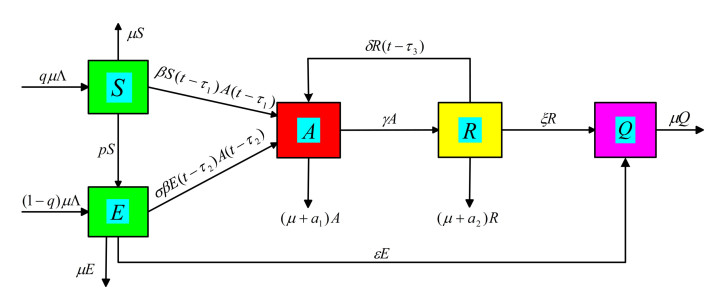
Citation: Jinhua Zeng, Xiulian Qiu, Shaopei Shi. Image processing effects on the deep face recognition system[J]. Mathematical Biosciences and Engineering, 2021, 18(2): 1187-1200. doi: 10.3934/mbe.2021064
[1] | Edil D. Molina, Paul Bosch, José M. Sigarreta, Eva Tourís . On the variable inverse sum deg index. Mathematical Biosciences and Engineering, 2023, 20(5): 8800-8813. doi: 10.3934/mbe.2023387 |
[2] | Wanlin Zhu, Minglei Fang, Xianya Geng . Enumeration of the Gutman and Schultz indices in the random polygonal chains. Mathematical Biosciences and Engineering, 2022, 19(11): 10826-10845. doi: 10.3934/mbe.2022506 |
[3] | Xinmei Liu, Xinfeng Liang, Xianya Geng . Expected Value of Multiplicative Degree-Kirchhoff Index in Random Polygonal Chains. Mathematical Biosciences and Engineering, 2023, 20(1): 707-719. doi: 10.3934/mbe.2023032 |
[4] | Saylé C. Sigarreta, Saylí M. Sigarreta, Hugo Cruz-Suárez . On degree–based topological indices of random polyomino chains. Mathematical Biosciences and Engineering, 2022, 19(9): 8760-8773. doi: 10.3934/mbe.2022406 |
[5] | Qi Wang, Lifang Huang, Kunwen Wen, Jianshe Yu . The mean and noise of stochastic gene transcription with cell division. Mathematical Biosciences and Engineering, 2018, 15(5): 1255-1270. doi: 10.3934/mbe.2018058 |
[6] | V. R. Kulli, J. A. Méndez-Bermúdez, José M. Rodríguez, José M. Sigarreta . Revan Sombor indices: Analytical and statistical study. Mathematical Biosciences and Engineering, 2023, 20(2): 1801-1819. doi: 10.3934/mbe.2023082 |
[7] | Ricai Luo, Khadija Dawood, Muhammad Kamran Jamil, Muhammad Azeem . Some new results on the face index of certain polycyclic chemical networks. Mathematical Biosciences and Engineering, 2023, 20(5): 8031-8048. doi: 10.3934/mbe.2023348 |
[8] | Xiujun Zhang, H. G. Govardhana Reddy, Arcot Usha, M. C. Shanmukha, Mohammad Reza Farahani, Mehdi Alaeiyan . A study on anti-malaria drugs using degree-based topological indices through QSPR analysis. Mathematical Biosciences and Engineering, 2023, 20(2): 3594-3609. doi: 10.3934/mbe.2023167 |
[9] | Mert Sinan Oz, Roberto Cruz, Juan Rada . Computation method of the Hosoya index of primitive coronoid systems. Mathematical Biosciences and Engineering, 2022, 19(10): 9842-9852. doi: 10.3934/mbe.2022458 |
[10] | Peng Gu, Dongrong Yang, Jin Zhu, Minhao Zhang, Xiaoliang He . Bioinformatics analysis identified hub genes in prostate cancer tumorigenesis and metastasis. Mathematical Biosciences and Engineering, 2021, 18(4): 3180-3196. doi: 10.3934/mbe.2021158 |
Alcoholism can be viewed as a social epidemic [1], which can bring great harm to individuals, families and society. The study of alcoholism has become an important aspect of social epidemic. Alcohol abuse can also lead to a range of negative social effects such as violence, antisocial and criminal behavior. Alcohol consumption has been identified as a major contributor to the global burden of chronic disease, injury and economic cost [2,3,4]. The World Health Organization reports the harmful use of alcohol causing approximately 3.3 million deaths every year (or 5.9% of all the global deaths), and 5.1% of the global burden of disease is attributable to alcohol consumption [5]. Therefore, alcoholism is the main targeted healthy risky behavior due to the high relevance of negative health and social effects. The spread of healthy risky behavior within a community can be viewed as a diffusion process with its own incidence rate. In this situation, the social interaction is considered to be the key factor in spreading the alcohol behavior which can result in adverse health effects. The prevention and control of alcoholism is an urgent problem.
Mathematical modelling is a powerful tool for solving problems in various fields [6,7,9,8,10]. In the last few decades, mathematical models for human behaviors related to addictions have been developed from epidemiological models for the spread of infectious diseases, including drinking, smoking and drug use, etc., see [11,12,13,14] and the references contained therein. In particular, there are several different mathematical models for alcohol problems that have been formulated and studied recently [15,16,17,18,19,20,21,22,23,24,25,26,27]. Benedict [15] modelled alcoholism as a contagious disease and studied how "infected" drinking buddies spread problem drinking. Manthey et al. [16] studied campus drinking and suggested that the reproductive numbers are not sufficient to predict whether drinking behavior will persist on campus and that the pattern of recruiting new members play a significant role in the reduction of campus alcohol problems. Santonja et al. [17] proposed a mathematical model for alcohol consumption in Spanish population. Predictions about the future behavior of the alcohol consumption in Spain are presented using this model. Environmental and peer influence combinations to create a culture of drinking were studied in [18,19,20,21]. In addition, the two-stage models: one stage where people who admit having the alcohol problem and other stage where people who do not admit to having the alcohol problem have been developed in [22,23]. Bhunu [24] studied the co-interaction of alcoholism and smoking in a community. Walters et al. [25] also discussed alcohol problems, and their results showed that an increase in the recovery rate decreased the proportion of binge drinkers in the population. Huo et al. [26] considered the effect of constant immigration on drinking behavior. Wang et al. [27] presented an alcoholism model with two control strategies to gain insights into this increasingly concern about health and social phenomenon. The optimal control strategies are derived by proposing an objective functional and using Pontryagins Maximum Principle.
It is well known that the prevalence of any epidemic is strongly dependent on the social behavior of individuals. Human behavior and social response play a very important role in the transmission of social epidemic. So, the rational way is to make people aware about the alcohol problems through the media, which can not only influence the individuals' behavior but also increase the governmental health care involvement to control the spread of heavy drinking. In recent years, many mathematical models have been used for studying the impact of public health education by media on epidemic outbreaks [28,29,30,31,32,33,34,35,36,37,38,39] and the references cited therein. These studies suggested that education and media have a huge impact in controlling the spread of infectious diseases. Recently, for drinking problem, Huo et al. [40] have studied drinking dynamics and focused on awareness programs and treatment in the modelling process. They have extended the model in [39] via including a treatment class and established some sufficient conditions for the stability of the alcohol-free and alcohol-present equilibria. Xiang et al. [41] also studied a drinking model with public health educational campaigns. Using Lyapunov function, the global stability of equilibria of the model is derived. Their results showed that the public health educational campaigns is one of the commonly used effective measures in order to prevent and reduce the alcohol problems. On the other hand, recently, Ma et al. [42,43] proposed that the multi-group alcoholism model can contribute to the control of alcohol problems in more realistic situations.
At the same time, time delays have been considered into the infections diseases models by many authors [44,45,46,47,48]. Delay differential equations exhibit much more complicated dynamics than ordinary differential equations since the time delay may affect the stability of the system, even lead to instability, oscillation or bifurcation phenomena. Because time delay is common in development of alcohol consumption habit in population, we should note that there are delays in the "infection" process which the alcohol consumption habit develops in a individual who is "infected" by alcoholics. So, it is more realistic to consider the time delay in the modelling alcoholism process. To our knowledge, the results about alcoholism epidemic model with delays are comparatively scarce. Recently, Huo et al. [49] introduced a more realistic binge drinking model with delay. They concluded that, regardless of the time delay length, the alcohol-free equilibrium is globally asymptotically stable. Numerical simulations showed that the alcohol-present equilibrium is globally asymptotically stable. Ma et al. [50] formulated a dynamic alcohol consumption model with awareness programs and one delay. The results showed that the time delay in alcohol consumption habit which develops in susceptible population may result in a Hopf bifurcation by increasing the value of time delay.
Motivated by the above works and based on the previous work in [41], we formulate an alcoholism model that incorporates both public health education and three delays to study the dynamics and control of drinking. We incorporate the delays τ1, τ2 to describe the time needed for a susceptible individual to become an alcohol user, and the delay τ3 to describe temporarily recovered population that will take a period of time to become a alcohol consumer again. Here, time delay for the "infection" and "recovery" are included together. The goal of this paper is to analyze the effects of public health education and three delays on alcohol control. So, the research work of this paper improves the existing results.
The remaining part of this paper is organized as follows. In next section, we formulate the delayed mathematical model. The positivity and ultimate boundedness of the solutions for system are shown in Section 3. In Section 4, for four different delay cases, the global dynamics of the delayed alcoholism model is discussed in detail. In Section 5, the impacts of public health education and delays on alcohol control will be discussed. The sensitivity analysis of system parameters is given in Section 6. Then, in order to support our theoretical predictions, some numerical simulations are included in Section 7. Finally, a brief conclusion is also given in the last section.
In 2015, Xiang et al. [41] investigated a SEARQ drinking model with the public health educational campaigns, as the following drinking dynamics:
{dS(t)dt=qμΛ−βS(t)A(t)−(μ+p)S(t),dE(t)dt=(1−q)μΛ+pS(t)−σβE(t)A(t)−(μ+ε)E(t),dA(t)dt=βS(t)A(t)+σβE(t)A(t)+δR(t)−(μ+a1+γ)A(t),dR(t)dt=γA(t)−(μ+a2+ξ+δ)R(t),dQ(t)dt=ξR(t)+εE(t)−μQ(t), | (2.1) |
where the total population in the model is divided into five types: at time t, S(t) denotes the numbers of susceptible individuals who consume alcohol in moderation and do not accept the public health education, but may develop problems with alcohol; E(t) is referred to educated susceptible individuals who consume alcohol in moderation and accept the public health education, in which the average rate of turning to drink is reduced relatively to the tuen rate of uneducated individuals; A(t) is alcoholics class who have drinking problems or addictions; R(t) is temporarily recovered drinkers, that is, former alcoholics who have entered treatment and are abstaining from alcohol; Q(t) represent quit drinkers who permanently quit drink. All parameters are assumed to be positive constants. It is also assumed that the drinking only spreads due to the direct contact between susceptible individuals or educated drinkers and alcoholics. The global stability of equilibria are obtained by constructing suitable Lyapunov functions (see Theorem 4.1 in [41]).
As is known to us, there are delays in the "infection" or "recovery" process of drinking. So we propose a mathematical model with health education and three delays to study the dynamics and control of alcoholism. In this paper, we incorporate three delays into the alcoholism model (2.1). Assume that homogenous mixing and each individual has the same chance to get infected. The variables and model structure are described in Figure 1.
Our model can be described by the following ordinary differential equations:
{dS(t)dt=qμΛ−βS(t)A(t)−(μ+p)S(t),dE(t)dt=(1−q)μΛ+pS(t)−σβE(t)A(t)−(μ+ε)E(t),dA(t)dt=βS(t−τ1)A(t−τ1)+σβE(t−τ2)A(t−τ2)+δR(t−τ3)−(μ+a1+γ)A(t),dR(t)dt=γA(t)−(μ+a2+ξ+δ)R(t),dQ(t)dt=ξR(t)+εE(t)−μQ(t). | (2.2) |
Here the meanings of parameters are the same as system (2.1). Time delay τ1≥0 to describe the time needed for a uneducated susceptible individual to become an heavy alcohol consumer, τ2≥0 to describe the time needed for a educated susceptible individual to become an heavy alcohol consumer, and time delay τ3≥0 to describe the time needed for a temporarily recovered drinkers re-enter the alcoholics groups. There is a time delay during which the alcohol consumption habit develops in his/her body. It is only after such time delay that he/she becomes a alcohol consumer.
Let τ=max{τ1,τ2,τ3}. We denote by C=C([−τ,0],R) the Banach space of continuous real-valued functions on the interval [−τ,0], with the sup-norm
||ϕ||=sup−τ≤θ≤0{|ϕ(θ)|},ϕ∈C. |
The nonnegative cone of C is defined as
C+=C([−τ,0],R+). |
We suppose that the initial conditions for system (2.2) take the forms:
S(θ)=ϕ1(θ),E(θ)=ϕ2(θ),A(θ)=ϕ3(θ),R(θ)=ϕ4(θ),Q(θ)=ϕ5(θ),ϕ=(ϕ1,ϕ2,ϕ3,ϕ4,ϕ5)∈C+×C+×C+×C+×C+,ϕi(0)>0,i=1,2,3,4,5. | (2.3) |
Remark 2.1. Assume τi≡0(i=1,2,3), the system (2.2) becomes the system (2.1) in [41]. Therefore, system (2.2) expands system (2.1).
Since the variable Q does not appear explicitly in the first four equations in (2.2), then the dynamics of system (2.2) is the same as the following reduced system:
dS(t)dt=qμΛ−βS(t)A(t)−(μ+p)S(t), | (3.1a) |
dE(t)dt=(1−q)μΛ+pS(t)−σβE(t)A(t)−(μ+ε)E(t), | (3.1b) |
dA(t)dt=βS(t−τ1)A(t−τ1)+σβE(t−τ2)A(t−τ2)+δR(t−τ3)−mA(t), | (3.1c) |
dR(t)dt=γA(t)−nR(t). | (3.1d) |
Thus, in the rest of the article, we are concerned with the above system (3.1). For system (3.1), it is important to prove that all the state variables remain nonnegative (for biological reasons). We give the following lemma.
Lemma 3.1. Consider system (3.1) with the initial conditions (2.3), we have
(i) All solutions of system (3.1) are strictly positive;
(ii) All solutions of system (3.1) are ultimately bounded if δ<μ.
Proof. (i) We prove the system (3.1) with initial conditions (2.3) has strictly positive solutions for all t≥0.
Assuming the contrary and letting t1>0 be the first time such that S(t1)=0. From Eq (3.1a), we have S′(t1)=qμΛ>0, hence for sufficiently small η>0, S(t)<0 for t∈(t1−η,t1). But this contradicts S(t)>0 for t∈[0,t1). It prove that S(t)>0 for all t≥0. Suppose that t2>0 be the first time such that E(t2)=0. From Eq (3.1b), we have E′(t2)=(1−q)μΛ+pS(t2)>0. We use the same method to prove E(t)>0 for all t≥0.
From Eq (3.1c), we can obtain
A′(t)≥[βS(t−τ1)+σβE(t−τ2)−m]A(t). |
By using the method of variation of constant and the step-to-step integration, we get
A(t)≥ϕ3(0)exp(∫t0[βS(ξ−τ1)+σβE(ξ−τ2)−m]dξ), |
which implies that A(t)>0 for all t>0.
Then from Eq (3.1d), we obtain
R′(t)=γA(t)−nR(t), |
hence
R(t)=ϕ4(0)e−nt+γ∫t0A(s)en(s−t)ds, |
which shows R(t)>0 for all t>0 because of R(0)=ϕ4(0)>0.
(ii) We show that the solutions of system (3.1) are ultimately bounded for all t≥0.
According to Eq (3.1a), we have
lim supt→∞S(t)≤qμΛμ+p. | (3.2) |
So, S(t) is ultimately bounded.
According to Eq (3.1b), we have
lim supt→∞E(t)≤μΛ[p+μ(1−q)](μ+ε)(μ+p), |
and E(t) is ultimately bounded, too. Then, we define
X(t)=S(t+τ2+τ3)+E(t+τ1+τ3)+A(t+τ1+τ2+τ3)+R(t+τ1+τ2+τ3), |
we have X(t)≥0, and yield
X′(t)≤μΛ+pS(t+τ1+τ3)−μX(t)+δR(t+τ1+τ2). | (3.3) |
From Eq (3.2), we choose T>0 so large such that t≥T−τ1−τ3>0, then
S(t+τ1+τ3)≤qμΛμ+p, |
and from the definition of X(t), we obtains
R(t+τ1+τ2)=X(t−τ3)−S(t+τ2)−E(t+τ1)−A(t+τ1+τ2). |
Then, we get from Eq (3.3) that
X′(t)≤M−μX(t)+δX(t−τ3), | (3.4) |
where M=μΛ(1+qμ+p). Following the method of Kuang [45], we assume that δ<μ, by considering the auxiliary system
z′(t)=M−μz(t)+δz(t−τ3), |
there is a unique positive steady state z∗=Mμ−δ is globally asymptotically stable. It follows from Eq (3.4) that
lim supt→∞X(t)≤Mμ−δ. |
Therefore, S(t),E(t),A(t) and R(t) are ultimately bounded. This proof is completed.
As a consequence of Lemma 3.1, we know that the dynamics of system (3.1) can be analyzed in the following bounded feasible region, namely, for sufficiently small ε>0 such that
Ω={(S,E,A,R)∈C+×C+×C+×C+:||S||≤qΛ+ε,||S+E||≤Λ+ε,||S+E+A+R||≤Mμ−δ+ε}. |
Hence, the region Ω is positive invariant set for system (3.1) and the system is well posed.
Remark 3.1. From the above discussion, we can see the parameter δ (i.e. the fraction of compartment R who relapse into drinking compartment A) could not be too large (δ<μ). The public health educational campaigns is an effective measure in reducing the value of return proportion δ.
Following the compartment approach, the alcoholism generation number R0 of the alcohol consumption model (2.2) can be easily obtained by the next generation matrix method [51], which is given by the following expression:
R0=β(S0+σE0)(μ+a2+ξ+δ)(μ+a1+γ)(μ+a2+ξ+δ)−γδ=:β(S0+σE0)nmn−γδ, |
where S0=qμΛμ+p,E0=μΛ[p+μ(1−q)](μ+ε)(μ+p), m=μ+a1+γ, n=μ+a2+ξ+δ, and mn>γδ. It acts as a threshold as is shown in the following results.
For convenience and simplicity, we still set P0 is the alcohol-free equilibrium and P∗ is the unique alcohol-present equilibrium for system (3.1). In addition, from the system (3.1), we have
S∗=qμΛμ+p+βA∗,E∗=[pq+(1−q)(μ+p+βA∗)]μΛ(μ+p+βA∗)(μ+ε+βσA∗),R∗=γA∗μ+a2+ξ+δ, |
where A∗ is the unique positive root of equation H(A)=0 (see Eq (13) in [41]). Then, we have the following results.
Lemma 4.1. Consider system (3.1) with the initial conditions (2.3), we have
(i) System (3.1) always has the alcohol-free equilibrium P0(S0,E0,0,0);
(ii) System (3.1) also has a unique alcohol-present equilibrium P∗(S∗,E∗,A∗,R∗) if R0>1.
In the following subsection, we investigate the stability of the equilibria of system (3.1). Considering the impacts of health education on the susceptible individuals and treatment on the temporarily recovered individuals, the time delays of three compartments are different, we will be divided into several cases to discuss.
1) Stability analysis of P0
We linearize the system (3.1) about P0, and the characteristic equation of system (3.1) at P0 is given by
(λ+p+μ)(λ+μ+ε){[λ+m−β(S0+σE0)e−λ˜τ](λ+n)−γδe−λτ3}=0. | (4.1) |
Obviously, we have
λ1=−(μ+p)<0,λ2=−(μ+ε)<0. |
So, our focus is to discuss the solution of the following equation
F(˜τ,τ3,λ)=[λ+m−β(S0+σE0)e−λ˜τ](λ+n)−γδe−λτ3=0. | (4.2) |
First, we set c1=β(S0+σE0)>0. If R0<1 and ˜τ=0,τ3>0, the Eq (4.2) becomes
F(0,τ3,λ)=[λ+m−β(S0+σE0)](λ+n)−γδe−λτ3=λ2+d1λ+d0−γδe−λτ3=0, | (4.3) |
where d1=m+n−c1>0, d0=n(m−c1)>0.
Notice that 0 is not a root of Eq (4.3) because of R0<1. Following the method in [54], we let λ=iω1(ω1>0) be a purely imaginary root of Eq (4.3). Substituting it into Eq (4.3) and separating the real and imaginary parts, then squaring and adding both equations, we can obtain
ω41+(d21−2d0)ω21+d20−(γδ)2=0. | (4.4) |
Let u1=ω21, then Eq (4.4) becomes
G(u1)=u21+(d21−2d0)u1+d20−(γδ)2=0, |
where d21−2d0=(m−c1)2+n2>0 and d20−(γδ)2>0 because of d0−γδ>0, so G(u1)=0 has no positive root. Hence, we easily obtain all roots of Eq (4.1) have negative real parts. The alcohol-free equilibrium P0 is locally asymptotically stable for any τ3>0 with τ1=τ2=˜τ=0. In the following, we prove P0 is globally attractive in Ω for any τ3>0 with τ1=τ2=˜τ=0.
We define the following Lyapunov function L1:C×C×C×C→R:
L1(St,Et,At,Rt)=At(0)+Rt(0)+δ∫0−τ3Rt(s)ds, |
where the notation xt(s)=x(t+s) for s∈[−τ,0), and xt(0)=x(t). The time derivative of L1 along the solution of system (3.1) (˜τ=0,τ3>0) is
dL1dt∣(3.1)=βS(t)A(t)+σβE(t)A(t)+δR(t−τ3)+(γ−m)A(t)+(δ−n)R(t)−δR(t−τ3)≤{β[S(t)+σE(t)]−(μ+a1)}A(t). | (4.5) |
Using Lemma 3.1, we know that S(t) and E(t) are bounded, that is, we obtain that S(t)≤S0 and E(t)≤E0. It is easy to see that dL1dt∣(3.1)≤0 if β(S0+σE0)<μ+a1. This condition is equivalent to the transmission rate β meets the following condition:
(H1): β<μ+a1S0+σE0.
Further, dL1dt=0 if and only if A(t)=0. It can be verified that the maximal invariant set in {dL1dt∣(3.1)=0} is the singleton {P0}. By the LaSalle's invariance principle, we can conclude that P0 is globally attractive in Ω.
Second, for R0>1, it is not difficult to verify that Eq (4.3) has a root with a positive real part. In fact, F(˜τ,τ3,0)<0 if R0>1, and limλ→∞F(˜τ,τ3,λ)=∞. Therefore, the following theorem is obtained.
Theorem 4.1. In Case I, we have
(i) If R0<1 and (H1) holds, then the alcohol-free equilibrium P0 is globally asymptotically stable for any τ3>0, ˜τ=0;
(ii) If R0>1, then the alcohol-free equilibrium P0 is unstable for any τ3>0,˜τ=0.
2) Stability analysis of P∗
We let s(t)=S(t)−S∗,e(t)=E(t)−E∗,a(t)=A(t)−A∗,r(t)=R(t)−R∗, the linearization of system (3.1) at P∗ is given by
{ds(t)dt=−(βA∗+μ+p)s(t)−βS∗a(t),de(t)dt=ps(t)−(μ+ε+σβA∗)e(t)−σβE∗a(t),da(t)dt=βA∗s(t−τ1)+σβA∗e(t−˜τ)+(βS∗+σβE∗)a(t−˜τ)+δr(t−τ3)−ma(t),dr(t)dt=γa(t)−nr(t). | (4.6) |
Further, the Jacobi matrix J at P∗ can be written as
J=J|P∗=(−(μ+p+βA∗)0−βS∗0p−(μ+ε+σβA∗)−σβE∗0βA∗e−λ˜τσβA∗e−λ˜τ(βS∗+σβE∗)e−λ˜τ−mδe−λτ300γ−n). |
The characteristic equation of system (3.1) at P∗ is det(λI−J)=0, where I is the identity matrix and λ is the eigenvalue of matrix J. By computation, the characteristic equation of system (3.1) at P∗ can be expressed by
λ4+n1λ3+n2λ2+n3λ+n4−(r1λ3+r2λ2+r3λ+r4)e−λ˜τ−(s2λ2+s3λ+s4)e−λτ3=0, | (4.7) |
where
n1=2μ+p+ε+m+n(σ+1)βA∗,
n2=σβ2(A∗)2+[(μ+n)(1+σ)+σp+ε+mσ+1]βA∗+σβ(μ+p)(μ+ε)+n(2μ+p+ε)+m(2μ+p+n+ε),
n3=(m+n)[σβ2(A∗)2+(μ+ε)βA∗+σ(p+μ)βA∗+(μ+p)(μ+ε)]+mn(σ+1)βA∗+n(2μ+p+ε),
n4=mn[σβ2(A∗)2+βA∗(μ+ε+σp+σμ)+(μ+p)(μ+ε)],
r1=βS∗+σβE∗,
r2=β2S∗A∗(σ+2)+σβ2E∗A∗(2σ+1)+β(2μ+p+n+ε)(S∗+σE∗),
r3=(βS∗+σβE∗)[σβ2(A∗)2+(σ(μ+p)+nσ+1)βA∗]+(μ+p)(μ+ε)+n(2μ+p+ε)+σ2β2E∗A∗(βA∗+μ+p+n)+β2S∗A∗(σβA∗+μ+ε+n),
r4=(βS∗+σβE∗)n[σβ2(A∗)2+βA∗(μ+ε+σp+σμ)+(μ+p)(μ+ε)]+nβ2S∗A∗(σβA∗+μ+ε)+npσβ2(A∗)2+nσ2β2E∗A∗(βA∗+μ+p),
s2=γδ,
s3=γδ[βA∗(1+σ)+(2μ+p+ε)],
s4=γδ[σβ2(A∗)2+βA∗(μ+ε+σμ+σp)+(μ+p)(μ+ε)].
Here ˜τ=0, τ3>0, so the characteristic equation Eq (4.7) can be simplified to
λ4+w1λ3+w2λ2+w3λ+w4−(s2λ2+s3λ+s4)e−λτ3=0, | (4.8) |
where w1=n1−r1,w2=n2−r2,w3=n3−r3,w4=n4−r4.
(H2): Suppose that the following conditions holds:
(i) w1>0,w1(w2−s2)>w3−s3,w4−s4>0;
(ii) w1[(w3−s3)(w2−s2)−w1(w4−s4)]>(w3−s3)2.
By the Routh-Hurwitz criteria, we will known that all roots of Eq (4.8) with τ3=0 have negative real parts. We now claim the locally stability of the alcohol-present equilibrium P∗ in Case I.
Theorem 4.2. In case I, the alcohol-present equilibrium P∗ is locally asymptotically stable for any τ3>0 if R0>1 and (H2) holds.
1) Stability analysis of P0
If R0<1 and ˜τ>0,τ3=0, from the discussion of part (1) in Case I, the characteristic equation F(˜τ,τ3,λ) of system (3.1) at P0 becomes
F(˜τ,0,λ)=[λ+m−β(S0+σE0)e−λ˜τ](λ+n)−γδ=λ2+b1λ+b0−(c1λ+c0)e−λ˜τ=0, | (4.9) |
where b1=m+n>0, b0=mn−γδ>0, c1=β(S0+σE0)>0, c0=β(S0+σE0)n=nc1>0.
Notice that 0 is not a root of Eq (4.9) because of R0<1. Let λ=iω2(ω2>0) be a purely imaginary root of Eq (4.9), we have
−ω22+ib1ω2+b0−(ic1ω2+c0)[cos(ω2˜τ)−isin(ω2˜τ)]=0. |
Separating the real and imaginary parts, we can get the following equations
{−ω22+b0=c0cos(ω2˜τ)+c1ω2sin(ω2˜τ),b1ω2=c1ω2cos(ω2˜τ)−c0sin(ω2˜τ). | (4.10) |
Squaring and adding both equations of Eq (4.10), it follows that
ω42+(b21−2b0−c21)ω22+(b20−c20)=0. | (4.11) |
Let u2=ω22, then Eq (4.11) becomes
G(u2)=u22+(b21−2b0−c21)u2+(b20−c20)=0. | (4.12) |
From m>c1, we easily get b20−c20>0 and b21−2b0−c21=n2+2γδ+m2−c21>0. So Eq (4.12) has no positive root. Thus, all roots of Eq (4.9) have negative real parts. The alcohol-free equilibrium P0 is locally asymptotically stable for any ˜τ>0 with τ3=0.
In the following, we prove P0 is globally attractive in Ω for any ˜τ>0, τ3=0. Define Lyapunov function L2:C×C×C×C→R:
L2(St,Et,At,Rt)=At(0)+Rt(0)+β∫0−˜τSt(s)At(s)ds+σβ∫0−˜τEt(s)At(s)ds, |
where the notation xt(s) and xt(0) are the same meaning as in L1. The time derivative of L2 along the solution of system (3.1) with τ1=τ2=˜τ>0,τ3=0 is
dL2dt∣(3.1)=βS(t−˜τ)A(t−˜τ)+σβE(t−˜τ)A(t−˜τ)+δR(t)+(γ−m)A(t)−nR(t)+βS(t)A(t)−βS(t−˜τ)A(t−˜τ)+σβE(t)A(t)−σβE(t−˜τ)A(t−˜τ)≤{β[S(t)+σE(t)]−(μ+a1)}A(t). | (4.13) |
If (H1) holds, it ensures that dL2dt∣(3.1)≤0, and dL2dt=0 if and only if A(t)=0. It can be verified that the maximal invariant set in {dL2dt∣(3.1)=0} is the singleton {P0}. By the LaSalle's invariance principle, we can conclude that P0 is globally attractive in Ω. Therefore, we have the following theorem.
Theorem 4.3. In case II, we have
(i) If R0<1 and (H1) holds, then the alcohol-free equilibrium P0 is globally asymptotically stable for any ˜τ>0, τ3=0;
(ii) If R0>1, then the alcohol-free equilibrium P0 is unstable for any ˜τ>0, τ3=0.
2) Stability analysis of P∗
Here ˜τ>0, τ3=0, similar to the discussion of part (2) in Case I, the characteristic equation Eq (4.7) can be simplified to
λ4+h1λ3+h2λ2+h3λ+h4−(r1λ3+r2λ2+r3λ+r4)e−λ˜τ=0, | (4.14) |
where h1=n1,h2=n2−s2,h3=n3−s3,h4=n4−s4.
(H3): The coefficients of Eq (4.14) satisfy the Routh-Hurwitz criteria when ˜τ=0.
Theorem 4.4. In case II, if R0>1 and (H3) holds, then the alcohol-present equilibrium P∗ is locally asymptotically stable for any ˜τ>0.
1) Stability analysis of P0
For τ1=τ2=τ3=τ>0, the characteristic equation of system (3.1) at P0 is given by
λ2+[m+n−β(S0+σE0)e−λτ]λ+mn−[γδ+β(S0+σE0)n]e−λτ=0. | (4.15) |
First, let λ=iω(ω>0) be a purely imaginary root of Eq (4.15). We have
−ω2+[m+n−β(S0+σE0)e−i(ωτ)]iω+mn−[γδ+β(S0+σE0)n]e−i(ωτ)=0. |
Then, we get
−ω2+(m+n)ωi−β(S0+σE0)[cos(ωτ)−isin(ωτ)]iω+mn−[γδ+β(S0+σE0)n][cos(ωτ)−isin(ωτ)]=0. |
Separating the real and imaginary parts, we can get the following equations
{−ω2+mn=β(S0+σE0)sin(ωτ)ω+[γδ+β(S0+σE0)n]cos(ωτ),(m+n)ω=β(S0+σE0)cos(ωτ)ω−[γδ+β(S0+σE0)n]sin(ωτ). | (4.16) |
Squaring and adding both equations of Eq (4.16), it follows that
ω4+[m2+n2−β2(S0+σE0)2]ω2+(mn)2−[γδ+β(S0+σE0)n]2=0. | (4.17) |
Let u=ω2, then Eq (4.17) becomes
G(u)=u2+[m2+n2−β2(S0+σE0)2]u+(mn)2−[γδ+β(S0+σE0)n]2=0. | (4.18) |
From R0<1, we easily obtain m2+n2−β2(S0+σE0)2>0 and (mn)2−[γδ+β(S0+σE0)n]2>0. So, Eq (4.18) has no positive root. Thus, all roots of Eq (4.15) have negative real parts, and the alcohol-free equilibrium P0 is locally asymptotically stable for any τ>0.
Second, we prove P0 is globally attractive in Ω for any τ>0. To prove this, we consider the following Lyapunov function L3:C×C×C×C→R:
L3(St,Et,At,Rt)=At(0)+Rt(0)+β∫0−τSt(s)At(s)ds+σβ∫0−τEt(s)At(s)ds+δ∫0−τRt(s)ds, |
where the notation xt(s) and xt(0) are the same meaning as in L1. The time derivative of L3 along the solution of system (3.1) with τi=τ>0(i=1,2,3) is
dL3dt∣(3.1)=−mA(t)+γA(t)−nR(t)+βS(t)A(t)+σβE(t)A(t)+δR(t)≤{β[S(t)+σE(t)]−(μ+a1)}A(t). | (4.19) |
If (H1) holds, then it ensures that dL3dt∣(3.1)≤0, and dL3dt=0 if and only if A(t)=0. It can be verified that the maximal invariant set in {dL3dt∣(3.1)=0} is the singleton {P0}. By the LaSalle's invariance principle, we can conclude that P0 is globally attractive in Ω. We claim the following result.
Theorem 4.5. For Case III, we get
(i) If R0<1 and (H1) holds, then the alcohol-free equilibrium P0 is globally asymptotically stable for any τi=τ>0(i=1,2,3);
(ii) If R0>1, then the alcohol-free equilibrium P0 is unstable for any τi=τ>0(i=1,2,3).
2) Stability analysis of P∗
For R0>1, system (3.1) has a unique positive equilibrium P∗(S∗,E∗,A∗,R∗). In the following, we will consider the stability of the alcohol-present equilibrium P∗ and concern about the effect of time delays on the stability of the alcohol-present equilibrium.
Let s(t)=S(t)−S∗,e(t)=E(t)−E∗,a(t)=A(t)−A∗,r(t)=R(t)−R∗, the linearization of system (3.1) at P∗ is given by
{ds(t)dt=−(βA∗+μ+p)s(t)−βS∗a(t),de(t)dt=ps(t)−(μ+ε+σβA∗)e(t)−σβE∗a(t),da(t)dt=βA∗s(t−τ)+σβA∗e(t−τ)+(βS∗+σβE∗)a(t−τ)+δr(t−τ)−ma(t),dr(t)dt=γa(t)−nr(t). | (4.20) |
Further, the Jacobi matrix J at P∗ can be written as
J=J|P∗=(−(μ+p+βA∗)0−βS∗0p−(μ+ε+σβA∗)−σβE∗0βA∗e−λτσβA∗e−λτ(βS∗+σβE∗)e−λτ−mδe−λτ00γ−n). |
By computation, the characteristic equation of system (3.1) at P∗ is given by
λ4+l1λ3+l2λ2+l3λ+l4−(u1λ3+u2λ2+u3λ+u4)e−λτ=0, | (4.21) |
where
l1=2μ+p+ε+m+n(σ+1)βA∗,
l2=σβ2(A∗)2+[(μ+n)(1+σ)+σp+ε+mσ+1]βA∗+σβ(μ+p)(μ+ε)+n(2μ+p+ε)+m(2μ+p+n+ε),
l3=(m+n)[σβ2(A∗)2+(μ+ε)βA∗+σ(p+μ)βA∗+(μ+p)(μ+ε)]+mn(σ+1)βA∗+n(2μ+p+ε),
l4=mn[σβ2(A∗)2+βA∗(μ+ε+σp+σμ)+(μ+p)(μ+ε)],
u1=βS∗+σβE∗,
u2=β2S∗A∗(σ+2)+σβ2E∗A∗(2σ+1)+β(2μ+p+n+ε)(S∗+σE∗)+γδ,
u3=(βS∗+σβE∗)[σβ2(A∗)2+(σ(μ+p)+nσ+1)βA∗]+(μ+p)(μ+ε)+n(2μ+p+ε)+σ2β2E∗A∗(βA∗+μ+p+n)+β2S∗A∗(σβA∗+μ+ε+n)+γδ[βA∗(1+σ)+(2μ+p+ε)],
u4=(βS∗+σβE∗)n[σβ2(A∗)2+βA∗(μ+ε+σp+σμ)+(μ+p)(μ+ε)]+nβ2S∗A∗(σβA∗+μ+ε)+npσβ2(A∗)2+nσ2β2E∗A∗(βA∗+μ+p)+γδ[σβ2(A∗)2+βA∗(μ+ε+σμ+σp)+(μ+p)(μ+ε)].
For τ>0, we assume that Eq (4.21) have root λ(τ)=ξ(τ)+iζ(τ), where ξ(τ),ζ(τ)∈R. Set τ∗ be such that ξ(τ∗)=0, ζ(τ∗)=ζ∗. Substituting λ(τ∗) into Eq (4.21) and separating the real and imaginary parts, we can get the following equations
{ζ4∗−l2ζ2∗+l4=(u4−u2ζ2∗)cos(ζ∗τ∗)+(u3ζ∗−u1ζ3∗)sin(ζ∗τ∗),−l1ζ3∗+l3ζ∗=(u3ζ∗−u1ζ3∗)cos(ζ∗τ∗)+(u2ζ2∗−u4)sin(ζ∗τ∗). | (4.22) |
Taking squares and adding the above equations of Eq (4.22), we get
ζ8∗+ρ1ζ6∗+ρ2ζ4∗+ρ3ζ2∗+ρ4=0, | (4.23) |
where ρ1=l21−2l2−u21,ρ2=l22−u22−2l1l3+2l4+2u1u3,ρ3=l23−u23−2l2l4+2u2u4,ρ4=l24−u24.
(H4): Equation (4.23) has at least one simple positive root.
Theorem 4.6. For Case III, if (H4) does not hold, then the alcohol-present equilibrium P∗ is locally asymptotically stable for any τi=τ>0(i=1,2,3).
Remark 4.1. If (H4) holds and set ζ∗ is the last such root. We will assert that there exists a τ∗>0 such that ξ(τ∗)=0,ζ(τ∗)=ζ∗. System (3.1) may exhibit a Hopf bifurcation at P∗ as τ passes upwards through τ∗=ζ−1(ζ∗)>0 with dξdτ∣τ=τ∗≠0.
Considering the delay of temporarily recovered people is likely to be different from the delay of two classes of susceptible populations, we assume that τ1=τ2=˜τ≠τ3 and show the stability in the following case.
In this case, we only study the stability analysis of P0. From the discussion of part (1) in Case I, the characteristic equation Eq (4.1) can be rewritten as
F(˜τ,τ3,λ)=[λ+m−β(S0+σE0)e−λ˜τ](λ+n)−γδe−λτ3=λ2+f1λ+f0−(g1λ+g0)e−λ˜τ−γδe−λτ3=0, | (4.24) |
where f1=m+n>0,f0=mn>0 and g1=β(S0+σE0)>0,g0=ng1>0. Let λ=iω3(ω3>0) be a purely imaginary root of Eq (4.24). It satisfies
−ω23+if1ω3+f0−ig1ω3cos(ω3˜τ)−g0cos(ω3˜τ)−g1ω3sin(ω3˜τ)+ig0sin(ω3˜τ)−h0cos(ω3τ3)+ih0sin(ω3τ3)=0. | (4.25) |
Separating the real and imaginary parts, we obtain
{Asin(ω3˜τ)+Bcos(ω3˜τ)=C,Acos(ω3˜τ)−Bsin(ω3˜τ)=D, | (4.26) |
where A=g1ω3,B=g0 and C=f0−ω23−h0cos(ω3τ3),D=f1ω3+h0sin(ω3τ3). Then, we obtain
C2+D2−A2−B2=0. |
Set
G(ω3)=C2+D2−A2−B2=ω43+m1ω33+m2ω23+m3ω3+m4=0. | (4.27) |
where m1=0,m2=f21−g21−2f0+2h0cos(ω3τ3),m3=2f1h0sin(ω3τ3),m4=f20−2f0h0cos(ω3τ3)+h20−g20.
We have
G′(ω3)=4ω33+2m2ω3+m3. |
Set
ω33+m22ω3+m34=ω33+p1ω3+q1=0, |
where p1=m22,q1=m34. Notice that there is no square term in the above equation. In order to analyze the roots of Eq (4.27), we cite the results in [55] about the existence of positive roots of the fourth degree polynomial equation. Define
Δ=(q12)2+(p13)3,η=−1+√3i2, |
ω(1)3=3√−q12+√Δ+3√−q12−√Δ, |
ω(2)3=3√−q12+√Δη+3√−q12−√Δη2, |
ω(3)3=3√−q12+√Δη2+3√−q12−√Δη. |
Then, we need the following lemma.
Lemma 4.2. (See [55]) Suppose that m4≥0.
(i) If Δ≥0, then Eq (4.27) has positive roots if and only if ω(1)3>0 and G(ω(1)3)<0;
(ii) If Δ<0, then Eq (4.27) has positive root if and only if there exists at least one ω∗∈{ω(1)3,ω(2)3,ω(3)3}, such that ω∗>0 and G(ω∗)≤0.
Notice that R0=nc1mn−γδ<1 (mn>γδ), we can get
G(0)=m4=f20−2f0h0cos(ωτ3)+h20−g20≥f20−2f0h0+h20−g20 |
=(f0−h0+g0)(f0−h0−g0)=(mn−γδ+nc1)(mn−γδ−nc1)>0. |
Supposing that condition (i) or (ii) of Lemma 4.2 is satisfied, then Eq (4.27) has finite positive roots ω31,...,ω3k,k≤4, and for every fixed ω3i,i=1,...,k,k≤4, there exists a sequence {τj3i∣i=1,...,k,k≤4,j=0,1,...} such that Eq (4.25) holds. Let
τ∗3(˜τ)=min{τ03i∣i=1,...,k}, |
and ω∗=ω3i, here i corresponding to the minimum value. Then Eq (4.24) has a pair of purely imaginary roots ±iω∗ when τ=τ∗3(˜τ), ˜τ∈[0,+∞).
Theorem 4.7. In Case IV, set ˜τ∈[0,+∞). If the condition (i) or (ii) of Lemma 4.2 is satisfied, then the alcohol-free equilibrium P0 is asymptotically stable when τ3∈[0,τ∗3(˜τ)].
Similarly, we can have the following results.
Theorem 4.8. In Case IV, set τ3∈[0,+∞). If exists
{˜τji∣i=1,...,k,k≤4,j=0,1,...} |
such that Eq (4.25) holds, then the alcohol-free equilibrium P0 is asymptotically stable when ˜τ∈[0,˜τ∗(τ3)].
Remark 4.2. We will leave the following studies as open problems:
1) The above research shows that delays do not destroy the globally asymptotical stability of alcohol-free equilibrium P0. However, delays can destroy the stability of alcohol-prsent equilibrium P∗. We assert that there may arise a Hopf bifurcation at P∗ by increasing the value of one or more delays.
2) The study of other situations is also very valuable. For example: τi>0,i=1,2,3 and τ1≠τ2≠τ3. However, it is well known that the analysis of the characteristic equation is a formidable and challenging task.
In the process of establishing the model, the public health education and three delay factors are introduced. In this section, the impact of these factors on the number of alcoholics will be discussed in detail.
First of all, we choose a set of values of parameters (parameter values are mainly taken from [5,41]):
μ=0.25,β=0.8,a1=0.01,a2=0.01,q=0.6,Λ=30.496,ε=0.2,δ=0.1,γ=0.7,
ξ=0.04,p=0.1,τ1=τ2=τ3=0.5.
Due to the greater the positive impact of media reporting measures on the population, the smaller the value of σ is. Figure 2 shows the number of alcoholics A(t) decreased with the decrease of the value of the media coverage impact factor σ. So, it is clear to see that the public health education (media reporting measure) is beneficial to control the spread of alcohol problems.
Next, the simulation results for different time delays are shown in Figure 3. Let σ=0.2, τi=0.01,1,2,3(i=1,2,3). We can see that the change trend of alcoholics A(t) for different values of τ1 in Figure 3(a). That is, as the value of τ1 increases, the value of A(t) increases rapidly in a short time. But the smaller the value of τ1 is, the smaller the corresponding peak value of A(t) is. Moreover, the larger the value of τ1, the longer it takes for the value of A(t) to reach the highest peak. This indicates that the higher the value of τ1, the more unfavorable it is to control the problem of drinking. From Figure 3(b), as the value of τ2 increases, the value of A(t) decreases rapidly in a short time. This shows that the higher the value of τ2, the more favorable the control of alcohol problem. From Figure 3(c), in the long term, as the value of τ3 increases, the value of A(t) decreases. This shows that the higher the value of τ3, the more favorable the control of alcohol problem.
In this section, we will use the Latin hypercube sampling method in [56,57,58] and Partial rank correlation coefficients method to study the sensitivity analysis of each parameter on the basic reproduction number R0 and the number of alcoholics A(t). By discussing the sensitivity analysis of each parameter, we can control the key parameters and take corresponding measures to control alcohol problems.
First, we will explore the effect of various parameters on the basic reproduction number R0, see Figure 4. Parameter values are mainly taken from [5,41] and given by Table 1.
Parameter | Value | Source |
μ: Natural death rate | 0.25 | [42] |
β: Rate of susceptible individuals turn to drink | 0.1 | [5] |
a1,a2: Death rates due to excessive drinking | 0.01 | [42] |
q: Proportion of these individuals is assumed to be uneducated | 0.6 | [42] |
Λ: New recruits enter the population | 0.8 | [5] |
σ: Overall effectiveness of the public health educational campaigns | 0.2 | [42] |
ε: Rate of transfer from educated individuals to quit drinkers | 0.2 | [42] |
δ: Rate of temporarily recovered drinkers enter into alcoholics compartment | 0.1 | [42] |
γ: Rate of transfer from alcoholics to temporarily recovered drinkers | 0.5 | [5] |
ξ: Rate of transfer from temporarily recovered drinkers to quit drinkers | 0.4 | [42] |
p: Rate of uneducated individuals enter into educated individuals | 0.1 | [Assumed] |
It is assumed that the parameters of the system obey normal distribution, and 2000 samples are taken within the 95% confidence interval (taking the upper and lower as 0.02). In what follows, we perform the numerical study. Namely, the PRCC-values of R0 with respect to each parameter of the system are given. According to the order of parameters μ,β,a1,a2,q,Λ,σ,ε,δ,γ,ξ,p, the corresponding PRCC-values are:
−0.4996,0.9968,−0.1318,−0.0254,0.8398,0.8220, |
0.6064,−0.3579,0.7078,−0.8267,−0.1571,−0.9330. |
Similarly, the P-values of R0 with respect to each parameter of the system are as follows. According to the order of parameters μ,β,a1,a2,q,Λ,σ,ε,δ,γ,ξ,p, the corresponding P-values are:
4.6463×10−126,0,3.6031×10−9,0.2584,0,0,4.4486×10−200, |
3.6482×10−61,2.9255×10−302,0,1.8615×10−12,0. |
Parameters with the highest (or lowest) PRCC values have the largest positive (or negative) impact on R0. On the contrary, R0 is more sensitive to the parameter with smaller P-value. Figure 4(a), (b) depict the parameters that have the most positive influence on R0 are β,q,Λ,σ and δ. While the parameters with the most negative impact on R0 are μ,a1,a2,ε,γ,ξ and p. It can be seen that alcohol transfer rate β has the greatest positive correlation with R0, and population transfer rate caused by media reports p has the greatest negative correlation with R0. Moreover, the sensitivity of a2 is the weakest among all parameters. Practical significance: by strengthening the news real-time report and cumulative report on alcohol related hazards, we can reduce the value of σ and δ, and increase the values of ε, ξ and p to make the value of R0 decreases to R0<1. so as to control the number of alcoholics in the population. Second, we study the sensitivity analysis of parameters to A(t). Focusing on the impact of media reporting measures on alcoholism. Therefore, the following mainly considers several parameters that are directly affected by media reports as shown in Figure 5. Figure 5(a) shows the influence of parameters μ and a1 on A(t) is always negatively correlated. And, the influence of parameter a2 on A(t) is positively correlated at first, and as time goes by, the influence of a2 on A(t) becomes negatively correlated because the relapsed person drinks again. However, the influence of parameter ε on A(t) is negatively. Because with the passage of time, the influence of media reports on people will weaken or even disappear, which also shows that it is necessary to carry out regular reports on the dangers of alcoholism to strengthen people's health awareness. In particular, Figure 5(b) shows the PRCC-values of parameter σ or p will be reduced to zero in the later stage, with slight positive and negative fluctuations. Research shows that the influence of media reports has saturation effect. This is also consistent with the reality. Regular media coverage of the dangers of alcoholism can control alcoholism in the community.
Remark 6.1. Among the abundant literature on sensitivity measures, the Sobols indices have received much attention since they provide accurate information for most models. Polynomial chaos-based Sobols indices are used in [60,61,62]. Recently, using deterministic, frequentist and Bayesian approaches, Calatayud et al. [63] studied the effect of social behavior on the increase of the obesity epidemic. And several sensitivity analyses are also conducted. These approaches are interesting alternatives for sensitivity analyses. We will leave these interesting work for the future.
In this section, some numerical results of system (3.1) are provided to substantiate the analytic results obtained in this paper.
In the following, we fix parameters:
μ=0.25,β=0.1,a1=0.01,a2=0.01,p=0.1,q=0.6,Λ=3.496,
σ=0.2,ε=0.2,δ=0.1,γ=0.5,ξ=0.4,
Choose τ1=τ2=0,τ3=0.5, we have R0=0.2478<1 and meet the previous conditions. Thus the alcohol-free equilibrium
P0=(1.4983,1.1098,0,0,0.8879) |
is globally asymptotically stable. The solutions of P0 are shown in Figure 6(a).
Other parameters remain unchanged and let β=0.8,γ=0.7, we have R0=1.7531>1, then the alcohol-free equilibrium P0 is unstable and the alcohol-present equilibrium
P∗=(0.8122,0.8462,0.3697,0.6467,0.9357) |
is locally asymptotically stable. The solutions of P∗ are shown in Figure 6(b).
Choose μ=0.7,τ1=τ2=0.7,τ3=0, we have R0=0.1792<1 and the alcohol-free equilibrium
P0=(1.8354,1.2916,0,0,0.3690) |
is globally asymptotically stable. The solutions of P0 are shown in Figure 7(a).
Choose μ=0.3,β=0.8, we get R0=1.9437>1 and the alcohol-present equilibrium
P∗=(1.0589,0.2687,0.4905,0.4039,0.6721) |
is locally asymptotically stable. The solutions of P∗ are shown in Figure 7(b).
Choose μ=0.25,β=0.05,τ1=τ2=τ3=0.5, we have R0=0.1239<1 and the alcohol-free equilibrium
P0=(1.4983,1.1098,0,0,0.8879) |
is globally asymptotically stable. The solutions of P0 are shown in Figure 8(a).
Choose μ=0.25,β=0.7, we get R0=1.7363>1 and the alcohol-present equilibrium
P∗=(1.0001,0.8793,0.3205,0.1527,0.7644) |
is locally asymptotically stable. The solutions of P∗ are shown in Figure 8(b).
Choose τ1=τ2=0.1,τ3=0.9, we have R0=0.0423<1 and the alcohol-free equilibrium
P0=(1.4983,1.1098,0,0,0.8879) |
is asymptotically stable. The solutions are shown in Figure 9(a).
Choose another set of values τ1=τ2=0.2,τ3=0.8, we get R0=0.1025<1 and the alcohol-free equilibrium
P0=(0.6992,1.5538,0,0,1.2430) |
is also asymptotically stable. The solutions are shown in Figure 9(b).
Through the above theoretical analysis and numerical simulation, it can be seen that different time delays have little effect on the global asymptotic behavior of the alcohol-free equilibrium point P0, but have a greater impact on the dynamic behavior of the alcoholic equilibrium point P∗.
There is a large literature (see [15,16,18,19,20,21,22,23,24,25,26,27] and [28,29,30,31,32,33,34,35,36,37,38,39,40,41,42,43]) that has been used for studying alcohol problems and describing the effects of awareness programs by media on the infectious disease or alcohol consumption behavior. From [41], we know that the global stability of system (2.1) without delays has been proved by using the Lyapunov function. That is, if R0<1, all solutions converge to the alcohol-free equilibrium P0, and the alcohol problems disappear eventually; if R0>1, the alcohol-present equilibrium P∗ is globally stable, i.e., the alcohol problems will persist in the population and the number of problem drinkers tends to a positive constant. However, most of these models are ODE models and do not incorporate the effects of the time delay. Recently, Huo et al. [49] and Ma et al. [50] incorporated one delay into alcoholism model and studied it's stability via the delay differential equations. Their results showed that the stability of system be destroyed by the delay and the system occurs a Hopf bifurcation at the alcohol-present equilibrium under certain conditions.
Compared to previous models, this paper considered an alcoholism model with health education and three delays. The main goal is to analyze the global dynamics of the model and discuss the impacts of health education and three delays on the alcohol consumption behavior. A key novelty of our model is that we do not only consider the impact of health education but also introduce three delays which describe the time needed that a susceptible or temporarily individual becomes an infectious alcohol user. It is necessary and practical significance to consider the dynamic behavior about delayed alcoholism model. A question is how the three delays affect the dynamics of the system (3.1). This is the main purpose of this paper.
Specifically, we discussed a FDE system (3.1) in four different cases respectively. If R0<1, our results showed that the alcohol-free P0 is still globally asymptotically stable after incorporating time delays, which dose not change the dynamic of P0. However, if R0>1, our results shows that incorporation of three delays will change the stability of the alcohol-present equilibrium P∗. This means that difference of the time delay between two classes of susceptible populations and temporarily recovered people can affect the spread and control of alcohol problems greatly. The higher the value of τ2 or τ3, the more conducive to the prevention and control of alcoholism. On the contrary, the higher the value of τ1, the more unfavorable the prevention and control of alcoholism. Under the point of view of the alcohol control, our results show that time delays have important influence in controlling alcohol problem. For condition (H1), that is, the transmission rate β plays an essential role in our study. Public health education can reduce the transmission rate β. Our model improves and generalizes the existing results.
In this article, we only considered several special cases. For the alcohol-present equilibrium P∗, we assert that there may arise a Hopf bifurcation at P∗ by increasing the value of delay. The study of other situations is more conform to reality the actual phenomenon. However, it is well known that the analysis of the characteristic equation is a formidable and challenging task. We will leave these studies as open problems.
For simplicity, the random variability is normally distributed in this paper. In fact, the value of the model key parameters can be obtained by using statistic data will make our paper more perfect. From Brauer [59], we know that a example (The Great Plague in Eyam): the village of Eyam near Sheffield, England suffered an outbreak of bubonic plague in 1665–1666. The actual data for the Eyam epidemic are remarkably close to the predictions of the model. Our model coefficients should be fixed from medical reports and the delays in model may have an estimation. At present, we lack statistical data on alcohol abuse. So that the parameters are given some values to test the theoretical results in Sections 5 and 7.
In Section 6, we use the Latin hypercube sampling method and Partial rank correlation coefficients method to study the sensitivity analysis. We know that the Sobol's index is a sensitivity grading method based on the proportion of parameters contribution to output variance. Among the abundant literature on sensitivity measures, the Sobol's indices have received much attention since they provide accurate information for most models. Polynomial chaos-based Sobol's indices are used in [60,61,62]. By deterministic, frequentist and Bayesian approaches, several sensitivity analyses are also conducted in [63]. These alternative methods of sensitivity analysis is effective. At the same time, compared with ordinary differential equation model, stochastic functional differential equation model (see [64,65,66,67]) will be more suitable for the actual situation. We will leave these interesting work for the future.
This work is supported by the National Natural Science Foundation of China (11861044 and 11661050), and the HongLiu first-class disciplines Development Program of Lanzhou University of Technology. The authors acknowledge the anonymous referees and editors very much for their fruitful comments and valuable suggestions which made this paper much improved.
The authors declare that there is no conflict of interests regarding the publication of this paper.
[1] |
U. Scherhag, C. Rathgeb, J. Merkle, R. Breithaupt, C. Busch, Face recognition systems under morphing attacks: A survey, IEEE Access, 7 (2019), 23012–23026. doi: 10.1109/ACCESS.2019.2899367
![]() |
[2] |
A. Fydanaki, Z. Geradts, Evaluating OpenFace: an open-source automatic facial comparison algorithm for forensics, Forensic Sci. Res., 3 (2018), 202–209. doi: 10.1080/20961790.2018.1523703
![]() |
[3] | M. P. Evison, R. W. V. Bruegge, The magna database: A database of three-dimensional facial images for research in human identification and recognition, Forensic Sci. Commun., 10 (2008), 1–11. |
[4] | J. Zeng, S. Shi, Q. Lu, Y. Li, X. Qiu, Face recognition techniques for forensic identification of human images, Int. J. Digital Content Technol. Its Appl., 11 (2017), 34–43. |
[5] |
J. Han, B. Bhanu, Individual recognition using gait energy image, IEEE Trans. Pattern Anal. Mach. Intell., 28 (2006), 316–322. doi: 10.1109/TPAMI.2006.38
![]() |
[6] | S. Bharadwaj, H. Bhatt, M. Vatsa, R. Singh, A. Noore, Quality assessment based denoising to improve face recognition performance, CVPR 2011 WORKSHOPS, Colorado Springs, CO, 2011,140–145. |
[7] | A. A. Dawood, M. F. Saleh, Review of different techniques for image denoising, Int. J. Innovative Res. Comput. Commun. Eng., 6 (2018), 2498–2505. |
[8] |
L. Fan, F. Zhang, H. Fan, C. Zhang, Brief review of image denoising techniques, Visual Comput. Ind. Biomed. Art, 2 (2019), 7. doi: 10.1186/s42492-019-0016-7
![]() |
[9] |
P. Janani, J. Premaladha, K. S. Ravichandran, Image enhancement techniques: A study, Indian J. Sci. Technol., 8 (2015), 125–145. doi: 10.17485/ijst/2015/v8iS6/71216
![]() |
[10] | G. Deng, L. W. Cahill, An adaptive Gaussian filtering for noise reduction and edge detection, Nuclear Science Symposium and Medical Imaging Conference, 3 (1993), 1615–1619. |
[11] | X. Jia, S. Liu, X. Feng,, L. Zhang, FOCNet: A fractional optimal control network for image denoising, 2019 IEEE/CVF Conference on Computer Vision and Pattern Recognition (CVPR), 2019. Available from: https://ieeexplore.ieee.org/document/8954104. |
[12] | C. Tian, Y. Xu, L. Fei, K. Yan, Deep learning for image denoising: a survey, International Conference on Genetic and Evolutionary Computing, 384 (2019), 563–572. |
[13] |
C. Knaus, M. Zwicker, Progressive image denoising, IEEE Trans. Image Process., 23 (2014), 3114–3125. doi: 10.1109/TIP.2014.2326771
![]() |
[14] | S. Cha, T. Moon, Fully convolutional pixel adaptive image denoiser, 2019 IEEE/CVF International Conference on Computer Vision (ICCV), 2019, 4160–4169. |
[15] | J. Xu, L. Zhang, D. Zhang, A trilateral weighted sparse coding scheme for real-world image denoising, The European Conference on Computer Vision (ECCV), 2018, 20–36. |
[16] | R. Wang, X. Xiao, B. Guo, Q. Qin, R. Chen, An effective image denoising method for UAV images via improved generative adversarial networks, Sensors, 18 (2018), 1985. |
[17] |
M. Diwakar, M. Kumar, A review on CT image noise and its denoising, Biomed. Signal Process. Control, 42 (2018), 73–88. doi: 10.1016/j.bspc.2018.01.010
![]() |
[18] | J. Benesty, J. D. Chen, Y. T. Huang, Study of the widely linear wiener filter for noise reduction, IEEE International Conference on Acoustics, Speech, and Signal Processing, Dallas, Texas, USA, 2010,205–208. |
[19] |
S. G. Mallat, A theory for multiresolution signal decomposition: the wavelet representation, IEEE Trans. Pattern. Anal. Mach. Intell., 11 (1989), 674–693. doi: 10.1109/34.192463
![]() |
[20] | W. Zhang, R. Li, K. He, J. Zhou, Approach to image denoising and enhancement based on 3DDCT, J. Univ. Electron. Sci. Technol. China, 40 (2011), 742–746. |
[21] | T. Yan, M. Qu, C. Zhou, Image denoising with BEMD and edge-preserving self-snake model, International Conference on Intelligent Computing, 8588 (2014), 435–442. |
[22] |
K. Nguyen, C. Fookes, S. Sridharan, M. Tistarelli, M. Nixon, Super-resolution for biometrics: A comprehensive survey, Pattern Recognit., 78 (2018), 23–42. doi: 10.1016/j.patcog.2018.01.002
![]() |
[23] | J. Jiang, J. Ma, C. Chen, X. Jiang, Z. Wang, Noise robust face image super-resolution through smooth sparse representation, IEEE Trans. Cybernetics, 47 (2016), 3991–4002. |
[24] | R. Tkachenko, P. Tkachenko, I. Izonin, Y. Tsymbal, Learning-based image scaling using neural-like structure of geometric transformation paradigm, in Advances in Soft Computing and Machine Learning in Image Processing, (2018), 537–565. |
[25] | I. Izonin, R. Tkachenko, D. Peleshko, T. Rak, D. Batyuk, Learning-based image super-resolution using weight coefficients of synaptic connections, 2015 Xth International Scientific and Technical Conference "Computer Sciences and Information Technologies" (CSIT), 2015, 25–29. |
[26] | Y. Rashkevych, D. Peleshko, O. Vynokurova, I. Izonin, N. Lotoshynska, Single-frame image super-resolution based on singular square matrix operator, 2017 IEEE First Ukraine Conference on Electrical and Computer Engineering, 2017,944–948. |
[27] | M. Emambakhsh, J. Gao, A.N. Evans, An evaluation of denoising algorithms for 3D face recognition, 5th International Conference on Imaging for Crime Detection and Prevention, London, 2013. |
[28] | L. Zhang, L. Zhang, L. Li, Illumination quality assessment for face images: A benchmark and a convolutional neural networks based model, in International Conference on Neural Information Processing, Springer, Cham, 2017,583–593. |
[29] |
N. Zhuang, Q. Zhang, C. Pan, B. Ni, Y. Xu, X. Yang, W. Zhang, Recognition oriented facial image quality assessment via deep convolutional neural network, Neurocomputing, 358 (2019), 109–118. doi: 10.1016/j.neucom.2019.04.057
![]() |
[30] |
J. Yu, K. Sun, F. Gao, S. Zhu, Face biometric quality assessment via light CNN, Pattern Recog. Lett., 107 (2018), 25–32. doi: 10.1016/j.patrec.2017.07.015
![]() |
[31] | V. V. Starovoitov, D. I. Samal, D. V. Briliuk, Image enhancement for face recognition, International Conference on Iconics, St. Petersburg, Russia, 2003. |
[32] |
T. Ojala, M. Pietikainen, T. Maenpaa, Multiresolution gray-scale and rotation invariant texture classification with local binary patterns, IEEE Trans. Pattern Anal. Mach. Intell., 24 (2002), 971–987. doi: 10.1109/TPAMI.2002.1017623
![]() |
[33] |
S. Chang, B. Yu, M. Vetterli, Adaptive wavelet thresholding for image denoising and compression, IEEE Trans. Image Process., 9 (2000), 1532–1546. doi: 10.1109/83.862633
![]() |
[34] | D. Andrews, C. Mallows, Scale mixtures of normal distributions, J. Royal Stat. Soc., 36 (1974), 99–102. |
[35] | A. Martinez, R. Benevento, The AR face database, CVC Technical Report #24, 1998. |
[36] |
P. J. Phillips, P. J. Flynn, T. Scruggs, K. W. Bowyer, J. Chang, K. Hoffman, et al., Overview of the face recognition grand challenge, 2005 IEEE Computer Society Conference on Computer Vision and Pattern Recognition (CVPR'05), 1(2005), 947–954. doi: 10.1109/CVPR.2005.268
![]() |
[37] | S. Mittal, S. Agarwal, M. J. Nigam, Real time multiple face recognition: A deep learning approach, Proceedings of the 2018 International Conference on Digital Medicine and Image Processing, 2018, 70–76. |
[38] | I. Masi, Y. Wu, T. Hassner, P. Natarajan, Deep face recognition: A survey, 2018 31st SIBGRAPI Conference on Graphics, Patterns and Images (SIBGRAPI), 2018. Available from: https://ieeexplore.ieee.org/document/8614364. |
[39] |
G. Gilboa, N. Sochen, Y. Y. Zeevi, Image enhancement and denoising by complex diffusion process, IEEE Trans. Patt. Anal. Mach. Intell., 26 (2004), 1020–1036. doi: 10.1109/TPAMI.2004.47
![]() |
[40] |
P. Perona, J. Malik, Scale space and edge detection using anisotropic diffusion, IEEE Trans. Pattern Anal. Mach. Intell., 12 (1990), 629–639. doi: 10.1109/34.56205
![]() |
1. | XinQi Dong, Ruijia Chen, Gender differences in the experience of loneliness in U.S. Chinese older adults, 2017, 29, 0895-2841, 115, 10.1080/08952841.2015.1080534 | |
2. | Xinqi Dong, Mengting Li, Yingxiao Hua, The Association Between Filial Discrepancy and Depressive Symptoms: Findings From a Community-Dwelling Chinese Aging Population, 2017, 72, 1079-5006, S63, 10.1093/gerona/glx040 | |
3. | XinQi Dong, Stephanie M. Bergren, E-Shien Chang, Traditional Chinese Medicine Use and Health in Community-Dwelling Chinese-American Older Adults in Chicago, 2015, 63, 00028614, 2588, 10.1111/jgs.13828 | |
4. | Naglaa A. El-Sherbiny, Asmaa Younis, Mohamed Masoud, A comprehensive assessment of the physical, nutritional, and psychological health status of the elderly populace in the Fayoum Governorate (Egypt), 2016, 66, 01674943, 119, 10.1016/j.archger.2016.06.001 | |
5. | Jinkyung Cho, Youngyun Jin, Inhwan Lee, Haeryun Hong, Donghyun Kim, Soohyun Park, Hyunsik Kang, Physical inactivity and cognitive impairment in Korean older adults: gender differences in potential covariates, 2017, 44, 0301-4460, 729, 10.1080/03014460.2017.1392604 | |
6. | Jinyu Liu, XinQi Dong, Duy Nguyen, Daniel W. L. Lai, Family Relationships and Depressive Symptoms Among Chinese Older Immigrants in the United States, 2017, 72, 1079-5006, S113, 10.1093/gerona/glw138 | |
7. | XinQi Dong, Dexia Kong, Dinesh Mendhe, Stephanie M. Bergren, Leveraging Technology to Improve Health Disparity Research: Trilingual Data Collection Using Tablets, 2019, 67, 0002-8614, 10.1111/jgs.16097 | |
8. | Xiaochuan Wang, Stephanie Bergren, XinQi Dong, Association Between Social Support and Depression Help-Seeking Behaviors Among U.S. Chinese Older Adults, 2023, 35, 0898-2643, 83, 10.1177/08982643221106870 | |
9. | Ethan Siu Leung Cheung, Ada C. Mui, Gender Variation and Late-life Depression: Findings from a National Survey in the USA, 2023, 48, 0163-5158, 263, 10.1007/s12126-021-09471-5 | |
10. | Li-Chi Huang, Ching-Ling Lin, Yao-Tsung Chang, Ruey-Yu Chen, Chyi-Huey Bai, Gender Impact on Diabetes Distress Focus at Medical Communication Concerns, Life and Interpersonal Stress, 2022, 19, 1660-4601, 15678, 10.3390/ijerph192315678 | |
11. | Sizhe Liu, Wei Zhang, Keqing Zhang, Bei Wu, The Association between Intergenerational Support and Self-Rated Health among Chinese Older Adults: Do Resilience and Gender Matter?, 2023, 42, 0733-4648, 111, 10.1177/07334648221127882 | |
12. | Magdalena Żebrowska, Susanne Strohmaier, Curtis Huttenhower, A. Heather Eliassen, Oana A. Zeleznik, Carri Westgarth, Tianyi Huang, Francine Laden, Jaime E. Hart, Bernard Rosner, Ichiro Kawachi, Jorge E. Chavarro, Olivia I. Okereke, Eva S. Schernhammer, Pet Attachment and Anxiety and Depression in Middle-Aged and Older Women, 2024, 7, 2574-3805, e2424810, 10.1001/jamanetworkopen.2024.24810 | |
13. | Ethan Siu Leung Cheung, The Association between Residential Environment and Self-Rated Mental Health among Older Canadians: The Moderating Effects of Education and Gender, 2024, 0714-9808, 1, 10.1017/S0714980824000230 |
Parameter | Value | Source |
μ: Natural death rate | 0.25 | [42] |
β: Rate of susceptible individuals turn to drink | 0.1 | [5] |
a1,a2: Death rates due to excessive drinking | 0.01 | [42] |
q: Proportion of these individuals is assumed to be uneducated | 0.6 | [42] |
Λ: New recruits enter the population | 0.8 | [5] |
σ: Overall effectiveness of the public health educational campaigns | 0.2 | [42] |
ε: Rate of transfer from educated individuals to quit drinkers | 0.2 | [42] |
δ: Rate of temporarily recovered drinkers enter into alcoholics compartment | 0.1 | [42] |
γ: Rate of transfer from alcoholics to temporarily recovered drinkers | 0.5 | [5] |
ξ: Rate of transfer from temporarily recovered drinkers to quit drinkers | 0.4 | [42] |
p: Rate of uneducated individuals enter into educated individuals | 0.1 | [Assumed] |
Parameter | Value | Source |
μ: Natural death rate | 0.25 | [42] |
β: Rate of susceptible individuals turn to drink | 0.1 | [5] |
a1,a2: Death rates due to excessive drinking | 0.01 | [42] |
q: Proportion of these individuals is assumed to be uneducated | 0.6 | [42] |
Λ: New recruits enter the population | 0.8 | [5] |
σ: Overall effectiveness of the public health educational campaigns | 0.2 | [42] |
ε: Rate of transfer from educated individuals to quit drinkers | 0.2 | [42] |
δ: Rate of temporarily recovered drinkers enter into alcoholics compartment | 0.1 | [42] |
γ: Rate of transfer from alcoholics to temporarily recovered drinkers | 0.5 | [5] |
ξ: Rate of transfer from temporarily recovered drinkers to quit drinkers | 0.4 | [42] |
p: Rate of uneducated individuals enter into educated individuals | 0.1 | [Assumed] |