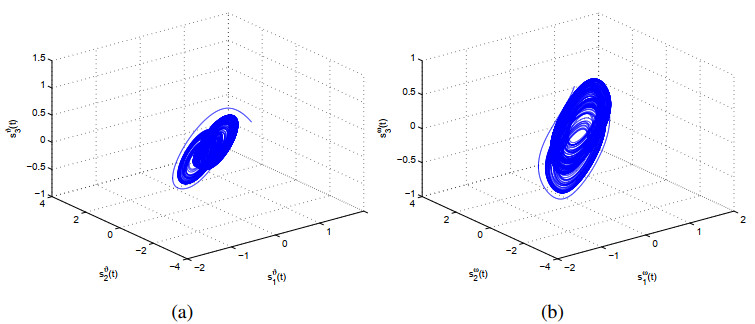
This paper investigates the practical generalized finite-time synchronization (PGFETS) of duplex networks with quantized and delayed couplings. Given that continuous transmission of signals will increase the load and cost of communication, we introduce quantized couplings in the model. Then, via the theorem of finite-time stability, the PGFETS is proposed based on the fact that PGFETS is much more extensive and practical than classical finite-time synchronization. Some sufficient criteria are formulated to achieve the goal of synchronization by utilizing quantized intermittent control schemes. Lastly, the validity of the theoretical results is illustrated by numerical simulations.
Citation: Ting Yang, Li Cao, Wanli Zhang. Practical generalized finite-time synchronization of duplex networks with quantized and delayed couplings via intermittent control[J]. AIMS Mathematics, 2024, 9(8): 20350-20366. doi: 10.3934/math.2024990
[1] | Chengqiang Wang, Xiangqing Zhao, Yang Wang . Finite-time stochastic synchronization of fuzzy bi-directional associative memory neural networks with Markovian switching and mixed time delays via intermittent quantized control. AIMS Mathematics, 2023, 8(2): 4098-4125. doi: 10.3934/math.2023204 |
[2] | Rakkiet Srisuntorn, Wajaree Weera, Thongchai Botmart . Modified function projective synchronization of master-slave neural networks with mixed interval time-varying delays via intermittent feedback control. AIMS Mathematics, 2022, 7(10): 18632-18661. doi: 10.3934/math.20221025 |
[3] | Tao Xie, Xing Xiong . Finite-time synchronization of fractional-order heterogeneous dynamical networks with impulsive interference via aperiodical intermittent control. AIMS Mathematics, 2025, 10(3): 6291-6317. doi: 10.3934/math.2025287 |
[4] | Ailing Li, Xinlu Ye . Finite-time anti-synchronization for delayed inertial neural networks via the fractional and polynomial controllers of time variable. AIMS Mathematics, 2021, 6(8): 8173-8190. doi: 10.3934/math.2021473 |
[5] | Dong Pan, Huizhen Qu . Finite-time boundary synchronization of space-time discretized stochastic fuzzy genetic regulatory networks with time delays. AIMS Mathematics, 2025, 10(2): 2163-2190. doi: 10.3934/math.2025101 |
[6] | Hongguang Fan, Jihong Zhu, Hui Wen . Comparison principle and synchronization analysis of fractional-order complex networks with parameter uncertainties and multiple time delays. AIMS Mathematics, 2022, 7(7): 12981-12999. doi: 10.3934/math.2022719 |
[7] | Changgui Wu, Liang Zhao . Finite-time adaptive dynamic surface control for output feedback nonlinear systems with unmodeled dynamics and quantized input delays. AIMS Mathematics, 2024, 9(11): 31553-31580. doi: 10.3934/math.20241518 |
[8] | Bin Hang, Weiwei Deng . Finite-time adaptive prescribed performance DSC for pure feedback nonlinear systems with input quantization and unmodeled dynamics. AIMS Mathematics, 2024, 9(3): 6803-6831. doi: 10.3934/math.2024332 |
[9] | Chengbo Yi, Rui Guo, Jiayi Cai, Xiaohu Yan . Pinning synchronization of dynamical neural networks with hybrid delays via event-triggered impulsive control. AIMS Mathematics, 2023, 8(10): 25060-25078. doi: 10.3934/math.20231279 |
[10] | N. Jayanthi, R. Santhakumari, Grienggrai Rajchakit, Nattakan Boonsatit, Anuwat Jirawattanapanit . Cluster synchronization of coupled complex-valued neural networks with leakage and time-varying delays in finite-time. AIMS Mathematics, 2023, 8(1): 2018-2043. doi: 10.3934/math.2023104 |
This paper investigates the practical generalized finite-time synchronization (PGFETS) of duplex networks with quantized and delayed couplings. Given that continuous transmission of signals will increase the load and cost of communication, we introduce quantized couplings in the model. Then, via the theorem of finite-time stability, the PGFETS is proposed based on the fact that PGFETS is much more extensive and practical than classical finite-time synchronization. Some sufficient criteria are formulated to achieve the goal of synchronization by utilizing quantized intermittent control schemes. Lastly, the validity of the theoretical results is illustrated by numerical simulations.
In the past, most of the research hotspots of complex networks theory focused on single-layer networks [1,2,3,4]. Considering that single-layer networks cannot describe the structure of two or more interconnected networks, many academics are now focusing on the structural model of multilayer networks [5,6,7,8], which is one of the most active topics in the field of complex networks research in recent years. Among them, the duplex networks (DNs) models can precisely depict the information exchange between two layers and accurately reflect the coupling relationship between layers. For example, Xu et al. [5] investigated the intralayer and interlayer quasi-synchronization of two-layer multi-weighted networks. The authors in [6] analyzed the interlayer synchronization for duplex networks by event-dependent intermittent control. At present, the research on DNs has made important progress and has been excellently applied in social networks analysis, transportation networks, and so on. And because the data information is transmitted over a network link, it is required to be quantized into a limited number of bits before transmission. This promotes us to study the stability and control of DNs with quantized couplings.
Many academics have assumed that all nodes between systems have exactly the same dynamics while studying the dynamical behaviors of complex networks. However, considering the complexity and changing topological nature of network structure, nodes in the networks usually have different dynamics. Thus, generalized synchronization has been proposed to study the synchronization of network systems with the identical or different dynamics, and some theoretical results have been investigated in [9,10,11,12]. Unlike other types of synchronization, generalized synchronization introduces a vector function between the systems, allowing the systems to exhibit richer dynamics. In particular, it can also be used to extend the coexistence of different synchronization types. Yang et al. [9] considered the generalized lag-synchronization of systems with uncertain parameters and unknown perturbations. Zheng et al. [10] investigated the generalized projective lag synchronization criteria of neural networks with delay. Nevertheless, most of the studies mentioned above have focused on the generalized synchronization of single-layer networks, while fewer studies have been conducted on multilayer networks. To our knowledge, in reality the states of systems usually converge to a neighborhood of the origin and not to the origin. From above, it can be seen that it is significant to explore the practical generalized finite-time synchronization (PGFETS) of DNs in this paper.
Obviously, a suitable control scheme is the key to achieve synchronization of the systems, which affects not only the settling time but also the synchronization performance. The control methods that are presented currently contain state feedback control [13], adaptive control [14], event-triggered control [15], intermittent control [16,17,18,19,20], etc., where intermittent control is a typical discontinuous control strategy. It is the discontinuity in working time that makes intermittent control less expensive to control and more suitable for practical applications. Furthermore, we introduce a quantizer in the controller to further conserve communication resources and improve communication efficiency. For example, the finite-time control issue of a class of hybrid systems under quantized intermittent control is proposed in [18]. In [19], the authors discussed the finite-time synchronization of stochastic complex networks via quantized aperiodically intermittent control. However, chattering is inevitable in the controllers with sign function designed above. In conclusion, this paper designs intermittent control methods without sign function, which effectively mitigate chattering phenomenon.
Motivated by the points discussed above, this paper explores the PGFETS of DNs with quantized and delayed couplings via intermittent control, and the following are the main highlights:
(1) To better capture the characteristics of realistic multiplex networks, a class of DNs with quantized and delayed couplings is considered in this study.
(2) Consider that in reality the error system will not converge to the origin ideally, but rather in an interval of the origin. Then, the PGFETS is presented in this paper.
(3) The quantized intermittent control strategies without sign function are utilized in this paper, which save the channel resources and control costs.
The outline of the remaining parts is organized as: Section 2 presents the system model and preliminary works. In Section 3, the PGFETS issue of DNs is discussed, and sufficient conditions are obtained through the control schemes. To verify the validity of the results, numerical examples are shown in Section 4. Finally, Section 5 draws the conclusions.
Notations: In this paper, R,Rn,Rn×m,N denote the set of real numbers, the vector space with n-dimensional, the set of n×m matrices, and the set of natural numbers, respectively. diag(⋅) represents the diagonal matrix. In is the n-dimensional unit matrix. ‖⋅‖ denotes the 2-norm of a vector or matrix. The superscript symbol T and −1 indicate the transposition and inverse. λmax(⋅)(or λmin(⋅)) is the largest (or smallest) eigenvalue of a matrix. ⊗ represents the Kronecker product.
A class of DNs with quantized and delayed couplings under control is constructed below:
{˙ϑı(t)=−Cϑı(t)+Dgϑ(Pϑϑı(t−σϑ1(t)))+ρϑN∑ȷ=1αϑıȷFϑ(ϑȷ(t−σϑ2(t)))+Υ(ωı(t)−ϑı(t))+uϑı(t),˙ωı(t)=−Cωı(t)+Dgω(Pωωı(t−σω1(t)))+ρωN∑ȷ=1αωıȷFω(ωȷ(t−σω2(t)))+Υ(ϑı(t)−ωı(t))+uωı(t), | (2.1) |
where ı,ȷ∈N={1,2,…,N},ϑı(t),ωı(t)∈Rn denote the state vectors of the ıth nodes in ϑ-layer and ω-layer, respectively. C=diag(c1,c2,…,cn), D∈Rn×m. gϑ(ω)(⋅):Rm→Rm is the activation function, where ϑ(ω) denotes ϑ-layer or ω-layer. Fϑ(ω)(⋅) is the quantizer defined below. Pϑ(ω)=[pϑ(ω)1,pϑ(ω)2,…,pϑ(ω)m]T, where pϑ(ω)k∈R1×n,k=1,2,…,m, Pϑϑı(t−σϑ1(t))=[pϑ1ϑı(t−σϑ1(t)),pϑ2ϑı(t−σϑ1(t)),…,pϑmϑı(t−σϑ1(t))]T, Pωωı(t−σω1(t))=[pω1ωı(t−σω1(t)),pω2ωı(t−σω1(t)),…,pωmωı(t−σω1(t))]T. gϑ(Pϑϑı(t−σϑ1(t)))=[gϑ1(pϑ1ϑı(t−σϑ1(t))),gϑ2(pϑ2ϑı(t−σϑ1(t))),…,gϑm(pϑmϑı(t−σϑ1(t)))]T, gω(Pωωı(t−σω1(t))) =[gω1(pω1ωı(t−σω1(t))),gω2(pω2ωı(t−σω1(t))),…,gωm(pωmωı(t−σω1(t)))]T. ρϑ(ω) denotes the coupling strength, the outer coupling matrix of each layer represented by Aϑ(ω)=(αϑ(ω)ıȷ)∈RN×N with αϑ(ω)ıȷ>0(ı≠ȷ) if node ı is related to node ȷ, otherwise, αϑ(ω)ıȷ=0, and αϑ(ω)ıı=−∑Nı=1,ȷ≠ıαϑ(ω)ıȷ. Υ represents the inner coupling matrix of the nodes within two layers. σϑ(ω)1(t) is time-varying delay satisfying 0≤σϑ(ω)1(t)≤σϑ(ω)1,˙σϑ(ω)1(t)≤ˉσϑ(ω)1<1. The coupling delay σϑ(ω)2(t) satisfies 0≤σϑ(ω)2(t)≤σϑ(ω)2, ˙σϑ(ω)2(t)≤ˉσϑ(ω)2<1. Let σϑ(ω)max=max{σϑ(ω)1,σϑ(ω)2}. uϑ(ω)ı(t) is the controller to be determined. The initial conditions of DNs (2.1) are ϑı(t)=φϑı(t)∈C([−σϑmax,0],Rn),ωı(t)=φωı(t)∈C([−σωmax,0],Rn). A quantizer Fϑ(ω)(o):R→Ψ={±γτ:γτ=ℓτγ0,τ=0,±1,±2,…,}∪{0} with γ0>0. For ∀o∈R, the quantizer Fϑ(ω)(o) is designed as:
Fϑ(ω)(o)={γτ,if11+∂γτ<o<11−∂γτ,0,ifo=0,−Fϑ(ω)(−o),ifo<0, |
in which ∂=1−ℓ1+ℓ,0<ℓ<1. Refer to [21], where there is a Filippov solution Λ∈[−∂,∂) that makes Fϑ(ω)(o)=(1+Λ)o. Let Fϑ(ω)(zı)=(Fϑ(ω)(zı1),Fϑ(ω)(zı2),…,Fϑ(ω)(zın))T, |Fϑ(ω)(zı)|χ=(|Fϑ(ω)(zı1)|χ,|Fϑ(ω)(zı2)|χ,…,|Fϑ(ω)(zın)|χ)T for zı∈Rn,χ>0.
It can be said that the networks (2.1) obtain synchronization when the states of nodes in ϑ-layer converges to the identical state sϑ(t), while all states in ω-layer reach the identical state sω(t). Hence, the target systems are modeled as
{˙sϑ(t)=−Csϑ(t)+Dgϑ(Pϑsϑ(t−σϑ1(t)))+Υ(sω(t)−sϑ(t)),˙sω(t)=−Csω(t)+Dgω(Pωsω(t−σω1(t)))+Υ(sϑ(t)−sω(t)), | (2.2) |
with the initial conditions sϑ(t)=Sϑ(t)∈C([−σϑ1,0],Rn),sω(t)=Sω(t)∈C([−σω1,0],Rn), and other parameters remain the same as in systems (2.1).
Remark 1. Systems (2.1) consider the quantized couplings and the different connections of network systems. It is more general than the single-layer networks in [1,2] and two-layer networks without quantized couplings such as [5,6,7].
Below, we provide the assumptions, lemmas, and definitions needed for this article before getting the theoretical results.
Definition 1. The PGFETS between networks (2.1) and (2.2) with respect to the vector map ψ(⋅):Rn→Rn is said to be realized, if there exist constants W>0 and Tf>0 such that
limt→Tf‖ϑı(t)−ψ(sϑ(t))‖≤W,and‖ϑı(t)−ψ(sϑ(t))‖≤W,fort>Tf,limt→Tf‖ωı(t)−ψ(sω(t))|≤W,and‖ωı(t)−ψ(sω(t))‖≤W,fort>Tf, |
where ı∈N, Tf is the settling time.
Remark 2. From Definition 1, it is clear that the finite-time synchronization in [3,4,5] is a special case of generalized finite-time synchronization when ψ(sϑ(ω)(t))=sϑ(ω)(t). However, it is not applicable for system nodes with different dynamic characteristics in reality. Consequently, the study of PGFETS is of more practical interest.
Assumption 1. For gϑ(ω)(⋅):Rn→Rn, there are nonnegative constants Lϑ and Lω that meet
‖gϑ(ϑı(t))−gϑ(sϑ(t))‖≤Lϑ‖ϑı(t)−sϑ(t)‖,‖gω(ωı(t))−gω(sω(t))‖≤Lω‖ωı(t)−sω(t)‖. |
Assumption 2. Let 0<q<1 and ε>0, then there is a continuous function f:[0,∞)→[0,∞) with f(0)≥0, for any 0≤μ≤t, that satisfies
f(t)−f(μ)≤−ε∫tt−μ(f(s))qds. |
Lemma 1. [22] There is a positive definite matrix Q∈Rn×n such that
2hT1Qh2≤hT1Qh1+hT2Q−1h2, |
for any h1,h2∈Rn.
Lemma 2. [23] Given that ν1,ν2,…,νN≥0,0<ζ1≤1,ζ2>1, then
N∑ı=1νζ1ı≥(N∑ı=1νı)ζ1,andN∑ı=1νζ2ı≥N1−ζ2(N∑ı=1νı)ζ2. |
Lemma 3. [24] For any ℵ∈R,β≫1, there is
0≤|ℵ|≤ℵtanh(βℵ)+♭β, |
where ♭=0.2785.
Lemma 4. [25] For y1,y2,…,yN∈Rn, there exists 0<p<2, which satisfies
‖y1‖p+‖y2‖p+⋯+‖yN‖p≥(‖y1‖2+‖y2‖2+⋯+‖yN‖2)p2. |
Definition 2. [26] Function V(x):Rn→R is called C-regular if V(x) is:
(ⅰ) regular in Rn;
(ⅱ) positive definite, i.e., V(x)>0 for x≠0 and V(0)=0;
(ⅲ) radially unbounded, i.e., V(x)→+∞ as ‖x‖→+∞.
Lemma 5. [27] Suppose that there exists a strict increasing time sequence {tk}k∈N, which satisfies t0=0,limk→+∞tk=+∞. Let us take £↓k=[t2k,t2k+1),£↑k=[t2k+1,t2k+2),ℑ↓k=t2k+1−t2k, ℑ↑k=t2k+2−t2k+1, and V(t):Rn→R is C-regular, satisfying:
˙V(t)≤{−ϱ1V(t)−ϱ2V℘(t)+ϱ0,t∈£↓k,ϱ3V(t)+ϱ0,t∈£↑k, |
in which t∈[0,+∞), ℘∈(0,1), ϱ0, ϱ1, ϱ2, and ϱ3 are positive constants. Furthermore, if there exists a k∈N such that
Γ(k)=(Ξ(k−1)+Δϵ)exp(−ϕ1ℑ↓k)−Δϵ≤0, |
then there exists a constant Tf>0 satisfying V(t)≤(ϱ0ϱ2(1−δ))1℘ for t>Tf, and
Tf=t2k∗+1ϕ1ln(1ΔϵΞ(k∗−1)+1), |
where ϕ1=(1−℘)ϱ1, ϕ2=(1−℘)ϱ2δ, ϕ3=(1−℘)ϱ3, ϕ4=(1−℘)ϱ2, δ∈(0,1), ϵ1=ϕ2ϕ1, ϵ2=ϕ4ϕ3, and ϵ1>ϵ2 with Δϵ=ϵ1−ϵ2, Ξ(k−1)=(V1−℘(0)+ϵ1)exp(∑k−1q=0(−ϕ1(t2q+1−t2q)+ϕ3(t2q+2−t2q+1))) with t−2=t−1=t0=0, k∗=min{k∈N:Γ(k)≤0}.
Based on the Lyapunov stability theorem, the PGFETS criteria of DNs are established by utilizing intermittent controllers in this part.
Let ςϑı(t)=(ςϑı1(t),ςϑı2(t),…,ςϑın(t))T=ϑı(t)−ψ(sϑ(t)), ςωı(t)=(ςωı1(t),ςωı2(t),…,ςωın(t))T=ωı(t)−ψ(sω(t))∈Rn, and combine systems (2.1) and (2.2). The error systems are deduced as
{˙ςϑı(t)=˙ϑı(t)−Πϑψ˙sϑ(t)=−Cϑı(t)+Dgϑ(Pϑϑı(t−σϑ1(t)))+ρϑN∑ȷ=1αϑıȷFϑ(ϑȷ(t−σϑ2(t)))+Υ(ωı(t)−ϑı(t))−Πϑψ(−Csϑ(t)+Dgϑ(Pϑsϑ(t−σϑ1(t)))+Υ(sω(t)−sϑ(t)))+uϑı(t),˙ςωı(t)=˙ωı(t)−Πωψ˙sω(t)=−Cωı(t)+Dgω(Pωωı(t−σω1(t)))+ρωN∑ȷ=1αωıȷFω(ωȷ(t−σω2(t)))+Υ(ϑı(t)−ωı(t))−Πωψ(−Csω(t)+Dgω(Pωsω(t−σω1(t)))+Υ(sϑ(t)−sω(t)))+uωı(t), | (3.1) |
where Πϑψ,Πωψ are the Jacobian matrices of the functions ψ(sϑ(t)) and ψ(sω(t)), respectively, which are defined as
Πϑψ=[∂ψ1(sϑ(t))∂sϑ1(t)∂ψ1(sϑ(t))∂sϑ2(t)…∂ψ1(sϑ(t))∂sϑn(t)∂ψ2(sϑ(t))∂sϑ1(t)∂ψ2(sϑ(t))∂sϑ2(t)…∂ψ2(sϑ(t))∂sϑn(t)⋮⋮⋱⋮∂ψn(sϑ(t))∂sϑ1(t)∂ψn(sϑ(t))∂sϑ2(t)…∂ψn(sϑ(t))∂sϑn(t)],Πωψ=[∂ψ1(sω(t))∂sω1(t)∂ψ1(sω(t))∂sω2(t)…∂ψ1(sω(t))∂sωn(t)∂ψ2(sω(t))∂sω1(t)∂ψ2(sω(t))∂sω2(t)…∂ψ2(sω(t))∂sωn(t)⋮⋮⋱⋮∂ψn(sω(t))∂sω1(t)∂ψn(sω(t))∂sω2(t)…∂ψn(sω(t))∂sωn(t)]. |
The intermittent controllers are formulated as
{uϑı(t)={−Θϑ(t)−ℜϑ1Fϑ(ςϑı(t))−ηϑ1(Fϑ(ςϑı(t)))χ1χ2−ηϑ2tanh(βFϑ(ςϑı(t))),t∈£↓k,−Θϑ(t)−ℜϑ2Fϑ(ςϑı(t))−ηϑ2tanh(βFϑ(ςϑı(t))),t∈£↑k,uωı(t)={−Θω(t)−ℜω1Fω(ςωı(t))−ηω1(Fω(ςωı(t)))χ1χ2−ηω2tanh(βFω(ςωı(t))),t∈£↓k,−Θω(t)−ℜω2Fω(ςωı(t))−ηω2tanh(βFω(ςωı(t))),t∈£↑k, | (3.2) |
where ηϑ(ω)1, ηϑ(ω)2, ℜϑ(ω)1,ℜϑ(ω)2 are positive constants, χ1<χ2, χ1,χ2 are positive odd constants, β is defined as in Lemma 3, and £↓k, £↑k are the same as that in Lemma 5. Take Θϑ(t)=−Cψ(sϑ(t))+Dgϑ(ψ(Pϑsϑ(t−σϑ1(t))))+Υ(ψ(sω(t))−ψ(sϑ(t)))−Πϑψ(−Csϑ(t)+Dgϑ(Pϑsϑ(t−σϑ1(t)))+Υ(sω(t)−sϑ(t))), Θω(t)=−Cψ(sω(t))+Dgω(ψ(Pωsω(t−σω1(t))))+Υ(ψ(sϑ(t))−ψ(sω(t)))−Πωψ(−Csω(t)+Dgω(Pωsω(t−σω1(t)))+Υ(sϑ(t)−sω(t))).
Remark 3. It is worth mentioning that compared with the intermittent control in [16,17], the above controllers (3.2) are without sign function, which effectively weakens the chattering phenomenon caused by the sign function. In addition, considering the quantization of information effectively reduces the control cost and alleviates the communication congestion.
Let ˉgϑ(Pϑςϑı(t−σϑ1(t)))=gϑ(Pϑϑı(t−σϑ1(t)))−gϑ(ψ(Pϑsϑ(t−σϑ1(t)))), ˉgω(Pωςωı(t−σω1(t)))=gω(Pωωı(t−σω1(t)))−gω(ψ(Pωsω(t−σω1(t)))), ˉFϑ(ςϑȷ(t−σϑ2(t)))=Fϑ(ϑȷ(t−σϑ2(t)))−Fϑ(ψ(s(t−σϑ2(t)))), ˉFω(ςωȷ(t−σω2(t)))=Fω(ωȷ(t−σω2(t)))−Fω(ψ(s(t−σω2(t)))), then systems (3.1) can be converted into
{˙ςϑı(t)={−Cςϑı(t)+Dˉgϑ(Pϑςϑı(t−σϑ1(t)))+ρϑN∑ȷ=1αϑıȷˉFϑ(ςϑȷ(t−σϑ2(t)))+Υ(ςωı(t)−ςϑı(t))−ℜϑ1Fϑ(ςϑı(t))−ηϑ1(Fϑ(ςϑı(t)))χ1χ2−ηϑ2tanh(βFϑ(ςϑı(t))),t∈£↓k,−Cςϑı(t)+Dˉgϑ(Pϑςϑı(t−σϑ1(t)))+ρϑN∑ȷ=1αϑıȷˉFϑ(ςϑȷ(t−σϑ2(t)))+Υ(ςωı(t)−ςϑı(t))−ℜϑ2Fϑ(ςϑı(t))−ηϑ2tanh(βFϑ(ςϑı(t))),t∈£↑k,˙ςωı(t)={−Cςωı(t)+Dˉgω(Pωςωı(t−σω1(t)))+ρωN∑ȷ=1αωıȷˉFω(ςωȷ(t−σω2(t)))+Υ(ςϑı(t)−ςωı(t))−ℜω1Fω(ςωı(t))−ηω1(Fω(ςωı(t)))χ1χ2−ηω2tanh(βFω(ςωı(t))),t∈£↓k,−Cςωı(t)+Dˉgω(Pωςωı(t−σω1(t)))+ρωN∑ȷ=1αϑıȷˉFω(ςωȷ(t−σω2(t)))+Υ(ςϑı(t)−ςωı(t))−ℜω2Fω(ςωı(t))−ηω2tanh(βFω(ςωı(t))),t∈£↑k. | (3.3) |
Theorem 1. Under Assumptions 1−2, there exist positive constants ϱ0, ϱ1, ϱ2, ϱ3, ε, δ∈(0,1), ℜϑ(ω)1, ℜϑ(ω)2, rϑ(ω)i, ˉrϑ(ω)i(i=1,2), and a k∈N, and the following factors are fulfilled:
Γ(k)=(Ξ(k−1)+Δϵ)exp(−ϕ1ℑ↓k)−Δϵ≤0, | (3.4) |
ϱ1−2λmin(C)+1+ρϑ(ω)(1+∂)+1−λmin(ΥT+Υ)+λmax(ΥTΥ)+rϑ(ω)1exp(ϱ1σϑ(ω)1)−2ˉrϑ(ω)1ε+rϑ(ω)2exp(ϱ1σϑ(ω)2)−2ˉrϑ(ω)2ε−2ℜϑ(ω)1(1−∂)≤0, | (3.5) |
(Lϑ(ω))2λmax(DTD)‖Pϑ(ω)‖2−rϑ(ω)1(1−ˉσϑ(ω)1)+2ˉrϑ(ω)1ε≤0, | (3.6) |
ρϑ(ω)(1+∂)λmax(Gϑ(ω))−rϑ(ω)2(1−ˉσϑ(ω)2)+2ˉrϑ(ω)2ε≤0, | (3.7) |
−ϱ3−2λmin(C)+1+ρϑ(ω)(1+∂)+1−λmin(ΥT+Υ)+λmax(ΥTΥ)+rϑ(ω)1exp(ϱ1σϑ(ω)1)+rϑ(ω)2exp(ϱ1σϑ(ω)2)−2ℜϑ(ω)2(1−∂)≤0, | (3.8) |
(Lϑ(ω))2λmax(DTD)‖Pϑ(ω)‖2−rϑ(ω)1(1−ˉσϑ(ω)1)≤0, | (3.9) |
ρϑ(ω)(1+∂)λmax(Gϑ(ω))−rϑ(ω)2(1−ˉσϑ(ω)2)≤0, | (3.10) |
where Gϑ(ω)=(Aϑ(ω))TAϑ(ω)⊗In, ϱ0=nN♭(ηϑ2+ηω2)β, ϱ2=min{2χ1+χ22χ2ηϑ1(1−∂)χ1χ2,2χ1+χ22χ2ηω1(1−∂)χ1χ2, ˉrϑ12χ1+χ22χ2(rϑ1exp(ϱ1σϑ1))−χ1+χ22χ2, ˉrϑ22χ1+χ22χ2(rϑ2exp(ϱ1σϑ2))−χ1+χ22χ2, ˉrω12χ1+χ22χ2(rω1exp(ϱ1σω1))−χ1+χ22χ2, ˉrω22χ1+χ22χ2(rω2exp(ϱ1σω2))−χ1+χ22χ2}. Moreover, ℑ↓k, ℑ↑k, ϕ1, ϕ2, ϕ3, ϕ4, ϵ1, ϵ2, Δϵ, Ξ(k−1), k∗ are defined as those in Lemma 5 and ℘=χ1+χ22χ2. Then, networks (2.1) and networks (2.2) are able to achieve PGFETS through the controllers (3.2), and the settling time is Tf=t2k∗+1ϕ1ln(1ΔϵΞ(k∗−1)+1).
Proof. Construct the Lyapunov functional as:
V(t)=V1(t)+V2(t)+V3(t)+V4(t)+V5(t)+V6(t),V1(t)=12N∑ı=1(ςϑı(t))Tςϑı(t),V2(t)=12N∑ı=1(ςωı(t))Tςωı(t),V3(t)=12N∑ı=1rϑ1exp(ϱ1σϑ1)∫tt−σϑ1(t)exp(ϱ1(s−t))(ςϑı(s))Tςϑı(s)ds,V4(t)=12N∑ı=1rϑ2exp(ϱ1σϑ2)∫tt−σϑ2(t)exp(ϱ1(s−t))(ςϑı(s))Tςϑı(s)ds,V5(t)=12N∑ı=1rω1exp(ϱ1σω1)∫tt−σω1(t)exp(ϱ1(s−t))(ςωı(s))Tςωı(s)ds,V6(t)=12N∑ı=1rω2exp(ϱ1σω2)∫tt−σω2(t)exp(ϱ1(s−t))(ςωı(s))Tςωı(s)ds. | (3.11) |
When t∈£↓k, differentiating V1(t) yields
˙V1(t)=N∑ı=1(ςϑı(t))T˙ςϑı(t)−ϱ1V1(t)+ϱ12N∑ı=1(ςϑı(t))Tςϑı(t)=−ϱ1V1(t)+ϱ12N∑ı=1(ςϑı(t))Tςϑı(t)+N∑ı=1(ςϑı(t))T(−Cςϑı(t)+Dˉgϑ(Pϑςϑı(t−σϑ1(t)))+ρϑN∑ȷ=1αϑıȷˉFϑ(ςϑȷ(t−σϑ2(t)))+Υ(ςωı(t)−ςϑı(t))−ℜϑ1Fϑ(ςϑı(t))−ηϑ1(Fϑ(ςωı(t)))χ1χ2−ηϑ2tanh(βFϑ(ςϑı(t)))). | (3.12) |
It's obvious to gain
N∑ı=1(ςϑı(t))T(−Cςϑı(t))≤−λmin(C)N∑ı=1‖ςϑı(t)‖2. | (3.13) |
By Assumption 1 and Lemma 1, it has
N∑ı=1(ςϑı(t))TDˉgϑ(Pϑςϑı(t−σϑ1(t)))≤12N∑ı=1(‖ςϑı(t)‖2+(Lϑ)2λmax(DTD)‖Pϑ‖2‖ςϑı(t−σϑ1(t))‖2). | (3.14) |
Moreover, it derives from Lemma 1 that
N∑ı=1(ςϑı(t))TρϑN∑ȷ=1αϑıȷˉFϑ(ςϑȷ(t−σϑ2(t)))≤N∑ı=1N∑ȷ=1ρϑ(1+∂)(ςϑı(t))Tαϑıȷςϑȷ(t−σϑ2(t))=ρϑ(1+∂)(ςϑ(t))T(Aϑ⊗In)ςϑ(t−σϑ2(t))≤ρϑ(1+∂)12(ςϑ(t))Tςϑ(t)+ρϑ(1+∂)12(ςϑ(t−σϑ2(t)))T((Aϑ)TAϑ⊗In)ςϑ(t−σϑ2(t))≤ρϑ(1+∂)2N∑ı=1‖ςϑı(t)‖2+ρϑ(1+∂)2λmax(Gϑ)N∑ı=1‖ςϑı(t−σϑ2(t))‖2, | (3.15) |
where ς(t)=(ςT1(t),ςT2(t),…,ςTN(t))T.
Same as above, a simple derivation yields
N∑ı=1(ςϑı(t))TΥ(ςωı(t)−ςϑı(t))=N∑ı=1(ςϑı(t))TΥςωı(t)−N∑ı=1(ςϑı(t))TΥςϑı(t)≤12N∑ı=1((ςϑı(t))Tςϑı(t)+(ςωı(t))TΥTΥςωı(t))−12N∑ı=1(ςϑı(t))T(ΥT+Υ)ςϑı(t)≤12(1−λmin(ΥT+Υ))N∑ı=1‖ςϑı(t)‖2+12λmax(ΥTΥ)N∑ı=1‖ςωı(t)‖2. | (3.16) |
It's not hard to get
−N∑ı=1(ςϑı(t))Tℜϑ1Fϑ(ςϑı(t))≤−ℜϑ1(1−∂)N∑ı=1‖ςϑı(t)‖2. | (3.17) |
Employing Lemma 2 and 0<χ1+χ22χ2<1, it follows that
−N∑ı=1(ςϑı(t))Tηϑ1(Fϑ(ςϑı(t)))χ1χ2≤−ηϑ1(1−∂)χ1χ2(N∑ı=1n∑k=1(ςϑık(t))2)χ1+χ22χ2=−2χ1+χ22χ2ηϑ1(1−∂)χ1χ2(12N∑ı=1‖ςϑı(t)‖2)χ1+χ22χ2. | (3.18) |
By simple calculation via Lemma 3, we get
−N∑ı=1(ςϑı(t))Tηϑ2tanh(βFϑ(ςϑı(t)))≤−ηϑ2N∑ı=1n∑k=1(ςϑık(t)tanh(β(1−∂)ςϑık(t)))≤ηϑ2(nN♭β(1−∂)−N∑ı=1n∑k=1|ςϑık(t)|)≤−√2ηϑ2(12N∑ı=1‖ςϑı(t)‖2)12+nN♭ηϑ2β(1−∂). | (3.19) |
The inequalities (3.13)–(3.19) guarantee that
˙V1(t)≤−ϱ1V1(t)+12N∑ı=1[ϱ1−2λmin(C)+1+ρϑ(1+∂)+1−λmin(ΥT+Υ)−2ℜϑ1(1−∂)]‖ςϑı(t)‖2+12λmax(ΥTΥ)N∑ı=1‖ςωı(t)‖2+12(Lϑ)2λmax(DTD)‖Pϑ‖2N∑ı=1‖ςϑı(t−σϑ1(t))‖2+ρϑ(1+∂)2λmax(Gϑ)N∑ı=1‖ςϑı(t−σϑ2(t))‖2−√2ηϑ2(12N∑ı=1‖ςϑı(t)‖2)12+nN♭ηϑ2β(1−∂)−2χ1+χ22χ2ηϑ1(1−∂)χ1χ2(12N∑ı=1‖ςϑı(t)‖2)χ1+χ22χ2. | (3.20) |
Similar to V1(t),
˙V2(t)≤−ϱ1V2(t)+12N∑ı=1[ϱ1−2λmin(C)+1+ρω(1+∂)+1−λmin(ΥT+Υ)−2ℜω1(1−∂)]‖ςωı(t)‖2+12λmax(ΥTΥ)N∑ı=1‖ςϑı(t)‖2+12(Lω)2λmax(DTD)‖Pω‖2N∑ı=1‖ςωı(t−σω1(t))‖2+ρω(1+∂)2λmax(Gω)N∑ı=1‖ςωı(t−σω2(t))‖2−√2ηω2(12N∑ı=1‖ςωı(t)‖2)12+nN♭ηω2β(1−∂)−2χ1+χ22χ2ηω1(1−∂)χ1χ2(12N∑ı=1‖ςωı(t)‖2)χ1+χ22χ2. | (3.21) |
Next, take the derivative of V3(t), which reaches that
˙V3(t)≤−ϱ1V3(t)+12N∑ı=1(rϑ1exp(ϱ1σϑ1)(‖ςϑı(t)‖2−exp(−ϱ1σϑ1)(1−ˉσϑ1)‖ςϑı(t−σϑ1(t))‖2))=−ϱ1V3(t)+12N∑ı=1(rϑ1exp(ϱ1σϑ1)‖ςϑı(t)‖2−rϑ1(1−ˉσϑ1)‖ςϑı(t−σϑ1(t))‖2). | (3.22) |
Then, by Assumption 2 and Lemma 4, we get
ˉrϑ1εN∑ı=1((ςϑı(t))Tςϑı(t)−(ςϑı(t−σϑ1(t)))Tςϑı(t−σϑ1(t)))≤−ˉrϑ1(N∑ı=1∫tt−σϑ1(t)(ςϑı(s))Tςϑı(s)ds)χ1+χ22χ2. | (3.23) |
Combining (3.22) and (3.23) yields
˙V3(t)≤−ϱ1V3(t)+12N∑ı=1(rϑ1exp(ϱ1σϑ1)‖ςϑı(t)‖2−rϑ1(1−ˉσϑ1)‖ςϑı(t−σϑ1(t))‖2)−ˉrϑ1εN∑ı=1(‖ςϑı(t)‖2−‖ςı(t−σϑ1(t))‖2)−ˉrϑ1(N∑ı=1∫tt−σϑ1(t)(ςϑı(s))Tςϑı(s)ds)χ1+χ22χ2. | (3.24) |
With the same analysis as above, we obtain
˙V4(t)≤−ϱ1V4(t)+12N∑ı=1((rϑ2exp(ϱ1σϑ2)−2ˉrϑ2ε)‖ςϑı(t)‖2−(rϑ2(1−ˉσϑ2)−2ˉrϑ2ε)‖ςϑı(t−σϑ2(t))‖2)−ˉrϑ2(N∑ı=1∫tt−σϑ2(t)(ςϑı(s))Tςϑı(s)ds)χ1+χ22χ2, | (3.25) |
˙V5(t)≤−ϱ1V5(t)+12N∑ı=1((rω1exp(ϱ1σω1)−2ˉrω1ε)‖ςωı(t)‖2−(rω1(1−ˉσω1)−2ˉrω1ε)‖ςωı(t−σω1(t))‖2)−ˉrω1(N∑ı=1∫tt−σω1(t)(ςωı(s))Tςωı(s)ds)χ1+χ22χ2, | (3.26) |
˙V6(t)≤−ϱ1V6(t)+12N∑ı=1((rω2exp(ϱ1σω2)−2ˉrω2ε)‖ςωı(t)‖2−(rω2(1−ˉσω2)−2ˉrω2ε)N∑ı=1‖ςωı(t−σω2(t))‖2)−ˉrω2(N∑ı=1∫tt−σω2(t)(ςωı(s))Tςωı(s)ds)χ1+χ22χ2. | (3.27) |
Refer to conditions (3.5)–(3.7), formulas (3.20)–(3.21) and (3.24)–(3.27), we have
˙V(t)=6∑i=1˙Vi(t)≤−ϱ1V1(t)+12N∑ı=1[ϱ1−2λmin(C)+1+ρϑ(1+∂)+1−λmin(ΥT+Υ)+λmax(ΥTΥ)+rϑ1exp(ϱ1σϑ1)−2ˉrϑ1ε+rϑ2exp(ϱ1σϑ2)−2ˉrϑ2ε−2ℜϑ1(1−∂)]‖ςϑı(t)‖2+12[(Lϑ)2λmax(DTD)‖Pϑ‖2−rϑ1(1−ˉσϑ1)+2ˉrϑ1ε]N∑ı=1‖ςϑı(t−σϑ1(t))‖2+12[ρϑ(1+∂)λmax(Gϑ)−rϑ2(1−ˉσϑ2)+2ˉrϑ2ε]N∑ı=1‖ςϑı(t−σϑ2(t))‖2−√2ηϑ2(12N∑ı=1‖ςϑı(t)‖2)12+nN♭ηϑ2β(1−∂)−2χ1+χ22χ2ηϑ1(1−∂)χ1χ2Vχ1+χ22χ21(t)−ϱ1V2(t)+12N∑ı=1[ϱ1−2λmin(C)+1+ρω(1+∂)+1−λmin(ΥT+Υ)+λmax(ΥTΥ)+rω1exp(ϱ1σω1)−2ˉrω1ε+rω2exp(ϱ1σω2)−2ˉrω2ε−2ℜω1(1−∂)]‖ςωı(t)‖2+12[(Lω)2λmax(DTD)‖Pω‖2−rω1(1−ˉσω1)+2ˉrω1ε]N∑ı=1‖ςωı(t−σω1(t))‖2+12[ρω(1+∂)λmax(Gω)−rω2(1−ˉσω2)+2ˉrω2ε]N∑ı=1‖ςωı(t−σω2(t))‖2−√2ηω2(12N∑ı=1‖ςωı(t)‖2)12+nN♭ηω2β(1−∂)−2χ1+χ22χ2ηω1(1−∂)χ1χ2Vχ1+χ22χ22(t)−ϱ1V3(t)−ˉrϑ12χ1+χ22χ2(rϑ1exp(ϱ1σϑ1))−χ1+χ22χ2Vχ1+χ22χ23(t)−ϱ1V4(t)−ˉrϑ22χ1+χ22χ2(rϑ2exp(ϱ1σϑ2))−χ1+χ22χ2Vχ1+χ22χ24(t)−ϱ1V5(t)−ˉrω12χ1+χ22χ2(rω1exp(ϱ1σω1))−χ1+χ22χ2Vχ1+χ22χ25(t)−ϱ1V6(t)−ˉrω22χ1+χ22χ2(rω2exp(ϱ1σω2))−χ1+χ22χ2Vχ1+χ22χ26(t)≤−ϱ1V(t)−ϱ2Vχ1+χ22χ2(t)+ϱ0. | (3.28) |
With the same analytical procedure as above, for t∈£↑k, in view of conditions (3.8)–(3.10), it is granted that
˙V(t)=6∑i=1˙Vi(t)≤−ϱ1V(t)+(ϱ1+ϱ3)V1(t)+(ϱ1+ϱ3)V2(t)+12N∑ı=1[−ϱ3−2λmin(C)+1+ρϑ(1+∂)+1−λmin(ΥT+Υ)+λmax(ΥTΥ)+rϑ1exp(ϱ1σϑ1)+rϑ2exp(ϱ1σϑ2)−2ℜϑ2(1−∂)]‖ςϑı(t)‖2+12[(Lϑ)2λmax(DTD)‖Pϑ‖2−rϑ1(1−ˉσϑ1)]N∑ı=1‖ςϑı(t−σϑ1(t))‖2+12[ρϑ(1+∂)λmax(Gϑ)−rϑ2(1−ˉσϑ2)]N∑ı=1‖ςϑı(t−σϑ2(t))‖2−√2ηϑ2(12N∑ı=1‖ςϑı(t)‖2)12+nN♭ηϑ2β(1−∂)+12N∑ı=1[−ϱ3−2λmin(C)+1+ρω(1+∂)+1−λmin(ΥT+Υ)+λmax(ΥTΥ)+rω1exp(ϱ1σω1)+rω2exp(ϱ1σω2)−2ℜω2(1−∂)]‖ςωı(t)‖2+12[(Lω)2λmax(DTD)‖Pω‖2−rω1(1−ˉσω1)]N∑ı=1‖ςωı(t−σω1(t))‖2+12[ρω(1+∂)λmax(Gω)−rω2(1−ˉσω2)]N∑ı=1‖ςωı(t−σω2(t))‖2−√2ηω2(12N∑ı=1‖ςωı(t)‖2)12+nN♭ηω2β(1−∂)≤−ϱ1V(t)+(ϱ1+ϱ3)V(t)+ϱ0=ϱ3V(t)+ϱ0. | (3.29) |
In view of Lemma 5, condition (3.4), and inequalities (3.28)–(3.29), one has V(t)≤(ϱ0ϱ2(1−δ))2χ2χ1+χ2, then V1(t)≤(ϱ0ϱ2(1−δ))2χ2χ1+χ2 and V2(t)≤(ϱ0ϱ2(1−δ))2χ2χ1+χ2, i.e., ‖ςϑı(t)‖≤√2(ϱ0ϱ2(1−δ))χ2χ1+χ2, ‖ςωı(t)‖≤√2(ϱ0ϱ2(1−δ))χ2χ1+χ2,ı∈N for t>Tf. It follows from Definition 1 that there is a settling time Tf at which the PGFETS of systems (2.1) and (2.2) is ensured. This concludes the proof.
Remark 4. Note that the two-layer networks have been studied in [6,7], and they belong to the problem of finite time synchronization for the error system, i.e., limt→TfV(t)=0, Tf is a settling time. Nevertheless, real systems cannot be strictly synchronized by various external factors, and the system states may oscillate in a certain region. Hence, the results in this paper are more consistent with real systems.
Corollary 1. Suppose Assumptions 1–2 hold if the mapping function ψ(sϑ(ω)(t))=Hsϑ(ω)(t), where H is a nonzero constant. Given positive constants ϱ0, ϱ1, ϱ2, ϱ3, ε, δ∈(0,1), ℜϑ(ω)1, ℜϑ(ω)2, rϑ(ω)i, ˉrϑ(ω)i(i=1,2), and a k∈N such that (3.4)–(3.10) hold, the parameters are similar to those in Theorem 3.1. Then, DNs (2.1) and (2.2) under controllers (3.2) can achieve finite-time projective synchronization. Moreover, it is a special case when H=−1, and the finite-time anti-synchronization is reached.
A numerical simulation is cited to corroborate the validity of Theorem 3.1. Take n=m=3,N=5, the time step is 0.001, and consider the systems (2.2) with sϑ(0)=(0.8,−0.5,0.4)T, sω(0)=(−0.2,−0.3,0.8)T. σϑ1(t)=σω1(t)=0.01. One has σϑ1=σω1=0.01,ˉσϑ1=ˉσω1=0. gϑ(x)=(tanh(x1),sin(x2),−1.5tanh(x3))T, while gω(x)=(tanh(x1),sin(x2),−2.5tanh(x3))T for x=(x1,x2,x3)T, thus Assumption 1 is satisfied with Lϑ=1.5,Lω=2.5. C=diag(1.9,1.5,1), Υ=diag(1,1,1), Pϑ=diag(1,1,1),Pω=diag(1,0,1), and
D=(2.5021.61.92.91.5−1.5−0.8). |
The trajectories of DNs (2.2) are demonstrated in Figure 1. Obviously, they are both chaotic.
In this paper, we consider DNs (2.1) with five nodes, the parameters are taken as ρϑ=5, ρω=6, σϑ2(t)=σω2(t)=0.02 with σϑ2=σω2=0.02,ˉσϑ2=ˉσω2=0,
Aϑ=(−0.150.1000.050.05−0.150.050.05000.15−0.30.15000.050.15−0.250.050.2000.05−0.25),Aω=(−0.150.1000.050.05−0.10.050000.05−0.10.050000.05−0.0500.05000−0.05), |
and others are consistent with systems (2.2). Then, we consider the PGFETS between DNs (2.1) and (2.2) with ψ(sϑ(ω)(t))=((sϑ(ω)1(t))2,(sϑ(ω)2(t))2,(sϑ(ω)3(t))2)T. There is a sequence {tk}k∈N satisfying the first control width t2k+1−t2k=0.15, and the second control width t2k+2−t2k+1=0.05. The time sequence is depicted in Figure 2, where the different control intervals are presented alternately. The control parameters of DNs (2.1) are chosen as χ1=1,χ2=3, ηϑ1=ηω1=ηϑ2=ηω2=2. Let γ0=1, ℓ=0.6, δ=0.65, ε=5, β=4000, then Assumption 2 is satisfied. In Theorem 3.1, we select ϱ1=10, ϱ3=16, and take rϑ1=51, rω1=141, rϑ2=1.5, rω2=0.5, ˉrϑ1=0.9, ˉrω1=1, ˉrϑ2=0.6, ˉrω2=0.3, ℜϑ1=48.6, ℜω1=115, ℜϑ2=32, ℜω2=98. Then, by a simple calculation, one has ϱ0=0.0042, ϱ2=0.0548, the left side of formula (3.5) is less than 0, and the condition (3.5) is satisfied. Similarly, conditions (3.6)–(3.10) hold. From above, we can get ϕ1=3.3333,ϕ2=0.0119,ϕ3=5.3333,ϕ4=0.0183, then ϵ1=0.0036, ϵ2=0.0034, that is, ϵ1>ϵ2 is satisfied. In addition, one has Γ(48)=−1.4269×10−6<0, satisfying condition (3.4). Then, Figure 3 shows the trajectories of ‖ςϑı(t)‖ and ‖ςωı(t)‖(ı=1,2,3,4,5). It can be seen that unlike other results in previous literatures, Figure 3 shows that after a period of time, ‖ςϑı(t)‖ and ‖ςωı(t)‖ will converge to a neighborhood near the origin instead of the origin. This means that the systems achieve PGFETS by Definition 1. Further, it concludes that DNs (2.1) with controllers (3.2) can be synchronized into networks (2.2) within Tf=9.7497. By Lemma 5, it has that V(t)≤0.1016, then V1(t)≤0.1016 and V2(t)≤0.1016. As a result, ‖ςϑı(t)‖≤W=0.4509,‖ςωı(t)‖≤W=0.4509 for t>Tf. In other words, the PGFETS of DNs (2.1) and (2.2) is achieved.
To sum up, this brief considers the PGFETS for a class of DNs with quantized and delayed couplings. Via the finite-time stability theory, the PGFETS is proposed. Based on the Lyapunov functionals and 2-norm, some synchronization criteria have been established by adopting quantized intermittent control methods without sign function. Here, those criteria guarantee the DNs can be synchronized within a neighborhood of the origin in a finite time, which is different from some existing results. The introduction of quantizer effectively alleviates the communication burden and reduces control cost. Moreover, the designed controllers minimize the effects of chattering generated by the sign function. Finally, numerical simulations demonstrate the correctness and reasonableness of the new results. Note that DNs can be expanded to multiplex networks which can be used to describe more complex real systems. In the future, our research direction is to extend our proposed criteria to multiplex networks.
Ting Yang: Investigation, Writing-original draft, Writing-review & editing; Li Cao: Software, Validation; Wanli Zhang: Methodology, Writing-review & editing, Supervision. All authors have read and approved the final version of the manuscript for publication.
The authors declare they have not used Artificial Intelligence (AI) tools in the creation of this article.
This work was jointly supported by the National Natural Science Foundation of China (NSFC) (Grant No. 62003065), Natural Science Foundation of Chongqing, China(Grant No. CSTB2023NSCQ-MSX0771), the Science and Technology Research Program of Chongqing Municipal Education Commission of China (Grant No. KJZD-K202300502).
The authors declare no conflicts of interest in this paper.
[1] |
S. B. Zhu, J. Zhou, J. H. Lü, J. A. Lu, Finite-time synchronization of impulsive dynamical networks with strong nonlinearity, IEEE Trans. Autom. Control, 66 (2021), 3550–3561. https://doi.org/10.1109/TAC.2020.3022532 doi: 10.1109/TAC.2020.3022532
![]() |
[2] |
A. L. Li, X. L. Ye, Finite-time anti-synchronization for delayed inertial neural networks via the fractional and polynomial controllers of time variable, AIMS Math., 6 (2021), 8173–8190. https://doi.org/10.3934/math.2021473 doi: 10.3934/math.2021473
![]() |
[3] |
T. H. Yu, J. D. Cao, L. Rutkowski, Y. P. Luo, Finite-time synchronization of complex-valued memristive-based neural networks via hybrid control, IEEE Trans. Neural Netw. Learn. Syst., 33 (2022), 3938–3947. https://doi.org/10.1109/TNNLS.2021.3054967 doi: 10.1109/TNNLS.2021.3054967
![]() |
[4] |
E. G. Tian, Y. Zou, H. T. Chen, Finite-time synchronization of complex networks with intermittent couplings and neutral-type delays, IEEE/CAA J. Autom. Sinica, 10 (2023), 2026–2028. https://doi.org/10.1109/JAS.2023.123171 doi: 10.1109/JAS.2023.123171
![]() |
[5] |
Y. H. Xu, X. Q. Wu, B. Mao, J. H. Lü, C. R. Xie, Finite-time intra-layer and inter-layer quasi-synchronization of two-layer multi-weighted networks, IEEE Trans. Circuits Syst. I, 68 (2021), 1589–1598. https://doi.org/10.1109/TCSI.2021.3050988 doi: 10.1109/TCSI.2021.3050988
![]() |
[6] |
C. J. Song, J. Zhou, J. S. Wang, Finite time inter-layer synchronization of duplex networks via event-dependent intermittent control, IEEE Trans. Circuits Syst. II, 69 (2022), 4889–4893. https://doi.org/10.1109/TCSII.2022.3187269 doi: 10.1109/TCSII.2022.3187269
![]() |
[7] |
T. Liang, W. L. Zhang, D. G. Yang, Fixed-time synchronization of switched duplex networks with stochastic disturbances and limited communication, Commun. Nonlinear Sci. Numer. Simul., 121 (2023), 107227. https://doi.org/10.1016/j.cnsns.2023.107227 doi: 10.1016/j.cnsns.2023.107227
![]() |
[8] |
X. F. Wu, H. B. Bao, J. D. Cao, Fixed-time synchronization of multiplex networks by sliding mode control, J. Frankl. Inst., 360 (2023), 5504–5523. https://doi.org/10.1016/j.jfranklin.2023.03.057 doi: 10.1016/j.jfranklin.2023.03.057
![]() |
[9] |
X. S. Yang, Q. X. Zhu, C. X. Huang, Generalized lag-synchronization of chaotic mix-delayed systems with uncertain parameters and unknown perturbations, Nonlinear Anal.-Real, 12 (2011), 93–105. https://doi.org/10.1016/j.nonrwa.2010.05.037 doi: 10.1016/j.nonrwa.2010.05.037
![]() |
[10] |
M. W. Zheng, Z. M. Wang, L. X. Li, H. P. Peng, J. H. Xiao, Y. X. Yang, et al., Finite-time generalized projective lag synchronization criteria for neutral-type neural networks with delay, Chaos Soliton. Fract., 107 (2018), 195–203. https://doi.org/10.1016/j.chaos.2018.01.009 doi: 10.1016/j.chaos.2018.01.009
![]() |
[11] |
Y. B. Wu, C. D. Wang, W. X. Li, Generalized quantized intermittent control with adaptive strategy on finite-time synchronization of delayed coupled systems and applications, Nonlinear Dyn., 95 (2019), 1361–1377. https://doi.org/10.1007/s11071-018-4633-z doi: 10.1007/s11071-018-4633-z
![]() |
[12] |
P. Anand, B. B. Sharma, Generalized finite-time synchronization scheme for a class of nonlinear systems using backstepping like control strategy, Int. J. Dynam. Control, 11 (2023), 258–270. https://doi.org/10.1007/s40435-022-00948-y doi: 10.1007/s40435-022-00948-y
![]() |
[13] |
D. S. Xu, Y. Liu, M. Liu, Finite-time synchronization of multi-coupling stochastic fuzzy neural networks with mixed delays via feedback control, Fuzzy Set. Syst., 411 (2021), 85–104. https://doi.org/10.1016/j.fss.2020.07.015 doi: 10.1016/j.fss.2020.07.015
![]() |
[14] |
H. W. Ren, Z. P. Peng, Y. Gu, Fixed-time synchronization of stochastic memristor-based neural networks with adaptive control, Neural Networks, 130 (2020), 165–175. https://doi.org/10.1016/j.neunet.2020.07.002 doi: 10.1016/j.neunet.2020.07.002
![]() |
[15] |
X. Wang, N. Pang, Y. W. Xu, T. W. Huang, J. Kurths, On state-constrained containment control for nonlinear multiagent systems using event-triggered input, IEEE Trans. Syst. Man Cybern.: Syst., 54 (2024), 2530–2538. https://doi.org/10.1109/TSMC.2023.3345365 doi: 10.1109/TSMC.2023.3345365
![]() |
[16] |
T. Y. Jing, D. Y. Zhang, T. L. Jing, Finite-time synchronization of hybrid-coupled delayed dynamic networks via aperiodically intermittent control, Neural Process. Lett., 52 (2020), 291–311. https://doi.org/10.1007/s11063-020-10245-4 doi: 10.1007/s11063-020-10245-4
![]() |
[17] |
Y. B. Wu, Y. X. Gao, W. X. Li, Finite-time synchronization of switched neural networks with state-dependent switching via intermittent control, Neurocomputing, 384 (2020), 325–334. https://doi.org/10.1016/j.neucom.2019.12.031 doi: 10.1016/j.neucom.2019.12.031
![]() |
[18] |
X. L. Xiong, X. S. Yang, J. D. Cao, R. Q. Tang, Finite-time control for a class of hybrid systems via quantized intermittent control, Sci. China Inf. Sci., 63 (2020), 192201. https://doi.org/10.1007/s11432-018-2727-5 doi: 10.1007/s11432-018-2727-5
![]() |
[19] |
Y. Ren, H. J. Jiang, J. R. Li, B. L. Lu, Finite-time synchronization of stochastic complex networks with random coupling delay via quantized aperiodically intermittent control, Neurocomputing, 420 (2021), 337–348. https://doi.org/10.1016/j.neucom.2020.05.103 doi: 10.1016/j.neucom.2020.05.103
![]() |
[20] |
R. Q. Tang, H. S. Su, Y. Zou, X. S. Yang, Finite-time synchronization of Markovian coupled neural networks with delays via intermittent quantized control: linear programming approach, IEEE Trans. Neural Netw. Learn. Syst., 33 (2022), 5268–5278. https://doi.org/10.1109/TNNLS.2021.3069926 doi: 10.1109/TNNLS.2021.3069926
![]() |
[21] |
C. Xu, X. S. Yang, J. Q. Lu, J. W. Feng, F. E. Alsaadi, T. Hayat, Finite-time synchronization of networks via quantized intermittent pinning control, IEEE Trans. Cybern., 48 (2018), 3021–3027. https://doi.org/10.1109/TCYB.2017.2749248 doi: 10.1109/TCYB.2017.2749248
![]() |
[22] | S. Boyd, L. El Ghaoui, E. Feron, V. Balakrishnan, Linear matrix inequalities in system and control theory, SIAM, 1994. https://doi.org/10.1137/1.9781611970777.bm |
[23] |
Z. Y. Zuo, Nonsingular fixed-time consensus tracking for second-order multi-agent networks, Automatica, 54 (2015), 305–309. https://doi.org/10.1016/j.automatica.2015.01.021 doi: 10.1016/j.automatica.2015.01.021
![]() |
[24] |
J. Liu, G. T. Ran, Y. B. Wu, L. Xue, C. Y. Sun, Dynamic event-triggered practical fixed-time consensus for nonlinear multiagent systems, IEEE Trans. Circuits Syst. II, 69 (2022), 2156–2160. https://doi.org/10.1109/TCSII.2021.3128624 doi: 10.1109/TCSII.2021.3128624
![]() |
[25] |
J. Mei, M. H. Jiang, W. M. Xu, B. Wang, Finite-time synchronization control of complex dynamical networks with time delay, Commun. Nonlinear Sci. Numer. Simul., 18 (2013), 2462–2478. https://doi.org/10.1016/j.cnsns.2012.11.009 doi: 10.1016/j.cnsns.2012.11.009
![]() |
[26] | F. H. Clarke, Optimization and nonsmooth analysis, Hoboken, NJ, USA: Wiley, 1983. |
[27] | L. Cao, W. L. Zhang, Practical finite-time synchronization of T-S fuzzy complex networks with different couplings via semi-intermittent control, Int. J. Fuzzy Syst., in press. https://doi.org/10.1007/s40815-024-01686-3 |