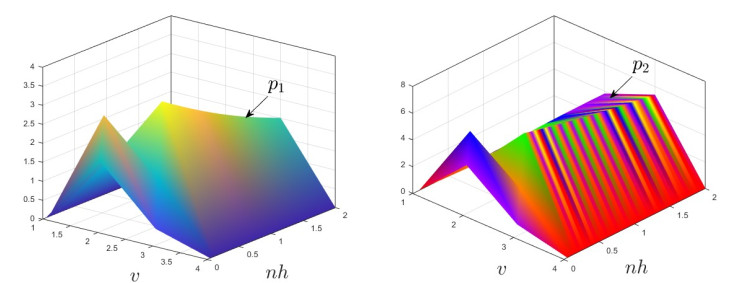
This paper presents an investigation into the phenomenon of global mean-squared finite-time synchronization within the context of two distinct schemes: The asymptotic and exponential forms. The subject matter encompasses space-time discrete stochastic fuzzy genetic regulatory networks, wherein Dirichlet controlled boundary values and time delays are taken into account. The findings presented therein pertain to global mean-squared finite-time synchronization for the aforementioned discrete stochastic fuzzy networks, which incorporate the Lyapunov-Krasovskii functional with a double sum representing the delay-dependent components. In addition, this study demonstrates that improved global mean-squared finite-time synchronization of space-time discrete stochastic fuzzy genetic regulatory networks with boundary controls can be achieved by optimizing the small diffusion intensities, the small fuzzy MIN and MAX parameters, and the large degradation rates of mRNA and proteins. It was unexpected to discover that the sizes of the time lags exert a direct influence on the value of the convergent rate of global mean-squared finite-time exponential synchronization of the networks. This paper presents a framework for exploring the issues of global mean-squared finite-time asymptotic and exponential synchronization for space-time discrete stochastic fuzzy genetic regulatory networks via the Dirichlet controlled boundaries. To conclude, an illustrative example is provided to demonstrate the efficacy of the aforementioned method.
Citation: Dong Pan, Huizhen Qu. Finite-time boundary synchronization of space-time discretized stochastic fuzzy genetic regulatory networks with time delays[J]. AIMS Mathematics, 2025, 10(2): 2163-2190. doi: 10.3934/math.2025101
[1] | Chengqiang Wang, Xiangqing Zhao, Yang Wang . Finite-time stochastic synchronization of fuzzy bi-directional associative memory neural networks with Markovian switching and mixed time delays via intermittent quantized control. AIMS Mathematics, 2023, 8(2): 4098-4125. doi: 10.3934/math.2023204 |
[2] | Nayika Samorn, Kanit Mukdasai, Issaraporn Khonchaiyaphum . Analysis of finite-time stability in genetic regulatory networks with interval time-varying delays and leakage delay effects. AIMS Mathematics, 2024, 9(9): 25028-25048. doi: 10.3934/math.20241220 |
[3] | Jingyang Ran, Tiecheng Zhang . Fixed-time synchronization control of fuzzy inertial neural networks with mismatched parameters and structures. AIMS Mathematics, 2024, 9(11): 31721-31739. doi: 10.3934/math.20241525 |
[4] | Zhengqi Zhang, Huaiqin Wu . Cluster synchronization in finite/fixed time for semi-Markovian switching T-S fuzzy complex dynamical networks with discontinuous dynamic nodes. AIMS Mathematics, 2022, 7(7): 11942-11971. doi: 10.3934/math.2022666 |
[5] | Ankit Kumar, Subir Das, Vijay K. Yadav, Rajeev, Jinde Cao, Chuangxia Huang . Synchronizations of fuzzy cellular neural networks with proportional time-delay. AIMS Mathematics, 2021, 6(10): 10620-10641. doi: 10.3934/math.2021617 |
[6] | Ailing Li, Xinlu Ye . Finite-time anti-synchronization for delayed inertial neural networks via the fractional and polynomial controllers of time variable. AIMS Mathematics, 2021, 6(8): 8173-8190. doi: 10.3934/math.2021473 |
[7] | Zhangir Nuriyev, Alfarabi Issakhanov, Jürgen Kurths, Ardak Kashkynbayev . Finite-time synchronization for fuzzy shunting inhibitory cellular neural networks. AIMS Mathematics, 2024, 9(5): 12751-12777. doi: 10.3934/math.2024623 |
[8] | Zhifeng Lu, Fei Wang, Yujuan Tian, Yaping Li . Lag synchronization of complex-valued interval neural networks via distributed delayed impulsive control. AIMS Mathematics, 2023, 8(3): 5502-5521. doi: 10.3934/math.2023277 |
[9] | Ting Yang, Li Cao, Wanli Zhang . Practical generalized finite-time synchronization of duplex networks with quantized and delayed couplings via intermittent control. AIMS Mathematics, 2024, 9(8): 20350-20366. doi: 10.3934/math.2024990 |
[10] | Shuang Li, Xiao-mei Wang, Hong-ying Qin, Shou-ming Zhong . Synchronization criteria for neutral-type quaternion-valued neural networks with mixed delays. AIMS Mathematics, 2021, 6(8): 8044-8063. doi: 10.3934/math.2021467 |
This paper presents an investigation into the phenomenon of global mean-squared finite-time synchronization within the context of two distinct schemes: The asymptotic and exponential forms. The subject matter encompasses space-time discrete stochastic fuzzy genetic regulatory networks, wherein Dirichlet controlled boundary values and time delays are taken into account. The findings presented therein pertain to global mean-squared finite-time synchronization for the aforementioned discrete stochastic fuzzy networks, which incorporate the Lyapunov-Krasovskii functional with a double sum representing the delay-dependent components. In addition, this study demonstrates that improved global mean-squared finite-time synchronization of space-time discrete stochastic fuzzy genetic regulatory networks with boundary controls can be achieved by optimizing the small diffusion intensities, the small fuzzy MIN and MAX parameters, and the large degradation rates of mRNA and proteins. It was unexpected to discover that the sizes of the time lags exert a direct influence on the value of the convergent rate of global mean-squared finite-time exponential synchronization of the networks. This paper presents a framework for exploring the issues of global mean-squared finite-time asymptotic and exponential synchronization for space-time discrete stochastic fuzzy genetic regulatory networks via the Dirichlet controlled boundaries. To conclude, an illustrative example is provided to demonstrate the efficacy of the aforementioned method.
Genetic regulatory networks (GRNs) [1,2,3] describe the complex networks of molecular regulators that control the expression of genes in a cell. They are key in understanding how genes interact with one another and how cells respond to internal and external stimuli. A typical GRN consists of genes, transcription factors, proteins, RNA molecules, and other regulatory components that work together to regulate gene expression, turning genes "on" or "of" in response to various signals. With the advent of high-throughput technologies like DNA microarrays, RNA sequencing (RNA-seq), and chromatin immunoprecipitation sequencing (ChIP-seq), scientists can now measure gene expression levels and map the interactions between regulatory proteins and DNA. This has led to the development of computational models and algorithms that simulate and predict GRN behavior. GRNs have several key applications, particularly in areas like biotechnology, medicine, and evolutionary biology. In particular, GRNs are foundational to synthetic biology, where scientists engineer or design new regulatory networks for specific purposes, such as producing therapeutic proteins, biofuels, or materials. Additionally, the misregulation of gene networks is implicated in various diseases, including cancer, neurodegenerative diseases, and autoimmune disorders. GRNs can help identify which genes are misregulated in these diseases. GRNs are a critical framework for understanding the regulation of gene expression and the interplay between various molecular components in cells. Their study has broad applications across biology and medicine [4], contributing to breakthroughs in disease treatment [5], agricultural improvements [6], synthetic biology [7,8,9], and personalized medicine [10,11].
A reaction-diffusion network [12,13,14] is a theoretical model that is used to describe the change in concentration of a substance or substances distributed in space. These changes occur due to two distinct processes: Local chemical reactions, which create or destroy the substances in question, and diffusion, whereby the substances in question spread out in space [15,16,17]. These networks play a pivotal role in elucidating the genesis of a multitude of pattern formation phenomena in biological, chemical, and physical systems. Reaction-diffusion networks provide an effective conceptual tool for elucidating the mechanisms by which intricate spatio-temporal patterns arise in natural and synthetic systems. The interdisciplinary applications of these networks make them an invaluable tool in theoretical and applied sciences alike, offering insights into the processes underlying pattern formation and spatial organization. Discrete-time and discrete-space systems (also referred to as DTDSSs) offer a robust framework for the modeling and comprehension of a plethora of dynamic processes across the realms of science and engineering. By decomposing time and space into discrete units, these models streamline the examination and emulation of intricate systems, facilitating insights that are challenging to discern from their continuous counterparts. The applications of these techniques are diverse, encompassing fields as varied as digital signal processing, control systems, ecology, epidemiology, and material science. The state of the system is updated at each discrete time step in accordance with the interactions within the spatial grid, as outlined in reports [9,18,19]. Furthermore, it is notable that while previous researches [13,14] have explored GRNs with reaction-diffusions, there is a dearth of literature examining diffusive GRNs in space-time discretized systems.
Fuzzy systems are a branch of artificial intelligence and control theory that uses fuzzy logic, which is an extension of classical logic. Unlike traditional binary logic (true or false), fuzzy logic allows for reasoning with degrees of truth or uncertainty. This concept was introduced in 1965 by Lotfi A. Zadeh, a professor at the University of California, Berkeley, who recognized the need for a system capable of handling ambiguous or imprecise information. In the real world, most phenomena do not operate strictly within the boundaries of "yes" or "no" but rather in a spectrum of possibilities. Fuzzy logic was created to address such situations. The integration of fuzzy systems into neural networks and genetic algorithms further expanded their capabilities, leading to the development of neuro-fuzzy systems and fuzzy evolutionary systems that combine fuzzy logic with machine learning for enhanced decision-making [10,20,21,22,23]. On the other hand, stochastic systems refer to systems that are inherently influenced by randomness or uncertainty. Unlike deterministic systems, which produce predictable outcomes based on initial conditions and precise equations, stochastic systems exhibit behavior that cannot be predicted with absolute certainty due to the presence of random variables or noise. This randomness can come from external factors (e.g., environmental fluctuations) or internal processes (e.g., thermal noise in physical systems or random mutations in biological processes). As is well-known, stochastic systems are applied in a wide range of fields, from engineering and finance to biology and climate science [24,25,26].
Synchronization [27,28,29,30] in dynamical systems refers to the process where the states (positions, velocities, etc.) of different systems converge and evolve identically over time. It can occur in various forms, e.g., complete synchronization, phase synchronization, and lag synchronization. In finite-time synchronization (FTS), the system reaches synchronization in a definite time, after which the system states remain synchronized [31]. Unlike asymptotic synchronization, this type of synchronization guarantees that the systems will be synchronized within a finite, predetermined time. It refers to a process where two or more dynamical systems, which may start from different initial conditions, achieve synchronization (i.e., their states become identical or follow the same trajectory) in a finite amount of time. This is different from asymptotic synchronization, where systems approach synchronization gradually over an infinite time horizon. Finite-time synchronization ensures that the systems synchronize within a fixed, finite period, regardless of their initial differences. FTS has applications across multiple fields, particularly in systems that require fast and robust synchronization in a specific time frame, such as communication systems, robotics, power systems, and biological networks. In GRNs, the expression of certain genes needs to be synchronized for a cell to maintain its functional state or transition between states, such as during development or differentiation. FTS allows the control of gene expression so that key genes are activated in unison, within a specific time frame, ensuring proper cellular function [7,8]. While previous works [7,8] have investigated various forms of GRNs' FTS, there is a noticeable dearth of research on the FTS topic for discrete GRNs within a space-time setting. It is clear that there is a significant research priority demanding investigation into the FTS of space-time discrete GRNs, which has not yet been adequately addressed within the existing literature.
The aforementioned discussions provide a rationale for our investigation into the issue of the FTS for space-time discrete stochastic fuzzy GRNs with time-varying delays via the boundary controls (BLs). The establishment of a Lyapunov-Krasovskii functional, comprising both delay-free and delay components, allows the FTS of space-time discrete stochastic fuzzy GRNs to be explored in depth, both asymptotically and exponentially. Summarily, the topic of finite-time synchronization of space-time stochastic GRNs with delays include the following difficulties: 1) Space-time variability requires advanced mathematical tools; 2) stochastic noise from biological and environmental factors complicates modeling and analysis; and 3) time delays destabilize systems and demand sophisticated stability criteria. The following part will present the primary findings of this investigation.
1) A framework of discrete stochastic fuzzy GRNs within a space-time context has been developed, which significantly extends the scope of reaction-diffusion GRNs as previously outlined [13,14]. Besides, this structure also complements recent studies on space-time discrete models [15,16].
2) By designing the controls at the Dirichlet boundaries of the GRNs, this article discusses the issue of the FTS for the discrete-time and discrete-space GRNs for the first time, which improves the feedback controls in previous works [29,30]. Additionally, in [18], authors studied the networks with the controls at the Neumann boundaries, but this study shows that the controls can be designed at the Dirichlet boundaries.
3) This shows a significant advance in the field of space-time discrete fuzzy GRNs' FTS, which represents a significant step forward in the controlling fields of biomedical research and medical practice.
The following part outlines the structure of this paper. Section 2 presents the model of discrete stochastic fuzzy GRNs within a space-time context, the concepts of the FTS, and a number of useful lemmas via the designation of the feedback boundary controls in the GRNs. Section 3 discusses the asymptotic and exponential FTS of the time-space discrete stochastic fuzzy GRNs with orderly time-varying delays. Section 4 provides illustrative numerical examples that substantiate the reliability of the main results. The final section of this paper is Section 5, in which the conclusions of this study are presented.
Denote R:=(−∞,∞), R0:=[0,∞), Z0:={0,1,2,…}, Z+:=Z0∖{0}, Z=Z0∪(−Z+). I represents some suitable dimensional identical matrix. For N-order matrix P, (P)⋁ and (P)⋀ denote a N-dimensional column vector whose element of the ith row is the number computed by making the operations ⋁ and ⋀, respectively, to the elements in the ith row of P, i=1,2,…,N. Also, denote
Διp[v]n=p[v+1]n−p[v]nι,Δ2ιp[v]n=Δι(Διp[v]n)=p[v+2]n−2p[v+1]n+p[v]nι2(finitedifference), |
Δ2c,ιp[v]n=Δι(Διp[v−1]n)=p[v+1]n−2p[v]n+p[v−1]nι2(centralfinitedifference), |
where z=p[v]n:Z2→RM, (v,k)∈Z2, ι>0.
This article mainly discusses the following stochastic fuzzy GRNs (SF-GRNs) with the space-time discrete forms:
{p[v]i,n+1=e−aihp[v]i,n+1−e−aihai[θiΔ2c,ιp[v]i,n+N∑j=1rijgj(m[v]j,τn)+N⋁j=1ξijgj(m[v]j,τn)+N⋀j=1ζijgj(m[v]j,τn)+N∑j=1sijσj(m[v]j,ηn)Δhωj,n],m[v]i,n+1=e−bihm[v]i,n+1−e−bihbi[ϑiΔ2c,ιm[v]i,n+cip[v]i,δn], | (2.1) |
where (v,n)∈(0,£)Z×Z0, i=1,2,…,N; pi and mi signify the concentrations of mRNA and proteins of the ith node; θi and ϑi denote the diffusion rates; ai and bi represent degradation rates of the mRNA and protein, respectively; gj,σj are the activation and noise functions described by the Hill functions with Hill constants ϰgj,ϰσj, respectively; that is,
gj(s)=sϰgj1+sϰgj,σj(s)=sϰσj1+sϰσj; |
rij,sij denote the couplings (see [32]), which can be described by
rij={˜rij,if transcription factor j is an activator of gene i;0,if there is no link between node j and gene i;−˜rij,if transcription factor j is a repressor of gene i, |
sij={˜sij,if transcription factor j is an activator of gene i;0,if there is no link between node j and gene i;−˜sij,if transcription factor j is a repressor of gene i, |
where ˜rij,˜sij express basal transcriptional rate; ⋁ and ⋀ represent the operations of fuzzy OR and fuzzy AND, respectively; ξij, ζij stand for the state parameters of fuzzy MIN feedback and fuzzy MAX feedback, respectively; Δhωj,n=ωj(nh+h)−ωj(nh)h, ωj denotes the one-dimensional real-valued Brownian motion on certain probability space satisfying E(ω2j)=1 and E(ωiωj)=0 for i≠j; i,j=1,2,…,N; n−τn,n−ηn,n−δn∈[0,∞) for all n∈Z0 are supposed to be included in set Z in the sequel. In SF-GRNs (2.1), we employ several highly effective, discrete methodologies that include the Euler time difference and the central finite space difference. For further information, please refer to the relevant papers [18,19].
In SF-GRNs (2.1), referring to articles [7,8], the two assumptions below can be provided.
(f1) The Hill constants ϰgj,ϰσj belong to [1,+∞).
(f2) τ0≤n−τn≤τ∞<∞, η0≤n−ηn≤η∞<∞, δ0≤n−δn≤δ∞<∞, ∀n∈Z0.
In addition, SF-GRNs (2.1) admit the initial and boundary conditions as follows
{p[v]i,s=φ[v]i,s,m[v]i,s=˜φ[v]i,s,∀(v,s)∈[0,£]Z×[−τ⋄,0]Z;p[0]i,n=p[£]i,n=0=m[0]i,n=m[£]i,n,∀n∈Z0, | (2.2) |
where τ⋄=max{τ∞,η∞,δ∞}, i=1,2,…,N.
Owing to assumption (f1), we can easily verify that the functions gj,σj meet Lipschitz conditions. In fact, it is not loss of generality to assume that x,y are nonnegative. By the mean valued theorem, it gives
|gj(x)−gj(y)|=|xϰgj1+xϰgj−yϰgj1+yϰgj|=ϰgjξϰgj−1(1+ξϰgj)2|x−y|(ξ is between x and y)=ϰgj(1+ξϰgj)2ϰgjξϰgj−1(1+ξϰgj)2−2ϰgj|x−y|≤ϰgj(1+0)2ϰgjξϰgj−122−2ϰgjξϰgj−1|x−y|=22ϰgj−2ϰgj|x−y|, |
similarly, |σj(x)−σj(y)|≤22ϰσj−2ϰσj|x−y|, where x,y∈R,j=1,2,…,N.
Considering SF-GRNs (2.1) as the master system, the corresponding slave system is described as
{q[v]i,n+1=e−aihq[v]i,n+1−e−aihai[θiΔ2c,ιq[v]i,n+N∑j=1rijgj(t[v]j,τn)+N⋁j=1ξijgj(t[v]j,τn)+N⋀j=1ζijgj(t[v]j,τn)+N∑j=1sijσj(t[v]j,ηn)Δhωj,n],t[v]i,n+1=e−biht[v]i,n+1−e−bihbi[ϑiΔ2c,ιt[v]i,n+ciq[v]i,δn], | (2.3) |
where (v,n)∈(0,£)Z×Z0, i=1,2,…,N. SF-GRNs (2.3) admit initial and boundary conditions with the control inputs, which are depicted by
{q[v]i,s=ϕ[v]i,s,t[v]i,s=˜ϕ[v]i,s,∀(v,s)∈[0,£]Z×[−τ⋄,0]Z;q[0]i,n=0=t[0]i,n,q[£]i,n=αi,n,t[£]i,n=βi,n,∀n∈Z0, | (2.4) |
where αi,n,βi,n denote the boundary control inputs in the ith node at time n, i=1,2,…,N.
Construct synchronized networks by setting ui=qi−pi,ρi=ti−mi, which are governed by the partial difference equations below
{u[v]i,n+1=e−aihu[v]i,n+1−e−aihai{θiΔ2c,ιu[v]i,n+N∑j=1rij˜gj(ρ[v]j,τn)+[N⋁j=1ξijgj(t[v]j,τn)−N⋁j=1ξijgj(m[v]j,τn)]+[N⋀j=1ζijgj(t[v]j,τn)−N⋀j=1ζijgj(m[v]j,τn)]+N∑j=1sij˜σj(ρ[v]j,ηn)Δhωj,n},ρ[v]i,n+1=e−bihρ[v]i,n+1−e−bihbi[ϑiΔ2c,ιρ[v]i,n+ciu[v]i,δn], | (2.5) |
where (v,n)∈(0,£)Z×Z0, ˜gj(ρj)=gj(qj)−gj(pj), ˜σj(ρj)=σj(qj)−σj(pj), i,j=1,2,…,N.
Via the matrix analysis, the scalar error system (2.5) can be transformed into the vector scheme below
{u[v]n+1=e−Ahu[v]n+I−e−AhA[ΘΔ2c,ιu[v]n+RG(ρ[v]τn)+G⋁(ρ[v]τn)+G⋀(ρ[v]τn)+SσωΔ(ρ[v]ηn)],ρ[v]n+1=e−Bhρ[v]n+I−e−BhB[ΦΔ2c,ιρ[v]n+Cu[v]δn], | (2.6) |
where (v,n)∈(0,£)Z×Z0, u=(u1,…,uN)T, ρ=(ρ1,…,ρN)T, A=diag(a1,…,aN)T, B=diag(b1,…,bN)T, C=diag(c1,…,cN), R=[rij]i,j=1,2,…,N, Θ=(θ1,…,θN)T, Φ=(ϑ1,…,ϑN)T, S=[sij]i,j=1,2,…,N, G(ρ)=(˜g1(ρ1),…,˜gN(ρN))T,
G⋁(ρ)=[N⋁j=1ξ1jgj(t[v]j)−N⋁j=1ξ1jgj(m[v]j)N⋁j=1ξ2jgj(t[v]j)−N⋁j=1ξ2jgj(m[v]j)⋮N⋁j=1ξNjgj(t[v]j)−N⋁j=1ξNjgj(m[v]j)],G⋀(ρ)=[N⋀j=1ζ1jgj(t[v]j)−N⋀j=1ζ1jgj(m[v]j)N⋀j=1ζ2jgj(t[v]j)−N⋀j=1ζ2jgj(m[v]j)⋮N⋀j=1ζNjgj(t[v]j)−N⋀j=1ζNjgj(m[v]j)], |
σωΔ(ρ)=[˜σ1(ρ1)Δhω1˜σ2(ρ2)Δhω2⋮˜σN(ρN)ΔhωN]. |
Define α=(α1,…,αN)T, β=(β1,…,βN)T. From initial and boundary conditions (2.2) and (2.4), the initial and boundary conditions of the error system (2.6) are given by
{u[v]s=ψ[v]s,ρ[v]s=˜ψ[v]s,∀(v,s)∈[0,£]Z×[−τ⋄,0]Z;u[0]n=0=ρ[0]n,u[£]n=αn,ρ[£]n=βn,∀n∈Z0, | (2.7) |
where ψ=(ϕ1−φ1,…,ϕN−φN)T, ˜ψ=(˜ϕ1−˜φ1,…,˜ϕN−˜φN)T.
Definition 2.1. (FTS) The master-slave networks (2.1) and (2.3) are called exponentially mean-squared finite-time synchronized (EMS-FTS) with respect to {ϵ,ς,N,κ} if
maxs∈[−τ⋄,0]Z£∑v=0E[‖ |
induces
\sum\limits_{v = 0}^{£}\mathbb{E}\Big[\left\|{\mathbf u}_{n}^{[v]}\right\|^2 +\left\|\boldsymbol\rho_{n}^{[v]}\right\|^2\Big]\leq \{(\varsigma)e^{-\kappa n} \leq \epsilon, \quad \forall n\in(0, \mathcal{N}]_{\mathbb{Z}}, |
where 0 < \varsigma < \epsilon , \kappa > 0 and \{ is a nonnegative function. If \{\equiv0 , then the master-slave networks (2.1) and (2.3) are called asymptotically mean-squared finite-time synchronized (AMS-FTS) with respect to \{\epsilon, \varsigma, \mathcal{N}\} .
Remark 2.1. In [31,33,34], the finite-time controls of various continuous-time systems were discussed. This study considers the finite-time dynamics of the GRNs in the space-time discrete frame, which aims to complement the works of papers [31,33,34].
In the lemmas below, \Delta_\iota and \Delta^2_\iota denote the first- and second-order finite difference operators with respect to v\in [0, £]_{\mathbb{Z}} , which are displayed at the beginning of Section 2.
Lemma 2.1. [35, Theorem 25, discrete formula of integration by parts] Let \{p^{[v]}\in\mathbb{R}^m:v\in [0, £]_{\mathbb{Z}}\} be the real-valued sequence and W be a m -order matrix, then
\sum\limits_{v = 1}^{£ -1}p^{[v]T}W\Delta_\iota^2p^{[v-1]} = \frac{1}{\iota}p^{[v]T}W\Delta_\iota p^{[v-1]}\bigg|_1^{£ } -\sum\limits_{v = 1}^{£ -1}\Delta_\iota p^{[v]T}W\Delta_\iota p^{[v]}. |
Lemma 2.2. [36, Corollary 2, discrete inequalities of Wirtinger's type] The inequalities below are valid for the m -dimensional real-valued sequence \{p^{[v]}\in\mathbb{R}^m:v\in [0, £]_{\mathbb{Z}}\} and m -order positive definitive matrix W . If p^{[0]} = 0 , then
4\sin^2\frac{\pi}{2(2£+1)}\sum\limits_{v = 0}^{£}p^{[v]T}Wp^{[v]}\leq\iota^2 \sum\limits_{v = 0}^{£-1}(\Delta_\iota p^{[v]})^TW\Delta_\iota p^{[v]}\leq 4\cos^2\frac{\pi}{2£+1} \sum\limits_{v = 0}^{£}p^{[v]T}Wp^{[v]}. |
If p^{[0]} = 0 may not be valid, then
\iota^2\sum\limits_{v = 1}^{£-1}(\Delta_\iota p^{[v]})^TW\Delta_\iota p^{[v]}\leq 4\cos^2\frac{\pi}{2£} \sum\limits_{v = 1}^{£}p^{[v]T}Wp^{[v]}. |
Lemma 2.3. [37] Supposing u, v\in\mathbb{R} , then
\bigg| \bigvee\limits_{j = 1}^{N}\xi_{ij}g_{j}(u)- \bigvee\limits_{j = 1}^{N}\xi_{ij}g_{j}(v)\bigg| \leq\sum\limits_{j = 1}^{N}|\xi_{ij}||g_{j}(u)-g_{j}(v)|, |
\bigg| \bigwedge\limits_{j = 1}^{N}\zeta_{ij}g_{j}(u)- \bigwedge\limits_{j = 1}^{N}\zeta_{ij}g_{j}(v)\bigg| \leq\sum\limits_{j = 1}^{N}|\zeta_{ij}||g_{j}(u)-g_{j}(v)|, |
where i = 1, 2, \ldots, N .
The objective of this section is to achieve the AMS-FTS and EMS-FTS of SF-GRNs (2.1) and (2.3) through the integration by parts formula, the inequalities of Wirtinger's type, the designations of the boundary feedback controls in the SF-GRNs (2.3), and the establishment of the Lyapunov-Krasovskii functional involving the delayed component.
Let \tau_* = \tau_\infty-\tau_0+1 , \delta_* = \delta_\infty-\delta_0+1 , \eta_* = \eta_\infty-\eta_0+1 , A_* = \frac{I-e^{-Ah}}{A}, B_* = \frac{I-e^{-Bh}}{B}. Design the feedback controls in the boundary (2.7) by
![]() |
(3.1) |
Hereby, and
are two N -order matrices denoting the gains of the controls.
Theorem 3.1. Let conditions (f_1) and (f_2) be fulfilled. Furthermore, it holds that
(f_3) there are matrices P > 0 and Q > 0 so that
16\cos^{2} \frac{\pi }{2£}\Theta A_{*}PA_{*}\Theta+\frac{\iota^{2} }{4\sin^{2} \frac{\pi }{4£ -2} }I \leq\iota^2\mathrm{sym}(e^{-Ah}PA_{*}\Theta), |
8\cos^{2} \frac{\pi }{2£}\Phi B_{*}QB_{*}\Phi+\frac{\iota^{2} }{4\sin^{2} \frac{\pi }{4£ -2} }I \leq \iota^2\mathrm{sym}(e^{-Bh}QB_{*}\Phi); |
(f_4) there are matrices \Pi_1 > 0, \Pi_2 > 0, \Pi_3 > 0 and positive constants \pi_1, \pi_2, \pi_3, \pi_4 so that
(\pi_1+\pi_2\xi_{\infty }N^2+\pi_3\zeta_{\infty }N^2)H^2\leq \Pi_1, \quad \pi_4K^2\leq \Pi_2, |
(2+\frac{1}{\varepsilon})C^TB_{*}QB_{*}C\leq \Pi_3, \quad S^TA_{*}PA_{*}S\leq \pi_4I, |
where \varepsilon > 0 , \xi_{\infty } = \max _{i, j} \xi _{i, j}^{2} , \zeta_{\infty } = \max _{i, j} \zeta _{i, j}^{2} , H = \mathrm{diag}\big(2^{\frac{2}{\varkappa_1^g}-2} \varkappa_1^g, \dots, 2^{\frac{2}{\varkappa_N^g}-2} \varkappa_N^g\big) , K = \frac{1}{h}\mathrm{diag}\big(2^{\frac{2}{\varkappa_1^\sigma}-2} \varkappa_1^\sigma, \dots, 2^{\frac{2}{\varkappa_N^\sigma}-2} \varkappa_N^\sigma\big) ;
(f_5) there are symmetric matrices Œ_1, Œ_2 and constant \lambda_\Omega\geq0 so that
\Omega = \left( \begin{array}{ccccccc} \Omega_{11} & \frac{1}{\iota^2}Œ_1 & 0 & 0 & e^{-Ah}PA_{*}R & e^{-Ah}PA_{*} & e^{-Ah}PA_{*} \\ * & -I & 0 & 0 & 0 & 0 & 0 \\ * & * &\Omega_{33} & \frac{1}{\iota^2}Œ_2 & 0 & 0 & 0 \\ * & * & * & -I & 0 & 0 & 0 \\ * & * & * & * & \Omega_{55} & R^T A_{*}PA_{*} & R^T A_{*}PA_{*} \\ * & * & * & * & * & \Omega_{66} & A_{*}PA_{*} \\ * & * & * & * & * & * & \Omega_{77} \\ \end{array} \right) < 0, |
where \Omega_{11} = -P+e^{-Ah}Pe^{-Ah}+\frac{2}{\iota^2}Œ_1+\delta_*\Pi_3-\lambda_\Omega I, \Omega_{33} = -Q+(1+\varepsilon)e^{-Bh}Qe^{-Bh}+\frac{2}{\iota^2}Œ_2+\tau_*\Pi_1+\eta_*\Pi_2-\lambda_\Omega I, \Omega_{55} = -\pi_1I+2R^TA_{*}PA_{*}R, \Omega_{66} = -\pi_2I+2A_{*}PA_{*}, \Omega_{77} = -\pi_3I+2A_{*}PA_{*}.
Then, the master-slave networks (2.1) and (2.3) are AMS-FTS with respect to \{\epsilon, \varsigma, \mathcal{N}\} , viz., for any \epsilon > 0 , there exists \varsigma > 0 so that
\max\limits_{s\in[-\tau_{\diamond}, 0]_{\mathbb{Z}}}\sum\limits_{v = 0}^{£}\mathbb{E}\Big[\big\|\psi_{s}^{[v]}\big\|^2+ \big\|\tilde{\psi}_{s}^{[v]}\big\|^2\Big]\leq \varsigma \Rightarrow \sum\limits_{v = 0}^{£}\mathbb{E}\Big[\left\|{\mathbf u}_{n}^{[v]}\right\|^2 +\left\|\boldsymbol\rho_{n}^{[v]}\right\|^2\Big] \leq \epsilon |
for all n\in(0, \mathcal{N}]_{\mathbb{Z}} . Hereby, the gains of the boundary controls are determined by
![]() |
Proof. Define a Lyapunov-Krasovskii functional, which is described by
V_{n} = V_{1, n}+ V_{2, n}+V_{3, n}+ V_{4, n}+V_{5, n}, |
where
V_{1, n} = \sum\limits_{v = 1}^{£ -1} ({\mathbf u}_{n}^{[v]} )^TP{\mathbf u}_{n}^{[v]}, \quad V_{2, n} = \sum\limits_{v = 1}^{£ -1} (\boldsymbol\rho _{n}^{[v]} )^TQ\boldsymbol\rho _{n}^{[v]}, \quad V_{3, n} = \sum\limits_{l = \tau_0}^{\tau_\infty}\sum\limits_{s = n-l}^{n -1}\sum\limits_{v = 1}^{£ -1}(\boldsymbol\rho_{s}^{[v]})^T \Pi_1\boldsymbol\rho_{s}^{[v]}, |
V_{4, n} = \sum\limits_{l = \eta_0}^{\eta_\infty}\sum\limits_{s = n-l}^{n -1}\sum\limits_{v = 1}^{£ -1}(\boldsymbol\rho_{s}^{[v]})^T \Pi_2\boldsymbol\rho_{s}^{[v]}, \quad V_{5, n} = \sum\limits_{l = \delta_0}^{\delta_\infty}\sum\limits_{s = n-l}^{n -1}\sum\limits_{v = 1}^{£ -1}({\mathbf u}_{s}^{[v]})^T \Pi_3{\mathbf u}_{s}^{[v]}, |
where n\in\mathbb{Z}_0 .
Calculating the first-order forward difference of V_1 with respect to time n , it gains
\mathbb{E}(\Delta V_{1, n}) = \mathbb{E}V_{1, n+1}-\mathbb{E}V_{1, n} = \underbrace{\mathbb{E}\sum\limits_{v = 1}^{£ -1} ({\mathbf u}_{n+1}^{[v]} )^TP{\mathbf u}_{n+1}^{[v]}}_{U_{1, n}} -\mathbb{E}\sum\limits_{v = 1}^{£ -1} ({\mathbf u}_{n}^{[v]} )^TP{\mathbf u}_{n}^{[v]}, | (3.2) |
where n\in\mathbb{Z}_0 . Compute the first term in the right side of the last equation, it follows
\begin{eqnarray*} U_{1, n}& = & \mathbb{E}\sum\limits_{v = 1}^{£ -1}({\mathbf u}_{n}^{[v]})^Te^{-Ah}Pe^{-Ah}{\mathbf u}_{n}^{[v]}+ \underbrace{\mathbb{E}\sum\limits_{v = 1}^{£ -1}(\Delta_{c, \iota}^{2}{\mathbf u}_{n}^{[v]})^T\Theta A_{*}PA_{*}\Theta\Delta_{c, \iota}^{2}{\mathbf u}_{n}^{[v]}}_{U_{2, n}}{{\nonumber}}\\ &&+\mathbb{E}\sum\limits_{v = 1}^{£ -1}(G(\boldsymbol\rho_{\tau_{n}}^{[v]}))^TR^TA_{*}PA_{*}RG(\boldsymbol\rho_{\tau_{n}}^{[v]}) +\mathbb{E}\sum\limits_{v = 1}^{£ -1}(G_{\bigvee}(\boldsymbol\rho_{\tau_{n}}^{[v]}))^TA_{*}PA_{*}G_{\bigvee}(\boldsymbol\rho_{\tau_{n}}^{[v]}){{\nonumber}}\\ &&+\mathbb{E}\sum\limits_{v = 1}^{£ -1}(G_{\bigwedge}(\boldsymbol\rho_{\tau_{n}}^{[v]}))^TA_{*}PA_{*}G_{\bigwedge}(\boldsymbol\rho_{\tau_{n}}^{[v]}) +\mathbb{E}\sum\limits_{v = 1}^{£ -1}(\sigma_\Delta^\omega(\boldsymbol\rho_{\eta_{n}}^{[v]}))^TS^TA_{*}PA_{*}S\sigma_\Delta^\omega(\boldsymbol\rho_{\eta_{n}}^{[v]}){{\nonumber}}\\ &&+\underbrace{\mathbb{E}\sum\limits_{v = 1}^{£ -1}\mathrm{sym}\big[({\mathbf u}_{n}^{[v]})^Te^{-Ah}PA_{*}\Theta\Delta_{c, \iota}^{2}{\mathbf u}_{n}^{[v]}\big]}_{U_{3, n}} +\mathbb{E}\sum\limits_{v = 1}^{£ -1}\mathrm{sym}\big[({\mathbf u}_{n}^{[v]})^Te^{-Ah}PA_{*}RG(\boldsymbol\rho_{\tau_{n}}^{[v]})\big]{{\nonumber}}\\ &&+\mathbb{E}\sum\limits_{v = 1}^{£ -1}\mathrm{sym}\big[({\mathbf u}_{n}^{[v]})^Te^{-Ah}PA_{*} G_{\bigvee}(\boldsymbol\rho_{\tau_{n}}^{[v]})\big] +\mathbb{E}\sum\limits_{v = 1}^{£ -1}\mathrm{sym}\big[({\mathbf u}_{n}^{[v]})^Te^{-Ah}PA_{*} G_{\bigwedge}(\boldsymbol\rho_{\tau_{n}}^{[v]})\big]{{\nonumber}}\\ &&+\underbrace{\mathbb{E}\sum\limits_{v = 1}^{£ -1}\mathrm{sym}\big[(\Delta_{c, \iota}^{2}{\mathbf u}_{n}^{[v]})^T\Theta A_{*}PA_{*}RG(\boldsymbol\rho_{\tau_{n}}^{[v]})\big]}_{U_{4, k}} {{\nonumber}}\\ && +\underbrace{\mathbb{E}\sum\limits_{v = 1}^{£ -1}\mathrm{sym}\big[(\Delta_{c, \iota}^{2} {\mathbf u}_{n}^{[v]})^T\Theta A_{*}PA_{*}G_{\bigvee}(\boldsymbol\rho_{\tau_{n}}^{[v]})\big]}_{U_{5, k}}{{\nonumber}}\\ &&+\underbrace{\mathbb{E}\sum\limits_{v = 1}^{£ -1}\mathrm{sym}\big[(\Delta_{c, \iota}^{2}{\mathbf u}_{n}^{[v]})^T\Theta A_{*}PA_{*}G_{\bigwedge}(\boldsymbol\rho_{\tau_{n}}^{[v]})\big]}_{U_{6, k}} {{\nonumber}}\\ && +\mathbb{E}\sum\limits_{v = 1}^{£ -1}\mathrm{sym}\big[(G(\boldsymbol\rho_{\tau_{n}}^{[v]}))^TR^T A_{*}PA_{*}G_{\bigvee}(\boldsymbol\rho_{\tau_{n}}^{[v]})\big]{{\nonumber}}\\ &&+\mathbb{E}\sum\limits_{v = 1}^{£ -1}\mathrm{sym}\big[(G(\boldsymbol\rho_{\tau_{n}}^{[v]}))^TR^T A_{*}PA_{*}G_{\bigwedge}(\boldsymbol\rho_{\tau_{n}}^{[v]})\big] {{\nonumber}}\\ && +\mathbb{E}\sum\limits_{v = 1}^{£ -1}\mathrm{sym}\big[(G_{\bigvee}(\boldsymbol\rho_{\tau_{n}}^{[v]}))^TA_{*}PA_{*}G_{\bigwedge}(\boldsymbol\rho_{\tau_{n}}^{[v]})\big], \quad \forall n\in\mathbb{Z}_0. \end{eqnarray*} | (3.3) |
According to the discrete formula of integration by parts in Lemma 2.1, it gets
![]() |
(3.4) |
Additionally,
\begin{eqnarray*} U_{4, k}& = &{\mathbb{E}\sum\limits_{v = 1}^{£ -1}\mathrm{sym}\big[(\Delta_{c, \iota}^{2}{\mathbf u}_{n}^{[v]})^T\Theta A_{*}PA_{*}RG(\boldsymbol\rho_{\tau_{n}}^{[v]})\big]}{{\nonumber}}\\ &\leq& \underbrace{\mathbb{E}\sum\limits_{v = 1}^{£ -1}(\Delta_{c, \iota}^{2}{\mathbf u}_{n}^{[v]})^T\Theta A_{*}PA_{*}\Theta\Delta_{c, \iota}^{2}{\mathbf u}_{n}^{[v]}}_{U_{2, n}} +\mathbb{E}\sum\limits_{v = 1}^{£ -1}(G(\boldsymbol\rho_{\tau_{n}}^{[v]}))^TR^TA_{*}PA_{*}RG(\boldsymbol\rho_{\tau_{n}}^{[v]}), \end{eqnarray*} | (3.5) |
\begin{eqnarray*} U_{5, k}& = & {\mathbb{E}\sum\limits_{v = 1}^{£ -1}\mathrm{sym}\big[(\Delta_{c, \iota}^{2} {\mathbf u}_{n}^{[v]})^T\Theta A_{*}PA_{*}G_{\bigvee}(\boldsymbol\rho_{\tau_{n}}^{[v]})\big]}{{\nonumber}}\\ &\leq& \underbrace{\mathbb{E}\sum\limits_{v = 1}^{£ -1}(\Delta_{c, \iota}^{2}{\mathbf u}_{n}^{[v]})^T\Theta A_{*}PA_{*}\Theta\Delta_{c, \iota}^{2}{\mathbf u}_{n}^{[v]}}_{U_{2, n}} +{\mathbb{E}\sum\limits_{v = 1}^{£ -1}(G_{\bigvee}(\boldsymbol\rho_{\tau_{n}}^{[v]})^TA_{*}PA_{*}G_{\bigvee}(\boldsymbol\rho_{\tau_{n}}^{[v]})}, \end{eqnarray*} | (3.6) |
as well
U_{6, k} = {\mathbb{E}\sum\limits_{v = 1}^{£ -1}\mathrm{sym}\big[(\Delta_{c, \iota}^{2}{\mathbf u}_{n}^{[v]})^T\Theta A_{*}PA_{*}G_{\bigwedge}(\boldsymbol\rho_{\tau_{n}}^{[v]})\big]} | (3.7) |
\leq \underbrace{\mathbb{E}\sum\limits_{v = 1}^{£ -1}(\Delta_{c, \iota}^{2}{\mathbf u}_{n}^{[v]})^T\Theta A_{*}PA_{*}\Theta\Delta_{c, \iota}^{2}{\mathbf u}_{n}^{[v]}}_{U_{2, n}} +{\mathbb{E}\sum\limits_{v = 1}^{£ -1}(G_{\bigwedge}(\boldsymbol\rho_{\tau_{n}}^{[v]}))^TA_{*}PA_{*}G_{\bigwedge}(\boldsymbol\rho_{\tau_{n}}^{[v]})}, | (3.8) |
where n\in\mathbb{Z}_0 .
Via Lemma 2.2, it computes
\begin{eqnarray*} 4U_{2, n}& = & 4\mathbb{E}\sum\limits_{v = 1}^{£ -1}(\Delta_{c, \iota}^{2}{\mathbf u}_{n}^{[v]})^T\Theta A_{*}PA_{*}\Theta\Delta_{c, \iota}^{2}{\mathbf u}_{n}^{[v]}{{\nonumber}}\\ & = &4\mathbb{E}\sum\limits_{v = 1}^{£ -1}\big[\Delta_{\iota}(\Delta_{\iota}{\mathbf u}_{n}^{[v-1]})\big]^T\Theta A_{*}PA_{*}\Theta\big[\Delta_{\iota}(\Delta_{\iota}{\mathbf u}_{n}^{[v-1]})\big]{{\nonumber}}\\ & = &4\mathbb{E}\sum\limits_{v = 0}^{£ -2}\big[\Delta_{\iota}(\Delta_{\iota}{\mathbf u}_{n}^{[v]})\big]^T\Theta A_{*}PA_{*}\Theta\big[\Delta_{\iota}(\Delta_{\iota}{\mathbf u}_{n}^{[v]})\big]{{\nonumber}}\\ &\leq& \frac{16}{\iota^{2} } \cos^{2} \frac{\pi }{2£}\mathbb{E}\sum\limits_{v = 0}^{£ -1}(\Delta_{\iota}{\mathbf u}_{n}^{[v]})^T\Theta A_{*}PA_{*}\Theta \Delta_{\iota}{\mathbf u}_{n}^{[v]}{{\nonumber}}\\ & = &\frac{16}{\iota^{4} } \cos^{2} \frac{\pi }{2£}\mathbb{E}({\mathbf u}_{n}^{[1]})^T\Theta A_{*}PA_{*}\Theta{\mathbf u}_{n}^{[1]} {{\nonumber}}\\ &&+\frac{16}{\iota^{2} } \cos^{2} \frac{\pi }{2£}\mathbb{E}\sum\limits_{v = 1}^{£ -1}(\Delta_{\iota}{\mathbf u}_{n}^{[v]})^T\Theta A_{*}PA_{*}\Theta \Delta_{\iota}{\mathbf u}_{n}^{[v]}, \quad\forall n\in\mathbb{Z}_0. \end{eqnarray*} | (3.9) |
By the definition of V_2 , it calculates
\mathbb{E}(\Delta V_{2, n}) = \mathbb{E}V_{2, n+1}-\mathbb{E}V_{2, n} = \underbrace{\mathbb{E}\sum\limits_{v = 1}^{£ -1} (\boldsymbol\rho _{n+1}^{[v]} )^TQ\boldsymbol\rho _{n+1}^{[v]}}_{\S_{1, n}}-\mathbb{E}\sum\limits_{v = 1}^{£ -1} (\boldsymbol\rho _{n}^{[v]} )^TQ\boldsymbol\rho _{n}^{[v]}, | (3.10) |
where
\begin{eqnarray*} \S_{1, n} & = & \mathbb{E}\sum\limits_{v = 1}^{£ -1}(\boldsymbol\rho_{n}^{[v]})^Te^{-Bh}Qe^{-Bh}\boldsymbol\rho_{n}^{[v]}+\underbrace{\mathbb{E}\sum\limits_{v = 1}^{£ -1}(\Delta_{c, \iota}^{2}\boldsymbol\rho_{n}^{[v]})^T\Phi B_{*}QB_{*}\Phi\Delta_{c, \iota}^{2}\boldsymbol\rho_{n}^{[v]}}_{\S_{2, n}}{{\nonumber}}\\ &&+\mathbb{E}\sum\limits_{v = 1}^{£ -1}({\mathbf u}_{\delta_{n}}^{[v]})^TC^TB_{*}QB_{*}C{\mathbf u}_{\delta_{n}}^{[v]} +\underbrace{\mathbb{E}\sum\limits_{v = 1}^{£ -1}\mathrm{sym}\big[(\boldsymbol\rho_{n}^{[v]})^T e^{-Bh}QB_{*} \Phi\Delta_{c, \iota}^{2}\boldsymbol\rho_{n}^{[v]}\big]}_{\S_{3, n}}{{\nonumber}}\\ &&+\underbrace{\mathbb{E}\sum\limits_{v = 1}^{£ -1}\mathrm{sym}\big[(\boldsymbol\rho_{n}^{[v]})^T e^{-Bh}QB_{*}C{\mathbf u}_{\delta_{n}}^{[v]}\big]}_{\S_{4, n}} +\underbrace{\mathbb{E}\sum\limits_{v = 1}^{£ -1}\mathrm{sym} \big[(\Delta_{c, \iota}^{2}\boldsymbol\rho_{n}^{[v]})^T\Phi B_{*} QB_{*}C{\mathbf u}_{\delta_{n}}^{[v]}\big]}_{\S_{5, n}}, \end{eqnarray*} | (3.11) |
where n\in\mathbb{Z}_0 .
Via the similar argument as that in (3.4), it contains
![]() |
(3.12) |
and similar to (3.5),
\begin{eqnarray*} \S_{4, n}& = &\mathbb{E}\sum\limits_{v = 1}^{£ -1}\mathrm{sym}\big[(\boldsymbol\rho_{n}^{[v]})^T e^{-Bh}QB_{*}C{\mathbf u}_{\delta_{n}}^{[v]}\big]{{\nonumber}}\\ &\leq& \varepsilon\mathbb{E}\sum\limits_{v = 1}^{£ -1}(\boldsymbol\rho_{n}^{[v]})^Te^{-Bh}Qe^{-Bh}\boldsymbol\rho_{n}^{[v]} +\frac{1}{\varepsilon}\mathbb{E}\sum\limits_{v = 1}^{£ -1}({\mathbf u}_{\delta_{n}}^{[v]})^TCB_{*}QB_{*}C{\mathbf u}_{\delta_{n}}^{[v]}, \end{eqnarray*} | (3.13) |
\begin{eqnarray*} \S_{5, n}& = &\mathbb{E}\sum\limits_{v = 1}^{£ -1}\mathrm{sym}\big[(\Delta_{c, \iota}^{2}\boldsymbol\rho_{n}^{[v]})^T\Phi B_{*}QB_{*}C{\mathbf u}_{\delta_{n}}^{[v]}\big]\\ &\leq& \underbrace{\mathbb{E}\sum\limits_{v = 1}^{£ -1} (\Delta_{c, \iota}^{2}\boldsymbol\rho_{n}^{[v]})^T \Phi B_{*}QB_{*}\Phi\Delta_{c, \iota}^{2}\boldsymbol\rho_{n}^{[v]}}_{\S_{2, n}}+\mathbb{E}\sum\limits_{v = 1}^{£ -1}({\mathbf u}_{\delta_{n}}^{[v]})^TCB_{*}QB_{*}C{\mathbf u}_{\delta_{n}}^{[v]}, \end{eqnarray*} | (3.14) |
and similar to (3.9),
\begin{eqnarray*} 2\S_{2, n}& = &2\mathbb{E}\sum\limits_{v = 1}^{£ -1}(\Delta_{c, \iota}^{2}\boldsymbol\rho_{n}^{[v]})^T\Phi B_{*}QB_{*}\Phi\Delta_{c, \iota}^{2}\boldsymbol\rho_{n}^{[v]}{{\nonumber}}\\ & = &2\mathbb{E}\sum\limits_{v = 1}^{£ -1}\big[\Delta_{\iota}(\Delta_{\iota}\boldsymbol\rho_{n}^{[v-1]})\big]^T\Phi B_{*}QB_{*}\Phi\big[\Delta_{\iota}(\Delta_{\iota}\boldsymbol\rho_{n}^{[v-1]})\big]{{\nonumber}}\\ & = &2\mathbb{E}\sum\limits_{v = 0}^{£ -2}\big[\Delta_{\iota}(\Delta_{\iota}\boldsymbol\rho_{n}^{[v]})\big]^T\Phi B_{*}QB_{*}\Phi\big[\Delta_{\iota}(\Delta_{\iota}\boldsymbol\rho_{n}^{[v]})\big]{{\nonumber}}\\ &\leq& \frac{8}{\iota^{2} } \cos^{2} \frac{\pi }{2£}\mathbb{E}\sum\limits_{v = 0}^{£ -1}(\Delta_{\iota}\boldsymbol\rho_{n}^{[v]})^T\Phi B_{*}QB_{*}\Phi \Delta_{\iota}\boldsymbol\rho_{n}^{[v]}{{\nonumber}}\\ & = &\frac{8}{\iota^{4} } \cos^{2} \frac{\pi }{2£}\mathbb{E}(\boldsymbol\rho_{n}^{[1]})^T\Phi B_{*}QB_{*}\Phi\boldsymbol\rho_{n}^{[1]} {{\nonumber}}\\ &&+\frac{8}{\iota^{2} } \cos^{2} \frac{\pi }{2£}\mathbb{E}\sum\limits_{v = 1}^{£ -1}(\Delta_{\iota}\boldsymbol\rho_{n}^{[v]})^T\Phi B_{*}QB_{*}\Phi \Delta_{\iota}\boldsymbol\rho_{n}^{[v]}, \quad\forall n\in\mathbb{Z}_0. \end{eqnarray*} | (3.15) |
Set \hat{{\mathbf u}} _{n}^{[v]} = {{\mathbf u}} _{n}^{[£]}-{{\mathbf u}} _{n}^{[v]} and \hat{\boldsymbol\rho} _{n}^{[v]} = {\boldsymbol\rho} _{n}^{[£]}-{\boldsymbol\rho} _{n}^{[v]} , \forall (v, n)\in[0, £]_{\mathbb{Z}}\times\mathbb{Z}_0 . So
![]() |
(3.16) |
as well
![]() |
(3.17) |
Through Lemma 2.2, it achieves
\begin{eqnarray*} 0& = &-\sum\limits_{v = 1}^{£ -1}(\hat{{\mathbf u}} _{n}^{[v]})^T\hat{{\mathbf u}} _{n}^{[v]}+ \sum\limits_{v = 1}^{£ -1}(\hat{{\mathbf u}} _{n}^{[v]})^T\hat{{\mathbf u}} _{n}^{[v]}{{\nonumber}}\\ & = &-\sum\limits_{v = 1}^{£ -1}(\hat{{\mathbf u}} _{n}^{[v]})^T\hat{{\mathbf u}} _{n}^{[v]}+ \sum\limits_{v = 1}^{£ }(\hat{{\mathbf u}} _{n}^{[v]})^T\hat{{\mathbf u}} _{n}^{[v]}{{\nonumber}}\\ &\underline{\underline {{{v' = £ -v}}}} & -\sum\limits_{v = 1}^{£ -1}(\hat{{\mathbf u}} _{n}^{[v]})^T\hat{{\mathbf u}} _{n}^{[v]}+ \sum\limits_{v' = 0}^{£ -1}(\hat{{\mathbf u}} _{n}^{[£ -v']})^T\hat{{\mathbf u}} _{n}^{[£ -v']}{{\nonumber}}\\ &\leq& -\sum\limits_{v = 1}^{£ -1}(\hat{{\mathbf u}} _{n}^{[v]})^T\hat{{\mathbf u}} _{n}^{[v]}+ \frac{\iota^{2} }{4\sin^{2} \frac{\pi }{4£ -2} } \sum\limits_{v' = 0}^{£ -2} (\Delta_{\iota}\hat{{\mathbf u}} _{n}^{[£ -v']})^T\Delta_{\iota}\hat{{\mathbf u}} _{n}^{[£ -v']}{{\nonumber}}\\ &\underline{\underline {{{v = £ -v'}}}} &-\sum\limits_{v = 1}^{£ -1}(\hat{{\mathbf u}} _{n}^{[v]})^T\hat{{\mathbf u}} _{n}^{[v]}+ \frac{\iota^{2} }{4\sin^{2} \frac{\pi }{4£ -2} } \sum\limits_{v = 1}^{£-1 }(\Delta_{\iota}\hat{{\mathbf u}} _{n}^{[v]})^T\Delta_{\iota}\hat{{\mathbf u}} _{n}^{[v]}{{\nonumber}}\\ & = &-\sum\limits_{v = 1}^{£ -1}(\hat{{\mathbf u}} _{n}^{[v]})^T\hat{{\mathbf u}} _{n}^{[v]}+ \frac{\iota^{2} }{4\sin^{2} \frac{\pi }{4£ -2} } \sum\limits_{v = 1}^{£-1 }(\Delta_{\iota}{{\mathbf u}} _{n}^{[v]})^T\Delta_{\iota}{{\mathbf u}} _{n}^{[v]}, \end{eqnarray*} | (3.18) |
and similarly,
\begin{eqnarray*} 0& = &-\sum\limits_{v = 1}^{£ -1}(\hat{\boldsymbol\rho} _{n}^{[v]})^T\hat{\boldsymbol\rho} _{n}^{[v]}+ \sum\limits_{v = 1}^{£ -1}(\hat{\boldsymbol\rho} _{n}^{[v]})^T\hat{\boldsymbol\rho} _{n}^{[v]}{{\nonumber}}\\ &\leq&-\sum\limits_{v = 1}^{£ -1}(\hat{\boldsymbol\rho} _{n}^{[v]})^T\hat{\boldsymbol\rho} _{n}^{[v]}+ \frac{\iota^{2} }{4\sin^{2} \frac{\pi }{4£ -2} } \sum\limits_{v = 1}^{£-1 } (\Delta_{\iota}{\boldsymbol\rho} _{n}^{[v]})^T\Delta_{\iota}{\boldsymbol\rho} _{n}^{[v]}, \quad\forall n\in\mathbb{Z}_0. \end{eqnarray*} | (3.19) |
Besides,
\begin{eqnarray*} 0& = &-\pi_1\sum\limits_{v = 1}^{£ -1}(G(\boldsymbol\rho_{\tau_{n}}^{[v]}))^T G(\boldsymbol\rho_{\tau_{n}}^{[v]}) +\pi_1\sum\limits_{v = 1}^{£ -1}(G(\boldsymbol\rho_{\tau_{n}}^{[v]}))^T G(\boldsymbol\rho_{\tau_{n}}^{[v]}){{\nonumber}}\\ &\leq&-\pi_1\sum\limits_{v = 1}^{£ -1}(G(\boldsymbol\rho_{\tau_{n}}^{[v]}))^T G(\boldsymbol\rho_{\tau_{n}}^{[v]}) +\pi_1\sum\limits_{v = 1}^{£ -1}(\boldsymbol\rho_{\tau_{n}}^{[v]})^TH^2\boldsymbol\rho_{\tau_{n}}^{[v]}, \end{eqnarray*} | (3.20) |
\begin{eqnarray*} 0& = &-\pi_2\sum\limits_{v = 1}^{£ -1}(G_{\bigvee}(\boldsymbol\rho_{\tau_{n}}^{[v]}))^TG_{\bigvee}(\boldsymbol\rho_{\tau_{n}}^{[v]}) +\pi_2\sum\limits_{v = 1}^{£ -1}(G_{\bigvee}(\boldsymbol\rho_{\tau_{n}}^{[v]}))^TG_{\bigvee}(\boldsymbol\rho_{\tau_{n}}^{[v]}){{\nonumber}}\\ &\leq&-\pi_2\sum\limits_{v = 1}^{£ -1}(G_{\bigvee}(\boldsymbol\rho_{\tau_{n}}^{[v]}))^T G_{\bigvee}(\boldsymbol\rho_{\tau_{n}}^{[v]}) +\pi_2\sum\limits_{v = 1}^{£ -1}\bigg[\bigg|\bigvee\limits_{j = 1}^N\xi_{1j}\tilde{g}_j (\rho_{j, \tau_{n}}^{[v]})\bigg| ^{2} + \dots +\bigg|\bigvee\limits_{j = 1}^N\xi_{Nj}\tilde{g}_j(\rho_{j, \tau_{n}}^{[v]}) \bigg| ^{2}\bigg]{{\nonumber}}\\ &\leq&-\pi_2\sum\limits_{v = 1}^{£ -1}(G_{\bigvee}(\boldsymbol\rho_{\tau_{n}}^{[v]}))^T G_{\bigvee}(\boldsymbol\rho_{\tau_{n}}^{[v]}) +\pi_2\xi_{\infty }N^2\sum\limits_{v = 1}^{£ -1}(\boldsymbol\rho_{\tau_{n}}^{[v]})^TH^2\boldsymbol\rho_{\tau_{n}}^{[v]}, \end{eqnarray*} | (3.21) |
\begin{eqnarray*} 0& = &-\pi_3\sum\limits_{v = 1}^{£ -1}(G_{\bigwedge}(\boldsymbol\rho_{\tau_{n}}^{[v]}))^TG_{\bigwedge}(\boldsymbol\rho_{\tau_{n}}^{[v]}) +\pi_3\sum\limits_{v = 1}^{£ -1}(G_{\bigwedge}(\boldsymbol\rho_{\tau_{n}}^{[v]}))^T G_{\bigwedge}(\boldsymbol\rho_{\tau_{n}}^{[v]}){{\nonumber}}\\ &\leq&-\pi_3\sum\limits_{v = 1}^{£ -1}(G_{\bigwedge}(\boldsymbol\rho_{\tau_{n}}^{[v]}))^T G_{\bigwedge}(\boldsymbol\rho_{\tau_{n}}^{[v]}) +\pi_3\zeta_{\infty }N^2\sum\limits_{v = 1}^{£ -1}(\boldsymbol\rho_{\tau_{n}}^{[v]})^TH^2\boldsymbol\rho_{\tau_{n}}^{[v]}, \end{eqnarray*} | (3.22) |
and
\begin{eqnarray*} 0& = &-\pi_4\mathbb{E}\sum\limits_{v = 1}^{£ -1}(\sigma_\Delta^\omega(\boldsymbol\rho_{\eta_{n}}^{[v]}))^T\sigma_\Delta^\omega(\boldsymbol\rho_{\eta_{n}}^{[v]}) +\pi_4\mathbb{E}\sum\limits_{v = 1}^{£ -1}(\sigma_\Delta^\omega(\boldsymbol\rho_{\eta_{n}}^{[v]}))^T\sigma_\Delta^\omega(\boldsymbol\rho_{\eta_{n}}^{[v]}){{\nonumber}}\\ &\leq&-\pi_4\mathbb{E}\sum\limits_{v = 1}^{£ -1}(\sigma_\Delta^\omega(\boldsymbol\rho_{\eta_{n}}^{[v]}))^T \sigma_\Delta^\omega(\boldsymbol\rho_{\eta_{n}}^{[v]}) +\pi_4\mathbb{E}\sum\limits_{v = 1}^{£ -1}(\boldsymbol\rho_{\eta_{n}}^{[v]})^TK^2\boldsymbol\rho_{\eta_{n}}^{[v]}, \quad \forall n\in\mathbb{Z}_0. \end{eqnarray*} | (3.23) |
Additionally,
\begin{eqnarray*} \mathbb{E}(\Delta V_{3, n})\leq\tau_* \sum\limits_{v = 1}^{£ -1}\mathbb{E}(\boldsymbol\rho_{n}^{[v]})^T \Pi_1\boldsymbol\rho_{n}^{[v]} -\sum\limits_{v = 1}^{£ -1}\mathbb{E}(\boldsymbol\rho_{\tau_n}^{[v]})^T \Pi_1\boldsymbol\rho_{\tau_n}^{[v]}, \end{eqnarray*} | (3.24) |
\begin{eqnarray*} \mathbb{E}(\Delta V_{4, n})\leq\eta_* \sum\limits_{v = 1}^{£ -1}\mathbb{E}(\boldsymbol\rho_{n}^{[v]})^T \Pi_2\boldsymbol\rho_{n}^{[v]} -\sum\limits_{v = 1}^{£ -1}\mathbb{E}(\boldsymbol\rho_{\eta_n}^{[v]})^T \Pi_2\boldsymbol\rho_{\eta_n}^{[v]}, \end{eqnarray*} | (3.25) |
and
\begin{eqnarray*} \label{eq.end} \mathbb{E}(\Delta V_{5, n})\leq\delta_* \sum\limits_{v = 1}^{£ -1}\mathbb{E}({\mathbf u}_{n}^{[v]})^T \Pi_3{\mathbf u}_{n}^{[v]} -\sum\limits_{v = 1}^{£ -1}\mathbb{E}({\mathbf u}_{\delta_n}^{[v]})^T \Pi_3{\mathbf u}_{\delta_n}^{[v]}, \quad\forall n\in\mathbb{Z}_0. \end{eqnarray*} | (3.26) |
Define a vector as follows
\boldsymbol\varpi_{n}^{[{v}]} = \Big( ({\mathbf u}_{n}^{[{v}]})^T, (\hat{{\mathbf u}}_{n}^{[{v}]})^T, (\boldsymbol\rho_{n}^{[{v}]})^T, (\hat{\boldsymbol\rho}_{n}^{[{v}]})^T, (G(\boldsymbol\rho_{\tau_{n}}^{[v]}))^T, (G_{\bigvee}(\boldsymbol\rho_{\tau_{n}}^{[v]}))^T, (G_{\bigwedge}(\boldsymbol\rho_{\tau_{n}}^{[v]}))^T \Big)^T, |
where ({v}, n)\in(0, £)_{\mathbb{Z}}\times\mathbb{Z}_0. By (3.2)–(3.26) and assumptions (f_3)–(f_5), it follows
\begin{eqnarray*} \label{dv1} \mathbb{E}(\Delta V_{n}) &\leq& \sum\limits_{{v} = 1}^{£-1}\mathbb{E} (\boldsymbol\varpi_{n}^{[{v}]})^{T}\Omega \boldsymbol\varpi_{n}^{[{v}]} +\lambda_\Omega\sum\limits_{{v} = 1}^{£-1}\mathbb{E} \|{\mathbf u}_{n}^{[{v}]}\|^2 +\lambda_\Omega\sum\limits_{{v} = 1}^{£-1}\mathbb{E} \|\boldsymbol\rho_{n}^{[{v}]}\|^2{{\nonumber}}\\ &\leq&\lambda_\Omega\sum\limits_{{v} = 1}^{£-1}\mathbb{E} \|{\mathbf u}_{n}^{[{v}]}\|^2 +\lambda_\Omega\sum\limits_{{v} = 1}^{£-1}\mathbb{E} \|\boldsymbol\rho_{n}^{[{v}]}\|^2, \quad \forall n\in\mathbb{Z}_0. \end{eqnarray*} | (3.27) |
Let \lambda_{+} denote the maximum eigenvalue of the matrices P, Q, \Pi_1, \Pi_2, \Pi_3, \lambda_{-} denote the minimum eigenvalue of the matrices P, Q , and \lambda_G denote the maximum eigenvalue of the matrices and
. For any \epsilon > 0 , taking
\varsigma = \bigg\{ \frac{3+2£\lambda_G}{\lambda_-} (1+\frac{\lambda_\Omega}{\lambda_-})^{\mathcal{N}-1}[\lambda_\Omega+(3\tau_\diamond^2+2)\lambda_+] \bigg\}^{-1}\epsilon. |
Assume that
\max\limits_{s\in[-\tau_{\diamond}, 0]_{\mathbb{Z}}}\sum\limits_{v = 0}^{£}\mathbb{E}\Big[\big\|\psi_{s}^{[v]}\big\|^2+ \big\|\tilde{\psi}_{s}^{[v]}\big\|^2\Big]\leq \varsigma. |
Taking n = 0, 1, 2 orderly in the inequality (3.27), it results in
\lambda_-\sum\limits_{{v} = 1}^{£-1}\mathbb{E} \big[\|{\mathbf u}_{1}^{[{v}]}\|^2 +\|\boldsymbol\rho_{1}^{[{v}]}\|^2\big] \leq \mathbb{E}V_1 \leq [\lambda_\Omega+(3\tau_\diamond^2+2)\lambda_+]\varsigma, |
\lambda_-\sum\limits_{{v} = 1}^{£-1}\mathbb{E} \big[\|{\mathbf u}_{2}^{[{v}]}\|^2 +\|\boldsymbol\rho_{2}^{[{v}]}\|^2\big] \leq \mathbb{E}V_2 \leq(1+\frac{\lambda_\Omega}{\lambda_-})\mathbb{E}V_1, |
\lambda_-\sum\limits_{{v} = 1}^{£-1}\mathbb{E} \big[\|{\mathbf u}_{3}^{[{v}]}\|^2 +\|\boldsymbol\rho_{3}^{[{v}]}\|^2\big] \leq \mathbb{E}V_3 \leq(1+\frac{\lambda_\Omega}{\lambda_-})^2\mathbb{E}V_1. |
Via the technique of mathematical induction, it directly gains
\sum\limits_{{v} = 1}^{£-1}\mathbb{E} \big[\|{\mathbf u}_{n}^{[{v}]}\|^2 +\|\boldsymbol\rho_{n}^{[{v}]}\|^2\big]\leq \frac{1}{\lambda_-} (1+\frac{\lambda_\Omega}{\lambda_-})^{n-1}[\lambda_\Omega+(3\tau_\diamond^2+2)\lambda_+] \varsigma, \quad \forall n\in\mathbb{Z}_0\backslash\{0\}. |
Together with (2.7) and (3.1), it gets
\begin{eqnarray*} \sum\limits_{{v} = 0}^{£}\mathbb{E} \big[\|{\mathbf u}_{n}^{[{v}]}\|^2 +\|\boldsymbol\rho_{n}^{[{v}]}\|^2\big]&\leq&\frac{3+2£\lambda_G}{\lambda_-} (1+\frac{\lambda_\Omega}{\lambda_-})^{\mathcal{N}-1}[\lambda_\Omega+(3\tau_\diamond^2+2)\lambda_+] \varsigma{{\nonumber}}\\ & = &\epsilon, \quad \forall n\in(0, \mathcal{N}]_{\mathbb{Z}_0}.{{\nonumber}} \end{eqnarray*} |
Thus, the master-slave networks (2.1) and (2.3) have finite-time asymptotic synchronization in the mean-square sense with respect to \{\epsilon, \varsigma, \mathcal{N}\} . This completes the proof.
Theorem 3.2. Let the gains be defined as those in Theorem 3.1, conditions (f_1), (f_2), (f_3), and (f_4) be fulfilled. Furthermore, it holds that
(f_6) there exists \kappa\in(0, \frac{1}{\tau_\diamond+1}] and constant \lambda_\Omega\geq0 so that
\Omega_* = \Omega+\left( \begin{array}{ccccccc} \kappa P+\delta_{\infty}\delta_*\Pi_3 & 0 & 0 & 0 & 0 & 0 & 0 \\ * & 0 & 0 & 0 & 0 & 0 & 0 \\ * & * &\kappa Q+\tau_{\infty}\tau_*\Pi_1+\eta_{\infty}\eta_*\Pi_2 & 0 & 0 & 0 & 0 \\ * & * & * & 0 & 0 & 0 & 0 \\ * & * & * & * & 0 & 0 & 0 \\ * & * & * & * & * & 0 & 0 \\ * & * & * & * & * & * & 0 \\ \end{array} \right) < 0, |
where \Omega is defined as that in Theorem 3.1.
Then, the master-slave networks (2.1) and (2.3) are EMS-FTS with respect to \{\epsilon, \varsigma, \mathcal{N}, \kappa_*\} , viz., for any \epsilon > 0 , it exists \varsigma > 0 , \kappa_* > 0 and function \{(\varsigma) > 0 so that
\max\limits_{s\in[-\tau_{\diamond}, 0]_{\mathbb{Z}}}\sum\limits_{v = 0}^{£}\mathbb{E}\Big[\big\|\psi_{s}^{[v]}\big\|^2+ \big\|\tilde{\psi}_{s}^{[v]}\big\|^2\Big]\leq \varsigma \Rightarrow \sum\limits_{v = 0}^{£}\mathbb{E}\Big[\left\|{\mathbf u}_{n}^{[v]}\right\|^2 +\left\|\boldsymbol\rho_{n}^{[v]}\right\|^2\Big]\leq\{(\varsigma)e^{-\kappa_*n} \leq \epsilon |
for all n\in(0, \mathcal{N}]_{\mathbb{Z}} .
Proof. Let us build a Lyapunov-Krasovskii functional, which is given by
V_n = V_{1, n}+V_{2, n}+V_{3, n}+V_{4, n}+V_{5, n}+V_{6, n}+V_{7, n}+V_{8, n}, |
where V_{1, n}, \ldots, V_{5, n} are defined as those in Theorem 3.1, and
V_{6, n} = \sum\limits_{l = \tau_0}^{\tau_\infty}\sum\limits_{s = n-l}^{n -1} \sum\limits_{s' = s}^{n-1}\sum\limits_{v = 1}^{£ -1}(\boldsymbol\rho_{s'}^{[v]})^T \Pi_1\boldsymbol\rho_{s'}^{[v]}, |
V_{7, n} = \sum\limits_{l = \eta_0}^{\eta_\infty}\sum\limits_{s = n-l}^{n -1} \sum\limits_{s' = s}^{n-1}\sum\limits_{v = 1}^{£ -1}(\boldsymbol\rho_{s'}^{[v]})^T \Pi_2\boldsymbol\rho_{s'}^{[v]}, \quad V_{8, n} = \sum\limits_{l = \delta_0}^{\delta_\infty}\sum\limits_{s = n-l}^{n -1} \sum\limits_{s' = s}^{n-1}\sum\limits_{v = 1}^{£ -1}({\mathbf u}_{s'}^{[v]})^T \Pi_3{\mathbf u}_{s'}^{[v]}, \quad \forall n\in\mathbb{Z}_0. |
It follows
\begin{eqnarray*} \label{v33} \left\{ \begin{array}{lll} \mathbb{E}(\Delta V_{6, n}) &\leq& \tau_{\infty}\tau_*\mathbb{E}\sum\limits_{v = 1}^{£ -1}(\boldsymbol\rho_{n}^{[v]})^T \Pi_1\boldsymbol\rho_{n}^{[v]} -\mathbb{E}V_{3, n}, \\ \mathbb{E}(\Delta V_{7, n}) &\leq& \eta_{\infty}\eta_*\mathbb{E}\sum\limits_{v = 1}^{£ -1}(\boldsymbol\rho_{n}^{[v]})^T \Pi_2\boldsymbol\rho_{n}^{[v]} -\mathbb{E}V_{4, n}, \\ \mathbb{E}(\Delta V_{8, n}) &\leq& \delta_{\infty}\delta_*\mathbb{E}\sum\limits_{v = 1}^{£ -1}({\mathbf u}_{n}^{[v]})^T \Pi_3{\mathbf u}_{n}^{[v]} -\mathbb{E}V_{5, n}, \quad \forall n\in\mathbb{Z}_0, \end{array} \right. \end{eqnarray*} | (3.28) |
and
\begin{eqnarray*} \label{v333} V_{6, n}\leq\tau_{\infty}V_{3, n}, \quad V_{7, n}\leq\eta_{\infty}V_{4, n}, \quad V_{8, n}\leq\delta_{\infty}V_{5, n}, \quad \forall n\in\mathbb{Z}_0. \end{eqnarray*} | (3.29) |
By (3.27)–(3.29), it has \kappa\in(0, \frac{1}{\tau_\diamond+1}] such that
\begin{eqnarray*} \label{es} \mathbb{E}(\Delta V_{n})+\kappa\mathbb{E}(V_{n}) &\leq&\sum\limits_{{v} = 1}^{£-1}\mathbb{E} (\boldsymbol\varpi_{n}^{[{v}]})^{T}\Omega_* \boldsymbol\varpi_{n}^{[{v}]} +\lambda_\Omega\sum\limits_{{v} = 1}^{£-1}\mathbb{E} \|{\mathbf u}_{n}^{[{v}]}\|^2 +\lambda_\Omega\sum\limits_{{v} = 1}^{£-1}\mathbb{E} \|\boldsymbol\rho_{n}^{[{v}]}\|^2 \nonumber\\ &&-(1-\kappa-\kappa \tau_{\infty}) V_{3, n} -(1-\kappa-\kappa \eta_{\infty}) V_{4, n} -(1-\kappa-\kappa \delta_{\infty}) V_{5, n}{{\nonumber}}\\ &\leq&\lambda_\Omega\sum\limits_{{v} = 1}^{£-1}\mathbb{E} \|{\mathbf u}_{n}^{[{v}]}\|^2 +\lambda_\Omega\sum\limits_{{v} = 1}^{£-1}\mathbb{E} \|\boldsymbol\rho_{n}^{[{v}]}\|^2, \quad \forall n\in\mathbb{Z}_0. \end{eqnarray*} | (3.30) |
For any \epsilon > 0 , taking
\varsigma = \bigg\{ \frac{3+2£\lambda_G}{\lambda_-(1-\kappa)} (1+\frac{\lambda_\Omega}{\lambda_-})^{\mathcal{N}}[\lambda_\Omega+(6\tau_\diamond^3+2)\lambda_+] \bigg\}^{-1}\epsilon. |
Assume that
\max\limits_{s\in[-\tau_{\diamond}, 0]_{\mathbb{Z}}}\sum\limits_{v = 0}^{£}\mathbb{E}\Big[\big\|\psi_{s}^{[v]}\big\|^2+ \big\|\tilde{\psi}_{s}^{[v]}\big\|^2\Big]\leq \varsigma. |
Taking n = 0, 1, 2 orderly in the inequality (3.30), it addresses
\lambda_-\sum\limits_{{v} = 1}^{£-1}\mathbb{E} \big[\|{\mathbf u}_{1}^{[{v}]}\|^2 +\|\boldsymbol\rho_{1}^{[{v}]}\|^2\big] \leq \mathbb{E}V_1 \leq(1-\kappa) [\lambda_\Omega+(6\tau_\diamond^3+2)\lambda_+]\varsigma, |
\lambda_-\sum\limits_{{v} = 1}^{£-1}\mathbb{E} \big[\|{\mathbf u}_{2}^{[{v}]}\|^2 +\|\boldsymbol\rho_{2}^{[{v}]}\|^2\big] \leq \mathbb{E}V_2 \leq(1-\kappa+\frac{\lambda_\Omega}{\lambda_-})\mathbb{E}V_1, |
\lambda_-\sum\limits_{{v} = 1}^{£-1}\mathbb{E} \big[\|{\mathbf u}_{3}^{[{v}]}\|^2 +\|\boldsymbol\rho_{3}^{[{v}]}\|^2\big] \leq \mathbb{E}V_3 \leq(1-\kappa+\frac{\lambda_\Omega}{\lambda_-})^2\mathbb{E}V_1. |
Via the technique of mathematical induction, it directly gains
\sum\limits_{{v} = 1}^{£-1}\mathbb{E} \big[\|{\mathbf u}_{n}^{[{v}]}\|^2 +\|\boldsymbol\rho_{n}^{[{v}]}\|^2\big]\leq \frac{1}{\lambda_-} (1-\kappa+\frac{\lambda_\Omega}{\lambda_-})^{n-1}[\lambda_\Omega+(6\tau_\diamond^3+2)\lambda_+] \varsigma, \quad \forall n\in\mathbb{Z}_0\backslash\{0\}. |
Together with (2.7) and (3.1), it gets
\begin{eqnarray*} \sum\limits_{{v} = 0}^{£}\mathbb{E} \big[\|{\mathbf u}_{n}^{[{v}]}\|^2 +\|\boldsymbol\rho_{n}^{[{v}]}\|^2\big]&\leq& \underbrace{\frac{3+2£\lambda_G}{\lambda_-(1-\kappa)} (1+\frac{\lambda_\Omega}{\lambda_-})^{\mathcal{N}}[\lambda_\Omega+(6\tau_\diamond^3+2)\lambda_+] \varsigma}_{\{(\varsigma)} e^{-\kappa_*n}{{\nonumber}}\\ &\leq&\epsilon, \quad \forall n\in(0, \mathcal{N}]_{\mathbb{Z}_0}, {{\nonumber}} \end{eqnarray*} |
where \kappa_* = -\mathrm{ln}\frac{1-\kappa+\frac{\lambda_\Omega}{\lambda_-}}{1+\frac{\lambda_\Omega}{\lambda_-}}. Thus, the master-slave networks (2.1) and (2.3) are EMS-FTS with respect to \{\epsilon, \varsigma, \mathcal{N}, \kappa_*\} . This completes the proof.
Remark 3.1. In [13,14], authors examined the diverse control issues associated with reaction-diffusion GRNs, including finite-time stability, state observer, and other pertinent aspects. Nevertheless, to date, there has been a paucity of published literature on discrete GRNs in space and time. In order to address this gap, this paper proposes a discrete-time stochastic fuzzy GRNs framework with discrete spatial diffusions. This constitutes a meaningful contribution to the research field.
This section considers a numerical example of the space-time discretized SF-GRNs with the time delays as noted below
\begin{eqnarray*} \label{exam.dri} \left\{ \begin{array}{lll} {p}_{1, n+1}^{[v]}& = & e^{-0.2h}{p}_{1, n}^{[v]}+\frac{1-e^{-0.2h}}{0.2} \bigg[0.1\Delta_{c, \iota}^{2}{p}_{1, n}^{[v]} +0.1g_2({m}_{2, n-1}^{[v]}) +\bigwedge\limits_{j = 1}^2\zeta_{1j}g_j({m}_{j, n-1}^{[v]})\bigg], \\ {p}_{2, n+1}^{[v]}& = & e^{0.1h}{p}_{2, n}^{[v]}+\frac{e^{0.1h}-1}{0.1} \bigg[0.2\Delta_{c, \iota}^{2}{p}_{2, n}^{[v]} +0.2g_1({m}_{1, n-1}^{[v]}) +0.15\sigma({m}_{1, n}^{[v]})\Delta_h\omega_{n}\bigg], \\ {m}_{1, n+1}^{[v]}& = & e^{-3h}{m}_{1, n}^{[v]}+ \frac{1-e^{-3h}}{3} \bigg[0.2\Delta_{c, \iota}^{2}{m}_{1, n}^{[v]} +0.15{p}_{1, n-2}^{[v]}\bigg], \\[0.2cm] {m}_{2, n+1}^{[v]}& = & e^{-5h}{m}_{2, n}^{[v]}+ \frac{1-e^{-5h}}{5} \bigg[0.1\Delta_{c, \iota}^{2}{m}_{2, n}^{[v]} +0.12{p}_{2, n-2}^{[v]}\bigg], \end{array} \right. \end{eqnarray*} | (4.1) |
where (v, n)\in(0, 3)_{\mathbb{Z}}\times\mathbb{Z}_0; h = 0.05, \iota = 1; g_1(s) = \frac{s^{1.1}}{1+s^{1.1}} = \sigma(s) , g_2(s) = \frac{s^{1.2}}{1+s^{1.2}} , \forall s\in\mathbb{R} ; \zeta_{11} = 0.1 , \zeta_{12} = 0.3 . Besides, the initial boundary values of SF-GRNs (4.1) are described by
\begin{eqnarray*} * \left\{ \begin{array}{ll} {p}_{1, s}^{[v]} = s'|\sin s'|, \, \, {p}_{2, s}^{[v]} = 2s'|\sin s'|, \\[0.25cm] {m}_{1, s}^{[v]} = s''|\sin s''|, \, \, {m}_{2, s}^{[v]} = 2s''|\sin s''|, \quad \forall s\in[-2, 0]_{\mathbb{Z}}, v\in(0, 3)_{\mathbb{Z}}; \\[0.25cm] {p}_{i, n}^{[0]} = 0 = {p}_{i, n}^{[3]}, \, \, {m}_{i, n}^{[0]} = 0 = {m}_{i, n}^{[3]}, \quad \forall n\in\mathbb{Z}_0, i = 1, 2, \end{array} \right. \end{eqnarray*} |
where s' = (s+3)(v+1) and s'' = s+3+v+1 , \forall s\in[-2, 0]_{\mathbb{Z}}, v\in(0, 3)_{\mathbb{Z}}.
In line with the master SF-GRNs (4.1), the slave SF-GRNs are described by
\begin{eqnarray*} \label{exam.res} \left\{ \begin{array}{lll} {q}_{1, n+1}^{[v]}& = & e^{-0.2h}{q}_{1, n}^{[v]}+\frac{1-e^{-0.2h}}{0.2} \bigg[0.1\Delta_{c, \iota}^{2}{q}_{1, n}^{[v]} +0.1g_2({t}_{2, n-1}^{[v]}) +\bigwedge\limits_{j = 1}^2\zeta_{1j}g_j({t}_{j, n-1}^{[v]})\bigg], \\ {q}_{2, n+1}^{[v]}& = & e^{0.1h}{q}_{2, n}^{[v]}+\frac{e^{0.1h}-1}{0.1} \bigg[0.2\Delta_{c, \iota}^{2}{q}_{2, n}^{[v]} +0.2g_1({t}_{1, n-1}^{[v]}) +0.15\sigma({t}_{1, n}^{[v]})\Delta_h\omega_{n}\bigg], \\ {t}_{1, n+1}^{[v]}& = & e^{-3h}{t}_{1, n}^{[v]}+ \frac{1-e^{-3h}}{3} \bigg[0.2\Delta_{c, \iota}^{2}{t}_{1, n}^{[v]} +0.15{q}_{1, n-2}^{[v]}\bigg], \\[0.2cm] {t}_{2, n+1}^{[v]}& = & e^{-5h}{t}_{2, n}^{[v]}+ \frac{1-e^{-5h}}{5} \bigg[0.1\Delta_{c, \iota}^{2}{t}_{2, n}^{[v]} +0.12{q}_{2, n-2}^{[v]}\bigg], \end{array} \right. \end{eqnarray*} | (4.2) |
where (v, n)\in(0, 3)_{\mathbb{Z}}\times\mathbb{Z}_0. Additionally, the initial and boundary values of the slave SF-GRNs (4.2) are depicted as
\begin{eqnarray*} * \label{exam.res.ibv} \left\{ \begin{array}{ll} {q}_{1, s}^{[v]} = s'|\sin s'|+0.1|\mathrm{randn(1)}|, \\[0.25cm] {q}_{2, s}^{[v]} = 2s'|\sin s'|+0.1|\mathrm{randn(1)}|, \\[0.25cm] {t}_{1, s}^{[v]} = s''|\sin s''|+0.1|\mathrm{randn(1)}|, \\[0.25cm] {t}_{2, s}^{[v]} = 2s''|\sin s''|+0.1|\mathrm{randn(1)}|, \quad \forall s\in[-2, 0]_{\mathbb{Z}}, v\in(0, 3)_{\mathbb{Z}}; \\[0.25cm] {q}_{i, n}^{[0]} = 0 = {t}_{i, n}^{[0]}, \, \, {q}_{i, n}^{[3]} = \alpha_{i, n}, {t}_{i, n}^{[3]} = \beta_{i, n}, \quad \forall n\in\mathbb{Z}_0, i = 1, 2. \end{array} \right. \end{eqnarray*} |
Hereby, \mathrm{randn(1)} represents the normally distributed random number with the means of 0 and variance of 1.
Taking \varepsilon = 0.1 , by computing the linear matrix inequalities in Theorem 3.1, it attains
P = \left[ \begin{array}{cc} 119.0654& 0\\ 0& 53.6820\\ \end{array} \right], \quad Q = \left[ \begin{array}{cc} 1836.6232& 0\\ 0 &803.0303\\ \end{array} \right], |
\Pi_1 = \left[ \begin{array}{cc} 46.4409& 0\\ 0& 52.7829\\ \end{array} \right], \quad \Pi_2 = \left[ \begin{array}{cc} 8.9593& 0\\ 0 &2.5156\\ \end{array} \right], |
\Pi_3 = \left[ \begin{array}{cc} 1.1278& 0\\ 0& 0.2857\\ \end{array} \right], \quad Œ_1 = -\left[ \begin{array}{cc} 0.9923& 0\\ 0 &0.9925\\ \end{array} \right], |
Œ_2 = -\left[ \begin{array}{cc} 0.9887& 0\\ 0& 0.9906\\ \end{array} \right], \quad \left[ \begin{array}{cc} \pi_1& \pi_2\\ \pi_3 &\pi_4\\ \end{array} \right] = \left[ \begin{array}{cc} 5.5006& 1817.8828\\ 25.2990 &0.0161\\ \end{array} \right], \quad \lambda_\Omega = 0.4789. |
So, the assumptions in Theorem 3.1 are valid, and the gains of the controls are calculated by
![]() |
According to Theorem 3.1, the master-slave SF-GRNs (4.1) and (4.2) are asymptotically mean-squared finite-time synchronized under the boundary controls below
\begin{eqnarray*} \label{exam.con} \left\{ \begin{array}{lll} {q}_{1, n}^{[3]}& = & -0.6920{u}_{1, n}^{[2]}-1.6920{u}_{1, n}^{[1]}, \\[0.15cm] {q}_{2, n}^{[3]}& = & -0.8351{u}_{2, n}^{[2]}-1.8351{u}_{2, n}^{[1]}, \\[0.15cm] {t}_{1, n}^{[3]}& = & 0.9326{\rho}_{1, n}^{[2]}-0.0674{\rho}_{1, n}^{[1]}, \\[0.15cm] {t}_{2, n}^{[3]}& = & 0.6420{\rho}_{2, n}^{[2]}- 0.3580{\rho}_{2, n}^{[1]}, \quad \forall n\in \mathbb{Z}_0. \end{array} \right. \end{eqnarray*} | (4.3) |
Hereby, u_{i} = q_{i}-p_{i} and \rho_{i} = t_{i}-m_{i} , i = 1, 2.
Figures 1 and 2 illustrate the 3D surfaces of the states in the master GRNs (4.1). The states of the slave GRNs (4.2) are shown in Figures 3 and 4 under the boundary feedback controller (4.3). In Figures 5 and 6, under the boundary feedback controller (4.3), we can see that the errors tend to be small as the time n increases in a finite-time interval. That is, the master-slave SF-GRNs (4.1) and (4.2) achieve global asymptotic finite-time synchronization in the mean-square sense via the boundary feedback controller (4.3). When the spatial variable v = 2 , Figures 7 and 8 show the 2D curves of the errors, which instinctively display the variations of the errors for the readers.
This article examines the subject of global asymptotic or exponential finite-time synchronization in the mean-squared sense of space-time discrete stochastic fuzzy genetic oscillator networks with Dirichlet controlled boundary conditions, accounting for the impact of time delays. By applying the appropriate boundary feedback controls in the slave genetic oscillator networks and utilizing the stochastic theory, this article successfully achieves global asymptotic or exponential finite-time synchronization in the mean-square sense of the space-time discrete genetic oscillator networks. The present study establishes a theoretical framework that will facilitate the global mean-squared finite-time synchronization of genetic oscillator networks within discrete schemes, taking into account both space and time domains. In future works, some interesting topics are worthy of further study, such as fixed/preassigned time stabilization/synchronization [38,39].
Dong Pan: Formal analysis, investigation, conceptualization, methodology, writing-original draft; Huizhen Qu: Funding acquisition, validation, writing-review and editing. All the authors have agreed and given their consent for the publication of this research paper.
The authors declare they have not used Artificial Intelligence (AI) tools in the creation of this article.
This work is supported by Yunnan University Graduate Research Innovation Fund Project under Grant No. KC-23233735.
All authors declare no conflict of interest in this paper.
[1] |
A. S. Ortiz, J. G. Nieto, J. F. A. Montes, I. N. Delgado, Multi-objective context-guided consensus of a massive array of techniques for the inference of gene regulatory networks, Comput. Biol. Med., 179 (2024), 108850. https://doi.org/10.1016/j.compbiomed.2024.108850 doi: 10.1016/j.compbiomed.2024.108850
![]() |
[2] |
G. Ai, C. He, S. T. Bi, Z. R. Zhou, A. K. Liu, X. Hu, et al., Dissecting the molecular basis of spike traits by integrating gene regulatory networks and genetic variation in wheat, Plant Commun., 5 (2024), 100879. https://doi.org/10.1016/j.xplc.2024.100879 doi: 10.1016/j.xplc.2024.100879
![]() |
[3] |
A. A. Brown, J. J. F. Tajes, M. G. Hong, C. A. Brorsson, R. W. Koivula, D, Davtian, et al., Genetic analysis of blood molecular phenotypes reveals common properties in the regulatory networks affecting complex traits, Nat. Commun., 14, (2023) 5062. https://doi.org/10.1038/s41467-023-40569-3 doi: 10.1038/s41467-023-40569-3
![]() |
[4] |
H. Jiang, Y. Wang, C. Yin, H. Pan, L. Chen, K. Feng, et al., SLIVER: Unveiling large scale gene regulatory networks of single-cell transcriptomic data through causal structure learning and modules aggregation, Comput. Biol. Med., 178 (2024), 108690. https://doi.org/10.1016/j.compbiomed.2024.108690 doi: 10.1016/j.compbiomed.2024.108690
![]() |
[5] |
P. J. Wei, J. J. Bao, Z. Gao, J. Y. Tan, R. F. Cao, Y. Su, et al., MEFFGRN: Matrix enhancement and feature fusion-based method for reconstructing the gene regulatory network of epithelioma papulosum cyprini cells by spring viremia of carp virus infection, Comput. biol. med., 179 (2024), 108835. https://doi.org/10.1016/j.compbiomed.2024.108835 doi: 10.1016/j.compbiomed.2024.108835
![]() |
[6] |
C. Wang, C. L. Chen, B. W. Lei, S. H. Qin, Y. Y. Zhang, K. Li, et al., Constructing eRNA-mediated gene regulatory networks to explore the genetic basis of muscle and fat-relevant traits in pigs, Genet. Sel. Evol., 56 (2024), 28. https://doi.org/10.1186/s12711-024-00897-4 doi: 10.1186/s12711-024-00897-4
![]() |
[7] |
M. Kchaou, G. Narayanan, M. S. Ali, S. Sanober, G. Rajchakit, B. Priya, Finite-time Mittag-Leffler synchronization of delayed fractional-order discrete-time complex-valued genetic regulatory networks: Decomposition and direct approaches, Inform. Sciences, 664 (2024), 120337. https://doi.org/10.1016/j.ins.2024.120337 doi: 10.1016/j.ins.2024.120337
![]() |
[8] |
J. Fan, X. Wan, Y. Wu, B. Ruan, Finite-time H_\infty asynchronous state estimation for stochastically switched delayed genetic regulatory networks with sojourn probabilities, J. Franklin I., 361 (2024), 106685. https://doi.org/10.1016/j.jfranklin.2024.106685 doi: 10.1016/j.jfranklin.2024.106685
![]() |
[9] |
S. Rao, X. Lv, Passivity-based control and asymptotic synchronization for multi-variable discrete stochastic genetic regulatory networks with complex network dynamics, Eur. Phys. J. Plus, 139 (2024), 76. https://doi.org/10.1140/epjp/s13360-024-04860-6 doi: 10.1140/epjp/s13360-024-04860-6
![]() |
[10] |
G. Narayanan, M. S. Ali, R. Karthikeyan, G. Rajchakit, A. Jirawattanapanit, Novel adaptive strategies for synchronization control mechanism in nonlinear dynamic fuzzy modeling of fractional-order genetic regulatory networks, Chaos Soliton. Fract., 165 (2022), 112748. https://doi.org/10.1016/j.chaos.2022.112748 doi: 10.1016/j.chaos.2022.112748
![]() |
[11] |
P. Anbalagan, E. Hincal, R. Ramachandran, D. Baleanu, J. Cao, M. Niezabitowski, An asymptotic state estimator design and synchronization criteria for fractional order time-delayed genetic regulatory networks, Asian J. Control, 24 (2022), 3163–3174. https://doi.org/10.1002/asjc.2733 doi: 10.1002/asjc.2733
![]() |
[12] |
M. Zamani, S. Mohammadi, Finite element solution of coupled multiphysics reaction-diffusion equations for fracture healing in hard biological tissues, Comput. Biol. Med., 179 (2024), 108829. https://doi.org/10.1016/j.compbiomed.2024.108829 doi: 10.1016/j.compbiomed.2024.108829
![]() |
[13] |
X. She, L. Wang, Finite-time stability of reaction-diffusion genetic regulatory networks with nondifferential time-varying mixed delays, Math. Method. Appl. Sci., 47 (2024), 7404–7417. https://doi.org/10.1002/mma.9978 doi: 10.1002/mma.9978
![]() |
[14] |
X. Song, X. Li, S. Song, C. K. Ahn, State observer design of coupled genetic regulatory networks with reaction-diffusion terms via time-space sampled-data communications, IEEE-ACM T. Comput. Bi., 19 (2022), 3704–3714. https://doi.org/10.1109/TCBB.2021.3114405 doi: 10.1109/TCBB.2021.3114405
![]() |
[15] | B. Hao, T. W. Zhang, Exponential convergence in the mean-square sense of nonlocal stochastic almost automorphic genetic regulatory lattice networks, T. I. Meas. Control, 46 (2024), 116–130. |
[16] |
S. B. Rao, T. W. Zhang, Almost automorphic behaviours of nonlocal stochastic fuzzy Cohen-Grossberg lattice neural networks, Int. J. Gen. Syst., 53 (2024), 1014–1041. https://doi.org/10.1080/03081079.2024.2340699 doi: 10.1080/03081079.2024.2340699
![]() |
[17] |
X. F. Hu, L. M. Wang, C. K. Zhang, X. B. Wan, Y. He, Fixed-time stabilization of discontinuous spatiotemporal neural networks with time-varying coefficients via aperiodically switching control, Sci. China Inform. Sci., 66 (2023), 152204. https://doi.org/10.1007/s11432-022-3650-8 doi: 10.1007/s11432-022-3650-8
![]() |
[18] |
T. W. Zhang, Z. H. Li, Switching clusters' synchronization for discrete space-time complex dynamical networks via boundary feedback controls, Pattern Recogn., 143 (2023), 109763. https://doi.org/10.1016/j.patcog.2023.109763 doi: 10.1016/j.patcog.2023.109763
![]() |
[19] |
T. W. Zhang, Y. Y. Yang, S. F. Han, Node-to-node clustering asymptotic synchronized discrete stochastic neural networks in time and space with Bernoulli switching delay, Chinese J. Phys., 92 (2024), 743–754. https://doi.org/10.1016/j.cjph.2024.09.007 doi: 10.1016/j.cjph.2024.09.007
![]() |
[20] |
G. Rajchakit, K. A. Banu, T. Aparna, C. P. Lim, Event-triggered secure control for Markov jump neural networks with time-varying delays and subject to cyber-attacks via state estimation fuzzy approach, Int. J. Syst. Sci., 56 (2025), 211–226. https://doi.org/10.1080/00207721.2024.2390694 doi: 10.1080/00207721.2024.2390694
![]() |
[21] |
C. Aouiti, Q. Hui, E. Moulay, F. Touati, Global dissipativity of fuzzy genetic regulatory networks with mixed delays, Int. J. Syst. Sci., 53 (2022), 2644–2663. https://doi.org/10.1080/00207721.2022.2056653 doi: 10.1080/00207721.2022.2056653
![]() |
[22] |
T. W. Zhang, Y. K. Li, Global exponential stability of discrete-time almost automorphic Caputo-Fabrizio BAM fuzzy neural networks via exponential Euler technique, Knowl.-Based Syst., 246 (2022), 108675. https://doi.org/10.1016/j.knosys.2022.108675 doi: 10.1016/j.knosys.2022.108675
![]() |
[23] |
T. W. Zhang, H. Z. Qu, J. W. Zhou, Asymptotically almost periodic synchronization in fuzzy competitive neural networks with Caputo-Fabrizio operator, Fuzzy Set. Syst., 471 (2023), 108676. https://doi.org/10.1016/j.fss.2023.108676 doi: 10.1016/j.fss.2023.108676
![]() |
[24] |
V. Gokulakrishnan, R. Srinivasan, M. S. Ali, G. Rajchakit, B. Priya, Novel LMI-based boundary stabilization of stochastic delayed reaction-diffusion Cohen-Grossberg BAM neural networks with impulsive effects, Neural Process. Lett., 56 (2024), 76. https://doi.org/10.1007/s11063-024-11494-3 doi: 10.1007/s11063-024-11494-3
![]() |
[25] |
J. Wichmann, On temporal regularity of strong solutions to stochastic p-Laplace systems, SIAM J. Math. Anal., 55 (2023), 3713–3730. https://doi.org/10.1137/21M1460491 doi: 10.1137/21M1460491
![]() |
[26] |
F. Tatari, H. Modares, Deterministic and stochastic fixed-time stability of discrete-time autonomous systems, IEEE-CAA J. Automatica, 10 (2023), 945–956. https://doi.org/10.1109/JAS.2023.123405 doi: 10.1109/JAS.2023.123405
![]() |
[27] |
T. W. Zhang, Y. Y. Yang, S. F. Han, Exponential heterogeneous anti-synchronization of multi-variable discrete stochastic inertial neural networks with adaptive corrective parameter, Eng. Appl. Artif. Intel., 142 (2025), 109871. https://doi.org/10.1016/j.engappai.2024.109871 doi: 10.1016/j.engappai.2024.109871
![]() |
[28] |
G. Rajchakit, R. Sriraman, C. P. Lim, P. Sam-ang, P. Hammachukiattikul, Synchronization in finite-time analysis of Clifford-valued neural networks with finite-time distributed delays, Mathematics, 9 (2021), 1163. https://doi.org/10.3390/math9111163 doi: 10.3390/math9111163
![]() |
[29] |
Y. Qin, J. Wang, X. Chen, K. Shi, H. Shen, Anti-disturbance synchronization of fuzzy genetic regulatory networks with reaction-diffusion, J. Franklin I., 359 (2022), 3733–3748. https://doi.org/10.1016/j.jfranklin.2022.03.031 doi: 10.1016/j.jfranklin.2022.03.031
![]() |
[30] |
X. Song, X. Li, C. K. Ahn, S. Song, Space-dividing-based cluster synchronization of reaction-diffusion genetic regulatory networks via intermittent control, IEEE T. Nanobiosci., 21 (2022), 55–64. https://doi.org/10.1109/TNB.2021.3111109 doi: 10.1109/TNB.2021.3111109
![]() |
[31] |
R. Q. Tang, X. S. Yang, P. Shi, Z. R. Xiang, L. B. Qing, Finite-time stabilization of uncertain delayed T-S fuzzy systems via intermittent control, IEEE T. Fuzzy Syst., 32 (2024), 116–125. https://doi.org/10.1109/TFUZZ.2023.3292233 doi: 10.1109/TFUZZ.2023.3292233
![]() |
[32] |
N. Padmaja, P. Balasubramaniam, Mixed H_\infty/passivity based stability analysis of fractional-order gene regulatory networks with variable delays, Math. Comput. Simulat., 192 (2022), 167–181. https://doi.org/10.1016/j.matcom.2020.09.019 doi: 10.1016/j.matcom.2020.09.019
![]() |
[33] |
X. Y. Chen, T. Y. Jia, Z. S. Wang, X. P. Xie, J. L. Qiu, Practical fixed-time bipartite synchronization of uncertain coupled neural networks subject to deception attacks via dual-channel event-triggered control, IEEE T. Cybernetics, 54 (2024), 3615–3625. https://doi.org/10.1109/TCYB.2023.3338165 doi: 10.1109/TCYB.2023.3338165
![]() |
[34] |
X. Y. Chen, H. W. Liu, G. H. Wen, Y. Liu, J. D. Cao, J. L. Qiu, Adaptive neural preassigned-time control for macro-micro composite positioning stage with displacement constraints, IEEE T. Ind. Inform., 20 (2024) 1103–1112. https://doi.org/10.1109/TII.2023.3254602 doi: 10.1109/TII.2023.3254602
![]() |
[35] | S. G. Georgiev, Integral equations on time scales, Dordrecht: Atlantis Press, 2016. |
[36] |
G. V. Milovanović, I. Z. Milovanović, On discrete inequalities of Wirtinger's type, J. Math. Anal. Appl., 88 (1982), 378–387. https://doi.org/10.1016/0022-247X(82)90201-3 doi: 10.1016/0022-247X(82)90201-3
![]() |
[37] |
T. Yang, L. Yang, The global stability of fuzzy cellular neural network, IEEE T. Circuits-I, 43 (1996), 880–883. https://doi.org/10.1109/81.538999 doi: 10.1109/81.538999
![]() |
[38] |
T. T. Cheng, L. M. Wang, Z. C. Wei, G. D. Zhang, Fixed/preassigned-time stabilization of discontinuous switched systems with time-varying delays, Appl. Math. Comput., 476 (2024), 128763. https://doi.org/10.1016/j.amc.2024.128763 doi: 10.1016/j.amc.2024.128763
![]() |
[39] |
X. F. Hu, L. M. Wang, C. K. Zhang, Y. He, Fixed-time synchronization of fuzzy complex dynamical networks with reaction-diffusion terms via intermittent pinning control, IEEE T. Fuzzy Syst., 32 (2024), 2307–2317. https://doi.org/10.1109/TFUZZ.2024.3349599 doi: 10.1109/TFUZZ.2024.3349599
![]() |