that for all
Proposition 9. The source term
(i)
(ii)
(iii) For all
(iv)
(v)
Proof. For conciseness, we denote
(i) Let
h[μ](Rd)=∫(Rd)q+1S(y0,⋯,yq)dμ0⋯dμq=12∫(Rd)q+1S(y0,⋯,yq)dμ0⋯dμq+12∫(Rd)q+1S(y0,⋯,yj,⋯,yi,⋯,yq)dμ0⋯dμq |
where we used the change of variables
(ii) The second property is immediate from the definition of
(iii) For the third point, let
∫Rdf(x)d(h[μ]−h[ν])=∫Rdf(x)∫RqdS(x,y)dμ1⋯dμqdμ−∫Rdf(x)∫RqdS(x,y)dν1⋯dνqdν=∫Rdf(x)∫RqdS(x,y)dμ1⋯dμqd(μ−ν)+q∑i=1∫Rdf(x)∫RqdS(x,y)dμ1⋯dμidνi+1⋯dνqdν−q∑i=1∫Rdf(x)∫RqdS(x,y)dμ1⋯dμi−1dνi⋯dνqdν=∫R(q+1)df(x)S(x,y)dμ1⋯dμqd(μ−ν)+q∑i=1∫R(q+1)df(x)S(x,y)dμ1⋯d(μi−νi)dνi+1⋯dνqdν. |
We begin by studying the first term
|ψ(x)|=|∫RqdS(x,y)dμ1⋯dμq|≤ˉS|μ|q≤ˉSQq. |
Furthermore, for all
|ψ(x)−ψ(z)|=|∫Rqd(S(x,y)−S(z,y))dμ1⋯dμq|≤LS|μ|q|x−z|, |
where we used the Lipschitz property (9) of
|f(x)ψ(x)−f(z)ψ(z)|=|f(x)(ψ(x)−ψ(z))+(f(x)−f(z))ψ(z)|≤(LS+ˉS)Qq|x−z|. |
This implies that the function
A(f)=Qq(LS+ˉS)∫Rdg(x)d(μ(x)−ν(x))≤Qq(LS+ˉS)ρ(μ,ν). |
Now, let
ζi:yi↦∫Rqdf(x)S(x,y1⋯,yq)dμ(y1)⋯dμ(yi−1)dν(yi+1)⋯dν(yq)dν(x) |
and
For all
|ζi(yi)−ζi(zi)|=|∫Rqdf(x)(S(x,y)−S(x,y1,⋯,zi,⋯,yq))dμ1⋯dμi−1dνi+1⋯dνqdν|≤‖f‖L∞LS|yi−zi||μ|i−1|ν|q−i+1≤LSQq|yi−zi|. |
Hence, the function
Bi(f)≤Qq(LS+ˉS)supf∈C0,Lipc,‖f‖∞≤1,‖f‖Lip≤1∫Rdf(x)d(μ(x)−ν(x))≤Qq(LS+ˉS)ρ(μ,ν). |
We conclude that for all
∫Rdf(x)d(h[μ](x)−h[ν](x))=A(f)+q∑i=1Bi(f)≤(q+1)Qq(LS+ˉS)ρ(μ,ν). |
(iv) Let
(v) Lastly, for all
h[μ](E)=∫E∫RdqS(x,y)dμ1⋯dμqdμ≥−ˉS|μ|qμ(E). |
In [21], existence of the solution to (14) was proven by showing that it is the limit of a numerical scheme discretizing time. It would seem natural to apply directly the results of [21] on well-posedness of the equation in
‖h[μ]−h[ν]‖≤Lh‖μ−ν‖,|h[μ]|≤P and supp(h[μ])⊂B0(R) | (18) |
uniformly for all
For this reason, in order to prove existence of the solution to (14), we design a new operator-splitting numerical scheme that conserves mass and positivity (hence total variation). The inequalities (18) will then hold for all solutions of the scheme, which will allow us to prove that it converges (with a technique very close to the techniques of [18,21]) in the space
Remark that the factor
As stated above, we begin by proving a key property of the scheme
Proposition 10. If
Proof. Let
● For all
μkt(Rd)=μknΔt(Rd)+2(t−nΔt)h[μknΔt](Rd)=1. |
● For all
μkt(Rd)=μk(n+12)Δt(ΦV[μknΔt]−2(t−(n+12)Δt)(Rd))=μk(n+12)Δt(Rd)=1. |
This proves that
● For all
μkt(E)≥μknΔt(E)−ΔtˉSμknΔt(Rd)kμknΔt(E)≥(1−2−kTˉS)μknΔt(E)≥0, |
where we used point
● For all
μkt(E)=μk(n+12)Δt(ΦV[μknΔt]−2(t−(n+12)Δt)(E))≥0 |
by definition of the push-forward.
The result is proven by induction on
We also prove another key property of the scheme: it preserves compactness of the support.
Proposition 11. Let
Proof. Let
supp(μkt)⊂B(0,Rn,k+ϕRn,kΔt)=B(0,Rn,k+(ϕ0+2LϕRn,k)Δt)=B(0,Rn+1,k), |
with
Rn,k=ϕ0Δtn∑i=0(1+2LϕΔt)i+R(1+2LϕΔt)n=(1+2LϕΔt)n(ϕ02Lϕ+R)−ϕ02Lϕ. |
Since
Moreover,
Propositions 10 and 11 allow us to state the main result of this section.
Proposition 12. Given
D(μ,ν):=supt∈[0,T]ρ(μt,νt). |
Proof. Let
|V[μkt](x)−V[μkt](z)|≤Lϕ|x−z| and ‖V[μkt]−V[μls]‖L∞≤LVρ(μkt,μls) |
where
ρ(μknΔt,μk(n+1)Δt)≤ρ(μknΔt,μk(n+12)Δt)+ρ(μk(n+12)Δt,μk(n+1)Δt)≤ρ(μknΔt,μknΔt+Δth[μknΔt])+MVΔt, | (19) |
from Proposition 4. Notice that
ρ(μknΔt,μknΔt+Δth[μknΔt])=Δtρ(0,h[μknΔt])≤Δt|h[μknΔt]|≤ΔtˉS |
from Equation (11), Proposition 3 and Proposition 9. Thus, coming back to (19),
If
ρ(μkt,μks)≤(ˉS+MV)˜t+p(ˉS+MV)+(ˉS+MV)˜s=(ˉS+MV)(s−t). | (20) |
We also estimate:
ρ(μk+1(n+12)Δt,μknΔt)≤ρ(μk+1(n+12)Δt,μk+1nΔt)+ρ(μk+1nΔt,μknΔt)≤Δt2(ˉS+MV)+ρ(μk+1nΔt,μknΔt). | (21) |
We now aim to estimate
μk(n+1)Δt=ΦV[μknΔt]Δt#(μknΔt+Δth[μknΔt])=νkn#νkn#(μknΔt+ΔtHkn),μk+1(n+1)Δt=ΦV[μk+1(n+12)Δt]Δt/2#(μk+1(n+12)Δt+Δt2h[μk+1(n+12)Δt])=νk+1n+12#(νk+1n#(μk+1nΔt+Δt2Hk+1n)+Δt2Hk+1n+12), |
it holds
{A1=ρ(νkn#νkn#μknΔt,νk+1n+12#νk+1n#μk+1nΔt),A2=ρ(νkn#νkn#Hkn,νk+1n+12#νk+1n#Hk+1n),A3=ρ(νkn#νkn#Hkn,νkn+12#Hk+1n+12). |
We study independently the three terms of the inequality. According to Proposition 4 (see also [18] and [21]),
A1≤eLϕΔt2ρ(νkn#μknΔt,νk+1n#μk+1nΔt)+eLϕΔt2−1Lϕ‖V[μknΔt]−V[μk+1(n+12)Δt]‖C0≤(1+LϕΔt)ρ(νkn#μknΔt,νk+1n#μk+1nΔt)+Δt‖V[μknΔt]−V[μk+1(n+12)Δt]‖C0. |
According to Proposition 8 and equation (21),
‖V[μknΔt]−V[μk+1(n+12)Δt]‖C0≤LVρ(μknΔt,μk+1(n+12)Δt)≤LV(Δt2(ˉS+MV)+ρ(μk+1nΔt,μknΔt)). |
Similarly,
ρ(νkn#μknΔt,νk+1n#μk+1nΔt)≤(1+LϕΔt)ρ(μknΔt,μk+1nΔt)+Δt‖V[μknΔt]−V[μk+1nΔt]‖C0≤(1+(Lϕ+LV)Δt)ρ(μknΔt,μk+1nΔt). |
Thus we obtain
A1≤(1+LϕΔt)(1+(Lϕ+LV)Δt)ρ(μknΔt,μk+1nΔt)+ΔtLV(Δt2(ˉS+MV)+ρ(μk+1nΔt,μknΔt))≤(1+2(Lϕ+LV)Δt+Lϕ(Lϕ+LV)Δt2)ρ(μknΔt,μk+1nΔt)+Lϕ2(ˉS+MV)Δt2. |
We treat the second term in a similar way.
A2≤(1+LϕΔt)ρ(νkn#Hkn,νk+1n#Hk+1n)+Δt‖V[μknΔt]−V[μk+1(n+12)Δt]‖C0. |
We have:
ρ(νkn#Hkn,νk+1n#Hk+1n)≤(1+LϕΔt)ρ(Hkn,Hk+1n)+Δt‖V[μknΔt]−V[μk+1nΔt]‖C0≤(1+(LϕLh+LV)Δt)ρ(μknΔt,μk+1nΔt). |
Thus,
A2≤(1+LϕΔt)(1+(LϕLh+LV)Δt)ρ(μknΔt,μk+1nΔt)+ΔtLV[Δt2(2ˉS+MV)+ρ(μk+1nΔt,μknΔt)]≤(1+(Lϕ(Lh+1)+2LV)Δt+Lϕ(LϕLh+LV)Δt2)ρ(μk+1nΔt,μknΔt)+LV2(ˉS+MV)Δt2. |
Lastly, for the third term we have:
A3≤(1+LϕΔt)ρ(νkn#Hkn,Hk+1n+12)+Δt‖V[μknΔt]−V[μk+1(n+12)Δt]‖C0≤(1+LϕΔt)[ρ(νkn#Hkn,Hkn)+ρ(Hkn,Hk+1n+12)]+ΔtLVρ(μknΔt,μk+1(n+12)Δt)≤Δt2(LS+2MV)+O(Δt2)+(1+(LϕLh+LV)Δt)ρ(μk+1nΔt,μknΔt). |
Gathering the three terms together, we have the following estimate:
ρ(μk(n+1)Δt,μk+1(n+1)Δt)≤(1+C1Δt)ρ(μk+1nΔt,μknΔt)+C2Δt2 |
where
ρ(μknΔt,μk+1nΔt)≤C2Δt2(1+C1Δt)n−11+C1Δt−1≤2nC2Δt. |
This allows us to prove the convergence of
ρ(μkT,μk+lT)≤2C2(12k+12k+1+⋯+12k+l−1)≤4C22k. |
A similar estimation holds for any
As an immediate consequence, since
limk→∞D(μk,ˉμ)=0. |
Let
∫T0(∫Rd(∂tf+V[ˉμt]⋅∇f)dˉμt+∫Rdfdh[ˉμt])dt=0. |
We begin by proving the following result:
Lemma 4.2. Let
limk→∞2k−1∑n=0∫(n+1)ΔtknΔtk(∫Rd(∂tf+V[μknΔtk]⋅∇f)dμkt+∫Rdfdh[μknΔtk])dt=0. |
Proof. Let
∫(n+1)ΔtnΔt(∫Rd(∂tf+V[μknΔt]⋅∇f)dμkt+∫Rdfdh[μknΔt])dt=∫(n+12)ΔtnΔt(∫Rd(∂tf+V[μknΔt]⋅∇f)d(μknΔt+2(t−nΔt)h[μknΔt]))dt+∫(n+1)Δt(n+12)Δt(∫Rd(∂tf+V[μknΔt]⋅∇f)d(ΦV[μknΔt]2(t−(n+12))Δt#μk(n+12)Δt))dt+∫(n+1)ΔtnΔt∫Rdfdh[μknΔt]dt=A1+A2+A3+A4 | (22) |
where
{A1=∫(n+12)ΔtnΔt(∫Rd∂tfd(μknΔt+2(t−nΔt)h[μknΔt]))dt,A2=∫(n+1)ΔtnΔt∫Rdfdh[μknΔt]dt,A3=∫(n+12)ΔtnΔt(∫Rd(V[μknΔt]⋅∇f)d(μknΔt+2(t−nΔt)h[μknΔt]))dt,A4=∫(n+1)Δt(n+12Δt(∫Rd(∂tf+V[μknΔt]⋅∇f)d(ΦV[μknΔt]2(t−(n+12))Δt#μk(n+12)Δt))dt. |
We begin by noticing that
A1=−2∫(n+12)ΔtnΔt∫Rdfdh[μknΔt]dt+∫Rdf((n+12)Δt)dμk(n+12)Δt−∫Rdf(nΔt)dμknΔt. | (23) |
We go back to the first two term of (22). Notice that from (23), we have
A1+A2=∫(n+12)ΔtnΔt∫Rd(f(t+Δt2)−f(t))dh[μknΔt]dt+∫Rdf((n+12)Δt)dμk(n+12)Δt−∫Rdf(nΔt)dμknΔt=∫(n+12)ΔtnΔt∫Rd(Δt2∂tf(t)+O(Δt2))dh[μknΔt]dt+∫Rdf((n+12)Δt)dμk(n+12)Δt−∫Rdf(nΔt)dμknΔt. |
Similarly, since
∫Δt0∫Rd∂τf(τ2+(n+12)Δt)dντdτ+∫Δt0∫Rd∇f(τ2+(n+12)Δt)⋅V[μknΔt]dντdτ=∫Rdf((n+1)Δt)dνΔt−∫Rdf((n+12)Δt)dν0 |
After the change of variables
∫(n+1)Δt(n+12)Δt∫Rd(∂tf(t)+2∇f(t)⋅V[μknΔt])d(ΦV[μknΔt]2(t−(n+12))Δt#μk(n+12)Δt)dt=∫Rdf((n+1)Δt)dμk(n+1)Δt−∫Rdf((n+12)Δt)dμk(n+12)Δt. |
We now use this to evaluate the fourth term of (22). We have:
A4=−∫(n+1)Δt(n+12)Δt∫Rd∇f⋅V[μknΔt]d(ΦV[μknΔt]2(t−(n+12))Δt#μk(n+12)Δt)dt+∫Rdf((n+1)Δt)dμk(n+1)Δt−∫Rdf((n+12)Δt)dμk(n+12)Δt. | (24) |
Adding together the second and third terms of (22) and using (24), we obtain:
A3+A4=∫(n+12)ΔtnΔt∫Rd∇f⋅V[μknΔt]dμktdt−∫(n+1)Δt(n+12)Δt∫Rd∇f⋅V[μknΔt]dμktdt+∫Rdf((n+1)Δt)dμk(n+1)Δt−∫Rdf((n+12)Δt)dμk(n+12)Δt. |
Now,
∫(n+12)ΔtnΔt∫Rd∇f⋅V[μknΔt]dμktdt−∫(n+1)Δt(n+12)Δt∫Rd∇f⋅V[μknΔt]dμktdt=∫(n+12)ΔtnΔt∫Rd∇f(t)⋅V[μknΔt]dμktdt−∫(n+12)ΔtnΔt∫Rd∇f(t+Δt2)⋅V[μknΔt]dμkt+Δt2dt=∫(n+12)ΔtnΔt∫Rd∇f(t)⋅V[μknΔt]d(μkt−μkt+Δt2)dt+∫(n+12)ΔtnΔt∫Rd(∇f(t)−∇f(t+Δt2))⋅V[μknΔt]dμkt+Δt2dt=B1+B2+B3 |
where
{B1:=∫(n+12)ΔtnΔt∫Rd∇f(t)⋅V[μknΔt]d(μkt−μk(n+12)Δt)dt,B2:=∫(n+12)ΔtnΔt∫Rd∇f(t)⋅V[μknΔt]d(μk(n+12)Δt−μkt+Δt2)dt,B3:=∫(n+12)ΔtnΔt∫Rd(∇f(t)−∇f(t+Δt2))⋅V[μknΔt]dμkt+Δt2dt. |
The first term gives:
|B1|=|∫(n+12)ΔtnΔt∫Rd∇f(t)⋅V[μknΔt]2((n+12)Δt−t)dh[μknΔt]dt|≤MVˉS‖∇f‖L∞Δt2. |
The second term gives:
|B2|≤|∫(n+12)ΔtnΔtL1ρ(μk(n+12)Δt,μkt+Δt2)dt|≤L1∫(n+12)ΔtnΔtMV(t+Δt2−(n+12)Δt)dt≤L1MVΔt2 |
where, denoting by
Lastly,
|B3|≤∫(n+12)ΔtnΔt∫RdΔt2|∂t(∇f(t))||V[μknΔt]|dμkt+Δt2dt≤MV‖∂t∇f‖L∞Δt24. |
We can finally go back to (22).
∫(n+1)ΔtnΔt(∫Rd(∂tf+V[μknΔt]⋅∇f)dμkt+∫Rdfdh[μknΔt])dt≤∫(n+12)ΔtnΔt∫Rd(Δt2∂tf(t)+O(Δt2))dh[μknΔt]dt+∫Rdf((n+12)Δt)dμk(n+12)Δt−∫Rdf(nΔt)dμknΔt+∫Rdf((n+1)Δt)dμk(n+1)Δt−∫Rdf((n+12)Δt)dμk(n+12)Δt+MV(ˉS‖∇f‖L∞+L1+14‖∂t∇f‖L∞)Δt2≤∫Rdf((n+1)Δt)dμk(n+1)Δt−∫Rdf(nΔt)dh[μknΔt]+CΔt2, |
with
limk→∞|2k−1∑n=0∫(n+1)ΔtnΔt(∫Rd(∂tf+V[μknΔt]⋅∇f)dμkt+∫Rdfdh[μknΔt])dt|≤limk→∞C2k−1∑n=0Δt2=limk→∞CT2−k=0. |
We can now prove the following:
Proposition 13. The limit measure
Proof. We will prove that for all
limk→∞2k−1∑n=0∫(n+1)ΔtnΔt(∫Rd(∂tf+V[μknΔt]⋅∇f)dμkt+∫Rdfdh[μknΔt])dt−∫T0(∫Rd(∂tf+V[ˉμt]⋅∇f)dˉμt+∫Rdfdh[ˉμt])dt=0. | (25) |
First, denoting by
2k−1∑n=0∫(n+1)ΔtnΔt∫Rd∂tfd(μkt−ˉμt)dt=F12k−1∑n=0∫(n+1)ΔtnΔt∫Rd∂tfF1d(μkt−ˉμt)dt≤F12k−1∑n=0∫(n+1)ΔtnΔt(supf∈C0,Lipc,‖f‖Lip≤1,‖f‖∞≤1∫Rdfd(μkt−ˉμt))dt≤F1TD(μk,ˉμ)→k→∞0. |
Secondly, denoting by
∫Rdfd(h[μknΔt]−h[ˉμt])=F2∫RdfF2d(h[μknΔt]−h[ˉμt])≤F2ρ(h[μknΔt],h[ˉμt])≤F2Lhρ(μknΔt,ˉμt)≤F2Lh(ρ(μknΔt,μkt)+ρ(μkt,ˉμt))≤F2Lh((ˉS+MV)Δt+D(μkt,ˉμt)) |
from Equation (19). Hence,
2k−1∑n=0∫(n+1)ΔtnΔt∫Rdfd(h[μknΔt]−h[ˉμt])dt≤F2Lh2k−1∑n=0∫(n+1)ΔtnΔt((ˉS+MV)Δt+D(μkt,ˉμt))dt≤(ˉS+MV)2k−1∑n=0Δt2+TD(μkt,ˉμt))=2−kT(ˉS+MV)+TD(μkt,ˉμt))→k→∞0. |
Thirdly, denoting by
∫RdV[μknΔt]⋅∇fdμkt−∫RdV[ˉμt]⋅∇fdˉμt=∫RdV[μknΔt]⋅∇fd(μkt−ˉμt)+∫Rd(V[μknΔt]−V[μkt])⋅∇fdˉμt+∫Rd(V[μkt]−V[ˉμt])⋅∇fdˉμt≤F3(MV+2LV)ρ(μkt,ˉμt)+F3LV(ˉS+MV)Δt. |
Hence,
∫T0(∫Rd(∂tf+V[ˉμt]⋅∇f)dˉμt+∫Rdfdh[ˉμt])dt=0. |
As remarked in [21], this weak formulation is equivalent to the Definition 4.1. This proves that
Proposition 14. Let
ρ(μt,νt)≤eCtρ(μ0,ν0). |
In particular, this implies uniqueness of the solution to (14).
Proof. Let
ε(t+τ)=ρ(μt+τ,νt+τ)≤A1+A2+A3 | (26) |
where
A3≤(1+2Lτ)ρ(μt+τh[μt],νt+τh[νt])+min{|μt+τh[μt]|,|νt+τh[νt]|}2τLVρ(μt,νt)≤(1+2(2Lϕ+Lh+2LV)τ)ρ(μt,νt). | (27) |
For
∀(t,τ)∈[0,T]×[0,T−t],ρ(μt+τ,ΦV[μt]τ#μt+τh[μt])≤Kτ2. | (28) |
We begin by proving (28) for solutions to the numerical scheme
Dl:=ρ(μk(n+l)Δt,ΦV[μknΔt]lΔt#(μknΔt+lΔth[μknΔt])). |
Notice that by definition of the numerical scheme, for
Let us now suppose that for some
Dl+1=ρ(Pkn+l#(μk(n+l)Δt+ΔtHkn+l),Pkn#ΦV[μknΔt]lΔt#(μknΔt+lΔtHkn+ΔtHkn))≤ρ(Pkn+l#μk(n+l)Δt,Pkn#ΦV[μknΔt]lΔt#(μknΔt+lΔtHkn))+Δtρ(Pkn+l#Hkn+l,Pkn#ΦV[μknΔt]lΔt#Hkn)≤(1+2LϕΔt)ρ(μk(n+l)Δt,ΦV[μknΔt]lΔt#(μknΔt+lΔtHkn))+2ΔtLVρ(μk(n+l)Δt,μknΔt)+Δt(1+2LϕΔt)ρ(Hkn+l,ΦV[μknΔt]lΔt#Hkn)+2Δt2LVρ(μk(n+l)Δt,μknΔt), |
where we used that from Proposition 10, for
Dl+1≤(1+2LϕΔt)K((l−1)2Δt2)+2ΔtLVlΔt(MV+ˉS)+Δt(1+2LϕΔt)(LhlΔt(MV+ˉS)+lΔtMVˉS)+2LVΔt2lΔt(MV+ˉS)≤Δt2[K(l−1)2+l((2LV+Lh)(MV+ˉS)+MVˉS)]+O(Δt3)≤Δt2[K(l−1)2+Kl]≤Kl2Δt2. |
Thus, by induction,
ρ(μt+τ,ΦV[μt]τ#μt+τh[μt])≤ρ(μkt+τ,ΦV[μt]τ#μkt+τh[μkt])+ρ(μt+τ,μkt+τ)+ρ(ΦV[μt]τ#μt+τh[μt],ΦV[μt]τ#μkt+τh[μkt]) |
and by taking the limit
Coming back to (26), and using (27) and (28), it holds
We have thus proven Theorem 1.1: Existence was obtained as the limit of the numerical scheme
We saw in Section 3.2 that the Bounded Lipschitz distance and the
Corollary 1. Let
W1(μt,νt)≤CRTeCtW1(μ0,ν0). |
Furthermore, for all
Wp(μt,νt)≤(2R)p−1pC1pRTeCptWp(μ0,ν0)1p. |
Proof. Let
W1(μt,νt)≤CRTρ(μt,νt)≤CRTeCtρ(μ0,ν0)≤CRTeCtW1(μ0,ν0), |
where
Wp(μNt,μt)≤(2R)p−1pW1(μNt,μt)1p≤(2R)p−1pC1pRTeCptW1(μN0,μ0)1p≤(2R)p−1pC1pRTeCptWp(μN0,μ0)1p. |
Having proven the well-posedness of both the microscopic and macroscopic models, we are now in a position to prove the convergence result stated in Theorem 1.2 that is central to this paper. The proof, as for the now classical proof of convergence of the microscopic dynamics without weights (1) to the non-local transport PDE (2) (see [10]), relies on two ingredients: the fact that the empirical measure satisfies the PDE and the continuity of the solution with respect to the initial data. We begin by defining the empirical measure for our microscopic system with weight dynamics and prove that it does satisfy the PDE (14).
The fact that (7) preserves indistinguishability allows us to define a generalized version of the empirical measure. For all
μNt=1MN∑i=1mi(t)δxi(t) | (29) |
be the generalized empirical measure. From Proposition 1, we know that for all
Proposition 15. Let
Proof. We show that
ddt∫Rdf(x)dμNt(x)=1MN∑i=1˙mi(t)f(xi(t))+1MN∑i=1mi(t)∇f(xi(t))⋅˙xi(t). | (30) |
The first part of the right-hand side of (17) gives
∫RdV[μt]⋅∇f(x)dμNt(x)=1M2N∑i=1N∑j=1mimjϕ(xj−xi)⋅∇f(xi)=1MN∑i=1mi∇f(xi)⋅˙xi. | (31) |
where the last equality comes from the fact that
∫Rdf(x)dh[μNt](x)=1MN∑i=1mif(xi)1MqN∑j1=1⋯N∑jq=1mj1⋯mjqS(xi,xj1,⋯xjq)=1MN∑i=1˙mif(xi). |
where the last equality comes from the fact that
We are finally equipped to prove Theorem 1.2, that we state again here in its full form:
Theorem 2. Let
For each
{˙xi=1MN∑j=1mjϕ(xj−xi),xi(0)=xN,0i˙mi=mi1MqN∑j1=1⋯N∑jq=1mj1⋯mjqS(xi,xj1,⋯xjq),mi(0)=mN,0i, |
and let
limN→∞D(μN0,μ0)=0, |
then for all
limN→∞D(μNt,μt)=0, |
where
∂tμt(x)+∇⋅(∫Rdϕ(x−y)dμt(y)μt(x))=(∫(Rd)qS(x,y1,⋯,yq)dμt(y1)⋯dμt(yq))μt(x), |
with initial data
Proof. Since
Let
To illustrate our convergence result, we provide numerical simulations for a specific model. We also refer the reader to the paper [2] for numerical simulations with a different model.
We recall the first model (M1) proposed in [16], "increasing weight by pairwise competition":
{˙xi(t)=1MN∑j=1mj(t)ϕ(xj(t)−xi(t)),xi(0)=x0i˙mi(t)=1Mmi(t)N∑j=1mj(t)β⟨˙xi(t)+˙xj(t)2,uji⟩,mi(0)=m0i | (32) |
where
With this choice of model, the evolution of each agent's weight depends on the dynamics of the midpoints
In order to ensure continuity, we slightly modify the model and replace
●
●
Then, by replacing
˙mi=1M2miN∑j=1N∑k=1mjmkβ⟨ϕ(xk−xi)+ϕ(xk−xj)2,h(xi−xj)⟩. |
Notice that it is in the form of System (7), with
We can then apply Theorem 1.2.
Consider
∂tμt(x)+∂x(∫Rϕ(y−x)dμt(y)μt(x))=(∫R2S(x,y,z)dμt(y)dμt(z))μt(x). | (33) |
Figures 1, 2 and 3 illustrate this convergence for the specific choices :
●
●
●
f(x):=[3.5√0.4πexp(−5(x−0.25)24)+1√0.4πexp(−5(x−0.90)24)]1[0,1](x) |
and
Remark 5. The interaction function
Figure 1 shows the evolution of
Figure 3 compares the evolutions of
We provide the proof of Proposition 2. It is modeled after the proof of existence and uniqueness of the Graph Limit equation provided in [2], but we write it fully here for self-containedness.
Proof. Let
{˙xi(t)=1MN∑j=1˜mj(t)ϕ(xj(t)−xi(t)),xi(0)=xini; | (34) |
{˙mi(t)=mi(t)1MqN∑j1=1⋯N∑jq=1mj1(t)⋯mjq(t)S(˜xi(t),˜xj1(t),⋯˜xjq(t)),mi(0)=mini. | (35) |
Existence and uniqueness of the solution to the Cauchy problem given by (34) comes from a simple fixed-point argument.
We now show existence and uniqueness of the solution to the second decoupled system (35). Let
(Kminm)i(t):=m0i+∫t0mi(τ)1MqN∑j1=1⋯N∑jq=1mj1(τ)⋯mjq(τ)S(˜xi(τ),˜xj1(τ),⋯˜xjq(τ))dτ |
for all
|(Kminm−Kminp)i|≤∫t01Mq|mi−pi|∑j1⋯jqmj1⋯mjq|S(˜xi,˜xj1,⋯˜xjq)|dτ+∫t01Mqpi∑j1⋯jq|mj1−pj1|mj2⋯mjq|S(˜xi,˜xj1,⋯˜xjq)|dτ+⋯+∫t01Mqpi∑j1⋯jqpj1⋯pjq−1|mjq−pjq||S(˜xi,˜xj1,⋯˜xjq)|dτ≤ˉS˜Tsup[0,˜T]|mi−pi|+qˉS˜T1Msup[0,˜T](piN∑j=1|mj−pj|)≤ˉS˜Tsup[0,˜T]|mi−pi|+qˉS˜Tsup[0,˜T]N∑j=1|mj−pj|. |
Thus,
Let us define the sequences
˙xni=1MN∑j=1mn−1jϕ(xnj−xni),˙mni=mni1MqN∑j1=1⋯N∑jq=1mnj1⋯mnjqS(xn−1i,xn−1j1,⋯xn−1jq) |
with initial conditions
Similarly, notice that for all
maxi∈{1,⋯,N}‖xni(t)‖≤maxi∈{1,⋯,N}‖xini‖+MTM(Φ0t+2Lϕ∫t0maxi∈{1,⋯,N}‖xni(τ)‖dτ) |
and from Gronwall's lemma, for all
maxi∈{1,⋯,N}‖xni(t)‖≤XT:=[maxi∈{1,⋯,N}‖xini‖+MTMΦ0T]e2LϕMTMT. |
We prove that
‖xn+1i−xni‖=‖∫t01MN∑j=1mnjϕ(xn+1j−xn+1i)dτ−∫t01MN∑j=1mn−1jϕ(xnj−xni)dτ‖ |
≤1M(Φ0+2LϕXT)∫t0N∑j=1|mnj−mn−1j|dτ+MTLϕM∫t0N∑j=1(‖xn+1j−xnj‖+‖xn+1i−xni‖)dτ. |
Thus
∑i‖xn+1i−xni‖≤NM(Φ0+2LϕXT)∫t0∑i|mni−mn−1i|dτ+2NMTLϕM∫t0∑i‖xn+1i−xni‖dτ. |
A similar computation, for
|mn+1i−mni|≤∫t0|mn+1i−mni|1Mq∑j1⋯jqmn+1j1⋯mn+1jqS(xni⋯xnjq)dτ+∫t0mni1Mq∑j1⋯jq|mn+1j1−mnj1|mn+1j2⋯mn+1jqS(xni⋯xnjq)dτ+⋯+∫t0mni1Mq∑j1⋯jqmnj1⋯mnjq−1|mn+1jq−mnjq|S(xni⋯xnjq)dτ+∫t0mni1Mq∑j1⋯jqmnj1⋯mnjq|S(xni⋯xnjq)−S(xn−1i⋯xn−1jq)|dτ. |
From (9), it holds
Thus,
N∑i=1|mn+1i−mni|≤ˉS(1+qNMTM)∫t0N∑j=1|mn+1j−mnj|dτ+MTLS(1+qNM)∫t0N∑i=1‖xnj−xn−1j‖dτ. |
Summarizing, we have
N∑i=1‖xn+1i−xni‖≤C1∫t0N∑i=1‖xn+1i−xni‖dτ+C2∫t0N∑i=1|mni−mn−1i|dτ;N∑i=1|mn+1i−mni|≤C3∫t0N∑i=1|mn+1i−mni|dτ+C3∫t0N∑i=1‖xni−xn−1i‖dτ. |
where
N∑i=1‖xn+pi−xni‖≤n+p−1∑k=nN∑i=1‖xk+1i−xki‖≤n+p−1∑k=nuk→n,p→+∞0. |
This proves that
Let us now deal with uniqueness. Suppose that
N∑i=1‖xi(t)−yi(t)‖+N∑i=1|mi(t)−pi(t)|≤AT∫t0(N∑i=1‖xi(τ)−yi(τ)‖+N∑i=1|mi(τ)−pi(τ)|)dτ. |
By Gronwall's lemma,
[1] |
A simulation study on the schooling mechanism in fish. Nippon Suisan Gakkaishi (1982) 48: 1081-1088. ![]() |
[2] |
Mean-field and graph limits for collective dynamics models with time-varying weights. Journal of Differential Equations (2021) 299: 65-110. ![]() |
[3] |
W. Braun and K. Hepp, The Vlasov dynamics and its fluctuations in the 1/N limit of interacting classical particles, Communications in Mathematical Physics, 56 (1977), 101–113, URL https://projecteuclid.org:443/euclid.cmp/1103901139. |
[4] |
F. Bullo, J. Cortés and S. Martínez, Distributed Control of Robotic Networks: A Mathematical Approach to Motion Coordination Algorithms, Princeton Series in Applied Mathematics. Princeton University Press, Princeton. |
[5] |
A Eulerian approach to the analysis of rendez-vous algorithms. IFAC Proceedings Volumes (2008) 41: 9039-9044. ![]() |
[6] |
Emergent behavior in flocks. IEEE Transactions on Automatic Control (2007) 52: 852-862. ![]() |
[7] |
P. Degond, Macroscopic limits of the Boltzmann equation: A review, Birkhäuser Boston, Boston, MA, 2004, 3–57. |
[8] |
Large scale dynamics of the persistent turning walker model of fish behavior. Journal of Statistical Physics (2008) 131: 989-1021. ![]() |
[9] |
Reaching a consensus. Journal of American Statistical Association (1974) 69: 118-121. ![]() |
[10] |
Vlasov equations. Functional Analysis and Its Applications (1979) 13: 115-123. ![]() |
[11] |
R. M. Dudley, Real Analysis and Probability, 2nd edition, Cambridge Studies in Advanced Mathematics, Cambridge University Press, 2002. |
[12] |
A formal theory of social power. Psychological Review (1956) 63: 181-194. ![]() |
[13] |
Collective behavior in animal groups: Theoretical models and empirical studies. Human Frontier Science Program Journal (2008) 2: 205-219. ![]() |
[14] |
From particle to kinetic and hydrodynamic descriptions of flocking. Kinetic and Related Models (2008) 1: 415-435. ![]() |
[15] |
R. Hegselmann, U. Krause et al., Opinion dynamics and bounded confidence models, analysis, and simulation, Journal of Artificial Societies and Social Simulation, 5. |
[16] |
Social dynamics models with time-varying influence. Mathematical Models and Methods in Applied Sciences (2019) 29: 681-716. ![]() |
[17] |
B. Piccoli and N. Pouradier Duteil, Control of collective dynamics with time-varying weights, in Recent Advances in Kinetic Equations and Applications (ed. F. Salvarani), Springer International Publishing, Cham, 2021,289–308. |
[18] |
Generalized Wasserstein distance and its application to transport equations with source. Archive for Rational Mechanics and Analysis (2014) 211: 335-358. ![]() |
[19] |
On properties of the generalized wasserstein distance. Archive for Rational Mechanics and Analysis (2016) 222: 1339-1365. ![]() |
[20] |
B. Piccoli and F. Rossi, Measure-theoretic models for crowd dynamics, Springer International Publishing, Cham, 2018,137–165. |
[21] |
B. Piccoli, F. Rossi and M. Tournus, A Wasserstein norm for signed measures, with application to nonlocal transport equation with source term, HAL, (2019), URL https://hal.archives-ouvertes.fr/hal-01665244, arXiv: 1910.05105. |
[22] |
Novel type of phase transition in a system of self-driven particles. Physical Review Letters (1995) 75: 1226-1229. ![]() |
[23] |
C. Villani, Optimal Transport: Old and New, Grundlehren der mathematischen Wissenschaften, Springer Berlin Heidelberg, 2008. |
1. | Benedetto Piccoli, Nastassia Pouradier Duteil, 2021, Chapter 12, 978-3-030-82945-2, 289, 10.1007/978-3-030-82946-9_12 | |
2. | Yurii Averboukh, Nonlocal Balance Equation: Representation and Approximation of Solution, 2024, 1040-7294, 10.1007/s10884-024-10373-8 | |
3. | Rico Berner, Thilo Gross, Christian Kuehn, Jürgen Kurths, Serhiy Yanchuk, Adaptive dynamical networks, 2023, 1031, 03701573, 1, 10.1016/j.physrep.2023.08.001 | |
4. | Marvin Lücke, Jobst Heitzig, Péter Koltai, Nora Molkenthin, Stefanie Winkelmann, Large population limits of Markov processes on random networks, 2023, 166, 03044149, 104220, 10.1016/j.spa.2023.09.007 | |
5. | Immanuel Ben-Porat, José A. Carrillo, Pierre-Emmanuel Jabin, The graph limit for a pairwise competition model, 2024, 413, 00220396, 329, 10.1016/j.jde.2024.08.069 | |
6. | Martin Gugat, Michael Herty, Chiara Segala, The turnpike property for mean-field optimal control problems, 2024, 35, 0956-7925, 733, 10.1017/S0956792524000044 | |
7. | Immanuel Ben-Porat, José A. Carrillo, Sondre T. Galtung, Mean field limit for one dimensional opinion dynamics with Coulomb interaction and time dependent weights, 2024, 240, 0362546X, 113462, 10.1016/j.na.2023.113462 | |
8. | Nathalie Ayi, Nastassia Pouradier Duteil, 2024, Chapter 3, 978-3-031-73422-9, 79, 10.1007/978-3-031-73423-6_3 | |
9. | Antonio Esposito, Georg Heinze, André Schlichting, Graph-to-local limit for the nonlocal interaction equation, 2025, 00217824, 103663, 10.1016/j.matpur.2025.103663 | |
10. | N. I. Pogodaev, M. V. Staritsyn, Optimal Control of Nonlocal Balance Equations in the Space of Nonnegative Measures, 2025, 66, 0037-4466, 576, 10.1134/S0037446625020223 |
Evolution of the positions for
Evolution of the weights for
Comparison of