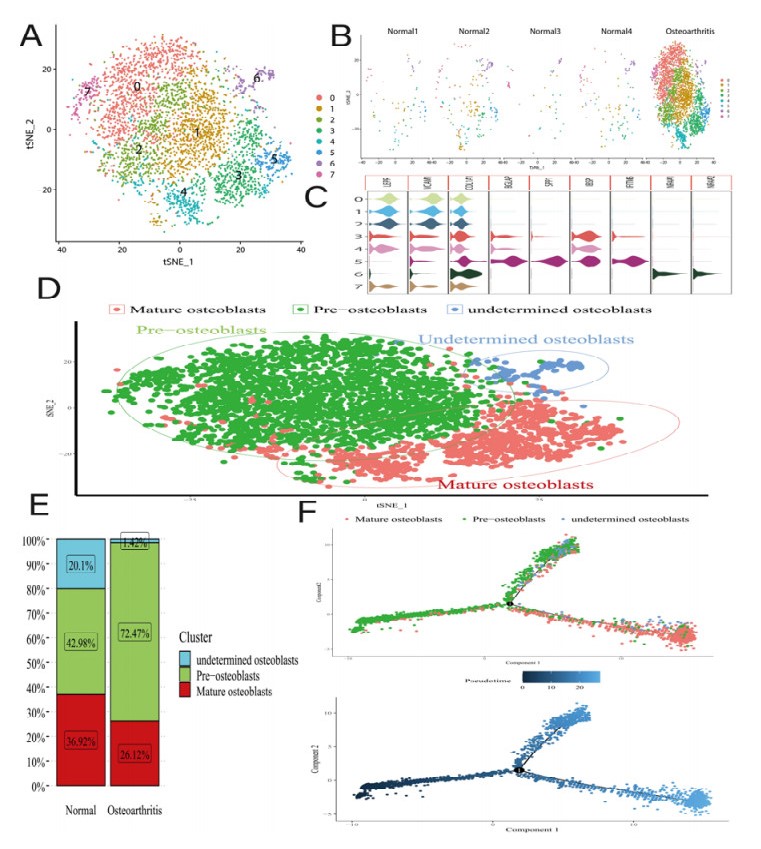
Osteoarthritis (OA) is the most common degenerative joint disease caused by osteoblastic lineage cells. However, a comprehensive molecular program for osteoblasts in human OA remains underdeveloped. The single-cell gene expression of osteoblasts and microRNA array data were from human. After processing the single-cell RNA sequencing (scRNA-seq) data, it was subjected to principal component analysis (PCA) and T-Stochastic neighbor embedding analysis (TSNE). Differential expression analysis was aimed to find marker genes. Gene-ontology (GO) enrichment, Kyoto encyclopedia of genes and genomes (KEGG) pathway enrichment analysis and Gene set enrichment analysis (GSEA) were applied to characterize the molecular function of osteoblasts with marker genes. Protein–protein interaction (PPI) networks and core module were established for marker genes by using the STRING database and Cytoscape software. All nodes in the core module were considered to be hub genes. Subsequently, we predicted the potential miRNA of hub genes through the miRWalk, miRDB and TargetScan database and experimentally verified the miRNA by GSE105027. Finally, miRNA-mRNA regulatory network was constructed using the Cytoscape software. We characterized the single-cell expression profiling of 4387 osteoblasts from normal and OA sample. The proportion of osteoblasts subpopulations changed dramatically in the OA, with 70.42% of the pre-osteoblasts. 117 marker genes were included and the results of GO analysis show that up-regulated marker genes enriched in collagen-containing extracellular matrix were highly expressed in the pre-osteoblasts cluster. Both KEGG and GSEA analyses results indicated that IL-17 and NOD-like receptor signaling pathways were enriched in down-regulated marker genes. We visualize the weight of marker genes and constructed the core module in PPI network. In potential mRNA-miRNA regulatory network, hsa-miR-449a and hsa-miR-218-5p may be involved in the development of OA. Our study found that alterations in osteoblasts state and cellular molecular function in the subchondral bone region may be involved in the pathogenesis of osteoarthritis.
Citation: Changxiang Huan, Jiaxin Gao. Insight into the potential pathogenesis of human osteoarthritis via single-cell RNA sequencing data on osteoblasts[J]. Mathematical Biosciences and Engineering, 2022, 19(6): 6344-6361. doi: 10.3934/mbe.2022297
[1] | Heyrim Cho, Ya-Huei Kuo, Russell C. Rockne . Comparison of cell state models derived from single-cell RNA sequencing data: graph versus multi-dimensional space. Mathematical Biosciences and Engineering, 2022, 19(8): 8505-8536. doi: 10.3934/mbe.2022395 |
[2] | Shuai Miao, Lijun Wang, Siyu Guan, Tianshu Gu, Hualing Wang, Wenfeng Shangguan, Weiding Wang, Yu Liu, Xue Liang . Integrated whole transcriptome analysis for the crucial regulators and functional pathways related to cardiac fibrosis in rats. Mathematical Biosciences and Engineering, 2023, 20(3): 5413-5429. doi: 10.3934/mbe.2023250 |
[3] | Rui-zhe Zheng, Jin Xing, Qiong Huang, Xi-tao Yang, Chang-yi Zhao, Xin-yuan Li . Integration of single-cell and bulk RNA sequencing data reveals key cell types and regulators in traumatic brain injury. Mathematical Biosciences and Engineering, 2021, 18(2): 1201-1214. doi: 10.3934/mbe.2021065 |
[4] | Jie Chen, Jinggui Chen, Bo Sun, Jianghong Wu, Chunyan Du . Integrative analysis of immune microenvironment-related CeRNA regulatory axis in gastric cancer. Mathematical Biosciences and Engineering, 2020, 17(4): 3953-3971. doi: 10.3934/mbe.2020219 |
[5] | Jinqi He, Wenjing Zhang, Faxiang Li, Yan Yu . Development of metastasis-associated seven gene signature for predicting lung adenocarcinoma prognosis using single-cell RNA sequencing data. Mathematical Biosciences and Engineering, 2021, 18(5): 5959-5977. doi: 10.3934/mbe.2021298 |
[6] | Linqian Guo, Qingrong Meng, Wenqi Lin, Kaiyuan Weng . Identification of immune subtypes of melanoma based on single-cell and bulk RNA sequencing data. Mathematical Biosciences and Engineering, 2023, 20(2): 2920-2936. doi: 10.3934/mbe.2023138 |
[7] | Xiaodong Xia, Manman Wang, Jiao Li, Qiang Chen, Heng Jin, Xue Liang, Lijun Wang . Identification of potential genes associated with immune cell infiltration in atherosclerosis. Mathematical Biosciences and Engineering, 2021, 18(3): 2230-2242. doi: 10.3934/mbe.2021112 |
[8] | Ana Isabel Muñoz, J. Ignacio Tello . On a mathematical model of bone marrow metastatic niche. Mathematical Biosciences and Engineering, 2017, 14(1): 289-304. doi: 10.3934/mbe.2017019 |
[9] | Xudan Ma, Qijun Zhang, Haihong Zhu, Kefeng Huang, Weina Pang, Qin Zhang . Establishment and analysis of the lncRNA-miRNA-mRNA network based on competitive endogenous RNA identifies functional genes in heart failure. Mathematical Biosciences and Engineering, 2021, 18(4): 4011-4026. doi: 10.3934/mbe.2021201 |
[10] | Yonghua Xue, Yiqin Ge . Construction of lncRNA regulatory networks reveal the key lncRNAs associated with Pituitary adenomas progression. Mathematical Biosciences and Engineering, 2020, 17(3): 2138-2149. doi: 10.3934/mbe.2020113 |
Osteoarthritis (OA) is the most common degenerative joint disease caused by osteoblastic lineage cells. However, a comprehensive molecular program for osteoblasts in human OA remains underdeveloped. The single-cell gene expression of osteoblasts and microRNA array data were from human. After processing the single-cell RNA sequencing (scRNA-seq) data, it was subjected to principal component analysis (PCA) and T-Stochastic neighbor embedding analysis (TSNE). Differential expression analysis was aimed to find marker genes. Gene-ontology (GO) enrichment, Kyoto encyclopedia of genes and genomes (KEGG) pathway enrichment analysis and Gene set enrichment analysis (GSEA) were applied to characterize the molecular function of osteoblasts with marker genes. Protein–protein interaction (PPI) networks and core module were established for marker genes by using the STRING database and Cytoscape software. All nodes in the core module were considered to be hub genes. Subsequently, we predicted the potential miRNA of hub genes through the miRWalk, miRDB and TargetScan database and experimentally verified the miRNA by GSE105027. Finally, miRNA-mRNA regulatory network was constructed using the Cytoscape software. We characterized the single-cell expression profiling of 4387 osteoblasts from normal and OA sample. The proportion of osteoblasts subpopulations changed dramatically in the OA, with 70.42% of the pre-osteoblasts. 117 marker genes were included and the results of GO analysis show that up-regulated marker genes enriched in collagen-containing extracellular matrix were highly expressed in the pre-osteoblasts cluster. Both KEGG and GSEA analyses results indicated that IL-17 and NOD-like receptor signaling pathways were enriched in down-regulated marker genes. We visualize the weight of marker genes and constructed the core module in PPI network. In potential mRNA-miRNA regulatory network, hsa-miR-449a and hsa-miR-218-5p may be involved in the development of OA. Our study found that alterations in osteoblasts state and cellular molecular function in the subchondral bone region may be involved in the pathogenesis of osteoarthritis.
Osteoarthritis is chronic degenerative joint disease, which may affect the cartilage, synovium, joint ligaments and subchondral bone [1]. In 2005, approximately 27 million people in the United States had OA [2]. The traits of joint lesions are joint space narrowing, osteophytosis, subchondral sclerosis and osteophyte formation. The traditional treatment in the later stages of OA is joint replacement surgery, obviously, its drawbacks include the inability to effectively treat and prevent early-stage OA and the need for invasive the surgery. The exploration of the pathogenesis of early OA, and timely prevention and treatment of OA will be beneficial to improve patient survival condition. Various biochemical compounds involved in OA development have been regarded as disease pathogenesis consisting of several inflammatory response proteins (e.g., interleukin 1β, interleukin 6 and tumor necrosis factor), matrix-degrading enzymes and toll-like receptors secreted by chondrocytes in cartilage [3]. However, some researchers used isotopes and radiography of OA joints to show that osteophyte and sclerosis formation in subchondral bone precede cartilage change [4]. These changes may be mediated by osteoblasts, osteocytes and osteoclast in subchondral bone. Abnormal bone remodeling, bone matrix mineralization and skeletal architecture construction are dysfunctions in osteoarthritic subchondral bone and directly effect on cartilage [5,6,7]. The irregular expression of RANKL had been found in OA osteoblasts [8,9]. osteoblasts may be acted in OA pathogenesis, by producing abnormal levels of A disintegrin and metalloproteinase with thrombospondin motifs (ADAMTS) and MMPs [10]. Therefore, the molecular mechanisms underlying osteoblasts in OA bone should be investigated.
After extensive research, various genes associated with disease treatment were established, including non-coding RNA, DNA methylation, histone modifications and regulatory RNAs [11,12]. Earlier studies have searched for potential target genes involved in OA in bulk transcriptome profiles [13,14,15]. The identification and exploration of differential expression genes in osteoblasts between normal and OA could expand our overall understanding of the role of osteoblasts in OA development. For samples with high heterogeneity such as the joint, traditional high-throughput sequencing technologies can only provide the average of transcript levels of all cells in the sample, but single-cell transcriptome sequencing can accurately characterize the transcriptome of each cell in the sample. Chenlu Li [16] successfully utilized single-cell RNA sequencing and found that COL6A3 and ACTG were validated as key marker genes of fibroblastic-like chondrocytes and fibroblasts in OA. Zhen Wu [17] found that fibronectin1 as key gene of synovial fibroblasts and the pathway of marker genes activated in differential state cells through single-cell expression profiling. Subsequently, we explored significant hub genes between subpopulations osteoblasts of normal and OA sample from single-cell expression profiling.
miRNA consists of 20–24 nucleotides, which binds to the target messenger RNA binding to the 3' untranslated region of the target RNA, and it can inhibit the translation of the target messenger RNA and affect protein expression [18]. miRNAs are recommended as circulating markers for osteoarthritis and are considered as novel therapeutic materials for in clinical treatment [19]. However, limited studies have reported the use of miRNA in OA. Coutinho de Almeida R found that 238 target mRNAs of 62miRNAs were mainly enriched in the 'nervous system development' mediated by miRNA regulatory mechanisms. TAO [20] found that miR34a is overexpressed in cartilage of OA patients and regulates the PI3K/AKT pathway. Therefore, an in-depth study of OA mRNA-miRNA regulatory mechanisms can provide a comprehensive understanding of the pathogenesis of OA and identify potential therapeutic loci.
In our research, we explored the genomic signatures and marker genes in osteoblasts between normal and OA osteoblasts by using scRNA-seq data from osteoblasts cluster derived from OA patient and healthy person. After differential expression analysis, we explored the biological functions of the differential expression genes. We constructed the PPI network and core module to accurately investigate differential gene interactions and screen for hub genes. Then, based on several credible databases, we rigorously screened several potential miRNAs and constructed mRNA-miRNA regulatory networks, in which partial miRNAs were experimentally validated by GSE175961.
The scRNA-seq data of human hip joint tissue of OA and normal were accessed from GSE169396 and GSE147390 with a reading depth of 10× genomics based on Illumina NextSeq 500 via the Gene Expression Omnibus (GEO) database (https://www.ncbi.nlm.nih.gov/geo/) [21,22]. Four normal samples and one OA sample were screened using Seurat package (version 4.0.2) [23]. The cells were subjected to 1000 highly variable genes by using the Normalization function and FindVariableFeatures function in the Seurat package. After using the FindNeighbors function, FindClusters function and RunTSNE function, cells were divided into different clusters according to highly variable genes. Finally, normal and osteoarthritic osteoblasts which remarked by marker genes (ALPL and RUNX2) were merged into one matrix by using the cbind function of R.
We utilized the Seurat package in R 4.1.1 to process osteoblasts matrix with subsequent analysis [23]. First, the osteoblasts matrix created the object and excluded low-quality cells by using the CreateSeuratObject and subset function. Low-quality osteoblasts were removed according to the following criteria: 1) genes identified < 3 cells; 2) cells of total cell expression genes < 200; 3) volume of total detected RNA < 500 or > 2500; and 4) The proportion of mitochondria and ribosomal expressed genes > 25%. The osteoblasts object was normalized using the NormalizeData function, and 1000 highly variable genes were screened out using the FindVariableFeatures function. To analyze multidmensinoal and complexity scRNA-seq data, we selected the ScaleData function and RunPCA function to linearly scale the data and reduction analysis [24].
To visualize and statistically analyze scRNA-seq data, we tested 20 principal component and were chosen for t-SNE. The function of FindNeighbors and FindClusters with the 0.5 clustering resolution were used for classification analysis of cell clusters. Then, we performed cluster identification and visualization in osteoblasts through RunTSNE function [25]. The osteoblast subpopulations, including pre-osteoblasts, mature osteoblasts and undetermined osteoblasts, were remarked through experimentally validated marker genes by manual annotation [22,26,27].
The differentially expressed genes (DEGS) between normal and OA osteoblasts were evaluated using the FindMarkers function as implemented to identify the DEGS of osteoblasts samples. We filtered the marker genes in accordance with cutoff of p_value_adj < 0.05 and |log2[fold change (FC)]| > 1. To further demonstrate the differences among different cell clusters, we used the Monocle package in R 4.1.1 to demonstrate the pseudotime trajectories analysis [28]. Using the differentialGeneTest function to selected test genes. Then, the prerequisite object was constructed as Monocle package, and the multi-dimensional expression profile of cell clusters was reduced to a low-dimensional data and presented in the form of different branches by using the method of DDRTree. By using the orderCells function, each cell was projected onto a dimension and tracked with branching points according to test genes.
ClusterProfiler package (version 4.0.5) in R 4.1.1 was utilized to explore the comprehensive molecular biology mechanisms and pathway of osteoblasts [29]. GO and KEGG analysis of marker genes were identified by enrichGO and enrichKEGG function in ClusterProfiler based on the cutoffvalue < 0.01 and cutoffvalue < 0.05. However, GSEA used for the analysis of the enrichment of total DEGS arranged by logFC from largest to smallest in ClusterProfiler package with the P-value < 0.05.
The Search Tool for the Retrieval of Interacting Genes (STRING V11.5, https://string-db.org/) database was used to identify the hub gene and visualize the PPI among the marker genes. The confidence PPI network was set minimum required interaction score > 0.4 and exported the result as a tsv.file. The original result was imported to the Cytoscape software (version 3.8.2), and a PPI network was constructed according to the co-expression active interaction source [30]. The core module was selected by Molecular Complex Detection (MCODE) plugin of Cytoscape with the degree Cutoff = 2, node score Cutoff = 0.2, K-core = 7 and max depth = 100. All nodes in the core module were selected as hub genes. The KEGG enrichment analysis of hub genes from top 1 module were applied using CluePedia plugin of Cytoscape. The KEGG enrichment analysis terms, it should have contained at least three genes from top 1 module and at least 3% of all gene characteristic to each term. Regarding the statistical method of the enrichment analyses, a cutoff of P value < 0.05 and kappa score of 0.4 were identified as screening criteria.
Hub genes were respectively predicted their potential miRNA depending on the three online miRNA databases including miRWalk (http://mirwalk.umm.uni-heidelberg.de/), TargetScan (http://www.targetscan.org/vert_80/) and miRDB (http://mirdb.org/). Not all miRNAs were selected, and the miRNA was found in at three databases to be considered as potential miRNA. The network was established according to bindingp score in Cytoscape software [30].
GSE175961 was downloaded from GEO database and used to analysis the miRNA expression levels of miRNA in human knee based on the platform of GPL20712 (Agilent-070156 Human miRNA). Significant differential expression levels of miRNAs between healthy and OA knee were analyzed by R language limma package [31]. The valid miRNA needs to meet two requirements simultaneously: 1) P value < 0.05; 2) The mRNA and miRNA have the opposite expression levels between healthy and OA knee.
After overlap the osteoblasts marker genes (ALPL, RUNX2) and each cluster marker genes with normal and osteoarthritis samples, we acquired 885 normal osteoblasts and 4221 osteoarthritic osteoblasts derived from four normal individuals and one patient (Figure S1, Table S1). After excluding low-quality cells with percentages of both ribosomal and mitochondrial sequencing count > 25%, we retained 577 normal osteoblasts and 3810 osteoarthritic osteoblasts for subsequent analysis. We combined the sequencing data of 5106 files into one matrix. The results of the quality control were presented in the form of violin diagrams, showing the detected gene numbers, the sequencing count and proportion of ribosomal and mitochondrial genes in each cell (Figure S2A). A sum of 19,535 corresponding genes were involved, from which 1000 highly variable genes were selected for cell subpopulation analysis, and the top 10 genes were displayed in Figure S2B. Then, we used principal component analysis (PCA) to selected optimal principal component and screened remarkably correlated genes in each component. According to the distribution of cell clusters in PC_1 and PC_2, scRNA-seq data from different sample have no significant batch effects and presented good cell clustering effects shown in Figure S2C. Furthermore, we selected 20 PCs with the P value < 0.05 for cell subpopulation visualization (Figure S2D). To directly visualize the multidimensional scRNA-seq data, we used TSNE projection to precisely classify osteoblasts into seven clusters (Figure 1A). And We used the split TSNE projection to show osteoblasts cells from different samples (Figure 1B). The manually annotated marker genes of three osteoblasts subpopulations are listed in Table S2. The marker genes of three osteoblasts subpopulations expression were shown in Figure 1C. We calculated the marker genes of seven clusters with criteria of p_val_adj < 0.05, in which LEPR enriched in clusters 0, 1, 2, VCAM1 enriched in clusters 0, 1; clusters; COL1A1, SPP1 and IFITM5 enriched in cluster 5; BGLAP and IBSP enriched in clusters 3, 4, 5 and NR4A1 and NR4A2 enriched in cluster 6 (Table S3). Taking into account the subpopulations marker genes at the cell cluster marker genes and cell expression levels, we annotated cell clusters 0, 1, 2 as pre-osteoblasts clusters 3, 4, 5 as mature osteoblast clusters, and 6 as undetermined osteoblast clusters. When the 7-cluster was analyzed for differences with pre-osteoblasts cells, most of the differential genes were ribosomal genes and therefore the 7-cluster was annotated as pre-osteoblasts clusters (Table S4). Pre-osteoblasts included 3009 cells; mature osteoblasts included 1208 cells and undetermined Osteoblasts included 170 cells (Table S5). Then, we exhibited the trajectory analysis to explore the osteoblasts subpopulations differentiation trends. A relatively high proportion of OA osteoblasts consisted of pro-osteoblasts compared with normal osteoblasts, but a relatively low proportion consisted of mature osteoblasts and undetermined osteoblasts (Figure 1E). Exactly as described by the cell annotation results, osteoblasts were correspondingly divided into three directions in the trajectory analysis of scRNA-seq results. From the left to the right of the diagram shows the differentiation of pre-osteoblasts to mature osteoblasts, with undetermined osteoblasts in the middle (Figure 1F).
A total of 117 marker genes were included through differential expression analysis between normal and osteoarthritic osteoblasts with |avg_log2FC| > 1 and p_value_adj < 0.05 (Table S6).
GO enrichment analysis was explored the molecular biology functions of marker genes in biological processes, cellular component and molecular function category (Figure 2A, B). In the biological processes category, up-regulated marker genes were remarkably related with post-translational protein modification, regulation of leukocyte migration and positive regulation of leukocytes migration, while the enrichment items of down-regulated marker genes included humoral immune response, defense response to bacterium and neutrophil activation. In the cellular component category, most up-regulated marker genes were associated with collagen-containing extracellular matrix and down-regulated marker genes were associated with secretory granule lumen, cytoplasmic vesicle lumen and vesicle lumen. In the molecular function category, significant enrichment items of up-regulated marker genes included enzyme inhibitor activity, receptor ligand activity and signaling receptor activator activity, while the enrichment items of down-regulated marker genes included glycosaminoglycan binding. The distribution of top-one GO analysis results was shown in Figure 3A, B.
KEGG enrichment analysis was explored the potential pathways of marker genes. The significantly enrichment item of up-regulated marker genes was cholesterol metabolism (P < 0.05, Figure 3C). Down-regulated marker genes were significantly activated in the IL-17 signaling pathway, malaria, NOD-like receptor signaling pathway, AGE-RAGE signaling pathway, TNF signaling pathway, transcriptional mis-regulation in cancer and osteoclast differentiation (P < 0.05, Figure 3D).
GSEA is a genetic statistical model that calculates significant biological pathways after ranking complete DEGS ordered avg_log2FC. The DEGS significantly enriched in IL-17 signaling pathway, NOD-like receptor signaling pathway and ribosome (P < 0.05, Figure 4). As shown in the figure, ribosome mostly enriched in down-regulated marker genes in DEGS. IL-17 and NOD-like receptor signaling pathway share the same linear trend and peak and they are mostly enriched in down-regulated marker genes in DEGS.
For a good understanding of the interaction correlation of marker genes, we performed STRING online database to construct PPI network. Based on the minimum required interaction score = 0.04, the network consists of 756 edges and 95 nodes. All the genes were imported into degree algorithm method on CytoHubba plugin, and the weight of marker genes with more interactions with other maker genes was visualized (Figure 5A). The most significant cluster with highly interconnected regions in the PPI network was obtained from MCODE plugin. The module involved 18 nodes and 134 edges (Figure 5B). All nodes in the module such as CXCL12, CXCL8, NR4A1, LCN2, JUN, S100A8, IER2, ZFP36, HP, SOCS3, CAMP, JUNB, BTG2, FOSB, RETN, IRF1, EGR1, and ATF3 were regarded as hub genes for the next functional enrichment analysis by using the ClueGO plugin in Cytoscape. Hub genes correspondingly involved in the IL-17 signaling pathway, osteoclast differentiation, TNF signaling pathway, AGE-RAGE signaling pathway in diabetic complications and Pertussis and rheumatoid arthritis in KEGG enrichment analysis (Figure 5C, Table S7). The connection genes between the different KEGG terms include CXCL8, JUN, JUNB, SOCS3, and FOSB.
To explore the potential regulatory pathways of miRNA, we searched three online databases, namely, TargetScan, miRWalk and miRDB, to predict the corresponding miRNA of hub genes. A miRNA can only be a potential miRNA if it is predicted by all three databases at the same time. Furthermore, we identified 189 potential miRNAs including SOCS3 intersects 2 potential miRNAs, BTG2 intersects 30 potential miRNAs, CXCL12 intersects 64 potential miRNAs, EGR1 intersects 4 potential miRNAs, FOSB intersects 10 potential miRNAs, IER2 intersects 4 potential miRNAs and JUN intersects 1 potential miRNA. To accurately visualize the regulatory network, we imported the intersections between mRNA and miRNA into Cytoscape software (Figure 6A). has-miR-449a and has-miR-218-5p were not only predicted by the database, but were also experimentally validated to be potentially regulated in relation to FOSB and SOCS3 based on the principle that miRNA inhibited the translation of the target messenger RNA. FOSB expressed high levels in normal sample than in OA sample (P < 0.01), but hsa-miR-449a has the opposite profile (P < 0.01, Figures 6B, C). SOCS3 expressed high levels in normal samples (P < 0.01), but hsa-miR-218-5p is highly expressed in OA samples (P < 0.05, Figures 6D, E).
Osteoarthritis is a chronic disease in which lesions in the subchondral bone area are characterized by osteophytes formation and sclerosis. OA lesions in the subchondral bone region are mediated by osteocytes and bone matrix. The osteoblasts act in bone production and remodeling, regulate skeletal architecture and bone matrix mineralization by producing extracellular matrix proteins and induce osteoclastogenesis by producing cytokines or direct cell contact [32]. However, with the onset of OA, their function changes dramatically in the subchondral bone region. Traditional RNA bulk-sequencing mainly obtains the average expression of gene transcripts of all cells in a tissue, but not the expression profile of individual cells or a certain type or state of cells, thus obscuring the role of key cell subpopulations or intermediate states. scRNA-seq allows us to obtain the gene expression profiles of individual cells and analyze them to identify the transcriptional profiles of cell types and subtypes, and thus understand the occurrence of OA and explore novel therapeutic loci. In our research, we combined osteoblasts clusters from single-cell datasets GSE169396 and GSE147390 into the same object to explore gene expression differences, molecular mechanisms and miRNA regulatory networks in OA.
In the present study, we identified seven clusters and manually annotated them as pro-osteoblasts cluster, mature osteoblasts cluster and undetermined osteoblasts cluster according to marker gene. After the proposed time series analysis, the osteoblasts subpopulations were divided into three directions, in which the pro-osteoblasts cluster was distributed in the lower left, the mature osteoblasts cluster was distributed in the lower right and the undetermined osteoblasts cluster was distributed in the upper part, which further validated the manual annotation results of the three osteoblasts subpopulations. Undetermined osteoblasts may be in an intermediate stage between pre-osteoblasts and mature osteoblasts. In the present study, osteoblasts in normal and OA samples shared the same subpopulation typology and no subpopulation appeared in separate samples. However, the proportion of subpopulations in the samples changed remarkably, and a higher proportion of mature osteoblasts and undetermined osteoblasts in normal samples and a high proportion of pre-osteoblasts in osteoarthritic samples. Upon the activation of IGF1 and PGE2 hormones, normal pre-osteoblasts further differentiates into mature osteoblasts, while the mineralization of the extracellular matrix occurred [33]. Indira Prasadam et al. [7] found that OA osteoblasts were unable to produce normal minerals and extracellular mesenchyme resulting in the inability to express SOST and DMP late markers, demonstrating that osteoblasts were in an immature state. As an essential survival environment, the extracellular mesenchyme regulates cell proliferation, differentiation and migration through multiple signaling pathways. Significant abnormalities in the extracellular mesenchyme have been observed in OA [34,35,36]. In the present study, the result of GO analysis show that marker genes were remarkably enriched in collagen-containing extracellular matrix. Subsequently, we found that collagen-containing extracellular matrix was highly expressed in the pre-osteoblasts and undetermined osteoblasts 2 clusters, which further validated the above results. Therefore, the undetermined osteoblasts 2 clusters may be a state between pre-osteoblasts and mature osteoblasts. Furthermore, alterations in osteoblasts status and function triggered by the abnormal mass of the extracellular mesenchyme may be involved in the occurrence of OA.
In the bone matrix, overloading of the joint causes microdamage and osteoblasts detecting microdamage can initiate repair by osteoblasts and osteoclasts [6]. IL-17 and TNF-a can mediate the RNAKL-RANK-OPG system to control the communication between osteoblasts and osteoclasts to achieve bone homeostasis [37,38,39,40]. Osteoblasts are important cells in the repair of inflammatory bone tissue damage. Michiel Croes [41] found that IL-17 greatly promotes osteogenic differentiation of human bone marrow mesenchymal stem cells. Zhongxiu Wang [38] treated primary osteoblasts with IL-17 with high expression of mRNA levels of ALP and RUNX2 (markers of early osteoblasts differentiation) and osteocalcin (marker of late differentiation) in a mouse model. Both GSEA and KEGG analyses results, IL-17 signaling pathway was highly activated in down-regulated marker genes. Obtained KEGG analysis of the core module and marker genes, IL-17 and TNF signaling pathway were are jointly regulated by JUN. Osteoclast differentiation was jointly regulated by IL-17 and TNF signaling pathway by JUN. Therefore, we speculate that the abnormal synthesis of IL-17A receptor and TNF-receptor on the surface of osteoblasts results in the inability of IL-17 and TNF to affect osteoblasts effectively, resulting in impaired bone remodeling and hypofractionation of primary osteoblasts, which in turn causes the clinical features of osteoarthritis (e.g., bone redundancy and subchondral osteosclerosis). We hypothesize that JUN may be potential therapeutic loci.
The expression of mRNAs was negatively regulated and repressed by miRNAs. Hence, mRNAs and miRNAs have opposite expression trends. miRNA expression can influence the development of OA [42,43,44]. In recent years, Numerous miRNAs have been identified in osteoarthritic cartilage cells [45]. Soyocak [46] found that miR-146a and miR-155 expressed higher levels in the osteoarthritis human than that of normal persons subjects and increases with the disease duration. Wang [47] found that miR-411 and matrix metalloproteinase 13 were negatively correlated in cartilage tissue in osteoarthritis, and that matrix metalloproteinase 13 was the target gene of miR-411 which overexpression inhibited the expression of matrix metalloproteinase 13, thereby delaying the development of OA. In our research, we searched for 130 potential miRNAs, among which hsa-miR-449a and hsa-miR-218-5p were obtained from the GSE175961 dataset for experimental validation. We found that hsa-miR-449a and FOSB, hsa-miR-218-5p with SOCS3 all exhibited opposite expression trends in OA samples. Thus, hsa-miR-449a and hsa-miR-218-5p may accompany the development and progression of OA. More experiments are still needed to validate and define it.
In addition, cementoblasts, a manner comparable to osteoblasts, expressed various functional TLRs and nucleotide-binding oligomerization domain (NOD) proteins [48]. The expressions of TLRs and NODs were upregulated upon differentiation in both cementoblasts and osteoblasts in a similar manner. Both GSEA and KEGG analysis, showed the NOD-like receptor signaling pathway located in down-regulated area. Therefore, low NOD-receptor expression may delay osteoblasts differentiation, which still needs further study.
Our current research still has limitations. Patient details are incomplete, and some clinical parameters such as laboratory tests, medical and surgical records cannot be added to the discussion, because they cannot be downloaded. Although the genetic profile of osteoblasts and miRNAs are well validated, complementary basic experiments are still necessary to reveal the specific mechanisms underlying the development of OA. We have shared the code on the GitHub website and expect more researchers to communicate with us via email (GitHub: https://github.com/huanchangxiang/Code-of-scRNA-in-osteoarthritis.git).
In conclusion, our study is the first to screen marker gene and potential miRNA for OA osteoblasts based on single-cell transcriptomics, and in addition to this, we have identified OA osteoblasts subpopulations and genetic signatures to explore the role of osteoblasts in the pathogenesis of OA at the genetic level.
All data in this study are already available from the Gene Expression Omnibus (https://www.ncbi.nlm.nih.gov/geo/).
The authors declare that there is no conflict of interest in this work.
[1] |
B. Abramoff, F. E. Caldera, Osteoarthritis: pathology, diagnosis, and treatment options, Med. Clin. North Am., 104 (2020), 293-311. https://doi.org/10.1016/j.mcna.2019.10.007 doi: 10.1016/j.mcna.2019.10.007
![]() |
[2] |
R. C. Lawrence, D. T. Felson, C. G. Helmick, L. M. Arnold, H. Choi, R. A. Deyo, et al., Estimates of the prevalence of arthritis and other rheumatic conditions in the United States: Part Ⅱ, Arthritis Rheum., 58 (2008), 26-35. https://doi.org/10.1002/art.23176 doi: 10.1002/art.23176
![]() |
[3] |
S. Glyn-Jones, A. J. Palmer, R. Agricola, A. J. Price, T. L. Vincent, H. Weinans, et al., Osteoarthritis, Lancet, 386 (2015), 376-387. https://doi.org/10.1016/s0140-6736(14)60802-3 doi: 10.1016/s0140-6736(14)60802-3
![]() |
[4] |
C. Buckland-Wright, Subchondral bone changes in hand and knee osteoarthritis detected by radiography, Osteoarthritis Cartilage, 12 (2004), S10-19. https://doi.org/10.1016/j.joca.2003.09.007 doi: 10.1016/j.joca.2003.09.007
![]() |
[5] |
E. Dall'Ara, C. Ohman, M. Baleani, M. Viceconti, Reduced tissue hardness of trabecular bone is associated with severe osteoarthritis, J. Biomech., 44 (2011), 1593-1598. https://doi.org/10.1016/j.jbiomech.2010.12.022 doi: 10.1016/j.jbiomech.2010.12.022
![]() |
[6] |
D. M. Findlay, G. J. Atkins, Osteoblast-chondrocyte interactions in osteoarthritis, Curr. Osteoporosis Rep., 12 (2014), 127-134. https://doi.org/10.1007/s11914-014-0192-5 doi: 10.1007/s11914-014-0192-5
![]() |
[7] |
I. Prasadam, S. Farnaghi, J. Q. Feng, W. Gu, S. Perry, R. Crawford, et al., Impact of extracellular matrix derived from osteoarthritis subchondral bone osteoblasts on osteocytes: role of integrinβ1 and focal adhesion kinase signaling cues, Arthritis Res. Ther., 15 (2013), R150. https://doi.org/10.1186/ar4333 doi: 10.1186/ar4333
![]() |
[8] |
S. K. Tat, J. P. Pelletier, N. Amiable, C. Boileau, D. Lajeunesse, N. Duval, et al., Activation of the receptor EphB4 by its specific ligand ephrin B2 in human osteoarthritic subchondral bone osteoblasts, Arthritis Rheum., 58 (2008), 3820-3830. https://doi.org/10.1002/art.24029 doi: 10.1002/art.24029
![]() |
[9] | S. K. Tat, J. P. Pelletier, D. Lajeunesse, H. Fahmi, M. Lavigne, J. Martel-Pelletier, The differential expression of osteoprotegerin (OPG) and receptor activator of nuclear factor kappaB ligand (RANKL) in human osteoarthritic subchondral bone osteoblasts is an indicator of the metabolic state of these disease cells, Clin. Exp. Rheumatol., 26 (2008), 295-304. |
[10] |
I. Prasadam, R. Crawford, Y. Xiao, Aggravation of ADAMTS and matrix metalloproteinase production and role of ERK1/2 pathway in the interaction of osteoarthritic subchondral bone osteoblasts and articular cartilage chondrocytes-possible pathogenic role in osteoarthritis, J. Rheumatol., 39 (2012), 621-634. https://doi.org/10.3899/jrheum.110777 doi: 10.3899/jrheum.110777
![]() |
[11] |
S. J. Rice, K. Cheung, L. N. Reynard, J. Loughlin, Discovery and analysis of methylation quantitative trait loci (mQTLs) mapping to novel osteoarthritis genetic risk signals, Osteoarthritis Cartilage, 27 (2019), 1545-1556. https://doi.org/10.1016/j.joca.2019.05.017 doi: 10.1016/j.joca.2019.05.017
![]() |
[12] |
S. J. Rice, F. Beier, D. A. Young, J. Loughlin, Interplay between genetics and epigenetics in osteoarthritis, Nat. Rev. Rheumatol., 16 (2020), 268-281. https://doi.org/10.1038/s41584-020-0407-3 doi: 10.1038/s41584-020-0407-3
![]() |
[13] |
P. Y. Huang, J. G. Wu, J. Gu, T. Q. Zhang, L. F. Li, S. Q. Wang, et al., Bioinformatics analysis of miRNA and mRNA expression profiles to reveal the key miRNAs and genes in osteoarthritis, J. Orthop. Surg. Res., 16 (2021), 63. https://doi.org/10.1186/s13018-021-02201-2 doi: 10.1186/s13018-021-02201-2
![]() |
[14] |
J. Luo, X. Luo, Z. Duan, W. Bai, X. Che, Z. Shan, et al., Comprehensive analysis of lncRNA and mRNA based on expression microarray profiling reveals different characteristics of osteoarthritis between Tibetan and Han patients, J. Orthop. Surg. Res., 16 (2021), 133. https://doi.org/10.1186/s13018-021-02213-y doi: 10.1186/s13018-021-02213-y
![]() |
[15] |
J. Xu, Y. Zeng, H. Si, Y. Liu, M. Li, J. Zeng, et al., Integrating transcriptome-wide association study and mRNA expression profile identified candidate genes related to hand osteoarthritis, Arthritis Res. Ther., 23 (2021), 81. https://doi.org/10.1186/s13075-021-02458-2 doi: 10.1186/s13075-021-02458-2
![]() |
[16] |
C. Li, J. Luo, X. Xu, Z. Zhou, S. Ying, X. Liao, et al., Single cell sequencing revealed the underlying pathogenesis of the development of osteoarthritis, Gene, 757 (2020), 144939. https://doi.org/10.1016/j.gene.2020.144939 doi: 10.1016/j.gene.2020.144939
![]() |
[17] |
Z. Wu, L. Shou, J, Wang, X. Xu, Identification of the key gene and pathways associated with osteoarthritis via single-cell RNA sequencing on synovial fibroblasts, Medicine (Baltimore), 99 (2020), e21707. https://doi.org/10.1097/md.0000000000021707 doi: 10.1097/md.0000000000021707
![]() |
[18] |
Q. Sun, S. Liu, J. Feng, Y. Kang, Y. Zhou, S. Guo, Current status of microRNAs that target the wnt signaling pathway in regulation of osteogenesis and bone metabolism: A review, Med. Sci. Monit., 27 (2021), e929510. https://doi.org/10.12659/msm.929510 doi: 10.12659/msm.929510
![]() |
[19] | T. E. Swingler, L. Niu, P. Smith, P. Paddy, L. Le, M. J. Barter, et al., The function of microRNAs in cartilage and osteoarthritis, Clin. Exp. Rheumatol., 37 (2019), 40-47. |
[20] |
H. Tao, L. Cheng, R. Yang, Downregulation of miR-34a promotes proliferation and inhibits apoptosis of rat osteoarthritic cartilage cells by activating PI3K/Akt pathway, Clin. Interv. Aging, 15 (2020), 373-385. https://doi.org/10.2147/cia.S241855 doi: 10.2147/cia.S241855
![]() |
[21] |
X. Qiu, Y. Liu, H. Shen, Z. Wang, Y. Gong, J. Yang, et al., Single-cell RNA sequencing of human femoral head in vivo, Aging (Albany NY), 13 (2021), 15595-15619. https://doi.org/10.18632/aging.203124 doi: 10.18632/aging.203124
![]() |
[22] |
Y. Gong, J. Yang, X. Li, C. Zhou, Y. Chen, Z. Wang, et al., A systematic dissection of human primary osteoblasts in vivo at single-cell resolution, Aging (Albany NY), 13 (2021), 20629-20650. https://doi.org/10.18632/aging.203452 doi: 10.18632/aging.203452
![]() |
[23] |
A. Butler, P. Hoffman, P. Smibert, E. Papalexi, R. Satija, Integrating single-cell transcriptomic data across different conditions, technologies, and species, Nat. Biotechnol., 36 (2018), 411-420. https://doi.org/10.1038/nbt.4096 doi: 10.1038/nbt.4096
![]() |
[24] |
S. Lall, D. Sinha, S. Bandyopadhyay, D. Sengupta, Structure-aware principal component analysis for single-cell RNA-seq data, J. Comput. Biol., (2018). https://doi.org/10.1089/cmb.2018.0027 doi: 10.1089/cmb.2018.0027
![]() |
[25] |
F. Pont, M. Tosolini, J. J. Fournié, Single-Cell Signature Explorer for comprehensive visualization of single cell signatures across scRNA-seq datasets, Nucleic Acids Res., 47 (2019), e133. https://doi.org/10.1093/nar/gkz601 doi: 10.1093/nar/gkz601
![]() |
[26] |
A. N. Tikhonova, I. Dolgalev, H. Hu, K. K. Sivaraj, E. Hoxha, Á. Cuesta-Domínguez, et al., The bone marrow microenvironment at single-cell resolution, Nature, 569 (2019), 222-228. https://doi.org/10.1038/s41586-019-1104-8 doi: 10.1038/s41586-019-1104-8
![]() |
[27] |
Y. Matsushita, M. Nagata, K. M. Kozloff, J. D. Welch, K. Mizuhashi, N. Tokavanich, et al., A Wnt-mediated transformation of the bone marrow stromal cell identity orchestrates skeletal regeneration, Nat. Commun., 11 (2020), 332. https://doi.org/10.1038/s41467-019-14029-w doi: 10.1038/s41467-019-14029-w
![]() |
[28] |
X. Qiu, Q. Mao, Y. Tang, L. Wang, R. Chawla, H. A. Pliner, et al., Reversed graph embedding resolves complex single-cell trajectories, Nat. Methods, 14 (2017), 979-982. https://doi.org/10.1038/nmeth.4402 doi: 10.1038/nmeth.4402
![]() |
[29] |
G. Yu, L. G. Wang, Y. Han, Q. Y. He, clusterProfiler: an R package for comparing biological themes among gene clusters, Omics, 16 (2012), 284-287. https://doi.org/10.1089/omi.2011.0118 doi: 10.1089/omi.2011.0118
![]() |
[30] |
P. Shannon, A. Markiel, O. Ozier, N. S. Baliga, J. T. Wang, D. Ramage, et al., Cytoscape: a software environment for integrated models of biomolecular interaction networks, Genome Res., 13 (2003), 2498-2504. https://doi.org/10.1101/gr.1239303 doi: 10.1101/gr.1239303
![]() |
[31] |
M. E. Ritchie, B. Phipson, D. Wu, Y. Hu, C. W. Law, W. Shi, et al., limma powers differential expression analyses for RNA-sequencing and microarray studies, Nucleic Acids Res., 43 (2015), e47. https://doi.org/10.1093/nar/gkv007 doi: 10.1093/nar/gkv007
![]() |
[32] |
N. Maruotti, A. Corrado, F. P. Cantatore, Osteoblast role in osteoarthritis pathogenesis, J. Cell Physiol., 232 (2017), 2957-2963. https://doi.org/10.1002/jcp.25969 doi: 10.1002/jcp.25969
![]() |
[33] |
I. Titorencu, V. Pruna, V. V. Jinga, M. Simionescu, Osteoblast ontogeny and implications for bone pathology: an overview, Cell Tissue Res., 355 (2014), 23-33. https://doi.org/10.1007/s00441-013-1750-3 doi: 10.1007/s00441-013-1750-3
![]() |
[34] |
A. D. Theocharis, D. Manou, N. K. Karamanos, The extracellular matrix as a multitasking player in disease, Febs J., 286 (2019), 2830-2869. https://doi.org/10.1111/febs.14818 doi: 10.1111/febs.14818
![]() |
[35] |
H. Z. Li, H. D. Lu, Transcriptome analyses identify key genes and potential mechanisms in a rat model of osteoarthritis, J. Orthop. Surg. Res., 13 (2018), 319. https://doi.org/10.1186/s13018-018-1019-3 doi: 10.1186/s13018-018-1019-3
![]() |
[36] |
Y. Shi, X. Hu, J. Cheng, X. Zhang, F. Zhao, W. Shi, et al., A small molecule promotes cartilage extracellular matrix generation and inhibits osteoarthritis development, Nat. Commun., 10 (2019), 1914. https://doi.org/10.1038/s41467-019-09839-x doi: 10.1038/s41467-019-09839-x
![]() |
[37] |
J. Y. Li, M. Yu, A. M. Tyagi, C. Vaccaro, E. Hsu, J. Adams, et al., IL-17 receptor signaling in osteoblasts/osteocytes mediates PTH-induced bone loss and enhances osteocytic RANKL production, J. Bone Miner Res., 34 (2019), 349-360. https://doi.org/10.1002/jbmr.3600 doi: 10.1002/jbmr.3600
![]() |
[38] |
Z. Wang, J. Tan, L. Lei, W. Sun, Y. Wu, P. Ding, et al., The positive effects of secreting cytokines IL-17 and IFN-γ on the early-stage differentiation and negative effects on the calcification of primary osteoblasts in vitro, Int. Immunopharmacol., 57 (2018), 1-10. https://doi.org/10.1016/j.intimp.2018.02.002 doi: 10.1016/j.intimp.2018.02.002
![]() |
[39] |
H. Kitaura, A. Marahleh, F. Ohori, T. Noguchi, W. R. Shen, J. Qi, et al., Osteocyte-related cytokines regulate osteoclast formation and bone resorption, Int. J. Mol. Sci., 21 (2020). https://doi.org/10.3390/ijms21145169 doi: 10.3390/ijms21145169
![]() |
[40] |
N. Udagawa, M. Koide, M. Nakamura, Y. Nakamichi, T. Yamashita, S. Uehara, et al., Osteoclast differentiation by RANKL and OPG signaling pathways, J. Bone Miner Metab., 39 (2021), 19-26. https://doi.org/10.1007/s00774-020-01162-6 doi: 10.1007/s00774-020-01162-6
![]() |
[41] |
M. Croes, F. C. Öner, D. van Neerven, E. Sabir, M. C. Kruyt, T. J. Blokhuis, et al., Proinflammatory T cells and IL-17 stimulate osteoblast differentiation, Bone, 84 (2016), 262-270. https://doi.org/10.1016/j.bone.2016.01.010 doi: 10.1016/j.bone.2016.01.010
![]() |
[42] |
S. Shen, Y. Wu, J. Chen, Z. Xie, K. Huang, G. Wang, et al., CircSERPINE2 protects against osteoarthritis by targeting miR-1271 and ETS-related gene, Ann. Rheum. Dis., 78 (2019), 826-836. https://doi.org/10.1136/annrheumdis-2018-214786 doi: 10.1136/annrheumdis-2018-214786
![]() |
[43] |
Y. Chao, L. Zhang, X. Zhang, C. Ma, Z. Chen, Expression of MiR-140 and MiR-199 in synovia and its correlation with the progression of knee osteoarthritis, Med. Sci. Monit., 26 (2020), e918174. https://doi.org/10.12659/msm.918174 doi: 10.12659/msm.918174
![]() |
[44] |
B. Zhang, M. Sun, J. Wang, C. Ma, T. Hao, G. Liu, et al., MiR-671 ameliorates the progression of osteoarthritis in vitro and in vivo, Pathol. Res. Pract., 215 (2019), 152423. https://doi.org/10.1016/j.prp.2019.04.015 doi: 10.1016/j.prp.2019.04.015
![]() |
[45] |
Z. Rasheed, H. A. Al-Shobaili, N. Rasheed, A. A. Al Salloom, O. Al-Shaya, A. Mahmood, et al., Integrated study of globally expressed microRNAs in IL-1β-stimulated human osteoarthritis chondrocytes and osteoarthritis relevant genes: A microarray and bioinformatics analysis, Nucleosides Nucleotides Nucleic Acids, 35 (2016), 335-355. https://doi.org/10.1080/15257770.2016.1163380 doi: 10.1080/15257770.2016.1163380
![]() |
[46] |
A. Soyocak, H. Kurt, M. Ozgen, D. Turgut Cosan, E. Colak, H. V. Gunes, miRNA-146a, miRNA-155 and JNK expression levels in peripheral blood mononuclear cells according to grade of knee osteoarthritis, Gene, 627 (2017), 207-211. https://doi.org/10.1016/j.gene.2017.06.027 doi: 10.1016/j.gene.2017.06.027
![]() |
[47] | G. Wang, Y. Zhang, X. Zhao, C. Meng, L. Ma, Y. Kong, MicroRNA-411 inhibited matrix metalloproteinase 13 expression in human chondrocytes, Am. J. Transl. Res., 7 (2015), 2000-2006. |
[48] |
E. Nemoto, T. Honda, S. Kanaya, H. Takada, H. Shimauchi, Expression of functional Toll-like receptors and nucleotide-binding oligomerization domain proteins in murine cementoblasts and their upregulation during cell differentiation, J. Periodontal. Res., 43 (2008), 585-593. https://doi.org/10.1111/j.1600-0765.2008.01096.x doi: 10.1111/j.1600-0765.2008.01096.x
![]() |
![]() |
![]() |
1. | Xueyao Cai, Wenjun Shi, Jie Lian, Guoyou Zhang, Yuchen Cai, Lian Zhu, Characterization of immune landscape and development of a novel N7-methylguanine-related gene signature to aid therapy in recurrent aphthous stomatitis, 2023, 72, 1023-3830, 133, 10.1007/s00011-022-01665-0 | |
2. | Amina Waheed, Muhammad Farooq Rai, Osteoarthritis year in review 2023: genetics, genomics, and epigenetics, 2024, 32, 10634584, 128, 10.1016/j.joca.2023.11.006 | |
3. | Ziqian Wang, Xianni Yang, Haopeng Yu, Songsong Zhu, Ruiye Bi, Single-cell transcriptome sequencing in synovial joint: insights of new progenitors and targets in joint development and disease, 2025, 43, 1066-5099, 10.1093/stmcls/sxaf008 |