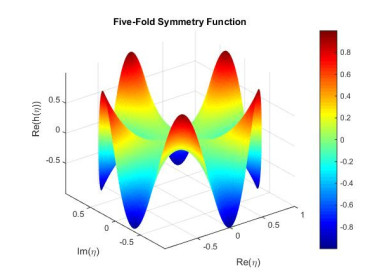
Attribute reduction of a decision information system (DIS) using multi-granulation rough sets is one of the important applications of granular computing. Constructing discernibility matrices by rough sets to get attribute reducts of a DIS is an important reduction method. By analyzing the commonalities between the multi-granulation reduction structure of decision multi-granulation spaces and that of incomplete DISs based on discernibility tool, this paper explored a general model for the multi-granulation reduction of DISs by the discernibility technique. First, the definition of the generalized neighborhood decision information system (GNDIS) was presented. Second, knowledge reduction of GNDISs by multi-granulation rough sets was discussed, and discernibility matrices and discernibility functions were constructed to characterize multi-granulation reduction structures of GNDISs. Third, the multi-granulation reduction structures of decision multi-granulation spaces and incomplete DISs were characterized by the reduction theory of GNDISs based on discernibility. Then, the multi-granulation reduction of GNDISs by the discernibility tool provided a theoretical foundation for designing algorithms of multi-granulation reduction of DISs.
Citation: Yanlan Zhang, Changqing Li. The discernibility approach for multi-granulation reduction of generalized neighborhood decision information systems[J]. AIMS Mathematics, 2024, 9(12): 35471-35502. doi: 10.3934/math.20241684
[1] | Syed Ghoos Ali Shah, Shahbaz Khan, Saqib Hussain, Maslina Darus . q-Noor integral operator associated with starlike functions and q-conic domains. AIMS Mathematics, 2022, 7(6): 10842-10859. doi: 10.3934/math.2022606 |
[2] | Hanen Louati, Afrah Al-Rezami, Erhan Deniz, Abdulbasit Darem, Robert Szasz . Application of q-starlike and q-convex functions under (u,v)-symmetrical constraints. AIMS Mathematics, 2024, 9(12): 33353-33364. doi: 10.3934/math.20241591 |
[3] | Humaira Kalsoom, Muhammad Amer Latif, Muhammad Idrees, Muhammad Arif, Zabidin Salleh . Quantum Hermite-Hadamard type inequalities for generalized strongly preinvex functions. AIMS Mathematics, 2021, 6(12): 13291-13310. doi: 10.3934/math.2021769 |
[4] | Huo Tang, Kadhavoor Ragavan Karthikeyan, Gangadharan Murugusundaramoorthy . Certain subclass of analytic functions with respect to symmetric points associated with conic region. AIMS Mathematics, 2021, 6(11): 12863-12877. doi: 10.3934/math.2021742 |
[5] | Jianhua Gong, Muhammad Ghaffar Khan, Hala Alaqad, Bilal Khan . Sharp inequalities for q-starlike functions associated with differential subordination and q-calculus. AIMS Mathematics, 2024, 9(10): 28421-28446. doi: 10.3934/math.20241379 |
[6] | İbrahim Aktaş . On some geometric properties and Hardy class of q-Bessel functions. AIMS Mathematics, 2020, 5(4): 3156-3168. doi: 10.3934/math.2020203 |
[7] | Bo Wang, Rekha Srivastava, Jin-Lin Liu . Certain properties of multivalent analytic functions defined by q-difference operator involving the Janowski function. AIMS Mathematics, 2021, 6(8): 8497-8508. doi: 10.3934/math.2021493 |
[8] | Haiyan Zhou, K. A. Selvakumaran, S. Sivasubramanian, S. D. Purohit, Huo Tang . Subordination problems for a new class of Bazilevič functions associated with k-symmetric points and fractional q-calculus operators. AIMS Mathematics, 2021, 6(8): 8642-8653. doi: 10.3934/math.2021502 |
[9] | Aisha M. Alqahtani, Rashid Murtaza, Saba Akmal, Adnan, Ilyas Khan . Generalized q-convex functions characterized by q-calculus. AIMS Mathematics, 2023, 8(4): 9385-9399. doi: 10.3934/math.2023472 |
[10] | Bakhtiar Ahmad, Muhammad Ghaffar Khan, Basem Aref Frasin, Mohamed Kamal Aouf, Thabet Abdeljawad, Wali Khan Mashwani, Muhammad Arif . On q-analogue of meromorphic multivalent functions in lemniscate of Bernoulli domain. AIMS Mathematics, 2021, 6(4): 3037-3052. doi: 10.3934/math.2021185 |
Attribute reduction of a decision information system (DIS) using multi-granulation rough sets is one of the important applications of granular computing. Constructing discernibility matrices by rough sets to get attribute reducts of a DIS is an important reduction method. By analyzing the commonalities between the multi-granulation reduction structure of decision multi-granulation spaces and that of incomplete DISs based on discernibility tool, this paper explored a general model for the multi-granulation reduction of DISs by the discernibility technique. First, the definition of the generalized neighborhood decision information system (GNDIS) was presented. Second, knowledge reduction of GNDISs by multi-granulation rough sets was discussed, and discernibility matrices and discernibility functions were constructed to characterize multi-granulation reduction structures of GNDISs. Third, the multi-granulation reduction structures of decision multi-granulation spaces and incomplete DISs were characterized by the reduction theory of GNDISs based on discernibility. Then, the multi-granulation reduction of GNDISs by the discernibility tool provided a theoretical foundation for designing algorithms of multi-granulation reduction of DISs.
Geometric Function Theory (GFT) is a field within complex analysis that focuses on the geometric and analytic properties of holomorphic (complex-differentiable) functions and their mappings. It focuses on topics such as conformal mappings, univalent (injective) functions, and the distortion of shapes induced by these mappings. Central concepts include the Riemann mapping theorem, Schwarzian derivatives, and extremal problems, which explore the limits of function behavior. GFT has wide-ranging applications in areas such as physics and engineering, and computational sciences, particularly in solving boundary value problems and modeling fluid dynamics. By blending geometry and analysis, GFT provides deep insights into the structure and behavior of complex functions. Let us define the collection of all functions that are analytic within the open unit disk, denoted as ˜G, by ¯H(˜G). Here, the open unit disk ˜G consists of all complex numbers η satisfying |η|<1. Within ¯H(˜G), we consider a specific subset ¯H, containing functions represented by the following power series:
ψ(η)=η+∞∑v=2avηv, |
where η lies in ˜G. The functions in ¯H are characterized by having the coefficient of the first-order term equal to 1, and all higher-order terms contribute via the coefficients av for v≥2. Let ψ,g∈¯H be two analytic functions, where ψ(η)=η+∑∞v=2avηv and g(η)=η+∑∞v=2bvηi. The convolution of ψ and g, denoted ψ∗g, is referred to as:
(ψ∗g)(η)=η+∞∑v=2avbvϑv. |
This operation involves multiplying the corresponding coefficients from the series expansions of ψ and g.
In complex analysis, (j,k)-symmetrical functions are a special class of analytic functions characterized by symmetry properties involving two integers j and k. These functions are defined in the unit disk ˜G and exhibit a form of rotational symmetry that relates their values under transformations by roots of unity.
In their influential 1995 study, Liczberski and Polubinski [1] proposed the concept of (j,k) -symmetrical functions, where j and k are integers with j being an element of {0,1,2,…,k−1} and k≥2. Let ˜U denote a domain possessing j -fold symmetry, and let j be an integer within the specified range. A function ψ:˜U→C is defined as (j,k) -it is considered symmetrical if it satisfies the following condition for every η∈˜U :
ψ(εη)=εjψ(η), |
where ε=e2πik represents any symmetry operation associated with the j -fold symmetry of ˜U.
The class of all (j,k)-symmetrical functions is represented by ¯Hkj. Within this structure, ¯H02 corresponds to the class of even functions, ¯H12 represents the class of odd functions, and ¯H1j consists of functions that exhibit symmetry under j -fold operations.
Based on the decomposition theorem proposed by Liczberski and Polubinski [1], any function ψ:˜U→C defined on a j -fold symmetric domain ˜U can be uniquely expressed as a combination of (j,k) -symmetrical components.
ψ(η)=k−1∑j=0ψj,k(η),ψj,k(η)=k−1k−1∑r=0ε−rjψ(εrη),∀η∈U. | (1.1) |
Alternatively, (1.1) can be expressed as
ψj,k(η)=∞∑v=1δvjavηv,whereδvj={1,v=lk+j;0,v≠lk+j;. | (1.2) |
To demonstrate the concept of (j,k)-symmetrical functions, let's examine some straightforward examples:
● Three-Fold Symmetry (j=1,k=3).
For k=3, functions exhibit symmetry under rotation by 2π/3, satisfying ψ(e2πi/3η)=e2πi/3ψ(η).
ψ(η)=η2sin(3arg(η)), |
where arg(η) is the argument of η. Under rotation η→e2πi/3η, the argument shifts by 2π/3, resulting in the required symmetry.
● Four-Fold Symmetry (j=2,k=4).
For k=4, functions are symmetric under rotation by π/2, satisfying ψ(eπi/2η)=−ψ(η).
ψ(η)=η2cos(2arg(η)). |
Under rotation η→eπi/2η, the symmetry condition ψ(eπi/2η)=−ψ(η) is satisfied.
● Five-Fold Symmetry (j=1,k=5).
For k=5, functions are symmetric under rotation by π/5, satisfying ψ(eπi/5η)=e2πi/5ψ(η), where e2πi/5 is the primitive 5th root of unity. One such function could be
ψ(η)=η4sin(5arg(η)). |
Geometrically, For different values of j and k, the image domains of the unit disk are illustrated below (see Figures 1 and 2).
The surface plot of the function f(η)=η4sin(5arg(η)) clearly shows its 5-fold symmetry, repeating every 2π5 rotation. The radial variation of the function's magnitude forms a smooth surface across the unit disk, with sinusoidal patterns emerging from the argument of η. This 3D visualization helps to understand how the function's symmetry influences its behavior in the complex plane. Such symmetry is widely applied in signal processing for analyzing periodic signals, fluid dynamics for modeling wave patterns, crystal structures for atomic arrangements, and electromagnetic theory for designing rotationally symmetric fields like antennas.
With the utmost respect, let us define the family Δ of Schwarz functions as follows:
¯Ω:={w∈¯H(˜G)∣|w(η)|<1,w(0)=0,∀η∈˜G}. |
Within the region ˜G, let ψ(η) and g(η) be two analytic functions. We define ψ(η) as subordinate to g(η), denoted as ψ(η)≺g(η), if there exists an analytic function w(η)∈¯Ω such that ψ(η)=g(w(η)) for all η∈˜G. Furthermore, if g(η) is univalent in ˜G, then subordination ψ(η)≺g(η) is equivalent to conditions ψ(0)=g(0) and ψ(˜G)⊆g(˜G). This result is discussed in [2]. This relation ensures that ψ(η) inherits important geometric and analytic properties from g(η), such as convexity, starlikeness, and boundedness. Discussing its implications further could involve exploring its role in function theory, its connection to differential subordination, and how it affects the growth, distortion, and coefficient estimates of ψ(η). Additionally, applications of subordination in complex analysis, geometric function theory, and operator theory can be elaborated upon.
Definition 1.1. [3] Let P represent the class of functions p∈¯H(˜G) that satisfy p(0)=1 and Re{p(η)}>0 for all η∈˜G. An equivalent characterization of p∈P is defined by its representation p(η)=1+w(η)1−w(η) for some w∈¯Ω.
Janowski [4] introduced the class P[A,B] for −1≤B<A≤1, defined as follows: A function ψ is considered to belong to the class P[A,B] if and only if it satisfies one of the following equivalent conditions:
ψ(η)≺1+Aη1+Bη |
or
ψ(η)=1+Aw(η)1+Bw(η), |
for some w(η)∈¯Ω. The study of q -Janowski type functions extends classical Janowski functions by incorporating q -calculus, offering a more flexible framework for geometric function theory. These functions are significant due to their applications in mathematical physics, combinatorics, and quantum calculus, where discrete and continuous structures interact.
With the utmost respect, to establish a new class of q -Janowski symmetrical functions within the framework of ˜G, we begin by revisiting the core principles of quantum calculus, often known as q -calculus. In [5,6], Jackson introduced and examined the q -derivative operator. ∂qψ(η) defined as:
∂qψ(η)={ψ′(η),q=1.ψ′(0),η=0,ψ(η)−ψ(qη)η(1−q),η≠0,0<q<1, | (1.3) |
and
[v]q=1+q+q2+...+qv−1=1−qv1−q. | (1.4) |
Equivalently, (1.3) may be written as
∂qψ(η)=1+∞∑v=2[v]qavηv−1η≠0, |
Quantum calculus has emerged as an essential tool for addressing mathematical challenges within the realms of discrete and quantum systems. By harnessing the unique properties of the parameter q, it extends and enriches traditional calculus techniques. The application of the q -derivative has led scholars to explore its potential in geometric function theory. For instance, Ismail et al. [7] offered significant insights into the association of quantum calculus with geometric function theory. More recently, researchers such as Srivastava et al. [8] have employed q -calculus to investigate the modification and radius of univalence and starlikeness within various subsets of q-starlike functions. Additionally, Naeem et al. [9] have explored subfamilies of q -convex functions associated with Janowski functions and domains that are q-conic. At the same time, in their work, Mohammed and Darus [10] studied the approximation and geometric characteristics of q-operators in particular subclasses of analytic functions within a compact disk. In parallel, significant advancements have been made in the study of q -calculus and its various classes [11,12]. In [13], a new subclass of analytic and bi-univalent functions was defined using the symmetric q-calculus. Bulboacˇa, Frasin, and their collaborators [14,15] have achieved numerous notable results across diverse classes that were constructed by making use of the (j,k)-symmetrical functions and the q-derivative. Moreover, Srivastava [16] has published an extensive survey and review paper that provides a valuable resource for researchers and scholars engaged in this rapidly evolving field.
Definition 1.2. Given arbitrary fixed numbers q, α, and β, 0<q<1, −1≤B<A≤1, let ψ∈¯H and ∂qψ be defined on a q-geometric, such that
|(B−1)η∂qψ(η)ψj,k(η)−(A−1)(B+1)η∂qψ(η)ψj,k(η)−(A+1)−11−q|<11−q, | (1.5) |
then ψ(η)∈¯Tj,kq(A,B). where ψj,k is defined in (1.1).
We can readily provide the equivalent form of Definition 1.2, which may prove beneficial for further exploration within the function class. ¯Tj,kq(A,B) as:
η∂qψ(η)ψj,k(η)≺2+[A(1+q)+(1−q)]η2+[B(1+q)+(1−q)]η. |
We define ¯Kj,kq(A,B) as the subclass of ¯H that includes all functions ψ for which
η∂qψ(η)∈¯Tj,kq(A,B). | (1.6) |
In specific cases involving the parameters j, k, q, A, and B, the class ¯Tj,kq(A,B) gives rise to several well-known subclasses of ¯H. For example, ¯T0,1q(A,B):=˜Sq(A,B) reduces to the class described by Srivastava et al. [17]. Similarly, ¯Tj,k1(A,B):=˜Sj,k(A,B) (introduced by Latha et al. [18]) and ¯T1,k1(A,B):=˜Sj(A,B) (motivated by the author Latha and Darus [19]) are notable subclasses. Additionally, ¯T1,1q(1−2λ,−1,0)=˜Sq(λ) (introduced by Agrawal and Sahoo in [20]) and ¯T1,11(1−2λ,−1,0)=˜S(λ) (the well-known class of starlike functions of order λ, which is introduced by Robertson [21]) are also important examples. Other significant subclasses include ¯T1,k1(1,−1,0):=˜Sk (inspired by Sakaguchi [22]) and ¯T1,1q(1,−1,0)=˜Sq (originally introduced by Ismail et al. [7]). Furthermore, ¯T1,11(A,B):=˜S[A,B] simplifies to the widely recognized class defined by Janowski [4], and ¯T1,11(1,−1)=˜S∗, the class introduced by Nevanlinna [23].
Consider the following functions as candidates for membership in the class ¯Tj,kq(A,B) :
1) Fractional exponential function
ψ(η)=eq(γη)=∞∑n=0(γη)n[n]q!,γ>0. |
The q -derivative of eq(γη) is:
∂qψ(η)=γeq(γη). |
Substituting this into the left-hand side of (1.5), we verify that the function belongs to ¯Tj,kq(A,B).
2) Deformed logarithmic function
ψ(η)=ηlnq(1+η),lnq(1+η)=∫η0dx1+qx. |
The q -derivative of ψ(η) is:
∂qψ(η)=1lnq(1+η)−η(1+qη)ln2q(1+η). |
By verifying Eq (1.5), we confirm that this function satisfies the required properties.
3) Rational function
ψ(η)=η(1−qη)γ,γ>0. |
The q -derivative is:
∂qψ(η)=(1−qγ)(1−qη)γ+1. |
Substituting this expression into (1.5), we check that it meets the subordination condition.
Thus, each of these functions serves as a valid example illustrating Definition 1.2.
In this study, we aim to explore the properties of functions within the classes ¯Tj,kq(A,B) and ¯Kj,kq(A,B) defined using q -derivatives and (j,k) -symmetrical functions. Our goals include deriving conditions for membership in these classes, understanding the impact of symmetry on function behavior, and extending classical results in complex analysis by examining the limit as q→1−. Through examples and new theorems, we seek to deepen our understanding of the role of symmetry in these mathematical frameworks and its applications in geometric function theory.
Theorem 2.1. A function ψ∈¯Kj,kq(A,B) if and only if
1ψ[ψ∗((η−qη3)(2+[B(1+q)+(1−q)]eiϕ)(1−η)(1−qη)(1−q2η)−(2+[A(1+q)+(1−q)]eiϕ)η(1−δjη)(1−ujqη))]≠0,|η|<R≤1, |
where 0≤ϕ<2π,−1≤B<A≤1,0<q<1 and uj is given by (2.7).
Proof. We have, ψ∈¯Kj,kq(A,B) if and only if
∂q(η∂qψ(η))∂qψj,k(η)≠2+[(1−q)+A(1+q)]eiϕ2+[(1−q)+B(1+q)]eiϕ,|η|<R, |
which implies
∂q(η∂qψ(η)){2+[(1−q)+B(1+q)]eiϕ}−∂qψj,k(η){2+[A(1+q)+(1−q)]eiϕ}≠0. | (2.1) |
Step 1: Expressing q-derivatives as series expansions. Assume that ψ has a power series representation:
ψ(η)=η+∞∑v=2avηv. | (2.2) |
Computing its first q-derivative:
∂qψ(η)=1+∞∑v=2[v]qavηv−1. | (2.3) |
For the second q-derivative:
∂q(η∂qψ(η))=1+∞∑v=2[v]2qavηv−1. | (2.4) |
This can be rewritten using convolution as:
∂q(η∂qψ)=∂qψ∗1(1−η)(1−qη). | (2.5) |
For the (j,k)-symmetrical component:
∂qψj,k(η)=∂qψ∗1(1−ujη)=∞∑v=1[v]quvjavηv−1, | (2.6) |
where
uvj=δv,j,andδv,jis given by(1.2). | (2.7) |
Step 2: Reformulating the main inequality. Substituting these into inequality (2.1):
∂qψ∗(2+[(1−q)+B(1+q)]eiϕ(1−η)(1−qη)−2+[(1−q)+A(1+q)]eiϕ1−ujη)≠0, | (2.8) |
simplifying (2.8), we obtain
1η[η∂qψ∗((2+[(1−q)+B(1+q)]eiϕ)η(1−η)(1−qη)−(2+[(1−q)+A(1+q)]eiϕ)η1−ujη)]≠0, | (2.9) |
since η∂qψ∗g=ψ∗η∂qg, we can write Eq (2.9) as
1η[ψ∗((η−qη3)(2+[B(1+q)+(1−q)]eiϕ)(1−η)(1−qη)(1−q2η)−(2+[A(1+q)+(1−q)]eiϕ)η(1−ujη)(1−ujqη))]≠0. |
Remark 2.2. When q→1− and for special values of j, k, A, and B, the following result, proven by Ganesan et al. in [24] and Silverman et al. in [25], holds true.
Corollary 2.3. [24] The function ψ∈K(A,B) in |η|<R≤1, if and only if
1η[ψ∗(Aρ+Bρ+2)B−Aη2+ρη(1−η)3]≠0. |
Theorem 2.4. A function ψ∈¯Tj,kq(A,B) if and only if
1η[ψ∗((2+[(1−q)+B(1+q)]eiϕ)η(1−η)(1−qη)−(2+[(1−q)+B(1+q)]eiϕ)η1−ujη)]≠0,|η|<1, | (2.10) |
where 0≤ϕ<2π, −1≤B<A≤1,0<q<1 and uj is given by (2.7).
Proof. Since ψ(η)∈¯Tj,kq(A,B)⟺g(η)=∫η0ψ(ϖ)ϖdqϖ∈¯Kj,kq(A,B), we have
1η[g∗((η−qη3)(2+[(1+q)B+(1−q)]eiϕ)(1−η)(1−qη)(1−q2η)−(2+[(1−q)+A(1+q)]eiϕ)η(1−ujη)(1−ujqη))]=1η[ψ∗((2+[(1+q)B+(1−q)]eiϕ)η(1−η)(1−qη)−(2+[(1−q)+A(1+q)]eiϕ)η1−ujη)]. |
Therefore, the result is a direct consequence of Theorem 2.4.
Remark 2.5. As q→1, the q -difference operator ∂q approaches the standard differentiation operator, leading to a transition from q -deformed function properties to their classical counterparts. This transition enables the recovery of well-known results in analytic function theory, including Janowski starlike and convex functions. Furthermore, we explicitly evaluate the subordination condition and key functional inequalities in the limiting case, confirming that they reduce to established classical results.
Example
Let us take a rational function, say:
ψ(η)=11−η,|η|<1. |
We will now demonstrate that this function satisfies the condition (2.4) in Theorem 2.10 for belonging to the class ¯Tj,kq(A,B). Substitute ψ(η)=11−η into this condition.
1η[((2+[(1−q)+(1+q)B]eiϕ)η(1−η)(1−qη)−(2+[(1−q)+(1+q)B]eiϕ)η1−ujη).11−η]≠0. |
Now simplify the expression:
=1η⋅((2+[(1−q)+(1+q)B]eiϕ)η(1−η)(1−qη)−(2+[(1−q)+(1+q)B]eiϕ)η1−ujη).11−η. |
Simplifying further:
=1(1−η)2((2+[(1−q)+(1+q)B]eiϕ)η(1−η)(1−qη)−(2+[(1−q)+(1+q)B]eiϕ)η1−ujη). |
Now, examine the term inside the parentheses:
(2+[(1−q)+(1+q)B]eiϕ)η(1−η)(1−qη)−(2+[(1−q)+(1+q)B]eiϕ)η1−ujη. |
Factor out the common terms:
(2+[(1−q)+(1+q)B]eiϕ)η[1(1−η)(1−qη)−11−ujη]. |
Now, we focus on the difference of the fractions:
1(1−η)(1−qη)−11−ujη. |
The denominator in each fraction is distinct, so the difference is generally non-zero for |η|<1. Thus, the term inside the parentheses is non-zero, and so is the overall expression. Finally, the factor 1(1−η)2 does not vanish for |η|<1, and therefore the whole expression is non-zero.
Theorem 2.6. A function ψ∈¯Tj,kq(A,B) if and only if
ψ(η)=1+∞∑v=1Avηv≠0, |
where Av=2(δvj−[v]q)+(B+1)[v]q−(A+1)δvj(B−A)xav.
Proof. A function ψ∈¯Tj,kq(A,B) if and only if
η∂qψ(η)ψj,k(η)≠2+[A(1+q)+(1−q)]x2+[B(1+q)+(1−q)]x,|η|<R,|x|=1,x≠−1, |
which implies
η∂qψ(η){2+[(1−q)+(1+q)B]x}−ψj,k(η){2+[(1−q)+(1+q)A]x}≠0, | (2.11) |
we have ∂qψ(η)=1+∑∞v=2[v]qavηv−1 and ψj,k(η)=∑∞v=1δvjavηv. Which implies
(B−A)xη+∞∑v=2[2(δvj−[v]q)+(B+1)[v]q−(A+1)δvj]avηv≠0. |
This
1+∞∑v=2[2(δvj−[v]q)+(B+1)[v]q−(A+1)δvj]avηv−1(B−A)x≠0. |
Thus, the desired result is established, completing the proof.
Remark 2.7. As q→1− and for particular values of j, k, A, and B, the following result, established by Ganesan et al. in [24] and Silverman et al. in [25], holds true:
In this paper, we have explored the properties of the function classes ¯Tj,kq(A,B) and ¯Kj,kq(A,B), which are defined using q -derivatives and (j,k) -symmetrical functions. Through our analysis, we have derived important conditions for membership in these classes, which are essential for understanding the geometric properties and behavior of the functions within them. Furthermore, we have extended classical results in complex analysis by incorporating the parameter q and considering the limit as q→1−. This approach has provided a novel perspective on the theory of symmetric functions and their applications in geometric function theory. In this study, we have explored the interplay between q -calculus and (j,k) -symmetrical functions, establishing fundamental results for the function classes ¯Tj,kq(A,B) and ¯Kj,kq(A,B). Our findings contribute to the broader understanding of geometric function theory in the context of q -calculus, offering new insights into their analytic and structural properties. Despite these advancements, several promising directions remain for future research. One potential avenue is the investigation of additional subclasses within ¯Tj,kq(A,B) and ¯Kj,kq(A,B) by incorporating more intricate conditions and generalizations. Furthermore, analyzing the influence of different values of j and k on the geometric and analytic behavior of these function classes may provide deeper theoretical insights.
Another important direction is the extension of our results to applications in quantum calculus and discrete mathematics. Exploring connections with combinatorial methods and special function theory could further enrich the applicability of (j,k) -symmetrical functions.
F. Alsarari: Conceptualization, Writing original draft, and Methodology. He was responsible for drafting the initial version of the manuscript and developing the research framework. F. Alanzi: Review and editing, Funding acquisition, and Supervision. She contributed to the critical revision of the manuscript, ensured the accuracy of the findings, and provided financial support for the research.
The authors declare that they have not used Artificial Intelligence (AI) tools in generating the research findings or mathematical results presented in this article.
The authors extend their appreciation to the Deanship of Scientific Research at Northern Border University, Arar, Kingdom of Saudi Arabia for funding this research work through the project number "NBU-FPEJ-2025-1102-02".
All authors declare no conflicts of interest in this paper.
[1] |
J. C. R. Alcantud, The semantics of N-soft sets, their applications, and a coda about three-way decision, Inform. Sciences, 606 (2022), 837–852. https://doi.org/10.1016/j.ins.2022.05.084 doi: 10.1016/j.ins.2022.05.084
![]() |
[2] |
D. G. Chen, C. Z. Wang, Q. H. Hu, A new approach to attribute reduction of consistent and inconsistent covering decision systems with covering rough sets, Inform. Sciences, 177 (2007), 3500–3518. https://doi.org/10.1016/j.ins.2007.02.041 doi: 10.1016/j.ins.2007.02.041
![]() |
[3] |
J. H. Dai, Z. Y. Wang, W. Y. Huang, Interval-valued fuzzy discernibility pair approach for attribute reduction in incomplete interval-valued information systems, Inform. Sciences, 642 (2023), 119215. https://doi.org/10.1016/j.ins.2023.119215 doi: 10.1016/j.ins.2023.119215
![]() |
[4] |
W. P. Ding, J. Nayak, B. Naik, D. Pelusi, M. Mishra, Fuzzy and real-coded chemical reaction optimization for intrusion detection in industrial big data environment, IEEE T. Ind. Inform., 17 (2021), 4298–4307. https://doi.org/10.1109/tii.2020.3007419 doi: 10.1109/tii.2020.3007419
![]() |
[5] |
H. Y. Gou, X. Y. Zhang, J. L. Yang, Z. Y. Lv, Three-way fusion measures and three-level feature selections based on neighborhood decision systems, Appl. Soft Comput., 148 (2023), 110842. https://doi.org/10.1016/j.asoc.2023.110842 doi: 10.1016/j.asoc.2023.110842
![]() |
[6] |
Q. H. Hu, D. R. Yu, J. F. Liu, C. X. Wu, Neighborhood rough set based heterogeneous feature subset selection, Inform. Sciences, 178 (2008), 3577–3594. https://doi.org/10.1016/j.ins.2008.05.024 doi: 10.1016/j.ins.2008.05.024
![]() |
[7] |
Y. Jiang, Y. Yu, Minimal attribute reduction with rough set based on compactness discernibility information tree, Soft Comput., 20 (2016), 2233–2243. https://doi.org/10.1007/s00500-015-1638-0 doi: 10.1007/s00500-015-1638-0
![]() |
[8] |
Q. Z. Kong, X. W. Zhang, W. H. Xu, S. T. Xie, Attribute reduction of multi-granulation information system, Artif. Intell. Rev., 53 (2020), 1353–1371. https://doi.org/10.1007/s10462-019-09699-3 doi: 10.1007/s10462-019-09699-3
![]() |
[9] |
M. Kryszkiewicz, Rough set approach to incomplete information systems, Inform. Sciences, 112 (1998), 39–49. https://doi.org/10.1016/S0020-0255(98)10019-1 doi: 10.1016/S0020-0255(98)10019-1
![]() |
[10] |
F. M. Ma, M. W. Ding, T. F. Zhang, J. Cao, Compressed binary discernibility matrix based incremental attribute reduction algorithm for group dynamic data, Neurocomputing, 344 (2019), 20–27. https://doi.org/10.1016/j.neucom.2018.01.094 doi: 10.1016/j.neucom.2018.01.094
![]() |
[11] |
F. M. Ma, T. F. Zhang, Generalized binary discernibility matrix for attribute reduction in incomplete information systems, J. China Univ. Posts Telecommun., 24 (2017), 57–68. https://doi.org/10.1016/s1005-8885(17)60224-3 doi: 10.1016/s1005-8885(17)60224-3
![]() |
[12] |
Z. Pawlak, Rough sets, Int. J. Comput. Inf. Sci., 11 (1982), 341–356. https://doi.org/10.1007/bf01001956 doi: 10.1007/bf01001956
![]() |
[13] | Z. Pawlak, Rough sets: Theoretical aspects of reasoning about data, Boston: Kluwer Academic Publishers, 2012. |
[14] |
Y. H. Qian, S. Y. Li, J. Y. Liang, Z. Z. Shi, F. Wang, Pessimistic rough set based decisions: A multi-granulation fusion strategy, Inform. Sciences, 264 (2014), 196–210. https://doi.org/10.1016/j.ins.2013.12.014 doi: 10.1016/j.ins.2013.12.014
![]() |
[15] |
Y. H. Qian, J. Y. Liang, C. Y. Dang, Incomplete multi-granulation rough set, IEEE T. Syst. Man Cybern., 40 (2010), 420–431. https://doi.org/10.1109/tsmca.2009.2035436 doi: 10.1109/tsmca.2009.2035436
![]() |
[16] |
Y. H. Qian, J. Y. Liang, W. Pedrycz, C. Y. Dang, Positive approximation: An accelerator for attribute reduction in rough set theory, Artif. Intell., 174 (2010), 597–618. https://doi.org/10.1016/j.artint.2010.04.018 doi: 10.1016/j.artint.2010.04.018
![]() |
[17] |
Y. H. Qian, J. Y. Liang, W. Pedrycz, C. Y. Dang, An efficient accelerator for attribute reduction from incomplete data in rough set framework, Pattern Recogn., 44 (2011), 1658–1670. https://doi.org/10.1016/j.patcog.2011.02.020 doi: 10.1016/j.patcog.2011.02.020
![]() |
[18] |
Y. H. Qian, J. Y. Liang, Y. Y. Yao, C. Y. Dang, MGRS: A multi-granulation rough set, Inform. Sciences, 180 (2010), 949–970. https://doi.org/10.1016/j.ins.2009.11.023 doi: 10.1016/j.ins.2009.11.023
![]() |
[19] | A. Skowron, Boolean reasoning for decision rules generation, In: Proceedings of the international symposium on methodologies for intelligent systems, 1993,295–305. https://doi.org/10.1007/3-540-56804-2_28 |
[20] | A. Skowron, C. Rauszer, The discernibility matrices and functions in information systems, In: R. Slowinski (ed), Intelligent decision support, Handbook of applications and advances of the rough sets theory, Kluwer Academic Publishers, Dordrecht, 1992. https://doi.org/10.1007/978-94-015-7975-9_21 |
[21] |
A. H. Tan, W. Z. Wu, J. J. Li, T. J. Li, Reduction foundation with multi-granulation rough sets using discernibility, Artif. Intell. Rev., 53 (2020), 2425–2452. https://doi.org/10.1007/s10462-019-09737-0 doi: 10.1007/s10462-019-09737-0
![]() |
[22] |
C. Z. Wang, Q. He, D. G. Chen, Q. H. Hu, A novel method for attribute reduction of covering decision systems, Inform. Sciences, 254 (2014), 181–196. https://doi.org/10.1016/j.ins.2013.08.057 doi: 10.1016/j.ins.2013.08.057
![]() |
[23] |
W. Z. Wu, Knowledge reduction in random incomplete decision tables via evidence theory, Fund. Inform., 115 (2012), 203–218. https://doi.org/10.3233/fi-2012-650 doi: 10.3233/fi-2012-650
![]() |
[24] |
W. H. Xu, D. D. Guo, J. S. Mi, Y. H. Qian, K. Y. Zheng, W. P. Ding, Two-way concept-cognitive learning via concept movement viewpoint, IEEE T. Neur. Netw. Lear. Syst., 34 (2023), 6798–6812. https://doi.org/10.1109/tnnls.2023.3235800 doi: 10.1109/tnnls.2023.3235800
![]() |
[25] |
T. Yang, Y. F. Deng, B. Yu, Y. H. Qian, J. H. Dai, Local feature selection for large-scale data sets limited labels, IEEE T. Knowl. Data En., 35 (2023), 7152–7163. https://doi.org/10.1109/tkde.2022.3181208 doi: 10.1109/tkde.2022.3181208
![]() |
[26] |
Y. Y. Yang, D. G. Chen, X. Zhang, Z. Y. Ji, Covering rough set-based incremental feature selection for mixed decision system, Soft Comput., 26 (2022), 2651–2669. https://doi.org/10.1007/s00500-021-06687-0 doi: 10.1007/s00500-021-06687-0
![]() |
[27] |
Y. Y. Yao, Constructive and algebraic methods of theory of rough sets, Inform. Sciences, 109 (1998), 21–47. https://doi.org/10.1016/S0020-0255(98)00012-7 doi: 10.1016/S0020-0255(98)00012-7
![]() |
[28] |
Y. Y. Yao, Y. H. She, Rough set models in multi-granulation spaces, Inform. Sciences, 327 (2016), 40–56. https://doi.org/10.1016/j.ins.2015.08.011 doi: 10.1016/j.ins.2015.08.011
![]() |
[29] |
Y. Y. Yao, Y. Zhao, Discernibility matrix simplification for constructing attribute reducts, Inform. Sciences, 179 (2009), 867–882. https://doi.org/10.1016/j.ins.2008.11.020 doi: 10.1016/j.ins.2008.11.020
![]() |
[30] |
E. A. K. Zaman, A. Mohamed, A. Ahmad, Feature selection for online streaming high-dimensional data: A state-of-the-art review, Appl. Soft Comput., 127 (2022), 109355. https://doi.org/10.1016/j.asoc.2022.109355 doi: 10.1016/j.asoc.2022.109355
![]() |
[31] |
C. C. Zhang, H. Liu, Z. X. Lu, J. H. Dai, Fast attribute reduction by neighbor inconsistent pair selection for dynamic decision tables, Int. J. Mach. Learn. Cyber., 15 (2024), 739–756. https://doi.org/10.1007/s13042-023-01931-5 doi: 10.1007/s13042-023-01931-5
![]() |
[32] |
C. L. Zhang, J. J. Li, Y. D. Lin, Knowledge reduction of pessimistic multi-granulation rough sets in incomplete information systems, Soft Comput., 25 (2021), 12825–12838. https://doi.org/10.1007/s00500-021-06081-w doi: 10.1007/s00500-021-06081-w
![]() |
[33] |
J. Zhang, G. Q. Zhang, Z. W. Li, L. D. Qu, C. F. Wen, Feature selection in a neighborhood decision information system with application to single cell RNA data classification, Appl. Soft Comput., 113 (2021), 107876. https://doi.org/10.1016/j.asoc.2021.107876 doi: 10.1016/j.asoc.2021.107876
![]() |
[34] |
X. Y. Zhang, W. C. Zhao, Uncertainty measures and feature selection based on composite entropy for generalized multi-granulation fuzzy neighborhood rough set, Fuzzy Set. Syst., 486 (2024), 108971. https://doi.org/10.1016/j.fss.2024.108971 doi: 10.1016/j.fss.2024.108971
![]() |
[35] |
Y. Zhao, Y. Y. Yao, F. Luo, Data analysis based on discernibility and indiscernibility, Inform. Sciences, 177 (2007), 4959–4976. https://doi.org/10.1016/j.ins.2007.06.031 doi: 10.1016/j.ins.2007.06.031
![]() |