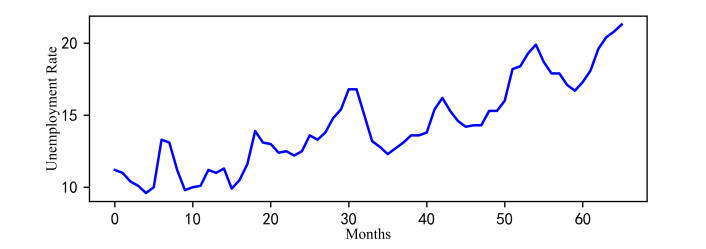
Granular computing is a novel method to solve complex tasks in the context of big data by simulating human problem-solving thinking, abstracting complex problems and dividing them into several simpler problems (i.e., granulation), which helps to better analyze and solve problems. In order to improve the accuracy of forecasting unemployment rates, this paper introduces the granulation idea of granular computing into the time series analysis of unemployment rates. Therefore, a novel method based on fuzzy information granules (FIGs) and grey system theory, namely FIG-GM(1,1) model, is proposed. This method not only reduces the dimensionality of the problem and computational complexity but also effectively reduces cumulative errors. In empirical analysis, three different performance indicators, mean absolute error (MAE), mean absolute percentage error (MAPE), and root mean squared error (RMSE), and seven comparative models are used to evaluate the forecasting performance of our proposed model. The empirical results indicate that the MAE, MAPE, and RMSE values of the FIG-GM(1,1) model are significantly lower than those of other models, indicating that the FIG-GM(1,1) model has better forecasting performance compared to other models.
Citation: Hong Yang, Jiangli Liu. A novel unemployment rate forecasting method based on fuzzy information granules and GM(1,1) model[J]. AIMS Mathematics, 2024, 9(4): 8689-8711. doi: 10.3934/math.2024421
[1] | Maolin Cheng . A new method for parameter estimation of extended grey GM(2, 1) model based on difference equation and its application. AIMS Mathematics, 2023, 8(7): 15993-16012. doi: 10.3934/math.2023816 |
[2] | Fengyi Chen . A new GM$ ^2(2,1) $ model based on a $ C^1 $ convexity-preserving rational quadratic interpolation spline. AIMS Mathematics, 2024, 9(7): 17917-17931. doi: 10.3934/math.2024872 |
[3] | James Daniel, Kayode Ayinde, Adewale F. Lukman, Olayan Albalawi, Jeza Allohibi, Abdulmajeed Atiah Alharbi . Optimised block bootstrap: an efficient variant of circular block bootstrap method with application to South African economic time series data. AIMS Mathematics, 2024, 9(11): 30781-30815. doi: 10.3934/math.20241487 |
[4] | Xiaolong Chen, Hongfeng Zhang, Cora Un In Wong . Optimization study of tourism total revenue prediction model based on the Grey Markov chain: a case study of Macau. AIMS Mathematics, 2024, 9(6): 16187-16202. doi: 10.3934/math.2024783 |
[5] | Yanlan Zhang, Changqing Li . The discernibility approach for multi-granulation reduction of generalized neighborhood decision information systems. AIMS Mathematics, 2024, 9(12): 35471-35502. doi: 10.3934/math.20241684 |
[6] | Halis Bilgil . New grey forecasting model with its application and computer code. AIMS Mathematics, 2021, 6(2): 1497-1514. doi: 10.3934/math.2021091 |
[7] | Dewang Li, Meilan Qiu, Shuiping Yang, Chao Wang, Zhongliang Luo . An optimal fractional-order accumulative Grey Markov model with variable parameters and its application in total energy consumption. AIMS Mathematics, 2023, 8(11): 26425-26443. doi: 10.3934/math.20231349 |
[8] | Dewang Li, Meilan Qiu, Zhongliang Luo . Huizhou resident population, Guangdong resident population and elderly population forecast based on the NAR neural network Markov model. AIMS Mathematics, 2024, 9(2): 3235-3252. doi: 10.3934/math.2024157 |
[9] | Yubin Cai, Xin Ma . A novel ensemble learning-based grey model for electricity supply forecasting in China. AIMS Mathematics, 2021, 6(11): 12339-12358. doi: 10.3934/math.2021714 |
[10] | Shahzaib Ashraf, Muhammad Shakir Chohan, Sameh Askar, Noman Jabbar . q-Rung Orthopair fuzzy time series forecasting technique: Prediction based decision making. AIMS Mathematics, 2024, 9(3): 5633-5660. doi: 10.3934/math.2024272 |
Granular computing is a novel method to solve complex tasks in the context of big data by simulating human problem-solving thinking, abstracting complex problems and dividing them into several simpler problems (i.e., granulation), which helps to better analyze and solve problems. In order to improve the accuracy of forecasting unemployment rates, this paper introduces the granulation idea of granular computing into the time series analysis of unemployment rates. Therefore, a novel method based on fuzzy information granules (FIGs) and grey system theory, namely FIG-GM(1,1) model, is proposed. This method not only reduces the dimensionality of the problem and computational complexity but also effectively reduces cumulative errors. In empirical analysis, three different performance indicators, mean absolute error (MAE), mean absolute percentage error (MAPE), and root mean squared error (RMSE), and seven comparative models are used to evaluate the forecasting performance of our proposed model. The empirical results indicate that the MAE, MAPE, and RMSE values of the FIG-GM(1,1) model are significantly lower than those of other models, indicating that the FIG-GM(1,1) model has better forecasting performance compared to other models.
The unemployment rate of any country is one of the most important economic indicators for financial market participants, and time series prediction of unemployment rate is crucial for financial market participants and has received widespread attention.
Unemployment is not only closely related to individuals, but also to the investment decisions of investors, social market economy, and national monetary policy. In recent years, external shocks such as the pandemic, influenza, and trade frictions have frequently affected employment, leading to increasingly serious unemployment problems and increasing negative impacts on individuals, society, and the country [1,9,33].
In order to deal with the unemployment problem, many countries and regions have adopted various policies and measures. For example, the policies adopted by the Chinese government include increasing the number of vocational education institutions, expanding the scale of the tertiary industry to optimize the current industrial structure of the country, establishing early warning system to improve the unemployment security system, and creating a variety of new employment opportunities actively. Although these policies have helped expand the scale of employment and reduce the negative consequences of unemployment to a certain extent, the employment situation in many countries and regions is still not optimistic and the unemployment problem is still relatively serious. Therefore, unemployment is an important factor that can-not be ignored in the national economic development, and is also an urgent unemployment problem to be solved [4,5].
Although the existing forecasting methods can forecast most macroeconomic indicators, it is difficult for the existing forecasting models to accurately forecast the unemployment rate due to the lack of time series data and indicators closely related to unemployment. It is important for the government to guide the next step by estimating the unemployment rate, so as to realize the precise docking of subsidies and make the whole society achieve the reasonable distribution and balance of human, material, and financial resources. Therefore, timely and accurate forecasting of the unemployment rate is conducive to relevant government departments to carry out macro-control and is also of great significance to understand the employment situation and do a good job in employment.
A review of the literature shows that studies on the unemployment rate broadly cover three areas.
The first is the influence factors of the unemployment rate. Since unemployment is closely connected with various economic and social realities, various factors will inevitably affect the unemployment rate, such as gross domestic product (GDP), industrial structure, economic growth rate, consumer price index (CPI), broadband speed of residents, etc [1,5,9,14,33].
The second is the effect generated by the unemployment rate. Unemployment affects not only individuals but also societies and nations. Research has shown that unemployment rates have a significant impact on mortality rates [2,3,23,29,30,31]. Specifically, for men, rising unemployment increases suicide rates, but falling unemployment does not reduce them and the opposite is true for women [2]. In addition, unemployment rate also has other implications. For example, T. Ikeda et al. [8] used a multilevel logistic model to examine the relationship between unemployment rate and low back pain, and the empirical results showed that unemployment rate has a significant effect on low back pain and women are more influenced by regional employment status than men. Unemployment also has a significant impact on security, with people feeling significantly more secure in their jobs in areas with the lowest unemployment rates, and people in areas with higher unemployment who worked on temporary or fixed-term contracts were more likely to feel less secure than those with permanent jobs [20].
The third is the forecasting methods of unemployment rate. In recent years, there are many models for forecasting unemployment rate. Not only include statistical models such as auto-regressive integrated moving average (ARIMA) model, nonlinear autoregressive (NAR), adaptive filtering method and trend extrapolation, but also machine learning models such as support vector regression (SVR), hidden markov model (HMM), long short-term memory network (LSTM), and so on [6,10,13,16,19,21,27]. However, the above mentioned methods on unemployment rate forecasting usually involve a single point advance forecasting, which may perform poorly in situation that requires predicting a sequence of values over a time horizon. Therefore, it is very necessary to design a multi-step forecasting model to improve the efficiency of forecast unemployment rate.
The ultimate purpose of the research in this paper is to construct a novel unemployment rate forecasting method and to provide a real reference for the actual state of employment and unemployment based on the forecasting results. The research covers the following three main areas. First, by referring to relevant literature, on the basis of fully understanding the research background and theoretical basis of unemployment rate, we can enrich the solid theoretical basis obtained by predecessors and supplement the knowledge of related fields that have not been deeply involved or not involved by predecessors. Second, by referring to the relevant forecasting methods of the unemployment rate at home and abroad, an accurate forecasting model of the unemployment rate is established to provide a reliable analysis tool for the empirical analysis. Finally, the empirical analysis is carried out, the accuracy of the forecasting model is evaluated by using the model performance related evaluation index, and the trend of unemployment rate is predicted.
The innovation of this paper is reflected in the forecasting method. Based on fuzzy information granules (FIGs) and grey forecasting model (GM), a novel forecasting method called FIG-GM(1,1) model is proposed. This is also the first proposal to combine FIGs with grey systems, combining the advantages of both FIGs and grey forecasting model. The construction method based on FIGs transforms the original time series into a meaningful and easy to operate granule time series. Analyzing on newly created granule time series instead of original numerical time series is more convenient for solving problems, which is equivalent to decomposing a very complex problem into several simple problems. This not only greatly reduces the dimensionality of the problem but also greatly reduces computational complexity. In addition, forecasting ground particles is meaningful, which makes it easier and more transparent for humans to explain the changes in this window. Moreover, forecasting based on granule time series is a multi-step forecasting, not a one-step prediction. In other words, a predicted granule usually contains several predicted values located in the future time window, which can effectively reduce cumulative errors.
The paper is organized as follows. In Section 2, the basic concepts and related theorems of fuzzy information granulation, grey forecasting principles and the algorithm of GM(1,1) model are introuced. Section 3 presents the granulation process of triangular FIGs, the process of constructing a grey forecasting model based on granule time series, and describes the residual correction process of the GM(1,1) model. In order to verify the effectiveness and feasibility of the proposed method, an empirical analysis is made in Section 4. Section 5 provides conclusions and offers some thoughts on future studies.
The concept and algorithm of fuzzy information granulation, the principle of grey prediction model and the algorithm of GM(1,1) model are introduced in this section.
Information granulation covers set theory, interval analysis, rough sets, fuzzy sets, probability theory, and quotient space theory [41]. There are three typical ways of information granulation: Information granulation based on rough set theory [17,18,22], information granulation based on fuzzy set theory [36,37,38,39,41,42], and information granulation based on quotient space theory [35].
Since L. A. Zadeh [36] proposed fuzzy set theory in 1965, he has focused on the definition of fuzzy systems and their states, laying the foundation for the framework of fuzzy system theory. The introduction of language variables, fuzzy conditional propositions, and basic features of fuzzy algorithms in 1973 [37] became a turning point work, laying the foundation for the theory of fuzzy information granulation. Zadeh [38] further elaborated on language variables, fuzzy "IF-THEN" rules, and fuzzy graphs. In 1979, Zadeh [39] proposed and discussed the problem of fuzzy information granulation, which promoted the development of fuzzy logic theory and applications. Then, the proposal of generalized constraints and "word computing theory" [40] marked the birth of the theory of fuzzy information granulation.
The theory of information granulation provides researchers with different perspectives and ways of problem-solving and has made tremendous progress in theoretical and applied research, giving rise to different methods and types of information granulation [43]. The information granulation based on fuzzy set theory is abbreviated as fuzzy information granulation, and the information granules derived from fuzzy information granulation theory are called FIGs.
The most critical issue in fuzzy information granulation is the characterization and description of FIGs. Professor Zadeh describes FIGs as
g≜(xisG)isλ, | (2.1) |
where x is a variable in the domain U, generally U is a real straight line (or Rn), G is a convex fuzzy subset of U characterized by a membership function μG, and λ represents fuzzy probability (or likelihood) and is a fuzzy subset of the unit interval [0, 1]. For example, g≜ (x is large) is possible, and g≜ (y is much smaller than x) is impossible.
Another proposition description of FIGs is
g≜xisG. | (2.2) |
Equation (2.2) is a special case of Eq (2.1) and does not have probabilistic characteristics. To distinguish between the two, the FIG described by the proposition in the form of Eq (2.1) is πp-granule, and the corresponding FIGs in Eq (2.2) is π-granule.
Let data X={x1,x2,...,xn} as an example to establish a FIG P that can reasonably describe the fuzzy concept G (a fuzzy set with X as the domain) of X. Once G is determined, the FIG can be determined
P≜xisG,xϵX. |
Establishing FIGs is equivalent to determining the membership function of fuzzy concept G, that is, A=μG. Except for special circumstances, this article uses FIG P instead of fuzzy concept G, that is,
P=A(x),xϵX. |
The basic forms of P in the formula include interval FIG, A(x;a,b); triangular FIG, A(x;a,m,b); ladder FIG, A(x;a,m,n,b); Gaussian FIG, A(x;μ,σ); etc.
Grey forecasting [32] is the forecasting of systems that contain both known and uncertain information and is the forecasting of grey processes that vary within a certain range and are time-dependent. Grey forecasting is performed by identifying the degree of dissimilarity of development trends among system factors (i.e., correlation analysis), processing raw data to find the pattern of system changes, and generating a data series with strong regularity. Then, the corresponding differential equation model is established to predict the future development trend of things.
Grey forecasting includes four types: Grey time series forecasting, distortion forecasting, system forecasting, and topological forecasting. The type of grey forecasting adopted in this paper is grey time series prediction, that is, the observed time series reflecting the characteristics of the prediction object is used to construct the grey forecasting model and predict the feature quantity at a certain time in the future, or the time to reach a certain feature quantity.
The grey forecasting model GM(n, h) is essentially a differential equation model that can be used to make a long-term, continuous, and dynamic reflection of the described object, where n represents the derivative order of the system and h represents the number of variables. In this paper, we use the GM(1,1) model.
The algorithm of the GM(1,1) model is as follows.
Let the time series X(0) have n observations, the X(0)={X(0)(1),X(0)(2),⋯,X(0)(n)}, generate the sequence by accumulating X(1)={X(1)(1),X(1)(2),⋯,X(1)(n)}. Then, the differential equation corresponding to the GM(1,1) model is
dX(1)dt+αX(1)=μ, | (2.3) |
where α is the developmental grey number and μ is the endogenous control grey number.
Let ˆα be the vector of parameters to be estimated, and ˆα=(αμ), which can be solved using the least squares method. Then, we obtain
ˆα=(DTD)−1DTYn. | (2.4) |
Of which
D=(−12[X(1)(1)+X(1)(2)]1−12[X(1)(2)+X(1)(3)]1⋯⋯−12[X(1)(n−1)+X(1)(n)]1),and Yn=(X(0)(2)X(0)(3)⋯X(0)(n)). | (2.5) |
By solving the differential Eq (2.3), the cumulative forecasting value ˆX(1)(k+1) of the GM(1,1) forecasting model is obtained:
ˆX(1)(k+1)=[X(0)(1)−μα]e−αk+μα,(k=0,1,⋯,n). | (2.6) |
Then, subtract ˆX(1)(k+1) to get the forecasting value ˆX(0)(k+1):
ˆX(0)(k+1)=ˆX(1)(k+1)−ˆX(1)(k),(k=1,2,3,⋯,n). | (2.7) |
In this section, we introduce the steps of constructing triangular FIGs on time series. Then, a grey forecasting method called FIG-GM(1,1) based on FIGs is proposed to overcome the shortcomings of traditional time series forecasting models. Finally, the residual correction steps of this new method are also introduced.
The triangular FIG (fuzzy set) will be used in this paper and its membership function A(x;a,m,b) is as follows:
A(x;a,m,b)={0,ifx<a,x−am−a,ifx∈[a,m],b−xb−m,ifx∈(m,b],0,ifx>b, | (3.1) |
where the input time series is represented by x; a, m, b are the parameters of the fuzzy granule. Specifically, a represents the minimum value of a fuzzy window, m reflects the average level, and b represents the maximum value.
The granulation process of FIGs includes the following steps:
Step 1: Determine the partition window T and divide the original time series into many subsequences. There are two methods for dividing window T: Equal length and unequal length, each with its own advantages.
Step 2: Establishing a FIG on the i-th subsequence Xi={x1,x2,⋯,xt} coming from the time series X, a sound fuzzy information granule should contain as much data as possible out of those contained in the time window, while at the same time, the granule should be as explicit and concise as possible so that its meaning (semantics) is easy to follow. According to these two requirements, calculate the values of the membership function parameters ai, mi, and bi of the subsequence Xi, and then the subsequence is transformed into a triangular FIG, A(ai,mi,bi).
Algorithm 1 introduces the pseudo code for granularize the original time series into a granule time series composed of triangular FIGs.
Algorithm 1 Granulation based on triangular FIGs |
Input: Original numerical time series X(i) and window width T. Output: A granule time series. 1: Determine the granulation window width T. 2: Divide the original numerical time series X(i) into s subsequences based on the window width T. 3: Granulate each subsegment into a triangular FIG. 4: A granule time series A={A1(a1,m1,b1),A2(a2,m2,b2),⋯,As(as,ms,bs)},i=1,2,⋯,s, composed of triangular FIGs is obtained. |
Example 3.1. We will give an example to illustrate in detail how to use triangular FIG to granulate time series X={x1,x2,⋯,xn} into granule time series.
Step 1: Let partition window T=k, where the window width T is divided into equal window lengths. Time series X is divided into s=nk equally long subseguences shown as below
{x1,x2,⋯,xk},{xk+1,xk+2,⋯,x2k},⋯,{x(s−1)k+1,x(s−1)k+2,⋯,xn}. |
Let Xi={x(i−1)k+1,x(i−1)k+2,⋯,xik},i=1,2,⋯,s. Then, X can be expressed as X={X1,X2,⋯,Xs}.
Step 2: Let the minimum data in the i−th subsequence of time series X be ai, the maximum data be bi, and the average value of all data be mi. Then, the subsequence can be represented as A(ai,mi,bi),i=1,2,⋯,s.
We will provide the steps and residual correction process for constructing a GM(1,1) model based on granule time series in this subsection.
The granulation algorithm of triangular FIG in Subsection 3.1 will be used to convert numerical time series X={x1,x2,⋯,xn} into granule time series A={A1(a1,m1,b1),A2(a2,m2,b2),⋯,As(as,ms,bs)} (s<n).
Theorem 1. [28] Let A be a triangular FIG such that, for any A(ai,mi,bi), A(ak,mk,bk), the following relationship is defined as:
A(ai,mi,bi)−A(ak,mk,bk)=A(ai−bk,mi−mk,bi−ak). |
The steps to construct the GM(1,1) model based on the granule time series A are as follows:
Step 1: Accumulate the parameter sequences a={a1,a2,⋯,as}, m={m1,m2,⋯,ms}, and b={b1,b2,⋯,bs} separately to generate the cumulative sequence a1={a11,a12,⋯,a1s}, m1={m11,m12,⋯,m1s}, and b1={b11,b12,⋯,b1s}.
Step 2: According to Eqs (2.4) and (2.5), estimate the parameter vectors ˆαa=(αaμa), ˆαm=(αmμm), and ˆαb=(αbμb) for sequences a1, m1, and b1 respectively.
Step 3: Substitute parameter vectors ˆαa=(αaμa), ˆαm=(αmμm), and ˆαb=(αbμb) into Eq (2.3), respectively, and solve these three differential equations. Then, obtain the expressions for the forecasting values of sequences a1, m1, and b1. They are expressed as follows:
ˆa1k+1=[a1−μaαa]e−αak+μaαa,(k=1,2,⋯,s), |
ˆm1k+1=[m1−μmαm]e−αmk+μmαm,(k=1,2,⋯,s), |
ˆb1k+1=[b1−μbαb]e−αbk+μbαb,(k=1,2,⋯,s). |
Step 4: Obtain a forecasting model for the parameter sequences a, m, and b of the granule time series A, which are:
ˆak+1=ˆak+11−ˆak1,(k=1,2,3,⋯,s), |
ˆmk+1=ˆak+11−ˆak1,(k=1,2,3,⋯,s), |
ˆbk+1=ˆak+11−ˆak1,(k=1,2,3,⋯,s). |
According to Subsection 2.2, the essence of the GM(1,1) model is a differential equation, and the grey forecasting model based on FIGs is actually solving differential Eq (2.3) on the granule time series. Algorithm 2 introduces the pseudo code for constructing a grey forecasting model based on granule time series.
Algorithm 2 Building a grey forecasting model based on granule time series |
Input: Evaluation indicators for accuracy level of granule time series and grey forecasting models. Output: The parameters of the constructed model. 1: Accumulating granule time series to generate accumulated granule time series. 2: Solving differential equations for accumulated granule time series. 3: Obtain grey forecasting model based on granule time series 4: Accumulate to obtain a forecasting model based on granule time series. 5: Calculate the residual value, correlation value, and posterior difference of the granule time series 6: if the residual value, correlation value, and posterior difference value all meet the conditions then 7: the forecasting model is output 8: else 9: residual correction will be applied to the forecasting model 10: end if 11: Update forecasting model |
To evaluate the forecasting performance of the GM(1,1) model constructed based on granular time series in Subsection 3.2.1, a residual test, correlation degree test, and posterior difference test (the process of these three testing methods can be found in [32]) are performed. If the constructed model is unqualified or has poor forecasting performance, residual correction can be applied. The detailed process is as follows:
Let the GM(1,1) model constructed based on the parameter sequence a={a1,a2,⋯,as} of granule time series A be:
ˆa1k+1=[a1−μaαa]e−αak+μaαa,ˆak+1=ˆak+11−ˆak1,(k=1,2,3,⋯,s). | (3.2) |
According to Eq (3.2), the forecasting value ˆa={ˆa1,ˆa2,⋯,ˆas} of the parameter generation sequence a={a1,a2,⋯,as} is obtained.
Definition 3.1. [32] The residual of the parameter sequence a={a1,a2,⋯,as} and the forecasting sequence ˆa={ˆa1,ˆa2,⋯,ˆas} is defined as:
e(0)(j)=aj−ˆaj. |
Let j=i,i+1,⋯,s. Then, the sequence of residuals corresponding to a={a1,a2,⋯,as} and ˆa={ˆa1,ˆa2,⋯,ˆas} is:
e(0)={e(0)(i),e(0)(i+1),⋯,e(0)(s)}. | (3.3) |
For ease of calculation, Eq (3.3) can be rewritten as
e(0)={e(0)(1′),e(0)(2′),⋯,e(0)(n′)},n′=s−i. |
The residual correction process is as follows.
Step 1. The residue sequence e(0) is added to produce the sequence e(1).
e(1)={e(1)(1′),e(1)(2′),⋯,e(1)(n′)},n′=s−i. |
Step 2. A GM(1,1) model is established for the sequence e(1), and the cumulative forecasting value of residual ˆe(1)(k+1) is obtained by substituting relevant data.
ˆe(1)(k+1)=[e(0)(1)−μeae]e−aek+μeae. |
Step 3. Derivative for ˆe(1)(k+1).
^e′(k+1)=(−ae)[e(0)(1)−μeae]e−ae(k−1),(k=1,2,⋯,n′). |
Step 4. A modified model for obtaining the cumulative parameter sequence a1i.
ˆak+11=[a1−μa]e−ak+μa]+δ(k−1)(−ae)[e(0)(1)−μeae]e−ae(k−1),(k=1,2,⋯,n′), |
where δ(k−1)={1,k≥2,0,k<2. is the correction factor.
Step 5. Residual correction model for obtaining parameter sequence ai of granule time series A.
ˆak+1=ˆak+11−ˆak1,(k=1,2,⋯,n′). | (3.4) |
Four time series data sets of unemployment rates, seven comparative models, and three commonly used indicators to evaluate the predictive performance of models for empirical analysis are introduced in Subsection 4.1. The empirical analysis and results of different data sets are presented in Subsection 4.2.
The four unemployment rate time series, three predictive performance evaluation indicators, seven comparative models, and the division of time series windows used for empirical analysis are introduced in this subsection.
Four unemployment rate time series with different characteristics are used for the forecasting*†, as shown below:
*https://data.stats.gov.cn/easyquery.htm?cn = A01
†https://www.macroview.club/data?code = us_unemployment
(1) China urban survey unemployment rate aged 16–24 time series, Jan.2018-Jun.2023, (see Figure 1);
(2) US unemployment rate time series, Jan.2000–Dec.2020, (see Figure 2);
(3) Japan unemployment rate time series, Jan.2000–Nov.2023, (see Figure 3);
(4) Germany unemployment rate time series, Jan.2000–Nov.2023, (see Figure 4).
In order to evaluate the forecasting accuracy of the FIG-GM(1,1) model and other seven models, three types of indexes [34] are considered as performance measures in this paper:
a) Mean absolute error (MAE):
MAE=1nf∑|Xforecasted(t)−Xactual(t)|. |
b) Mean absolute percentage error (MAPE):
MAPE=1nf∑|Xforecasted(t)−Xactual(t)|Xactual(t)×100. |
c) Root mean square error (RMSE):
RMSE=√∑(Xforecasted(t)−Xactual(t))2nf, |
where nf is the number of future values to be predicted, Xforecasted(t) and Xactual(t) are the predicted and actual value in the next t-th moment, respectively.
The following seven time series forecasting models are used to compare with the proposed model in this paper.
a) Exponential smoothing (ES)
ES model [32] calculates st=αxt+(1−α)s(t−1), where st is regarded as a prediction of x(t+1) and 0<α<1 is the smoothing factor.
b) Auto-regressive integrated moving average (ARIMA)
In an ARIMA (p, d, q) model [12], the time series with the mean μ is generated as follows:
ϕ(B)(1−B)d(xt−μ)=θ(B)αt, |
where xt and αt are respectively the actual value and random error at time t, ϕ(B) and θ(B) are the polynomials in B of degrees p and q, B denotes the lag operator, and d indicates the degree of ordinary differencing.
c) Prophet model
Prophet model [26] is an additive regression model, which consists of four parts: Trend, seasonality, holidays, and error. The expression is as follows:
y(t)=g(t)+s(t)+h(t)+ϵ(t), |
where g(t) represents the trend, s(t) represents the seasonality, h(t) represents the holidays, and ϵ(t) represents the error.
d) Support vector regression (SVR)
SVR [24] is a regression method of support vector machines, which is mainly based on the principle of maximizing function intervals, selecting the optimal separating hyperplane, minimizing the sum of squares of regression residuals, and constructing the optimal regression curve.
e) Long short-term memory neural network (LSTM)
The LSTM model [27] consists of input-gate, forget-gate, and output-gate, which are expressed as
ft=σ(W(fx)xt+W(fh)h(t−1)+bf),it=σ(W(ix)xt+W(ih)h(t−1)+bi),~ct=tanh(W(cx)xt+W(ch)h(t−1)+bc), |
Ct=ft∘C(t−1)+it∘~ct,ot=σ(W(ox)xt+W(oh)h(t−1)+bo),ht=ot∘tanh(Ct), |
where xt is the input at moment t, h(t−1) is the output at moment t-1, σ is the sigmoid activation function, and ∘ is the Hadamard product.
f) Artificial neural network (ANN)
Artificial neural network [21], consists of input layer, hidden layer, and output layer. It is an information processing system established based on imitating the structure and function of brain neural networks.
g) GM(1,1) model
The GM(1,1) model is essentially a differential equation, as detailed in Subsection 2.2 of this paper.
In our proposed FIG-GM(1,1) model algorithm, the granule window length T is an important parameter. Improper selection may lead to poor data analysis results and render the grey forecasting model worthless.
There are two ways to divide the time window T: Divide it into fixed time intervals or divide it into non fixed time intervals. Due to the fact that the second method can not only segment the molecular sequence based on the fluctuation points of the time series, but also retain the trend information of the original time series, and because constructing fuzzy information granules with unequal length subsequences can more effectively utilize the role of information granules, this paper will use the ℓ1 trend filtering [11] method to segment the time series. Specifically, for China urban survey unemployment rate aged 16–24 time series, divided into 11 sequences; for United States unemployment rate time series, divided into 16 sequences; and for Japan unemployment rate time series, divided into 20 sequences; for Germany unemployment rate time series, divided into 17 sequences.
In this subsection, we will conduct empirical analysis on four selected unemployment rate data sets with different characteristics.
The China urban survey unemployment rate aged 16–24 time series from January 2018 to June 2023 are used for this experiment. The whole time series can be divided into 11 temporal windows based on ℓ1 trend filtering. The first 10 temporal windows data are used as training data to predict values in the last one temporal window data. The forecasting values of the FIG-GM(1,1) model are closest to the actual values. Also, these predicted FIGs describe changes in the next one windows in a way that is easy for humans to understand. In detail, the one outputs ˆAi=G(ˆai,ˆmi,ˆbi), i = 11, where
(ˆa11,ˆm11,ˆb11)=(17.55,19,21.22) |
predict that the data for this time series in the next time window will be between 17.55 and 21.22, the average value is 19. These predictions are consistent with the practial situation, which shows that the predicted FIGs serve as fairly good tools to describe the future data.
Table 1 and Figure 5 compare MAE, MAPE, and RMSE values of the FIG-GM(1,1) model with other 7 comparative models. We can clearly see that whether predicting for 3 or 6 months, the three performance indicators of the FIG-GM(1,1) model have the smallest values. Overall, in this empirical study, the prediction accuracy of the FIG-GM(1,1) model performed the best among these eight models. This is because the FIG-GM(1,1) model proposed in this paper effectively utilizes the fact that a FIG can contain a large amount of data, achieving multi-step prediction instead of single step prediction, and thereby effectively reducing the cumulative error of single step prediction and obtaining more accurate prediction results. Also, FIG has high interpretability, making it easier for people to understand. Thus, the proposed model is more suitable for practical applications.
Model | 3 months ahead forecast | 6 months ahead forecast | |||||
MAE | MAPE | RMSE | MAE | MAPE | RMSE | ||
ES | 0.489 | 0.026 | 0.433 | 0.594 | 0.030 | 0.644 | |
ARIMA | 1.340 | 0.071 | 1.098 | 2.420 | 0.119 | 2.719 | |
Prophet | 0.900 | 0.048 | 0.767 | 1.485 | 0.074 | 1.681 | |
SVR | 0.747 | 0.041 | 0.574 | 2.217 | 0.107 | 2.893 | |
LSTM | 0.379 | 0.020 | 0.377 | 0.503 | 0.025 | 0.610 | |
ANN | 1.093 | 0.058 | 0.947 | 1.878 | 0.109 | 2.564 | |
GM | 0.746 | 0.041 | 0.574 | 1.318 | 0.066 | 1.463 | |
FIG-GM | 0.293 | 0.016 | 0.255 | 0.276 | 0.013 | 0.296 |
The US unemployment rate time series from January 2000 to December 2020 are used for this experiment. The whole time series can be divided into 16 temporal windows based on ℓ1 trend filtering. The first 15 temporal windows data are used as training data to predict values in the last one temporal window data. The predicted values of the FIG-GM(1,1) model is closest to the actual values. Also, these predicted FIGs describe changes in the next one windows in a way easy that is for humans to understand. In detail, the one outputs ˆAi=G(ˆai,ˆmi,ˆbi), i = 16, where
(ˆa16,ˆm16,ˆb16)=(5.32,7.35,9.94) |
predict that the data for this time series in the next time window will be between 5.32 and 9.94, the average value is 7.35, and these predictions are consistent with the real situation.
Table 2 and Figure 6 summarize the performances of these models. From the results in Table 2 and Figure 6, whether predicting for 3 or 6 months, the MAE, MAPE, and RMSE values of FIG-GM(1,1) model are smaller than those of other 7 comparative models, indicating the forecasting accuracy of the proposed model is better than that of other models.
Model | 3 months ahead forecast | 6 months ahead forecast | |||||
MAE | MAPE | RMSE | MAE | MAPE | RMSE | ||
ES | 5.329 | 0.452 | 2.051 | 3.077 | 0.276 | 4.020 | |
ARIMA | 2.783 | 0.255 | 2.246 | 4.360 | 0.518 | 4.714 | |
Prophet | 8.160 | 0.705 | 4.041 | 6.450 | 0.659 | 6.735 | |
SVR | 4.293 | 0.359 | 1.293 | 2.195 | 0.185 | 3.316 | |
LSTM | 6.477 | 0.566 | 3.123 | 5.288 | 0.546 | 5.501 | |
ANN | 4.713 | 0.401 | 1.951 | 2.782 | 0.253 | 3.539 | |
GM | 5.643 | 0.483 | 2.489 | 3.727 | 0.357 | 4.313 | |
FIG-GM | 2.630 | 0.226 | 1.201 | 2.040 | 0.207 | 2.158 |
The Japan unemployment rate time series from January 2000 to November 2023 are used for this experiment, taking China as an example. The whole time series can be divided into 20 temporal windows based on ℓ1 trend filtering. The first 19 temporal windows data are used as training data to predict values in the last one temporal window data. A few methods give precise predictions in this experiment, and the FIG-GM(1,1) model has the best prediction accuracy. In detail, the one outputs ˆAi=G(ˆai,ˆmi,ˆbi), i = 20, where
(ˆa20,ˆm20,ˆb20)=(2.4,2.7,2.9) |
predict that the data for this time series in the next time window will be between 2.4 and 2.9, the average value is 2.7. This prediction is consistent with the practical situation, which shows that the predicted FIG serve as fairly good tools to describe the future data.
The MAE, MAPE, and RMSE values of the FIG-GM(1,1) model are compared with the other 7 results in Table 3 and Figure 7. The experimental results show that among these 8 models, when predicting 6 months, the MAE value of the FIG-GM(1,1) model is the smallest, the MAPE value is the smallest consistent with the SVR model, and the RMSE value is slightly larger than the SVR model; when predicting 12 months, the three performance indicators of the FIG-GM(1,1) model have the smallest values, reflecting that the predictive performance of FIG-GM(1,1) model is better than other models.
Model | 6 months ahead forecast | 12 months ahead forecast | |||||
MAE | MAPE | RMSE | MAE | MAPE | RMSE | ||
ES | 0.102 | 0.041 | 0.121 | 0.091 | 0.036 | 0.109 | |
ARIMA | 0.075 | 0.029 | 0.090 | 0.118 | 0.044 | 0.147 | |
Prophet | 0.122 | 0.048 | 0.145 | 0.163 | 0.062 | 0.208 | |
SVR | 0.065 | 0.026 | 0.066 | 0.300 | 0.119 | 0.848 | |
LSTM | 0.088 | 0.035 | 0.107 | 0.103 | 0.039 | 0.127 | |
ANN | 0.068 | 0.028 | 0.085 | 0.084 | 0.032 | 0.105 | |
GM | 0.175 | 0.070 | 0.189 | 0.131 | 0.051 | 0.150 | |
FIG-GM | 0.060 | 0.026 | 0.076 | 0.079 | 0.030 | 0.085 |
The Germany unemployment rate time series from January 2018 to November 2023 are used for this experiment. Then, the whole time series can be divided into 17 temporal windows based on ℓ1 trend filtering. The first 16 temporal windows data are used as training data to predict values in the last one temporal window data. The FIG-GM(1,1) model has the best prediction accuracy among these prediction methods. In detail, the one outputs ˆAi=G(ˆai,ˆmi,ˆbi), i = 17, where
(ˆa17,ˆm17,ˆb17)=(2.9,3,3.3) |
predict that the data for this time series in the next time window will be between 2.9 and 3.3, the average value is 3. This prediction is consistent with the practical situation, which shows that the predicted FIG serve as fairly good tools to describe the future data.
Table 4 and Figure 8 summarize the performances of these models. From the results in Table 4 and Figure 8, whether predicting for 6 or 12 months, the MAE, MAPE, and RMSE values of FIG-GM(1,1) model are smaller than those of other 7 comparative models, indicating the forecasting accuracy of the proposed model is better than that of other models.
Model | 6 months ahead forecast | 12 months ahead forecast | |||||
MAE | MAPE | RMSE | MAE | MAPE | RMSE | ||
ES | 0.205 | 0.069 | 0.206 | 0.128 | 0.043 | 0.151 | |
ARIMA | 0.158 | 0.054 | 0.167 | 0.115 | 0.039 | 0.133 | |
Prophet | 0.330 | 0.112 | 0.355 | 0.286 | 0.096 | 0.309 | |
SVR | 0.210 | 0.071 | 0.214 | 0.173 | 0.058 | 0.180 | |
LSTM | 0.083 | 0.028 | 0.096 | 0.112 | 0.037 | 0.125 | |
ANN | 0.157 | 0.053 | 0.163 | 0.104 | 0.035 | 0.125 | |
GM | 0.357 | 0.121 | 0.359 | 0.428 | 0.142 | 0.436 | |
FIG-GM | 0.010 | 0.003 | 0.014 | 0.050 | 0.016 | 0.100 |
This paper proposes a novel forecasting method called the FIG-GM(1,1) model based on fuzzy information granules and grey forecasting model. In the FIG-GM(1,1) model, fuzzy information granulation substantially reduces the sample complexity while effectively preserving the sample characteristics and significantly improves the efficiency of the model by transforming the original time series into a meaningful and easily manipulated granule time series. In empirical analysis, the forecasting performance of our proposed model is evaluated by comparing the MAE, MAPE, and RMSE values of three performance indicators between our proposed model and seven comparative models (i.e., ES, ARIMA, Prophet, SVR, LSTM, ANN, and GM). The four empirical results indicate that the MAE, MAPE, and RMSE values of the FIG-GM(1,1) model are significantly lower than those of other models, indicating that the FIG-GM(1,1) model has better forecasting performance compared to other models. Along with the better forecasting accuracy, another benefit of our method is using granules to forecast future data, allowing us to describe future periods in a way that is easy for humans to understand.
In addition, the forecasting effect of the method will be affected by the type of fuzzy granules, the type of kernel function, the size of the granulation window, etc. In the future, these factors need to be discussed and further optimized in order to improve the application effect of the forecasting method.
The authors declare they have not used Artificial Intelligence (AI) tools in the creation of this article.
This work is supported by National Natural Science Foundation of China (12161082) and Natural Science Foundation of Gansu Province (21JR7RA134).
The authors are very grateful to the anonymous referees for their valuable suggestions.
The authors declare that they have no conflict of interest.
[1] | A. Badulescu, Unemployment in Romania. A retrospective study, Theor. Appl. Econ., 2 (2006), 71–76. |
[2] |
F. Botha, V. H. Nguyen, Opposite nonlinear effects of unemployment and sentiment on male and female suicide rates: Evidence from Australia, Soc. Sci. Med., 292 (2022), 114536. http://dx.doi.org/10.1016/j.socscimed.2021.114536 doi: 10.1016/j.socscimed.2021.114536
![]() |
[3] |
K. A. Bender, I. Theodossiou, A reappraisal of the unemployment-mortality relationship: Transitory and permanent effects, J. Public Health Pol., 36 (2015), 81–94. http://dx.doi.org/10.1057/jphp.2014.40 doi: 10.1057/jphp.2014.40
![]() |
[4] |
M. Bussiere, M. Fratzscher, Towards a new early warning system of financial crises, J. Int. Money Financ., 25 (2006), 953–973. http://dx.doi.org/10.1016/j.jimonfin.2006.07.007 doi: 10.1016/j.jimonfin.2006.07.007
![]() |
[5] |
F. Cai, M. Wang, Growth and structural changes in employment in transition China, J. Comp. Econ., 38 (2010), 71–81. http://dx.doi.org/10.1016/j.jce.2009.10.006 doi: 10.1016/j.jce.2009.10.006
![]() |
[6] |
T. Chakraborty, A. K. Chakraborty, M. Biswas, S. Banerjee, S. Bhattacharya, Unemployment rate forecasting: A hybrid approach, Comput. Econ., 57 (2021), 183–201. http://dx.doi.org/10.1007/s10614-020-10040-2 doi: 10.1007/s10614-020-10040-2
![]() |
[7] |
A. A. Davidescu, S. A. Apostu, L. A. Stoica, Socioeconomic effects of COVID-19 pandemic: Exploring uncertainty in the forecast of the romanian unemployment rate for the period 2020–2023, Sustainability, 13 (2021), 7078. http://dx.doi.org/10.3390/su13137078 doi: 10.3390/su13137078
![]() |
[8] |
T. Ikeda, K. Sugiyama, J. Aida, T. Tsuboya, K. Osaka, The contextual effect of Area-Level unemployment rate on lower back pain: A multilevel analysis of three consecutive surveys of 962,586 workers in Japan, Int. J. Env. Res. Pub. He., 16 (2019), 4016. http://dx.doi.org/10.3390/ijerph16204016 doi: 10.3390/ijerph16204016
![]() |
[9] | A. Kavkler, D. E. Dncic, A. G. Babucea, I. Bicanic, B. Bohm, D. Tevdovski, et al., Cox regression models for unemployment duration in Romania, Austria, Slovenia, Croatia and Macedonia, Rom. J. Econ. Forecast., 10 (2009), 81–104. |
[10] |
C. Katris, Prediction of unemployment rates with time series and machine learning techniques, Comput. Econ., 55 (2019), 1–34. http://dx.doi.org/10.1007/s10614-019-09908-9 doi: 10.1007/s10614-019-09908-9
![]() |
[11] | S. Kim, K. Koh, S. Boyd, D. Gorinevsky, ℓ1 trend filtering, SIAM Rev., 51 (2009), 339–360. |
[12] |
B. Seetanah, N. U. H. Sookia, P. N. Gonpot, B. Seetanah, Out-of-sample forecasting of the Canadian unemployment rates using univariate models, Appl. Econ. Lett., 24 (2017), 1097–1101. http://dx.doi.org/10.1080/13504851.2016.1257208 doi: 10.1080/13504851.2016.1257208
![]() |
[13] |
A. Lahiani, O. Scaillet, Testing for threshold effect in ARFIMA models: Application to US unemployment rate data, Int. J. Forecast., 25 (2009), 418–428. http://dx.doi.org/10.1016/j.ijforecast.2009.01.004 doi: 10.1016/j.ijforecast.2009.01.004
![]() |
[14] | B. J. Loboa, M. R. Alama, B. E. Whitacreb, Broadband speed and unemployment rates: Data and measurementissues, Telecommun. Policy, 44 (2020), 101829. |
[15] |
F. Li, C. F. Wang, Develop a multi-linear-trend fuzzy information granule based short-term time series forecasting model with k-medoids clustering, Inform. Sciences, 629 (2023), 358–375. http://dx.doi.org/10.1016/j.ins.2023.01.122 doi: 10.1016/j.ins.2023.01.122
![]() |
[16] |
H. Lai, Y. A. Khan, A. Thaljaoui, W. Chammam, S. Z. Abbas, COVID-19 pandemic and unemployment rate: A hybrid unemployment rate prediction approach for developed and developing countries of Asia, Soft Comput., 27 (2023), 615. http://dx.doi.org/10.1007/s00500-021-05871-6 doi: 10.1007/s00500-021-05871-6
![]() |
[17] | T. Y. Lin, Granular computing on binary relations Ⅰ: datamining and neighborhood systems, Ⅱ: Rough setrepresentations and belief functions, rough sets in knowledge discovery, Heidelberg: Physica-Verlag, 1998,107. |
[18] | T. Y. Lin, Granular computing rough set perspective, Newsl. IEEE Comput. Intell. Soc., 2 (2005), 1543. |
[19] |
Z. Li, W. Xu, L. Zhang, R. Y. K. Lau, An ontology-based Web mining method for unemployment rate prediction, Decis. Support Syst., 66 (2014), 114–122. http://dx.doi.org/10.1016/j.dss.2014.06.007 doi: 10.1016/j.dss.2014.06.007
![]() |
[20] |
A. Milner, A. Kavanagh, L. Krnjacki, R. Bentley, A. D. LaMontagne, Area-level unemployment and perceived job insecurity: Evidence from a longitudinal survey conducted in the Australian working-age population, Ann. Occupat. Hyg., 58 (2014), 171–181. http://dx.doi.org/10.1093/annhyg/met066 doi: 10.1093/annhyg/met066
![]() |
[21] |
E. Olmedo, Forecasting spanish unemployment using near neighbour and neural net techniques, Comput. Econ., 43 (2014), 183–197. http://dx.doi.org/10.1007/s10614-013-9371-1 doi: 10.1007/s10614-013-9371-1
![]() |
[22] | Z. Pawlak, Rough sets, Int. J. Comput. Inform. Sci., 11 (1982), 341. |
[23] |
D. J. Roelfs, E. Shor, A. Blank, J. E. Schwartz, Misery loves company? A meta-regression examining aggregate unemployment rates and the unemployment-mortality association, Ann. Epidemiol., 25 (2015), 312–322. http://dx.doi.org/10.1016/j.annepidem.2015.02.005 doi: 10.1016/j.annepidem.2015.02.005
![]() |
[24] |
N. I. Sapankevych, R. Sankar, Time series prediction using support vector machines: A survey, IEEE Comput. Intell. M., 4 (2009), 24–38. http://dx.doi.org/10.1109/MCI.2009.932254 doi: 10.1109/MCI.2009.932254
![]() |
[25] | T. Sun, Y. Liu, Chaotic time series prediction based on fuzzy information granulation and hybrid neural networks, Inform. Control, 2022, 1–8. |
[26] |
S. J. Taylor, B. Letham, Forecasting at scale, Am. Stat., 72 (2018), 37–45. http://dx.doi.org/10.1080/00031305.2017.1380080 doi: 10.1080/00031305.2017.1380080
![]() |
[27] |
Y. Tang, F. Yu, W. Pedrycz, X. Yang, J. Wang, S. Liu, Building trend fuzzy granulation based LSTM recurrent neural network for long-term time series forecasting, IEEE T. Fuzzy Syst., 30 (2022), 1599–1613. http://dx.doi.org/10.1109/TFUZZ.2021.3062723 doi: 10.1109/TFUZZ.2021.3062723
![]() |
[28] |
F. Wang, H. Yang, Expansion of the existence range of the difference between two fuzzy numbers, Pure Math., 11 (2021), 1451–1463. http://dx.doi.org/10.12677/PM.2021.118163 doi: 10.12677/PM.2021.118163
![]() |
[29] | M. C. Wang, F. L. Lin, H. H. Su, P. L. Kuo, Revisiting the relationship between suicide and unemployment in Mexico: Evidence from linear and nonlinear Co-integration, Front. Public Health, 8 (2020), 60. |
[30] | Q. Wang, The effects of unemployment rate on health status of Chinese people, Iran. J. Public Health, 44 (2015), 28–35. |
[31] |
Q. Wang, J. J. Shen, C. Cochran, Unemployment rate, smoking in China: Are they related, Env. Res. Pub. He., 13 (2016), 113. http://dx.doi.org/10.3390/ijerph13010113 doi: 10.3390/ijerph13010113
![]() |
[32] | G. X. Xu, Statistical prediction and decision, Shanghai: Shanghai University of Finance and Economics Press, 2008. |
[33] |
D. S. Yamacli, S. Yamacli, Estimation of the unemployment rate in Turkey: A comparison of the ARIMA and machine learning models including Covid-19 pandemic periods, Heliyon, 9 (2023), e12796. http://dx.doi.org/10.1016/j.heliyon.2023.e12796 doi: 10.1016/j.heliyon.2023.e12796
![]() |
[34] |
X. Yang, F. Yu, W. Pedrycz, Long-term forecasting of time series based on linear fuzzy information granules and fuzzy inference system, Int. J. Approx. Reason., 81 (2017), 1–27. http://dx.doi.org/10.1016/j.ijar.2016.10.010 doi: 10.1016/j.ijar.2016.10.010
![]() |
[35] | L. Zhang, B. Zhang, A quotient space approximationmodel of multiresolution signal analysis, J. Comput. Sci. Technol., 2015, 90. |
[36] | L. A. Zadeh, Fuzzy sets, Inform. Control, 8 (1965), 338. |
[37] |
L. A. Zadeh, Outline of a new approach to the analysis of complex systems and decision processes, IEEE T. Syst., 3 (1973), 28–44. http://dx.doi.org/10.1109/TSMC.1973.5408575 doi: 10.1109/TSMC.1973.5408575
![]() |
[38] |
L. A. Zadeh, Concept of a linguistic variable and itsapplication to approximate reasoning—Ⅱ, Inform. Sciences, 8 (1975), 301–357. http://dx.doi.org/10.1016/0020-0255(75)90046-8 doi: 10.1016/0020-0255(75)90046-8
![]() |
[39] | L. A. Zadeh, Fuzzy sets and information granularity, Adv. Fuzzy Set Th. Appl., 1979, 3–18. |
[40] |
L. A. Zadeh, Fuzzy logic = computing with words, IEEE T. Fuzzy Syst., 4 (1996), 103–111. http://dx.doi.org/10.1109/91.493904 doi: 10.1109/91.493904
![]() |
[41] | L. A. Zadeh, Toward a theory of fuzzy information granulation and its centrality in human reasoning and fuzzylogic, Fuzzy Set. Syst., 90 (1997), 111–127. |
[42] |
L. A. Zadeh, Some reflections on soft computing, granular computing and their roles in the conception, design and utilization of information/intelligent systems, Soft Comput., 2 (1998), 23–25. http://dx.doi.org/10.1007/s005000050030 doi: 10.1007/s005000050030
![]() |
[43] |
Y. Q. Zhang, Constructive granular systems with universal approximation and fast knowledge discovery, IEEE T. Fuzzy Syst., 13 (2005), 48–57. http://dx.doi.org/10.1109/TFUZZ.2004.839657 doi: 10.1109/TFUZZ.2004.839657
![]() |
1. | Pengfei Zhang, Yuxin Zhao, Dexian Wang, Yujie Zhang, Zheng Yu, Fuzzy Rough Set Models Based on Fuzzy Similarity Relation and Information Granularity in Multi-Source Mixed Information Systems, 2024, 12, 2227-7390, 4039, 10.3390/math12244039 | |
2. | Saptaporni Karmakar, Arkaprovo Chakraborty, Deterministic, Stochastic, and Deep Learning Approaches to Understand the Economic Fluctuations in India, 2025, 0170-4214, 10.1002/mma.10700 |
Model | 3 months ahead forecast | 6 months ahead forecast | |||||
MAE | MAPE | RMSE | MAE | MAPE | RMSE | ||
ES | 0.489 | 0.026 | 0.433 | 0.594 | 0.030 | 0.644 | |
ARIMA | 1.340 | 0.071 | 1.098 | 2.420 | 0.119 | 2.719 | |
Prophet | 0.900 | 0.048 | 0.767 | 1.485 | 0.074 | 1.681 | |
SVR | 0.747 | 0.041 | 0.574 | 2.217 | 0.107 | 2.893 | |
LSTM | 0.379 | 0.020 | 0.377 | 0.503 | 0.025 | 0.610 | |
ANN | 1.093 | 0.058 | 0.947 | 1.878 | 0.109 | 2.564 | |
GM | 0.746 | 0.041 | 0.574 | 1.318 | 0.066 | 1.463 | |
FIG-GM | 0.293 | 0.016 | 0.255 | 0.276 | 0.013 | 0.296 |
Model | 3 months ahead forecast | 6 months ahead forecast | |||||
MAE | MAPE | RMSE | MAE | MAPE | RMSE | ||
ES | 5.329 | 0.452 | 2.051 | 3.077 | 0.276 | 4.020 | |
ARIMA | 2.783 | 0.255 | 2.246 | 4.360 | 0.518 | 4.714 | |
Prophet | 8.160 | 0.705 | 4.041 | 6.450 | 0.659 | 6.735 | |
SVR | 4.293 | 0.359 | 1.293 | 2.195 | 0.185 | 3.316 | |
LSTM | 6.477 | 0.566 | 3.123 | 5.288 | 0.546 | 5.501 | |
ANN | 4.713 | 0.401 | 1.951 | 2.782 | 0.253 | 3.539 | |
GM | 5.643 | 0.483 | 2.489 | 3.727 | 0.357 | 4.313 | |
FIG-GM | 2.630 | 0.226 | 1.201 | 2.040 | 0.207 | 2.158 |
Model | 6 months ahead forecast | 12 months ahead forecast | |||||
MAE | MAPE | RMSE | MAE | MAPE | RMSE | ||
ES | 0.102 | 0.041 | 0.121 | 0.091 | 0.036 | 0.109 | |
ARIMA | 0.075 | 0.029 | 0.090 | 0.118 | 0.044 | 0.147 | |
Prophet | 0.122 | 0.048 | 0.145 | 0.163 | 0.062 | 0.208 | |
SVR | 0.065 | 0.026 | 0.066 | 0.300 | 0.119 | 0.848 | |
LSTM | 0.088 | 0.035 | 0.107 | 0.103 | 0.039 | 0.127 | |
ANN | 0.068 | 0.028 | 0.085 | 0.084 | 0.032 | 0.105 | |
GM | 0.175 | 0.070 | 0.189 | 0.131 | 0.051 | 0.150 | |
FIG-GM | 0.060 | 0.026 | 0.076 | 0.079 | 0.030 | 0.085 |
Model | 6 months ahead forecast | 12 months ahead forecast | |||||
MAE | MAPE | RMSE | MAE | MAPE | RMSE | ||
ES | 0.205 | 0.069 | 0.206 | 0.128 | 0.043 | 0.151 | |
ARIMA | 0.158 | 0.054 | 0.167 | 0.115 | 0.039 | 0.133 | |
Prophet | 0.330 | 0.112 | 0.355 | 0.286 | 0.096 | 0.309 | |
SVR | 0.210 | 0.071 | 0.214 | 0.173 | 0.058 | 0.180 | |
LSTM | 0.083 | 0.028 | 0.096 | 0.112 | 0.037 | 0.125 | |
ANN | 0.157 | 0.053 | 0.163 | 0.104 | 0.035 | 0.125 | |
GM | 0.357 | 0.121 | 0.359 | 0.428 | 0.142 | 0.436 | |
FIG-GM | 0.010 | 0.003 | 0.014 | 0.050 | 0.016 | 0.100 |
Model | 3 months ahead forecast | 6 months ahead forecast | |||||
MAE | MAPE | RMSE | MAE | MAPE | RMSE | ||
ES | 0.489 | 0.026 | 0.433 | 0.594 | 0.030 | 0.644 | |
ARIMA | 1.340 | 0.071 | 1.098 | 2.420 | 0.119 | 2.719 | |
Prophet | 0.900 | 0.048 | 0.767 | 1.485 | 0.074 | 1.681 | |
SVR | 0.747 | 0.041 | 0.574 | 2.217 | 0.107 | 2.893 | |
LSTM | 0.379 | 0.020 | 0.377 | 0.503 | 0.025 | 0.610 | |
ANN | 1.093 | 0.058 | 0.947 | 1.878 | 0.109 | 2.564 | |
GM | 0.746 | 0.041 | 0.574 | 1.318 | 0.066 | 1.463 | |
FIG-GM | 0.293 | 0.016 | 0.255 | 0.276 | 0.013 | 0.296 |
Model | 3 months ahead forecast | 6 months ahead forecast | |||||
MAE | MAPE | RMSE | MAE | MAPE | RMSE | ||
ES | 5.329 | 0.452 | 2.051 | 3.077 | 0.276 | 4.020 | |
ARIMA | 2.783 | 0.255 | 2.246 | 4.360 | 0.518 | 4.714 | |
Prophet | 8.160 | 0.705 | 4.041 | 6.450 | 0.659 | 6.735 | |
SVR | 4.293 | 0.359 | 1.293 | 2.195 | 0.185 | 3.316 | |
LSTM | 6.477 | 0.566 | 3.123 | 5.288 | 0.546 | 5.501 | |
ANN | 4.713 | 0.401 | 1.951 | 2.782 | 0.253 | 3.539 | |
GM | 5.643 | 0.483 | 2.489 | 3.727 | 0.357 | 4.313 | |
FIG-GM | 2.630 | 0.226 | 1.201 | 2.040 | 0.207 | 2.158 |
Model | 6 months ahead forecast | 12 months ahead forecast | |||||
MAE | MAPE | RMSE | MAE | MAPE | RMSE | ||
ES | 0.102 | 0.041 | 0.121 | 0.091 | 0.036 | 0.109 | |
ARIMA | 0.075 | 0.029 | 0.090 | 0.118 | 0.044 | 0.147 | |
Prophet | 0.122 | 0.048 | 0.145 | 0.163 | 0.062 | 0.208 | |
SVR | 0.065 | 0.026 | 0.066 | 0.300 | 0.119 | 0.848 | |
LSTM | 0.088 | 0.035 | 0.107 | 0.103 | 0.039 | 0.127 | |
ANN | 0.068 | 0.028 | 0.085 | 0.084 | 0.032 | 0.105 | |
GM | 0.175 | 0.070 | 0.189 | 0.131 | 0.051 | 0.150 | |
FIG-GM | 0.060 | 0.026 | 0.076 | 0.079 | 0.030 | 0.085 |
Model | 6 months ahead forecast | 12 months ahead forecast | |||||
MAE | MAPE | RMSE | MAE | MAPE | RMSE | ||
ES | 0.205 | 0.069 | 0.206 | 0.128 | 0.043 | 0.151 | |
ARIMA | 0.158 | 0.054 | 0.167 | 0.115 | 0.039 | 0.133 | |
Prophet | 0.330 | 0.112 | 0.355 | 0.286 | 0.096 | 0.309 | |
SVR | 0.210 | 0.071 | 0.214 | 0.173 | 0.058 | 0.180 | |
LSTM | 0.083 | 0.028 | 0.096 | 0.112 | 0.037 | 0.125 | |
ANN | 0.157 | 0.053 | 0.163 | 0.104 | 0.035 | 0.125 | |
GM | 0.357 | 0.121 | 0.359 | 0.428 | 0.142 | 0.436 | |
FIG-GM | 0.010 | 0.003 | 0.014 | 0.050 | 0.016 | 0.100 |