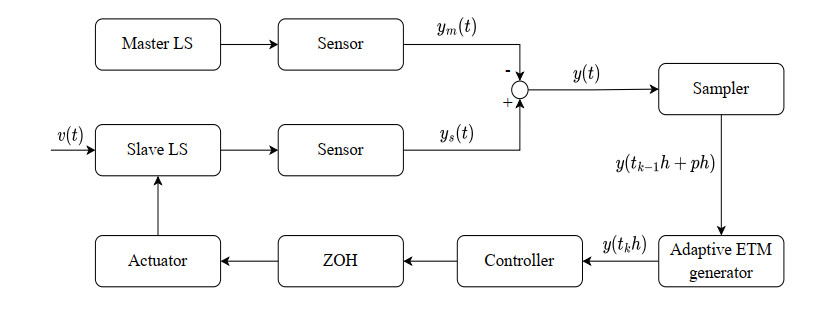
We explore the master-slave chaos synchronization of stochastic time-delay Lur'e systems within a networked environment. To tackle the challenges posed by potential mode-mismatch behavior and limited networked channel resources, an asynchronous and adaptive event-triggered (AAET) controller is employed. A criterion on the stochastic stability and L2−L∞ disturbance-suppression performance of the synchronization-error system is proposed by using a Lyapunov-Krasovskii functional, a Wirtinger-type inequality, the Itô formula, as well as a convex combination inequality. Then, a method for determining the desired AAET controller gains is proposed by decoupling the nonlinearities that arise from the Lyapunov matrices and controller gains. Finally, the applicability of the AAET control approach is validated by a Chua's circuit.
Citation: Xinling Li, Xueli Qin, Zhiwei Wan, Weipeng Tai. Chaos synchronization of stochastic time-delay Lur'e systems: An asynchronous and adaptive event-triggered control approach[J]. Electronic Research Archive, 2023, 31(9): 5589-5608. doi: 10.3934/era.2023284
[1] | Yawei Liu, Guangyin Cui, Chen Gao . Event-triggered synchronization control for neural networks against DoS attacks. Electronic Research Archive, 2025, 33(1): 121-141. doi: 10.3934/era.2025007 |
[2] | Chengbo Yi, Jiayi Cai, Rui Guo . Synchronization of a class of nonlinear multiple neural networks with delays via a dynamic event-triggered impulsive control strategy. Electronic Research Archive, 2024, 32(7): 4581-4603. doi: 10.3934/era.2024208 |
[3] | Dandan Zuo, Wansheng Wang, Lulu Zhang, Jing Han, Ling Chen . Non-fragile sampled-data control for synchronizing Markov jump Lur'e systems with time-variant delay. Electronic Research Archive, 2024, 32(7): 4632-4658. doi: 10.3934/era.2024211 |
[4] | Zongying Feng, Guoqiang Tan . Dynamic event-triggered $ H_{\infty} $ control for neural networks with sensor saturations and stochastic deception attacks. Electronic Research Archive, 2025, 33(3): 1267-1284. doi: 10.3934/era.2025056 |
[5] | Xingyue Liu, Kaibo Shi, Yiqian Tang, Lin Tang, Youhua Wei, Yingjun Han . A novel adaptive event-triggered reliable $ H_\infty $ control approach for networked control systems with actuator faults. Electronic Research Archive, 2023, 31(4): 1840-1862. doi: 10.3934/era.2023095 |
[6] | Jun Guo, Yanchao Shi, Weihua Luo, Yanzhao Cheng, Shengye Wang . Exponential projective synchronization analysis for quaternion-valued memristor-based neural networks with time delays. Electronic Research Archive, 2023, 31(9): 5609-5631. doi: 10.3934/era.2023285 |
[7] | Jiaqi Chang, Xiangxin Yin, Caoyuan Ma, Donghua Zhao, Yongzheng Sun . Estimation of the time cost with pinning control for stochastic complex networks. Electronic Research Archive, 2022, 30(9): 3509-3526. doi: 10.3934/era.2022179 |
[8] | Yu-Jing Shi, Yan Ma . Finite/fixed-time synchronization for complex networks via quantized adaptive control. Electronic Research Archive, 2021, 29(2): 2047-2061. doi: 10.3934/era.2020104 |
[9] | Yilin Li, Jianwen Feng, Jingyi Wang . Mean square synchronization for stochastic delayed neural networks via pinning impulsive control. Electronic Research Archive, 2022, 30(9): 3172-3192. doi: 10.3934/era.2022161 |
[10] | Xiaoming Wang, Yunlong Bai, Zhiyong Li, Wenguang Zhao, Shixing Ding . Observer-based event triggering security load frequency control for power systems involving air conditioning loads. Electronic Research Archive, 2024, 32(11): 6258-6275. doi: 10.3934/era.2024291 |
We explore the master-slave chaos synchronization of stochastic time-delay Lur'e systems within a networked environment. To tackle the challenges posed by potential mode-mismatch behavior and limited networked channel resources, an asynchronous and adaptive event-triggered (AAET) controller is employed. A criterion on the stochastic stability and L2−L∞ disturbance-suppression performance of the synchronization-error system is proposed by using a Lyapunov-Krasovskii functional, a Wirtinger-type inequality, the Itô formula, as well as a convex combination inequality. Then, a method for determining the desired AAET controller gains is proposed by decoupling the nonlinearities that arise from the Lyapunov matrices and controller gains. Finally, the applicability of the AAET control approach is validated by a Chua's circuit.
Chaotic systems exhibit intricate nonlinear dynamics, characterized by features such as pseudo-random behavior and a heightened sensitivity to initial conditions. Lur'e systems (LSs), which encompass a wide range of chaotic systems such as Chua's circuits [1] neural networks [2], and n-scroll attractors [3], have attracted significant research attention in the past decades. Chaos synchronization of LSs has found applications in diverse areas including image encryption [4,5,6], cryptography [7,8] and confidential communication [9,10,11]. The existence of time delay and stochastic perturbations are often unavoidable in a real-world control system and can cause the system to be unstable. Correspondingly, substantial efforts have been devoted to chaos synchronization of stochastic time-delay LSs, and a few results have been reported [12,13,14,15].
When implementing chaos synchronization in a networked environment, network-induced phenomena, such as packet losses [16], link failures [17] and cyber attacks [18,19,20], can introduce inconsistencies between the system and the controller/filter modes [21]. Therefore, researchers have shown interest in studying chaos synchronization using asynchronous/mode-unmatched controllers. The hidden Markov model (HMM), proposed by Rabiner [22], serves as a suitable tool for describing the asynchronous phenomena. Building upon the HMM framework, Li et al. [23] investigated the synchronization control in Markov-switching neural networks, while Ma et al. [24] explored the drive-response synchronization in fuzzy complex dynamic networks. Despite these advancements, to our knowledge, there is a scarcity of research addressing chaos synchronization in stochastic time-delay LSs under asynchronous controllers.
Moreover, in networked control systems, the bandwidth of the communication network is usually limited, which can impact the control performance significantly. To eliminate unnecessary resource waste and achieve efficient resource allocation, recent studies on chaos synchronization of LSs have utilized event-triggered mechanism (ETM)-based control methods. For instance, Wu et al. [25] conducted research on exponential synchronization and joint performance issues of chaotic LSs by designing a switching ETM based on perturbation terms. He et al. [26] proposed a secure communication scheme through synchronized chaotic neural networks based on quantized output feedback ETM. Besides, several studies on memory-based ETM for chaos synchronization in LSs have been reported in [27,28,29]. Notably, the above literature employed fixed thresholds in the designed ETM, limiting their ability to conserve communication resources.
Motivated by the above discussion, we explore the master-slave chaos synchronization of stochastic time-delay LSs within an asynchronous and adaptive event-triggered (AAET) control framework. Unlike previous works [25,26,27,28,29], the thresholds of the ETM used can be adjusted adaptively with real-time system states. The objective is to determine the required AAET controller gains to ensure that the synchronization-error system (SES) has both stochastic stability (SS) and L2−L∞ disturbance-suppression performance (LDSP) [30]. The contributions of this paper are:
1) The AAET controller is first applied to tackle the chaos synchronization issue of stochastic time-delay LSs;
2) A criterion on the SS and LDSP is proposed using a Lyapunov-Krasovskii functional (LKF), a Wirtinger-type inequality, the Itô formula, as well as a convex combination inequality (CCI);
3) A method for determining the desired AAET controller is proposed by decoupling the nonlinearities that arise from the Lyapunov matrices and controller gains.
Throughout, we employ Rn1, Rn1×n2 and N to stand for the n1-dimensional Euclidean space, the set of all n1×n2 real matrices, and the set of natural numbers, respectively. The symbol ‖⋅‖ is the Euclidean vector norm, (⋅)T represents the matrix transposition, He(G) means the sum of matrix G and its transpose GT, ∗ indicates the symmetric term in a matrix and E{⋅} and Prob{⋅} indicate, respectively, the expectation and probability operator. Furthermore, we utilize col{⋅} to denote a column vector, diag{⋅} to stand for a block-diagonal matrix and sup{⋅} and inf{⋅} to indicate the supremum and infimum of a set of real numbers, respectively.
Consider the following master-slave stochastic time-delay LSs:
{dxm(t)=[Aα(t)xm(t)+Bα(t)xm(t−τ(t))+Wα(t)ψ(Fxm(t))]dt+Dα(t)xm(t)dϖ(t),ym(t)=Cα(t)xm(t),xm(t)=ξm0,t∈[−τ2,0], | (2.1) |
{dxs(t)=[Aα(t)xs(t)+Bα(t)xs(t−τ(t))+Wα(t)ψ(Fxs(t))+u(t)+Eα(t)v(t)]dt+Dα(t)xs(t)dϖ(t),ys(t)=Cα(t)xs(t),xs(t)=ξs0,t∈[−τ2,0] | (2.2) |
with xm(t)∈Rnx and xs(t)∈Rnx being the state vectors, ξm0 and ξs0 being the initial values, ym(t)∈Rny and ys(t)∈Rny being the output vectors, ψ(⋅)∈Rq being the nonlinear term with all components within [ˊgr,ˊgr] for r=1,2,⋯,q, u(t)∈Rq being the control signal to be designed, v(t) being the disturbance in L2[0,∞) [31], one-dimension Brownian motion ϖ(t) satisfying E{dϖ(t)}=0 and E{dϖ2(t)}=dt, and time-varying delay τ(t) satisfying 0<τ1⩽τ(t)⩽τ2 and μ1⩽˙τ(t)⩽μ2, where τ1, τ2, μ1, and μ2 are constants. {α(t),t⩾0} is the Markovian process and belongs to the state space N={1,2,⋯,N}. The transition rate (TR) matrix and transition probability is given by Π=[πij]N×N and
Prob{α(t+ς)=j|α(t)=i}={πijς+o(ς),i≠j.1+πiiς+o(ς),i=j, |
with ς>0, limς→0o(ς)/ς=0, πij⩾0, i≠j and πii=−∑Ni=1,i≠jπij [32,33,34]. Aα(t), Bα(t), Cα(t), Dα(t), Eα(t) and Wα(t), which can be abbreviated as Ai, Bi, Ci, Di, Ei, and Wi for α(t)=i∈N, are matrices with appropriate dimensions.
In order to minimize information transmission, the adaptive ETM is employed to determine whether the current sampled data should be transmitted to the controller, as illustrated in Figure 1. Let tkh denote the latest transmission time of the output signal, where h>0 is the sampling period. y(tkh) and y(t) denote the latest output signal and the current one, respectively. Defining e(t)=y(tkh)−y(t), the event-triggered condition is designed as follows:
t0h=0,tk+1h=tkh+infp∈N{ph|eT(t)Ψie(t)<ρ(t)yT(tkh)Ψiy(tkh)},t∈[tkh,tk+1h), | (2.3) |
where Ψi>0 is the trigger matrix and ρ(t) is a threshold parameter adjusted by an adaptive law as
ρ(t)=ρ1+(ρ2−ρ1)2πarccot(κ‖e(t)‖2), | (2.4) |
where ρ1 and ρ2 are predetermined parameters with 0<ρ1⩽ρ2<1, κ is a positive scalar used to adjust the sensitivity of the function ‖e(k)‖2.
Remark 1. The value of ρ(t) has a certain influence on the trigger condition (2.3). The trigger condition will be stricter with the value of ρ(t) being higher, resulting in less data being transmitted to the controller. Conversely, the trigger condition will be relaxed with the value of ρ(t) being lower, allowing more data to be transmitted. Furthermore, it is worth to mention that, when ρ(t) takes a constant on (0,1], the adaptive ETM will be transformed into the periodic ETM (PETM); when ρ(t) is set as 0, it will be transformed into the sampled-data mechanism (SDM).
Remark 2. The arccot function, incorporated in the adaptive law (2.4), enables the threshold parameter of ETM to be dynamically adjusted as the output error changes within the range of (ρ1,ρ2]. The presence of the arccot function results in an inverse relationship between the threshold parameter ρ(t) and the output error e(t). It can be observed that ρ(t) tends to ρ2 as ‖e(t)‖2 approaches 0, and ρ(t) tends to ρ1 as ‖e(t)‖2 approaches ∞.
Consider the following controller:
u(t)=Kβ(t)(ym(tkh)−ys(tkh)),t∈[tkh,tk+1h). | (2.5) |
Unlike [35,36,37], the controller is based on output feedback, which is known to be more easily implemented. In the controller, we introduce another stochastic variable with state space M={1,2,⋯,M} to describe this stochastic process {β(t),t⩾0}. The conditional transition probability (CTP) matrix is signed as Φ=[φiι]N×M with
φiι=Prob{β(t)=ι|α(t)=i} |
and ∑Mι=1φiι=1. Kβ(t), which will be abbreviated as Kι, is the controller gain to be designed. Note that {{α(t),t⩾0}{β(t),t⩾0}} constitutes a HMM [38,39].
Define x(t)=xm(t)−xs(t). Then, from (2.1), (2.2) and (2.5), one can establish the following SES:
dx(t)=F(t)dt+G(t)dϖ(t),t∈[tkh,tk+1h), | (2.6) |
where F(t)=(Ai−KιCi)x(t)+Bix(t−τ(t))+Wi˜ψ(Fx(t))−Kιe(t)−Eiv(t), G(t)=Dix(t), ˜ψ(Fx(t))=ψ(F(x(t)+xs(t)))−ψ(Fxs(t)) that satisfies
ˊgr⩽ψr(fTr(x+xs))−ψr(fTrxs)fTrx=˜ψr(fTrx)fTrx⩽ˊgr,∀x,xs,r=1,2,⋯,q, | (2.7) |
where fTr indicates the r-th row of F. From (2.7), one can get
[˜ψr(fTrx)−ˊgrfTrx][˜ψr(fTrx)−ˊgrfTrx]⩽0. | (2.8) |
Remark 3. The constants ˊgr and ˊgr can be taken as positive, negative, or zero. By allowing ˊgr and ˊgr to have a wide range of values, the sector bounded nonlinearity can flexibly adapt to the needs of different systems and provide a more flexible regulation and control mechanism.
To streamline the subsequent analysis, we define
ζ(t)=col{x(t),x(t−τ1),x(t−τ(t)),x(t−τ2),ϕ1,ϕ2,ϕ3},ϱd=[0n×(d−1)nIn×n0n×(9−d)n](d=1,2,⋯,9),ϕ1=1τ1∫tt−τ1x(s)ds,ϕ2=1τ(t)−τ1∫t−τ1t−τ(t)x(s)ds,ϕ3=1τ2−τ(t)∫t−τ(t)t−τ2x(s)ds,τ12=τ2−τ1, |
and provide two definitions and four lemmas.
Definition 1. The SES (2.6) is said to be with SS if there exists a scalar M(α0,ξ(⋅)) satisfying
E{∫∞0‖x(t)‖2dt|α0,x(t)=ξ0,t∈[−τ2,0]}<M(α0,ξ(⋅)) |
when v(t)≡0.
Definition 2. Under the zero initial condition, for a given scalar γ>0, the SES (2.6) is said to have a LDSP if
E{supt⩾0{‖y(t)‖2}}⩽γ2E{∫t0‖v(s)‖2ds} |
holds for v(t)≠0.
Lemma 1. Given stochastic differential equation
dx(t)=F(t)dt+G(t)dϖ(t), | (2.9) |
where ϖ(t) is one-dimension Brownian motion, for scalars a, b (b>a), and a matrix R, one has
∫baFT(s)RF(s)ds⩾1b−aΩT(a,b)˜RΩ(a,b)+2b−aΩT(a,b)˜Rμ(a,b), |
where ˜R=diag{R,3R} and
Ω(a,b)=[x(b)−x(a)x(b)+x(a)−2b−a∫bax(s)ds],μ(a,b)=[−∫baG(s)dϖ(s)1b−a∫ba(b+a−2s)G(s)dϖ(s)]. |
Remark 4. Following the approaches used in [40,41], the Wirtinger-type inequality of Lemma 1 can be readily established. It should be noted that the integral term in μ(a,b) should be 1b−a∫ba(b+a−2s)G(s)dϖ(s) instead of 1b−a∫ba(b−a+2s)G(s)dϖ(s).
Lemma 2. [40] Consider the stochastic differential equation (2.9). For n×n real matrix R>0 and the piecewise function τ(t) satisfying 0<τ1⩽τ(t)⩽τ2, where τ1 and τ2 are two constants, and for S∈R2n×2n satisfying [˜RST∗˜R]⩾0 with ˜R=diag{R,3R}, the following CCI holds:
−τ12∫t−τ1t−τ2FT(s)RF(s)ds⩽−2℧T2S℧1−℧T1˜R℧1−℧T2˜R℧2−2℘(dϖ(t)), |
where
℧1=Ω(t−τ(t),t−τ1),℧2=Ω(t−τ2,t−τ(t)),℘(dϖ(t))=τ12τ(t)−τ1℧T1˜Rμ(t−τ(t),t−τ1)+τ12τ2−τ(t)℧T2˜Rμ(t−τ2,t−τ(t)). |
Lemma 3. [42] For any two matrices G and R>0 with appropriate dimensions,
−GTR−1G⩽R−GT−G |
holds true.
Lemma 4. [43] For a given scalar ε>0, suppose there are matrices Λ, U, V and W ensuring
[ΛU+εV∗−εX−εXT]<0. |
Then, one gets
Λ+He(UX−1VT)<0. |
In contrast to the common H∞ performance, LDSP imposes a limitation on the energy-to-peak gain from disturbance to the output signal, ensuring it does not exceed a specified disturbance-suppression index [44]. In this paper, we intend to develop an asynchronous controller in (2.5) with the adaptive ETM in (2.3) to guarantee the SS and LDSP of SES (2.6). First, we give a condition to ensure the SS and LDSP of SES (2.6).
Theorem 1. Given scalars γ>0 and ρ2, suppose that there exist matrices Pi>0, Q1>0, Q2>0, Q3>0, R1>0, R2>0, Ψi>0, diagonal matrix L>0, and matrices S, Kι, for any i∈N, ι∈M satisfying
[˜R2ST∗˜R2]⩾0, | (3.1) |
CTiCi−Pi<0, | (3.2) |
Λi=M∑c=1φiι([Λ1,1iιΛ1,2i∗−γ2I]+[˜ATiι−ETi](τ21R1+τ212R2)[˜ATiι−ETi]T)<0. | (3.3) |
Then the SES (2.6) has the SS and LDSP, where
Λ1,1iι=[Υ1,1iιΥ1,2iΥ1,3iι∗−He(L)0∗∗(ρ2−1)Ψi],Λ1,2i=[−ϱT1PiEi00],Υ1,1iι=He(ϱT1PiAiι−ϱT1FTˊGTLˊGFϱ1)+ϱT1(DTiPiDi+˜Pi)ϱ1+˜Q−2GT2SG1−GT0˜R1G0−GT1˜R2G1−GT2˜R2G2+ϱT1ρ2CTiΨiCiϱ1,Υ1,2i=ϱT1PiWi+ϱT1FT(ˊGT+FT)L,Υ1,3iι=ϱT1ρ2CTiΨi−ϱT1PiKι,˜Rd=diag{Rd,3Rd}(d=1,2),˜Aiι=[AiιWi−Kι],˜Q=diag{Q1,−Q1+Q2,(1−μ1)Q3−(1−μ2)Q2,−Q3,03n×3n},Aiι=[Ai−KιCi0Bi0000],˜Pi=N∑i=1πijPj,G0=[ϱ1−ϱ2ϱ1+ϱ2−2ϱ5],G1=[ϱ2−ϱ3ϱ2+ϱ3−2ϱ6],G2=[ϱ3−ϱ4ϱ3+ϱ4−2ϱ7]. |
Proof. Select a LKF candidate as:
V(t,x(t),α(t),β(t))=4∑k=1Vk(t,x(t),α(t),β(t)), | (3.4) |
where
V1(t,x(t),α(t),β(t))=xT(t)Pα(t)x(t),V2(t,x(t),α(t),β(t))=∫tt−τ1xT(s)Q1x(s)ds+∫t−τ1t−τ(t)xT(s)Q2x(s)ds+∫t−τ(t)t−τ2xT(s)Q3x(s)ds,V3(t,x(t),α(t),β(t))=τ1∫tt−τ1∫tvFT(s)R1F(s)dsdv+τ12∫t−τ1t−τ2∫tvFT(s)R2F(s)dsdv. |
Assume that α(t)=i, α(t+ς)=j, β(t)=ι and define L as the weak infinitesimal operator of the stochastic process {x(t),α(t)}. Then, along SES (2.6), we get
LV1(t,x(t),i,ι)=2xT(t)PiF(t)+GT(t)PiG(t)+xT(t)N∑i=1πijPjx(t)=ζT(t)[He(ϱT1PiAiι)+ϱT1(DTiPiDi+˜Pi)ϱ1]ζ(t)+2ζT(t)ϱT1PiWi˜ψ(Fx(t))−2ζT(t)ϱT1PiKιe(t)−2ζT(t)ϱT1PiEiv(t), | (3.5) |
LV2(t,x(t),i,ι)=xT(t)Q1x(t)+xT(t−τ1)(−Q1+Q2)x(t−τ1)+(1−˙τ(t))xT(t−τ(t))(−Q2+Q3)x(t−τ(t))−xT(t−τ2)Q3x(t−τ2)⩽ζT(t)˜Qζ(t), | (3.6) |
LV3(t,x(t),i,ι)=FT(t)(τ21R1+τ212R2)F(t)−τ1∫tt−τ1FT(s)R1F(s)ds−τ12∫t−τ1t−τ2FT(s)R2F(s)ds. | (3.7) |
Define an augmented vector as ˜ζ(t)=col{ζ(t),˜ψ(Fx(t)),e(t)}. We can derive that
FT(t)(τ21R1+τ212R2)F(t)=[˜ζ(t)v(t)]T[˜ATiι−ETi](τ21R1+τ212R2)[˜ATiι−ETi]T[˜ζ(t)v(t)]. | (3.8) |
Based on (3.1), applying Lemmas 1 and 2 to the integral terms in (3.7) yields
−τ1∫tt−τ1FT(s)R1F(s)ds⩽−ζT(t)GT0˜R1G0ζ(t)−2ζT(t)GT0˜R1μ(t−τ1,t), | (3.9) |
−τ12∫t−τ1t−τ2FT(s)R2F(s)ds⩽ζT(t)[−2GT2SG1−GT1˜R2G1−GT2˜R2G2]ζ(t)−2℘(dϖ(t)), | (3.10) |
where ℘(dϖ(t))=τ12τ(t)−τ1ζT(t)GT1˜R2μ(t−τ(t),t−τ1)+τ12τ2−τ(t)ζT(t)GT2˜R2μ(t−τ2,t−τ(t)). Recalling (3.7)–(3.10), we have
LV3(t,x(t),i,ι)⩽[˜ζ(t)v(t)]T[˜ATiι−ETi](τ21R1+τ212R2)[˜ATiι−ETi]T[˜ζ(t)v(t)]+˜℘(dϖ(t))+ζT(t)[−GT0˜R1G0−2GT2SG1−GT1˜R2G1−GT2˜R2G2]ζ(t), | (3.11) |
where ˜℘(dϖ(t))=−2ζT(t)GT0˜R1μ(t−τ1,t)−2℘(dϖ(t)). It follows from (2.8) that
−2(˜ψ(Fx(t))−ˊGFx(t))TL(˜ψ(Fx(t))−ˊGFx(t))⩾0, |
which means
0⩽−2(˜ψ(Fx(t))−ˊGFϱ1ζ(t))TL(˜ψ(Fx(t))−ˊGFϱ1ζ(t))=−˜ψT(Fx(t))He(L)˜ψ(Fx(t))+2ζT(t)ϱT1FT(ˊGT+ˊGT)L˜ψ(Fx(t))−ζT(t)He(ϱT1FTˊGTLˊGFϱ1)ζ(t). | (3.12) |
In addition, recalling the adaptive ETM (2.3), we obtain
0⩽ρ(t)yT(tkh)Ψiy(tkh)−eT(t)Ψie(t)⩽ρ2[y(t)+e(t)]TΨi[y(t)+e(t)]−eT(t)Ψie(t)=ρ2[xT(t)CTiΨiCix(t)+2xT(t)CTiΨie(t)+eTΨie(t)]−eT(t)Ψie(t)=ζT(t)ϱT1ρ2CTiΨiCiϱ1ζ(t)+2ζT(t)ϱT1ρ2CTiΨie(t)+eT(t)(ρ2−1)Ψie(t). | (3.13) |
Combining (3.5), (3.6), (3.11), (3.12) and (3.13), we find that
LV(t,x(t),i,ι)⩽ζT(t)Υ1,1iιζ(t)+2ζT(t)Υ1,2i˜ψ(Fx(t))+2ζT(t)Υ1,3iιe(t)−2ζT(t)ϱT1PiEiv(t)+eT(t)(ρ2−1)Ψie(t)−˜ψT(Fx(t))He(L)˜ψ(Fx(t))+˜℘(dϖ(t)+[˜ζ(t)v(t)]T[˜ATiι−ETi](τ21R1+τ212R2)[˜ATiι−ETi]T[˜ζ(t)v(t)]. | (3.14) |
Noting E{˜℘(dϖ(t))}=0, we can calculate E{LV(t,x(t),i,ι)} by (3.14) that
E{LV(t,x(t),i,ι)}⩽[˜ζ(t)v(t)]TD∑c=1φiι([Λ1,1iιΛ2,2i∗0]+[˜ATiι−ETi](τ21R1+τ212R2)[˜ATiι−ETi]T)[˜ζ(t)v(t)]T. | (3.15) |
Next, we discuss the SS of (2.6) under the condition of v(t)≡0 and the LDSP of (2.6) under the condition of v(t)≠0, respectively.
i) v(t)≡0, we can get the following inequality from (3.15):
E{LV(t,x(t),i,ι)}⩽˜ζT(t)Λ0i˜ζ(t), |
where Λ0i=∑Mc=1φiι(Λ1,1iι+˜ATiι(τ21R1+τ212R2)˜Aiι). From (3.3), we can find that there exists a>0 such that for any
E{LV(t,x(t),i,ι)}⩽−a‖x(t)‖2,i∈N. |
Utilizing the Itô formula, we obtain
E{V(t,x(t),α(t))}−E{V(0,x0,α0)}=E{∫t0V(s,x(s),α(s))ds}⩽−aE{∫t0‖x(s)‖2ds}, |
which means
E{∫t0‖x(s)‖2ds}⩽1aV(0,x0,α0). |
Thus, the SS of (2.6) is proved.
ii) v(t)≠0, under the zero initial condition, defining J(t)=yT(t)y(t)−γ2∫t0vT(s)v(s)ds leads to
J(t)=yT(t)y(t)−γ2∫t0vT(s)v(s)ds+E{∫t0LV(s,x(s),i,ι)ds}−(V(t,x(t),i,ι)−V(0,x0,α0,β0))⩽xT(t)(CTiCi−Pi)x(t)+∫t0[˜ζ(s)v(s)]TΛi[˜ζ(s)v(s)]ds. |
From conditions (3.2) and (3.3), we know that J(t)⩽0. According to Definition 2, the SES (2.6) has a LDSP γ.
On the basis of Theorem 1, a design method of the AAET controller can be proposed.
Theorem 2. Given scalars ε, ρ2 and γ>0, suppose that there exist matrices Pi>0, Q1>0, Q2>0, Q3>0, R1>0, R2>0, Ψi>0, diagonal matrix L>0, matrices S, Xι, Yι, and Tiι for any i∈N, ι∈M satisfying (3.1), (3.2), and
[−He(Tiι+Yι)Pi−Xι−εYTι∗−εXι−εXTι]<0, | (3.16) |
Θi=M∑c=1φiι[Θ1,1iιΛ1,2iΘ1,3iΘ1,4i∗−γ2IΘ2,3iΘ2,4i∗∗Θ3,3i0∗∗∗Θ4,4i]<0, | (3.17) |
where
Θ1,1iι=[˜Υ1,1iιΥ1,2i˜Υ1,3iι∗−He(L)0∗∗(ρ2−1)Ψi],Λ1,2i=[−ϱT1PiEi00],Θ1,3i=[τ1√φi1˜ℵTi1⋯τ1√φiM˜ℵTiM],Θ1,4i=[τ12√φi1˜ℵTi1⋯τ12˜ℵTiM],Θ2,3i=[−τ1√φi1ETiPi⋯−τ1√φiMETiPi],Θ2,4i=[−τ12√φi1ETiPi⋯−τ12√φiMETiPi],Θ3,3i=diag{R1−2Pi,⋯,R1−2Pi},Θ4,4i=diag{R2−2Pi,⋯,R2−2Pi},˜Υ1,1iι=He(ϱT1ℵiι−ϱT1FTˊGTLˊGFϱ1)+ϱT1(DTiPiDi+˜Pi)ϱ1+˜Q−2GT2SG1−GT0˜R1G0−GT1˜R2G1−GT2˜R2G2+ϱT1ρ2CTiΨiCiϱ1,Υ1,2i=ϱT1PiWi+ϱT1FT(ˊGT+ˊGT)L,˜Υ1,3iι=ϱT1ρ2CTiΨi+ϱT1Tiι,˜ℵiι=[ℵiιPiWiTiι],ℵiι=[PiAi+TiιCi0PiBi0000],˜Pi=N∑i=1πijPj,˜Rd=diag{Rd,3Rd}(d=1,2),˜Q=diag{Q1,−Q1+Q2,(1−μ1)Q3−(1−μ2)Q2,−Q3,03n×3n},G0=[ϱ1−ϱ2ϱ1+ϱ2−2ϱ5],G1=[ϱ2−ϱ3ϱ2+ϱ3−2ϱ6],G2=[ϱ3−ϱ4ϱ3+ϱ4−2ϱ7]. |
Then, AAET controller (2.5) with gains
(3.18) |
enables the SES (2.6) to have both SS and LDSP.
Proof. According to (3.18), we have by applying Lemma 4 to (3.16). Then, we can conclude that and lead to
(3.19) |
(3.20) |
(3.21) |
where
Based on Lemma 3, we can get that and , which means
(3.22) |
(3.23) |
where , . Due to , combining (3.19)–(3.23) yields
(3.24) |
where
By applying Schur's complement to (3.24), we find that (3.3) can be guaranteed by (3.17). The proof is completed.
Remark 5. The coupling between parameter and the controller gain in Theorem 1 is addressed by introducing a slack matrix . It is worth mentioning that directly setting the coupling term equal to the matrix (i.e. ) would result in non-uniqueness of controller gains . In this paper, we introduce such that . By designing the controller gains, as given in equation (3.18), and combining it with Lemma 4, the aforementioned issue is avoided.
Remark 6. Theorem 1 provides a analysis result of the SS and LDSP for SES (2.6) based on HMM, while Theorem 2 presents a design scheme for the needed AAET controller. The proofs of these theorems involve the use of the LKF in (3.4), Itô formula, as well as the inequalities in Lemmas 1-4. To further reduce the conservatism of the obtained results, one may refer to the augmented LKFs in [45,46], the free-matrix-based approaches in [47,48,49], the refined CCIs in [50,51] and the decoupling methods in [52].
Example 1. In this example, we consider a three-mode Chua's circuit given by
where parameters , , , and , , , are listed in Table 1. The nonlinear characteristics belonging to sector , and . The circuit model can be re-expressed as LS (2.1) with
i | ||||
1 | 9 | 14.28 | 0.1 | 0.01 |
2 | 8 | 10 | 0.05 | 0.01 |
3 | 9 | 14 | 0.15 | 0.01 |
The delay parameters are specified as , , and , the parameters related to the adaptive law are given by , , and the TR and CTP matrices are chosen as
By solving the LMIs in Theorem 2, the optimal LDSP is found to be , and the controller gains and adaptive ETM weight matrices are calculated as
We set the initial states as , and the disturbance as . The sampling period is chosen to be . A possible mode evolution of system and AAET controller is drawn in Figure 2. When there is no controller applied, we can find from Figure 3 that the system is unstable. By utilizing the devised AAET controller shown in Figure 4, the state response curves of the SES (2.6) are exhibited in Figure 5. It can be seen that the synchronization error between the master and slave LSs approaches zero over time, indicating that the master and slave LSs can successfully achieve synchronization under the presented AAET controller. The trajectory of the adaptive law and release time intervals between two trigger moments are depicted in Figures 6 and 7, respectively. Based on the simulation results, it can be observed that as the SES (2.6) stabilizes, the threshold function gradually converges to a fixed value. Define the function for LDSP. Then the trajectory of under zero initial condition is depicted in Figure 8. It is evident from Figure 8 that the maximum value of is , which is lower than .
Table 2 presents the data transmission rates under different triggering mechanisms in the same conditions. The comparison demonstrates that the adaptive ETM employed in this study can effectively reduce data transmission to achieve the goal of conserving channel resources.
SDM in [14] | PETM in [53] | Adaptive ETM in this paper | |
Number of transmitted data | 500 | 236 | 194 |
Data transmission rates | 100% | 47.20% | 38.80% |
The master-slave chaos synchronization of stochastic time-delay LSs (2.1) and (2.2) within a networked environment has been considered. To tackle the challenges posed by potential mode-mismatch behavior and limited networked channel resources, the AAET controller in (2.5) has been employed. A criterion on the SS and LDSP of the SES (2.6) has been proposed in Theorem 1 using a LKF, a Wirtinger-type inequality, the Itô formula, as well as a CCI. Then, a method for determining the desired AAET controller gains has been proposed in Theorem 2 by decoupling the nonlinearities that arise from the Lyapunov matrices and controller gains. Finally, simulation results have confirmed that the designed controller can achieve chaos synchronization between the master LS (2.1) and slave LS (2.2), while significantly reducing data transmission.
The authors declare they have not used Artificial Intelligence (AI) tools in the creation of this article.
This work was supported by the Key Research and Development Project of Anhui Province (Grant No. 202004a07020028).
All authors declare no conflicts of interest in this paper.
[1] |
L. O. Chua, L. Kocarev, K. Eckert, M. Itoh, Experimental chaos synchronization in Chua's circuit, Int. J. Bifurcat. Chaos, 2 (1992), 705–708. https://doi.org/10.1142/S0218127492000811 doi: 10.1142/S0218127492000811
![]() |
[2] |
H. Sompolinsky, A. Crisanti, H. J. Sommers, Chaos in random neural networks, Phys. Rev. Lett., 61 (1988), 259. https://doi.org/10.1103/PhysRevLett.61.259 doi: 10.1103/PhysRevLett.61.259
![]() |
[3] | J. A. K. Suykens, A. Huang, L. O. Chua, A family of n-scroll attractors from a generalized Chua's circuit, AEU Int. J. Electron. Commun., 51 (1997), 131–137. |
[4] |
Y. Fan, Z. Wang, X. Huang, H. Shen, Using partial sampled-data information for synchronization of chaotic Lur'e systems and its applications: an interval-dependent functional method, Inform. Sci., 619 (2023), 358–373. https://doi.org/10.1016/j.ins.2022.11.066 doi: 10.1016/j.ins.2022.11.066
![]() |
[5] |
L. Wang, S. Jiang, M. F. Ge, C. Hu, J. Hu, Finite-/fixed-time synchronization of memristor chaotic systems and image encryption application, IEEE Trans. Circuits-I, 68 (2021), 4957–4969. https://doi.org/10.1109/TCSI.2021.3121555 doi: 10.1109/TCSI.2021.3121555
![]() |
[6] |
S. Moon, J. J. Baik, J. M. Seo, Chaos synchronization in generalized Lorenz systems and an application to image encryption, Commun. Nonlinear Sci., 96 (2021), 105708. https://doi.org/10.1016/j.cnsns.2021.105708 doi: 10.1016/j.cnsns.2021.105708
![]() |
[7] |
M. Roohi, C. Zhang, Y. Chen, Adaptive model-free synchronization of different fractional-order neural networks with an application in cryptography, Nonlinear Dyn., 100 (2020), 3979–4001. https://doi.org/10.1007/s11071-020-05719-y doi: 10.1007/s11071-020-05719-y
![]() |
[8] |
V. K. Yadav, V. K. Shukla, S. Das, Exponential synchronization of fractional-order complex chaotic systems and its application, Chaos Solitons Fractals, 147 (2021), 110937. https://doi.org/10.1016/j.chaos.2021.110937 doi: 10.1016/j.chaos.2021.110937
![]() |
[9] |
S. Lakshmanan, M. Prakash, C. P. Lim, R. Rakkiyappan, P. Balasubramaniam, S. Nahavandi, Synchronization of an inertial neural network with time-varying delays and its application to secure communication, IEEE Trans. Neural Net. Learn. Syst., 29 (2018), 195–207. https://doi.org/10.1109/TNNLS.2016.2619345 doi: 10.1109/TNNLS.2016.2619345
![]() |
[10] |
A. M. Gonzalez-Zapata, E. Tlelo-Cuautle, I. Cruz-Vega, W. D. León-Salas, Synchronization of chaotic artificial neurons and its application to secure image transmission under MQTT for IoT protocol, Nonlinear Dyn., 104 (2021), 4581–4600. https://doi.org/10.1007/s11071-021-06532-x doi: 10.1007/s11071-021-06532-x
![]() |
[11] |
W. Shao, Y. Fu, M. Cheng, L. Deng, D. Liu, Chaos synchronization based on hybrid entropy sources and applications to secure communication, IEEE Photonic. Tech. Lett., 33 (2021), 1038–1041. https://doi.org/10.1109/LPT.2021.3093584 doi: 10.1109/LPT.2021.3093584
![]() |
[12] |
Q. Li, X. Liu, Q. Zhu, S. Zhong, J. Cheng, Stochastic synchronization of semi-Markovian jump chaotic Lur'e systems with packet dropouts subject to multiple sampling periods, J. Franklin I., 356 (2019), 6899–6925. https://doi.org/10.1016/j.jfranklin.2019.06.005 doi: 10.1016/j.jfranklin.2019.06.005
![]() |
[13] |
Y. Li, J. Feng, J. Wang, Mean square synchronization for stochastic delayed neural networks via pinning impulsive control, Electron. Res. Arch., 30 (2022), 3172–3192. http://dx.doi.org/10.3934/era.2022161 doi: 10.3934/era.2022161
![]() |
[14] |
T. Yang, Z. Wang, J. Xia, H. Shen, Sampled-data exponential synchronization of stochastic chaotic Lur'e delayed systems, Math. Comput. Simulation, 203 (2023), 44–57. https://doi.org/10.1016/j.matcom.2022.06.010 doi: 10.1016/j.matcom.2022.06.010
![]() |
[15] |
Y. Zhou, X. H. Chang, W. Huang, Z. M. Li, Quantized extended dissipative synchronization for semi-Markov switching Lur'e systems with time delay under deception attacks, Commun. Nonlinear Sci., 117 (2023), 106972. https://doi.org/10.1016/j.cnsns.2022.106972 doi: 10.1016/j.cnsns.2022.106972
![]() |
[16] |
X. H. Chang, Y. Liu, Quantized output feedback control of AFS for electric vehicles with transmission delay and data dropouts, IEEE Trans. Intell. Transp. Syst., 23 (2022), 16026–16037. https://doi.org/10.1109/TITS.2022.3147481 doi: 10.1109/TITS.2022.3147481
![]() |
[17] |
C. Jia, J. Hu, H. Liu, J. Du, S. Feng, Recursive state estimation for a class of nonlinear uncertain coupled complex networks subject to random link failures and packet disorders, ISA Trans., 127 (2022), 88–98. https://doi.org/10.1016/j.isatra.2021.12.036 doi: 10.1016/j.isatra.2021.12.036
![]() |
[18] |
D. Xu, X. Li, W. Tai, J. Zhou, Event-triggered stabilization for networked control systems under random occurring deception attacks, Math. Biosci. Eng., 20 (2023), 859–878. http://dx.doi.org/10.3934/mbe.2023039 doi: 10.3934/mbe.2023039
![]() |
[19] |
J. Zhou, D. Xu, W. Tai, C. K. Ahn, Switched event-triggered security control for networked systems vulnerable to aperiodic DoS attacks, IEEE Trans. Net. Sci. Eng., 10 (2023), 2109–2123. https://doi.org/10.1109/TNSE.2023.3243095 doi: 10.1109/TNSE.2023.3243095
![]() |
[20] | Y. Fan, X. Huang, Y. Li, H. Shen, Sampled-data-based secure synchronization control for chaotic Lur'e systems subject to Denial-of-Service attacks, IEEE Trans. Neural Net. Learn. Syst., 2022 (2022). https://doi.org/10.1109/TNNLS.2022.3203382 |
[21] |
Y. Xu, Z. G. Wu, Y. J. Pan, J. Sun, Resilient asynchronous state estimation for Markovian jump neural networks subject to stochastic nonlinearities and sensor saturations, IEEE Trans. Cybern., 52 (2022), 5809–5818. https://doi.org/10.1109/TCYB.2020.3042473 doi: 10.1109/TCYB.2020.3042473
![]() |
[22] |
L. R. Rabiner, A tutorial on hidden Markov models and selected applications in speech recognition, Proc. IEEE, 77 (1989), 257–286. https://doi.org/10.1109/5.18626 doi: 10.1109/5.18626
![]() |
[23] |
F. Li, S. Song, J. Zhao, S. Xu, Z. Zhang, Synchronization control for Markov jump neural networks subject to HMM observation and partially known detection probabilities, Appl. Math. Comput., 360 (2019), 1–13. https://doi.org/10.1016/j.amc.2019.04.032 doi: 10.1016/j.amc.2019.04.032
![]() |
[24] |
C. Ma, L. Hao, H. Fu, Neural network based asynchronous synchronization for fuzzy hidden Markov jump complex dynamical networks, Complex Intell. Syst., 8 (2022), 1941–1948. https://doi.org/10.1007/s40747-021-00370-5 doi: 10.1007/s40747-021-00370-5
![]() |
[25] |
W. Wu, L. He, J. Zhou, Z. Xuan, S. Arik, Disturbance-term-based switching event-triggered synchronization control of chaotic Lurie systems subject to a joint performance guarantee, Commun. Nonlinear Sci., 115 (2022), 106774. https://doi.org/10.1016/j.cnsns.2022.106774 doi: 10.1016/j.cnsns.2022.106774
![]() |
[26] |
W. He, T. Luo, Y. Tang, W. Du, Y. C. Tian, F. Qian, Secure communication based on quantized synchronization of chaotic neural networks under an event-triggered strategy, IEEE Trans. Neural Net. Learn. Syst., 31 (2020), 3334–3345. https://doi.org/10.1109/TNNLS.2019.2943548 doi: 10.1109/TNNLS.2019.2943548
![]() |
[27] |
Y. Ni, Z. Wang, Y. Fan, X. Huang, H. Shen, Memory-based event-triggered control for global synchronization of chaotic Lur'e systems and its application, IEEE Trans. Syst. Man Cybern. Syst., 53 (2023), 1920–1931. https://doi.org/10.1109/TSMC.2022.3207353 doi: 10.1109/TSMC.2022.3207353
![]() |
[28] | Y. Ni, Z. Wang, Y. Fan, J. Lu, H. Shen, A switching memory-based event-trigger scheme for synchronization of Lur'e systems with actuator saturation: A hybrid Lyapunov method, IEEE Trans. Neural Net. Learn. Syst., 2023. https://doi.org/10.1109/TNNLS.2023.3273917 |
[29] |
Z. Gu, S. Yan, J. H. Park, X. Xie, Event-triggered synchronization of chaotic Lur'e systems via memory-based triggering approach, IEEE Trans. Circuits-II, 69 (2022), 1427–1431. https://doi.org/10.1109/TCSII.2021.3113955 doi: 10.1109/TCSII.2021.3113955
![]() |
[30] |
W. Tai, D. Zuo, Z. Xuan, J. Zhou, Z. Wang, Non-fragile filtering for a class of switched neural networks, Math. Comput. Simulation, 185 (2021), 629–645. https://doi.org/10.1016/j.matcom.2021.01.014 doi: 10.1016/j.matcom.2021.01.014
![]() |
[31] |
P. Selvaraj, O. Kwon, S. Lee, R. Sakthivel, Robust fault-tolerant control design for polynomial fuzzy systems, Fuzzy Sets Syst., 464 (2023), 108406. https://doi.org/10.1016/j.fss.2022.09.012 doi: 10.1016/j.fss.2022.09.012
![]() |
[32] |
X. Li, X. Ma, W. Tai, J. Zhou, Designing an event-triggered filter with possibly inconsistent modes for Markov jump systems, Digital Signal Process., 139 (2023), 104092. https://doi.org/10.1016/j.dsp.2023.104092 doi: 10.1016/j.dsp.2023.104092
![]() |
[33] |
J. Gu, H. Wang, W. Li, Output-feedback stabilization for stochastic nonlinear systems with Markovian switching and time-varying powers, Math. Biosci. Eng., 19 (2022), 11071–11085. http://dx.doi.org/10.3934/mbe.2022516 doi: 10.3934/mbe.2022516
![]() |
[34] |
W. Tai, X. Li, J. Zhou, S. Arik, Asynchronous dissipative stabilization for stochastic Markov-switching neural networks with completely-and incompletely-known transition rates, Neural Net., 161 (2023), 55–64. https://doi.org/10.1016/j.neunet.2023.01.039 doi: 10.1016/j.neunet.2023.01.039
![]() |
[35] |
R. Vadivel, P. Hammachukiattikul, N. Gunasekaran, R. Saravanakumar, H. Dutta, Strict dissipativity synchronization for delayed static neural networks: An event-triggered scheme, Chaos Solitons Fractals, 150 (2021), 111212. https://doi.org/10.1016/j.chaos.2021.111212 doi: 10.1016/j.chaos.2021.111212
![]() |
[36] |
X. Liu, K. Shi, Y. Tang, L. Tang, Y. Wei, Y. Han, A novel adaptive event-triggered reliable control approach for networked control systems with actuator faults, Electron. Res. Arch., 31 (2023), 1840–1862. http://dx.doi.org/10.3934/era.2023095 doi: 10.3934/era.2023095
![]() |
[37] |
L. Yao, X. Huang, Memory-based adaptive event-triggered secure control of Markovian jumping neural networks suffering from deception attacks, Sci. China Technol. Sci., 66 (2023), 468–480. https://doi.org/10.1007/s11431-022-2173-7 doi: 10.1007/s11431-022-2173-7
![]() |
[38] | J. Zhou, J. Dong, S. Xu, Asynchronous dissipative control of discrete-time fuzzy Markov jump systems with dynamic state and input quantization, IEEE Trans. Fuzzy Syst., 2023. https://doi.org/10.1109/TFUZZ.2023.3271348 |
[39] |
S. Dong, M. Liu, Adaptive fuzzy asynchronous control for nonhomogeneous Markov jump power systems under hybrid attacks, IEEE Trans. Fuzzy Syst., 31 (2023), 1009–1019. https://doi.org/10.1109/TFUZZ.2022.3193805 doi: 10.1109/TFUZZ.2022.3193805
![]() |
[40] |
J. Wang, X. M. Zhang, Y. Lin, X. Ge, Q. L. Han, Event-triggered dissipative control for networked stochastic systems under non-uniform sampling, Inform. Sci., 447 (2018), 216–228. https://doi.org/10.1016/j.ins.2018.03.003 doi: 10.1016/j.ins.2018.03.003
![]() |
[41] |
F. Zeng, Y. Wang, G. Zhuang, F. Chen, Dynamic-memory event-triggered-based controller design for singular stochastic semi-Markov jump systems against multiple cyber-attacks, Nonlinear Dyn., 110 (2022), 1559–1582. https://doi.org/10.1007/s11071-022-07728-5 doi: 10.1007/s11071-022-07728-5
![]() |
[42] | E. K. Boukas, Control of singular systems with random abrupt changes, Springer, Berlin, 2008. |
[43] |
X. H. Chang, J. H. Park, J. Zhou, Robust static output feedback control design for linear systems with polytopic uncertainties, Syst. Control Lett., 85 (2015), 23–32. https://doi.org/10.1016/j.sysconle.2015.08.007 doi: 10.1016/j.sysconle.2015.08.007
![]() |
[44] |
I. Kucukdemiral, X. Han, M. S. Erden, Robust induced optimal control of discrete-time systems having magnitude and rate-bounded actuators, ISA Trans., 129 (2022), 73–87. https://doi.org/10.1016/j.isatra.2022.02.025 doi: 10.1016/j.isatra.2022.02.025
![]() |
[45] |
H. B. Zeng, Z. L. Zhai, Y. He, K. L. Teo, W. Wang, New insights on stability of sampled-data systems with time-delay, Appl. Math. Comput., 374 (2020), 125041. https://doi.org/10.1016/j.amc.2020.125041 doi: 10.1016/j.amc.2020.125041
![]() |
[46] |
T. S. Peng, H. B. Zeng, W. Wang, X. M. Zhang, X. G. Liu, General and less conservative criteria on stability and stabilization of T–S fuzzy systems with time-varying delay, IEEE Trans. Fuzzy Syst., 31 (2023), 1531–1541. https://doi.org/10.1109/TFUZZ.2022.3204899 doi: 10.1109/TFUZZ.2022.3204899
![]() |
[47] |
W. Wang, H. B. Zeng, K. L. Teo, Y. J. Chen, Relaxed stability criteria of time-varying delay systems via delay-derivative-dependent slack matrices, J. Franklin I., 360 (2023), 6099–6109. https://doi.org/10.1016/j.jfranklin.2023.04.019 doi: 10.1016/j.jfranklin.2023.04.019
![]() |
[48] |
H. B. Zeng, Y. He, M. Wu, J. She, New results on stability analysis for systems with discrete distributed delay, Automatica, 60 (2015), 189–192. https://doi.org/10.1016/j.automatica.2015.07.017 doi: 10.1016/j.automatica.2015.07.017
![]() |
[49] |
H. B. Zeng, X. G. Liu, W. Wang, A generalized free-matrix-based integral inequality for stability analysis of time-varying delay systems, Appl. Math. Comput., 354 (2019), 1–8. https://doi.org/10.1016/j.amc.2019.02.009 doi: 10.1016/j.amc.2019.02.009
![]() |
[50] |
H. B. Zeng, H. C. Lin, Y. He, K. L. Teo, W. Wang, Hierarchical stability conditions for time-varying delay systems via an extended reciprocally convex quadratic inequality, J. Franklin I., 357 (2020), 9930–9941. https://doi.org/10.1016/j.jfranklin.2020.07.034 doi: 10.1016/j.jfranklin.2020.07.034
![]() |
[51] | H. C. Lin, H. B. Zeng, X. M. Zhang, W. Wang, Stability analysis for delayed neural networks via a generalized reciprocally convex inequality, IEEE Trans. Neural Net. Learn. Syst., 2022. https://doi.org/10.1109/TNNLS.2022.3144032 |
[52] |
W. Wang, H. B. Zeng, A looped functional method to design state feedback controllers for Lurie networked control systems, IEEE/CAA J. Autom. Sinica, 10 (2023), 1093–1095. https://doi.org/10.1109/JAS.2023.123141 doi: 10.1109/JAS.2023.123141
![]() |
[53] |
E. Tian, C. Peng, Memory-based event-triggering load frequency control for power systems under deception attacks, IEEE Trans. Cybern., 50 (2020), 4610–4618. https://doi.org/10.1109/TCYB.2020.2972384 doi: 10.1109/TCYB.2020.2972384
![]() |
1. | Dandan Zuo, Wansheng Wang, Lulu Zhang, Jing Han, Ling Chen, Non-fragile sampled-data control for synchronizing Markov jump Lur'e systems with time-variant delay, 2024, 32, 2688-1594, 4632, 10.3934/era.2024211 |
i | ||||
1 | 9 | 14.28 | 0.1 | 0.01 |
2 | 8 | 10 | 0.05 | 0.01 |
3 | 9 | 14 | 0.15 | 0.01 |