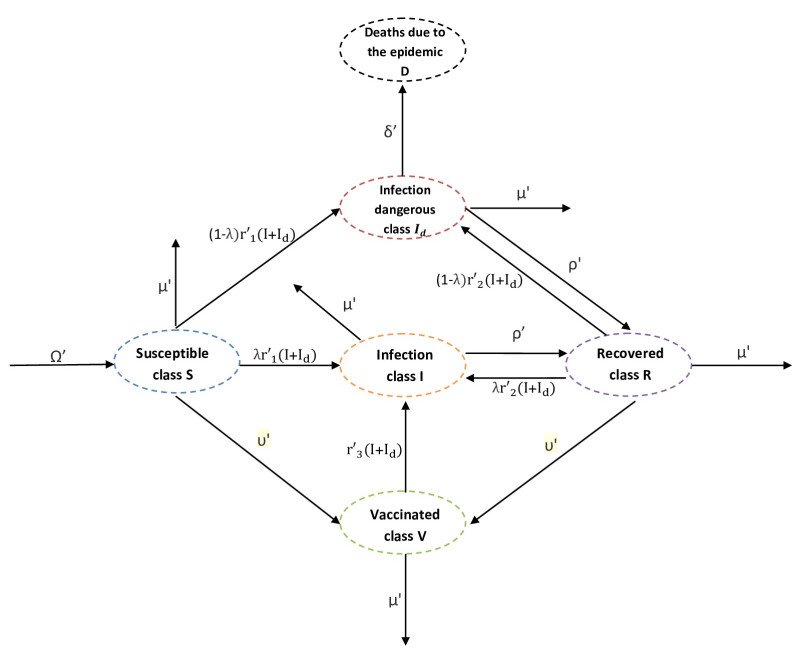
Referring tothe study of epidemic mathematical models, this manuscript presents a noveldiscrete-time COVID-19 model that includes the number of vaccinated individuals as an additional state variable in the system equations. The paper shows that the proposed compartment model, described by difference equations, has two fixed points, i.e., a disease-free fixed point and an epidemic fixed point. By considering both the forward difference system and the backward difference system, some stability analyses of the disease-free fixed point are carried out.In particular, for the backward difference system a novel theorem is proved, which gives a condition for the disappearance of the pandemic when an inequality involving some epidemic parameters is satisfied. Finally, simulation results of the conceived discrete model are carried out, along with comparisons regarding the performances of both the forward difference system and the backward difference system.
Citation: A Othman Almatroud, Noureddine Djenina, Adel Ouannas, Giuseppe Grassi, M Mossa Al-sawalha. A novel discrete-time COVID-19 epidemic model including the compartment of vaccinated individuals[J]. Mathematical Biosciences and Engineering, 2022, 19(12): 12387-12404. doi: 10.3934/mbe.2022578
[1] | A. Q. Khan, M. Tasneem, M. B. Almatrafi . Discrete-time COVID-19 epidemic model with bifurcation and control. Mathematical Biosciences and Engineering, 2022, 19(2): 1944-1969. doi: 10.3934/mbe.2022092 |
[2] | Sarafa A. Iyaniwura, Rabiu Musa, Jude D. Kong . A generalized distributed delay model of COVID-19: An endemic model with immunity waning. Mathematical Biosciences and Engineering, 2023, 20(3): 5379-5412. doi: 10.3934/mbe.2023249 |
[3] | Pannathon Kreabkhontho, Watchara Teparos, Thitiya Theparod . Potential for eliminating COVID-19 in Thailand through third-dose vaccination: A modeling approach. Mathematical Biosciences and Engineering, 2024, 21(8): 6807-6828. doi: 10.3934/mbe.2024298 |
[4] | Tao Chen, Zhiming Li, Ge Zhang . Analysis of a COVID-19 model with media coverage and limited resources. Mathematical Biosciences and Engineering, 2024, 21(4): 5283-5307. doi: 10.3934/mbe.2024233 |
[5] | Glenn Ledder . Incorporating mass vaccination into compartment models for infectious diseases. Mathematical Biosciences and Engineering, 2022, 19(9): 9457-9480. doi: 10.3934/mbe.2022440 |
[6] | Cheng-Cheng Zhu, Jiang Zhu . Spread trend of COVID-19 epidemic outbreak in China: using exponential attractor method in a spatial heterogeneous SEIQR model. Mathematical Biosciences and Engineering, 2020, 17(4): 3062-3087. doi: 10.3934/mbe.2020174 |
[7] | Hamdy M. Youssef, Najat A. Alghamdi, Magdy A. Ezzat, Alaa A. El-Bary, Ahmed M. Shawky . A new dynamical modeling SEIR with global analysis applied to the real data of spreading COVID-19 in Saudi Arabia. Mathematical Biosciences and Engineering, 2020, 17(6): 7018-7044. doi: 10.3934/mbe.2020362 |
[8] | Beatriz Machado, Liliana Antunes, Constantino Caetano, João F. Pereira, Baltazar Nunes, Paula Patrício, M. Luísa Morgado . The impact of vaccination on the evolution of COVID-19 in Portugal. Mathematical Biosciences and Engineering, 2022, 19(1): 936-952. doi: 10.3934/mbe.2022043 |
[9] | Sarita Bugalia, Jai Prakash Tripathi, Hao Wang . Estimating the time-dependent effective reproduction number and vaccination rate for COVID-19 in the USA and India. Mathematical Biosciences and Engineering, 2023, 20(3): 4673-4689. doi: 10.3934/mbe.2023216 |
[10] | Quentin Griette, Jacques Demongeot, Pierre Magal . What can we learn from COVID-19 data by using epidemic models with unidentified infectious cases?. Mathematical Biosciences and Engineering, 2022, 19(1): 537-594. doi: 10.3934/mbe.2022025 |
Referring tothe study of epidemic mathematical models, this manuscript presents a noveldiscrete-time COVID-19 model that includes the number of vaccinated individuals as an additional state variable in the system equations. The paper shows that the proposed compartment model, described by difference equations, has two fixed points, i.e., a disease-free fixed point and an epidemic fixed point. By considering both the forward difference system and the backward difference system, some stability analyses of the disease-free fixed point are carried out.In particular, for the backward difference system a novel theorem is proved, which gives a condition for the disappearance of the pandemic when an inequality involving some epidemic parameters is satisfied. Finally, simulation results of the conceived discrete model are carried out, along with comparisons regarding the performances of both the forward difference system and the backward difference system.
Epidemic models have received considerable attention over the last years [1]. They can be represented by either dynamic continuous-time or discrete-time systems, i.e., they are described by either differential or difference equations [1]. Recently, several epidemic models have been proposed with the aim to understand, describe and control the spread of the COVID-19 pandemic. These models analyse the evolution of the disease over time by dividing the communities into some compartments, which mainly include the susceptible class (S), the exposed class (E), the infected class (I) and the removed class (R). For example, in [2] a SIR model is utilized for estimating the infectivity and recovery rates from COVID-19 real data. Then, the estimated rates have been exploited to analyse the evolution of the pandemic over time. In [3] another SIR model is presented, with the aim to describe the spread of the COVID-19 epidemic in Wuhan. The model is updated with real-time input data in order to derive clinical parameters that could support public officials in decision-makings. In [4] a dynamic continuous-time model of the COVID-19 pandemic is introduced. By using phase portraits and time-series plots, the authors of reference [4] highlight the chaotic dynamics of their epidemic model. In [5] a nonlinear dynamic SIR model, which includes the effect of social distancing, is proposed to carefully analyse the spread of the COVID-19 pandemic. In [6] an accurate model that includes eight compartments (i.e., susceptible (S), infected (I), diagnosed (D), ailing (A), recognized (R), threatened (T), healed (H) and extinct (E)) is presented. The model is called SIDARTHE and introduces the distinction between diagnosed/non-diagnosed individuals, being the former isolated and, consequently, less likely to spread the infection.In [7] a COVID-19 model is illustrated, which includes the effects of media on public awareness as well as a removal rate based on the hospital-bed population ratio. The approaches to modelling the spread of the COVID-19 pandemic have become to change, at the beginning of the year 2021, by virtue of the introduction of the vaccines. Namely, a number of papers have been published, which take into account (in different ways) the role of vaccination for controlling the pandemic. Most of these models are described by differential equations, whereas very few models involve difference equations. Referring to continuous-time models, in [8] a novel SEIRS system that includes the vaccine rate is illustrated. The model clearly shows that, when the vaccine rate increases, the infection decreases and the recovered population increases over time. In [9] a continuous-time model based on eight state variables is presented, where the vaccination is considered as preventive action, rather than as a system variable. In particular, the approach in [9] highlights that vaccination is a key solution to eliminate the coronavirus among healthcare workers. In [10] an extended Kalman filter is developed to estimate the state variables of the model. In particular, the spread of COVID-19 is modelled by a set of ordinary differential equations where vaccinations represent the control input signals. In [11] a nonlinear robust control policy is exploited to analyse the role of vaccinations on the spread of the COVID-19 pandemic. The proposed continuous-time dynamic model includes eight state variables, i.e., susceptible, exposed, infected, quarantined, hospitalized, recovered, deceased and insusceptible populations. In [12] an innovative SEIR model that takes into account the effect of vaccination on the spread of COVID-19 is illustrated. Some properties of the model, including output-reach ability and non-negativity of the solution, are carefully analysed. In [13] a continuous-time SEIR model, which includes both a feedback vaccination law and an antiviral treatment control law, is illustrated. In particular, the existence of a unique disease-free equilibrium point is proved, along with the attainability of the endemic equilibrium point. In [14] a SEIR-type epidemic model with time delay and vaccination control is developed. The vaccination strategy is expressed as a state delayed feedback, which is related to the current and previous state of the epidemic model.Reference [15] is devoted to modelling and predicting COVID-19 confirmed cases through a multiple linear regression. In particular, a novel trial is illustrated, which combines both growth rates and vaccination rates in modelling the spread of the COVID-19 pandemic.
It is worth noting that discrete-time epidemic models present the advantage, over the continuous-time model, to allows a direct acquisition of recorded data, given that the sampling period (i.e., the unity), may correspond to one day or one week, depending on the available data [16]. In spite of these considerations, just a few papers have been published so far (to the best of the authors' knowledge) regarding discrete-time models that take into account the effect of vaccination on the COVID-19 pandemic. Namely, in [17] a novel discrete SEIR COVID-19 model is developed. In particular, a feedback vaccination control law is introduced to control the \textquotedblleft susceptible class\textquotedblright, in [18] the authors present a separate new epidemiological model for susceptible to have recovered infection (SEIR) subject to a two-dose feedback vaccination efforts, in [19] the authors developed a susceptible- infected- recovered- vaccine-1- vaccine-2- death model to analyze the behavior of the epidemic in Japan. Besides the fact that the topic of discrete-time models including vaccination is almost unexplored, it should be also noted that most the COVID-19 models (both continuous-time and discrete-time) published to date consider the vaccination as a control law or as a preventive action. Based on these considerations, this paper makes a contribution to the topic of discrete mathematical modelling of epidemics by introducing a new discrete-time COVID-19 model, which includes the number of vaccinated individuals as an additional state variable describing the system dynamics and it has the same characteristics as the Susceptible class. The paper shows the existence of two fixed points at most in the proposed compartment model described by difference equations, i.e., the disease-free fixed point and the epidemic fixed point. By considering both the forward difference system and the backward difference system, some stability analyses of the disease-free fixed point are carried out. In particular, for the backward difference system a theorem is proved, which gives a condition for the disappearance of the pandemic when an inequality involving some epidemic parameters is satisfied. The paper is organized as follows. In Section 2 a new discrete-time compartment model for describing the spread of the COVID-19 pandemic is illustrated. The discrete system involves five state variables, i.e., the Susceptible class S, the Recovered class R, the Infection class I, the Infection dangerous class Id and the Vaccinated class V.In Section 3it is shown that the system possesses two fixed points, i.e., the disease-free fixed point and the epidemic fixed point. Moreover, the basic reproduction number is computed. In Section 4 a stability analysis for the forward difference system is conducted, whereas in Section 5 a similar analysis is carried out for the backward difference system. In particular, in Section 5 a novel theorem is proved, which assures the global stability of the disease-free fixed point, indicating that the pandemic disappears provided that an inequality involving some epidemic parameters is satisfied. Finally, in Section 6 simulation results of the conceived discrete COVID-19 compartment model are carried out, along with comparisons regarding the performances of both the forward difference system and the backward difference system.
For the proposed model, the study population (N) is divided into two main classes: the class of individuals exposed to infection and the class of individuals infected. Each of the previous classes is also divided into secondary class, so the class of people who are exposed to infection is divided into three sub-class: people who are exposed to infection and were not previously infected and did not receive vaccination (S), people who were previously infected and recovered from the disease and are at risk of being infected again (R), and the class of vaccinated people against the epidemic (V). As for the class of infected persons, it is divided into two secondary classes: The people with good immunity and for whom infection does not pose a great risk and suppose that their ratio in the society is λ,(λ≤1), so the class of infected people from this group are going to I. The infected persons for whom the infection is dangerous and consists of the elderly, pregnant women and people with chronic diseases (whose their ratio in the society is (1−λ))Id. The transition between classes is described in the following diagram: So the mathematical model that we will be interested in, which is given in detail in [20] as follow:
{dSdt=Ω′−r′1(I(t)+Id(t))S(t)−(μ′+υ′)S(t),dRdt=ρ′(I(t)+Id(t))−r′2(I(t)+Id(t))R(t)−(υ′+μ′)R(t),dVdt=υ′(S(t)+R(t))−r′3(I(t)+Id(t))V(t)−μ′V(t),dIdt=(λ(r′1S(t)+r′2R(t))+r′3V(t))(I(t)+Id(t))−(μ′+ρ′)I(t),dIddt=(1−λ)(r′1S(t)+r′2R(t))(I(t)+Id(t))−(μ′+δ′+ρ′)Id(t).t∈R+. | (2.1) |
The proposed model's flowchart and parameters descriptors are well explained in (2.2).
![]() |
(2.2) |
Where r′i=pikN,i=1,2,3, k is the average numbers of contacts per capita (per unit of time), pi is the probabilities of contagion (p1>p2>p3) and N is the total population (It can be considered as the maximum value of the population)
Adding up the equations given in (2.1), we find
N=S+R+V+I+Id. |
In addition, the following initial conditions take into consideration:
S(0),R(0),V(0),I(0),Id(0)≥0. | (2.3) |
In real life, the statistics are discrete, so the discrete system is closer to modeling the spread of Corona virus, and in light of this we will use the following approximation:
dX(t)dt≃X(t+h)−X(t)h. | (2.4) |
System 2.1 becomes:
{S(t+h)−S(t)h=Ω′−r′1(I(t)+Id(t))S(t)−(μ′+υ′)S(t),R(t+h)−R(t)h=ρ′(I(t)+Id(t))−r′2(I(t)+Id(t))R(t)−(υ′+μ′)R(t),V(t+h)−V(t)h=υ′(S(t)+R(t))−r′3(I(t)+Id(t))V(t)−μ′V(t),I(t+h)−I(t)h=(λ(r′1S(t)+r′2R(t))+r′3V(t))(I(t)+Id(t))−(μ′+ρ′)I(t),Id(t+h)−Id(t)h=(1−λ)(r′1S(t)+r′2R(t))(I(t)+Id(t))−(μ′+δ′+ρ′)Id(t).t∈R+. |
We multiply both sides by h, and put
X(t)=X(n), |
and
X(t+h)=X(n+1), |
where n∈N,X(t)=(S(t),R(t),V(t),I(t),Id(t))t. And we also put
Ω=hΩ′,μ=hμ′,ri=hr′i,i=1,2,3,ρ=hρ′,υ=hυ′,δ=hδ′. |
We finally get the following system:
ΔX(n)=F(X(n)),n∈N, | (2.5) |
where
F(X(n))=(F1(X(n))F2(X(n))F3(X(n))F4(X(n))F5(X(n)))=(Ω−r1(I(n)+Id(n))S(t)−(μ+υ)S(n)ρ(I(n)+Id(n))−r2(I(n)+Id(n))R(n)−(υ+μ)R(n)υ(S(n)+R(n))−r3(I(n)+Id(n))V(n)−μV(n)(λ(r1S(n)+r2R(n))+r3V(n))(I(n)+Id(n))−(μ+ρ)I(n)(1−λ)(r1S(n)+r2R(n))(I(n)+Id(n))−(μ+δ+ρ)Id(n)), |
and where Δ is the forward difference operator (ΔX(n)=X(n+1)−X(n)).
We can also use the following approximation:
dX(t)dt≃X(t)−X(t−h)h, | (2.6) |
and by following similar steps to the above, we get:
∇X(n)=F(X(n)),n∈N−{1}, | (2.7) |
where ∇ is the backward difference operator (∇X(n)=X(n)−X(n−1)).
To study the dynamics of the systems 2.5 and 2.7, one must first find the fixed points, and to find the fixed points must be solved the following nonlinear system:
{Ω−r1(I∗+I∗d)S∗−(μ+υ)S∗=0,ρ(I∗+I∗d)−r2(I∗+I∗d)R∗−(υ+μ)R∗=0,υ(S∗+R∗)−r3(I∗+I∗d)V∗−μV∗=0,(λ(r1S∗+r2R∗)+r3V∗)(I∗+I∗d)−(μ+ρ)I∗=0(1−λ)(r1S∗+r2R∗)(I∗+I∗d)−(μ+δ+ρ)I∗d=0. | (3.1) |
The previous equation has the point E0=(Ω(μ+υ),0,υΩμ(μ+υ),0,0) as a solution. It can be seen that the disease at this point is non-exist and therefore it is called the disease-free fixed point which we will focus on studying later.
If we suppose that (I∗+I∗d)≠0, So we will get:
Ωr1(I∗+I∗d)+(μ+υ)=S∗,ρ(I∗+I∗d)r2(I∗+I∗d)+(υ+μ)=R∗,υ(S∗+R∗)r3(I∗+I∗d)+μ=V∗,(λ(r1S∗+r2R∗)+r3V∗)(μ+ρ)=I∗(I∗+I∗d),(1−λ)(r1S∗+r2R∗)(μ+δ+ρ)=I∗d(I∗+I∗d). | (3.2) |
This system is complex and difficult to solve in the abstract case. Even if we can solve it, studying the stability of this fixed point contains many obstacles. In general, this point is called the endemic equilibrium point E∗=(S∗,R∗,V∗,I∗,I∗d) and its existence and stability can be studied numerically.
The basic reproduction number is a very important number for studying the behavior of epidemic systems. According to [21] the basic reproduction number is defined as the spectral radius of the next generation matrix FV−1, where F and V are the Jacobian matrices for F and V respectively at the disease-free fixed point, and where F the rate of appearance of new infections:
F=((λ(r1S(t)+r2R(t))+r3V(t))(I(t)+Id(t))(1−λ)(r1S(t)+r2R(t))(I(t)+Id(t))), |
and V the disappearance rate of infections:
V=((μ+ρ)I(t)(μ+δ+ρ)Id(t)). |
The Jacobite matrices are:
F=(Ω(μ+υ)(λr1+υr3μ)Ω(μ+υ)(λr1+υr3μ)(1−λ)(Ωr1(μ+υ))(1−λ)(Ωr1(μ+υ))), |
and
V=((μ+ρ)00(μ+δ+ρ)). |
So the next generation matrix is:
FV−1=(Ωμυr3+λμr1(μ+υ)(μ+ρ)Ωμυr3+λμr1(μ+υ)(μ+δ+ρ)−Ωr1λ−1(μ+υ)(μ+ρ)−Ωr1λ−1(μ+υ)(μ+δ+ρ)). |
According to it the basic reproductive number is given by:
R0=Ω(μ+υ)((1−λ)r1(μ+δ+ρ)+υr3+λμr1μ(μ+ρ)). | (3.3) |
System 2.5 can be written as:
X(n+1)=X(n)+F(X(n)),n∈N. | (4.1) |
Thus, it is defined by a regression relationship, we can notice that knowing each term allows us to know the term after it, so existence and uniqueness are trivial in this case. It cannot be shown that the solution is positive for system 2.5. In fact, the solution to system 2.5 is not always positive even when the initial conditions are positive.
We are now studying the stability of the disease-free fixed point, we mention the following theorem that we need in the study of stability:
Theorem 1. [22] System 4.1 is asymptotically stable if each eigenvalue λ∗ of the Jacobite matrix J∗ of X(n)+F(X(n)) at the fixed point is satisfied
|λ∗|<1. | (4.2) |
Using the above theorem we result:
Theorem 2. Suppose that R0<1, if
μ+υ<2,A2<4B, | (4.3) |
where
A=(μ+ρ)+(μ+δ+ρ)(1−R0)+Ωδ(υr3+λμr1)μ(μ+ρ)(μ+υ),B=(μ+ρ)(μ+δ+ρ)(1−R0). |
Then the disease-free fixed point E0 of system 2.5 is locallyasymptotically stable.
Proof. System 2.5 is equivalent to system 4.1, and the Jacobian matrix of X(n)+F(X(n)) at E0 is:
J∗=I+J, |
where I is the identity matrix and J is the Jacobian matrix of F(X(n)) at E0:
J=(−(μ+υ)00−Ωr1(μ+υ)−Ωr1(μ+υ)0−(υ+μ)0ρρυυ−μ−Ωυr3μ(μ+υ)−Ωυr3μ(μ+υ)000(λr1Ω(μ+υ)+υr3Ωμ(μ+υ))−(μ+ρ)(λr1Ω(μ+υ)+υr3Ωμ(μ+υ))000(1−λ)r1Ω(μ+υ)(1−λ)r1Ω(μ+υ)−(μ+δ+ρ)). | (4.4) |
The characteristic polynomial of J∗ is:
(X−1+μ)(X−1+μ+υ)2(X2+(A−2)X+(B−A+1)). |
So the matrix J∗ has λ1=1−μ as normal eigenvalue and λ2=1−(μ+υ) as a double eigenvalue, so according to conditions 4.3 and because all parameters are positives (μ+υ<2 and μ,υ>0), we get:
|λ1|<|λ2|<1, |
so λ1 and λ2 satisfy the condition of Theorem 1. Stayed the roots of the polynomial:
X2+(A−2)X+(B−A+1). |
If R0<1 then A,B>0, we have from the conditions 4.3 (A2<4B):
(A−2)2−4(B−A+1)=A2−4B<0. |
So B−A+1>(A−2)24>0, and the roots are complex and conjugated, we not them λ and ˉλ and we have
|λ|=|ˉλ|=√B−A+1=√1−Ωδ(υr3+λμr1)μ(μ+ρ)(μ+υ)<1, |
(because Ωδ(υr3+λμr1)μ(μ+ρ)(μ+υ)>0). So all the eigenvalues of the characteristic equation of J∗ satisfy the condition of Theorem 1. Accordingly the disease-free fixed point E0 of system 2.5 is locally asymptotically stable.
The structure of this system does not allow us to study the global stability, therefore we will move to the backward difference system.
System 2.7 can be written as:
X(n+1)−F(X(n+1))=X(n),n∈N. | (5.1) |
So, to calculate each term, the term before it must belong to the image of the function x→x−F(x), and this makes the existence not trivial like the previous case, for this purpose, we give the following result:
Theorem 3. For any n∗∈N, there exists δ∗>0, so that the solution of system 2.7 (for n≤n∗) is exists when ‖x(0)−E0‖<δ∗. Also, this solution is positive when the initialcondition is positive.
Proof. System 2.7 is equivalent to system 5.1, and from the system 4.5:
X(n)=X(0)+n∑i=1F(X(i)), |
so the solution is the fixed point of T(X(n)), where:
T(X(n))=X(0)+n∑i=1F(X(i)), |
in the space ℓ∞([0,n∗]∩N), with its norm (‖.‖∞), so for x1,x2∈ℓ∞([0,n∗]∩N), we have:
‖T(x1)−T(x2)‖≤n∑i=1‖F(x1(i))−F(x2(i))‖, |
since F continues in the neighborhood of E0, we have ∀ϵ>0,∃δ>0:x1(i),x2(i)∈B(E0,δ) for i≤n⟹‖F(x1(i))−F(x2(i))‖<ϵ‖x1(i)−x2(i)‖. Take ϵ∗>0 check that nϵ∗<1 for n∈[0,n∗]∩N, then ∃δ∗>0, check that:
‖T(x1)−T(x2)‖∞≤nϵ‖x1−x2‖∞. |
So T is contraction map in ℓ∞([0,n∗]∩N), and from it, by Banach fixed point theorem there exists one solution for the system 5.1 for n≤n∗.
To prove the positivity of the solution we assume the opposite. Let S be the first component to become negative at n1∈N, (i.e R(n1),V(n1),I(n1),Id(n1)≥0 and S(n1)≤0), then:
S(n1)−S(n1−1)=Ω−r1(I(n1)+Id(n1))S(n1)−(μ+υ)S(n1)≥0, |
this means
S(n1)≥S(n1−1)≥0, |
a contradiction so S(n)≥0 for n≤n∗. If R the first component to become negative at n2∈N, (i.e S(n2),V(n2),I(n2),Id(n2)≥0 and R(n2)≤0), then:
R(n2)−R(n2−1)=ρ(I(n2)+Id(n2))−r2(I(n2)+Id(n2))R(n2)−(υ+μ)R(n2)≥0, |
this means
R(n2)≥R(n2−1)≥0, |
a contradiction so R(n)≥0 for n≤n∗. If V the first component to become negative at n3∈N, (i.e S(n3),R(n3),I(n3),Id(n3)≥0 and V(n3)≤0) then:
V(n3)−V(n3−1)=υ(S(n3)+R(n3))−r3(I+Id)V(n3)−μV(n3)≥0, |
this means
V(n3)≥V(n3−1)≥0, |
a contradiction so V(n)≥0 for n≤n∗. If I the first component to become negative at n4∈N, (i.e S(n4),R(n4),V(n4),Id(n4)≥0 and I(n4)≤0) then:
I(n4)−I(n4−1)=(λ(r1S(n4)+r2R(n4))+r3V(n4))(I(n4)+Id(n4))−(μ+ρ)I(n4), |
so
I(n4)=I(n4−1)+(λ(r1S(n4)+r2R(n4))+r3V(n4))Id(n4)(1+μ+ρ−(λ(r1S(n4)+r2R(n4))+r3V(n4)))≥0, |
because we know that:
λ(r1S(n4)+r2R(n4))+r3V(n4)<λkp1<1, |
a contradiction, so I(n)≥0 for n≤n∗. If Id the first component to become negative at n5∈N, (i.e S(n5),R(n5),V(n5),I(n5)≥0 and Id(n5)≤0) then:
Id(n5)−Id(n5−1)=(1−λ)(r1S(n5)+r2R(n5))(I(n5)+Id(n5))−(μ+δ+ρ)Id(n5), |
so
Id(n5)=Id(n5−1)+(1−λ)(r1S(n5)+r2R(n5))I(n5)(1+μ+δ+ρ−(1−λ)(r1S(n5)+r2R(n5)))≥0, |
because we know that:
(1−λ)(r1S(n5)+r2R(n5))<(1−λ)kp1<1, |
a contradiction, so I(n)≥0 for n≤n∗.In the end, we conclude that:
R(n)≥0,V(n)≥0,I(n)≥0,Id(n)≥0. |
Theorem 4. The System 2.7 have
Ψ={(S,R,V,I,Id)∈R5+andS+R+V+I+Id≤Ωμ}, |
as invariant region, where R5+={(x1,x2,x3,x4,x5)∈R5andxi≥0fori=1..5}.
Proof. Adding the equations of system 2.7, we get:
∇N(n)=Ω−μN(n)−δId(n). |
Since Id is positive, we get:
∇N(n)≤Ω−μN(n) |
where
N(0)=S(0)+R(0)+V(0)+I(0)+Id(0), |
and from it we find:
N(n)≤Ω+N(n−1)1+μ. |
Let N(0)≤Ωμ, and suppose that N(n)≤Ωμ for a natural number n, we get:
N(n+1)≤Ω+N(n)1+μ≤Ω+Ωμ1+μ=Ωμ, |
then by induction for all n when the solution exists:
0≤N(n)≤Ωμ. |
Therefore, the solution belongs to the invariant region:
Ψ={(S,R,V,I,Id)∈R5+andS+R+V+I+Id≤Ωμ}. |
We are now studying the stability of the disease-free fixed point, so we present the following result:
Theorem 5. Suppose that R0<1. Then the disease-free fixed point E0 of thesystem 2.7 is locally asymptotically stable.
Proof. Locally, system 2.7 behaves the same as that of:
∇X(n)=JX(n),n≥1, | (5.2) |
⇔
X(n+1)−JX(n+1)=X(n), |
since
det(Id−J)=(1+μ)(1+μ+υ)2(A+B+1)>0, |
when R0<1, so
X(n+1)=(Id−J)−1X(n). | (5.3) |
According to Theorem 1, the system 5.3 is stable when each eigenvalue λ∗ of (I−J)−1 is satisfied
|λ∗|<1, | (5.4) |
its meaning that each eigenvalue λ−1∗ of (I−J) is satisfied
|λ−1∗|>1. | (5.5) |
and whereas
|λ−1∗|=|1−λ|, |
where λ an eigenvalue for J, the condition of stability becomes:
|1−λ|>1. | (5.6) |
The characteristic polynomial of J is :
(X+μ)(X+μ+υ)2(X2+AX+B). |
So the matrix J has λ1=−μ, as normal eigenvalue and λ2=−(μ+υ), as a double eigenvalue, and from it:
|1−λ1|=1+μ>1, |
and
|1−λ2|=1+υ+μ>1. |
Stayed the roots of the polynomial:
X2+AX+B, |
if R0<1, then A,B>0, so according to Routh-Hurwitz criterion, the roots of this polynomial called λ3 and λ3 have a negative real part, so:
|1−λ3|>1−Re(λ3)>1, |
and
|1−λ4|>1−Re(λ3)>1, |
where Re(λ) is the real part of λ. Therefore, all eigenvalues of matrix J satisfying the condition 5.6, so the disease-free fixed point is locally asymptotically stable.
The main purpose of studying epidemic models is to find applicable conditions in the real life and contribute to the disappearance of the epidemic, so we will now give a condition related to reducing friction to get rid of the epidemic.
Theorem 6. If
(1−p1k+(μ+ρ))>1, | (5.6) |
then the disease will disappear.
Proof. Adding the last two equations in the system 2.7 together, we find that the infected class is described by the following equation:
∇(I+Id)(n)=(λ(r1S(n)+r2R(n))+r3V(n))(I(n)+Id(n))−(μ+ρ)I(n)+(1−λ)(r1S(n)+r2R(n))(I(n)+Id(n))−(μ+δ+ρ)Id(n),=(r1S(n)+r2R(n)+r3V(n)−(μ+ρ))(I(n)+Id(n))−δId(n). |
Since I is positive and ri=pikN,i=1,2,3,(p3≤p2≤p1):
∇(I+Id)(n)≤(r1S(n)+r2R(n)+r3V(n))(I(n)+Id(n))−(μ+ρ)(I(n)+Id(n)),≤(p1kNS(n)+p2kNR(n)+p3kNV(n))(I(n)+Id(n))−(μ+ρ)(I(n)+Id(n)),≤kmax1≤i≤3{pi}(S(n)+R(n)+V(n)N)(I(n)+Id(n))−(μ+ρ)(I(n)+Id(n)),≤(kp1−(μ+ρ))(I(n)+Id(n)), |
⇔
(I+Id)(n)−(I+Id)(n−1)≤(kp1−(μ+ρ))(I(n)+Id(n)),n=1,2,3,⋯, |
⇔
(I+Id)(n)≤(1−p1k+(μ+ρ))−1(I+Id)(n−1),n=1,2,3,⋯, |
⇔
(I+Id)(n)≤(1−p1k+(μ+ρ))−n(I+Id)(0). |
We note that if (1−p1k+(μ+ρ))−1<1, then (I+Id)(t)→0 when t→∞.
In this section we will compare systems 2.5 and 2.7, note that the solution of the system 2.5 has always existed, but it is not always positive and also it cannot be proven that it is bounded. In system 2.7 the solution does not always exist, but if it is exists by taking a positive initial condition the solution is positive, and it also belongs to an invariant region. As for stability, the system 2.5 and even when R0<1, stability is not guaranteed except by adding other conditions, contrary to system 2.7 the only condition for local stability is R0<1 and therefore it is the one who maintains the characteristics of the continuous system 2.1. In the system 2.5 We couldn't find a condition for global stability, which was so easily formulated in the system 2.7.
In the following, we will do some numerical simulations with the same initial value and the same parameters, and note the essential differences between the two systems 2.5 and 2.7. Therefore, we choose a divided population as follows:
S(0)=500000,R(0)=23000,V(0)=100000,I(0)=3000,Id(0)=170. | (6.1) |
And we take the parameters as:
Ω=38;μ=4.4×10−6;λ=7×10−6;r1=4.4×10−7;r2=3.215×10−7;r3=1.4925×10−7;ρ=0.4;υ=0.08;δ=1.3×10−5. | (6.2) |
We get the simulation as:
We notice that there is no difference in the previous example. In fact, essential differences sometimes do not appear in short periods of time. We now take the same system as before and make
r1=4.4×10−6.3;r2=3.215×10−6.3;r3=1.4925×10−6.3; |
we get
There is no difference at the beginning, but when we take long periods of time, we notice the differences. We have increased the rate of spread of the disease. We note that the disease is spreading quickly. In this case, we notice an increase in I and a decrease in S for the forward difference system, but the opposite happens to the backward difference system.
Referring to the epidemic models that take into account the effect of vaccination on the COVID-19 pandemic, all the models (both continuous-time and discrete-time) published to date consider the vaccination as a control law or as a preventive action. No paper includes the vaccination as an additional compartment, to be added to the remaining compartments when describing the system dynamics. This manuscript has presented a novel discrete-time COVID-19 model, which includes the number of vaccinated individuals as an additional state variable in the system equations. The paper has shown that the proposed compartment model, described by difference equations, has a disease-free fixed point and an epidemic fixed point. By considering both the forward difference system and the backward difference system, some stability analyses of the disease-free fixed point have been carried out. In particular, for the backward difference system a novel theorem has been proved, which has provided a condition for the disappearance of the pandemic when an inequality involving some epidemic parameters is satisfied.This result represents a remarkable finding of the proposed approach, which may help decision-makers to better understand the epidemiological behaviour of the COVID-19 over time.Finally, numerical simulations of the proposed discrete model have been carried out, along with comparisons regarding the performances of both the forward difference system and the backward difference system.
This research has been funded by Scientific Research Deanship at University of Ha'il - Saudi Arabia through project number RG-21067.
The authors declare no conflict of interest.
[1] |
H. W. Hethcote, The mathematics of infectious diseases, SIAM Rev., 42 (2000), 599–653. https://doi.org/10.1137/S0036144500371907 doi: 10.1137/S0036144500371907
![]() |
[2] |
T. T. Marinov, R. S. Marinova, Dynamics of COVID-19 using inverse problem for coefficient identification in SIR epidemic models, Chaos Solit. Fract., 5 (2020). https://doi.org/10.1016/j.csfx.2020.100041 doi: 10.1016/j.csfx.2020.100041
![]() |
[3] |
J. T. Wu, K. Leung, M. Bushman, N. Kishore, R. Niehus, P. M. de Salazar, et al., Estimating clinical severity of COVID-19 from the transmission dynamics in Wuhan, China, Nat. Med., 26 (2020), 506–510. https://doi.org/10.1038/s41591-020-0822-7 doi: 10.1038/s41591-020-0822-7
![]() |
[4] |
S. Mangiarotti, M. Peyre, Y. Zhang, M. Huc, F. Roger, Y. Kerr, Chaos theory applied to the outbreak of COVID-19: An ancillary approach to decision making in pandemic context, Epidem. Infect, 148 (2020), 1–29. https://doi.org/10.1017/S0950268820000990 doi: 10.1017/S0950268820000990
![]() |
[5] |
S. Gounane, Y. Barkouch, A. Atlas, M. Bendahmane, F. Karami, D. Meskine, An adaptive social distancing SIR model for COVID-19 disease spreading and forecasting, Epidem. Methods, 10 (2021), 20200044. https://doi.org/10.1515/em-2020-0044 doi: 10.1515/em-2020-0044
![]() |
[6] |
G. Giordano, F. Blanchini, R. Bruno, P. Colaneri, A. Di Filippo, A. Di Matteo, et al., Modelling the COVID-19 epidemic and implementation of population-wide interventions in Italy, Nat. Med., 26 (2020), 855–860. https://doi.org/10.1038/s41591-020-0883-7 doi: 10.1038/s41591-020-0883-7
![]() |
[7] |
A. Ajbar, R. T. Alqahtani, M. Boumaza1, Dynamics of an SIR-Based COVID-19 Model With Linear Incidence Rate, Nonlinear Removal Rate, and Public Awareness, Front. Phys., (2021). https://doi.org/10.3389/fphy.2021.634251 doi: 10.3389/fphy.2021.634251
![]() |
[8] |
P. Kumara, V. S. Erturk, M. Murillo-Arcila, A new fractional mathematical modelling of COVID-19 with the availability of vaccine, Results Phys., 24 (2021), 2211–3797. https://doi.org/10.1016/j.rinp.2021.104213 doi: 10.1016/j.rinp.2021.104213
![]() |
[9] |
N. Gozalpour, E. Badfar, A. Nikoofard, Transmission dynamics of novel coronavirus SARS-CoV-2 among healthcare workers, a case study in Iran, Nonlinear Dynam., 105 (2021), 3749–-3761. https://doi.org/10.1007/s11071-021-06778-5 doi: 10.1007/s11071-021-06778-5
![]() |
[10] |
E. Badfar, E. J. Zaferani, A. Nikoofard, Design a robust sliding mode controller based on the state and parameter estimation for the nonlinear epidemiological model of Covid-19, Nonlinear Dynam., (2021), 5–-18. https://doi.org/10.1007/s11071-021-07036-4 doi: 10.1007/s11071-021-07036-4
![]() |
[11] |
A. Rajaei, M. Raeiszadeh, V. Azimi, M. Sharifi, State estimation-based control of COVID-19 epidemic before and after vaccine development, J. Pro. Control, 102 (2021), 1–14. https://doi.org/10.1016/j.jprocont.2021.03.008 doi: 10.1016/j.jprocont.2021.03.008
![]() |
[12] |
M. De la Sen, A. Ibeas, R. Nistal, About partial reachability issues in an SEIR epidemic model and related infectious disease tracking in finite time under vaccination and treatment controls, Discrete Dynam. Nat. Soc., (2021). https://doi.org/10.1155/2021/5556897 doi: 10.1155/2021/5556897
![]() |
[13] |
M. De la Sen, A. Ibeas, On an SE(Is)(Ih)AR epidemic model with combined vaccination and antiviral controls for COVID-19 pandemic, Adv. Difference Equ., 92 (2021). https://doi.org/10.1186/s13662-021-03248-5 doi: 10.1186/s13662-021-03248-5
![]() |
[14] |
S. Zhai, G. Luo, T. Huang, X. Wang, J. Tao, P. Zhou, Vaccination control of an epidemic model with time delay and its application to COVID-19, Nonlinear Dynam., 106 (2021), 1279–1292. https://doi.org/10.1007/s11071-021-06533-w doi: 10.1007/s11071-021-06533-w
![]() |
[15] |
E. Hwang, Prediction intervals of the COVID-19 cases by HAR models with growth rates and vaccination rates in top eight affected countries: Bootstrap improvement, Chaos Solit. Fract., 155 (2022). https://doi.org/10.1016/j.chaos.2021.111789 doi: 10.1016/j.chaos.2021.111789
![]() |
[16] |
P. Mahmood, M. Saeed, Stability of the equilibria in a discrete-time sivs epidemic model with standard incidence, Filomat, 33 (2019), 2393–2408. https://doi.org/10.1016/j.chaos.2021.111789 doi: 10.1016/j.chaos.2021.111789
![]() |
[17] |
M. De la Sen, S. Alonso-Quesada, A. Ibeas, On a Discrete SEIR Epidemic Model with Exposed Infectivity, Feedback Vaccination and Partial Delayed Re-Susceptibility, Mathematics, 9 (2021), 5–9. https://doi.org/10.3390/math9050520 doi: 10.3390/math9050520
![]() |
[18] |
M. De la Sen, S. Alonso-Quesada, A. Ibeas, R. Nistal, On a Discrete SEIR Epidemic Model with Two-Doses Delayed Feedback Vaccination Control on the Susceptible, Vaccines, 9 (2021). https://doi.org/10.3390/vaccines9040398 doi: 10.3390/vaccines9040398
![]() |
[19] |
Y. Omae, Y. Kakimoto, M. Sasaki, J. Toyotani, K. Hara, Y. Gon, et al., SIRVVD model-based verification of the effect of first and second doses of COVID-19/SARS-CoV-2 vaccination in Japan, Math. Biosci. Eng., 19 (2021), 1026–1040. https://doi.org/10.3934/mbe.2022047 doi: 10.3934/mbe.2022047
![]() |
[20] | N. Djenina, I. Rezzoug, A. Ouannas, T-E. Oussaeif, Giuseppe Grassi, A new COVID-19 pandemic model including the compartment of vaccinated individuals: Global stability of the disease-free fixed point, Submitted to CMMM, 2022 (2022). |
[21] |
P. van den Driesschea, J. Watmough, Reproduction numbers and sub-threshold endemic equilibria for compartmental models of disease transmission, Math. Biosci., 180 (2002). https://doi.org/10.1016/S0025-5564(02)00108-6 doi: 10.1016/S0025-5564(02)00108-6
![]() |
[22] |
S. Elaydi, An introduction to difference equations, Springer SBM, 3 (2005). https://doi.org/10.1007/0-387-27602-5 doi: 10.1007/0-387-27602-5
![]() |
1. | A Othman Almatroud, Noureddine Djenina, Adel Ouannas, Giuseppe Grassi, M Mossa Al-sawalha, A novel discrete-time COVID-19 epidemic model including the compartment of vaccinated individuals, 2022, 19, 1551-0018, 12387, 10.3934/mbe.2022578 | |
2. | Abdallah Al-Husban, Noureddine Djenina, Rania Saadeh, Adel Ouannas, Giuseppe Grassi, A New Incommensurate Fractional-Order COVID 19: Modelling and Dynamical Analysis, 2023, 11, 2227-7390, 555, 10.3390/math11030555 | |
3. | Noureddine Djenina, Adel Ouannas, 2023, Chapter 9, 978-3-031-37969-7, 147, 10.1007/978-3-031-37970-3_9 | |
4. | Amel Hioual, Adel Ouannas, Taki Eddine Oussaeif, 2023, Chapter 10, 978-3-031-37969-7, 169, 10.1007/978-3-031-37970-3_10 | |
5. | Amer Dababneh, Noureddine Djenina, Adel Ouannas, Giuseppe Grassi, Iqbal M. Batiha, Iqbal H. Jebril, A New Incommensurate Fractional-Order Discrete COVID-19 Model with Vaccinated Individuals Compartment, 2022, 6, 2504-3110, 456, 10.3390/fractalfract6080456 | |
6. | Limin Zhang, Jiaxin Gu, Guangyuan Liao, Bifurcations and model fitting of a discrete epidemic system with incubation period and saturated contact rate, 2025, 1023-6198, 1, 10.1080/10236198.2025.2457987 | |
7. | Shaher Momani, Iqbal M. Batiha, Issam Bendib, Abeer Al-Nana, Adel Ouannas, Mohamed Dalah, On finite-time stability of some COVID-19 models using fractional discrete calculus, 2025, 26669900, 100188, 10.1016/j.cmpbup.2025.100188 |