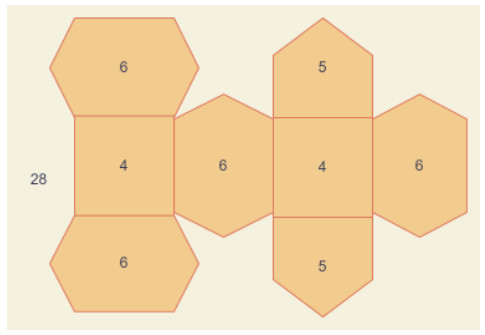
The recognition of denatured biological tissue is an indispensable part in the process of high intensity focused ultrasound treatment. As a nonlinear method, multi-scale permutation entropy (MPE) is widely used in the recognition of denatured biological tissue. However, the traditional MPE method neglects the amplitude information when calculating the time series complexity. The disadvantage will affect the recognition effect of denatured tissues. In order to solve the above problems, the method of multi-scale rescaled range permutation entropy (MRRPE) is proposed in this paper. The simulation results show that the MRRPE not only includes the amplitude information of the signal when calculating the signal complexity, but also extracts the extreme volatility characteristics of the signal effectively. The proposed method is applied to the HIFU echo signals during HIFU treatment, and the support vector machine (SVM) is used for recognition. The results show that compared with MPE and the multi-scale weighted permutation entropy (MWPE), the recognition rate of denatured biological tissue based on the MRRPE is higher, up to 96.57%, which can better recognize the non-denatured biological tissues and the denatured biological tissues.
Citation: Bei Liu, Wenbin Tan, Xian Zhang, Ziqi Peng, Jing Cao. Recognition study of denatured biological tissues based on multi-scale rescaled range permutation entropy[J]. Mathematical Biosciences and Engineering, 2022, 19(1): 102-114. doi: 10.3934/mbe.2022005
[1] | Huilin Ge, Yuewei Dai, Zhiyu Zhu, Biao Wang . Robust face recognition based on multi-task convolutional neural network. Mathematical Biosciences and Engineering, 2021, 18(5): 6638-6651. doi: 10.3934/mbe.2021329 |
[2] | Chii-Dong Ho, Gwo-Geng Lin, Thiam Leng Chew, Li-Pang Lin . Conjugated heat transfer of power-law fluids in double-pass concentric circular heat exchangers with sinusoidal wall fluxes. Mathematical Biosciences and Engineering, 2021, 18(5): 5592-5613. doi: 10.3934/mbe.2021282 |
[3] | José M. Sigarreta . Extremal problems on exponential vertex-degree-based topological indices. Mathematical Biosciences and Engineering, 2022, 19(7): 6985-6995. doi: 10.3934/mbe.2022329 |
[4] | Qingqun Huang, Muhammad Labba, Muhammad Azeem, Muhammad Kamran Jamil, Ricai Luo . Tetrahedral sheets of clay minerals and their edge valency-based entropy measures. Mathematical Biosciences and Engineering, 2023, 20(5): 8068-8084. doi: 10.3934/mbe.2023350 |
[5] | Hao Wang, Guangmin Sun, Kun Zheng, Hui Li, Jie Liu, Yu Bai . Privacy protection generalization with adversarial fusion. Mathematical Biosciences and Engineering, 2022, 19(7): 7314-7336. doi: 10.3934/mbe.2022345 |
[6] | Meili Tang, Qian Pan, Yurong Qian, Yuan Tian, Najla Al-Nabhan, Xin Wang . Parallel label propagation algorithm based on weight and random walk. Mathematical Biosciences and Engineering, 2021, 18(2): 1609-1628. doi: 10.3934/mbe.2021083 |
[7] | Xinmei Liu, Xinfeng Liang, Xianya Geng . Expected Value of Multiplicative Degree-Kirchhoff Index in Random Polygonal Chains. Mathematical Biosciences and Engineering, 2023, 20(1): 707-719. doi: 10.3934/mbe.2023032 |
[8] | Fengwei Li, Qingfang Ye, Juan Rada . Extremal values of VDB topological indices over F-benzenoids with equal number of edges. Mathematical Biosciences and Engineering, 2023, 20(3): 5169-5193. doi: 10.3934/mbe.2023240 |
[9] | Stefano Cosenza, Paolo Crucitti, Luigi Fortuna, Mattia Frasca, Manuela La Rosa, Cecilia Stagni, Lisa Usai . From Net Topology to Synchronization in HR Neuron Grids. Mathematical Biosciences and Engineering, 2005, 2(1): 53-77. doi: 10.3934/mbe.2005.2.53 |
[10] | Qiming Li, Tongyue Tu . Large-pose facial makeup transfer based on generative adversarial network combined face alignment and face parsing. Mathematical Biosciences and Engineering, 2023, 20(1): 737-757. doi: 10.3934/mbe.2023034 |
The recognition of denatured biological tissue is an indispensable part in the process of high intensity focused ultrasound treatment. As a nonlinear method, multi-scale permutation entropy (MPE) is widely used in the recognition of denatured biological tissue. However, the traditional MPE method neglects the amplitude information when calculating the time series complexity. The disadvantage will affect the recognition effect of denatured tissues. In order to solve the above problems, the method of multi-scale rescaled range permutation entropy (MRRPE) is proposed in this paper. The simulation results show that the MRRPE not only includes the amplitude information of the signal when calculating the signal complexity, but also extracts the extreme volatility characteristics of the signal effectively. The proposed method is applied to the HIFU echo signals during HIFU treatment, and the support vector machine (SVM) is used for recognition. The results show that compared with MPE and the multi-scale weighted permutation entropy (MWPE), the recognition rate of denatured biological tissue based on the MRRPE is higher, up to 96.57%, which can better recognize the non-denatured biological tissues and the denatured biological tissues.
To exemplify the phenomena of compounds scientifically, researchers utilize the contraption of the diagrammatic hypothesis, it is a well-known branch of geometrical science named graph theory. This division of numerical science provides its services in different fields of sciences. The particular example in networking [1], from electronics [2], and for the polymer industry, we refer to see [3]. Particularly in chemical graph theory, this division has extra ordinary assistance to study giant and microscope-able chemical compounds. For such a study, researchers made some transformation rules to transfer a chemical compound to a discrete pattern of shapes (graph). Like, an atom represents as a vertex and the covalent bonding between atoms symbolized as edges. Such transformation is known as molecular graph theory. A major importance of this alteration is that the hydrogen atoms are omitted. Some chemical structures and compounds conversion are presented in [4,5,6].
In cheminformatics, the topological index gains attraction due to its implementations. Various topological indices help to estimate a bio-activity and physicochemical characteristics of a chemical compound. Some interesting and useful topological indices for various chemical compounds are studied in [3,7,8]. A topological index modeled a molecular graph or a chemical compound into a numerical value. Since 1947, topological index implemented in chemistry [9], biology [10], and information science [11,12]. Sombor index and degree-related properties of simplicial networks [13], Nordhaus–Gaddum-type results for the Steiner Gutman index of graphs [14], Lower bounds for Gaussian Estrada index of graphs [15], On the sum and spread of reciprocal distance Laplacian eigenvalues of graphs in terms of Harary index [16], the expected values for the Gutman index, Schultz index, and some Sombor indices of a random cyclooctane chain [17,18,19], bounds on the partition dimension of convex polytopes [20,21], computing and analyzing the normalized Laplacian spectrum and spanning tree of the strong prism of the dicyclobutadieno derivative of linear phenylenes [22], on the generalized adjacency, Laplacian and signless Laplacian spectra of the weighted edge corona networks [23,24], Zagreb indices and multiplicative Zagreb indices of Eulerian graphs [25], Minimizing Kirchhoff index among graphs with a given vertex bipartiteness, [26], asymptotic Laplacian energy like invariant of lattices [27]. Few interesting studies regarding the chemical graph theory can be found in [28,29,30,31,32].
Recently, the researchers of [33] introduced a topological descriptor and called the face index. Moreover, the idea of computing structure-boiling point and energy of a structure, motivated them to introduced this parameter without heavy computation. They computed these parameters for different models compare the results with previous literature and found approximate solutions with comparatively less computations. This is all the blessings of face index of a graph. The major concepts of this research work are elaborated in the given below definitions.
Definition 1.1. [33] Let a graph G=(V(G),E(G),F(G)) having face, edge and vertex sets notation with F(G),E(G),V(G), respectively. It is mandatory that the graph is connected, simple and planar. If e from the edge set E(G), is one of those edges which surrounds a face, then the face f from the face set F(G), is incident to the edge e. Likewise, if a vertex α from the vertex set V(G) is at the end of those incident edges, then a face f is incident to that vertex. This face-vertex incident relation is symbolized here by the notation α∼f. The face degree of f in G is described as d(f)=∑α∼fd(α), which are elaborated in the Figure 1.
Definition 1.2. [33] The face index FI(G), for a graph G, is formulated as
FI(G)=∑f∈F(G)d(f)=∑α∼f,f∈F(G)d(α). |
In the Figure 1, we can see that there are two faces with degree 4, exactly two with five count and four with count of 6. Moreover, there is an external face with count of face degree 28, which is the count of vertices.
As the information given above that the face index is quite new and introduced in the year 2020, so there is not so much literature is available. A few recent studies on this topic are summarized here. A chemical compound of silicon carbides is elaborated with such novel definition in [34]. Some carbon nanotubes are discussed in [35]. Except for the face index, there are distance and degree-based graphical descriptors available in the literature. For example, distance-based descriptors of phenylene nanotube are studied in [36], and in [37] titania nanotubes are discussed with the same concept. Star networks are studied in [38], with the concept of degree-based descriptors. Bounds on the descriptors of some generalized graphs are discussed in [39]. General Sierpinski graph is discussed in [40], in terms of different topological descriptor aspects. The study of hyaluronic acid-doxorubicin ar found in [41], with the same concept of the index. The curvilinear regression model of the topological index for the COVID-19 treatment is discussed in [42]. For further reading and interesting advancements of topological indices, polynomials of zero-divisor structures are found in [43], zero divisor graph of commutative rings [44], swapped networks modeled by optical transpose interconnection system [45], metal trihalides network [46], some novel drugs used in the cancer treatment [47], para-line graph of Remdesivir used in the prevention of corona virus [48], tightest nonadjacently configured stable pentagonal structure of carbon nanocones [49]. In order to address a novel preventive category (P) in the HIV system known as the HIPV mathematical model, the goal of this study is to offer a design of a Morlet wavelet neural network (MWNN) [50].
In the next section, we discussed the newly developed face index or face-based index for different chemical compounds. Silicate network, triangular honeycomb network, carbon sheet, polyhedron generalized sheet, and generalized chain of silicate network are studied with the concept of the face-based index. Given that the face index is more versatile than vertex degree-based topological descriptors, this study will aid in understanding the structural characteristics of chemical networks. Only the difficulty authors will face to compute the face degree of a generalized network or structure, because it is more generalized version and taking degree based partition of edges into this umbrella of face index.
Silicates are formed when metal carbonates or metal oxides react with sand. The SiO4, which has a tetrahedron structure, is the fundamental chemical unit of silicates. The central vertex of the SiO4 tetrahedron is occupied by silicon ions, while the end vertices are occupied by oxygen ions [51,52,53]. A silicate sheet is made up of rings of tetrahedrons that are joined together in a two-dimensional plane by oxygen ions from one ring to the other to form a sheet-like structure. The silicate network SLn symbol, where n represents the total number of hexagons occurring between the borderline and center of the silicate network SLn. The silicate network of dimension one is depicted in Figure 2. It contain total 3n(5n+1) vertices are 36n2 edges. Moreover, the result required is detailed are available in Table 1.
Dimension | |f12| | |f15| | |f36| |
1 | 24 | 48 | 7 |
2 | 32 | 94 | 14 |
3 | 40 | 152 | 23 |
4 | 48 | 222 | 34 |
5 | 56 | 304 | 47 |
6 | 64 | 398 | 62 |
7 | 72 | 504 | 79 |
8 | 80 | 622 | 98 |
. | . | . | . |
. | . | . | . |
. | . | . | . |
n | 8n+16 | 6n2+28n+14 | n2+4n+2 |
Theorem 2.1. Let SLn be the silicate network of dimension n≥1. Then the face index of SLn is
FI(SLn)=126n2+720n+558. |
Proof. Consider SLn the graph of silicate network with dimension n. Suppose fi denotes the faces of graph SLn having degree i. that is, d(fi)=∑α∼fid(α)=i and |fi| denotes the number of faces with degree i. The graph SLn contains three types of internal faces f12, f15, f36, and single external face which is usually denoted by f∞.
If SLn has one dimension then sum of degree of vertices incident to the external face is 144 and when SLn has two dimension then sum of degree of incident vertices to the external face is 204 whenever SLn has three dimension then sum of degree of incident vertices to the external face is 264. Similarly, SLn has n−dimension then sum of degree of incident vertices to the external face is 60n+84.
The number of internal faces with degree in each dimension is mentioned in Table 1.
By using the definition of face index FI we have
FI(SLn)=∑α∼f∈F(SLn)d(α)=∑α∼f12∈F(SLn)d(α)+∑α∼f15∈F(SLn)d(α)+∑α∼f36∈F(SLn)d(α)+∑α∼f∞∈F(SLn)d(α)=|f12|(12)+|f15|(15)+|f36|(36)+(60n+84)=(8n+16)(12)+(6n2+28n+14)(15)+(n2+4n+2)(36)+60n+84=126n2+72n+558. |
Hence, this is our required result.
A chain silicate network of dimension (m,n) is symbolized as CSL(m,n) which is made by arranging (m,n) tetrahedron molecules linearly. A chain silicate network of dimension (m,n) with m,n≥1 where m denotes the number of rows and each row has n number of tetrahedrons. The following theorem formulates the face index FI for chain silicate network.
Theorem 2.2. Let CSL(m,n) be the chain of silicate network of dimension m,n≥1. Then the face index FI of the graph CSL(m,n) is
FI(CSL(m,n))={48n−12if m=1, n≥1;96m−12if n=1, m≥2;168m−60if n=2,m≥2;45m−9n+36mn−42if both m,n are even45m−9n+36mn−21otherwise. |
Proof. Let CSL(m,n) be the graph of chain silicate network of dimension (m,n) with m,n≥1 where m represents the number of rows and n is the number of tetrahedrons in each row. A graph CSL(m,n) for m=1 contains three type of internal faces f9, f12 and f15 with one external face f∞. While for m≥2, it has four type of internal faces f9, f12, f15 and f36 with one external face f∞. We want to evaluate the algorithm of face index FI for chain silicate network. We will discuss it in two different cases.
Case 1: When CSL(m,n) has one row (m=1) with n number of tetrahedrons as shown in the Figure 3.
The graph has three type of internal faces f9, f12 and f15 with one external face f∞. The sum of degree of incident vertices to the external face is 9n and number of faces are |f9|=2, |f12|=2n and |f15|=n−2. Now the face index FI of the graph CSL(m,n) is given by
FI(CSL(m,n))=∑α∼f∈F(CSL(m,n))d(α)=∑α∼f9∈F(CSL(m,n))d(α)+∑α∼f12∈F(CSL(m,n))d(α)+∑α∼f15∈F(CSL(m,n))d(α)+∑α∼f∞∈F(CSL(m,n))d(α)=|f9|(9)+|f12|(12)+|f15|(15)+(9n)=(2)(9)+(2n)(12)+(n−2)(15)+9n=48n−12. |
Case 2: When CSL(m,n) has more than one rows (m≠1) with n number of tetrahedrons in each row as shown in the Figure 4.
The graph has four type of internal faces f9, f12, f15 and f36 with one external face f∞. The sum of degree of incident vertices to the external face is
∑α∼f∞∈F(CSL(m,n))d(α)={18mif n=1, m≥1;27mif n=2, m≥1;30m+15n−30if both m,n are even30m+15n−33otherwise. |
The number of faces are |f9|, |f12|, f15 and |f36| are given by
|f9|={2if m is odd3+(−1)nif m is even.|f12|={2(2m+n−1)if m is odd4(⌊n+12⌋+2m−1)if m is even|f15|=(3m−2)n−m|f36|={(m−12)(n−1)if m is odd(2n+(−1)n−14)(m−22)nif m is even. |
Now the face index FI of the graph CSL(m,n) is given by
FI(CSL(m,n))=∑α∼f∈F(CSL(m,n))d(α)=∑α∼f9∈F(CSL(m,n))d(α)+∑α∼f12∈F(CSL(m,n))d(α)+∑α∼f15∈F(CSL(m,n))d(α)+∑α∼f36∈F(CSL(m,n))d(α)+∑α∼f∞∈F(CSL(m,n))d(α)=|f9|(9)+|f12|(12)+|f15|(15)+|f36|(36)+∑α∼f∞∈F(CSL(m,n))d(α). |
After some mathematical simplifications, we can get
FI(CSL(m,n))={48n−12if m=196m−12if n=1,∀m168m−60if n=2,∀m45m−9n+36mn−42if both m,n are even45m−9n+36mn−21otherwise. |
There are three regular plane tessellations known to exist, each constituted from the same type of regular polygon: triangular, square, and hexagonal. The triangular tessellation is used to define the hexagonal network, which is extensively studied in [54]. A dimensioned hexagonal network THk has 3k2−3k+1 vertices and 9k2−15k+6 edges, where k is the number of vertices on one side of the hexagon. It has 2k−2 diameter. There are six vertices of degree three that are referred to as corner vertices. Moreover, the result required detailed are available in the Table 2.
Dimension | |f12| | |f14| | |f17| | |f18| |
1 | 6 | 0 | 0 | 0 |
2 | 6 | 12 | 12 | 12 |
3 | 6 | 24 | 24 | 60 |
4 | 6 | 36 | 36 | 144 |
5 | 6 | 48 | 48 | 264 |
6 | 6 | 60 | 60 | 420 |
7 | 6 | 72 | 72 | 612 |
8 | 6 | 84 | 84 | 840 |
. | . | . | . | . |
. | . | . | . | . |
. | . | . | . | . |
k | 6 | 12(k−1) | 12(k−1) | 18k2−42k+24 |
Theorem 2.3. Let THk be the triangular honeycomb network of dimension k≥1. Then the face index of graph THk is
FI(THk)=324k2−336k+102. |
Proof. Consider THk be a graph of triangular honeycomb network. The graph TH1 has one internal and only one external face while graph THk with k≥2, contains four types of internal faces f12, f14, f17, and f18 with one external face f∞.
For TH1 the sum of degree of incident vertices to the external face is 18 and in TH2 the sum of degree of incident vertices to the external face is 66. Whenever the graph TH3, the sum of degree of incident vertices to the external face is 114. Similarly, for THk has n−dimension then sum of degree of incident vertices to the external face is 48k−30.
The number of internal faces with degree in each dimension is given in Table 2.
By using the definition of face index FI we have
FI(THk)=∑α∼f∈F(THk)d(α)=∑α∼f12∈F(THk)d(α)+∑α∼f14∈F(THk)d(α)+∑α∼f17∈F(THk)d(α)+∑α∼f18∈F(THk)d(α)+∑α∼f∞∈F(THk)d(α)=|f12|(12)+|f14|(14)+|f17|(17)+|f18|(18)+(48k−30)=(6)(12)+(12(k−1))(14)+(12(k−1))(17)+(18k2−42k+24)(18)+48k−30=324k2−336k+102. |
Hence, this is our required result.
Given carbon sheet in the Figure 6, is made by grid of hexagons. There are few types of carbon sheets are given in [55,56]. The carbon sheet is symbolize as HCSm,n, where n represents the total number of vertical hexagons and m denotes the horizontal hexagons. It contain total 4mn+2(n+m)−1 vertices and 6nm+2m+n−2 edges. Moreover, the result required detailed are available in Tables 3 and 4.
Dimension m | |f15| | |f16| | |f18| | |f∞| |
2 | 3 | 2(n−1) | n−1 | 20n+7 |
Dimension m | |f15| | |f16| | |f17| | |f18| | |f∞| |
2 | 3 | 2(n−1) | 0 | n−1 | 20n+7 |
3 | 2 | 2n | 1 | 3(n−1) | 20n+17 |
4 | 2 | 2n | 3 | 5(n−1) | 20n+27 |
5 | 2 | 2n | 5 | 7(n−1) | 20n+37 |
6 | 2 | 2n | 7 | 9(n−1) | 20n+47 |
. | . | . | . | . | . |
. | . | . | . | . | . |
. | . | . | . | . | . |
m | 2 | 2n | 2m−5 | 2mn−2m−3n+3 | 20n+10m−13 |
Theorem 2.4. Let HCSm,n be the carbon sheet of dimension (m,n) and m,n≥2. Then the face index of HCSm,n is
FI(HCSm,n)={70n+2ifm=236mn−14−2(n−4m)ifm≥3. |
Proof. Consider HCSm,n be the carbon sheet of dimension (m,n) and m,n≥2. Let fi denotes the faces of graph HCSm,n having degree i, which is d(fi)=∑α∼fid(α)=i, and |fi| denotes the number of faces with degree i. A graph HCSm,n for a particular value of m=2 contains three types of internal faces f15, f16, f17 and f18 with one external face f∞. While for the generalize values of m≥3, it contain four types of internal faces f15, f16 and f17 with one external face f∞ in usual manner. For the face index of generalize nanotube, we will divide into two cases on the values of m.
Case 1: When HCSm,n has one row or HCS2,n.
A graph HCSm,n for a this particular value of m=2 contains three types of internal faces |f15|=3, |f16|=2(n−1) and |f18|=n−1 with one external face f∞. For the face index of carbon sheet, details are given in the Table 3. Now the face index FI of the graph NT2,n is given by
FI(HCS2,n)=∑α∼f∈F(HCS2,n)d(α)=∑α∼f15∈F(HCS2,n)d(α)+∑α∼f16∈F(HCS2,n)d(α)+∑α∼f18∈F(HCS2,n)d(α)+∑α∼f∞∈F(HCS2,n)d(α)=|f15|(15)+|f16|(16)+|f18|(18)+20n+7.=3(15)+2(n−1)(16)+(n−1)(18)+20n+7.=70n+2. |
Case 2: When HCSm,n has m≥3 rows.
A graph HCSm,n for generalize values of m≥3 contains four types of internal faces |f15|=2, |f16|=2n, |f17|=2m−5 and |f18|=2mn−2m−3n+3 with one external face f∞. For the face index of carbon sheet, details are given in the Table 4. Now the face index FI of the graph NTm,n is given by
FI(HCSm,n)=∑α∼f∈F(HCSm,n)d(α)=∑α∼f15∈F(HCSm,n)d(α)+∑α∼f16∈F(HCSm,n)d(α)+∑α∼f17∈F(HCSm,n)d(α)+∑α∼f18∈F(HCSm,n)d(α)+∑α∼f∞∈F(HCSm,n)d(α)=|f15|(15)+|f16|(16)+|f17|(17)+|f18|(18)+20n+10m−13.=36mn−2n+8m−14. |
Given structure of polyhedron generalized sheet of C∗28 in the Figure 7, is made by generalizing a C∗28 polyhedron structure which is shown in the Figure 8. This particular structure of C∗28 polyhedron are given in [57]. The polyhedron generalized sheet of C∗28 is as symbolize PHSm,n, where n represents the total number of vertical C∗28 polyhedrons and m denotes the horizontal C∗28 polyhedrons. It contain total 23nm+3n+2m vertices and 33nm+n+m edges. Moreover, the result required detailed are available in Tables 3 and 5.
m | |f14| | |f15| | |f16| | |f17| | |f18| | |f20| | |f35| |
1 | 2n+1 | 2 | 4n−2 | 0 | 0 | 2n−1 | 0 |
2 | 2n+2 | 2 | 8n−2 | 2 | 2n−2 | 4n−2 | 2n−1 |
3 | 2n+3 | 2 | 12n−2 | 4 | 4n−4 | 6n−3 | 4n−2 |
. | . | . | . | . | . | . | . |
. | . | . | . | . | . | . | . |
. | . | . | . | . | . | . | . |
m | 2n+m | 2 | 4mn−2 | 2m−2 | 2mn−2(m+n)+2 | 2mn−m | 2mn−(m+2n)+1 |
Theorem 2.5. Let PHSm,n be the polyhedron generalized sheet of C∗28 of dimension (m,n) and m,n≥1. Then the face index of PHSm,n is
FI(PHSm,n)=210mn−2(3m+5n). |
Proof. Consider PHSm,n be the polyhedron generalized sheet of C∗28 of dimension (m,n) and m,n≥1. Let fi denotes the faces of graph PHSm,n having degree i, which is d(fi)=∑α∼fid(α)=i, and |fi| denotes the number of faces with degree i. A graph PHSm,n for the generalize values of m,n≥1, it contain seven types of internal faces f14,f15,f16,f17,f18,f20 and f35 with one external face f∞ in usual manner. For the face index of polyhedron generalized sheet, details are given in the Table 5.
A graph PHSm,n for generalize values of m,n≥1 contains seven types of internal faces |f14|=2n+m, |f15|=2, |f16|=4nm−2, |f17|=2(m−1), |f18|=2nm−2(m+n)+2, |f20|=2nm−2mn−m, and |f35|=2mn−m−2n+1 with one external face f∞. Now the face index FI of the graph PHSm,n is given by
FI(PHSm,n)=∑α∼f∈F(PHSm,n)d(α)=∑α∼f14∈F(PHSm,n)d(α)+∑α∼f15∈F(PHSm,n)d(α)+∑α∼f16∈F(PHSm,n)d(α)+∑α∼f17∈F(PHSm,n)d(α)+∑α∼f18∈F(PHSm,n)d(α)+∑α∼f20∈F(PHSm,n)d(α)+∑α∼f35∈F(PHSm,n)d(α)+∑α∼f∞∈F(PHSm,n)d(α)=|f14|(14)+|f15|(15)+|f16|(16)+|f17|(17)+|f18|(18)+|f20|(20)+|f35|(35)+37m+68n−35.=210mn−6m−10n. |
With the advancement of technology, types of equipment and apparatuses of studying different chemical compounds are evolved. But topological descriptors or indices are still preferable and useful tools to develop numerical science of compounds. Therefore, from time to time new topological indices are introduced to study different chemical compounds deeply. In this study, we discussed a newly developed tool of some silicate type networks and generalized sheets, carbon sheet, polyhedron generalized sheet, with the face index concept. It provides numerical values of these networks based on the information of faces. It also helps to study physicochemical characteristics based on the faces of silicate networks.
M. K. Jamil conceived of the presented idea. K. Dawood developed the theory and performed the computations. M. Azeem verified the analytical methods, R. Luo investigated and supervised the findings of this work. All authors discussed the results and contributed to the final manuscript.
This work was supported by the National Science Foundation of China (11961021 and 11561019), Guangxi Natural Science Foundation (2020GXNSFAA159084), and Hechi University Research Fund for Advanced Talents (2019GCC005).
The authors declare that they have no conflicts of interest.
[1] | M. Diana, L. Schiraldi, Y. Y. Liu, R. Memeo, D. Mutter, P. Pessaux, et al., High intensity focused ultrasound (HIFU) applied to hepato-bilio-pancreatic and the digestive system-current state of the art and future perspectives, Hepatobiliary Surg. Nutr., 5 (2016), 329. doi: 10.21037/hbsn.2015.11.03. |
[2] |
L. Hallez, F.Touyeras, J. Y. KHihn, J. L. Guey, M. Spajer, Y. Bailly, Characterization of HIFU transducers designed for sonochemistry application: Acoustic streaming, Ultrason. Sonochem., 29 (2016), 420-427. doi: 10.1016/j.ultsonch.2015.10.019. doi: 10.1016/j.ultsonch.2015.10.019
![]() |
[3] | M. Marinova, M. Rauch, M. Mücke, High-intensity focused ultrasound (HIFU) for pancreatic carcinoma: evaluation of feasibility, reduction of tumour volume and pain intensity, Eur. Radiol., 26 (2016), 1-10. doi: 10.1007/s00330-016-4239-0. |
[4] |
W. Lei, J. Hu, Y. Liu, W. Liu, X. Chen, Numerical evaluation of high-intensity focused ultrasound-induced thermal lesions in atherosclerotic plaques, Math. Biosci. Eng., 18 (2021), 1154-1168. doi: 10.3934/mbe.2021062. doi: 10.3934/mbe.2021062
![]() |
[5] |
D. Cranston, A review of high intensity focused ultrasound in relation to the treatment of renal tumours and other malignancies, Ultrason. Son., 27 (2015), 654-658. doi: 10.1016/j.ultsonch.2015.05.035. doi: 10.1016/j.ultsonch.2015.05.035
![]() |
[6] |
N. Ellens, K. Hynynen, Frequency considerations for deep ablation with high-intensity focused ultrasound: A simulation study, Med. Phys., 42 (2015), 4896-4910. doi: 10.1118/1.4927060. doi: 10.1118/1.4927060
![]() |
[7] |
R. P. Ramaekers, M. D. Greef, J. M. M. Breugel, C. T. W. Moonen, M. Ries, Increasing the HIFU ablation rate through an MRI-guided sonication strategy using shock waves: Feasibility in the in vivo porcine liver, Phys. Med. Biol., 61 (2016), 1057-1077. doi: 10.1088/0031-9155/61/3/1057. doi: 10.1088/0031-9155/61/3/1057
![]() |
[8] |
H. Dong, G. Liu, X. Tong, Influence of temperature-dependent acoustic and thermal parameters and nonlinear harmonics on the prediction of thermal lesion under HIFU ablation, Math. Biosci. Eng., 18 (2021), 1340-1351. doi: 10.3934/mbe.2021070. doi: 10.3934/mbe.2021070
![]() |
[9] | B. Liu, R. Wang, Z. Peng, L. Qin, Identification of denatured biological tissues based on compressed sensing and improved multiscale dispersion entropy during HIFU treatment, Entropy, 22 (2020), 944. doi: 10.3390/e22090944. |
[10] |
J. S. Lee, T. E. Kim, J. H. Kim, B. J. Park, Unintended pregnancies with term delivery following ultrasound-guided high-intensity focused ultrasound (USgHIFU) ablation of uterine fibroid and adenomyosis, Clin. Exp. Obstet. Gyn., 45 (2018), 842-844. doi: 10.12891/ceog4472.2018. doi: 10.12891/ceog4472.2018
![]() |
[11] | S. Rahimian, J. Tavakkoli, Estimating dynamic changes of tissue attenuation coefficient during high-intensity focused ultrasound treatment, J. Ther. Ultrasound., 1 (2013), 14. doi: 10.1186/2050-5736-1-14. |
[12] | S. Q. Yan, H. Zhang, B. Liu, H. Tang, S. Y. Qian, Identification of denatured and normal biological tissues based on compressed sensing and refined composite multi-scale fuzzy entropy during high intensity focused ultrasound treatment, Chin. Phys. B, 30 (2021), 028704. doi: 10.1088/16741056/abcfa7. |
[13] | R. Seip, J. Tavakkoli, R. F. Carlson, A. Wunderlich, N. T. Sanghvi, K. A. Dines, et al., High-intensity focused ultrasound (HIFU) multiple lesion imaging: comparison of detection algorithms for real-time treatment control, in 2002 IEEE Ultrasonics Symposium, 2 (2002), 1427-1430. doi: 10.1109/ULTSYM.2002.1192564. |
[14] | T. Shishitani, S. Yoshizawa, S. Umemura, Change in acoustic impedance and sound speed of HIFU-exposed chicken breast muscle, in 2010 IEEE International Ultrasonics Symposium, (2010), 1384-1387. doi: 10.1109/ULTSYM.2010.5935709. |
[15] | S. Mobasheri, H. Behnam, P. Rangraz, Radio frequency ultrasound time series signal analysis to evaluate high-intensity focused ultrasound lesion formation status in tissue. J. Med. Signals. Sens., 6 (2016), 91. doi: 10.4103/2228-7477.181032. |
[16] | P. H. Tsui, Y. L. Wan, Effects of fatty infiltration of the liver on the Shannon entropy of ultrasound backscattered signals, Entropy, 18 (2016), 341. doi: 10.3390/e18090341. |
[17] | M. M. Monfared, H. Behnam, P. Rangraz, High-intensity focused ultrasound thermal lesion detection using entropy imaging of ultrasound radio frequency signal time series, J. Med. Ultrasound., 26 (2018), 24. doi: 10.4103/JMU.JMU_3_17. |
[18] | C. Bandt, B. Pompe, Permutation entropy: a natural complexity measure for time series, Phys. Rev. Lett., 88 (2002), 174102. doi: 10.1103/PhysRevLett.88.174102. |
[19] | Y. Gao, F. Villecco, M. Li, W. Song, Multi-scale permutation entropy based on improved LMD and HMM for rolling bearing diagnosis, Entropy, 19 (2017), 176. doi: 10.3390/e19040176. |
[20] | W. P. Yao, T. B. Liu, J. F. Dai, J. Wang, Multiscale permutation entropy analysis of electroencephalogram, Acta. Phys. Sin, 63 (2014), 078704. doi: 10.7498/aps.63.078704. |
[21] |
J. Murillo-Escobar, Y. E. Jaramillo-Munera, D. A. Orrego-Metaute, E. Delgado-Trejos, D. Cuesta-Frau, Muscle fatigue analysis during dynamic contractions based on biomechanical features and Permutation Entropy, Math. Biosci. Eng., 17 (2020), 2592-2615. doi: 10.3934/mbe.2020142. doi: 10.3934/mbe.2020142
![]() |
[22] | B. Liu, W. P. Hu, X. Zou, Y. J. Ding, S. Y. Qian, Recognition of denatured biological tissue based on variational mode decomposition and multi-scale permutation entropy, Acta. Phys. Sin, 68 (2019), 028702. doi: 10.7498/aps.68.20181772. |
[23] |
S. D. Wu, C. W. Wu, S. G. Lin, C. C. Wang, K. Y. Lee, Time series analysis using composite multiscale entropy, Entropy, 15 (2013), 1069-1084. doi: 10.3390/e15031069. doi: 10.3390/e15031069
![]() |
[24] |
S. D. Wu, C. W. Wu, S. G. Lin, K. Y. Lee, C. K. Peng, Analysis of complex time series using refined composite multiscale entropy, Phys. Lett. A, 378 (2014), 1369-1374. doi: 10.1016/j.physleta.2014.03.034. doi: 10.1016/j.physleta.2014.03.034
![]() |
[25] |
D. Cuesta-Frau, Permutation entropy: Influence of amplitude information on time series classification performance, Math. Biosci. Eng., 16 (2019), 6842-6857. doi: 10.3934/mbe.2019342. doi: 10.3934/mbe.2019342
![]() |
[26] | B. Fadlallah, B. Chen, A. Keil, J. Principe, Weighted-permutation entropy: A complexity measure for time series incorporating amplitude information, Phys. Rev. E, 87 (2013) 022911. doi: 10.1103/PhysRevE.87.022911. |
[27] | B. Liu, S. Qian, W. Hu, Identification of denatured biological tissues based on time-frequency entropy and refined composite multi-scale weighted permutation entropy during HIFU treatment, Entropy, 21 (2019), 666. doi: 10.3390/e21070666. |
[28] | J. C. Zhang, W. K.Ren, N. D. Jin, Rescaled range permutation entropy: a method for quantifying the dynamical complexity of extreme volatility in chaotic time series, Chinese. Phys. Lett., 37 (2020), 090501. doi: 10.1088/0256-307X/37/9/090501. |
1. | Shabana Anwar, Muhammad Kamran Jamil, Amal S. Alali, Mehwish Zegham, Aisha Javed, Extremal values of the first reformulated Zagreb index for molecular trees with application to octane isomers, 2023, 9, 2473-6988, 289, 10.3934/math.2024017 | |
2. | Ali N. A. Koam, Ali Ahmad, Raed Qahiti, Muhammad Azeem, Waleed Hamali, Shonak Bansal, Enhanced Chemical Insights into Fullerene Structures via Modified Polynomials, 2024, 2024, 1076-2787, 10.1155/2024/9220686 | |
3. | Ali Ahmad, Ali N. A. Koam, Muhammad Azeem, Reverse-degree-based topological indices of fullerene cage networks, 2023, 121, 0026-8976, 10.1080/00268976.2023.2212533 | |
4. | Muhammad Waheed Rasheed, Abid Mahboob, Iqra Hanif, Uses of degree-based topological indices in QSPR analysis of alkaloids with poisonous and healthful nature, 2024, 12, 2296-424X, 10.3389/fphy.2024.1381887 | |
5. | Shriya Negi, Vijay Kumar Bhat, Face Index of Silicon Carbide Structures: An Alternative Approach, 2024, 16, 1876-990X, 5865, 10.1007/s12633-024-03119-0 | |
6. | Haseeb AHMAD, Muhammad AZEEM, Face-degree-based topological descriptors of germanium phosphide, 2024, 52, 18722040, 100429, 10.1016/j.cjac.2024.100429 | |
7. | Belman Gautham Shenoy, Raghavendra Ananthapadmanabha, Badekara Sooryanarayana, Prasanna Poojary, Vishu Kumar Mallappa, 2024, Rational Wiener Index and Rational Schultz Index of Graphs, 180, 10.3390/engproc2023059180 |
Dimension | |f12| | |f15| | |f36| |
1 | 24 | 48 | 7 |
2 | 32 | 94 | 14 |
3 | 40 | 152 | 23 |
4 | 48 | 222 | 34 |
5 | 56 | 304 | 47 |
6 | 64 | 398 | 62 |
7 | 72 | 504 | 79 |
8 | 80 | 622 | 98 |
. | . | . | . |
. | . | . | . |
. | . | . | . |
n | 8n+16 | 6n2+28n+14 | n2+4n+2 |
Dimension | |f12| | |f14| | |f17| | |f18| |
1 | 6 | 0 | 0 | 0 |
2 | 6 | 12 | 12 | 12 |
3 | 6 | 24 | 24 | 60 |
4 | 6 | 36 | 36 | 144 |
5 | 6 | 48 | 48 | 264 |
6 | 6 | 60 | 60 | 420 |
7 | 6 | 72 | 72 | 612 |
8 | 6 | 84 | 84 | 840 |
. | . | . | . | . |
. | . | . | . | . |
. | . | . | . | . |
k | 6 | 12(k−1) | 12(k−1) | 18k2−42k+24 |
Dimension m | |f15| | |f16| | |f18| | |f∞| |
2 | 3 | 2(n−1) | n−1 | 20n+7 |
Dimension m | |f15| | |f16| | |f17| | |f18| | |f∞| |
2 | 3 | 2(n−1) | 0 | n−1 | 20n+7 |
3 | 2 | 2n | 1 | 3(n−1) | 20n+17 |
4 | 2 | 2n | 3 | 5(n−1) | 20n+27 |
5 | 2 | 2n | 5 | 7(n−1) | 20n+37 |
6 | 2 | 2n | 7 | 9(n−1) | 20n+47 |
. | . | . | . | . | . |
. | . | . | . | . | . |
. | . | . | . | . | . |
m | 2 | 2n | 2m−5 | 2mn−2m−3n+3 | 20n+10m−13 |
m | |f14| | |f15| | |f16| | |f17| | |f18| | |f20| | |f35| |
1 | 2n+1 | 2 | 4n−2 | 0 | 0 | 2n−1 | 0 |
2 | 2n+2 | 2 | 8n−2 | 2 | 2n−2 | 4n−2 | 2n−1 |
3 | 2n+3 | 2 | 12n−2 | 4 | 4n−4 | 6n−3 | 4n−2 |
. | . | . | . | . | . | . | . |
. | . | . | . | . | . | . | . |
. | . | . | . | . | . | . | . |
m | 2n+m | 2 | 4mn−2 | 2m−2 | 2mn−2(m+n)+2 | 2mn−m | 2mn−(m+2n)+1 |
Dimension | |f12| | |f15| | |f36| |
1 | 24 | 48 | 7 |
2 | 32 | 94 | 14 |
3 | 40 | 152 | 23 |
4 | 48 | 222 | 34 |
5 | 56 | 304 | 47 |
6 | 64 | 398 | 62 |
7 | 72 | 504 | 79 |
8 | 80 | 622 | 98 |
. | . | . | . |
. | . | . | . |
. | . | . | . |
n | 8n+16 | 6n2+28n+14 | n2+4n+2 |
Dimension | |f12| | |f14| | |f17| | |f18| |
1 | 6 | 0 | 0 | 0 |
2 | 6 | 12 | 12 | 12 |
3 | 6 | 24 | 24 | 60 |
4 | 6 | 36 | 36 | 144 |
5 | 6 | 48 | 48 | 264 |
6 | 6 | 60 | 60 | 420 |
7 | 6 | 72 | 72 | 612 |
8 | 6 | 84 | 84 | 840 |
. | . | . | . | . |
. | . | . | . | . |
. | . | . | . | . |
k | 6 | 12(k−1) | 12(k−1) | 18k2−42k+24 |
Dimension m | |f15| | |f16| | |f18| | |f∞| |
2 | 3 | 2(n−1) | n−1 | 20n+7 |
Dimension m | |f15| | |f16| | |f17| | |f18| | |f∞| |
2 | 3 | 2(n−1) | 0 | n−1 | 20n+7 |
3 | 2 | 2n | 1 | 3(n−1) | 20n+17 |
4 | 2 | 2n | 3 | 5(n−1) | 20n+27 |
5 | 2 | 2n | 5 | 7(n−1) | 20n+37 |
6 | 2 | 2n | 7 | 9(n−1) | 20n+47 |
. | . | . | . | . | . |
. | . | . | . | . | . |
. | . | . | . | . | . |
m | 2 | 2n | 2m−5 | 2mn−2m−3n+3 | 20n+10m−13 |
m | |f14| | |f15| | |f16| | |f17| | |f18| | |f20| | |f35| |
1 | 2n+1 | 2 | 4n−2 | 0 | 0 | 2n−1 | 0 |
2 | 2n+2 | 2 | 8n−2 | 2 | 2n−2 | 4n−2 | 2n−1 |
3 | 2n+3 | 2 | 12n−2 | 4 | 4n−4 | 6n−3 | 4n−2 |
. | . | . | . | . | . | . | . |
. | . | . | . | . | . | . | . |
. | . | . | . | . | . | . | . |
m | 2n+m | 2 | 4mn−2 | 2m−2 | 2mn−2(m+n)+2 | 2mn−m | 2mn−(m+2n)+1 |