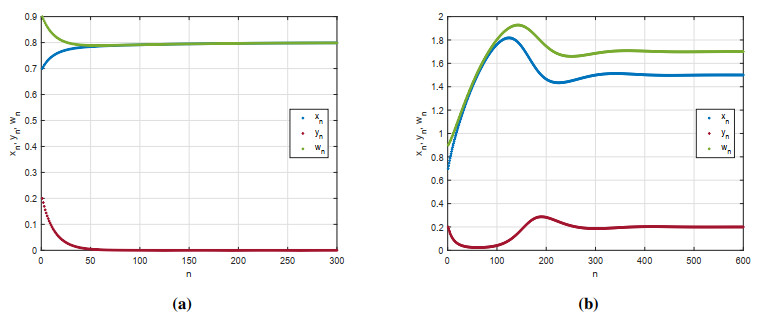
In this paper we introduce and analyze a non-standard discretized SIS epidemic model for a homogeneous population. The presented model is a discrete version of the continuous model known from literature and used by us for building a model for a heterogeneous population. Firstly, we discuss basic properties of the discrete system. In particular, boundedness of variables and positivity of solutions of the system are investigated. Then we focus on stability of stationary states. Results for the disease-free stationary state are depicted with the use of a basic reproduction number computed for the system. For this state we also manage to prove its global stability for a given condition. It transpires that the behavior of the disease-free state is the same as its behavior in the analogous continuous system. In case of the endemic stationary state, however, the results are presented with respect to a step size of discretization. Local stability of this state is guaranteed for a sufficiently small critical value of the step size. We also conduct numerical simulations confirming theoretical results about boundedness of variables and global stability of the disease-free state of the analyzed system. Furthermore, the simulations ascertain a possibility of appearance of Neimark-Sacker bifurcation for the endemic state. As a bifurcation parameter the step size of discretization is chosen. The simulations suggest the appearance of a supercritical bifurcation.
Citation: Marcin Choiński, Mariusz Bodzioch, Urszula Foryś. A non-standard discretized SIS model of epidemics[J]. Mathematical Biosciences and Engineering, 2022, 19(1): 115-133. doi: 10.3934/mbe.2022006
[1] | Hui Cao, Yicang Zhou, Zhien Ma . Bifurcation analysis of a discrete SIS model with bilinear incidence depending on new infection. Mathematical Biosciences and Engineering, 2013, 10(5&6): 1399-1417. doi: 10.3934/mbe.2013.10.1399 |
[2] | Jianquan Li, Zhien Ma, Fred Brauer . Global analysis of discrete-time SI and SIS epidemic models. Mathematical Biosciences and Engineering, 2007, 4(4): 699-710. doi: 10.3934/mbe.2007.4.699 |
[3] | Yicang Zhou, Zhien Ma . Global stability of a class of discrete age-structured SIS models with immigration. Mathematical Biosciences and Engineering, 2009, 6(2): 409-425. doi: 10.3934/mbe.2009.6.409 |
[4] | A. Q. Khan, M. Tasneem, M. B. Almatrafi . Discrete-time COVID-19 epidemic model with bifurcation and control. Mathematical Biosciences and Engineering, 2022, 19(2): 1944-1969. doi: 10.3934/mbe.2022092 |
[5] | Ke Guo, Wanbiao Ma . Global dynamics of an SI epidemic model with nonlinear incidence rate, feedback controls and time delays. Mathematical Biosciences and Engineering, 2021, 18(1): 643-672. doi: 10.3934/mbe.2021035 |
[6] | Yoichi Enatsu, Yukihiko Nakata, Yoshiaki Muroya . Global stability for a class of discrete SIR epidemic models. Mathematical Biosciences and Engineering, 2010, 7(2): 347-361. doi: 10.3934/mbe.2010.7.347 |
[7] | Ceyu Lei, Xiaoling Han, Weiming Wang . Bifurcation analysis and chaos control of a discrete-time prey-predator model with fear factor. Mathematical Biosciences and Engineering, 2022, 19(7): 6659-6679. doi: 10.3934/mbe.2022313 |
[8] | Parvaiz Ahmad Naik, Muhammad Amer, Rizwan Ahmed, Sania Qureshi, Zhengxin Huang . Stability and bifurcation analysis of a discrete predator-prey system of Ricker type with refuge effect. Mathematical Biosciences and Engineering, 2024, 21(3): 4554-4586. doi: 10.3934/mbe.2024201 |
[9] | John E. Franke, Abdul-Aziz Yakubu . Periodically forced discrete-time SIS epidemic model with disease induced mortality. Mathematical Biosciences and Engineering, 2011, 8(2): 385-408. doi: 10.3934/mbe.2011.8.385 |
[10] | Xin-You Meng, Tao Zhang . The impact of media on the spatiotemporal pattern dynamics of a reaction-diffusion epidemic model. Mathematical Biosciences and Engineering, 2020, 17(4): 4034-4047. doi: 10.3934/mbe.2020223 |
In this paper we introduce and analyze a non-standard discretized SIS epidemic model for a homogeneous population. The presented model is a discrete version of the continuous model known from literature and used by us for building a model for a heterogeneous population. Firstly, we discuss basic properties of the discrete system. In particular, boundedness of variables and positivity of solutions of the system are investigated. Then we focus on stability of stationary states. Results for the disease-free stationary state are depicted with the use of a basic reproduction number computed for the system. For this state we also manage to prove its global stability for a given condition. It transpires that the behavior of the disease-free state is the same as its behavior in the analogous continuous system. In case of the endemic stationary state, however, the results are presented with respect to a step size of discretization. Local stability of this state is guaranteed for a sufficiently small critical value of the step size. We also conduct numerical simulations confirming theoretical results about boundedness of variables and global stability of the disease-free state of the analyzed system. Furthermore, the simulations ascertain a possibility of appearance of Neimark-Sacker bifurcation for the endemic state. As a bifurcation parameter the step size of discretization is chosen. The simulations suggest the appearance of a supercritical bifurcation.
In most cases mathematical modeling of epidemic dynamics is based on formulating continuous systems. Discrete systems are less often applied. However, using computer software for simulations is becoming increasingly common. Furthermore, the epidemiological data reflect values of particular magnitudes only for determined moments. Because of these reasons, the discrete approach is gaining attention. The examples of discrete models describing epidemic phenomena can be found e.g., in [1,2].
Generally discrete models are constructed on the grounds of their continuous counterparts. One of the easiest method of discretization is the explicit Euler method (EEM). However, applying this method can lead to dynamical inconsistency of discrete models with their continuous analogues. In particular, there can arise biologically unreasonable bifurcating behavior. Moreover, negativity of system's solutions can occur. To avoid these nuisances, one can apply the non-standard discretization method (NSDM), which is presented and discussed in [3]. Discrete models exploiting this method are dynamically consistent with their continuous counterparts [4].
Even in systems discretized using the NSDM bifurcations can occur. Here we will focus on the possibility of appearing Neimark-Sacker bifurcation (NSB) in the analyzed system. We say that in a system of difference equations there can occur NSB if the Jacobian matrix for a given stationary state has two coupled complex eigenvalues with non-zero imaginary parts and modulus equal to 1 [5]. This bifurcation is a discrete counterpart for Hopf bifurcation in continuous systems. However, the Hopf bifurcation is a term used also for a discrete case [6].
In NSB we identify a bifurcation parameter, which critical value gives desirable eigenvalues. There are two types of NSB: supercritical and subcritical one. In the supercritical NSB for bifurcation parameter's values below critical one a given stationary state is a sink. After exceeding this critical value this state loses stability and there arises a stable isolated closed invariant curve which surrounds the stationary state. In the subcritical bifurcation before exceeding critical value there is an analogous unstable curve surrounding a stable stationary state. After exceeding this value, the curve disappears and the stationary state loses stability [5].
Here we will consider a system belonging to the class of SIS (susceptible-infected-susceptible) models. In this kind of models it is assumed that there is no long-lasting immunity and immediately after infection individuals become susceptible again. The exemplary basic SIS models were presented in [7,8].
Authors in [9] analyze a discrete SIS model with the use of the EEM. The authors use a disease incidence function relied on the mass-action law and assume that growth of a population is constant. Dynamically consistent SIS models are presented in [10]. Here the standard incidence function was chosen. The discrete SIS model from [11] with the NSDM is analyzed in the context of the basic reproduction number. A similar approach is used in [12], where authors use a variation of the backward Euler discretization. Currently the main contribution of analysis of SIS models is related to epidemic spread in complex networks. In [13] authors investigate a continuous model for such a network, assuming that infection rates of multiple edges interfere with one another. A continuous model is analyzed also in [14], where an infective medium is considered. A discrete approach with the EEM is presented in [15]. However, in this three papers immigration, birth and death processes are neglected. Dynamics of the current COVID-19 pandemic is also an issue investigated from the mathematical point of view. In [16] both discrete and continuous models are introduced. Here the author includes also an additional group of recovered people, proposing SIR type (susceptible-infected-recovered) model. An incubation period is considered and an incidence function for a continuous case is built with the use of an integral.
In our previous papers (see [17,18]) we analyzed continuous SIS models differing in a type of inflow into subpopulations. Basing on the system from [17], we constructed the discrete model with the use of the EEM. This model was discussed in [19]. Here we will show a modification of this discrete model with the use of the NSDM.
This paper is organized as follows. In the next section we quote the continuous model from [17] and the discrete model with the use of the EEM from [19]. Then we introduce the non-standard discretized model and show the basic properties of this model. Later we focus on the stability analysis of stationary states of the system. Finally, we discuss the possibility of NSB appearance in the system. In the last part we give conclusions. Our theoretical results are complemented with numerical simulations with the use of the Matlab software.
In the paper we will assume that N means the set of all natural numbers including zero and N+:=N∖{0}.
Let us first remind the continuous model analyzed in [17]. This model describes the epidemic spread in a homogeneous population in which we consider two exclusive groups: healthy (susceptible) and infected people. The sizes of these groups at time t are denoted by S(t) and I(t), accordingly. Naturally, these sizes can be interpreted as densities. The model has the form:
˙S=C−βSI+γI−μS,˙I=βSI−(γ+α+μ)I, | (2.1) |
where C reflects an inflow into the population and coefficients: β, γ, μ and α correspond to illness transmission, recovery, natural death and disease-related death, respectively. The parameters appearing in system (2.1) are constant and positive. Because of the meaning of the parameters, it is reasonable to assume C≫μ.
We conducted scaling of system (2.1) in order to reduce the number of parameters. Eliminating β and γ, we obtain
x′=C−xy+y−μx,y′=xy−ky, | (2.2) |
where x=βS, y=βI, k=1+α+μ and the coefficients in system (2.2) are scaled accordingly.
Let us remind the basic properties of system (2.2). The form of the right-hand side of system (2.2) guarantees existence, uniqueness and positiveness of solutions for positive initial conditions. The basic reproduction number R0 for system (2.2) is equal to
R0=Cμk. | (2.3) |
We will refer to this value also in the case of discrete systems.
System (2.2) has two stationary states:
1) disease-free: Ed=(xd,yd)=(Cμ,0), always existing,
2) endemic: Ee=(xe,ye)=(k,C−μkk−1) which exists for C>μk, that is for R0>1.
The state Ed is locally stable for Cμ<k. If Cμ>k (i.e., when Ee exists), then Ed is a saddle point. The state Ee is always locally stable under its existence. Furthermore, the Poincaré Theorem implies global stability – either Ed is globally stable whenever Ee does not exist, or Ee is globally stable whenever exists.
Now let us remind the discrete version of system (2.2) with the use of the EEM. This model was introduced and analyzed in [19]. Let n∈N be an n-th node in a discrete timescale. After discretization we obtained the system
xn+1=xn+h(C−xnyn+yn−μxn),yn+1=yn+h(xnyn−kyn), | (2.4) |
where h is a step size of discretization.
Now we quote the properties of system (2.4). Basing on the approach presented in [20] dedicated for discrete systems, we computed R0 for system (2.4) and obtained again system (2.3). Let us define a variable wn as
wn:=xn+yn, |
meaning the size of the whole population for the n-th point in the discrete timescale. Adding by sides the equations from system (2.4), we get
wn+1=wn+h(C−μwn−αyn)≤wn+h(C−μwn). | (2.5) |
Solving inequality (Eq 2.5) for
h<1μ | (2.6) |
yields
wn≤(1−hμ)nw0+(1−(1−hμ)n)Cμ | (2.7) |
and hence we have
wn≤w0+Cμ. | (2.8) |
It means that the size of the whole population is bounded from above. Moreover, if inequality (Eq 2.6) holds and w0≤Cμ, then wn≤Cμ for every n∈N+.
Now let us remind the property related to the boundedness of the particular variables of system (2.4).
Proposition 1. If
h<min(1k,1Cμ+μ+1), | (2.9) |
and
x0>0,y0>0,x0+y0≤Cμ, | (2.10) |
then we have xn>0, yn>0 and xn+yn≤Cμ for any n∈N+.
The forms of stationary states and conditions for their existence are the same in both systems (2.2) and (2.4). Let us present the conditions for stability of the stationary states in system (2.4).
Proposition 2. If inequality (Eq 2.9) holds, then Ed is a sink for R0<1, non-hyperbolic for R0=1 and a saddle for R0>1. Moreover, if inequality (Eq 2.10) holds, then Ed is globally stable for R0<1.
For the next proposition we define
ha:=4(k−1)C−μ+√δ,hb:=4(k−1)C−μ−√δ,hc:=C−μ(k−1)(C−μk) | (2.11) |
and
δ:=(C−μ)2−4(C−μk)(k−1)2=(C−μ)2−4(C−μk)(α+μ)2. | (2.12) |
Now we formulate the statement.
Proposition 3. Let us assume that Ee exists. If δ≥0, then Ee is a sink for h<ha, a saddle for ha<h<hb, a source for h>hb and non-hyperbolic for h∈{ha,hb}. If δ<0, then Ee is a spiral sink for h<hc, a spiral source h>hc and non-hyperbolic for h=hc.
Comparing the conditions for the local stability of Ee in system (2.2) to those in system (2.4), we state that the behavior of Ee in the discrete system with the explicit Euler method is more complex than in the continuous system. Furthermore, in the discrete case there arises additional dependence of the conditions for the stability on the step size of discretization.
Let us introduce the discrete version of system (2.2) with the use of the NSDM, following the approach presented in [3].
In our case applying the approach from [3] means replacing a bilinear term xnyn occurring in system (2.4) with a term xn+1yn or xnyn+1 in the first, second or both equations. In order to keep xn terms (meaning the scaled number of healthy people) positive, we introduce xn+1yn in the first equation. The positivity of yn terms is easily preserved without any modifications of xnyn in the second equation. Based on these assumptions, we modify system (2.4) and obtain the system having form
xn+1=xn+h(C−xn+1yn+yn−μxn), | (3.1a) |
yn+1=yn+h(xnyn−kyn)=yn(1+h(xn−k)). | (3.1b) |
Transforming Eq (3.1a), we write system (3.1) as
xn+1=xn(1−hμ)+hC+hyn1+hyn, | (3.2a) |
yn+1=yn(1+h(xn−k)). | (3.2b) |
Now we have the clear dependence of the variables' values for the (n+1)-th step on the variables' values for the previous one.
Let us first present some basic properties of system (3.2).
Basing again on the approach from [20], we compute R0 for system (3.2) and obtained (2.3).
System (3.2) is a discrete dynamical system described by the function
H(x,y)=(F(x,y)G(x,y))=(x(1−hμ)+hC+hy1+hyy(1+h(x−k))), | (3.3) |
that is the orbit of any arbitrary point (x0,y0), indicated by O, has the form O=((x0,y0),H(x0,y0), H2(x0,y0),…). Because of the meaning of the system variables, we should have Fn(x,y)≥0 and Gn(x,y)≥0 for nonnegative x and y. Note that F(x,y)>0 for inequality (Eq 2.6) held and G(x,y)>0 for
h<1k. | (3.4) |
Since k>μ, inequality (Eq 3.4) is stronger than inequality (Eq 2.6). From system (3.3) we conclude the following
Corollary 1. Let inequality (Eq 3.4) hold and x0≥0. Then
1. if y0>0, then xn,yn>0 for n∈N+,
2. if y0=0, then xn>0 and yn=0 for n∈N+.
One should note that thanks to inequality (Eq 3.4) and the non-negative initial sizes of both healthy and infected subpopulations, our model is biologically reasonable. The number of the healthy people is always positive and the number of the infected ones is always non-negative – it is equal to zero, however, in case of the lack of the infection in the population.
In the following we assume that inequality (Eq 3.4) holds and consider system (3.2) for x,y≥0.
Now we estimate values of the x variable. This variable increases if F(x,y)x>1 for x≠0, that is equivalet to
(1−hμ)+hCx+hyx1+hy>1, |
giving
x<˜x(y):=C+yy+μ=C+y+μ−μy+μ=1+C−μμ+y. |
In the considered domain the function ˜x(y) has its maximal value for y=0 equal to
˜x|y=0=1+C−μμ=Cμ. | (3.5) |
Hence, in the following we assume x≤Cμ. Substituting this inequality in F(x,y) yields
F(x,y)≤Cμ(1−hμ)+hC+hy1+hy=1+Cμ−11+hy. | (3.6) |
Since Cμ≫1, F(x,y) has the maximal value for y=0 equal to
1+Cμ−11=Cμ |
and we conclude:
Corollary 2. Let inequality (Eq 3.4) hold. If xq≤Cμ for a given q∈N, then xq+s≤Cμ for any s∈N.
In other words, if the number of healthy people in any iteration of system (3.2) is smaller than Cμ, then this value will be an upper bound for further iterations. Having given x0≤Cμ, we rephrase Corollary 2.
Corollary 3. If inequality (Eq 3.4) holds and x0≤Cμ, then xn≤Cμ for any n∈N+.
In the following we consider system (3.2) in the invariant subset
Ω:={(x,y)∈R2:0≤x≤Cμ,y≥0}. |
Now we focus on the y variable. For y≠0, this variable decreases if
G(x,y)y=1+h(x−k)<1 |
giving
x<k. | (3.7) |
Note that if Cμ<k, then inequality (Eq 3.7) is satisfied in the invariant subset Ω.
Combining this analysis with the notion of R0, we get
Corollary 4. If x0≤Cμ and R0<1, then for system (3.2) we have yn+1<yn for n∈N.
Now let us check if the size of the whole population is bounded from above. We rewrite system (3.2) to get difference quotients
xn+1−xnh=C−xn+1yn+yn−μxn,yn+1−ynh=yn(xn−k). | (3.8) |
Adding by sides the equations from system (3.8), we get
wn+1−wnh=C−xn+1yn+yn−μxn+ynxn−ynk | (3.9) |
giving
wn+1−wnh=C+yn(xn−xn+1)−μwn−αyn. | (3.10) |
We consider two cases:
∙ Let xn+1≥xn. From Eq (3.10) we have
wn+1−wnh≤C−μwn, | (3.11) |
that is equivalent to
wn+1≤h(C−μwn)+wn, | (3.12) |
what appears in system (2.5). Analogously like in system (2.4) solving inequality (Eq 3.12) with inequality (Eq 2.6) gives inequality (Eq 2.7), what implies inequality (Eq 2.8). Naturally, if we additionally assume w0≤Cμ, we get wn≤Cμ for every n∈N+.
∙ Now let xn+1<xn be true.
First we assume that R0<1 and x0≤Cμ. From Corollary 4 we have yn+1<yn, and with xn+1<xn it gives wn+1<wn. Hence the population size is bounded from above.
If R0>1 and xn<k, then yn+1<yn, giving again the boundedness of the population size from above.
The case R0>1 and xn>k is complicated and we will omit its analysis in this paper.
Graphs in Figure 1 present the boundedness of the whole population which dynamics is described by system (3.2). The initial condition (x0,y0)=(0.7;0.2) and the values of parameters μ=0.1, α=0.4 and h=0.1 were chosen. For Figure 1a we assumed that C=0.08 and for Figure 1b we chose C=0.25 so that R0<1 and R0>1 are fulfilled, accordingly. For each inequality 300 and 600 first iterations of system (3.2) were conducted, respectively.
Let us focus on stability analysis. System (3.2) has the same stationary states, and consequently the same conditions for their existence, as in systems (2.2) and (2.4). Jacobian matrix of system (3.2) has the following form
M(x,y)=(1−hμ1+hyh(1−x(1−hμ)−hC)(1+hy)2hy1+h(x−k)). |
Let us start from the stability of Ed.
Theorem 1. If inequality (Eq 3.4) holds, then the disease-free state Ed of system (3.2) is a sink for R0<1, non-hyperbolic for R0=1 and a saddle for R0>1. Moreover, if x0<Cμ and R0<1, then Ed is globally stable.
Proof. Inequality (Eq 3.4) guarantees the positivity of x and y. The Jacobian matrix for the Ed state has the form
M(Ed)=(1−hμh(1−Cμ)01+h(Cμ−k)). |
The conditions for Ed stability are |λi|<1, i=1, 2. Note that λ1=1−hμ satisfies it under the assumption inequality (Eq 3.4). On the other hand, λ2=1−hk+hCμ is positive for inequality (Eq 3.4) and λ2<1 holds if Cμ<k, that is R0<1.
Now we show the global stability of Ed for x0<Cμ and R0<1. From Corollary 3 we have xn≤Cμ. Moreover, Corollary 3 and Eq (3.2b) give
yn=y0n−1∏j=0(1+h(xj−k))≤y0(1+h(Cμ−k))n=y0(1+hk(Cμk−1))n=y0(1−hk(1−R0))n. | (3.13) |
From R0<1 and inequality (Eq 3.4) we get 0<1−hk(1−R0)<1 and
limn→∞yn=limn→∞y0(1−hk(1−R0))n=0. | (3.14) |
Now let us add by sides the equations from system (3.1). We get
wn+1=wn+h(C−xn+1yn+yn−μxn)+ynh(xn−k)=wn+h(C−xn+1yn+xnyn−αyn−μwn). | (3.15) |
This yields
wn+1≤wn+h(C+xnyn−αyn−μwn)≤wn+h(C+Cμyn−αyn−μwn). | (3.16) |
Let us take ε>0. Since Eq (3.14) holds, we state that
∀ε1>0∃N1∈N∀n>N1(Cμ−α)yn<ε1. | (3.17) |
Combining inequalities (Eq 3.16) and (Eq 3.17), we get for n>N1
wn+1<(1−hμ)wn+h(C+ε1) |
giving
wn<(1−hμ)n(w0−C+ε1μ)+C+ε1μ. |
Becuase
limn→∞(1−hμ)n(w0−C+ε1μ)=0, |
we can choose sufficiently large n (>N1) and we get
wn<Cμ+ε. | (3.18) |
Using Eq (3.15) again we obtain
wn+1≥wn+h(C−xn+1yn−αyn−μwn)≥wn+h(C−Cμyn−αyn−μwn). |
From Eq (3.14) for sufficiently large n we have
(Cμ+α)yn<ε1 |
and
wn+1>(1−hμ)wn+h(C−ε1) |
yielding
wn>(1−hμ)n(w0−C−ε1μ)+C−ε1μ. |
We state that for sufficiently large n
wn>Cμ−ε | (3.19) |
holds. Combining inequalities (Eq 3.18) and (Eq 3.19), for sufficiently large n we get
|wn−Cμ|<ε, |
implying
limn→∞wn=Cμ. |
Clearly, we have
limn→∞wn=limn→∞(xn+yn)=limn→∞xn+limn→∞yn. | (3.20) |
Both Eqs (3.14) and (3.20) yield
limn→∞xn=Cμ, | (3.21) |
proving global stability of Ed.
Note that conditions of stability of Ed do not depend on the step size of discretization h, while this is not the case of the endemic state Ee we discuss below.
Figure 2 includes a phase portrait illustrating the global stability of Ed for system (3.2). The values of parameters C=0.05, μ=0.1, α=0.4 and h=0.1 were chosen so that the condition R0<1 is fulfilled. Four initial conditions (x0,y0)=(0.8;0.2), (x0,y0)=(0.15;0.05), (x0,y0)=(0.2;0.25) and (x0,y0)=(0.3;0.5) were chosen. For each initial condition 50000 iterations were conducted.
Now we investigate the stability of the endemic stationary state Ee. Hence, in the following analysis we assume R0>1. Let us define parameters
h1:=C−μkα+μ−α+√(C−μkα+μ−α)2+4(C−μ)2(C−μk) | (3.22) |
and
h2:=δ4(C−μk)2(α+μ). | (3.23) |
Furthermore, we will refer to the δ value presented in Eq (2.12). See that because C≫μ, we have h1>0 for R0>1. Positivity of h2 is guaranteed for δ>0. In order to present the results transparently, we first consider the case δ>0. The condition δ<0 will be discussed later. The case δ=0 is not generic and will be not analyzed.
Let us formulate the theorem.
Theorem 2. Suppose that the state Ee of system (3.2) exists. Moreover, assume that δ>0. The state Ee is then a sink for h<h2, a spiral sink for h∈(h2,h1) and h2<h1, a spiral source for h>h1>h2, and non-hyperbolic for h=h1>h2, where h1 and h2 are defined in Eqs (3.22) and (3.23), respectively.
Proof. The Jacobian matrix of system (3.2) for Ee has the form
M(Ee)=(1−hμ1+hyeh(1−k(1−hμ)−hC)(1+hye)2hye1). |
To simplify the notation, we define
υ:=1−hμ1+hye, | (3.24) |
ϖ:=hC+k(1−hμ)−1=h(C−μk)+α+μ. | (3.25) |
From inequality (Eq 2.6) and R0>1 we have υ>0 and ϖ>0. Using Eqs (3.24) and (3.25), we rewrite M(Ee) as
M(Ee)=(υ−hϖ(1+hye)2hye1). | (3.26) |
The characteristic polynomial of M(Ee) reads
P(λ)=λ2−(1+υ)λ+υ+h2yeϖ(1+hye)2, | (3.27) |
while eigenvalues are equal to
λ1,2=12⋅(1+υ±√ˆδ), |
where
ˆδ=(1+υ)2−4(υ+h2yeϖ(1+hye)2)=(1−v)2−4h2yeϖ(1+hye)2. |
Let us check for which h a condition ˆδ>0 holds. We get the sequence of equivalent inequalities
(1−υ)2>4h2yeϖ(1+hye)2, |
(1−1−hμ1+hye)2>4h2yeϖ(1+hye)2, |
(ye+μ)2h2>4h2yeϖ, |
(C−μα+μ)2>4C−μkα+μ(h(C−μk)α+μ), |
(C−μ)24(C−μk)(α+μ)>h(C−μk)+α+μ, |
h<(C−μ)24(C−μk)2(α+μ)−α+μC−μk, |
leading to
h<δ4(C−μk)2(α+μ). | (3.28) |
From δ>0 we have the positivity of the right-hand side of inequality (Eq 3.28). Combining inequality (Eq 3.28) and Eq (3.23), we get h<h2.
Analogously we have ˆδ<0 for
h>h2. | (3.29) |
The further analysis considers different signs of ˆδ. We omit the case ˆδ=0.
∙ Let us consider ˆδ>0, for which we get the real eigenvalues such that λ1>λ2. Note that Polynomial Eq (3.27) has two real zeros and the coefficient from its linear term is negative. Hence, λ1,λ2>0 and for the local stability of Ee it is enough to fulfill λ1<1. Looking for this inequality we obtain
12⋅(1+υ+√ˆδ)<1 |
giving
√ˆδ<1−υ. | (3.30) |
The right-hand side of inequality (Eq 3.30) can be written as
1−υ=1−1−hμ1+hye=hye+hμ1+hye. |
The above expression is always positive, so we can square both sides of inequality (Eq 3.30) obtaining
ˆδ<(1−υ)2, |
which is obvious from the definition of ˆδ.
From λ1<1 we do not get additional conditions for the local stability. Hence, we state that existing Ee is locally stable if δ>0 and h<h2 hold.
∙ Now we consider the case ˆδ<0, making the eigenvalues complex. We have
λ1λ2=|λ|2=14(1+υ)2−14ˆδ=υ+h2yeϖ(1+hye)2. | (3.31) |
The condition |λ|<1 can be written as
1−hμ1+hye+h2yeh(C−μk)+α+μ(1+hye)2<1, |
giving
ye(C−μk)h2−ye(ye−α)h−(ye+μ)<0. | (3.32) |
We denote the left-hand side of inequality (Eq 3.32) as ˉP(h), which is a quadratic trinomial with a negative constant term. From the existence of Ee we have ye(C−μk)>0. Hence, ˉP(h) has one positive zero equal to
ye(ye−α)+√(ye(ye−α))2+4ye(C−μk)(ye+μ)2ye(C−μk)=C−μkα+μ−α+√(C−μkα+μ−α)2+4(C−μ)2(C−μk). | (3.33) |
From Eq (3.22) we state that this zero is equal to h1. We conclude that h<h1 should hold in order to fulfil ˉP(h)<0. Combining h<h1 and inequality (Eq 3.29), we get h∈(h2,h1), being true for h2<h1.
Let us focus again on Theorem 2. Note that after exceeding h2 the state Ee changes from a sink to a spiral sink, while the local stability is preserved. Moreover, h1 is a critical value in the context of stability – after exceeding h1 the state Ee stops being stable.
Now let us assume δ<0. Then we have h2<0, corresponding to ˆδ<0. Using that and Theorem 2, we conclude:
Corollary 5. Suppose that the stationary state Ee of system (3.2) exists. Let us also assume that δ<0. The state Ee is then a spiral sink for h<h1, a spiral source for h>h1 and non-hyperbolic for h=h1.
Now we assume that inequality (Eq 3.4) is true, guaranteeing the positivity of solutions of system (3.2). We check conditions for which the threshold values of h defined in the previous subsection satisfy this inequality. Let us first check the condition giving
h2<1k. | (3.34) |
After some calculations this inequality is equivalent to
W(C):=a2C2+a1C+a0<0, | (3.35) |
where
a2:=1−3(α+μ),a1:=2k(2(μ+α)(μ−α)−μ),a0:=kμ(μ+4kα(α+μ)). | (3.36) |
Note that a0>0. Let δC be a discriminant of W(C). After some tedious calculations we obtain
δC=16k(α+μ)3(α+μ2+αμ+α2). | (3.37) |
Because δC>0, we have two real zeros of W(C) equal to
Ca,b=2k(μ−2(μ+α)(μ−α))∓√δC2(1−3(α+μ)). | (3.38) |
The inequalities a2>0 and a1<0 can be written as
α+μ<13 | (3.39) |
and
2(μ+α)(μ−α)<μ. | (3.40) |
Let us take m∈(0,13). Assuming inequality (Eq 3.39), from inequality (Eq 3.40) we have
2m(μ−α)<μ⇒2mμ−2mα<μ⇒0<(1−2m)μ+2mα. |
We have 1−2m>0, so the last inequality from above is always true.
Hence, a2>0 implies a1<0. We also see that a2>0 and a1>0 cannot hold simultaneously.
The fulfillment of inequality (Eq 3.35) depends on the sign of a2 solely. Because inequalities (Eq 3.34) and (Eq 3.35) are equivalent, we state that:
1) for a2>0 inequality (Eq 3.34) holds for C>0 and C∈(Ca,Cb),
2) for a2<0 inequality (Eq 3.34) holds for C>Cb>0.
Now let us focus on the inequality
h1<1k. | (3.41) |
Using the ye notation, we rewrite h1 as
h1=ye−α+√(ye−α)2+4(C−μ)2(C−μk)=ye−α+√(ye−α)2+4(C−μ)2ye(k−1). | (3.42) |
Substituting Eq (3.42) into inequality (Eq 3.41), we get
(ye−α)+√(ye−α)2+4(C−μ)2(C−μk)<1k, |
that is
√(ye−α)2+4(C−μ)<2(C−μk)k−(ye−α). | (3.43) |
Inequality (Eq 3.43) is justified for
2(C−μk)k−ye+α=2ye(k−1)k−ye+α=ye(1−2k)+α=(C−μk)(k−2)k(k−1)+α>0. | (3.44) |
If the condition contrary to Eq (3.44) holds, then we have
h1>1k. | (3.45) |
Let us assume that Eq (3.44) is true. We can then square both sides of inequality (Eq 3.43) obtaining
(ye−α)2+4(C−μ)<4(C−μk)2k2−4(C−μk)(ye−α)k+(ye−α)2, |
what gives
k2(C−μ)<−(C−μk)2α+μ+kα(C−μk), |
k(1+α+μ)(C−μ)<−(C−μk)2α+μ+kα(C−μ(1+μ+α)), |
k(1+μ)(C−μ)+(C−μk)2α+μ+kαμ(μ+α)<0. | (3.46) |
Note that from C≫μ we have k(1+μ)(C−μ)≫0. Hence we state that inequality (Eq 3.46) never holds. It means that inequality (Eq 3.41) is contradictory and we shall assume inequality (Eq 3.45).
Now let us consider Theorem 2 and Corollary 5 in the context of positivity of solutions of system (3.2). Let us emphasize again that the condition h>h1 does not hold. We consider the sign of a2 defined in Eq (3.36). From Theorem 2 we obtain the following corollary:
Corollary 6. Assume that the stationary state Ee of system (3.2) exists and define Ca and Cb as in Eq (3.38). If δ>0 and one set of the conditions:
α+μ<13,0<C∈(Ca,Cb) |
or
α+μ>13,C>Cb, |
holds, then Ee is a sink for h<h2 and a spiral sink for h∈(h2,1k). Moreover, the solutions of system (3.2) are positive for x0≥0 and y0>0.
Let us look at Corollary 5. Remind that δ<0 is equivalent to h2<0. We see that then inequality (Eq 3.34) is always true and we should only consider inequality (Eq 3.45). We reject the case when h>h1. Hence we have:
Corollary 7. Let the state Ee of system (3.2) exist and inequality (Eq 3.4) be true. Then Ee is a spiral sink and the solutions of system (3.2) are positive for x0≥0 and y0>0.
Let us consider the possibility of the Neimark-Sacker bifurcation appearance for the Ee state in system (3.2). The necessary condition giving this possibility is |λ1|=|λ2|=1. Using the calculations presented above, we determine the necessary conditions for NSB:
Corollary 8. Let us assume that either δ>0 and ˆδ<0 or δ<0. Neimark-Sacker bifurcation for the state Ee in system (3.2) can happen if h=h1.
Determining the conditions for NSB in systems based on the NSDM is in general difficult. Because of that, we only numerically illustrate the possibility of the NSB appearance for system (3.2).
We conduct numerical simulations which results are presented in Figures 3 and 4. In each figure first 50,000 iterations of system (3.2) were presented. For simulations the values of parameters: C=0.19, μ=0.1 and α=0.3 were taken. The values were chosen so that the condition δ<0 is fulfilled. For Figure 3 (x0,y0)=(1.52;0.2) and h=4.5 were taken. In case of Figure 4 the step size h=4.65 was assumed and for each Figures 4a and 4b the initial condition (x0,y0)=(1.52;0.2) and (x0,y0)=(1.49;0.3) was respectively chosen. Note that for h=4.5 the state Ee is a sink and for h=4.65 we get the invariant curve attracting the solutions from both inside and outside the curve, what is shown in Figures 4a, b. Analysing the behavior illustrated in Figures 3 and 4, we guess that in system (3.2) there is a possibility of NSB for the endemic stationary state for h=h1.
In this paper we analyzed the non-standard discretized system describing epidemic spread in the population consisting of two groups: susceptible (healthy) and infected people. We presented basic properties of the assumed system of difference equations, focusing on positivity and boundedness of the variables. In both discrete model (2.4) with the EEM and system (3.2) with the NSDM we obtained boundedness of the variables with a proper initial condition (besides the unchecked case R0>1 and xn>k for the NSDM). We also proved the global stability of the disease-free stationary state Ed and the local stability of the endemic state Ee.
The results for Ed for sufficiently small step of discretization are analogous to those for this state in case of the continuous model. Note that these results are nearly the same as for system (2.4).
The state Ee for the continuous case is globally stable if it exists (see [17]). In case of the NSDM we proved the local stability of Ee for h<h1. These results are in accordance with the fact that the behavior of a discrete system for a sufficiently small step of discretization is analogous to the behavior of its continuous counterpart. Even the analysis of local stability of the endemic state occurred complex for system (3.2), so we expect that global stability analysis would be much more complex, which is in general the case for systems based on the NSDM. This is mainly related to the dependence of stability of Ee on the step of discretization and the possibility of bifurcations with respect to this parameter. Note that that behavior of systems (3.2) and (2.4) is analogous near accordingly h1 and hc. We conclude that in both discrete models for the endemic state Ee there is a critical value of the step size of discretization and after exceeding this value the state Ee loses the local stability. After necessary computations, we got that h1>hc. It means that the loss of Ee stability happens in system (3.2) for a bigger value of h than in system (2.4). Hence, we state that using the NSDM in a discrete system gives better approximation of the analogical continuous counterpart comparing to using the EEM.
Comparing the results for the continuous model to those for both discrete ones, we conclude that the conditions for the stability of the stationary states requires the bounded value of the step size of discretization from above for the discrete models. In case of the NSDM the conditions are weaker than in case of the EEM.
We checked the possibility of the Neimark-Sacker bifurcation appearance in the analyzed system. We presented one specific example for which an isolated invariant closed curve seems to appear. Looking at Figures 3 and 4, we state that the critical value for the NSB belongs to the interval (4.5;4.65). Note that after exceeding the critical value, the stationary state loses its stability and there arises a stable closed invariant curve surrounding this state. We conclude that in system (3.2) there can be a supercritcal NSB. We conducted numerical simulations for possibility of NSB appearance in the discrete model with the EEM. The simulations suggest the appearance of a subcritical NSB for the same stationary state Ee, differently to the model with the non-standard discretization. In order to prove that for two types of discretization we have different types of NSB, mathematical analysis should be conducted.
We would like to thank the constructive feedback provided by the reviewers.
The authors declare that there are no conflicts of interest regarding the publication of this article.
[1] | J. Liu, B. Peng, T. Zhang, Effect of discretization on dynamical behavior of SEIR and SIR models with nonlinear incidence, Appl. Math. Lett., 39 (2015), 60–66. doi: 10.1016/j.aml.2014.08.012. |
[2] | S. Side, A. M. Utami, Sukarna, M. I. Pratama, Numerical solution of SIR model for transmission of tuberculosis by Runge–Kutta method, J. Phys. Conf. Ser., 1040 (2018). doi: 10.1088/1742-6596/1040/1/012021. |
[3] | R. E. Mickens, Nonstandard Finite Difference Models of Differential Equations, World Scientific, Atlanta, 1993. doi: 10.1142/2081. |
[4] | H. Al-Kahby, F. Dannan, S. Elaydi, Non-standard discretization methods for some biological models, in Applications of Nonstandard Finite Difference Schemes, (2000), 155–180. doi: 10.1142/9789812813251_0004. |
[5] | Y. A. Kuznetsov, Elements of Applied Bifurcation Theory, 2nd edition, Springer-Verlag, New York, 1998. doi: 10.1007/b98848. |
[6] | Z. Enatsu, Z. Teng, C. Jia, C. Zhang, L. Zhang, Dynamical analysis and chaos control of a discrete SIS epidemic model, Adv. Differ. Equations, 58 (2014), 1–20. doi: 10.1186/1687-1847-2014-58. |
[7] | D. A. Kessler, Epidemic size in the SIS model of endemic infections, J. Appl. Probab., 45 (2008), 757–778. doi: 10.1239/jap/1222441828. |
[8] | M. Martcheva, An Introduction to Mathematical Epidemiology, Springer, New York, 2015. doi: 10.1007/978-1-4899-7612-3. |
[9] | R. N. Shalan, R. Shireen, A. H. Lafta, Discrete an SIS model with immigrants and treatment, J. Interdiscip. Math., 24 (2021), 1201–1206. doi: 10.1080/09720502.2020.1814496. |
[10] | W. L. I. Roeger, Dynamically consisent discrete-time SI and SIS epidemic models, Discrete Contin. Dyn. Syst., 2013 (2013), {653–662}. doi: 10.3934/proc.2013.2013.653. |
[11] | M. T. Hoang, O. F. Egbelowo, Nonstandard finite difference schemes for solving an SIS epidemic model with standard incidence, Rend. Circolo Mat. Palermo Ser. 2, 69 (2020), 753–769. doi: 10.1007/s12215-019-00436-x. |
[12] | Y. Enatsu, Y. Nakata, Y. Muroya, Global stability for a discrete SIS epidemic model with immigration of infectives, J. Differ. Equations Appl., 18 (2012), 1913–1924. doi: 10.1080/10236198.2011.602973. |
[13] | Y. Xie, Z. Wang, J. Lu, Y. Li, Stability analysis and control strategies for a new SIS epidemic model in heterogeneous networks, Appl. Math. Comput., 383 (2020). doi: 10.1016/j.amc.2020.125381. |
[14] | Y. Xie, Z. Wang, Transmission dynamics, global stability and control strategies of a modified SIS epidemic model on complex networks with an infective medium, Math. Comput. Simul., 188 (2021), 23–34. doi: 10.1016/j.matcom.2021.03.029. |
[15] | X. Wang, Z. Wang, H. Shen, Dynamical analysis of a discrete-time SIS epidemic model on complex networks, Appl. Math. Lett., 94 (2019), 292–299. doi: 10.1016/j.aml.2019.03.011. |
[16] | D. B. Saakian, A simple statistical physics model for the epidemic with incubation period, Chin. J. Phys., 73 (2021), 546–551. doi: 10.1016/j.cjph.2021.07.007. |
[17] | M. Bodzioch, M. Choiński, U. Foryś, SIS criss-cross model of tuberculosis in heterogeneous population, Discrete Contin. Dyn. Syst. Ser. B, 24 (2019), 2169–2188. doi: 10.3934/dcdsb.2019089. |
[18] | M. Choiński, M. Bodzioch, U. Foryś, Simple criss-cross model of epidemic for heterogeneous populations, Commun. Nonlinear Sci. Numer. Simul., 79 (2019), 1–17. doi: 10.1016/j.cnsns.2019.104920. |
[19] | M. Choiński, M. Bodzioch, U. Foryś, Simple discrete SIS criss-cross model of tuberculosis in heterogeneous population of homeless and non-homeless people, Math. Appl., 47 (2019), 103–115. doi: 10.14708/ma.v47i1.6496. |
[20] | L. J. S. Allen, P. van den Driessche, The basic reproduction number in some discrete time epidemic models, J. Differ. Equations Appl., 14 (2008), 1127–1147. doi: 10.1080/10236190802332308. |
1. | R. A. Yakhina, Modification of Epidemiological Model for Predicting the Development of a Socially Significant Infection (by the Example of Chronic Viral Hepatitis C), 2022, 19, 2500-3925, 87, 10.21686/2500-3925-2022-4-87-96 | |
2. | Marcin Choiński, A discrete SIS-model built on the strictly positive scheme, 2024, 35, 0938-1279, 17, 10.1007/s00200-023-00607-5 |