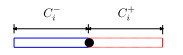
We study the modeling and simulation of gas pipeline networks, with a focus on fast numerical methods for the simulation of transient dynamics. The obtained mathematical model of the underlying network is represented by a system of nonlinear differential algebraic equations (DAEs). With our modeling approach, we reduce the number of algebraic constraints, which correspond to the (2,2) block in our semi-explicit DAE model, to the order of junction nodes in the network, where a junction node couples at least three pipelines. We can furthermore ensure that the (1,1) block of all system matrices including the Jacobian is block lower triangular by using a specific ordering of the pipes of the network. We then exploit this structure to propose an efficient preconditioner for the fast simulation of the network. We test our numerical methods on benchmark problems of (well-)known gas networks and the numerical results show the efficiency of our methods.
Citation: Yue Qiu, Sara Grundel, Martin Stoll, Peter Benner. Efficient numerical methods for gas network modeling and simulation[J]. Networks and Heterogeneous Media, 2020, 15(4): 653-679. doi: 10.3934/nhm.2020018
[1] | Yue Qiu, Sara Grundel, Martin Stoll, Peter Benner . Efficient numerical methods for gas network modeling and simulation. Networks and Heterogeneous Media, 2020, 15(4): 653-679. doi: 10.3934/nhm.2020018 |
[2] | Michael Herty . Modeling, simulation and optimization of gas networks with compressors. Networks and Heterogeneous Media, 2007, 2(1): 81-97. doi: 10.3934/nhm.2007.2.81 |
[3] | Mapundi K. Banda, Michael Herty, Axel Klar . Gas flow in pipeline networks. Networks and Heterogeneous Media, 2006, 1(1): 41-56. doi: 10.3934/nhm.2006.1.41 |
[4] | Mapundi K. Banda, Michael Herty, Axel Klar . Coupling conditions for gas networks governed by the isothermal Euler equations. Networks and Heterogeneous Media, 2006, 1(2): 295-314. doi: 10.3934/nhm.2006.1.295 |
[5] | Martin Gugat, Falk M. Hante, Markus Hirsch-Dick, Günter Leugering . Stationary states in gas networks. Networks and Heterogeneous Media, 2015, 10(2): 295-320. doi: 10.3934/nhm.2015.10.295 |
[6] | Fabian Rüffler, Volker Mehrmann, Falk M. Hante . Optimal model switching for gas flow in pipe networks. Networks and Heterogeneous Media, 2018, 13(4): 641-661. doi: 10.3934/nhm.2018029 |
[7] | Raul Borsche, Axel Klar, T. N. Ha Pham . Nonlinear flux-limited models for chemotaxis on networks. Networks and Heterogeneous Media, 2017, 12(3): 381-401. doi: 10.3934/nhm.2017017 |
[8] | Gunhild A. Reigstad . Numerical network models and entropy principles for isothermal junction flow. Networks and Heterogeneous Media, 2014, 9(1): 65-95. doi: 10.3934/nhm.2014.9.65 |
[9] | Michael Herty, Veronika Sachers . Adjoint calculus for optimization of gas networks. Networks and Heterogeneous Media, 2007, 2(4): 733-750. doi: 10.3934/nhm.2007.2.733 |
[10] | M. D. König, Stefano Battiston, M. Napoletano, F. Schweitzer . On algebraic graph theory and the dynamics of innovation networks. Networks and Heterogeneous Media, 2008, 3(2): 201-219. doi: 10.3934/nhm.2008.3.201 |
We study the modeling and simulation of gas pipeline networks, with a focus on fast numerical methods for the simulation of transient dynamics. The obtained mathematical model of the underlying network is represented by a system of nonlinear differential algebraic equations (DAEs). With our modeling approach, we reduce the number of algebraic constraints, which correspond to the (2,2) block in our semi-explicit DAE model, to the order of junction nodes in the network, where a junction node couples at least three pipelines. We can furthermore ensure that the (1,1) block of all system matrices including the Jacobian is block lower triangular by using a specific ordering of the pipes of the network. We then exploit this structure to propose an efficient preconditioner for the fast simulation of the network. We test our numerical methods on benchmark problems of (well-)known gas networks and the numerical results show the efficiency of our methods.
Natural gas is one of the most widely used energy sources in the world, as it is easily transportable, storable and usable to generate heat and electricity. Even though research on transient gas networks dates back to the 1980s [26,27], often only stationary solutions of the gas network are computed. This is also reasonable as the variation in a classically operated gas transportation networks enforces no need for a truly transient simulation. However, as we move from classical to renewable energy sources in which we may use the gas pipelines to deal with flexibility from volatile energy creation, the need for fast transient simulation will increase. In recent years, research on natural gas networks focuses on a variety of topics: transient simulations [26,27,39,14,17], optimization and control [37,45,18], time splitting schemes for solving the parabolic flow equations [44], discretization methods [8,28], and model sensitivity [6] to mention a few. It is obvious that efficient simulation techniques are needed both for design and for control.
The objective of this paper is to speed up the computations at the heart of each simulation. For that we make use of a discretization that respects the hyperbolic nature of the problem by using a finite volume method (FVM), as well as exploiting the network structure to create good properties for the computations. The use of the finite volume method is not entirely new in the literature [5], but typically comes with a Riemann solver, which by definition is an explicit time integration and therefore often slow. It is also not clear how to extend such a numerical scheme to a network with circles, which is straightforward in our approach. We start as it is standard for modeling gas transport in a pipeline by the one-dimensional isothermal Euler equation, which is a coupled partial differential equation (PDE). We introduce the necessary discrete variables for the pressure and the flux on each pipe and make sure to use as little algebraic equations as possible when considering a network of pipes. Other discretization schemes were studied in [3,23], but our setup is favorable for the speedup of the DAE simulation with an implicit time integration scheme. By further exploiting the structure of the system, we propose a preconditioner that enables fast solution of such a nonlinear equation using a preconditioned Krylov solver at each Newton iteration.
The structure of this paper is as follows. We introduce the incompressible isothermal Euler equation for gas dynamics modeling of each pipeline of the network in Section 2, where we also apply the finite volume method (FVM) to discretize the incompressible isothermal Euler equation. In Section 3, we introduce the details of gas network modeling starting from assembling all pipelines. This results in a set of nonlinear DAEs for the network model. We propose numerical algorithms to solve the resulting nonlinear DAE in Section 4 to simulate the gas network. We use benchmark problems from gas pipeline networks to show the efficiency and the advantage of our numerical algorithms in Section 5, and we draw conclusions in the last section.
In a typical gas transport network, the main components are pipelines (or pipes, for short). In this section, we will discuss the dynamics of gas transported along pipes. In Section 2.1, we discuss the isothermal Euler equation, which is the partial differential equation used for modeling transport in the pipe. Some standard simplifications lead to equation (4) and after some more assumptions, equation (5) can be derived, which is the basis for our simulations. In Section 2.2, equation (5) is discretized in space by a standard finite volume scheme leading to (7).
The dynamics of gas transported along pipes is described by the Euler equation, which represents the laws of mass conservation, momentum conservation, and energy conservation. In this paper, we assume that the temperature is constant throughout the gas network, leading to the isothermal Euler equations. Therefore, the energy equation can be neglected. This may seem unrealistic, but for onshore gas networks, in which the pipes are buried underground, the temperature along pipes does not vary much. This assumption greatly reduces the complexity of modeling and is widely used in the simulation of gas networks [19,22,13,17,11].
Consider the 1D isothermal Euler equation over the spatial domain
∂∂tρ=−∂∂xφ, | (1a) |
∂∂tφ=−∂∂xp−∂∂x(ρv2)−gρ∂∂xh−λ(φ)2dρv|v|, | (1b) |
p=γ(T)z(p,T)ρ. | (1c) |
Here,
By using the mass flow
∂∂tρ=−1a∂∂xq, | (2a) |
1a∂∂tq=−∂∂xp−1a2∂∂xq2ρ−gρ∂∂xh−λ(q)2da2q|q|ρ, | (2b) |
p=γ(T)z(p,T)ρ. | (2c) |
For the isothermal case, the temperature
1γ0∂∂tpz0(p)=−1a∂∂xq, | (3a) |
1a∂∂tq=−∂∂xp−γ0a2∂∂xq2z0(p)p⏟inertia term−gγ0pz0(p)∂∂xh⏟gravity term−λ(q)γ02da2z0(p)q|q|p⏟friction term. | (3b) |
For the inertia term, it is concluded in [19] that for realistic gas pipes
γ0a2∂∂xq2z0(p)p≈10−3∂∂xp. |
Therefore, the inertia term can be neglected and this neglecting greatly simplifies the model, which is standard in the study of gas networks [18,14,45]. In this paper, we also use this simplification. Meanwhile, we will often assume that the elevation of pipes is homogeneous. The gravity term in (3b) then vanishes. However, this term is easily treatable within our framework, which we will illustrate later in this section.
Now, we get the model that describes the dynamics of isothermal gas transported along homogeneous elevation pipes given by
∂∂tpz0(p)=−γ0a∂∂xq, | (4a) |
∂∂tq=−a∂∂xp−λ(q)γ02daz0(p)q|q|p. | (4b) |
The details of modeling the compressibility factor
In this paper, the dynamics of the gas transported along pipes are described by the 1D isothermal incompressible Euler equation (
∂∂tp+ca∂∂xq=0, | (5a) |
∂∂tq+a∂∂xp+cλ2daq|q|p=0. | (5b) |
For simplification of notation we introduce
{p=ps,at x=0,q=qd,at x=L. | (6) |
For the well-posedness and the regularity of the solution of the system (5)–(6), we refer to [9].
More advanced numerical schemes for hyperbolic PDEs, such as the total variation diminishing (TVD) method [40], or the discontinuous Galerkin method (DG) [24], could be implemented at the next step of our research to investigate more complicated dynamics of the gas networks. The scope of our paper is to develop a systematic numerical methodology for the fast simulation of the network dynamics while taking numerical accuracy into account. Thus, here we stick to this simple discretization scheme for the purpose of exposition.
To apply the FVM to discretize the PDE and the boundary condition, we integrate (5a)–(5b) over each control volume. The
After the FVM discretization, we get
[MpMq]⏟M[∂tp∂tq]=[0KpqKqp0]⏟K[pq]+right BC⏞[Bq0]⏟Bqqd+left BC⏞[0Bp]⏟Bpps+[0g(ps,p,q)], | (7) |
where the mass matrices
Mp=[h1+h22h2+h32⋱hn−2+hn−12hn−183hn−18],Mq=[3h18h18h1+h22h2+h32⋱hn−2+hn−12], |
and
Kpq=−c2[−1a11a1−1a21a2−1a21a2−1a31a3⋱⋱⋱−1an−31an−3−1an−21an−2−1an−21an−2−1an−1−1an−1], |
is an upper-triangular matrix with 3 diagonals and
Kqp=−12[a1a1−a2a2−a2a2−a3a3−a3a3−a4a4⋱⋱⋱−an−2an−2−an−1an−1], |
is a lower-triangular matrix with 3 diagonals. Meanwhile,
Bq=−c2[0⋮01an−11an−1],Bp=12[a1a10⋮0],g(ps,p,q)=−c4[h1λ1a1d1q1|q1|ps(h1λ1a1d1+h2λ2a2d2)q2|q2|p2(h2λ2a2d2+h3λ3a3d3)q3|q3|p3⋮(hn−2λn−2an−2dn−2+hn−1λn−1an−1dn−1)qn−1|qn−1|pn−1]. | (8) |
The vectors
p=[p2p3⋯pn]T,q=[q1q2⋯qn−1]T, |
represent the discretized analog of
Remark 1. The obtained model in (7) results from the discretization of the incompressible isothermal Euler equation of homogeneous elevation (5a)–(5b). However, we note that for the heterogeneous elevation case, the gravity term in (3b) is linear in the pressure
Remark 2. In this discretization, the cross-sectional area of the pipe does not have to be constant. This will be needed later on in the modeling of the network.
Note that we impose the boundary condition (6) by using the prescribed pressure at the inflow point and the given mass flow at the outflow point. However one can also impose a negative mass flow at the outflow point making it an inflow point, and for more complex topology of the network with more than one supply node, it can happen that the gas flows out at a so called inflow point. Computational results in the numerical experiments show this.
We have introduced the single pipeline model for the gas dynamics in Section 2. In this section, we introduce the gas pipeline network using a graph in which the pipelines are the edges (Section 3.1). We simplify this graph by smoothing. This procedure is explained in detail for readers that are not familiar with this concept in Section 3.2. The dynamics of gas transport through this network are described by combining (7) on each edge with the nodal conditions on each node (Section 3.3), which are then put together into one large differential-algebraic system resulting in equation (13) in Section 3.4. The smoothing of the graph gets rid of internal nodes, which are not junction nodes and in turn cuts down the number of algebraic constraints for the global network model assembly so that the complexity of the global network model is reduced.
Within this paper, we focus on passive networks to demonstrate how advanced numerical linear algebra can benefit the fast simulation of such a network. When we say passive network we mean a network that does not contain active elements, such as compressors, valves, etc. [12]. This simplification allows us to maintain a differential algebraic model without combinatorial aspects. For the modeling of the network with compressors and valves we refer to [3,20].
The abstract gas network is described by a directed graph
G=(E, N), | (9) |
where
Definition 3.1. The nodes inside a graph
Starting from the network, we use the so called smoothing of a graph to end up with a smoothed graph in which all interior nodes are junction nodes.
Denote by
An example network is shown in Figure 2. Here, nodes
By making use of the smoothed graph, the number of algebraic constraints is kept small, while the edges which represent several pipes become long. More details on the number of algebraic constraints will be presented in Proposition 1 at the end of this section. For each such long pipe
Remark 3. Our gas network is modeled by a directed graph
Remark 4. An edge in the smoothed graph can represent more than one pipe. This means that the cross-sectional area is not necessarily constant on one edge.
There are two types of constraints concerning the connection of edges, namely the pressure constraint, and the mass flow constraint. These two types of constraints represent the equality of the dynamic pressure and conservation of mass at junction nodes [21].
The so-called pressure nodal condition describes the pressure continuity among pipes connected at a joint junction node, which is given by
p(i)n(i)=p(j)s,if a node connects the incoming pipe i and the outgoing pipe j. | (10) |
The pressure nodal condition states that the pressure at the end of the outflow pipes should equal the pressure at the beginning of the inflow pipes that connect to the same junction node and ensures that there is only one pressure value at each node.
The second type of nodal condition i.e., the mass flow nodal condition, states the conservation of mass flow at the junction nodes, and it is given by
∑i∈Ikq(i)d=∑i∈Okq(i)1 for every node k, | (11) |
where
In the discretized model (7) describing the dynamics of gas transported along one single pipe, the variables
q(1)d+q(2)d=q(3)1,p(1)n1=p(2)n2, | (12) |
are the added algebraic constraints.
By using the single pipe model (7), we obtain the mathematical model for the network in Figures 2–3,
[M(1)M(2)M(3)00]⏟M∂∂t[u(1)u(2)u(3)q(1)dq(2)d]=[B(1)pB(2)p]⏟Bp[p(1)sp(2)s]+[00B(3)q00]⏟Bqq(3)d+[K(1)B(1)qK(2)B(2)qˉB(3)pK(3)e311e1e20]⏟K[u(1)u(2)u(3)q(1)dq(2)d]+[G1(u(1),p(1)s)G2(u(2),p(2)s)G3(u(3),ˉe3u(1))00]⏟g(∗). | (13) |
Here
ˉB3p=[0,0,…,,1,0,…,]⊗B3p,Gi(u(i),p(i)s)=[0g(p(i)s,p(i),q(i))]. | (14) |
The mass flow nodal condition is represented by the 4th block row in (13), and the pressure nodal condition is given by the 5th block row and also the (3, 1) block of
Note that the matrix
Although we need extra variables for both, the pressure and mass flow, to assemble a global network model, we only introduce extra mass flow variables explicitly while the extra pressure variables can be obtained via applying some pressure nodal conditions directly. This reduces the redundancy in the network modeling.
There is a degenerate case that a network has only one long pipe, i.e., this network has one supply node and one demand node, but no junction node. For such a degenerate network, which is equivalent to one pipe, we do not need to introduce extra variables since we already have the left and right boundary conditions. For a non-degenerate network, we have the following proposition.
Proposition 1. Suppose that the network
ne=na=ns+nj. |
Proof. As stated before, we only introduce extra variables for the mass flow of each supply and junction pipe, since the extra pressure variables are directly included at the process of network assembling. Then we have
ne=ns+nj, |
which is due to the fact that the mass flows at the demand pipes are already prescribed.
The algebraic constraints are obtained via applying nodal conditions at the junction nodes. Suppose that the junction node
na=∑in(i)in. |
On the other hand,
∑in(i)in=ns+nj, |
as incoming pipes are never demand pipes and the sum over all incoming pipes is the number of all supply and all junction pipes.
Proposition 1 states that the total number of extra variables is equal to the total number of algebraic constraints. This is very important for us to simulate the network model in the form of (13), which will be shown in the next section.
Based on the discretization of the one-dimensional isothermal Euler equation, using the smoothed graph, we are able to reduce the algebraic constraints to a very small number and obtain the nonlinear DAE system (13).
In this section, we introduce the direction following ordering method to order the edges of the smoothed graph to exploit the matrix structure of the DAE for fast computations. We integrate the DAE by a simple implicit Euler method, such that we have to solve a nonlinear system in each time step, which is done by Newton's method (Section 4.1). Looking closely at the matrix structure of the system that has to be solved in each Newton iteration (Section 4.2), we develop a special ordering of the nodes and edges in the graph such that the Jacobian is block lower triangular (Section 4.3), which is then illustrated in an example (Section 4.4). Last but not least, this linear system is solved with a preconditioned iterative method to achieve maximal speedup of the numerical computation (Section 4.5). The entire workflow is shown in Figure 7.
Here, we reuse the notation from (13) with simplifications. We obtain the general mathematical model,
M∂tx=Kx+Bu(t)+f(x,u(t)), | (15) |
where the mass matrix
Mxk−xk−1τ=Kxk+Buk+f(xk,uk), |
i.e., we need to solve the following nonlinear system of equations,
F(x)=(M−τK)x−τf(x,uk)−Mxk−1−τBuk=0, | (16) |
at each time step
Algorithm 1: Newton's method to solve (16) |
1: Input: maximal number of Newton steps 2: 3: while 4: Compute the Jacobian matrix 5: Solve 6: 7: end while 8: Output: solution |
The biggest challenge for Algorithm 1 is to solve the linear system in line
The Jacobian matrix is given by
DF(x)=(M−τK)+τ∂∂xf(x,u), | (17) |
where the matrices
M=[ˉM0],K=[K11K12K21K22]. | (18) |
Here
∂∂xf=[Df000], | (19) |
since the nonlinear term only acts on the differential part of the DAE (13).
In the following, we will present a concept that allows us to order the nodes and edges of the graph
Definition 4.1. A directed acyclic graph (DAG) is a finite, directed graph, which has no directed cycles, meaning there is no vertex from which we can start a path along directed edges that ends up at that vertex again.
Lemma 4.2. Given a graph with directed and undirected edges, such that the directed edges do not create cycles, we can always direct the undirected edges such that the resulting graph is a directed acyclic graph (DAG).
Proof. Take a graph satisfying the assumptions and remove all undirected edges. This results in a graph that may not be connected. However, it is a DAG so that we can order the nodes in a topological ordering [2]. Applying such a topological ordering to the original graph, direct the undirected edges according to that topological ordering, which induces another DAG.
This means, even by fixing the direction of the supply and demand pipes, we can redirect all the other edges in our graph
Lemma 4.3. Given a DAG, we can order the edges in such a way that at every node all incoming edges have a lower order than all the outgoing edges, or it does not have an incoming edge. We call this ordering direction following (DF) ordering.
Proof. Since we have a DAG, there exists a topological ordering of the nodes. This means: if an edge goes from node
The network in Figure 4a is a smoothed graph that represents a gas network. Only the directions of the supply and demand pipes are fixed. We start ordering the nodes of the graph from the supply nodes, and end up with the demand nodes, which gives a topological ordering of the nodes. Now, we can plot the graph along a line as in Figure 4b. The directions of the undirected edges of the graph are picked up by pointing away from lower order nodes to higher order nodes, which induces a DAG. This in turn leads to an ordering of the edges, which is the index above each edge in Figure 4b. Note that the DF ordering is not unique.
Definition 4.4 A smoothed direction following (SDF) gas network graph is a directed acyclic graph, whose boundary nodes are supply or demand nodes, directions are away from supply nodes and towards demand nodes. All edges are sorted with the DF ordering and there are no nodes in the graph that connect exactly two edges.
From now on, we assume that our modeling is such that
Proposition 2. Given a SDF gas network graph, we can construct the DAE system in such a way that
Proof. The block of
fi=Gi=[0g(pin,p(i),q(i))], |
where the structure of
pin={ps,when the i-th pipe is a supply pipe,p(j)out,when pipe j is an incoming pipe of the node where pipe i is outgoing. |
By the selected ordering, we always have
[0000g(pin,p(i),q(i))∂p(i)g(pin,p(i),q(i))∂q(i)], |
which is again block lower triangular and in particular, with an easy structure of the diagonal blocks, since
Similar to Proposition 2, we can also show that the
Proposition 3. Given an SDF gas network graph,
Proof. For the
If we partition the Jacobian matrix (17) by a 2-by-2 block structure as in (18), then we have the following theorem to illustrate the structure of the
Theorem 4.5. Given an SDF gas network graph, we are able to model the system in such a way, that the
Proof. According to (17)–(19), the
ˉM−τK11+τDf. |
According to Proposition 2 and Proposition 3,
Next, we use a benchmark network from [34], given by Figure 5, to show the structure of the Jacobian matrix
Figure 6 shows that the Jacobian matrix
The specific structure of the Jacobian matrix can be exploited to solve the Jacobian system fast for the simulation of the gas network. Recall that in order to simulate the discretized gas network model, we need to apply Algorithm 1, where we need to solve a Jacobian system at each Newton iteration for each time step
DF(x)=[DF11DF12DF21DF22]. |
Note that the Jacobian matrix
We can compute a block LU factorization by
DF(x)=[DF11DF21S][ID−1F11DF12I]. | (20) |
Here
P=[DF11DF21S], | (21) |
to solve the Jacobian system using a preconditioned Krylov solver. Associated with the block LU factorization (20), we can immediately see that the preconditioned spectrum satisfies
At each iteration of the Krylov solver, we need to solve the system
[DF11DF21S][y1y2]=[r1r2], |
which can be solved easily since
P1=[D1F11D1F21S1], | (22) |
where
Next, we show the performance of the DF ordering for the Schur complement
with DF | without DF | ||
20 | 2.01e+05 | 8.75 | |
10 | 3.97e+05 | 19.14 | |
5 | 7.91e+05 | 41.75 | |
2.5 | 1.58e+06 | 87.77 |
We have noticed that for medium problem sizes, the advantage of computing
Since
By applying the preconditioner
In this section, we report the performance of our numerical algorithms for the simulation of gas networks. We apply our numerical algorithms to the benchmark problems of several gas networks given in [13,16,15,14] to show the performance of our methods. All numerical experiments are performed in MATLAB 2017a on a desktop with Intel(R) Core(TM)2 Quad CPU Q8400 of 2.66GHz, 8 GB memory and the Linux 4.9.0-6-amd64 kernel.
In this section, we compare the performance of the finite volume method (FVM) with that of the finite difference method (FDM) for the discretization of the gas networks. We apply both the FVM and FDM to a pipeline network illustrated in Figure 8. Parameter settings for this pipeline network are given in [14].
We discretize the pipeline network using FVM and FDM with different mesh sizes, and the discretized pipeline networks result in ordinary differential equations (ODEs) since there is no algebraic constraint. We simulate the ODE systems using the routine ode15s in MATLAB over the time horizon
Figure 9a shows the number of time steps that ode15s uses to simulate the ODEs given by FVM and FDM discretizations over the time horizon
Next, we use another network to show that with the same mesh, the model given by the FVM discretization gives more accurate results than the FDM discretization. The test network is given in Figure 10, where the network parameters are given in [16]. We use the FVM and FDM methods to discretize the network in Figure 10 and apply the computational method depicted in Figure 7. We choose different mesh sizes for the discretization, and fix the step size for the time discretization to be one second, i.e.,
The mass flow at node 57 computed by using different discretized DAE models (16) is plotted in Figure 11a, showing similar dynamical behavior of the different models. However, when we look at the dynamics of the mass flow during the first 5 seconds, we can see quite a big difference in Figure 11b. With the mesh refinement, the solutions of the model given by both the FVM and the FDM discretizations converge. Moreover, we can infer that we can use a bigger mesh size for the FVM discretization than for the FDM discretization to get the same accuracy.
The computational results given by Figure 9 and Figure 11 show that when we use the same mesh size to discretize the network, the model given by the FVM discretization is more accurate than the model by the FDM discretization. To get the same model accuracy, we can use a bigger mesh size to discretize the network by FVM than that by FDM. This in turn yields a smaller model given by the FVM discretization than the model given by the FDM discretization. This in turn means that the FVM discretized model is easier to solve than the FDM discretized model.
We also plot the condition number of the Jacobian matrix (
Newton iter. | 1 | 2 | 3 | 4 |
FVM | 1.56e+07 | 1.57e+07 | 1.57e+07 | 1.57e+07 |
FDM | 1.24e+08 | 1.25e+08 | 1.25e+08 | 1.25e+08 |
Computational results in Figure 9 and Table 2 show that the FVM offers a big advantage over the FDM. When using the same mesh size for discretization, FVM gives a more accurate model than the FDM discretization. Moreover, the Jacobian matrix from the FVM discretized model has a better condition number than the Jacobian matrix from the FDM discretized model, which makes it easier to simulate the FVM discretized model. To get the same model accuracy, the size of the FVM discretized model is smaller than the size of the FDM discretized model, and it is therefore computationally cheap. For the comparison of the finite element method (FEM) with FDM for the gas network simulation, we refer to an early study in [28], where the authors preferred FDM due to the comparable accuracy with FEM and less computational time.
The basis of our modeling is a directed graph, with the implicit assumption that the gas flow follows that direction. However, the flow direction may change due to the change of operation conditions of the gas network. In this part, we show that the direction is just a theoretical construction but that the gas is allowed to flow in either direction meaning that the mass flow can be negative and does not influence the performance of our methods. The change of the flow direction does not change the mathematical formulation of algebraic constraints. Therefore, the structure of the system stays unchanged with respect to the change of the flow direction. This means that we do not require the foreknowledge of the flow direction.
Note that the nonlinear term
First, we test two different cases, which corresponds to two different flow direction profiles of the network, cf. Figure 2. Case 1 corresponds to
Figure 12 shows that the mass flow at both supply nodes approaches the steady state after oscillation for a short while, and both input mass flows have a positive sign. This represents that both supply nodes inject gas flow into the network to supply gas to the demand node 6. After changing the operation condition of the network, e.g., changing the pressure at supply nodes, the mass flow is redistributed as shown in Figure 13. The mass flow at supply node
We also apply two different cases to a more complicated network given in Figure 10 to test the robustness of our methods. Case 1 corresponds to
The simulation results in Figure 14 show that the flow direction at pipe
As introduced in the previous section, the biggest challenge for applying Algorithm 1 to simulate a gas network lies in the effort spent to solve the linear system at each Newton iteration. For large-scale networks, we need smaller mesh sizes to discretize such networks and this results in larger sizes of the DAEs. Therefore, we need to employ iterative solvers to compute the solution of such a large-scale linear system at each Newton iteration, while preconditioning is essential to accelerate the convergence of such iterative solvers. In this part, we study the performance of the preconditioner (22).
We test the performance of the preconditioner for the network in Figure 5 using different mesh sizes for the FVM discretization. At each Newton (outer) iteration, we solve a linear system by applying an (inner) Krylov solver, e.g., the IDR(s) solver [41,36], and this is called Newton-Krylov method. Note that the Newton-Krylov method is an inexact Newton method, and at each Newton iteration, we apply the IDR(s) method to solve the linear system up to an accuracy
‖F(xm)+DF(xm)(x−xm)‖≤εtol‖F(xm)‖, |
where
The computational results of the nonlinear residual
The computational results in Figure 16a show that the total number of IDR(
We have already observed that the performance of our modeling is robust and convergence of the preconditioned Krylov solver happens quickly. Next, we report the computational time for computing the preconditioner
IDR( |
backslash | |||
40 | 1.03e+05 | 0.25 | 0.13 | |
20 | 2.01e+05 | 0.52 | 0.36 | |
10 | 3.97e+05 | 1.06 | 1.18 | |
5 | 7.91e+05 | 2.13 | 1054.62 | |
2.5 | 1.58e+06 | 4.34 | - |
The computational results in Table 3 show the advantage of our preconditioner in solving the large-scale Jacobian system over the direct solver. The time to solve the preconditioned system using the IDR(4) solver scales linearly with the system size, and is much smaller than the time to apply the direct solver when the mesh sizes are smaller than
In this paper, we studied the modeling and simulation of gas networks. We applied the finite volume method (FVM) to discretize the incompressible isothermal Euler equation, and compared it with the finite difference method (FDM). Numerical results show the advantage of the FVM over the FDM. To model gas networks, we introduced the smoothed gas network concept, which represents the topology of the network interconnection and reduces the size of the algebraic constraints of the resulting system of differential algebraic equations (DAEs) compared with current research. To simulate such a DAE system, we proposed the direction following (DF) ordering of the edges of the smoothed network. Through such a DF ordering, we exploited the structure of the system matrix and proposed an efficient preconditioner to solve the DAE. Numerical results show the advantage of our algorithms.
[1] | N. Banagaaya, S. Grundel and P. Benner, Index-aware MOR for gas transport networks with many supply inputs, in IUTAM Symposium on Model Order Reduction of Coupled Systems (eds. J. Fehr and B. Haasdonk), Springer International Publishing, Cham, 2020,191–207. |
[2] |
J. Bang-Jensen and G. Z. Gutin, Digraphs: Theory, Algorithms and Applications, Springer-Verlag, London, 2008. doi: 10.1007/978-1-84800-998-1
![]() |
[3] | P. Benner, S. Grundel, C. Himpe, C. Huck, T. Streubel and C. Tischendorf, Gas network benchmark models, in Differential-Algebraic Equations Forum, Springer, Berlin, Heidelberg, 2018. |
[4] |
Numerical solution of saddle point problems. Acta Numer. (2005) 14: 1-137. ![]() |
[5] |
Finite volume methods for multi-component Euler equations with source terms. Comput. Fluids (2017) 156: 113-134. ![]() |
[6] |
Sensitivity of pipeline gas flow model to the selection of the equation of state. Chem. Eng. Res. Des. (2009) 87: 1596-1603. ![]() |
[7] |
Inexact Newton methods. SIAM J. Numer. Anal. (1982) 19: 400-408. ![]() |
[8] |
H. Egger, A robust conservative mixed finite element method for isentropic compressible flow on pipe networks, SIAM J. Sci. Comput., 40 (2018), A108–A129. doi: 10.1137/16M1094373
![]() |
[9] |
H. Egger, T. Kugler and N. Strogies, Parameter identification in a semilinear hyperbolic system, Inverse Probl., 33 (2017), 055022. doi: 10.1088/1361-6420/aa648c
![]() |
[10] |
(2014) Finite Elements and Fast Iterative Solvers. New York: Oxford University Press. ![]() |
[11] | A. Fügenschuh, et al., Physical and technical fundamentals of gas networks, in Evaluating Gas Network Capacities |
[12] |
A joint model of probabilistic/robust constraints for gas transport management in stationary networks. Comput. Manag. Sci. (2017) 14: 443-460. ![]() |
[13] |
S. Grundel, N. Hornung, B. Klaassen, P. Benner and T. Clees, Computing surrogates for gas network simulation using model order reduction, in Surrogate-Based Modeling and Optimization doi: 10.1007/978-1-4614-7551-4_9
![]() |
[14] | S. Grundel, N. Hornung and S. Roggendorf, Numerical aspects of model order reduction for gas transportation networks, in Simulation-Driven Modeling and Optimization, Springer Proceedings in Mathematics & Statistics, 153, 2016, 1–28. |
[15] |
S. Grundel and L. Jansen, Efficient simulation of transient gas networks using IMEX integration schemes and MOR methods, in 2015 54th IEEE Conference on Decision and Control (CDC), 2015, 4579–4584. doi: 10.1109/CDC.2015.7402934
![]() |
[16] |
S. Grundel, L. Jansen, N. Hornung, T. Clees, C. Tischendorf and P. Benner, Model order reduction of differential algebraic equations arising from the simulation of gas transport networks, in Progress in Differential-Algebraic Equations, Differential-Algebraic Equations Forum, Springer Berlin Heidelberg, 2014,183–205. doi: 10.1007/978-3-642-34928-7_2
![]() |
[17] |
Stationary states in gas networks. Netw. Heterog. Media (2015) 10: 295-320. ![]() |
[18] | F. M. Hante, G. Leugering, A. Martin, L. Schewe and M. Schmidt, Challenges in Optimal Control Problems for Gas and Fluid Flow in Networks of Pipes and Canals: From Modeling to Industrial Applications, Springer Verlag, Singapore, 2017, 77–122. |
[19] | Modeling and simulation of a gas distribution pipeline network. Appl. Math. Model. (2009) 33: 1584-1600. |
[20] |
Modeling, simulation and optimization of gas networks with compressors. Netw. Heterog. Media (2007) 2: 81-97. ![]() |
[21] |
Coupling conditions for networked systems of Euler equations. SIAM J. Sci. Comput. (2008) 30: 1596-1612. ![]() |
[22] |
A new model for gas flow in pipe networks. Math. Methods Appl. Sci. (2010) 33: 845-855. ![]() |
[23] |
A topology based discretization of PDAEs describing water transportation networks. Proc. Appl. Math. Mech. (2014) 14: 923-924. ![]() |
[24] |
An analysis of the discontinuous Galerkin method for a scalar hyperbolic equation. Math. Comp. (1986) 46: 1-26. ![]() |
[25] |
C. Kelley, Solving Nonlinear Equations with Newton's Method, Society for Industrial and Applied Mathematics, Philadelphia, 2003. doi: 10.1137/1.9780898718898
![]() |
[26] |
Simulation of transient gas flows in networks. Internat. J. Numer. Methods Fluids (1984) 4: 13-24. ![]() |
[27] | A. Osiadacz, Simulation and Analysis of Gas Networks, Gulf Publishing, Houston, TX, 1987. |
[28] |
A comparison of a finite element method and a finite difference method for transient simulation of a gas pipeline. Appl. Math. Model. (1989) 13: 79-85. ![]() |
[29] | On the development of parameter-robust preconditioners and commutator arguments for solving Stokes control problems. Electron. Trans. Numer. Anal. (2015) 44: 53-72. |
[30] |
Natural preconditioning and iterative methods for saddle point systems. SIAM Rev. (2015) 57: 71-91. ![]() |
[31] |
M. Porcelli, V. Simoncini and M. Tani, Preconditioning of active-set Newton methods for PDE-constrained optimal control problems, SIAM J. Sci. Comput., 37 (2015), S472–S502. doi: 10.1137/140975711
![]() |
[32] | Y. Qiu, Preconditioning Optimal Flow Control Problems Using Multilevel Sequentially Semiseparable Matrix Computations, Ph.D thesis, Delft Institute of Applied Mathematics, Delft University of Technology, 2015. |
[33] | T. Rees, Preconditioning Iterative Methods for PDE-Constrained Optimization, Ph.D thesis, University of Oxford, 2010. |
[34] | S. Roggendorf, Model Order Reduction for Linearized Systems Arising from the Simulation of Gas Transportation Networks, Master's thesis, Rheinischen Friedrich-Wilhelms-Universität Bonn, Germany, 2015. |
[35] |
Y. Saad, Iterative Methods for Sparse Linear Systems, Society for Industrial and Applied Mathematics, Philadelphia, 2003. doi: 10.1137/1.9780898718003
![]() |
[36] |
IDR(s): A family of simple and fast algorithms for solving large nonsymmetric systems of linear equations. SIAM J. Sci. Comput. (2008) 31: 1035-1062. ![]() |
[37] |
On PDE solution in transient optimization of gas networks. J. Comput. Appl. Math. (2007) 203: 345-361. ![]() |
[38] |
M. Stoll and T. Breiten, A low-rank in time approach to PDE-constrained optimization, SIAM J. Sci. Comput., 37 (2015), B1–B29. doi: 10.1137/130926365
![]() |
[39] |
Transient analysis of gas pipeline network. Chem. Eng. J. (1998) 69: 47-52. ![]() |
[40] |
Centred TVD schemes for hyperbolic conservation laws. IMA J. Numer. Anal. (2000) 20: 47-79. ![]() |
[41] |
M. B. van Gijzen and P. Sonneveld, Algorithm 913: An elegant IDR(s) variant that efficiently exploits biorthogonality properties, ACM Trans. Math. Software, 38 (2011), 5: 1–5: 19. doi: 10.1145/2049662.2049667
![]() |
[42] |
Preconditioning. Acta Numer. (2015) 24: 329-376. ![]() |
[43] |
M. Wathen, C. Greif and D. Schötzau, Preconditioners for mixed finite element discretizations of incompressible MHD equations, SIAM J. Sci. Comput., 39 (2017), A2993–A3013. doi: 10.1137/16M1098991
![]() |
[44] |
Simulation of transients in natural gas pipelines using hybrid TVD schemes. Internat. J. Numer. Methods Fluids (2000) 32: 407-437. ![]() |
[45] |
A. Zlotnik, M. Chertkov and S. Backhaus, Optimal control of transient flow in natural gas networks, in 2015 54th IEEE Conference on Decision and Control (CDC), 2015, 4563–4570. doi: 10.1109/TCNS.2014.2367360
![]() |
1. | Zlatinka Dimitrova, Flows of Substances in Networks and Network Channels: Selected Results and Applications, 2022, 24, 1099-4300, 1485, 10.3390/e24101485 | |
2. | Björn Liljegren-Sailer, Nicole Marheineke, On Snapshot-Based Model Reduction Under Compatibility Conditions for a Nonlinear Flow Problem on Networks, 2022, 92, 0885-7474, 10.1007/s10915-022-01901-z | |
3. | Jianguo Huang, Yue Qiu, Resolution invariant deep operator network for PDEs with complex geometries, 2025, 522, 00219991, 113601, 10.1016/j.jcp.2024.113601 |
Algorithm 1: Newton's method to solve (16) |
1: Input: maximal number of Newton steps 2: 3: while 4: Compute the Jacobian matrix 5: Solve 6: 7: end while 8: Output: solution |
with DF | without DF | ||
20 | 2.01e+05 | 8.75 | |
10 | 3.97e+05 | 19.14 | |
5 | 7.91e+05 | 41.75 | |
2.5 | 1.58e+06 | 87.77 |
Newton iter. | 1 | 2 | 3 | 4 |
FVM | 1.56e+07 | 1.57e+07 | 1.57e+07 | 1.57e+07 |
FDM | 1.24e+08 | 1.25e+08 | 1.25e+08 | 1.25e+08 |
IDR( |
backslash | |||
40 | 1.03e+05 | 0.25 | 0.13 | |
20 | 2.01e+05 | 0.52 | 0.36 | |
10 | 3.97e+05 | 1.06 | 1.18 | |
5 | 7.91e+05 | 2.13 | 1054.62 | |
2.5 | 1.58e+06 | 4.34 | - |
Algorithm 1: Newton's method to solve (16) |
1: Input: maximal number of Newton steps 2: 3: while 4: Compute the Jacobian matrix 5: Solve 6: 7: end while 8: Output: solution |
with DF | without DF | ||
20 | 2.01e+05 | 8.75 | |
10 | 3.97e+05 | 19.14 | |
5 | 7.91e+05 | 41.75 | |
2.5 | 1.58e+06 | 87.77 |
Newton iter. | 1 | 2 | 3 | 4 |
FVM | 1.56e+07 | 1.57e+07 | 1.57e+07 | 1.57e+07 |
FDM | 1.24e+08 | 1.25e+08 | 1.25e+08 | 1.25e+08 |
IDR( |
backslash | |||
40 | 1.03e+05 | 0.25 | 0.13 | |
20 | 2.01e+05 | 0.52 | 0.36 | |
10 | 3.97e+05 | 1.06 | 1.18 | |
5 | 7.91e+05 | 2.13 | 1054.62 | |
2.5 | 1.58e+06 | 4.34 | - |