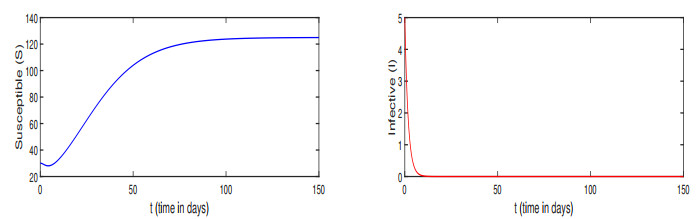
A delay differential equation model of an infectious disease is considered and analyzed. In this model, the impact of information due to the presence of infection is considered explicitly. As information propagation is dependent on the prevalence of the disease, the delay in reporting the prevalence is an important factor. Further, the time lag in waning immunity related to protective measures (such as vaccination, self-protection, responsive behaviour etc.) is also accounted. Qualitative analysis of the equilibrium points of the model is executed and it is observed that when the basic reproduction number is less unity, the local stability of the disease free equilibrium (DFE) depends on the rate of immunity loss as well as on the time delay for the waning of immunity. If the delay in immunity loss is less than a threshold quantity, the DFE is stable, whereas, it loses its stability when the delay parameter crosses the threshold value. When, the basic reproduction number is greater than unity, the unique endemic equilibrium point is found locally stable irrespective of the delay effect under certain parametric conditions. Further, we have analyzed the model system for different scenarios of both delays (i.e., no delay, only one delay, and both delay present). Due to these delays, oscillatory nature of the population is obtained with the help of Hopf bifurcation analysis in each scenario. Moreover, at two different time delays (delay in information's propagation), the emergence of multiple stability switches is investigated for the model system which is termed as Hopf-Hopf (double) bifurcation. Also, the global stability of the endemic equilibrium point is established under some parametric conditions by constructing a suitable Lyapunov function irrespective of time lags. In order to support and explore qualitative results, exhaustive numerical experimentations are carried out which lead to important biological insights and also, these results are compared with existing results.
Citation: Anuj Kumar, Yasuhiro Takeuchi, Prashant K Srivastava. Stability switches, periodic oscillations and global stability in an infectious disease model with multiple time delays[J]. Mathematical Biosciences and Engineering, 2023, 20(6): 11000-11032. doi: 10.3934/mbe.2023487
[1] | Shunyi Li . Hopf bifurcation, stability switches and chaos in a prey-predator system with three stage structure and two time delays. Mathematical Biosciences and Engineering, 2019, 16(6): 6934-6961. doi: 10.3934/mbe.2019348 |
[2] | Jinhu Xu, Yicang Zhou . Bifurcation analysis of HIV-1 infection model with cell-to-cell transmission and immune response delay. Mathematical Biosciences and Engineering, 2016, 13(2): 343-367. doi: 10.3934/mbe.2015006 |
[3] | Huan Kong, Guohong Zhang, Kaifa Wang . Stability and Hopf bifurcation in a virus model with self-proliferation and delayed activation of immune cells. Mathematical Biosciences and Engineering, 2020, 17(5): 4384-4405. doi: 10.3934/mbe.2020242 |
[4] | Sarita Bugalia, Jai Prakash Tripathi, Hao Wang . Mathematical modeling of intervention and low medical resource availability with delays: Applications to COVID-19 outbreaks in Spain and Italy. Mathematical Biosciences and Engineering, 2021, 18(5): 5865-5920. doi: 10.3934/mbe.2021295 |
[5] | Yuan Ma, Yunxian Dai . Stability and Hopf bifurcation analysis of a fractional-order ring-hub structure neural network with delays under parameters delay feedback control. Mathematical Biosciences and Engineering, 2023, 20(11): 20093-20115. doi: 10.3934/mbe.2023890 |
[6] | Jiawei Deng, Ping Jiang, Hongying Shu . Viral infection dynamics with mitosis, intracellular delays and immune response. Mathematical Biosciences and Engineering, 2023, 20(2): 2937-2963. doi: 10.3934/mbe.2023139 |
[7] | Yu Yang, Gang Huang, Yueping Dong . Stability and Hopf bifurcation of an HIV infection model with two time delays. Mathematical Biosciences and Engineering, 2023, 20(2): 1938-1959. doi: 10.3934/mbe.2023089 |
[8] | LanJiang Luo, Haihong Liu, Fang Yan . Dynamic behavior of P53-Mdm2-Wip1 gene regulatory network under the influence of time delay and noise. Mathematical Biosciences and Engineering, 2023, 20(2): 2321-2347. doi: 10.3934/mbe.2023109 |
[9] | Bruno Buonomo, Marianna Cerasuolo . The effect of time delay in plant--pathogen interactions with host demography. Mathematical Biosciences and Engineering, 2015, 12(3): 473-490. doi: 10.3934/mbe.2015.12.473 |
[10] | Zenab Alrikaby, Xia Liu, Tonghua Zhang, Federico Frascoli . Stability and Hopf bifurcation analysis for a Lac operon model with nonlinear degradation rate and time delay. Mathematical Biosciences and Engineering, 2019, 16(4): 1729-1749. doi: 10.3934/mbe.2019083 |
A delay differential equation model of an infectious disease is considered and analyzed. In this model, the impact of information due to the presence of infection is considered explicitly. As information propagation is dependent on the prevalence of the disease, the delay in reporting the prevalence is an important factor. Further, the time lag in waning immunity related to protective measures (such as vaccination, self-protection, responsive behaviour etc.) is also accounted. Qualitative analysis of the equilibrium points of the model is executed and it is observed that when the basic reproduction number is less unity, the local stability of the disease free equilibrium (DFE) depends on the rate of immunity loss as well as on the time delay for the waning of immunity. If the delay in immunity loss is less than a threshold quantity, the DFE is stable, whereas, it loses its stability when the delay parameter crosses the threshold value. When, the basic reproduction number is greater than unity, the unique endemic equilibrium point is found locally stable irrespective of the delay effect under certain parametric conditions. Further, we have analyzed the model system for different scenarios of both delays (i.e., no delay, only one delay, and both delay present). Due to these delays, oscillatory nature of the population is obtained with the help of Hopf bifurcation analysis in each scenario. Moreover, at two different time delays (delay in information's propagation), the emergence of multiple stability switches is investigated for the model system which is termed as Hopf-Hopf (double) bifurcation. Also, the global stability of the endemic equilibrium point is established under some parametric conditions by constructing a suitable Lyapunov function irrespective of time lags. In order to support and explore qualitative results, exhaustive numerical experimentations are carried out which lead to important biological insights and also, these results are compared with existing results.
Mathematical models have been found a successful tool to understand the underlying dynamics of infectious diseases. This eventually helps in controlling epidemic threats and related consequences so that effective and applicable control measures can be developed [1,2,3]. A large number of mathematical models, after the fundamental work of Kermack and McKendrick [4], have been formulated and analyzed to comprehend the dynamics of disease transmission and progression [1,2,3,5,6,7,8,9]. It is important to mention here that in the last few years, various infectious diseases such as SARS, influenza, Ebola, Zika and most recent COVID-19 have posed serious challenges and socio-economic consequences worldwide. Therefore, researchers are attracted to study various control strategies so that limited resources can be best utilized. For that various control interventions: pharmaceutical and non-pharmaceutical and their suitable combination are an important area of study [5,8,10,11,12]. Education, awareness, information, isolation, social distancing etc. are considered as the non-pharmaceutical controls [10,11,13,14,15,16].
Nowadays, during and after the outbreaks of diseases, it has been observed that individuals change their behaviour due to the effect of information generated via global connectivity of social media, educational campaigns etc. which eventually alters the progression of infectious diseases [17,18,19,20,21,22]. Therefore, researchers have intended to study the effect of behaviour influencing factors induced by information on the spread of infectious diseases. Researchers have studied the effect of information on model dynamics, using it either on the force of infection or by making a subclass of aware population [14,20,21,23,24,25,26,27,28,29].
The time lags/delays are inherent in natural, biological as well as man made systems and they play a crucial role in the disease dynamics as well. Moreover, the effect of time delays, appeared in various interactions during the disease progression, has been well studied and explored in literature. Delay differential equation models have been used to explain different kinds of time lags in biological system for example delay in infection (disease transmission), maturation, waning immunity etc. [30,31,32,33,34,35,36,37]. These delay models show rich and complex dynamics leading to different kind of bifurcations, instability of equilibria, periodic solutions etc. [33,35,36,38,39,40,41,42]. However, study of multiple delay models is challenging as it increases the complexity of the system and analysis.
In 1999, an epidemic model which accounts for the effect of maturation delay in the growth of population was proposed and analyzed by Cooke et al. [30]. For R0>1, authors found that the disease will remain endemic in the population for all the time, either at the equilibrium value or show oscillations around it. Further, in 2005, Greenhalgh et al. proposed an SIRS model with vaccination effect on the susceptible population and the effect of delay on waning the vaccine-induced immunity [31]. Analytically, the existence of Hopf bifurcation was established by the authors. In 2005, Kyrychko et al. proposed and analyzed a delay differential equation model in which they used a nonlinear force of infection along with delay effect in loss of vaccine immunity [34]. When the time lag in loss of vaccine immunity crosses a threshold quantity, the global stability of the infected equilibrium was established by authors. In 2008, a delayed SIRS model along with temporary immunity was formulated and explored by Wen et al. and the global stability of the infected equilibrium was shown by constructing a Lyapunov function [37]. Further, in 2010, various epidemiological models such as SIR, SIS, SEIR and SEI along with time delay effect and general force of infection term were proposed by Huang et al. and they studied their global stability properties under time delay effect [32].
There are some recent developments in this direction that are related to our study and some of them are discussed in the following [43,44,45,46]. In 2022, Mezouaghi and their coworkers proposed a delayed epidemic model in which they included a separate class of protected individuals [45]. They have established the global stability of disease free and endemic equilibrium points by constructing Lyapunov functions. Further, Yang and others formulated an epidemic model which quantifies a convex type of force of infection and a delay effect in recovery rate [46]. They proved the global exponential stability of endemic equilibrium point along with the existence of Hopf bifurcation and their stability and direction. Recently, Lv et al. developed an SVIR model which accounts for the effect of two delays related to COVID-19 booster vaccination and failure of antibody (waning immunity) [43]. The authors have explored the dynamic properties of the model consisting of local and Hopf bifurcation analysis. They estimated the model parameters from the real data set and numerically validated the obtained analytical results.
In 2017, Kumar et al. [11] have considered the growth of information as function of infective at instantaneous time. However, in practice, reporting the disease may not be possible instantaneously and hence, there is always a time lag in the report by media and health agencies after the outbreak. Thus, the dissemination/propagation of information about the disease prevalence may be affected by this time lag. So this delay in reporting these infected individuals may have an impact on generated information. In order to quantify this factor, Kumar et al. [47] have considered this delay effect in their model and observed important insights due to delay in reporting. As we are aware that vaccination and recovery provide a certain immunity to fight with infection but this immunity may not have long-term effects and hence, waning takes place. Therefore, the waning immunity is one of the crucial factors which leads to the high reoccurrence of infectious diseases such as measles, chickenpox etc. because recovered or immunized individuals will again join the susceptible class [48,49]. Moreover, it is very difficult to determine the duration of protection level through any kind of immunity either vaccine-induced or natural and it may vary from the case to case [50]. Therefore, time lag in waning the immunity becomes very important to quantify in the modeling process. In some of the studies, it is found that this kind of delay gives complex dynamics [48,49]. Keeping, these crucial factors in mind, in this study, we consider two discrete time delays in the model. First, we consider the delay effect in the growth of information but assume the growth function as a linear function of infective which is meaningful if we consider relatively low infection persistence. Second, we quantify another delay in the waning immunity of individuals in the recovered class related to loss of protective measures (such as vaccination, self-protection, responsive behaviour etc.) with the aim to explore its impact mathematically and biologically. Thus, the main objective of this study is to explore the impact of above mentioned time delays on the disease dynamics and we would like to mention here that to the best of the author's knowledge, this type of particular setup has not been explored much in the literature though delay models have been analyzed but not the impact of information and immunity loss together in delay context. Consideration of two delays in a model itself increases huge mathematical complexity which will be more challenging. One can refer to articles [51,52] for more details of mathematical difficulties that arise in two delay model systems.
The delay models concerning information have been studied in the literature in different modeling sets up. For example see references [19,21,42,53,54,55,56]. Misra et al. in 2011, proposed a model for the dynamics of infectious diseases in which susceptible individuals become aware due to awareness driven by media with delay effect [21]. Authors have shown the occurrence of oscillations via Hopf bifurcation due to the delay effect. Zhao et al. in 2014, considered an SIRS model which accounts for the media impact on the force of infection along with delay effect [42]. They found that the disease free equilibrium is locally stable and also observed that the periodic solutions bifurcate around the infected equilibrium via Hopf bifurcation at a critical value of the delay. Further global Hopf bifurcation is also established for the model system. Liu et al. in 2015, proposed and analyzed an SIS epidemic model which accounts for the effect of delayed behavioural response on the force of infection [35]. They found that different forms of the delayed behavioural response exhibit various kinds of characteristics such as oscillations via Hopf and epidemic bubble.
Greenhalgh et al. in 2015, formulated an epidemic model in which the effect of awareness programs on the dynamics of diseases has been incorporated [19]. Further, the authors have extended their model to the corresponding delay model and observed that the delay induces oscillations via Hopf bifurcation. Uniform persistence of the disease with delay effect has also been established by authors. In 2017, Zhao et al. formulated an SIR epidemic model by quantifying the delayed effect of awareness driven by media in force of infection term [56]. They performed the model analysis and found that at the critical value of the delay, an endemic equilibrium loses its stability and bifurcates into periodic oscillations via Hopf bifurcation. But these models have incorporated the effect of information differently. However, the results obtained in our study match with them in principle, i.e., we observe oscillations in the populations due to the presence of delays. Li and others, in 2020, proposed an epidemic model which accounts for the effect of media publicity and found that shortening the delay of media reports may gradually decrease the infection from the population [53]. Further, in 2021, an SIRS epidemic model along with delay effect in information-induced force of infection term was formulated by Yadav and Srivastava in which treatment is in saturation type [54]. They found that the model system exhibits the existence of oscillatory behaviour due to the delay effect whereas, the saturation in treatment leads to the existence of multiple endemic equilibrium points. Recently, in 2022, Zhang and others formulated a compartmental model in which the susceptible population was divided into three subclasses due to the effect of awareness along with delay in immunity loss [55]. Authors explored the local dynamical properties such as local stability and Hopf bifurcation.
The rest of the paper is presented in the following order: in the next section, a delay mathematical model is proposed which contains two discrete delays. Further, the model analysis is performed for different cases of time delays in consecutive sections followed by numerical validation. The local bifurcations analysis along with the global stability of the equilibrium point is established in each case.
A mathematical model is formulated, in this section, which accounts for the effect of two different time delays: one in the growth of information and another in the waning of immunity. The model given by Kumar et al. [11] is modified to study the dynamics along with the modified rate equation of the information's dynamics. As in practice, there is always a time needed to lose the immunity related to protection. Therefore, in this model, the effect of delay is accounted for in the waning immunity of the recovered population. For this, a time delay τ1>0 is considered when recovered individuals will move to the susceptible class after losing the immunity. Also, there is always a significant time required for health agencies to measure the approximate cumulative density of the infective population and thus naturally a time delay comes in the dissemination of information generated by them. Therefore, during the outbreak, the effect of time lags or delays must be quantified in the growth factor of information to make the model dynamics more realistic. Therefore, a time delay τ2>0 is quantified in the growth of information as the information at time t will be measured by the infective population present at time t−τ2. Keeping the above facts, the proposed delay differential equation model is given by
dS(t)dt=Λ−βS(t)I(t)−μS(t)−u1dZ(t)S(t)+δ0R(t−τ1),dI(t)dt=βS(t)I(t)−(μ+δ+γ)I(t),dR(t)dt=γI(t)+u1dZ(t)S(t)−μR(t)−δ0R(t−τ1),dZ(t)dt=aI(t−τ2)−a0Z(t), | (2.1) |
with the initial population size S(θ)=S0≥0,I(θ)=I0≥0,R(θ)=R0≥0 and Z(θ)=Z0≥0 where θ∈[−τ,0], where τ=max{τ1,τ2}. Also, the initial condition lies in the Banach space of continuous functions C([−τ,0],R4+).
In this study, three sub-populations (S(t)-susceptible, I(t)-infective, R(t)-removed) of the entire population (N(t)) are considered to study at any given time t. Also, the density of the information is measured by the variable Z(t) which depends on the cumulative count of the infective as well as other social media/activities [11,39]. Thus, the information variable Z(t) dimensionally follows the number of infected individuals or prevalence of the disease [39]. Here, the growth of the susceptible population is given by parameter Λ. Natural mortality and disease related deaths are measured by the parameters μ and δ respectively whereas γ represents the recovery of the infected population. A homogeneously mixed population and a mass action type interaction between susceptible and infective are assumed for the disease transmission with the rate β. The loss of immunity is represented by the parameter δ0 which consists of the loss of natural immunity as well as immunity of protective measures. The factor u1dZ(t)S(t) is quantified as the information-induced behavioural response of susceptible individuals with response rate u1d. Where the parameter u1 is the response intensity with 0≤u1≤1 and the d denotes the information interaction rate that influences an individual's behaviour. The growth of information (Z) and their natural degradation are measured by the parameters a and a0 respectively.
This section focuses to study the qualitative insight of the mathematical model by investigating the stability of equilibrium points, and the existence of bifurcations such as Hopf bifurcation and Hopf-Hopf bifurcation due to considered delays. For this purpose, first, the boundedness and positivity of the solutions are established.
From the model system (2.1) for t∈[0,τ], the solution are given as
S(t)=e−∫t0(βI+μ+dZ)dξ[S(0)+∫t0(Λ+δ0R(t−τ1))e−∫t0(βI+μ+dZ)dξdξ],I(t)=I(0)e−∫t0(μ+δ+γ−βI)dξ,R(t)=e−μt[R(0)+∫t0(γI+u1dZS−δ0R(t−τ1))e−μξdξ],Z(t)=e−a0t[Z(0)+∫t0aI(t−τ2)e−a0ξdξ], | (3.1) |
Clearly, from the second equation of (3.1), we have I(t)≥0. It is also clear from the third equation, R(t) may or may not be positive (refer to Claim 1 of [57]). Following similar argument from Lv et al. [43], during the outbreak, the count of I(t) and R(t) may be very large than the R(t−τ1), thus it is reasonable to assume γI+u1dZS−δ0R(t−τ1) positive which leads the R(t)>0 and hence, R(t−τ1)>0. Using, the positivity of R(t) and I(t), we have S(t)>0 and Z(t)>0. Further, in order to establish the bound of the population, we consider from above that all the sub-populations will remain positive for all time when the initial populations are positive. Further, notice that the entire population N(t), in the model system (2.1), follows the following differential equation
dN(t)dt=Λ−μN(t)−δI(t)≤Λ−μN(t). |
Therefore, we have lim supt→∞N(t)≤Λμ. Clearly, all the sub-populations (S(t),I(t) and R(t)) are bounded by Λμ. Moreover, using the bound of I, the last equation of the model system (2.1) implies lim supt→∞Z(t)≤aΛa0μ. Using the above facts, the following positive invariant set describes the biologically feasible region for the model system (2.1):
Σ={(S(t),I(t),R(t),Z(t))∈R4+∣0≤S(t),I(t),R(t)≤Λμ,0≤Z(t)≤aΛa0μ}. |
The basic reproduction number of the model system (2.1) (as computed in Kumar et al. [11]) is given below:
R0=Λβμ(μ+δ+γ). |
The model (2.1) have following equilibrium points:
(i) a disease free equilibrium E1=(Λμ,0,0,0) which exists always and unconditionally, and
(ii) a unique endemic equilibrium E2=(S∗,I∗,R∗,Z∗), which exists if and only if R0>1. Here, S∗=(μ+δ+γ)β, R∗=I∗μ+δ0(γ+du1a(μ+δ+γ)a0β), Z∗=aI∗a0 and I∗=−CB, where B=μ(μ+δ+γ)+δ0(μ+δ)(μ+δ0)+μdu1a(μ+δ+γ)βa0(μ+δ0) and C=Λ(1R0−1).
The stability results obtained in Kumar et al. [11] are restated below for the case of no delay (τ1=τ2=0).
Theorem 1. [11] For τ1=τ2=0,
(i) the disease free equilibrium E1 of the system (2.1) is locally asymptotically stable if R0<1 and is unstable if R0>1,
(ii) if R0>1, then the unique endemic equilibrium E2 is locally asymptotically stable provided following conditions are satisfied:
P1P2>P3andP1(P2P3−P1P4)>P23. |
Here, P1=a0+2μ+δ0+βI∗+du1Z∗, P2=a0(μ+δ0)+(μ+a0)(μ+βI∗+du1Z∗)+δ0(μ+βI∗)+β2S∗I∗, P3=βI∗((a0+μ)(μ+δ+γ)+δ0(μ+δ))+μa0(μ+βI∗+du1Z∗)+a0δ0(μ+βI∗)+adu1βS∗I∗ and P4=βa0I∗(μ(μ+δ+γ)+δ0(μ+δ))+aμβdu1S∗I∗.
Stability of the equilibrium points of the delay model (2.1) are established in the subsequent parts when delays are present.
Theorem 2. (i) The disease free equilibrium E1 is locally asymptotically stable for any τ1,τ2≥0, if R0<1 and δ0≤μ.
(ii) The disease free equilibrium E1 is locally asymptotically stable for 0≤τ1<^τ1 and τ2≥0, if R0<1 and δ0>μ. Here
^τ1=1√δ20−μ2arctan(−√δ20−μ2μ). |
(iii) If R0>1, the disease free equilibrium E1 is unstable for any τ1,τ2≥0.
Proof. The characteristic equation at E1 is given by,
Δ(λ,τ1,τ2)=det(λI4−(JE1+e−λτ1J2+e−λτ2J3))=0. |
where, JE1=(−μ−βΛμ0−du1Λμ0βΛμ−(μ+δ+γ)000γ−μdu1Λμ000−a0), J2=(00δ00000000−δ000000), J3=(0000000000000a00) and I4 is the identity matrix of order four. Now
Δ(λ,τ1,τ2)=(λ+μ)(λ+a0)(λ−(μ+δ+γ)(R0−1))(λ+μ+δ0e−λτ1)=0. |
Hence, the eigenvalues are λ=−a0,−μ,(μ+δ+γ)(R0−1) which are negative if R0<1. The remaining eigenvalues are solution to
λ+μ+δ0e−λτ1=0. | (3.2) |
When τ1=0, the solution is λ=−μ−δ0<0. For τ1>0, let us suppose that λ=iω(ω>0) is a pure imaginary root of the Equation 3.2. Further, the real and imaginary parts are separated as follows
μ+δ0cos(ωτ1)=0,ω−δ0sin(ωτ1)=0, | (3.3) |
which provides ω2=δ20−μ2>0 if δ0>μ.
Corresponding to ω=√δ20−μ2 for δ0>μ, the Eq (3.3) gives
τ1=^τ1=1√δ20−μ2arctan(−√δ20−μ2μ). |
When δ0≤μ, the Eq (3.3) has no positive solution. Hence, the theorem is proven.
Example 1. Here, we shall numerically support the aforesaid analytical results for the stability properties of the disease free equilibrium with the help of model parameters which are selected as: Λ=5,β=0.003,μ=0.04, d=0.17,δ=0.5,δ0=0.02,a=0.1,a0=0.1,γ=0.1,u1=0.9. For this set of model parameters, the delay model induces only disease free equilibrium E1=(125,0,0,0) as in this case R0=0.585<1. Clearly, μ>δ0 which ensures the local stability of the disease free equilibrium E1 for all time lags τ1,τ2≥0 (Theorem 2(i)) and the corresponding numerical result is given in Figure 1.
Further, we choose δ0=0.4(>μ=0.04) except with the same parameters as above. In this case, the model has the same disease free equilibrium (E1) and R0 along with the threshold value of the time delay τ1 as ^τ1=4.198. Clearly from Theorem 2(ii), when the delay τ1<^τ1, the disease free equilibrium E1 is locally stable for all τ2≥0 and the corresponding obtained results are shown in Figure 2. Whereas, when τ1>^τ1, the disease free equilibrium E1 loses its stability and Figure 3 shows the corresponding result.
Remark 1. As we have assumed previously that the population is positive, so Figure 3(a) is not biologically feasible due to oscillations in the negative zone though it is mathematically sound to give the instability nature or property of the disease free equilibrium E1.
Here, we shall establish the local stability of the endemic equilibrium E2 when both the time lags are present in the system. For this, around endemic equilibrium E2, we linearise the delay model (2.1) as.
dY(t)dt=J4Y(t)+J2Y(t−τ1)+J3Y(t−τ2). | (3.4) |
Here, J4=(−(μ+βI∗+du1Z∗)−βS∗0−du1S∗βI∗000du1Z∗γ−μdu1S∗000−a0),
Y(t)=(S(t),I(t),R(t),Z(t))T. Here, J2 and J3 are defined as in the previous subsection.
For the linearized system (3.4), the characteristic equation is given by,
D(λ,τ1,τ2)=det(λI4−(J4+e−λτ1J2+e−λτ2J3))=0, |
with four order identity matrix I4. Further, it can be rewritten as
D(λ,τ1,τ2)=λ4+A1λ3+A2λ2+A3λ+A4+e−λτ1(B1λ3+B2λ2+B3λ+B4)+e−λτ2(C1λ+C2)=0, | (3.5) |
where
A1=a0+2μ+βI∗+du1Z∗>0A2=a0μ+(μ+a0)(μ+βI∗+du1Z∗)+β2S∗I∗>0A3=βI∗(a0+μ)(μ+δ+γ)+μa0(μ+βI∗+du1Z∗)>0A4=βa0I∗μ(μ+δ+γ)>0B1=δ0>0B2=δ0(a0+μ+βI∗)>0B3=a0δ0(μ+βI∗)+βI∗δ0(μ+δ)>0B4=βa0I∗δ0(μ+δ)>0C1=adu1βS∗I∗>0C2=μadu1βS∗I∗>0. |
Notice that, infinitely many complex roots can be obtained from the characteristic Eq (3.5) due to its transcendental nature in λ. We consider a similar argument for the stability of E2 as discussed in [58]. Thus, the sign of real parts of the roots of the characteristic Eq (3.5) will determine the stability nature of endemic equilibrium E2. The negative real parts will lead to local stability whereas the instability will rise in the case when a root crosses the imaginary axis (existence of a purely imaginary root). Also, the transcendental nature of Eq (3.5) infers that it is very tedious to determine the sign of roots of the Eq (3.5). Thus, the sign will change when the roots of the Eq (3.5) cross the imaginary axis as per Rouche's Theorem and continuity in time delays (τ1 and τ2).
The subsequent sections will determine the stability properties of the endemic equilibrium point E2 in different scenarios.
In this section, the stability properties of E2 under τ1>0 and τ2=0 will be examined and the corresponding characteristic Eq (3.5) is given by,
D(λ,τ1)=λ4+A1λ3+A2λ2+(A3+C1)λ+(A4+C2)+e−λτ1(B1λ3+B2λ2+B3λ+B4)=0. | (4.1) |
It is noted that when τ1=0, the Eq (4.1) possess roots with negative real parts (Theorem 1) under the parametric conditions P1P2>P3andP1(P2P3−P1P4)>P23 where P1=A1+B1,P2=A2+B2,P3=A3+B3+C1 and P4=A4+B4+C2. Further, in order to get the purely imaginary roots when τ1>0, we replace λ=iω in the Eq (4.1) and the corresponding real and imaginary parts are listed below:
ω4−A2ω2+A4+C2=(B2ω2−B4)cosωτ1+(B1ω3−B3ω)sinωτ1. | (4.2) |
(A3+C1)ω−A1ω3=(B1ω3−B3ω)cosωτ1−(B2ω2−B4)sinωτ1. | (4.3) |
Further, we square and add both the Eqs (4.2) and (4.3), we have
ω8+A11ω6+A12ω4+A13ω2+A14=0. | (4.4) |
Here, A11=A21−2A2−B21,A12=A22+2(A4+C2)−2A1(A3+C1)−B22+2B1B3, A13=(A3+C1)2−2A2(A4+C2)+2B2B4−B23 and A14=(A4+C2)2−B24.
By substituting m=ω2 in Eq (4.4), we have
ψ(m)=m4+A11m3+A12m2+A13m+A14=0. | (4.5) |
It is very clear that the Eq (4.5) will have all roots with negative real part if the Routh-Hurwitz criterion holds true for (4.5) which gives the following result.
Theorem 3. The unique endemic equilibrium E2 of the delay system (2.1) will be locally asymptotically stable for all τ1>0 and τ2=0 provided following conditions hold
A11>0,A13>0,A14>0andA11A12A13>A213+A211A14. |
In this part, the occurrence of Hopf bifurcation (periodic solutions) is established when an endemic equilibrium loses its stability. For this, the delay parameter τ1 is considered as a bifurcation parameter. There exists a Hopf bifurcation at a threshold value of the delay τ10 if
(H1) λ1,2(τ10)=±iω10(ω10>0) and all other eigenvalues are with negative real parts at τ=τ10.
(H2) [Re(dλ1,2dτ1)−1]|λ=iω10≠0.
For the (H1) condition, we require that there exists at least one positive root of the Eq (4.5). Descartes' rule of signs [59] will determine the conditions for at least one positive root of the Eq (4.5) in following result.
Lemma 1. The Eq (4.5) has
(i) at least one positive root (either one or three) if
(a) A11>0,A12<0,A13>0,A14<0,
(b) A11<0,A12<0,A13>0,A14<0,
(c) A11<0,A12>0,A13>0,A14<0,
(d) A11<0,A12>0,A13<0,A14<0.
(ii) exactly one positive root if
(a) A11<0,A12<0,A13<0,A14<0,
(b) A11>0,A12<0,A13<0,A14<0,
(c) A11>0,A12>0,A13<0,A14<0,
(d) A11>0,A12>0,A13>0,A14<0.
(iii) at most two positive roots if
(a) A11>0,A12<0,A13>0,A14>0,
(b) A11>0,A12>0,A13<0,A14>0,
(c) A11<0,A12>0,A13>0,A14>0.
Assume that the Eq (4.5) holds one of the conditions of Lemma 1, then, the Eq (4.5) has at least one positive root say m10=ω120. Then, for a threshold value of the delay τ1, there will be a pair of purely imaginary roots (±iω10) for the Eq (4.1). We obtain the following threshold value for the delay τ1 with the help of Eqs (4.2) and (4.3) as:
τ10=1ω10[arccos(Υ(ω10))], | (4.6) |
where
Υ(ω10)=(B2ω120−B4)(ω140−A2ω120+A4+C2)+(B1ω130−B3ω10)((A3+C1)ω10−A1ω130)(B2ω120−B4)2+(B1ω130−B3ω10)2. | (4.7) |
Further, we differentiate the Eq (4.1) with respect to τ1 to get the transversality condition (H2) as:
[Re(dλdτ1)−1]|λ=iω10=ψ′(m)(B2ω120−B4)2+(B1ω130−B3ω10)2. | (4.8) |
Lemma 2. Let iω10 be a purely imaginary root with m10=ω120 such that ψ(ω10)=0 and ψ′(ω10)≠0, then [Re(dλdτ1)−1]|λ=iω10≠0 and its sign is the same as ψ′(ω10)≠0.
Above results ensure that the transversality condition holds true. The following result summarises the above discussion.
Theorem 4. The unique endemic equilibrium E2 is locally asymptotically stable for τ1<τ10 and is unstable for τ1>τ10. At τ1=τ10, a Hopf bifurcation occurs, i.e., a family of periodic solutions bifurcates from the endemic equilibrium E2 as delay parameter τ1 crosses the threshold value τ10 [60,61].
For numerical validation of the Hopf bifurcation result, a set of parameters are chosen as in Example 1 except β=0.0125,μ=0.02 and δ0=0.05 so that the basic reproduction number is R0=5.04>1. In this case, the model has the unique endemic equilibrium E2=(49.59,1.47,162.04,1.47) along with the unstable disease free equilibrium E1=(250,0,0,0). Further, we notice that the coefficients of the Eq (4.5) are A11>0,A12<0,A13>0 and A14>0, and hence, the condition iii(a) of Lemma 1 holds. This gives that the Eqs (4.5) and (3.5) have a positive root (0.04509) and a pair of purely imaginary root (±0.212i with ω10=0.212) respectively. Further, by using Eq (4.6), the threshold value of the delay τ1 is calculated as τ10=7.97 along with transversality condition [Re(dλdτ1)−1]|λ=iω10=2.2×102>0. Thus, Theorem 4 ensures that the delay system (2.1) will be stable for the delay range τ1∈[0,τ10) and unstable for τ1>τ10. Whereas periodic oscillations bifurcate at τ1=τ10=7.97 around E2 when τ1 crosses τ10.
Further, we numerically exhibit the stability and instability of the unique endemic equilibrium E2 by solving the delay system (2.1) with the help of DDE23 in MATLAB. The delay model is solved for the delay τ1=7<τ10 with initial population size S(θ)=70,I(θ)=5,R(θ)=10 and Z(θ)=5 for θ∈[−τ1,0] and model parameters taken as above. Figure 4 describes the corresponding result which infers that the unique endemic equilibrium E2 is asymptotically stable.
Furthermore, Theorem 4 infers that the unique endemic equilibrium E2 loses its stability leading to the existence of a family of periodic solutions when delay τ1 crosses the threshold value τ10=7.97. To show this numerically, the delay system (2.1) is further solved for the delay parameter τ=9>τ10. The corresponding periodic solutions are plotted in Figure 5 which mimics the obtained analytical result. Therefore, our study infers that the disease will survive in oscillatory nature due to the delay effect considered in the waning of the immunity. Moreover, if this delay takes more than eight days, then it will be very challenging to estimate the actual size of the epidemic due to oscillations which leads the difficulty in control implementation. In addition, in this case, disease elimination may be very critical and challenging due to oscillations and hence, the actual estimation of an epidemic cannot be measured. A similar kind of observation is found in the study of Barbarossa and others [48] though in this case, authors have taken waning immunity in a different context other than in our case. In addition, recently in 2022, Zhang and others also established the existence of such oscillations (Hopf bifurcation) when the delay in waning crosses a threshold value when the susceptible population is divided based on the constant effect of awareness. A similar kind of observation has also been encountered by Yadav and Srivastava when a constant effect of information with delay is quantified [54]. Whereas, in this proposed study, a dynamic effect of information is considered along with a delay in waning immunity. Thus, this proposed study gives more generalised biological and mathematical insights. Our obtained Hopf bifurcation result and study also have good agreement in results claimed by Lv et al. [43]. In 2023, Lv et al. investigated the delay effects related to the failure of antibody and COVID-19 booster vaccination dose, and they found the existence of periodic oscillations for a real data set.
In addition, the bifurcation diagrams are plotted to show the periodic orbits when the unique endemic equilibrium loses its stability at the threshold value of τ1. The delay parameter is varied in $ \tau_1\in[7,11] $ to plot the diagrams and are shown in Figures 6–8. One can easily see from Figures 6 and 7 that when the delay parameter τ1 lies in the range [7,7.97), the unique endemic equilibrium E2 is stable and as τ1 crosses the threshold value τ10=7.97 bifurcation takes place. In addition, we also plot the occurrence of periodic orbits as the delay parameter τ1 varies, and the corresponding bifurcation diagram is shown in Figure 8.
This particular part explores the global properties of endemic equilibrium E2 by proposing a suitable Lyapunov function.
Theorem 5. If R0>1, τ1>0 and τ2=0, the unique endemic equilibrium E2 of the model system (2.1) is globally asymptotically stable under following parametric conditions a2<2a0(μ+δ), δ20<4γμ23(2μ+δ), (du1)2<2a0γμ33Λ2(2μ+δ) and ((2μ+δ)du1Z∗−2μγ)2<43γμ2(2μ+δ).
Proof. In order to establish the global stability, we construct the following positive definite function in ∑,
U1(S,I,R,Z)=12[(S−S∗)+(I−I∗)+(R−R∗)]2+K12(R−R∗)2+K2(I−I∗−I∗lnII∗)+12(Z−Z∗)2+μ∫tt−τ1(R(ν)−R∗)2dν, |
The positive constants K1 and K2 will be selected suitably later. Now, we shall differentiate the function U1 with respect to t along the solution trajectories of the model (2.1) and using parametric relationship of equilibrium points.
We have,
˙U1=[(S−S∗)+(I−I∗)+(R−R∗)]d(S+I+R)dt+K1(R−R∗)dRdt+K2(I−I∗)IdIdt+(Z−Z∗)dZdt+μ((R−R∗)2−(R(t−τ1)−R∗)2)=[(S−S∗)+(I−I∗)+(R−R∗)][Λ−μ(S+I+R)−δI]+K1(R−R∗)[γI−μR+du1ZS−δ0R(t−τ1)]+K2(I−I∗)I(βSI−(μ+δ+γ)I)+(Z−Z∗)(aI−a0Z)+μ((R−R∗)2−(R(t−τ1)−R∗)2)=[(S−S∗)+(I−I∗)+(R−R∗)][−μ(S−S∗)−(μ+δ)(I−I∗)−μ(R−R∗)]+K1(R−R∗)[γ(I−I∗)−μ(R−R∗)−δ0(R(t−τ1)−R∗)+du1(ZS−Z∗S∗)]+K2(I−I∗)(βS−βS∗)+(Z−Z∗)(a(I−I∗)−a0(Z−Z∗))+μ((R−R∗)2−(R(t−τ1)−R∗)2)=−μ(S−S∗)2−(μ+δ)(I−I∗)2−μ(R−R∗)2−(2μ+δ)(S−S∗)(I−I∗)−2μ(S−S∗)(R−R∗)−(2μ+δ)(R−R∗)(I−I∗)+K1γ(R−R∗)(I−I∗)−K1μ(R−R∗)2−K1δ0(R−R∗)(R(t−τ1)−R∗)+K1du1Z∗(S−S∗)(R−R∗)+K1du1S(Z−Z∗)(R−R∗)+K2β(S−S∗)(I−I∗)+a(I−I∗)(Z−Z∗)−a0(Z−Z∗)2+μ(R−R∗)2−μ(R(t−τ1)−R∗)2. |
Further, selecting and replacing the values of positive constants as: K1=2μ+δγ and K2=2μ+δβ, we have
˙U1=−μ(S−S∗)2−(μ+δ)(I−I∗)2−2μ+δγμ(R−R∗)2−2μ+δγδ0(R−R∗)(R(t−τ1)−R∗)+(2μ+δγdu1Z∗−2μ)(S−S∗)(R−R∗)+2μ+δγdu1S(Z−Z∗)(R−R∗)+a(I−I∗)(Z−Z∗)−a0(Z−Z∗)2−μ(R(t−τ1)−R∗)2=−F1((S−S∗)(R−R∗))−F2((R−R∗)(R(t−τ1)−R∗))−F3((Z−Z∗)(R−R∗))−F4((I−I∗)(Z−Z∗)), |
where F1((S−S∗)(R−R∗))=[μ(S−S∗)2−(2μ+δγdu1Z∗−2μ)(S−S∗)(R−R∗)+2μ+δ3γμ(R−R∗)2], F2((R−R∗)(R(t−τ1)−R∗))=[2μ+δ3γμ(R−R∗)2+2μ+δγδ0(R−R∗)(R(t−τ1)−R∗)+μ(R(t−τ1)−R∗)2], F3((Z−Z∗)(R−R∗))=[a02(Z−Z∗)2−2μ+δγdu1S(Z−Z∗)(R−R∗)+2μ+δ3γμ(R−R∗)2] and F4((I−I∗)(Z−Z∗))=[(μ+δ)(I−I∗)2−a(I−I∗)(Z−Z∗)+a02(Z−Z∗)2]. Note that within the feasible region ∑, ˙U1≤0 if following parametric conditions a2<2a0(μ+δ), δ20<4γμ23(2μ+δ), (du1)2<2a0γμ33Λ2(2μ+δ) and ((2μ+δ)du1Z∗−2μγ)2<43γμ2(2μ+δ) hold true. It is observed that ˙U1=0 if and only if at endemic equilibrium E2 and ˙U1<0 otherwise within the feasible region ∑, therefore the singleton set {E2} is the largest positively invariant set contained in {(S,I,R,Z)∈∑:˙U1=0}. Hence, in the interior of ∑, Lyapunov LaSalle's theorem [62] ensures that the endemic equilibrium E2 is globally asymptotically stable.
Example 2. In this part, we shall numerically support the aforesaid global stability property of the unique endemic equilibrium with the help of model parameters which are selected as: Λ=5,β=0.0125,μ=0.02,d=0.0017,δ=0.5,δ0=0.005,a=0.01,a0=0.1,γ=0.1,u1=0.009. For this set of model parameters, the delay model has the unique endemic equilibrium E2=(49.6,6.67,26.73,0.66) along with the unstable disease free equilibrium E1=(250,0,0,0) and in this case, R0=5.0403>1. Clearly, the parametric conditions for global stability a2−2a0(μ+δ)=−0.1039<0, δ20−4γμ23(2μ+δ)=−7.3765×10−5<0, (du1)2−2a0γμ33Λ2(2μ+δ)=−3.7165×10−9 and ((2μ+δ)du1Z∗−2μγ)2−43γμ2(2μ+δ)=−1.2844×10−5<0 hold true. This confirms the global stability of the endemic equilibrium E2 for all time lags τ1,τ2>0 (Theorem 5).
From biological point of view, our global stability result infers that irrespective of time lag in immunity loss (even if for larger delay), the disease will persist in stable sense and it can be eradicated by bringing the basic reproduction number below one. Thus, oscillations may appear locally and will disappear in larger context and this is one of the important biological as well as mathematical insight.
The characteristic equation corresponding to this case is defined as follows:
D(λ,τ2)=λ4+(A1+B1)λ3+(A2+B2)λ2+(A3+B3)λ+(A4+B4)+e−λτ2(C1λ+C2)=0. | (5.1) |
Consider P1=A1+B1,Q1=A2+B2,W1=A3+B3 and D1=A4+B4. Using the similar argument as in Case-I, in the Eq (5.1), we further replace λ=iω and the corresponding real and imaginary parts are given by.
ω4−Q1ω2+D1=−C1ωsinωτ2−C2cosωτ2. | (5.2) |
W1ω−P1ω3=C2sinωτ2−C1ωcosωτ2. | (5.3) |
Now, we square and add both the above Eqs (5.2) and (5.3), we have
ω8+B11ω6+B12ω4+B13ω2+B14=0. | (5.4) |
Here, B11=P21−2Q1,B12=Q21+2D1−2P1W1,B13=W21−2D1Q1−C21 and B14=D21−C22. Further, we substitute m=ω2 in Eq (5.4), we get
Ψ(m)=m4+B11m3+B12m2+B13m+B14=0. | (5.5) |
Clearly, the Eq (5.5) will have all roots with negative real part if the Routh-Hurwitz criterion holds true for (5.5) which gives the following result.
Theorem 6. The unique endemic equilibrium E2 of the delay system (2.1) will be locally asymptotically stable for all τ2>0 provided following conditions hold
B11>0,B13>0,B14>0andB11B12B13>B213+B211B14. |
Following similarly as in Case-I, the delay parameter τ2 is taken as a bifurcation parameter and at the threshold value of delay τ=τ20, the assumptions (H1) and (H2) must hold for Hopf bifurcation.
At least one positive root of the Eq (5.5) is needed to get assumption (H1) which can be obtained using Descartes' rule of signs and following the same result as discussed in Lemma 1 for Bij for i,j=1−4 in place of Aij for i,j=1−4. Assume that the Eq (5.5) satisfies one of the conditions given in Lemma 1 to have at least one positive root say m20=ω220. Hence, the Eq (5.1) will have a pair of purely imaginary root (±iω20) at the threshold of the delay τ20. And the corresponding τ20 is calculated using Eqs (5.2) and (5.3) and is given by.
τ20=1ω20[arccos(Φ(ω20))], | (5.6) |
where,
Φ(ω20)=ω240(P1C1−C2)+ω220(C2Q1−C1W1)−C2D1C22+C21ω220. | (5.7) |
Further, the Eq (5.1) is differentiated with respect to τ2 to get the transversality condition (H2) as.
[Re(dλdτ2)−1]|λ=iω20=Ψ′(m)C22ω220+C21. | (5.8) |
Lemma 3. Let iω20 be a purely imaginary root with m20=ω220 such that ψ(ω20)=0 and Ψ′(ω20)≠0, then [Re(dλdτ2)−1]|λ=iω20≠0 and its sign is the same as Ψ′(ω20)≠0.
Above discussion ensures that the transversality condition holds true which leads the following result.
Theorem 7. The unique endemic equilibrium E2 is locally asymptotically stable for τ2<τ20 and is unstable for τ2>τ20. At τ2=τ20, a Hopf bifurcation occurs, i.e., a family of periodic solutions bifurcates from the endemic equilibrium E2 as delay parameter τ2 crosses the threshold value τ20 [60,61].
For the numerical experimentation of the Hopf bifurcation, a set of parameters as given in Example 1 are considered expect β=0.03 so that the basic reproduction number becomes R0=5.859>1. For this set of parameters, the model has a unique endemic equilibrium E2=(21.33,1.49,83.54,1.49) along with the unstable disease free equilibrium E1=(125,0,0,0). For the numerical simulation, we consider the initial population size S(θ)=30,I(θ)=5,R(θ)=85 and Z(θ)=1 for θ∈[−τ2,0]. Notice that the coefficients B11,B12 of the Eq (5.5) are positive and B13,B14 are negative, and hence, the condition Lemma 1ii(c) holds true. Therefore, the Eqs (5.5) and (5.1) have exactly one positive root (0.04005) and a pair of purely imaginary root (±0.20012i with ω20=0.20012) respectively. Also, in this case, τ20=1.537 and [Re(dλdτ2)−1]|λ=iω20=2.11>0. Thus, Theorem 7 infers that the delay system (2.1) will remain stable for the delay range τ2∈[0,τ20) and unstable for τ2>τ20. Whereas, when τ2 crosses τ20, the E2 loses stability and leads the occurrence of periodic oscillations at τ2=τ20=1.537.
Further, the delay model is examined numerically for delay parameter τ2=1<τ20 to check the stability of the unique endemic equilibrium E2 and the results are plotted in Figure 10 which infers that E2 is locally stable. In this case, the disease can be eradicated if the time lag in propagation of information (τ2) is less than couple of days after outbreak of the disease by bringing down the basic reproduction number R0 less than one (Theorem 2). From Theorem 7, as τ2 crosses τ20=1.537, the periodic solutions will appear around E2. To show this, the delay model (2.1) is further solved for τ2=3 and the oscillatory trajectories are plotted in Figure 11. Clearly, due to the delay effect considered in information growth, the disease will survive oscillatory in behaviour within the population and hence the implementation of suitable control is complicated.
It is important to notice here that, if the propagation of information takes more than couple of days after the outbreaks to make aware the population, then the population will experience the oscillatory behaviour of the disease. Therefore, in this case, it will be very difficult to account the actual size of the epidemic and hence, the control will be very challenging due to high and low peaks of the disease. Therefore, in order to control the spread of the disease, health agencies and governments have to propagate the information very quickly after the disease outbreak.
Now to further get insight into the model numerically, we wish to plot the bifurcation diagram to depict the oscillations and instability around E2. For this purpose, we vary τ2∈[0.5,3] and the corresponding bifurcation diagrams are shown in Figure 15. It is very clear from Figure 15 that the unique endemic equilibrium E2 is stable for the range τ2∈[0.5,1.537) whereas it loses its stability at τ20=1.537 leads the occurrence of oscillations in the population for τ2>1.537. Some authors such as Misra et al. and Greenhalgh et al. [19,21] have investigated similar kind of results for Hopf bifurcation using different modeling techniques.
This particular section focuses to study a different kind of bifurcation named as a Hopf-Hopf bifurcation which appears at two different values of the delay. It can be easily seen that the Eq (5.5) may have more than one positive root depending on the signs of the coefficients B11,B12,B13 and B14. Let us consider that the Eqs (5.5) and (3.5) have two positive roots (m21=ω221 and m22=ω222 and two pair of purely imaginary roots (±iω21 and ±iω22) respectively. Also, the critical values of the delay τ2 are obtained as.
τ2j=1ω2j[arccos(Φ(ω2j))],j=1,2. | (5.9) |
where Φ(ω2j)=ω24j(P1C1−C2)+ω22j(C2Q1−C1W1)−C2D1C22+C21ω22j, j=1,2. The corresponding transversality conditions are given by,
[Re(dλdτ2)−1]|λ=iω2j=Ψ′(m)C22ω22j+C21,j=1,2. | (5.10) |
We state the following result using the results given in [33,63,64].
Lemma 4. Let iω2j be the purely imaginary roots with m2j=ω22j such that ψ(ω2j)=0 and Ψ′(ω2j)≠0, \; j = 1, 2, then [Re(dλdτ2)−1]|λ=iω21>0 if Ψ′(ω21)>0 and [Re(dλdτ2)−1]|λ=iω22<0 if Ψ′(ω22)<0.
Using the Lemma 4, the existence of Hopf-Hopf (double) bifurcation is ensured in the following.
Theorem 8. The delay model undergoes Hopf-Hopf (double) bifurcation at τ21 and τ22 respectively. The unique endemic equilibrium E2 is locally asymptotically stable for τ2<τ21. At τ2=τ21 the system undergoes Hopf bifurcation and the endemic equilibrium E2 loses its stability. Further as delay parameter τ2 increases, the endemic equilibrium E2 regains its stability at τ2=τ22 and again system undergoes Hopf bifurcation. The endemic equilibrium E2 remains stable for τ2>τ22.
The Hopf-Hopf (double) bifurcation result is validated numerically in this part, for this, a set of parameters as given in Example 1 except β=0.03 and δ0=0.5. In this case, we note R0=5.859>1 and the unique endemic equilibrium E2=(21.33,5.25,32.73,5.25) is obtained for the delay model along with unstable disease free equilibrium E1=(125,0,0,0). Notice that the Eq (5.5) consists two positive roots m21=0.0452,m22=0.0097 as B11 and B14 are positive, and B12 and B13 are negative. Hence, two pair of purely imaginary roots ±0.212i and ±0.0985i with ω21=0.212,ω22=0.0985 are found for the characteristic Eq (5.1). The corresponding critical values of the delay parameter τ2 are calculated by using (9.1) and given as: τ21=6.18 and τ22=29.79. Also The transversality conditions are determined as: [Re(dλdτ2)−1]|λ=iω21=1.75>0 and [Re(dλdτ2)−1]|λ=iω22=−0.792<0. Hence Theorem 8 follows.
To show the stability and bifurcation, we simulate the delay system for the initial population size: S(θ)=30,I(θ)=7,R(θ)=30 and Z(θ)=4 for θ∈[−τ2,0] along with above parameters. Figures 16 and 17 describe the corresponding Hopf-Hopf bifurcation result numerically. Notice from Figures 16 and 17 that the unique endemic equilibrium E2 is locally stable for τ2∈[0,τ21=6.18) and at τ2=τ21=6.18 periodic oscillations appear through first Hopf bifurcation as E2 loses its stability. Further, as the bifurcation parameter increases, at τ2=τ22=29.79, periodic oscillations die out through second Hopf bifurcation and for τ2>τ22=29.79, the endemic equilibrium E2 regains its stability and remains stable. Thus, the delay in information propagation leads to the complex behaviour of the diseases.
Moreover, our finding suggests that Hopf-Hopf bifurcation gives a region for a delay that not only exhibits stability but also oscillations with different periods in population as shown in Figure 16. It is very clear from Figure 18 that E2 is stable for τ2=6<τ21=6.18 and τ2=31>τ22=29.79 and also oscillations appear around E2 for τ21<τ2=20<τ22. Therefore, multiple stability switches are observed due to the delay effect taken into account in the model system. We further infer that the system undergoes the existence of periodic orbits for τ2∈[6.18,29.79] as given in Figure 17 and hence infection will persist oscillatory within the population. In this case, it is clear from Figure 19 that the solution trajectories approach to periodic orbit for a fix τ2=15.
Thus, it is important to notice here that the control of disease spread will be very challenging when the delay in the dissemination of information lies τ2∈[6.18,29.79] due to oscillations (high and low peaks of an epidemic). Hence, if information propagates in the early phase of the epidemic, a reduction in disease transmission can be obtained. Therefore, biologically this Hopf-Hopf bifurcation result is very important which provides a range of specific delays where the infection will persist oscillatory and as discussed above this is a crucial range for delay parameter. In order to control the oscillations within the population, a reduction in delay value (propagation of information) is needed that is early dissemination of information is required. This kind of similar stability switches results were discussed in Barbarossa and others [48] but in a different context whereas a similar kind of observation is found in Kumar et al. [47]. In Liu et al. [35], authors obtained a closed loop shaped curve during Hopf-Hopf bifurcation which they named as 'endemic bubble'. In this section, we have also obtained a similar 'endemic bubble' by varying the bifurcation parameter. All the above studies include only a single delay effect in different contexts whereas, in this proposed model, we have considered two delays which gives the more generalised version of existing studies as two delays lead the high mathematical complexity.
Here, by formulating a suitable Lyapunov function, we shall explore the global properties of unique endemic equilibrium E2.
Theorem 9. If R0>1, τ1=0 and τ2>0, the unique endemic equilibrium E2 of the model system (2.1) is globally asymptotically stable under following parametric conditions a<min{δ,2a0}, (du1)2<a0γμ2(μ+δ)δΛ2 and (δdu1Z∗−2μγ)2<2γμδ(μ+δ0).
Proof. For this purpose, we consider the positive definite function in ∑ which is given by,
U2(S,I,R,Z)=12[(S−S∗)+(I−I∗)+(R−R∗)]2+K12(R−R∗)2+K2(I−I∗−I∗lnII∗)+12(Z−Z∗)2+a∫tt−τ2(I(ν)−I∗)2dν, |
The positive constants K1 and K2 will be selected suitably later. Now, we shall differentiate the function U1 with respect to t along the solution trajectories of the model (2.1) and using the parametric relationship of equilibrium points. We have,
˙U2=[(S−S∗)+(I−I∗)+(R−R∗)]d(S+I+R)dt+K1(R−R∗)dRdt+K2(I−I∗)IdIdt+(Z−Z∗)dZdt+a((I−I∗)2−(I(t−τ2)−I∗)2)=[(S−S∗)+(I−I∗)+(R−R∗)][Λ−μ(S+I+R)−δI]+K1(R−R∗)[γI−(μ+δ0)R+du1ZS]+K2(I−I∗)I(βSI−(μ+δ+γ)I)+(Z−Z∗)(aI(t−τ2)−a0Z)+a((I−I∗)2−(I(t−τ2)−I∗)2)=[(S−S∗)+(I−I∗)+(R−R∗)][−μ(S−S∗)−(μ+δ)(I−I∗)−μ(R−R∗)]+K1(R−R∗)[γ(I−I∗)−(μ+δ0)(R−R∗)+du1(ZS−Z∗S∗)]+K2(I−I∗)(βS−βS∗)+(Z−Z∗)(a(I(t−τ2)−I∗)−a0(Z−Z∗))+a((I−I∗)2−(I(t−τ2)−I∗)2)=−μ(S−S∗)2−δ(I−I∗)2−μ[(I−I∗)+(R−R∗)]2−(2μ+δ)(S−S∗)(I−I∗)−2μ(S−S∗)(R−R∗)−δ(R−R∗)(I−I∗)+K1γ(R−R∗)(I−I∗)−K1(μ+δ0)(R−R∗)2+K1du1Z∗(S−S∗)(R−R∗)+K1du1S(Z−Z∗)(R−R∗)+K2β(S−S∗)(I−I∗)+a(I(t−τ2)−I∗)(Z−Z∗)−a0(Z−Z∗)2+a((I−I∗)2−(I(t−τ2)−I∗)2). |
Now, choosing and replacing the values of positive constants as: K1=δγ and K2=2μ+δβ, we get
˙U2=−μ(S−S∗)2−μ[(I−I∗)2+(R−R∗)2]−(δ−a)(I−I∗)2−δγ(μ+δ0)(R−R∗)2+(δγdu1Z∗−2μ)(S−S∗)(R−R∗)+δγdu1S(Z−Z∗)(R−R∗)−a0(Z−Z∗)2+a(I(t−τ2)−I∗)(Z−Z∗)−a(I(t−τ2)−I∗)2=−G1((S−S∗)(R−R∗))−G2((Z−Z∗)(R−R∗))−G3((I(t−τ2)−I∗)(Z−Z∗))−(δ−a)(I−I∗)2, |
where G1((S−S∗)(R−R∗))=[μ(S−S∗)2−(δdu1Z∗γ−2μ)(S−S∗)(R−R∗)+δ(μ+δ0)2γ(R−R∗)2], G2((Z−Z∗)(R−R∗))=[a02(Z−Z∗)2−δdu1Sγ(Z−Z∗)(R−R∗)+δ(μ+δ0)2γ(R−R∗)2] and G3((I(t−τ2)−I∗)(Z−Z∗))=[a(I(t−τ2)−I∗)2−a(I(t−τ2)−I∗)(Z−Z∗)+a02(Z−Z∗)2]. Clearly, within the feasible region ∑, if following parametric conditions a<min{δ,2a0}, (du1)2<a0γμ2(μ+δ)δΛ2 and (δdu1Z∗−2μγ)2<2γμδ(μ+δ0) hold true, then ˙U2≤0. We found that ˙U2=0 if and only if at endemic equilibrium E2 and ˙U2<0 otherwise within the feasible region ∑, therefore the singleton set {E2} is the largest positively invariant set contained in {(S,I,R,Z)∈∑:˙U2=0}. Hence, in the interior of ∑, Lyapunov LaSalle's theorem [62] ensures that the endemic equilibrium E2 is globally asymptotically stable.
Example 3. In this example, the aforesaid global stability result of the unique endemic equilibrium is validated numerically for a set of the same parameters as considered in the Example 2. Similarly, the delay model have the unique endemic equilibrium E2=(49.6,6.67,26.73,0.66) and a disease free equilibrium E1=(250,0,0,0) (unstable) along with the basic reproduction number R0=5.0403>1. The parametric conditions for global stability are defined as: a−min{δ,2a0}=−0.19<0, (du1)2−a0γμ2(μ+δ)δΛ2=−3.1977×10−7<0 and (δdu1Z∗−2μγ)2−2γμδ(μ+δ0)=−3.4041×10−5<0. Notice that all the conditions are satisfied for this set of parameters which insures that the endemic equilibrium E2 is globally stable for all time lags τ1,τ2>0 (Theorem 9).
Here, we study the stability of endemic equilibrium E2 in the presence of both delays τ1 and τ2. In the following, we first state the result that infers about the sign of the real part of roots of the characteristic Eq (3.5).
Lemma 5. [65] If all roots of Eq (5.1) have negative real parts for τ2>0, then there exists a τ10(τ2)>0 such that all roots of Eq (3.5) have negative real parts when τ1<τ10(τ2).
Proof. Proof follows similarly as of Lemma 6 of [65].
Theorem 10. Let R0>1 and τ20=1ω20[arccos(Φ(ω20))], where Φ(ω20) is given in Eq (5.7). Then for any τ2<τ20 there exists a τ10(τ2)>0 such that the unique endemic equilibrium E2 is locally asymptotically stable for τ1<τ10(τ2) and τ2<τ20.
Proof. It follows from Theorem 7 that the Eq (5.1) has all negative real parts for τ2<τ20. Now using Lemma 5, the result follows.
Similarly, we state the following result for the delay τ1.
Theorem 11. Let R0>1 and τ10=1ω10[arccos(Υ(ω10))], where Υ(ω10) is given in Eq (4.7). Then for any τ1<τ10 there exists a τ20(τ1)>0 such that the unique endemic equilibrium E2 is locally asymptotically stable for τ2<τ20(τ1).
Remark 2. From Theorem 10, for the stability, an explicit form for threshold value of the τ10 can not obtain rather it only informs the existence of such value. Using numerical experimentation, we shall find the region of stability in the presence of both delays.
In order to find the stability region when both delays are present, we simulate the delay model system for parametric values as considered in the pervious section (Hopf-Hopf bifurcation).
Further, we shall vary both delays as 0.25≤τ1≤15 and 0.25≤τ2≤50 to obtain the stability region numerically and the corresponding result is shown in Figure 20. The stable endemic equilibrium is plotted in '∗' in τ2τ1-plane and ⋄ is showing otherwise.
This result is more precise for the above-considered set of parameters than the one established in Theorem 10. In addition, we would like to discuss here that, in general delay destabilises the system but from Theorems 10 and 11, it is clear that the system is still stable when both delays are present under some restrictions. Also, from the stability region (Figure 20), if we consider τ1=2 (small) and τ2=32 (large), one can find the system is stable whereas if we change it, the stability may change. Thus, stability or stability switches are important insights from the biological point of view as eradication of disease may be possible in the case of stability whereas, in the case of oscillations, it will be challenging.
In the previous sections, we have performed the local analysis of the delayed model system but due to mathematical complexity, it is very difficult to obtain the explicit parametric relation for local stability. Therefore, in this section, we shall establish the global stability of the unique endemic equilibrium E2 by constructing a suitable Lyapunov function.
Theorem 12. If R0>1, τ1>0 and τ2>0, the unique endemic equilibrium E2 of the model system (2.1) is globally asymptotically stable under following parametric conditions a<min{δ,2a0}, δ20<4γμ23(2μ+δ), (du1)2<2a0γμ23Λ2(2μ+δ) and ((2μ+δ)du1Z∗−2μγ)2<43γμ2(2μ+δ).
Proof. For global stability, we consider the following positive definite function in ∑,
U3(S,I,R,Z)=12[(S−S∗)+(I−I∗)+(R−R∗)]2+K12(R−R∗)2+K2(I−I∗−I∗lnII∗)+12(Z−Z∗)2+μ∫tt−τ1(R(ν)−R∗)2dν+a∫tt−τ2(I(ν)−I∗)2dν. |
The positive constants K1 and K2 will be selected suitably later. Now, we shall differentiate the function U1 with respect to t along the solution trajectories of the model (2.1) and using the parametric relationship of equilibrium points. We have,
˙U3=[(S−S∗)+(I−I∗)+(R−R∗)]d(S+I+R)dt+K1(R−R∗)dRdt+K2(I−I∗)IdIdt+(Z−Z∗)dZdt+μ((R−R∗)2−(R(t−τ1)−R∗)2)+a((I−I∗)2−(I(t−τ2)−I∗)2)=[(S−S∗)+(I−I∗)+(R−R∗)][Λ−μ(S+I+R)−δI]+K1(R−R∗)[γI−μR+du1ZS−δ0R(t−τ1)]+K2(I−I∗)I(βSI−(μ+δ+γ)I)+(Z−Z∗)(aI(t−τ2)−a0Z)+μ((R−R∗)2−(R(t−τ1)−R∗)2)+a((I−I∗)2−(I(t−τ2)−I∗)2)=[(S−S∗)+(I−I∗)+(R−R∗)][−μ(S−S∗)−(μ+δ)(I−I∗)−μ(R−R∗)]+K1(R−R∗)[γ(I−I∗)−μ(R−R∗)−δ0(R(t−τ1)−R∗)+du1(ZS−Z∗S∗)]+K2(I−I∗)(βS−βS∗)+(Z−Z∗)(a(I(t−τ2)−I∗)−a0(Z−Z∗))+μ(R−R∗)2−μ(R(t−τ1)−R∗)2+a((I−I∗)2−(I(t−τ2)−I∗)2)=−μ(S−S∗)2−(μ+δ)(I−I∗)2−μ(R−R∗)2−(2μ+δ)(S−S∗)(I−I∗)−2μ(S−S∗)(R−R∗)−(2μ+δ)(R−R∗)(I−I∗)+K1γ(R−R∗)(I−I∗)−K1μ(R−R∗)2−K1δ0(R−R∗)(R(t−τ1)−R∗)+K1du1Z∗(S−S∗)(R−R∗)+K1du1S(Z−Z∗)(R−R∗)+K2β(S−S∗)(I−I∗)+a(I(t−τ2)−I∗)(Z−Z∗)−a0(Z−Z∗)2+μ(R−R∗)2−μ(R(t−τ1)−R∗)2+a((I−I∗)2−(I(t−τ2)−I∗)2). |
Now, selecting and replacing the values of positive constants as: K1=2μ+δγ and K2=2μ+δβ, we have
˙U3=−μ(S−S∗)2−μ(I−I∗)2−(δ−a)(I−I∗)2−2μ+δγμ(R−R∗)2−2μ+δγδ0(R−R∗)(R(t−τ1)−R∗)+(2μ+δγdu1Z∗−2μ)(S−S∗)(R−R∗)+2μ+δγdu1S(Z−Z∗)(R−R∗)+a(I(t−τ2)−I∗)(Z−Z∗)−a0(Z−Z∗)2−μ(R(t−τ1)−R∗)2−a(I(t−τ2)−I∗)2=−H1((S−S∗)(R−R∗))−H2((R−R∗)(R(t−τ1)−R∗))−H3((Z−Z∗)(R−R∗))−H4((I(t−τ2)−I∗)(Z−Z∗)), |
where H1((S−S∗)(R−R∗))=[μ(S−S∗)2−(2μ+δγdu1Z∗−2μ)(S−S∗)(R−R∗)+2μ+δ3γμ(R−R∗)2], H2((R−R∗)(R(t−τ1)−R∗))=[2μ+δ3γμ(R−R∗)2+2μ+δγδ0(R−R∗)(R(t−τ1)−R∗)+μ(R(t−τ1)−R∗)2], H3((Z−Z∗)(R−R∗))=[a02(Z−Z∗)2−2μ+δγdu1S(Z−Z∗)(R−R∗)+2μ+δ3γμ(R−R∗)2] and H4((I(t−τ2)−I∗)(Z−Z∗))=[a(I(t−τ2)−I∗)2−a(I(t−τ2)−I∗)(Z−Z∗)+a02(Z−Z∗)2]. Note that within the feasible ∑, ˙U3≤0 if following parametric conditions a<min{δ,2a0}, δ20<4γμ23(2μ+δ), (du1)2<2a0γμ23Λ2(2μ+δ) and ((2μ+δ)du1Z∗−2μγ)2<43γμ2(2μ+δ) hold true. It is observed that ˙U3=0 if and only if at endemic equilibrium E2 and ˙U3<0 otherwise within the feasible region ∑, therefore the singleton set {E2} is the largest positively invariant set contained in {(S,I,R,Z)∈∑:˙U3=0}. Hence, in the interior of ∑, Lyapunov LaSalle's theorem [62] ensures that the endemic equilibrium E2 is globally asymptotically stable.
Example 4. Here, we perform the numerical validation of the aforesaid global stability result stated in Theorem 12 when the model has a unique endemic equilibrium when R0>1. For this purpose, we consider a set of the same parameters as mentioned in Example 2. For this set of parameters, the delay model has the unique endemic equilibrium E2=(49.6,6.67,26.73,0.66) and a disease free equilibrium E1=(250,0,0,0) (unstable) along with the basic reproduction number R0=5.0403>1. Now, we calculate the parametric conditions numerically and are given as: a−min{δ,2a0}=−0.19<0, δ20−4γμ23(2μ+δ)=−7.3765×10−5<0, (du1)2−2a0γμ23Λ2(2μ+δ)=−1.9730×10−7<0 and ((2μ+δ)du1Z∗−2μγ)2−43γμ2(2μ+δ)=−1.2844×10−5<0. Clearly, for this set of parameters, all the parametric relations hold true which insures that the endemic equilibrium E2 is globally stable for all time lags τ1,τ2>0 (Theorem 12).
Finally, a rich and complex dynamics are obtained for the model in the presence of time delays. Whereas, in the absence of one time delay either τ1 or τ2, the delay model system shows the occurrence of oscillations through Hopf bifurcation. Further, in the presence of τ2 only (τ1=0), the existence of Hopf-Hopf bifurcation is established at two different delays for the delay model. In addition, global analysis is also established for various cases by constructing suitable Lyapunov functions which is itself very mathematically challenging due to high mathematical complexity.
The effects of delayed information (τ2) and the delay in immunity loss (τ1) are studied for infectious diseases by formulating a delay mathematical model. To describe the dynamics of the delayed information, a rate equation is considered in which the density of information depends on the infective population as well as social media. Model analysis is performed and stability of equilibrium points is carried out for different scenarios of delay parameters. We observed that when the rate of immunity loss is small, the disease free equilibrium will be always stable otherwise it becomes unstable at a large rate. This happens due to the delay effect considered in the waning of immunity, if the delay parameter is smaller than a threshold quantity for R0<1, the disease free equilibrium is stable whereas it loses its stability as the delay crosses the threshold quantity. Generally, such type of phenomenon does not appear in non-delay models. In addition, when the effect of both delays is small enough, the unique endemic equilibrium is found locally stable for R0>1 and under some parametric conditions. Moreover, when both the delays (τ1 and τ2) cross critical values (either in presence of a single delay only or both delays), the existence of periodic solutions (via Hopf bifurcation) is investigated in this study. This leads to the existence of oscillatory persistence of disease within the population and hence, execution of control measures becomes challenging due to high and low epidemic peaks. Such Hopf bifurcation results follow the observation posed by a few researchers such as Yadav and Srivastava [54], and Zhang et al. [55] (single delay cases with the constant effect of information) but in the more general context as our model considered the dynamic effect of information with two discrete time delays. Our findings also follow similar facts as discussed by Lv et al. [43] for the delay considered in COVID-19 booster dose and antibody failure. Furthermore, the existence of multiple stability switches, termed as Hopf-Hopf (double) bifurcation at two different values of delay, is also examined for the case when only τ2 is present (τ1=0). In this case, at the first critical value of the τ2, the system is destabilised and undergoes the first Hopf bifurcation (the endemic equilibrium loses stability) whereas as τ2 increases, the system again undergoes the second Hopf bifurcation (the endemic equilibrium regains the stability) at another critical value of the delay. Therefore, both delays (reporting of infective and delay in waning the immunity) not only destabilise the system but also retain its stability which is very important as the contraction of infection is possible. Here, we infer that our obtained stability switch result falls in line with the result discussed by Kumar and others [47] and by Barbarossa and others [48] but with only single delay cases and different biological context. Therefore, our study also establishes similar kinds of important results under a simple mathematical form of delay factors considered in the model system to the other studies. Moreover, the global stability of the unique endemic equilibrium point is established by constructing Lyapunov functions in various cases for time delays which is itself difficult and challenging under high mathematical complexity raised by two crucial delays. The global stability result infers that the disease will persist in an endemic sense irrespective of delay impacts and can be suppressed if the basic reproduction number is below one. Mostly, delay models with a single delay, in this line of work, explore the local bifurcation analysis, not global properties. Finally, our study infers that the model system exhibits rich and complex dynamics locally as well as globally, and provides important insight. As, during the outbreak, optimal usage and allocation of suitable control measures with minimum economic damage are very difficult in any delay environment, hence, the corresponding optimal control problems may be possible pathways to solve such problems. In coming future, we shall try to address such problems.
The financial support of Anuj Kumar is from TIET, Patiala project no. TU/DORSP/57/7284. Yasuhiro Takeuchi acknowledges the financial support from JSPS "Grand-in-Aid 20K03755".
The authors declare there is no conflict of interest.
[1] | F. Brauer, C. Castillo-Chavez, Mathematical Models in Population Biology and Epidemiology, Springer, 2001. |
[2] | O. Diekmann, J. A. P. Heesterbeek, Mathematical Epidemiology of Infectious Diseases: Model Building, Analysis and Interpretation, John Wiley & Sons, 2000. |
[3] |
H. W. Hethcote, The mathematics of infectious diseases, SIAM Rev., 42 (2000), 599–653. https://doi.org/10.1137/S0036144500371907 doi: 10.1137/S0036144500371907
![]() |
[4] |
W. O. Kermack, A. G. McKendrick, A contribution to the mathematical theory of epidemics, Proc. R. Soc. Lond. A, 115 (1927), 700–721. https://doi.org/10.1098/rspa.1927.0118 doi: 10.1098/rspa.1927.0118
![]() |
[5] |
M. E. Alexander, C. Bowman, S. M. Moghadas, R. Summers, A. B. Gumel, B. M. Sahai, A vaccination model for transmission dynamics of influenza, SIAM J. Appl. Dyn. Syst., 3 (2004), 503–524. https://doi.org/10.1137/03060037 doi: 10.1137/03060037
![]() |
[6] |
A. B. Gumel, S. Ruan, T. Day, J. Watmough, F. Brauer, P. van den Driessche, et al., Modelling strategies for controlling SARS outbreaks, Proc. R. Soc. B: Biol. Sci., 271 (2004), 2223–2232. https://doi.org/10.1098/rspb.2004.2800 doi: 10.1098/rspb.2004.2800
![]() |
[7] |
S. Lee, G. Chowell, C. Castillo-Chávez, Optimal control for pandemic influenza: the role of limited antiviral treatment and isolation, J. Theor. Biol., 265 (2010), 136–150. https://doi.org/10.1016/j.jtbi.2010.04.003 doi: 10.1016/j.jtbi.2010.04.003
![]() |
[8] |
X. Liu, Y. Takeuchi, S. Iwami, SVIR epidemic models with vaccination strategies, J. Theor. Biol., 253 (2008), 1–11. https://doi.org/10.1016/j.jtbi.2007.10.014 doi: 10.1016/j.jtbi.2007.10.014
![]() |
[9] |
Z. Qiu, Z. Feng, Transmission dynamics of an influenza model with vaccination and antiviral treatment, Bull. Math. Biol., 72 (2010), 1–33. https://doi.org/10.1007/s11538-009-9435-5 doi: 10.1007/s11538-009-9435-5
![]() |
[10] |
A. Kumar, P. K. Srivastava, Vaccination and treatment as control interventions in an infectious disease model with their cost optimization, Commun. Nonlinear Sci. Numer. Simul., 44 (2017), 334–343. https://doi.org/10.1016/j.cnsns.2016.08.005 doi: 10.1016/j.cnsns.2016.08.005
![]() |
[11] |
A. Kumar, P. K. Srivastava, Y. Takeuchi, Modeling the role of information and limited optimal treatment on disease prevalence, J. Theor. Biol., 414 (2017), 103–119. https://doi.org/10.1016/j.jtbi.2016.11.016 doi: 10.1016/j.jtbi.2016.11.016
![]() |
[12] |
Y. Yuan, N. Li, Optimal control and cost-effectiveness analysis for a COVID-19 model with individual protection awareness, Phys. A: Stat. Mech. Appl., 603 (2022), 127804. https://doi.org/10.1016/j.physa.2022.127804 doi: 10.1016/j.physa.2022.127804
![]() |
[13] |
P. A. Gonzˊalez-Parra, S. Lee, L. Velazquez, C. Castillo-Chavez, A note on the use of optimal control on a discrete time model of influenza dynamics, Math. Biosci. Eng., 8 (2011), 183–197. doi: 10.3934/mbe.2011.8.183 doi: 10.3934/mbe.2011.8.183
![]() |
[14] |
S. M. Kassa, A. Ouhinou, The impact of self-protective measures in the optimal interventions for controlling infectious diseases of human population, J. Math. Biol., 70 (2015), 213–236. https://doi.org/10.1007/s00285-014-0761-3 doi: 10.1007/s00285-014-0761-3
![]() |
[15] |
A. Kumar, P. K. Srivastava, Y. Dong, Y. Takeuchi, Optimal control of infectious disease: Information-induced vaccination and limited treatment, Physica A: Stat. Mech. Appl., 542 (2020), 123196. https://doi.org/10.1016/j.physa.2019.123196 doi: 10.1016/j.physa.2019.123196
![]() |
[16] |
A. Yadav, P. K. Srivastava, A. Kumar, Mathematical model for smoking: Effect of determination and education, Int. J. Biomath., 8 (2015), 1550001. https://doi.org/10.1142/S1793524515500011 doi: 10.1142/S1793524515500011
![]() |
[17] | World Health Organization (WHO), Government of Senegal boosts Ebola awareness through SMS campaign, 2014. Avaliable from: http://http://www.who.int/features/2014/senegal-ebola-sms/en/. |
[18] |
A. Ahituv, V. J. Hotz, T. Philipson, The responsiveness of the demand for condoms to the local prevalence of AIDS, J. Hum. Resour., 31 (1996), 869–897. https://doi.org/10.2307/146150 doi: 10.2307/146150
![]() |
[19] |
D. Greenhalgh, S. Rana, S. Samanta, T. Sardar, S. Bhattacharya, J. Chattopadhyay, Awareness programs control infectious disease-multiple delay induced mathematical model, Appl. Math. Comput., 251 (2015), 539–563. https://doi.org/10.1016/j.amc.2014.11.091 doi: 10.1016/j.amc.2014.11.091
![]() |
[20] |
Y. Liu, J. Cui, The impact of media coverage on the dynamics of infectious disease, Int. J. Biomath., 1 (2008), 65–74. https://doi.org/10.1142/S1793524508000023 doi: 10.1142/S1793524508000023
![]() |
[21] |
A. K. Misra, A. Sharma, V. Singh, Effect of awareness programs in controlling the prevalence of an epidemic with time delay. J. Biol. Syst., 19 (2011), 389–402. https://doi.org/10.1142/S0218339011004020 doi: 10.1142/S0218339011004020
![]() |
[22] |
T. Philipson, Private vaccination and public health: an empirical examination for US measles, J. Hum. Resour., 31 (1996), 611–630. https://doi.org/10.2307/146268 doi: 10.2307/146268
![]() |
[23] |
J. Cui, Y. Sun, H. Zhu, The impact of media on the control of infectious diseases, J. Dyn. Differ. Equations, 20 (2008), 31–53. https://doi.org/10.1007/s10884-007-9075-0 doi: 10.1007/s10884-007-9075-0
![]() |
[24] |
A. d'Onofrio, P. Manfredi, Information-related changes in contact patterns may trigger oscillations in the endemic prevalence of infectious diseases, J. Theor. Biol., 256 (2009), 473–478. https://doi.org/10.1016/j.jtbi.2008.10.005 doi: 10.1016/j.jtbi.2008.10.005
![]() |
[25] |
S. Funk, E. Gilad, C. Watkins, V. A. A. Jansen, The spread of awareness and its impact on epidemic outbreaks, Proc. Natl. Acad. Sci. U.S.A., 106 (2009), 6872–6877. https://doi.org/10.1073/pnas.08107621 doi: 10.1073/pnas.08107621
![]() |
[26] |
I. Z. Kiss, J. Cassell, M. Recker, P. L. Simon, The impact of information transmission on epidemic outbreaks, Math. Biosci., 225 (2010), 1–10. https://doi.org/10.1016/j.mbs.2009.11.009 doi: 10.1016/j.mbs.2009.11.009
![]() |
[27] |
Y. Li, C. Ma, J. Cui, The effect of constant and mixed impulsive vaccination on SIS epidemic models incorporating media coverage, Rocky Mt. J. Math., 38 (2008), 1437–1455. DOI: 10.1216/RMJ-2008-38-5-1437 doi: 10.1216/RMJ-2008-38-5-1437
![]() |
[28] |
R. Liu, J. Wu, H. Zhu, Media/psychological impact on multiple outbreaks of emerging infectious diseases, Comput. Math. Methods Med., 8 (2007), 153–164. https://doi.org/10.1080/17486700701425870 doi: 10.1080/17486700701425870
![]() |
[29] | P. Manfredi, A. d'Onofrio, Modeling the Interplay Between Human Behavior and the Spread of Infectious Diseases, Springer Science & Business Media, 2013. |
[30] |
K. Cooke, P. Van. den Driessche, X. Zou, Interaction of maturation delay and nonlinear birth in population and epidemic models, J. Math. Biol., 39 (1999), 332–352. https://doi.org/10.1007/s002850050194 doi: 10.1007/s002850050194
![]() |
[31] |
D. Greenhalgh, Q. J. A. Khan, F. I. Lewis, Recurrent epidemic cycles in an infectious disease model with a time delay in loss of vaccine immunity, Nonlinear Anal. Theory Methods Appl., 63 (2005), e779–e788. https://doi.org/10.1016/j.na.2004.12.018 doi: 10.1016/j.na.2004.12.018
![]() |
[32] |
G. Huang, Y. Takeuchi, W. Ma, D. Wei, Global stability for delay SIR and SEIR epidemic models with nonlinear incidence rate, Bull. Math. Biol., 72 (2010), 1192–1207. https://doi.org/10.1007/s11538-009-9487-6 doi: 10.1007/s11538-009-9487-6
![]() |
[33] | Y. Kuang, Delay Differential Equations: with Applications in Population Dynamics, Academic Press, 1993. |
[34] |
N. Kyrychko, K. B. Blyuss, Global properties of a delayed SIR model with temporary immunity and nonlinear incidence rate, Nonlinear Anal. Real World Appl., 6 (2005), 495–507. https://doi.org/10.1016/j.nonrwa.2004.10.001 doi: 10.1016/j.nonrwa.2004.10.001
![]() |
[35] |
M. Liu, E. Liz, G. Röst, Endemic bubbles generated by delayed behavioral response: global stability and bifurcation switches in an SIS model, SIAM J. Appl. Math., 75 (2015), 75–91. https://doi.org/10.1137/140972652 doi: 10.1137/140972652
![]() |
[36] |
Y. Song, J. Wei, Bifurcation analysis for chen's system with delayed feedback and its application to control of chaos, Chaos, Solitons Fractals, 22 (2004), 75–91. https://doi.org/10.1016/j.chaos.2003.12.075 doi: 10.1016/j.chaos.2003.12.075
![]() |
[37] |
L. Wen, X. Yang, Global stability of a delayed SIRS model with temporary immunity, Chaos, Solitons Fractals, 38 (2008), 221–226. https://doi.org/10.1016/j.chaos.2006.11.010 doi: 10.1016/j.chaos.2006.11.010
![]() |
[38] |
T. Cheng, X. Zou, A new perspective on infection forces with demonstration by a DDE infectious disease model, Math. Biosci. Eng., 19 (2022), 4856–4880. doi: 10.3934/mbe.2022227 doi: 10.3934/mbe.2022227
![]() |
[39] |
A. d'Onofrio, P. Manfredi, E. Salinelli, Vaccinating behaviour, information, and the dynamics of SIR vaccine preventable diseases, Theor. Popul. Biol., 71 (2007), 301–317. https://doi.org/10.1016/j.tpb.2007.01.001 doi: 10.1016/j.tpb.2007.01.001
![]() |
[40] |
P. K. Srivastava, M. Banerjee, P. Chandra, A primary infection model for HIV and immune response with two discrete time delays, Differ. Equations Dyn. Syst., 18 (2010), 385–399. https://doi.org/10.1007/s12591-010-0074-y doi: 10.1007/s12591-010-0074-y
![]() |
[41] |
P. K. Srivastava, P. Chandra, Hopf bifurcation and periodic solutions in a dynamical model for HIV and immune response, Differ. Equations Dyn. Syst., 16 (2008), 77–100. https://doi.org/10.1007/s12591-008-0006-2 doi: 10.1007/s12591-008-0006-2
![]() |
[42] |
H. Zhao, Y. Lin, Y. Dai, An SIRS epidemic model incorporating media coverage with time delay, Comput. Math. Methods Med., 2014 (2014). https://doi.org/10.1155/2014/680743 doi: 10.1155/2014/680743
![]() |
[43] |
Z. Lv, X. Liu, Y. Ding, Dynamic behavior analysis of an SVIR epidemic model with two time delays associated with the COVID-19 booster vaccination time, Math. Biosci. Eng., 20 (2023), 6030–6061. https://doi.org/10.3934/mbe.2023261 doi: 10.3934/mbe.2023261
![]() |
[44] |
Y. Ma, Y. Cui, M. Wang, Global stability and control strategies of a SIQRS epidemic model with time delay, Math. Methods Appl. Sci., 45 (2022), 8269–8293. https://doi.org/10.1002/mma.8309 doi: 10.1002/mma.8309
![]() |
[45] |
A. Mezouaghi, S. Djillali, A. Zeb, K.S. Nisar, Global proprieties of a delayed epidemic model with partial susceptible protection, Math. Biosci. Eng., 19 (2022), 209–224. https://doi.org/10.3934/mbe.2022011 doi: 10.3934/mbe.2022011
![]() |
[46] |
H. Yang, Y. Wang, S. Kundu, Z. Song, Z. Zhang, Dynamics of an SIR epidemic model incorporating time delay and convex incidence rate, Results Phys., 32 (2022), 105025. https://doi.org/10.1016/j.rinp.2021.105025 doi: 10.1016/j.rinp.2021.105025
![]() |
[47] |
A. Kumar, P. K. Srivastava, A. Yadav, Delayed information induces oscillations in a dynamical model for infectious disease, Int. J. Biomath., 12 (2019), 1950020. https://doi.org/10.1142/S1793524519500207 doi: 10.1142/S1793524519500207
![]() |
[48] |
M. V. Barbarossa, M. Polner, G. Röst, Stability switches induced by immune system boosting in an sirs model with discrete and distributed delays, SIAM J. Appl. Math., 77 (2017), 905–923. https://doi.org/10.1137/16M1077234 doi: 10.1137/16M1077234
![]() |
[49] |
M. V. Barbarossa, G. Röst, Immuno-epidemiology of a population structured by immune status: a mathematical study of waning immunity and immune system boosting, J. Math. Biol., 71 (2015), 1737–1770. https://doi.org/10.1007/s00285-015-0880-5 doi: 10.1007/s00285-015-0880-5
![]() |
[50] | D. Wodarz, Killer Cell Dynamics: Mathematical and Computational Approaches to Immunology, Springer, 2007. |
[51] |
Q. An, E. Beretta, Y. Kuang, C. Wang, H. Wang, Geometric stability switch criteria in delay differential equations with two delays and delay dependent parameters, J. Differ. Equations, 266 (2019), 7073–7100. https://doi.org/10.1016/j.jde.2018.11.025 doi: 10.1016/j.jde.2018.11.025
![]() |
[52] | X. Lin, H. Wang, Stability analysis of delay differential equations with two discrete delays, Can. Appl. Math. Q., 20 (2012), 519–533. |
[53] |
D. Li, B. Chai, W. Liu, P. Wen, R. Zhang, Qualitative analysis of a class of SISM epidemic model influenced by media publicity, Math. Biosci. Eng., 17 (2020), 5727–5751. https://doi.org/10.3934/mbe.2020308 doi: 10.3934/mbe.2020308
![]() |
[54] |
A. Yadav, P. K. Srivastava, The impact of information and saturated treatment with time delay in an infectious disease model, J. Appl. Math. Comput., 66 (2021), 277–305. https://doi.org/10.1007/s12190-020-01436-2 doi: 10.1007/s12190-020-01436-2
![]() |
[55] |
Z. Zhang, G. ur Rahman, J. F. Gómez-Aguilar, J. Torres-Jiménez, Dynamical aspects of a delayed epidemic model with subdivision of susceptible population and control strategies, Chaos, Solitons Fractals, 160 (2022), 112194. https://doi.org/10.1016/j.chaos.2022.112194 doi: 10.1016/j.chaos.2022.112194
![]() |
[56] |
H. Zhao, M. Zhao, Global hopf bifurcation analysis of an susceptible-infective-removed epidemic model incorporating media coverage with time delay, J. Biol. Dyn., 11 (2017), 8–24. https://doi.org/10.1080/17513758.2016.1229050 doi: 10.1080/17513758.2016.1229050
![]() |
[57] |
Y. Liu, J. Wei, Bifurcation analysis in delayed nicholson blowflies equation with delayed harvest, Nonlinear Dyn., 105 (2021), 1805–1819. https://doi.org/10.1007/s11071-021-06651-5 doi: 10.1007/s11071-021-06651-5
![]() |
[58] | S. Ruan, J. Wei, On the zeros of transcendental functions with applications to stability of delay differential equations with two delays, Dyn. Contin. Discrete Impuls. Syst. A: Math. Anal., 10 (2003), 863–874. |
[59] |
X. Wang, A simple proof of descartes's rule of signs, Am. Math. Mon., 111 (2004), 525. https://doi.org/10.1080/00029890.2004.11920108 doi: 10.1080/00029890.2004.11920108
![]() |
[60] |
H. I. Freedman, V. S. H. Rao, The trade-off between mutual interference and time lags in predator-prey systems, Bull. Math. Biol., 45 (1983), 991–1004. https://doi.org/10.1016/S0092-8240(83)80073-1 doi: 10.1016/S0092-8240(83)80073-1
![]() |
[61] | J. K. Hale, Functional Differential Equations, Springer, New York, 1977. |
[62] | J. P. La Salle, The Stability of Dynamical Systems, Society for Industrial and Applied Mathematics, SIAM, Philadelphia, 1976. |
[63] |
H. Jiang, T. Zhang, Y. Song, Delay-induced double hopf bifurcations in a system of two delay-coupled van der pol-duffing oscillators, Int. J. Bifurcation Chaos, 25 (2015), 1550058. https://doi.org/10.1142/S0218127415500583 doi: 10.1142/S0218127415500583
![]() |
[64] |
H. Zang, T. Zhang, Y. Zhang, Stability and bifurcation analysis of delay coupled van der pol-duffing oscillators, Nonlinear Dyn., 75 (2014), 35–47. https://doi.org/10.1007/s11071-013-1047-9 doi: 10.1007/s11071-013-1047-9
![]() |
[65] |
M. Adimy, F. Crauste, S. Ruan, Periodic oscillations in leukopoiesis models with two delays, J. Theor. Biol., 242 (2006), 288–299. https://doi.org/10.1016/j.jtbi.2006.02.020 doi: 10.1016/j.jtbi.2006.02.020
![]() |
1. | Akanksha Rajpal, Sumit Kaur Bhatia, Shashank Goel, Sanyam Tyagi, Praveen Kumar, Epidemic and unemployment interplay through bi-level multi delayed mathematical model, 2025, 229, 03784754, 758, 10.1016/j.matcom.2024.10.027 | |
2. | Nabeela Anwar, Iftikhar Ahmad, Adiqa Kausar Kiani, Muhammad Shoaib, Muhammad Asif Zahoor Raja, Novel neuro-stochastic adaptive supervised learning for numerical treatment of nonlinear epidemic delay differential system with impact of double diseases, 2024, 0228-6203, 1, 10.1080/02286203.2024.2303577 | |
3. | Steve Martin Anthony, Sumit Kaur Bhatia, Mathematical modeling and dynamical analysis of an SPIR epidemic model with fuzzy parameters under environmental pollution, 2024, 2363-6203, 10.1007/s40808-024-02164-3 | |
4. | Moumita Ghosh, Arvind Kumar Misra, Pritha Das, Analysis of a delayed MISCR rumor spread model with refutation mechanism, 2024, 139, 2190-5444, 10.1140/epjp/s13360-024-05339-0 | |
5. | Shashank Goel, Sumit Kaur Bhatia, Jai Prakash Tripathi, Sarita Bugalia, Mansi Rana, Vijay Pal Bajiya, SIRC epidemic model with cross-immunity and multiple time delays, 2023, 87, 0303-6812, 10.1007/s00285-023-01974-w | |
6. | Ye Xia, An individual-level probabilistic model and solution for control of infectious diseases, 2024, 21, 1551-0018, 7253, 10.3934/mbe.2024320 | |
7. | Sanyam Tyagi, Sumit Kaur Bhatia, Adira Das, Tripti Mittal, Dynamic analysis of a diabetes mathematical model including heredity parameter and multiple time delays, 2024, 0924-090X, 10.1007/s11071-024-10776-8 | |
8. | Saduri Das, Tapan Sarkar, Pankaj Biswas, Exploring the stability of equilibria and Hopf-bifurcation in a tuberculosis model with delayed treatment, 2025, 100, 0031-8949, 015271, 10.1088/1402-4896/ad9d91 | |
9. | Deepika Solanki, Sumit Kaur Bhatia, Harendra Pal Singh, Praveen Kumar, Dynamic analysis of a communicable disease fractional order model incorporating vaccination and multiple time delays, 2025, 116, 11100168, 147, 10.1016/j.aej.2024.12.055 | |
10. | Bharat Kaushik, Sumit Kaur Bhatia, Sanyam Tyagi, Shashank Goel, Understanding the impact of media and latency in information response on the disease propagation: a mathematical model and analysis, 2025, 14, 2161-962X, 10.1515/em-2024-0011 |