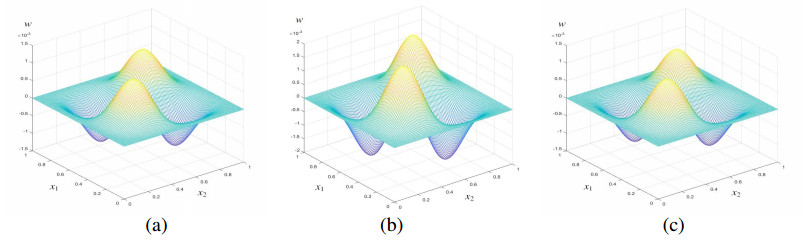
The main purpose of this paper was to study the reduced-dimension of unknown classical two-grid finite element (CTGFE) solution coefficient vectors for the nonlinear time-fractional wave (NTFW) equation by using proper orthogonal decomposition (POD). For this purpose, a CTGFE method with unconditional stability for the NTFW equation and the error estimates of CTGFE solutions were reviewed. Then, the CTGFE method was rewritten into matrix form, and the unknown solution coefficient vectors in the matrix CTGFE method were reduced by the POD method, so a new reduced-dimension TGFE (RDTGFE) method was created. The biggest contribution of this paper consists in analyzing theoretically the existence, stability, and errors of the RDTGFE solutions, and in applications, verifying the correctness of the obtained theoretical results and the advantages of the RDTGFE method. The RDTGFE method can not only greatly reduce the unknowns of the CTGFE method and the simplify computational process but also greatly save CPU runtime and improve the computational efficiency. Therefore, the RDTGFE method is worth studying and spreading.
Citation: Liang He, Yihui Sun, Zhenglong Chen, Fei Teng, Chao Shen, Zhendong Luo. The POD-based reduced-dimension study on the two-grid finite element method for the nonlinear time-fractional wave equation[J]. AIMS Mathematics, 2025, 10(2): 3408-3427. doi: 10.3934/math.2025158
[1] | Huiqin Zhang, Yanping Chen . Two-grid finite element method with an H2N2 interpolation for two-dimensional nonlinear fractional multi-term mixed sub-diffusion and diffusion wave equation. AIMS Mathematics, 2024, 9(1): 160-177. doi: 10.3934/math.2024010 |
[2] | Zhichao Fang, Ruixia Du, Hong Li, Yang Liu . A two-grid mixed finite volume element method for nonlinear time fractional reaction-diffusion equations. AIMS Mathematics, 2022, 7(2): 1941-1970. doi: 10.3934/math.2022112 |
[3] | Changling Xu, Hongbo Chen . A two-grid $ P_0^2 $-$ P_1 $ mixed finite element scheme for semilinear elliptic optimal control problems. AIMS Mathematics, 2022, 7(4): 6153-6172. doi: 10.3934/math.2022342 |
[4] | Yanjie Zhou, Xianxiang Leng, Yuejie Li, Qiuxiang Deng, Zhendong Luo . A novel two-grid Crank-Nicolson mixed finite element method for nonlinear fourth-order sin-Gordon equation. AIMS Mathematics, 2024, 9(11): 31470-31494. doi: 10.3934/math.20241515 |
[5] | Jeong-Kweon Seo, Byeong-Chun Shin . Reduced-order modeling using the frequency-domain method for parabolic partial differential equations. AIMS Mathematics, 2023, 8(7): 15255-15268. doi: 10.3934/math.2023779 |
[6] | Mario Ohlberger, Barbara Verfürth . Localized Orthogonal Decomposition for two-scale Helmholtz-typeproblems. AIMS Mathematics, 2017, 2(3): 458-478. doi: 10.3934/Math.2017.2.458 |
[7] | Zuliang Lu, Fei Cai, Ruixiang Xu, Lu Xing . Convergence and proposed optimality of adaptive finite element methods for nonlinear optimal control problems. AIMS Mathematics, 2022, 7(11): 19664-19695. doi: 10.3934/math.20221079 |
[8] | Ailing Zhu, Yixin Wang, Qiang Xu . A weak Galerkin finite element approximation of two-dimensional sub-diffusion equation with time-fractional derivative. AIMS Mathematics, 2020, 5(5): 4297-4310. doi: 10.3934/math.2020274 |
[9] | Zuliang Lu, Xiankui Wu, Fei Huang, Fei Cai, Chunjuan Hou, Yin Yang . Convergence and quasi-optimality based on an adaptive finite element method for the bilinear optimal control problem. AIMS Mathematics, 2021, 6(9): 9510-9535. doi: 10.3934/math.2021553 |
[10] | Zhongdi Cen, Jian Huang, Aimin Xu . A posteriori grid method for a time-fractional Black-Scholes equation. AIMS Mathematics, 2022, 7(12): 20962-20978. doi: 10.3934/math.20221148 |
The main purpose of this paper was to study the reduced-dimension of unknown classical two-grid finite element (CTGFE) solution coefficient vectors for the nonlinear time-fractional wave (NTFW) equation by using proper orthogonal decomposition (POD). For this purpose, a CTGFE method with unconditional stability for the NTFW equation and the error estimates of CTGFE solutions were reviewed. Then, the CTGFE method was rewritten into matrix form, and the unknown solution coefficient vectors in the matrix CTGFE method were reduced by the POD method, so a new reduced-dimension TGFE (RDTGFE) method was created. The biggest contribution of this paper consists in analyzing theoretically the existence, stability, and errors of the RDTGFE solutions, and in applications, verifying the correctness of the obtained theoretical results and the advantages of the RDTGFE method. The RDTGFE method can not only greatly reduce the unknowns of the CTGFE method and the simplify computational process but also greatly save CPU runtime and improve the computational efficiency. Therefore, the RDTGFE method is worth studying and spreading.
The partial differential equations (PDEs) with fractional derivatives have an important physics background, can be used to describe some natural phenomena, and have been applied in many fields, such as nonlinear dynamics [1], physics [2], and bioengineering [3]. Due to the complexity of the fractional PDEs, their analytical solutions are usually not available. Hence, the numerical methods of the fractional PDEs have received much attention. For instance, a high-order finite difference (FD) scheme of the two-dimensional (2D) time-space tempered fractional diffusion wave equation was studied in [4], a high-order FD algorithm for the fractional diffusion wave equation with the Caputo fractional derivative was addressed in [5], a higher order FD algorithm of the fractional-order reaction and anomalous diffusion equation was presented in [6], a reduced-order FD scheme of the fractional-order parabolic-type sine-Gordon equations was posed in [7], an optimized FD scheme of the fractional-order parabolic-type sine-Gordon equations was proposed in [8], a Crank–Nicolson finite element (FE) method of the symmetric tempered fractional diffusion equation was constructed in [9], and a meshless method for solving a wide class of time-fractional PDEs was developed in [10]. However, these time-fractional PDEs are linear, so only single-layer grid numerical methods are needed to solve them. Recently, a two-grid FE (TGFE) method for the nonlinear time-fractional wave (NTFW) equation with two strong nonlinear terms was established in [11], which was composed of a nonlinear FE system of equations on the coarser grids and a linear FE system of equations on the finer grids with sufficient accuracy, thus simplifying the calculation and improving the computational efficiency.
The TGFE method was initially used to solve nonlinear elliptic equations (see [12]). Afterward, Shi's and Liu's teams [13,14,15] used the method to solve some more complicated nonlinear PDEs. Though the classical TGFE (CTGFE) method for solving the NTFW equations in [11] can greatly simplify the calculation and improve the computational efficiency, when it is used for the actual engineering calculations, the unknowns can be as high as tens of millions. Therefore, the main purpose of this paper is to create a new reduced-dimension TGFE (RDTGFE) method by using proper orthogonal decomposition (POD) in [16, Sec. 5.1] to reduce the dimension of unknown coefficient vectors in the CTGFE solutions in [11] and keep the FE basic functions unchanged. Thus, the created RDTGFE method can not only have the advantages of the CTGFE method, but also greatly reduce unknowns, mitigate calculation burden, retard the accumulation of computation errors, and enhance computation efficiency. Therefore, it is a study with practical application value.
A large number of numerical tests, such as the natural boundary element space reduced-dimension method in [17], the FE space reduced-dimension methods in [18,19], and the Galerkin space reduced-dimension methods in [20,21,22], show that the POD method is one of the most effective approaches for reducing the unknowns of numerical methods. However, the above reduced-dimension methods all belong to the category of dimensionality reduction methods of FE space, i.e., FE basic functions (since FE subspace is spanned by the FE basic functions) for the Galerkin and FE methods, which are thoroughly distinct from the RDTGFE method in this article and are further explained as follows.
It is well known that the unknown Galerkin and FE solutions wkh at moment tk are equal to the linear combination of the known FE basis functions ζ1(x), ζ2(x),⋯, ζMh(x) and unknown solution coefficients wk1, wk2, ⋯,wkMh at moment tk, i.e., are equal to the inner product of the known FE bases function vectors ζ=(ζ1(x),ζ2(x),⋯, ζMh(x))T and unknown solution coefficient vectors wk=(wk1,wk2, ⋯,wkMh)T, namely that
wkh=ζ⋅wk,1⩽k⩽K, | (1.1) |
where Mh is the number of FE basic functions, which is equal to the number of FE grid nodes, and K is the number of time nodes.
Both the Galerkin reduced-dimension methods and FE reduced-dimension methods mentioned above are designed by reducing the dimension of the FE basic function vectors ζ, while the RDTGFE method of this paper is created by reducing the dimension of unknown solution coefficient vectors wk and keeping the FE basic function vectors ζ unchanged. Therefore, the RDTGFE method is quite different from the previous reduced-dimension method of the FE basic function vectors, i.e., FE subspace.
Although the RDTGFE methods for the nonlinear wave equation [23], the 3D nonlinear elastodynamic sin-Gordon problem [24], the unsaturated soil water flow problem [25], the nonlinear fourth-order reaction diffusion equation with a temporal fractional derivative [26], and the spatial fractional nonlinear Allen-Cahn equations [27] have been developed, the NTFW equation with two strong nonlinear terms and a time-fractional derivative as well as a second time derivative in this paper is much more complicated that the above five equations with only a nonlinear term. Hence, both the design of the RDTGFE format and the theory analysis for the existence, unconditional stableness, and error estimations for the RDTGFE solutions are confronted with more difficulties and require more techniques than all the previous works. But, as just mentioned above, the RDTGFE method for the equation with two strong nonlinear terms has more important applications than the previous works. Hence, it is very valuable to study the RDTGFE method of the NTFW equation.
The rest of this article is organized as follows. First, in Section 2, we review the CTGFE method in [11] and provide the existence and unconditional stableness as well as error estimates of the CTGFE solutions. Thereafter, in Section 3, we create a new RDTGFE method by using the POD method only to reduce the dimension of unknown coefficient vectors in the CTGFE solutions and keep the FE basis functions unchanged, and prove the existence and stableness as well as error estimates of the RDTGFE solutions by using matrix analysis. Afterward, in Section 4, we use some numerical tests to confirm the effectiveness of the RDTGFE method and correctness of our theory results. Finally, in Section 5, we generalize the main conclusions to this article and provide some expectations for the future research.
The NTFW equation with two strong nonlinear terms is found in [11], which is restated as follows:
Problem 1. Find w:[0,te)→C2(ˉΩ) such that
{wtt(x,t)+∂γtw(x,t)−÷(a(w(x,t))∇w(x,t))=f(w(x,t))+g(x,t),∀(x,t)∈Ω×(0,te),w(x,t)=0,∀(x,t)∈∂Ω×(0,te),w(0,x)=w0(x),wt(0,x)=w1(x),∀x∈Ω, | (2.1) |
where te is the given last moment, C2(ˉΩ) represents a space consisting of functions with second-order continuous derivatives, Ω⊂Rn (n=1,2,3) is a connected bounded region with boundary ∂Ω, wt=∂w/∂t, wtt=∂2w/∂t2, ∂γtw(x,t) (1<γ<2) is the Caputo time-fractional derivative, defined as follows:
∂γtw(x,t)=1Γ(2−γ)∫t0∂2w(x,s)∂s21(t−s)γ−1ds, | (2.2) |
a(w)>0 and f(w) are two nonlinear functions that can be arbitrarily selected according to the needs of the object of study and are bounded second-order derivatives, namely, there are two positive numbers αi>0 (i=1,2) such that
α1⩽a(w)+|a′(w)|+|a″(w)|+|f′(w)|+|f″(w)|⩽α2, |
and the source term g(x,t), and the initial values w0(x) and w1(x) are three sufficiently smooth known functions.
The Sobolev spaces and norms used hereinafter are standard (see [16,28,29]). For example, the Sobolev space Hm(Ω) is also a Hilbert space, which is defined as follows:
Hm(Ω)={υ:∫Ω∑0⩽|α|⩽m|∂αυ∂xα|2dx<∞},α=(α1,α2,⋯,αn),|α|=n∑i=1αi, |
where m⩾0 and αi⩾0 (i=1,2,⋯,n) are integers. Especially, the subspace Hm0(Ω) of Hm(Ω) is defined as follows:
Hm0(Ω)={υ∈Hm(Ω):∑0⩽|α|<m|∂αυ∂xα|∂Ω=0}. |
The norm and semi-norm are respectively defined as follows:
‖υ‖l={∫Ω∑0⩽|α|⩽m|∂αυ∂xα|2dx}1/2and|υ|l={∫Ω∑|α|=m|∂αυ∂xα|2dx}1/2. |
Let U=H10(Ω). Thus, by Green's formula, we can build the following variational format of Problem 1.
Problem 2. For any t∈(0,te), find w∈U such that
{(wtt,υ)+(∂γtw,υ)+(a(w)∇w,∇υ)=(f(w),υ)+(g,υ),∀υ∈U,w(x,0)=w0(x),wt(x,0)=w1(x),x∈Ω, | (2.3) |
where (w,υ)=∫Ωw(x,t)υ(x,t)dx is the L2 inner product.
The existence and stability for the generalized solutions to Problem 2 can be proved by the proof method of Theorem 3.3.1 in [16].
To create the CTGFE method, we need to employ, respectively, difference quotient and the two-grid FE method to discretize the time derivative and the spatial variables in Problem 2. For this end, we first assume that K>0 is an integer, Δt=te/K is the time step increment, wk (0⩽k⩽K) is the approximations to w(x,t) at tk=kΔt, and
ˉwk=wk+wk−12,ˆwk=wk+wk−22,ˉ∂twk=wk−wk−1Δt,ˆ∂twk=wk−wk−22Δt,ˉ∂2twk=wk−2wk−1+wk−2Δt2. |
Thus, when w∈c3[0,te], by Taylor's formula, we get
wk−1t=ˆ∂twk+O(Δt2)=ˆwkt+O(Δt2),w1t=w0t+w0ttΔt+O(Δt2). | (2.4) |
When w∈c3[0,te], if we set that
ˆ∂γtwk=12(∂γtwk+∂γtwk−2),k⩾3;ˆ∂γtwk=12∂γtwk,k=2, | (2.5) |
then, by the technique in [11], the mean value ˆ∂γtwk of the Caputo time-fractional derivative ∂γtw(x,t) (1<γ<2) can be approximated by
ˆ∂γtwk=Δt1−γΓ(3−γ)(b0ˆ∂twk−k−1∑j=2(bk−j−1−bk−j)ˆ∂twj−bk−2−bk−12w0tt−bk−2w0t)+O(Δt3−γ):=ˆDγtwk+O(Δt3−γ),k⩾2, | (2.6) |
where bj=(j+1)2−γ−j2−γ>0, satisfying
1=b0>b1>b2>⋯>bk>0,bk→0(k→∞);k−1∑j=1(bj−1−bj)=b0−bk−1<1,1⩽k⩽K. | (2.7) |
Then, we assume that JH is a coarse grid on ˉΩ that is quasi-uniformly divided, which is composed of two-dimensional triangles or quadrangles and three-dimensional tetrahedrons or hexahedrons, and H represents the maximum diameter of all E∈JH. Thus, the FE space defined on the coarse grids is expressed by
UH={vH∈C(ˉΩ)∩U:vH|E∈Pl(E),∀E∈JH}, |
where Pl(E) (l⩾1) stands for the space of polynomials with degree ⩽l defined on the coarse grid element E∈JH.
Afterward, we assume that Jh is a fine grid on ˉΩ that is a quasi-uniform division and h represents the maximum diameter of all e∈Jh (h≪H). Likewise, the FE space defined on the fine grids Jh is denoted by
Uh={vh∈C(ˉΩ)∩U:vh|e∈Pl(e),∀e∈Jh}. |
If we assume that Rδ:U→Uδ (δ=H,h) are two Ritz projections, i.e., for any u∈U, there are two unique Rδu∈Uδ such that
(∇(u−Rδu),∇ϑδ)=0,∀ϑδ∈Uδ,δ=H,h, | (2.8) |
then we get the following error estimates:
|u−Rδu|r⩽Cδl+1−r,ifu∈U∩Hl+1(Ω),δ=H,h,r=−1,0,1. | (2.9) |
Thus, the CTGFE method can be created in the following.
Problem 3. Step 1. Find wkH∈UH (1⩽k⩽K) defined on the coarse grid JH such that they satisfy the following nonlinear system of equations:
{(ˉ∂2twkH+ˆDγtwkH,υH)+(a(ˆwkH)∇wkH,∇υH)=(f(ˆwkH),υH)+(ˆgk,υH),∀υH∈UH,2⩽k⩽K;w0H=RHw0,w1H=w0H+ΔtRHw1+Δt22RHwtt,inΩ. | (2.10) |
Step 2. Find wkh∈Uh (1⩽k⩽K) defined on the fine grid Jh such that they satisfy the following linear system of equations:
{(ˉ∂2twkh+ˆDγtwkh,υh)+(a(ˆwkH)∇ukh,∇υh)=(f(ˆwkH),υh)+(ˆgk,υh),∀υh∈Uh,2⩽k⩽K;w0h=Rhw0,w1h=w0h+ΔtRhw1+Δt22Rhwtt,inΩ. | (2.11) |
The following results of existence, stability, and error estimates of the CTGFE solutions for Problem 3 have been provided in [11, Theorems 4.1 and 4.2].
Theorem 1. Problem 3 has a unique set of solutions {wkH}Kk=1⊂UH defined on the coarse grid JH and a unique set of solutions {wkh}Kk=1⊂Uh defined on the fine grid Jh, respectively, satisfying the following unconditional boundedness, i.e., unconditional stability:
‖wkH‖1+‖wkh‖1⩽c(‖w0‖1+‖w1‖1+‖f(0)‖0+‖g‖0),1⩽k⩽K, | (2.12) |
where and hereinafter c is also a positive constant independent of H, h, and Δt. Furthermore, when H=O(Δt3−γ) and h=O(H1+1/l), they have the following error estimations:
‖w(tk)−wkH‖0+H‖∇(w(tk)−wkH)‖0⩽c(Δt3−γ+Hl+1),1⩽k⩽K, | (2.13) |
‖w(tk)−wkh‖0+h‖∇(w(tk)−wkh)‖0⩽c(Δt3−γ+hl+1),1⩽k⩽K, | (2.14) |
where w(tk)=w(x,tk).
Remark 1. Theorem 1 shows that the CTGFE solutions are unconditionally stable and can theoretically achieve an optimal order error estimate.
The most critical step in creating the RDTGFE method is to rewrite the CTGFE method in matrix form. By the orthogonalization principle in functional analysis in [30, Sect. 1.6.3] and the existence of orthonormal basis functions in [30, Proposition 1.6.21], we claim that the FE subspaces UH and Uh have, respectively, a set of orthonormal basis functions {ζi}MHi=1 and {ξi}Mhi=1 under the L2 inner product (⋅,⋅), where MH and Mh are the dimensions of the FE subspaces UH and Uh, respectively. Thus, the FE subspaces UH and Uh can be reexpressed by
UH={wH∈[C(ˉΩ)]∩U:wH|E∈[Pl(E)],∀E∈JH}=span{ζi:1⩽i⩽MH};Uh={wh∈[C(ˉΩ)]∩V:wh|e∈[Pl(e)],∀e∈Jh}=span{ξi:1⩽i⩽Mh}. |
By using the basis functions {ζi}MHi=1 and {ξi}Mhi=1, the CTGFE solutions wkH and wkh can be respectively denoted by
wkH=MH∑i=1Wkiζi=ζTWk,wkh=Mh∑i=1wkiξi=ξTwk, | (3.1) |
where ζ=(ζ1,ζ2,⋯,ζMH)T and ξ=(ξ1,ξ2,⋯,ξMh)T are, respectively, the basis function vectors formed by the orthonormal bases {ζi}MHi=1 and {ξi}Mhi=1, and Wk=(Wk1,Wk2,⋯, WkMH)T and wk=(wk1,wk2,⋯, wkMh)T are, respectively, vectors formed by the unknown coefficients in the CTGFE solutions wkH and wkh. Thus, Problem 3 may be rewritten as the follow matrix form.
Problem 4. Step 1. Find Wk∈RMH and wkH∈UH (1⩽k⩽K) such that they satisfy the following nonlinear system of equations:
{W0=((RHw0,ζ)),W1=W0+Δt((RHw1,ζ))+0.5Δt2((RHwtt(x,0),ζ)),(Wk−2Wk−1+Wk−2)+Δt2ˆDγtWk+Δt2BH(ˆWk)=Δt2FH(ˆWk)+Δt2GkH,2⩽k⩽K,wkH=ζTWk,1⩽k⩽K, | (3.2) |
where ˆDγtWk are obtained by replacing wk in (2.6) with Wk, and
BH(ˆWk)=0.5((a(0.5(ζTWk+ζTWk−2))(ζTWk+ζTWk−2),ζ)), |
FH(ˆWk)=((f(0.5(ζTWk+ζTWk−2)),ζ)), |
GkH=0.5((gk+gk−2,ζ)) |
are three MH-dimensional vectors.
Step 2. Find wk∈RMh and wkh∈Uh (1⩽k⩽K) such that they satisfy the following linear system of equations:
{w0=((Rhw0,ξ)),w1=w0+Δt((RHw1,ξ))+0.5Δt2((RHwtt(x,0),ξ)),(wk−2wk−1+wk−2)+Δt2ˆDγtwk+Δt2Bh(ˆwk)=Δt2Fh(^Wk)+Δt2Gh,2⩽k⩽K,wkh=ξTwk,1⩽k⩽K, | (3.3) |
where ˆDγtwk are obtained by replacing wk in (2.6) with wk, and
Bh(ˆwk)=0.5((a(0.5(ζTWk+ζTWk−2))(ξTwk+ξTwk−2),ξ)), |
Fh(ˆWk)=((f(0.5(ζTWk+ζTWk−2)),ξ)), |
Gkh=0.5((gk+gk−2,ξ)) |
are three Mh-dimensional vectors.
For Problem 4, we get the following conclusion.
Theorem 2. Under the same conditions as Theorem 1, Problem 4 has two unique sets of CTGFE solution coefficient vectors {Wk}Kk=1⊂RMH and {wk}Kk=1⊂RMh, and two unique sets of CTGFE solutions {wkH}Kk=1⊂UH and {wkh}Kk=1⊂Uh, defined respectively on the coarse grid division ℑH and the fine grid division ℑh, satisfying the following unconditional boundedness, i.e., unconditional stability:
‖Wk‖+‖wk‖⩽c(‖w0‖1+‖w1‖1+‖f(0)‖0+‖g‖0),1⩽k⩽K, | (3.4) |
where ‖Wk‖ (1⩽k⩽K) are the Euclidean norm for the vectors Wk.
Proof. Respectively multiplying (3.1) by the FE basis vectors ζ and ξ yields
Wk=wkHζ/‖ζ‖2,wk=wkhξ/‖ξ‖2,1⩽k⩽K. |
Thus, by Theorem 1, we assert that Problem 4 has two unique sets of CTGFE solution coefficient vectors {Wk}Kk=1⊂RMH and {wk}Kk=1⊂RMh. Further, by the third subsystem of equations in (3.2) and (3.3), we claim that Problem 4 has two unique sets of CTGFE solutions {wkH}Kk=1⊂UH and {wkh}Kk=1⊂Uh.
With the inverse estimate theorem and Theorem 1, we get
‖Wk‖+‖wk‖=|wkH|‖ζ‖/‖ζ‖+|wkh|‖ξ‖/‖ξ‖⩽c(‖wkH‖0,∞+‖wkh‖0,∞)⩽c(|wkH|1+|wkh|1)⩽c(‖w0‖1+‖w1‖1+‖f(0)‖0+‖g‖0),1⩽k⩽K. |
Thereupon, the CTGFE solution coefficient vectors Wk and wk (1⩽k⩽K) of Problem 4 are unconditionally bounded, i.e., unconditionally stable. This completes the proof of Theorem 2.
Remark 2. If the time-step increment Δt, the coarse and fine division parameters H and h, a(⋅), f(⋅), g(x,t), and the initial functions w0(x) and w1(x) are given, a set of CTGFE solutions {wkh}Kk=1⊂Uh can be solved by Problem 4. However, when Problem 4 is used to solve an actual engineering problem, it could contain many unknowns (often more than millions). Thereby, it is extremely necessary to employ the POD technique to reduce the dimension of the unknown coefficient vectors of the CTGFE solutions in the CTGFE method, i.e., Problem 4, and create a new RDTGFE method.
The POD basis vectors can be constructed by the following three steps.
(1) Find two sets of coefficient vectors of CTGFE solutions {Wk}Lk=1 and {wk}Lk=1 by the first and second equations of (3.3) and (3.4) at the first L time steps and make up two matrices AH=(W1,W2, ⋯,WL) and Ah=(w1,w2, ⋯,wL). When solving a practical engineering problem, AH and Ah may be formed by observations on the coarse grid division JH and fine grid division Jh without solving the CTGFE method (Problem 4).
(2) Find two sets of normalized eigenvectors ˆφκi (1⩽i⩽rκ =:rank(Aκ)) of matrices ATκAκ corresponding to two sets of positive eigenvalues λκ1⩾λκ2⩾⋯⩾λκrκ>0 (κ=H,h).
(3) Find two most-important sets of dκ (dκ⩽rκ) normalized vectors {φκ1,φκ2,⋯, φκdκ} of matrices AκATκ by the formulas φκi= Aκˆφκi/√λκi (1⩽i⩽dκ) to construct two matrices Φκ=(φκ1,φκ2, ⋯, φκdκ) (κ=H,h), which are called two sets of POD basis vectors.
By Sect. 5.1.2 in [16], we get that Φκ have the following properties:
‖Aκ−ΦκΦTκAκ‖2,2=√λκ(dκ+1),κ=H,h, | (3.5) |
in which ‖Aκ‖2,2=supv∈RL‖Aκv‖/‖v‖ (κ=H,h) and ‖v‖ still represents the Euclidean norm of vector v.
By (3.5), we get
‖ωkκ−ΦκΦTκωkκ‖=‖(Aκ−ΦκΦTκAκ)ek‖⩽‖Aκ−ΦκΦTκAκ‖2,2‖ek‖⩽√λκ(dκ+1),1⩽k⩽L,κ=H,h,ωH=W,ωh=w, | (3.6) |
where ek (1⩽k⩽L) represent the L-dimension normalized vectors with k-th element 1.
If we assume that
Wkd=(Wk1d,Wk2d,⋯,WkMHd)T,wkd=(wk1d,wk2d,⋯,wkMhd)T, |
βkH=(βkH1,βkH2,⋯,βkHdH)T,βkh=(βkh1,βkh2,⋯,βkhdh)T, |
then the RDTGFE solutions can be denoted by
wkHd=ζ⋅ΦHβkH,wkhd=ξ⋅Φhβkh,1⩽k⩽K. |
Thus, the first L coefficient vectors of RDTGFE solutions are immediately obtained by
Wkd=ΦHΦTHWk=:ΦHβkH,wkd=ΦhΦThwk=:Φhβkh,1⩽k⩽L. |
Thus, by replacing Wk and wk in Problem 4 with Wkd=ΦHβkH and wkd=Φhβkh (L+1⩽k⩽K) and using the orthogonality of vectors in Φκ, respectively, a novel RDTGFE method is created as follows.
Problem 5. Step 1. Find βkH∈RdH and wkHd∈UH (1⩽k⩽K) such that they satisfy the following system of nonlinear equations:
{βkH=ΦTHWk,1⩽k⩽L,βkH−2βk−1H+βk−2H+Δt2ΦTHˆDγtβkH+Δt2BH(βkH)=Δt2ΦTHFH(βkH)+Δt2ΦTHˆGkH,L+1⩽k⩽K,wkHd=ζT(ΦHβkH),1⩽k⩽K, | (3.7) |
where ˆDγtβkH are obtained by replacing wk in (2.6) with βkH, and
BH(βHk)=0.5((a(0.5(ζT(ΦHβkH)+ζT(ΦHβk−2H)))(ζT(ΦHβkH)+ζT(ΦHβk−2H),ζ)), |
FH(βkH)=((f(0.5(ζT(ΦHβkH)+ζT(ΦHβk−2H)),ζ)), |
ˆGkH=0.5(g(ζT(ΦHβkH)+ζT(ΦHβk−2H),ζ) |
are three MH-dimensional vectors.
Step 2. Find βkh∈Rdh and (wkhd,ukhd)∈Uh×Uh (1⩽k⩽K), satisfying the following system of linear equations:
{βkh=ΦThwk,1⩽k⩽L,βkh−2βk−1h+βk−2h+Δt2ΦThˆDγtβkh+Δt2Bh(βkh)=Δt2ΦThFh(βkH)+Δt2ΦThˆGkh,L+1⩽k⩽K,wkh=ξT(Φhβkh),1⩽k⩽K, | (3.8) |
where ˆDγtβkh are obtained by replacing wk in (2.6) with βkh, and
Bh(βhk)=0.5((a(0.5(ζT(ΦHβkH)+ζT(ΦHβk−2H)))(ξT(Φhβkh)+ξT(Φhβk−2H),ξ)), |
Fh(βkH)=((f(0.5(ζT(ΦHβkH)+ζT(ΦHβk−2H)),ζ)), |
ˆGkh=0.5(g(ζT(ΦHβkH)+ζT(ΦHβk−2H),ζ) |
are three MH-dimensional vectors.
Remark 3. It is clear that Problem 4 has (MH+Mh) unknowns per time step, while Problem 5 has only (dH+dh) unknowns per time step, and dH≪MH and dh≪Mh (for example, the numerical tests in Sect. 4, dH=dh=6, but MH=103 and Mh=106). Therefore, Problem 5 can greatly lessen the unknowns, thus greatly alleviating the computation load and saving the CPU time. In particular, Problem 5 has the same basis functions and accuracy as Problem 4. In other words, not only are the unknowns of Problem 5 greatly reduced, but the precision of the RDTGFE solutions of Problem 5 remains unchanged. Hence, the RDTGFE method is far superior over the CTGFE method.
The theoretical analysis of the existence, stability, and error estimates of RDTGFE solutions needs the following lemma, see [11, Lemma 3.1].
Lemma 1 (Discrete Gronwall's inequality). Let {ak} be a series of nonnegative real numbers, {ck} be a non-descending series of nonnegative numbers, and {δk} be a series of nonnegative real numbers, satisfying
ak⩽ck+k−1∑j=1δjaj,k⩾1. |
Then they also satisfy
ak⩽ckexp(k−1∑j=1δj),k⩾1. |
For Problem 5, we get the following results:
Theorem 3. Under the same conditions as Theorem 1, Problem 5 has two unique sets of RDTGFE solutions {wkHd}Kk=1⊂UH and {wkhd}Kk=1⊂Uh, satisfying the following unconditional stability:
‖wkHd‖1+‖wkhd‖1⩽c(‖w0‖1+‖w1‖1+‖f(0)‖0+‖g‖0),1⩽k⩽K. | (3.9) |
Furthermore, when h=O(H1+1/l), they satisfy the following error estimates:
‖w(tk)−wkHd‖0+H‖∇(w(tk)−wkHd)‖0⩽c(Δt3−γ+Hl+√λH(dH+1)),1⩽k⩽K, | (3.10) |
‖w(tk)−wkh‖0+h‖∇(w(tk)−wkhd)‖0⩽c(Δt3−γ+hl+1+√λH(dH+1)+√λh(dh+1)),1⩽k⩽K, | (3.11) |
where w(tk)=w(x,tk) (1⩽k⩽K).
Proof. The demonstration of Theorem 3 is divided into the following two steps.
(1) Discuss the existence as well as stability of RDTGFE solutions.
(ⅰ) When 1⩽k⩽L, by the first and third subsystems of (3.7) and (3.8), and Theorems 1 and 2, we assert that Problem 5 has two unique sets of RDTGFE solutions {wkHd}Lk=1⊂UH and {wkhd}Lk=1⊂Uh, satisfying (3.9) when 1⩽k⩽L.
(ⅱ) When L+1⩽k⩽K, by using Wkd=ΦHβkH and wkd=Φhβkh, we can rewrite (3.7) and (3.8) as the following two systems of equations:
{Wkd=ΦHΦTHWk,1⩽k⩽L,Wkd−2Wk−1d+Wk−2d+Δt2ˆDγtWkd+Δt2BH(Wkd)=Δt2FH(Wkd)+Δt2ˆGkH,L+1⩽k⩽K,wkHd=ζT(Wkd),1⩽k⩽K. | (3.12) |
{wkd=ΦHΦTHwk,1⩽k⩽L,wkd−2wk−1d+wk−2d+Δt2ˆDγtwkd+Δt2Bh(Wkd)=Δt2Fh(Wkd)+Δt2ˆGkh,L+1⩽k⩽K,wkhd=ζT(wkd),1⩽k⩽K. | (3.13) |
Noting that the second and third equations in (3.12) and (3.13) have the same form as the second and third equations in (3.2) and (3.3) of Problem 4, by using the same proof as Theorems 1 and 2, we can prove that when L+1⩽k⩽K, Problem 5 has two unique sets of RDTGFE solutions {wkHd}Kk=L+1⊂UH and {wkhd}Kk=L+1⊂Uh, satisfying (3.9) when L+1⩽k⩽K.
Synthesizing (ⅰ) and (ⅱ), we assert that Problem 5 has two unique sets of RDTGFE solutions {wkHd}Kk=1⊂UH and {wkhd}Kk=1⊂Uh, satisfying (3.9).
(2) Discuss the errors of the RDTGFE solutions.
(a) When 1⩽k⩽L, noting that wkH=ζTW, wkh=ξTw, ‖ζ‖1⩽c, and ‖ξ‖1⩽c, and using (3.6), we obtain
|wkH−wkHd|1=|ζT(Wk−Wkd)|1⩽|ζ|1‖Wk−Wkd‖⩽c√λH(dH+1), | (3.14) |
|wkh−wkhd|1=|ξT(wk−wkd)|1⩽|ξ|1‖wk−wkd‖⩽c√χh(dh+1). | (3.15) |
(b) When L+1⩽k⩽K, by subtracting the second equations of (3.12) and (3.13) from the second equations of (3.2) and (3.3), respectively, setting θk=Wk−Wkd and ˜θk=wk−wkd, taking the inner product by left multiplying with (θk−θk−2)T and (˜θk−˜θk−2)T, respectively, and using the Lagrange differential mean value theorem, (2.7), and (3.6), we get
(θk−θk−1)T(θk−θk−1)=(θk−1−θk−2)T(θk−1−θk−2)−Δt2(θk−θk−2)TˆDγt(Wk−Wkd)−Δt2(θk−θk−2)T(BH(Wk)−BH(Wkd))+Δt2(θk−θk−2)T(FH(Wk)−FH(Wkd))⩽(θk−1−θk−2)T(θk−1−θk−2)+cλH(dH+1)+cΔt3−γ(θk−θk−2)T(θk−θk−2)+cΔt2−γk−1∑j=L+1(bk−j−1−bk−j)(θk−θk−2)T(θj−θj−2)+cΔt2(‖θk‖+‖θk−2‖)(‖θk‖+‖θk−2‖)⩽(θk−1−θk−2)T(θk−1−θk−2)+cΔt3−γλH(dH+1)+cΔt3−γ(‖θk‖2+‖θk−2‖2)+cΔt3−γk−1∑j=L+1(bk−j−1−bk−j)(‖θk‖+‖θk−2‖)(‖θj‖+‖θj−2‖)+cΔt2(‖θk‖2+‖θk−2‖2), | (3.16) |
(˜θk−˜θk−1)T(˜θk−θk−1)=(˜θk−1−˜θk−2)T(˜θk−1−˜θk−2)−Δt2(˜θk−˜θk−2)TˆDγt(wk−wkd)−Δt2(˜θk−˜θk−2)T(Bh(Wk)−Bh(Wkd))+Δt2(˜θk−˜θk−2)T(Fh(Wk)−Fh(Wkd))⩽(˜θk−1−˜θk−2)T(˜θk−1−˜θk−2)+cλh(dh+1)+cΔt3−γ(˜θk−˜θk−2)T(˜θk−˜θk−2)+cΔt2−γk−1∑j=L+1(bk−j−1−bk−j)(˜θk−˜θk−2)T(˜θj−˜θj−2)+cΔt2(‖˜θk‖+‖˜θk−2‖)(‖˜θk‖+‖˜θk−2‖)⩽(˜θk−1−˜θk−2)T(˜θk−1−˜θk−2)+cΔt3−γλh(dh+1)+cΔt3−γ(‖˜θk‖2+‖˜θk−2‖2)+cΔt3−γk−1∑j=L+1(bk−j−1−bk−j)(‖˜θk‖+‖˜θk−2‖)(‖˜θj‖+‖˜θj−2‖)+cΔt2(‖θk‖2+‖θk−2‖2+‖˜θk‖2+‖˜θk−2‖2). | (3.17) |
Summing (3.16) and (3.17) from L+2 to k (k⩽K), by (3.6), we obtain
‖θk‖2⩽λH(dH+1)+cΔt2k∑i=L‖θi‖2+cΔt3−γk∑i=L‖θi‖2+cΔt3−γλH(dH+1)+cΔt3−γk∑i=L+2k−1∑j=L+1(bi−j−1−bi−j)‖θi‖‖θj‖⩽cλH(dH+1)+cΔt3−γk∑i=L‖θi‖2+cΔt3−γk∑i=L+2k−1∑j=L+1(bi−j−1−bi−j)‖θi‖‖θj‖, | (3.18) |
‖˜θk‖2⩽λh(dh+1)+cΔt2k∑i=L(‖˜θi‖2+‖θi‖2)+cΔt3−γk∑i=L‖˜θi‖2+cΔt3−γλh(dh+1)+cΔt3−γk∑i=L+2k−1∑j=L+1(bi−j−1−bi−j)‖˜θi‖‖˜θj‖⩽cλh(dh+1)+cΔt3−γk∑i=L(‖˜θi‖2+‖θi‖2)+cΔt3−γk∑i=L+2k−1∑j=L+1(bi−j−1−bi−j)‖˜θi‖‖˜θj‖, | (3.19) |
where L+1⩽k⩽K. Thus, when Δt is sufficiently small such that cΔt3−γ⩽1/2, simplifying (3.18) and (3.19) yields
‖θk‖2⩽cλH(dH+1)+cΔt3−γk−1∑i=L‖θi‖2+cΔt3−γk−1∑i=L+2k−1∑j=L+1(bi−j−1−bi−j)‖θi‖‖θj‖, | (3.20) |
‖˜θk‖2⩽cλh(dh+1)+cΔt3−γk−1∑i=L−1(‖˜θi‖2+‖θi+1‖2)+cΔt3−γk∑i=L+2k−1∑j=L+1(bi−j−1−bi−j)‖˜θi‖‖˜θj‖, | (3.21) |
where L+1⩽k⩽K.
By Lemma 1, from (3.20) and (3.21) we get
‖Wk−Wkd‖=‖θk‖⩽c√λH(dH+1)exp(cΔt3−γ(k−L+1)+cΔt3−γk∑i=L+2k−1∑j=L+1(bi−j−1−bi−j))⩽c√λH(dH+1),L+1⩽k⩽K; | (3.22) |
‖wk−wkd‖=‖˜θk‖⩽c(λh(dh+1)+Δtk∑i=L‖θi‖)1/2exp(cΔt3−γ(k−L+1)+cΔt3−γk∑i=L+2k−1∑j=L+1(bi−j−1−bi−j))⩽c(√λh(dh+1)+√λH(dH+1)),L+1⩽k⩽K. | (3.23) |
By (3.22) and (3.23), we get
‖wkH−wkHd‖1=‖ζT(Wk−Wkd)‖1⩽‖ζ‖1‖Wk−Wkd‖⩽c√λH(dH+1),L+1⩽k⩽K, | (3.24) |
‖wkh−wkhd‖1=‖ξT(wk−wkd)‖1⩽‖ξ‖1‖wk−wkd‖⩽c(√λh(dh+1)+√λH(dH+1)),L+1⩽k⩽K. | (3.25) |
By combining Theorem 1 with (3.14), (3.15), (4.1), and (3.25), we immediately get (3.10) and (3.11), which finishes the demonstration of Theorem 3.
Remark 4. By comparison, we find that the errors of Theorem 3 have two more terms √λH(dH+1) and √λh(dh+1) than those of Theorem 1. Fortunately, the two extra terms √λH(dH+1) and √λh(dh+1) serve as suggestions for selecting the number of POD base vectors. In fact, as long as the selected dh and dH satisfy √λh(dh+1)+√λH(dH+1)⩽(Δt2+hl+1), the total errors will not be affected. A large number of numerical tests conducted in [16] demonstrate that the eigenvalue rapidly drops to 0. Generally, when dh and dH=5∼7, they are already very small and satisfy √λh(dh+1)+√λH(dH+1)⩽(Δt2+hl+1). The biggest advantage of the RDTGFE method is that it can calculate the numerical solutions at all time nodes satisfying the appointed accuracy by updating the POD base vectors and reconstructing the RDTGFE method. The specific way is that if the RDTGFE solution (wk0+1hd,uk0+1hd) at time node tk0+1 does not meet the required accuracy, but (wkhd,ukhd) at time nodes tk⩽tk0 still meet accuracy requirements, then we can retake two sets of new solution vectors to form two new matrices AH=(Wk0−L+1,Wk0−L+2,⋯,Wk0) and Ah=(wk0−L+1,wk0−L+2,⋯,wk0) and reconstruct two sets of new POD bases and a new RDTGFE method to find the RDTGFE solutions satisfying the appointed accuracy. In this way, we can obtain the RDTGFE solutions satisfying the specified accuracy at all time nodes, which is unmatched by the CTGFE method.
In this section, we employ some numerical experiments to verify the correctness of our theoretical results and demonstrate the advantage of the RDTGFE method.
To facilitate the calculation of the errors of CTGFE and RDTGFE solutions, we use the 2D NTFW equation with analytic solutions as an example. In general, there is no analytical solution to the NTFW equation. If we take
ˉΩ=[0,1]×[0,1],a(w)=(1+w),f(w)=w2,w0(x)=sin(2πx1)sin(2πx2),w1(x)=−sin(2πx1)sin(2πx2),g(x,t)=sin(2πx1)sin(2πx2)exp(−t)−sin2(2πx1)sin2(2πx2)exp(−2t)+4π2exp(−t)[cos2(2πx1)sin2(2πx2)+sin2(2πx1)cos2(2πx2)−2sin(2πx1)sin(2πx2)(1+sin(2πx1)sin(2πx2)exp(−t))]+sin(2πx1)sin(2πx2)Γ(2−γ)∫t0exp(−s)(t−s)1−γds, |
then Problem 1 has an analytical solution w(x,t)=sinx1sinx2exp(−t).
The fine grid division Jh is composed of the squares with diagonal h=√2×10−3. When l=1, in order to satisfy h=O(H1+1/l), i.e., h=O(H2), the coarse grid division JH is composed of the squares with diagonal H=4√2/√1000. When Δt=10−2−2γ3−γ and √λh(dh+1)+√λH(dH+1)=O(10−6), according to Theorems 1 and 3.2, the L2 norm errors of the CTGFE and RDTGFE solutions of the NTFW equation can theoretically achieve O(10−6).
The RDTGFE solutions can be found by the following flowchart.
(1) Experientially, calculate two sets of the first 20 (L=20) CTGFE solution vectors W1,W2,⋯, W20 and w1,w2,⋯,w20 when γ=1.5 to make up two matrices AH=(W1,W2,⋯,W20) and Ah=(w1,w2,⋯,w20).
(2) Calculate two sets of orthonormal eigenvectors ˆφϵi (1⩽i⩽20) for matrices ATϵAϵ associated with two sets of eigenvalues λϵ1⩾λϵ2⩾⋯⩾λϵ20⩾0 (ϵ=H,h).
(3) By estimation, we get that √λH7 ⩽√λH7+√λh7 ⩽3.126×10−6. Thus, we only need to extract two sets of the first six orthonormal eigenvectors ˆφϵi (1⩽i⩽6) to form two sets of POD bases Φϵ=(φϵ1,φϵ2,⋯, φϵ6) by formulas φϵi=Aϵˆφϵi/√λϵi (ϵ=H,h and 1⩽i⩽6).
(4) Substitute Φϵ (ϵ=H,h) into Problem 5 and find the RDTGFE solutions wkhd when γ=1.5, t=0.5, 1.0, 1.5, 2.0, 2.5, and 3.0, as shown in Figures 1(a)–6(a).
To show that the RDTGFE method precedes the CTGFE method, we also calculate the CTGFE solutions wkh of the NTFW equation when γ=1.5, t=0.5, 1.0, 1.5, 2.0, 2.5, and 3.0, as shown in Figures 1(b)–6(b). In practical application, it is unnecessary to find the CTGFE solutions, which may be replaced by the observations on the coarse grid JH and fine grid Jh and to find the RDTGFE solutions by the above four steps.
To expediently compare the difference between the RDTGFE solutions and the CTGFE solutions with the analytical solutions, we also provide the analytical solutions w(x,t) when γ=1.5, t=0.5, 1.0, 1.5, 2.0, 2.5, and 3.0, in Figures 1(c) to 6(c).
By comparing each set of graphs in Figures 1–6, it can be easy to see that when γ=1.5, t=0.5, 1.0, 1.5, 2.0, 2.5, and 3.0, the RDTGFE solutions are very close to the analytical solutions, but the CTGFE solutions deviate from the analytical solutions. This deviation is caused by the accumulation of truncation errors. Because the CTGFE method has (103+106) unknowns per time step, while the RDTGFE method has only 2×6 unknowns per time step, the RDTGFE method can greatly reduce the unknowns and is obviously superior to the CTGFE method.
To truly showcase the benefits of the RDTGFE method, we record, when γ=1.5, t=0.5, 1.0, 1.5, 2.0, 2.5, and 3.0, the CPU running time for finding the CTGFE and RDTGFE solutions by using MATLAB R2024a software on a laptop and their errors under the L2 norm, shown in Table 1.
t | TGFE solutions errors | RDTGFE solutions errors | TGFE method | RDTGFE method |
‖w(tk)−wkh‖0 | ‖w(tk)−wkhd‖0 | CPU Runtime | CPU Runtime | |
0.5 | 1.3153×10−6 | 3.4352×10−6 | 1213.262 s | 24.265 s |
1.0 | 3.4367×10−6 | 3.6674×10−6 | 2426.523 s | 48.531 s |
1.5 | 5.5581×10−6 | 3.8985×10−6 | 4853.051 s | 97.061 s |
2.0 | 7.6795×10−6 | 4.1298×10−6 | 9706.103 s | 194.121 s |
2.5 | 8.7881×10−6 | 4.3586×10−6 | 19412.216 s | 388.244 s |
3.0 | 9.9652×10−6 | 4.5172×10−6 | 38824.432 s | 776.489 s |
The data in Table 1 show that when γ=1.5, t=0.5, 1.0, 1.5, 2.0, 2.5, and 3.0, the numerical errors of CTGFE and RDTGFE solutions achieve O(10−6), which matches with the obtained theoretical errors, but the CPU runtime for finding CTGFE solutions is almost fifty times as long as that for finding RDTGFE solutions. Hence, the RDTGFE method is far superior to the CTGFE method and the RDTGFE method is very valid to find the numerical solutions of the NTFW equation.
In this paper, we have created a new RDTGFE method of the NTFW equation, and have strictly proved the existence, stability, and error estimates of the RDTGFE solutions, theoretically. We have also employed some numerical experiments to verify the correctness of our theoretical results and demonstrate the superiorities of the RDTGFE method. The RDTGFE method of the NTFW equation is first proposed in this paper, which is completely different from the existing methods, including those in [23,24,25,26,27]. Therefore, they are completely original.
Although we have developed the RDTGFE method only for the NTFW equation, the method and ideas in this paper can be extended to more complicated unsteady nonlinear PDEs, for example, the nonlinear Schrödinger equation and Cahn-Hilliard equation, even to real engineering nonlinear problems. Hence, it has a wide range of applications.
Liang He: Conceptualization, Investigation, Methodology, Validation, Writing-original draft, Formal analysis; Yihui Sun: Conceptualization, Methodology, Formal analysis, Writing-review and editing; Zhenglong Chen: Conceptualization, Methodology, Formal analysis, Writing-review and editing; Fei Teng: Validation, Visualization, Writing-review and editing; Chao Shen: Inspection, Writing-review and editing; Zhendong Luo: Conceptualization, Investigation, Methodology, Formal analysis, Writing-original draft, Writing-review, and editing. All authors have read and approved the final version of the manuscript for publication.
The authors declare that they have not used Artificial Intelligence (AI) tools in the creation of this article.
This work is jointly supported by the Natural Science Foundation of Changsha City (No. kq2208085), the Guangxi Key Laboratory of Special Engineering Equipment and Control, Guilin University of Aerospace Technology (No. 2411KFYB03), and the National Natural Science Foundation of China (No. 11671106).
The authors declare that they have no conflicts of interest to report regarding the present study.
[1] | C. Cattani, H. M. Srivastava, X. J. Yang, Fractional dynamics, Berlin: Emerging Science Publishers, 2015. |
[2] | R. Hilfer, Applications of fractional calculus in physics, Singapore: World Scientific, 2000. |
[3] | R. L. Magin, Fractional calculus in bioengineering, Danbury: Begell House Publishers, 2006. |
[4] |
H. Ding, A high-order numerical algorithm for two-dimensional time-space tempered fractional diffusion-wave equation, Appl. Numer. Math., 135 (2019), 30–46. https://doi.org/10.1016/j.apnum.2018.08.005 doi: 10.1016/j.apnum.2018.08.005
![]() |
[5] |
R. Du, Y. Yan, Z. Liang, A high-order scheme to approximate the Caputo fractional derivative and its application to solve the fractional diffusion wave equation, J. Comput. Phys., 376 (2019), 1312–1330. https://doi.org/10.1016/j.jcp.2018.10.011 doi: 10.1016/j.jcp.2018.10.011
![]() |
[6] |
Z. Xing, L. Wen, Numerical analysis and fast implementation of a fourth-order difference scheme for two-dimensional space-fractional diffusion equations, Appl. Math. Comput., 346 (2019), 155–166. https://doi.org/10.1016/j.amc.2018.10.057 doi: 10.1016/j.amc.2018.10.057
![]() |
[7] |
Z. Luo, H. Wang, A highly efficient reduced-order extrapolated finite difference algorithm for time-space tempered fractional diffusion-wave equation, Appl. Math. Lett., 102 (2020), 106090. https://doi.org/10.1016/j.aml.2019.106090 doi: 10.1016/j.aml.2019.106090
![]() |
[8] |
Y. Zhou, Z. Luo, An optimized Crank-Nicolson finite difference extrapolating model for the fractional-order parabolic-type sine-Gordon equations, Adv. Differ. Equ., 2019 (2019), 1. https://doi.org/10.1186/s13662-018-1939-6 doi: 10.1186/s13662-018-1939-6
![]() |
[9] |
C. Çelik, M. Duman, Finite element method for a symmetric tempered fractional diffusion equation, Appl. Numer. Math., 120 (2017), 270–286. https://doi.org/10.1016/j.apnum.2017.05.012 doi: 10.1016/j.apnum.2017.05.012
![]() |
[10] |
J. Lin, J. Bai, S. Reutskiy, J. Lu, A novel RBF-based meshless method for solving time-fractional transport equations in 2D and 3D arbitrary domains, Eng. Comput., 39 (2023), 1905–1922. https://doi.org/10.1007/s00366-022-01601-0 doi: 10.1007/s00366-022-01601-0
![]() |
[11] |
K. Li, Z. Tan, A two-grid fully discrete Galerkin finite element approximation for fully nonlinear time-fractional wave equations, Nonlinear Dyn., 111 (2023), 8497–8521. https://doi.org/10.1007/s11071-023-08265-5 doi: 10.1007/s11071-023-08265-5
![]() |
[12] |
J. Xu, A novel two-grid method for semilinear elliptic equations, SIAM J. Sci. Comput., 15 (1994), 231–237. https://doi.org/10.1137/0915016 doi: 10.1137/0915016
![]() |
[13] |
D. Shi, Q. Liu, An efficient nonconforming finite element two-grid method for Allen-Cahn equation, Appl. Numer. Math., 98 (2019), 374–380. https://doi.org/10.1016/j.aml.2019.06.037 doi: 10.1016/j.aml.2019.06.037
![]() |
[14] |
D. Shi, R. Wang, Unconditional superconvergence analysis of a two-grid finite element method for nonlinear wave equations, Appl. Numer. Math., 150 (2020), 38–50. https://doi.org/10.1016/j.apnum.2019.09.012 doi: 10.1016/j.apnum.2019.09.012
![]() |
[15] |
Y. Liu, Y. Du, H. Li, J. Li, S. He, A two-grid mixed finite element method for a nonlinear fourth-order reaction-diffusion problem with time-fractional derivative, Comput. Math. Appl., 70 (2015), 2474–2492. https://doi.org/10.1016/j.camwa.2015.09.012 doi: 10.1016/j.camwa.2015.09.012
![]() |
[16] | Z. Luo, Finite element and reduced dimension methods for partial differential equations, Beijing: Springer and Science Press of China, 2024. |
[17] |
F. Teng, Z. Luo, A natural boundary element reduced-dimension model for uniform high-voltage transmission line problem in an unbounded outer domain, Comput. Appl. Math., 43 (2024), 106. https://doi.org/10.1007/s40314-024-02617-6 doi: 10.1007/s40314-024-02617-6
![]() |
[18] | Z. Luo, G. Chen, Proper orthogonal decomposition methods for partial differential equations, London: Academic Press of Elsevier, 2019. |
[19] |
H. Li, Z. Song, A reduced-order finite element method based on proper orthogonal decomposition for the Allen-Cahn model, J. Math. Anal. Appl., 500 (2021), 125103. https://doi.org/10.1016/j.jmaa.2021.125103 doi: 10.1016/j.jmaa.2021.125103
![]() |
[20] |
A. K. Alekseev, D. A. Bistrian, A. E. Bondarev, I. M. Navon, On linear and nonlinear aspects of dynamic mode decomposition, Int. J. Numer. Meth. Fluids, 82 (2016), 348–371. https://doi.org/10.1002/fld.4221 doi: 10.1002/fld.4221
![]() |
[21] |
J. Du, I. M. Navon, J. Zhu, F. Fang, A. K. Alekseev, Reduced order modeling based on POD of a parabolized Navier-Stokes equations model Ⅱ: Trust region POD 4D VAR data assimilation, Comput. Math. Appl., 65 (2013), 380–394. https://doi.org/10.1016/j.camwa.2012.06.001 doi: 10.1016/j.camwa.2012.06.001
![]() |
[22] |
K. Li, T. Huang, L. Li, S. Lanteri, L. Xu, B. Li, A reduced-order discontinuous Galerkin method based on POD for electromagnetic simulation, IEEE Trans. Antennas Propag., 66 (2018), 242–254. https://doi.org/10.1109/TAP.2017.2768562 doi: 10.1109/TAP.2017.2768562
![]() |
[23] |
Y. Li, H. Li, Y. Zeng, Z. Luo, A preserving accuracy two-grid reduced-dimensional Crank-Nicolson mixed finite element method for nonlinear wave equation, Appl. Numer. Math., 202 (2024), 1–20. https://doi.org/10.1016/j.apnum.2024.04.010 doi: 10.1016/j.apnum.2024.04.010
![]() |
[24] |
L. Jing, F. Teng, M. Feng, H. Li, J. Yang, Z. Luo, A novel dimension reduction model based on POD and two-grid Crank-Nicolson mixed finite element methods for 3D nonlinear elastodynamic sin-Gordon problem, Commun. Nonlinear Sci. Numer. Simul., 140 (2025), 108409. https://doi.org/10.1016/j.cnsns.2024.108409 doi: 10.1016/j.cnsns.2024.108409
![]() |
[25] |
X. Hou, Y. Li, Q. Deng, Z. Luo, A novel dimensionality reduction iterative method for the unknown coefficient vectors in TGFECN solutions of unsaturated soil water flow problem, J. Math. Anal. Appl., 543 (2025), 128930. https://doi.org/10.1016/j.jmaa.2024.128930 doi: 10.1016/j.jmaa.2024.128930
![]() |
[26] |
Y. Li, F. Teng, Y. Zeng, Z. Luo, Two-grid dimension reduction method of Crank-Nicolson mixed finite element solution coefficient vectors for the fourth-order extended Fisher-Kolmogorov equation, J. Math. Anal. Appl., 538 (2024), 128168. https://doi.org/10.1016/j.jmaa.2024.128168 doi: 10.1016/j.jmaa.2024.128168
![]() |
[27] |
H. Li, Y. Li, Y. Zeng, Z. Luo, A reduced-dimension extrapolation two-grid Crank-Nicolson finite element method of unknown solution coefficient vectors for spatial fractional nonlinear Allen-Cahn equations, Comput. Math. Appl., 167 (2024), 110–122. https://doi.org/10.1016/j.camwa.2024.05.007 doi: 10.1016/j.camwa.2024.05.007
![]() |
[28] |
H. Li, R. Yang, Analysis of two spectral Galerkin approximation schemes for solving the perturbed FitzHugh-Nagumo neuron model, Comput. Math. Appl., 143 (2023), 1–9. https://doi.org/10.1016/j.camwa.2023.04.033 doi: 10.1016/j.camwa.2023.04.033
![]() |
[29] |
Z. Song, D. Li, D. Wang, H. Li, A modified Crank Nicolson finite difference method preserving maximum-principle for the phase-field model, J. Math. Anal. Appl., 526 (2023), 127271. https://doi.org/10.1016/j.jmaa.2023.127271 doi: 10.1016/j.jmaa.2023.127271
![]() |
[30] | G. Zhang, Y. Lin, Notes on functional analysis, in Chinese, Beijing: Peking University Press, 2011. |
t | TGFE solutions errors | RDTGFE solutions errors | TGFE method | RDTGFE method |
‖w(tk)−wkh‖0 | ‖w(tk)−wkhd‖0 | CPU Runtime | CPU Runtime | |
0.5 | 1.3153×10−6 | 3.4352×10−6 | 1213.262 s | 24.265 s |
1.0 | 3.4367×10−6 | 3.6674×10−6 | 2426.523 s | 48.531 s |
1.5 | 5.5581×10−6 | 3.8985×10−6 | 4853.051 s | 97.061 s |
2.0 | 7.6795×10−6 | 4.1298×10−6 | 9706.103 s | 194.121 s |
2.5 | 8.7881×10−6 | 4.3586×10−6 | 19412.216 s | 388.244 s |
3.0 | 9.9652×10−6 | 4.5172×10−6 | 38824.432 s | 776.489 s |
t | TGFE solutions errors | RDTGFE solutions errors | TGFE method | RDTGFE method |
‖w(tk)−wkh‖0 | ‖w(tk)−wkhd‖0 | CPU Runtime | CPU Runtime | |
0.5 | 1.3153×10−6 | 3.4352×10−6 | 1213.262 s | 24.265 s |
1.0 | 3.4367×10−6 | 3.6674×10−6 | 2426.523 s | 48.531 s |
1.5 | 5.5581×10−6 | 3.8985×10−6 | 4853.051 s | 97.061 s |
2.0 | 7.6795×10−6 | 4.1298×10−6 | 9706.103 s | 194.121 s |
2.5 | 8.7881×10−6 | 4.3586×10−6 | 19412.216 s | 388.244 s |
3.0 | 9.9652×10−6 | 4.5172×10−6 | 38824.432 s | 776.489 s |