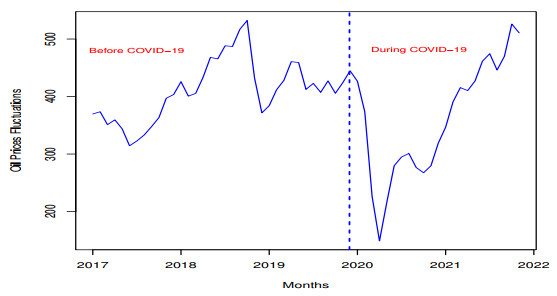
The COVID-19 epidemic has had a profound effect on almost every aspect of daily life, including the financial sector, education, transportation, health care, and so on. Among these sectors, the financial and health sectors are the most affected areas by COVID-19. Modeling and predicting the impact of the COVID-19 epidemic on the financial and health care sectors is particularly important these days. Therefore, this paper has two aims, (i) to introduce a new probability distribution for modeling the financial data set (oil prices data), and (ii) to implement a machine learning approach to predict the oil prices. First, we introduce a new approach for developing new probability distributions for the univariate analysis of the oil price data. The proposed approach is called a new reduced exponential-X (NRE-X) family. Based on this approach, two new statistical distributions are introduced for modeling the oil price data and its log returns. Based on certain statistical tools, we observe that the proposed probability distributions are the best competitors for modeling the prices' data sets. Second, we carry out a multivariate analysis while considering some covariates of oil price data. Dual well-known machine learning algorithms, namely, the least absolute shrinkage and absolute deviation (Lasso) and Elastic net (Enet) are utilized to achieve the important features for oil prices based on the best model. The best model is established through forecasting performance.
Citation: Huda M. Alshanbari, Zubair Ahmad, Faridoon Khan, Saima K. Khosa, Muhammad Ilyas, Abd Al-Aziz Hosni El-Bagoury. Univariate and multivariate analyses of the asset returns using new statistical models and penalized regression techniques[J]. AIMS Mathematics, 2023, 8(8): 19477-19503. doi: 10.3934/math.2023994
[1] | Zubair Ahmad, Zahra Almaspoor, Faridoon Khan, Sharifah E. Alhazmi, M. El-Morshedy, O. Y. Ababneh, Amer Ibrahim Al-Omari . On fitting and forecasting the log-returns of cryptocurrency exchange rates using a new logistic model and machine learning algorithms. AIMS Mathematics, 2022, 7(10): 18031-18049. doi: 10.3934/math.2022993 |
[2] | Naif Alotaibi, A. S. Al-Moisheer, Ibrahim Elbatal, Salem A. Alyami, Ahmed M. Gemeay, Ehab M. Almetwally . Bivariate step-stress accelerated life test for a new three-parameter model under progressive censored schemes with application in medical. AIMS Mathematics, 2024, 9(2): 3521-3558. doi: 10.3934/math.2024173 |
[3] | Jumanah Ahmed Darwish, Saman Hanif Shahbaz, Lutfiah Ismail Al-Turk, Muhammad Qaiser Shahbaz . Some bivariate and multivariate families of distributions: Theory, inference and application. AIMS Mathematics, 2022, 7(8): 15584-15611. doi: 10.3934/math.2022854 |
[4] | Alanazi Talal Abdulrahman, Khudhayr A. Rashedi, Tariq S. Alshammari, Eslam Hussam, Amirah Saeed Alharthi, Ramlah H Albayyat . A new extension of the Rayleigh distribution: Methodology, classical, and Bayes estimation, with application to industrial data. AIMS Mathematics, 2025, 10(2): 3710-3733. doi: 10.3934/math.2025172 |
[5] | Baishuai Zuo, Chuancun Yin . Stein’s lemma for truncated generalized skew-elliptical random vectors. AIMS Mathematics, 2020, 5(4): 3423-3433. doi: 10.3934/math.2020221 |
[6] | Aisha Fayomi, Ehab M. Almetwally, Maha E. Qura . A novel bivariate Lomax-G family of distributions: Properties, inference, and applications to environmental, medical, and computer science data. AIMS Mathematics, 2023, 8(8): 17539-17584. doi: 10.3934/math.2023896 |
[7] | Walid Emam . Benefiting from statistical modeling in the analysis of current health expenditure to gross domestic product. AIMS Mathematics, 2023, 8(5): 12398-12421. doi: 10.3934/math.2023623 |
[8] | Qian Li, Qianqian Yuan, Jianhua Chen . An efficient relaxed shift-splitting preconditioner for a class of complex symmetric indefinite linear systems. AIMS Mathematics, 2022, 7(9): 17123-17132. doi: 10.3934/math.2022942 |
[9] | Yanting Xiao, Wanying Dong . Robust estimation for varying-coefficient partially linear measurement error model with auxiliary instrumental variables. AIMS Mathematics, 2023, 8(8): 18373-18391. doi: 10.3934/math.2023934 |
[10] | Guangshuai Zhou, Chuancun Yin . Family of extended mean mixtures of multivariate normal distributions: Properties, inference and applications. AIMS Mathematics, 2022, 7(7): 12390-12414. doi: 10.3934/math.2022688 |
The COVID-19 epidemic has had a profound effect on almost every aspect of daily life, including the financial sector, education, transportation, health care, and so on. Among these sectors, the financial and health sectors are the most affected areas by COVID-19. Modeling and predicting the impact of the COVID-19 epidemic on the financial and health care sectors is particularly important these days. Therefore, this paper has two aims, (i) to introduce a new probability distribution for modeling the financial data set (oil prices data), and (ii) to implement a machine learning approach to predict the oil prices. First, we introduce a new approach for developing new probability distributions for the univariate analysis of the oil price data. The proposed approach is called a new reduced exponential-X (NRE-X) family. Based on this approach, two new statistical distributions are introduced for modeling the oil price data and its log returns. Based on certain statistical tools, we observe that the proposed probability distributions are the best competitors for modeling the prices' data sets. Second, we carry out a multivariate analysis while considering some covariates of oil price data. Dual well-known machine learning algorithms, namely, the least absolute shrinkage and absolute deviation (Lasso) and Elastic net (Enet) are utilized to achieve the important features for oil prices based on the best model. The best model is established through forecasting performance.
The worldwide COVID-19 outbreak has affected the production and lives of society, in addition to posing a serious threat to human health [1]. Governments have implemented countermeasures such as, lock-downs, to circumvent the transmission of the disease as the number of confirmed cases continues to rise. Global supply networks were disrupted as a result of these actions, and the world experienced an economic blockade [2]. It resulted in the collapse of the stock market crisis, the energy market, and a downturn in the world economy [3,4]. One of the financial markets most influenced by COVID-19 is WTI (West Texas Intermediate) crude oil futures [5]. WTI crude oil futures fall to a negative value on April 20, 2020, from $18 per barrel to -$38, experienced for the first time in history [6].
The global market economy was seriously affected by the drop in crude oil futures prices. Coal futures declined from 70.68 (on March 27) to $51.62 (on April 27), indicating that they are alternatives to each other. Since then, the market has descended into depression. The US government updated a fresh round of energy perspectives in order to stabilize the market economy and mitigate the influence of the crises. However, since June, the coal prices and WTI crude oil have risen steadily. Inflationary pressures have also sparked worries about the energy market's future growth.
From Figures 1 and 2, it can be observed that the series of oil prices and oil returns are highly volatile and noisy. The existing statistical tools are not capable to fit both series of oil efficiently, and thereby this study modifies the statistical tool in order to approximate the oil prices and their return accurately. In addition, our study adopts dual penalization techniques, namely the Least absolute shrinkage and selection operator (Lasso) and elastic net (Enet) to select the important features that drive the oil prices in the case of China.
In the financial and other closed connected sectors, statistical distributions play a vital role to provide the best description of the data under consideration. The next section offers a review of probability models that are implemented for analyzing data sets in different sectors.
Probability/statistical models play a vital role in almost every sector. The applications of statistical models can be found (i) in financial sectors [7,8], (ii) on social media platforms [9], (iii) oceanology and metrology [10], (iv) engineering-related areas [11], (v) medicine and healthcare-related sectors [12], and (vi) hydrology [13], among others.
Among these sectors, the statistical models play an important role in data modeling in different sectors related to the financial phenomena. For example, (i) Bhati and Ravi [14] introduced a log-Moyal distribution for modeling the financial and insurance data sets, (ii) Ahmad et al. [15] implemented a heavy-tailed extension of the Weibull model for analyzing the vehicle insurance loss data, (iii) Li et al. [16] proposed a generalized log-Moyal distribution for modeling the loss data, (vi) Bielak et al. [17] implemented a multidimensional and heavy-tailed-based model for market risk factors analysis. For furthermore details, we refer the interested readers to [18,19,20,21,22,23,24].
The development of new statistical distributions for modeling data in the above-mentioned sectors is a prominent research area. Therefore, numerous new statistical distributions have been introduced for providing the best description of the metrology, oceanology, engineering, hydrology, medical, and financial data sets [25]. In this paper, we also introduce a novel statistical approach for modeling data in the financial sector. The proposed approach is called, a new reduced exponential-X (NRE-X) family of distributions.
Genesis: Suppose a random variable, say T, with probability density function (PDF) f(t), where T∈[r1,r2] for −∞≤r1<r2≤∞. Let X is another random variable with CDF (cumulative distribution function) represented by K(x). Let L[K(x)] represent a function of CDF of X, satisfying the following three conditions
(i) L[K(x)]∈[r1,r2],
(ii) L[K(x)] is differentiable and monotonically increasing,
(iii) L[K(x)]→r1 as x→−∞ and L[K(x)]→r2 as x→∞.
According to the findings of [26], the CDF of the T-X distributions approach is defined by
M(x)=∫L[K(x)]r1f(t)dt,x∈R. | (2.1) |
Corresponding to M(x), the PDF m(x) of the T-X distributions is given by
m(x)={ddxL[K(x)]}k{L[K(x)]},x∈R. |
Consider the CDF F(t) of the exponential distribution given by
F(t)=1−e−ϕt,t≥0,ϕ∈R+. | (2.2) |
Let ϕ=1, then, Eq (2.2) becomes
F(t)=1−e−t,t≥0. | (2.3) |
Corresponding to F(t), the PDF f(t) is given by
f(t)=e−t,t>0. | (2.4) |
Using Eq (2.4) and L[K(x)]=λ2[1−K(x)](λ−log[1−K(x)])2 in Eq (2.1), we get the CDF of the proposed the NRE-X family, given by
M(x;λ)=1−λ2ˉK(x)(λ−log[ˉK(x)])2,x∈R,λ∈R+, | (2.5) |
where, ˉK(x)=1−K(x) is the SF (survival function) of the baseline random variable.
Based on our search of the existing literature, the NRE-X approach defined in Eq (2.5) has not been used/proposed so far. This is one of the key motivations of the current work. Therefore, using the NRE-X approach numerous new models can also be introduced for data modeling in different sectors. Corresponding to M(x;λ), the PDF m(x;λ) is given by
m(x;λ)=λ2k(x)(λ−log[ˉK(x)])3(2+λ−log[ˉK(x)]),x∈R, | (2.6) |
where ddxK(x)=k(x).
In link to Eqs (2.5) and (2.6), the SF ˉM(x;λ)=1−M(x;λ) and hazard function (HF) h(x;λ)=m(x;λ)ˉM(x;λ), are given, respectively, by
ˉM(x;λ)=λ2ˉK(x)(λ−log[ˉK(x)])2,x∈R, |
and
h(x;λ)=k(x)ˉK(x)(λ−log[ˉK(x)])(2+λ−log[ˉK(x)]),x∈R. |
In this paper, we implement the proposed NRE-X distributions and introduce updated versions of the logistic and Fréchet distributions. The updated version of the logistic distribution is called, a new reduced exponential-logistic (NRE-logistic) model. Whereas, the updated version of the Fréchet model is called, a new reduced exponential-Fréchet (NRE-Fréchet) model. In the next section, we provide expressions for the CDF, PDF, SF, and HF of the NRE-logistic and NRE-Fréchet distributions. Furthermore, different PDF behaviors of the NRE-logistic and NRE-Fréchet distributions are also presented.
This section is devoted to introducing two special models of the NRE-X method by taking the logistic and Fréchet distributions as baseline models.
Consider the CDF K(x;θ,σ) of the two parameters (θ∈R,σ∈R+) logistic distribution is given by
K(x;θ,σ)=(1+e−(x−θσ))−1x∈R. | (3.1) |
For θ∈R and σ∈R+, the PDF, SF and HF of the logistic distribution are given by
k(x;θ,σ)=1σe−(x−θσ)(1+e−(x−θσ))−2,x∈R, |
ˉK(x;θ,σ)=1−(1+e−(x−θσ))−1,x∈R, |
and
h(x;θ,σ)=e−(x−θσ)(1+e−(x−θσ))−21−(1+e−(x−θσ))−1,x∈R, |
respectively.
Using Eq (3.1) in Eq (2.5), we get the CDF of the NRE-logistic distribution, given by
M(x;λ,θ,σ)=1−λ2(1−(1+e−(x−θσ))−1)(λ−log[1−(1+e−(x−θσ))−1])2,x∈R, | (3.2) |
with PDF
m(x;λ,θ,σ)=λ2σe−(x−θσ)(1+e−(x−θσ))−2(λ−log[1−(1+e−(x−θσ))−1])3(2+λ−log[1−(1+e−(x−θσ))−1]),x∈R. | (3.3) |
Some possible plots for the PDF of the NRE-logistic distribution are presented in Figure 3. In the plots in Figure 3, the additional parameter λ has a positive impact on the shape of the PDF of the NRE-logistic distribution. These plots reveal that as the value of λ increases, the NRE-logistic distribution possesses the heavy-tailed characteristics which are very useful in the financial and other related sectors.
Furthermore, the SF and HF of the NRE-logistic distribution are, respectively, given by
ˉM(x;λ,θ,σ)=λ2(1−(1+e−(x−θσ))−1)(λ−log[1−(1+e−(x−θσ))−1])2, |
and
h(x;λ,θ,σ)=1σe−(x−θσ)(1+e−(x−θσ))−2(2+λ−log[1−(1+e−(x−θσ))−1])(λ−log[1−(1+e−(x−θσ))−1])(1−(1+e−(x−θσ))−1). |
Let K(x;η,φ) be the CDF of the two parameters (η∈R+,φ∈R+) Fréchet distribution, given by
K(x;η,φ)=e−φx−η,x∈R+. | (3.4) |
For η∈R+ and φ∈R+, the PDF, SF, and HF of the Fréchet distribution are given by
k(x;η,φ)=ηφx−η−1e−φx−η,x∈R+, |
ˉK(x;η,φ)=1−e−φx−η,x∈R+, |
and
h(x;η,φ)=ηφx−η−1e−φx−η1−e−φx−η,x∈R+, |
respectively.
Using Eq (3.4) in Eq (2.5), we get the CDF of the NRE-Fréchet distribution, given by
M(x;λ,η,φ)=1−λ2(1−e−φx−η)[λ−log(1−e−φx−η)]2,x∈R+,λ∈R+, | (3.5) |
with PDF
m(x;λ,η,φ)=λ2ηφx−η−1e−φx−η[λ−log(1−e−φx−η)]3[2+λ−log(1−e−φx−η)],x∈R+. | (3.6) |
Some possible behaviors for the PDF of the NRE-Fréchet distribution are presented in Figure 4. The plots in Figure 4, show that the additional parameter λ, has a significant impact on the PDF shape of the NRE-Fréchet distribution. In a nutt shell, we can see that as the value of λ increases, the NRE-Fréchet distribution tends to be a heavy-tailed distribution.
Furthermore, the SF and HF of the NRE-Fréchet distribution are given by
ˉM(x;λ,η,φ)=λ2(1−e−φx−η)[λ−log(1−e−φx−η)]2,x∈R,λ∈R+, |
and
h(x;λ,η,φ)=ηφx−η−1e−φx−η[2+λ−log(1−e−φx−η)][λ−log(1−e−φx−η)](1−e−φx−η),x∈R+, |
respectively.
In this section, we use the maximum likelihood estimation method to obtain the maximum likelihood estimators (MLEs) for the parameters of the NRE-logistic and NRE-Fréchet distributions. Furthermore, simulation studies are also provided to assess the performances of the MLEs of the NRE-logistic and NRE-Fréchet distributions.
Assume a set of random samples, say X1,X2,...,Xn, taken from the NRE-logistic distribution with PDF m(x;λ,θ,σ) in Eq (3.3). Then, corresponding to m(x;λ,θ,σ), the log-likelihood function (LLF), say ℓ(λ,θ,σ), is given by
ℓ(λ,θ,σ)=2nlogλ−nlogσ−n∑i=1(xi−θσ)−2n∑i=1log(1+e−(xi−θσ))+n∑i=1log(2+λ−log[1−(1+e−(xi−θσ))−1])+3n∑i=1log(λ−log[1−(1+e−(xi−θσ))−1]). |
Corresponding to ℓ(λ,θ,σ), the partial derivatives with respect to θ,σ, and λ are given, respectively, by
∂∂θℓ(λ,θ,σ)=+nσ−2nσn∑i=1e−(xi−θσ)(1+e−(xi−θσ))−n∑i=1nσe−(xi−θσ)(1+e−(xi−θσ))−2[1−(1+e−(xi−θσ))−1](2+λ−log[1−(1+e−(xi−θσ))−1])−3nσn∑i=1e−(xi−θσ)(1+e−(xi−θσ))−2[1−(1+e−(xi−θσ))−1](λ−log[1−(1+e−(xi−θσ))−1]), |
∂∂σℓ(λ,θ,σ)=−nσ+n∑i=1xiσ2−2n∑i=1xiσ2e−(xi−θσ)(1+e−(xi−θσ))−n∑i=1xiσ2e−(xi−θσ)(1+e−(xi−θσ))−2[1−(1+e−(xi−θσ))−1](2+λ−log[1−(1+e−(xi−θσ))−1])−3n∑i=1xiσ2e−(xi−θσ)(1+e−(xi−θσ))−2[1−(1+e−(xi−θσ))−1](λ−log[1−(1+e−(xi−θσ))−1]) |
and
∂∂λℓ(λ,θ,σ)=2nλ+n∑i=11(2+λ−log[1−(1+e−(xi−θσ))−1])+3n∑i=11(λ−log[1−(1+e−(xi−θσ))−1]). |
On solving ∂∂θℓ(λ,θ,σ)=0, ∂∂σℓ(λ,θ,σ)=0, and ∂∂λℓ(λ,θ,σ)=0, we get the MLEs (ˆθMLE,ˆσMLE,ˆλMLE) of the parameters (θ,σ,λ) of the of the NRE-logistic distribution.
Next, we provide a Monte Carlo simulation study (MCSS) to evaluate the performances of ˆθMLE,ˆσMLE, and ˆλMLE. For the NRE-logistic distribution, the MCSS is conducted by generating the random numbers such as n=25,50,...,500 using the inverse CDF method. The MCSS s conducted for two different combinations of θ,σ, and λ. These combination values are given by (i) θ=1.5,σ=0.9,λ=0.5 and (ii) θ=1.3,σ=0.7,λ=1.1.
The performances of ˆθMLE,ˆσMLE, and ˆλMLE are evaluated using two decisive tools. The numerical values of the selected decisive tools are computed as
● Bias
Bias(ˆθMLE)=n∑i=1(ˆθi−θ). |
● Mean square error (MSE)
MSE(ˆθMLE)=n∑i=1(ˆθi−θ)2. |
The MLEs and the above evaluation criteria are computed using the optim()R with argument method = "L-BFGS-B". After carrying out the MCSS, the numerical results are provided in Tables 1 and 2. Whereas, the results of the MCSS are illustrated visually in Figures 5 and 6.
n | Parameters | MLEs | MSEs | Biases |
θ | 1.5306540 | 0.0845499 | 0.03065429 | |
25 | σ | 1.6616360 | 1.6801157 | 0.76163600 |
λ | 2.1413169 | 6.5317397 | 1.64131690 | |
θ | 1.4878190 | 0.0405659 | -0.01218063 | |
50 | σ | 1.3621325 | 0.8178465 | 0.46213251 |
λ | 1.7129257 | 4.8094673 | 1.21292570 | |
θ | 1.4833020 | 0.0266625 | -0.01669770 | |
75 | σ | 1.2395189 | 0.5670010 | 0.33951893 |
λ | 1.3942232 | 3.2730729 | 0.89422320 | |
θ | 1.4907440 | 0.0200039 | -0.00925587 | |
100 | σ | 1.1644847 | 0.4874031 | 0.26448468 |
λ | 1.2066578 | 2.6246481 | 0.70665780 | |
θ | 1.4939300 | 0.0128720 | -0.00606980 | |
200 | σ | 1.0687798 | 0.3153811 | 0.16877977 |
λ | 0.9406648 | 1.4242122 | 0.44066480 | |
θ | 1.4850080 | 0.0079217 | -0.01499184 | |
300 | σ | 1.0421690 | 0.2105968 | 0.14216899 |
λ | 0.7456199 | 0.7993679 | 0.30561990 | |
θ | 1.4895150 | 0.0067249 | -0.01048548 | |
400 | σ | 1.0060013 | 0.1530510 | 0.10600131 |
λ | 0.5966013 | 0.3541581 | 0.19660130 | |
θ | 1.4963270 | 0.0048694 | -0.00367308 | |
500 | σ | 0.9351764 | 0.1209646 | 0.07517640 |
λ | 0.5406189 | 0.2645338 | 0.14061890 |
n | Parameters | MLEs | MSEs | Biases |
θ | 1.3409810 | 0.06089320 | 4.0981e-02 | |
25 | σ | 0.9684732 | 0.32269754 | 0.26847323 |
λ | 2.4804880 | 6.35740400 | 1.58048760 | |
θ | 1.3207820 | 0.03098274 | 2.0781e-02 | |
50 | σ | 0.8257357 | 0.18517215 | 0.12573567 |
λ | 2.0018830 | 4.78013720 | 1.10188330 | |
θ | 1.3131030 | 0.02263240 | 1.3103e-02 | |
75 | σ | 0.8056960 | 0.16232288 | 0.10569596 |
λ | 1.8454520 | 3.95911760 | 0.94545150 | |
θ | 1.2969670 | 0.01678957 | -3.0325e-03 | |
100 | σ | 0.8074029 | 0.13781325 | 0.10740290 |
λ | 1.8371310 | 3.76135120 | 0.93713060 | |
θ | 1.3000190 | 0.01000074 | 1.9164e-05 | |
200 | σ | 0.7502970 | 0.09277251 | 0.05029699 |
λ | 1.4371740 | 2.04954990 | 0.53717410 | |
θ | 1.2876490 | 0.00735997 | -1.2350e-02 | |
300 | σ | 0.7879704 | 0.07972821 | 0.08797043 |
λ | 1.4682940 | 1.76350150 | 0.56829450 | |
θ | 1.2992960 | 0.00527875 | -7.0367e-04 | |
400 | σ | 0.7273118 | 0.05311106 | 0.02731182 |
λ | 1.1906500 | 0.97227000 | 0.29065020 | |
θ | 1.3000400 | 0.00404874 | 3.9596e-05 | |
500 | σ | 0.7113346 | 0.04542952 | 0.01133456 |
λ | 1.1060500 | 0.72525460 | 0.20605010 |
The results of the MCSS of the NRE-logistic distribution presented in Tables 1 and 2, Figures 5 and 6, reveal that as the size of the sample n increases
● The MLEs ˆθMLE,ˆσMLE, and ˆλMLE tend to stable.
● The MSEs of ˆθMLE,ˆσMLE, and ˆλMLE decreases.
● The biases of ˆθMLE,ˆσMLE, and ˆλMLE decay to zero.
Consider a random sample, say X1,X2,...,Xn, observed from the NRE-Fréchet distribution with PDF m(x;λ,η,φ) expressed by Eq (3.6). Then, corresponding to m(x;λ,η,φ), the LLF, say ℓ(λ,η,φ), is given by
m(x;λ,η,φ)=λ2ηφx−η−1e−φx−η[λ−log(1−e−φx−η)]3[2+λ−log(1−e−φx−η)], |
ℓ(λ,η,φ)=2nlogλ+nlogη+nlogφ−(η+1)n∑i=1logxi−n∑i=1φx−ηi+n∑i=1log[2+λ−log(1−e−φx−ηi)]−3n∑i=1log[λ−log(1−e−φx−ηi)]. |
Corresponding to ℓ(λ,η,φ), the partial derivatives with respect to η,φ, and λ are given, respectively, by
∂∂ηℓ(λ,η,φ)=nη−n∑i=1logxi+n∑i=1(logxi)φx−ηi+n∑i=1(logxi)φx−ηie−φx−ηi(1−e−φx−ηi)[2+λ−log(1−e−φx−ηi)]−3n∑i=1(logxi)φx−ηie−φx−ηi(1−e−φx−ηi)[λ−log(1−e−φx−ηi)], |
∂∂φℓ(λ,η,φ)=nφ−n∑i=1x−ηi−n∑i=1x−ηie−φx−ηi(1−e−φx−ηi)[2+λ−log(1−e−φx−ηi)]+3n∑i=1x−ηie−φx−ηi(1−e−φx−ηi)[λ−log(1−e−φx−ηi)], |
and
∂∂λℓ(λ,η,φ)=2nλ+n∑i=11(1−e−φx−ηi)[2+λ−log(1−e−φx−ηi)]−3n∑i=11(1−e−φx−ηi)[λ−log(1−e−φx−ηi)]. |
Equating the expressions ∂∂ηℓ(λ,η,φ), ∂∂φℓ(λ,η,φ), and ∂∂λℓ(λ,η,φ) to zero and solving we get the MLEs (ˆηMLE,ˆφMLE,ˆλMLE) of the parameters (η,φ,λ) of the of the NRE-Fréchet distribution.
After obtaining the MLEs ˆηMLE,ˆφMLE, and ˆλMLE, now we check their performances through a Monte Carlo simulation study (MCSS). The evaluation of ˆηMLE,ˆφMLE, and ˆλMLE is carried out by generating random numbers from the NRE-Fréchet distribution by implementing the NRE-Fréchet distribution. For the NRE-Fréchet distribution, the MCSS is conducted for two sets of parameters, such as (i) θ=1.5,σ=0.9,λ=0.5 and (ii) θ=0.9,σ=1.1,λ=1.5.
Again, we use the Bias and MSE as decisive tools to judge the performances of ˆηMLE,ˆφMLE, and ˆλMLE. After conducting the MCSS, the numerical results for the MLEs, MSEs, and biases of the NRE-Fréchet distribution are presented in Tables 3 and 4. Whereas, the results of the MCSS are illustrated visually in Figures 7 and 8.
n | Parameters | MLEs | MSEs | Biases |
η | 0.8295898 | 0.08826282 | 0.12958978 | |
25 | ϕ | 1.6435660 | 0.20131711 | 0.04356598 |
λ | 1.8830760 | 1.75815404 | 0.58307614 | |
η | 0.7636202 | 0.03381550 | 0.06362024 | |
500 | ϕ | 1.6144310 | 0.11071804 | 0.01443136 |
λ | 1.5688950 | 0.60710519 | 0.26889488 | |
η | 0.7413941 | 0.02058671 | 0.04139414 | |
75 | ϕ | 1.6032170 | 0.07300298 | 0.00321683 |
λ | 1.4934430 | 0.38750257 | 0.19344311 | |
η | 0.7308403 | 0.01473447 | 0.03084032 | |
100 | ϕ | 1.6124770 | 0.05724885 | 0.01247658 |
λ | 1.4101360 | 0.15857094 | 0.11013555 | |
η | 0.7159275 | 0.00628118 | 0.01592747 | |
200 | ϕ | 1.5983640 | 0.02611875 | -0.00163616 |
λ | 1.3440490 | 0.02956159 | 0.04404876 | |
η | 0.7083867 | 0.00356276 | 0.00838667 | |
300 | ϕ | 1.6127650 | 0.01877270 | 0.01276520 |
λ | 1.3214120 | 0.01317318 | 0.02141173 | |
η | 0.7037929 | 0.00282884 | 0.00379289 | |
400 | ϕ | 1.6012280 | 0.01354377 | 0.00122802 |
λ | 1.3194250 | 0.01055359 | 0.01942524 | |
η | 0.7062376 | 0.00234377 | 0.00623763 | |
500 | ϕ | 1.5949220 | 0.01018247 | -0.00507846 |
λ | 1.3100780 | 0.00762077 | 0.01907786 |
n | Parameters | MLEs | MSEs | Biases |
η | 1.0040981 | 0.083711686 | 0.10409810 | |
25 | ϕ | 1.2140710 | 0.098602278 | 0.11407104 |
λ | 1.8282900 | 0.975918910 | 0.3282901 | |
η | 0.9628862 | 0.037615081 | 0.06288619 | |
500 | ϕ | 1.1532450 | 0.036819558 | 0.05324535 |
λ | 1.6785700 | 0.420855040 | 0.17856980 | |
η | 0.9416555 | 0.026231108 | 0.04165548 | |
75 | ϕ | 1.1467750 | 0.027392599 | 0.04677475 |
λ | 1.5794770 | 0.139978210 | 0.07947676 | |
η | 0.9243457 | 0.015663488 | 0.02434573 | |
100 | ϕ | 1.1333150 | 0.021317467 | 0.03331549 |
λ | 1.5717710 | 0.105736930 | 0.07177117 | |
η | 0.9111239 | 0.007281454 | 0.01112393 | |
200 | ϕ | 1.1173830 | 0.012048905 | 0.01738310 |
λ | 1.5347830 | 0.045606720 | 0.03478277 | |
η | 0.9082706 | 0.005246932 | 0.00827062 | |
300 | ϕ | 1.1059050 | 0.007748683 | 0.00590497 |
λ | 1.5355600 | 0.032318420 | 0.03556033 | |
η | 0.9051241 | 0.003407605 | 0.00512410 | |
400 | ϕ | 1.1116230 | 0.006835205 | 0.01162262 |
λ | 1.5194740 | 0.024970670 | 0.01947445 | |
η | 0.9068855 | 0.003215347 | 0.00688549 | |
500 | ϕ | 1.1049340 | 0.005313549 | 0.00493448 |
λ | 1.5219570 | 0.020792440 | 0.02195670 |
From the results of the MCSS of the NRE-Fréchet distribution provided in Tables 3 and 4, Figures 7 and 8, we can see that the MLEs of the NRE-Fréchet distribution tend to sable as the size of the sample increases. With the increase in the sample size, the MSEs of the ˆηMLE,ˆφMLE, and ˆλMLE decreases and biases of ˆηMLE,ˆφMLE, and ˆλMLE decay to zero.
In this this section, we implement the NRE-logistic and NRE-Fréchet distributions for analyzing a data set taken from the healthcare economic sector. The data set consists of fifty-nine (59) observations and is taken from an oil company in China during the COVID-19 pandemic. First, we analyze the price data taken from the oil company of China. Then, we obtain the price returns of the same oil company in China during the COVID-19 epidemic.
The comparison of the NRE-logistic is made with the (i) logistic (a two-parameter model; see Eq (7)), (ii) exponentiated logistic (Exp-logistic) which is a three-parameter competing distribution, and (iii) Kumaraswamy logistic (Kum-logistic) which is a four-parameter competing distribution. The CDFs of the Exp-logistic and Kum-logistic distributions are given by
K(x;α,θ,σ)=((1+e−(x−θσ))−1)α,x∈R,α∈R+, |
and
K(x;α,β,θ,σ)=1−(1−[(1+e−(x−θσ))−1]α)β,x∈R,α∈R+,β∈R+, |
respectively.
Whereas, the results of the NREi-Fréchet distribution are compared with (i) the Fréchet distribution (a two-parameter model; see Eq (10)) and alpha power transformed Fréchet (APT-Fréchet) which is a three-parameter competing distribution. The CDF of the APT-Fréchet distribution is given by
K(x;α1,η,φ)=αe−φx−η1−1α1−1,x∈R,α1∈R+,α1≠1. |
To figure out analytically/numerically which competing model provides the close fit to the economic data collected during the COVID-19 pandemic, three goodness-of-fit tests such as (i) Anderson-Darling (AD) test, (ii) Cramér-von Mises (CVM) test, and (iii) Kolmogorov-Smirnov (KS) test, are considered. Besides these tests, the p-value of the competing models has also been calculated. The numerical values of the goodness-of-fit tests and MLEs of the fitted distributions are obtained using the R-script Adequacy Model with BFGS algorithm.
This section is devoted to implementing the NRE-Fréchet distribution for modeling the oil prices data, given by: 369.66,373.55,351.07,359.46,343.74,314.33,322.73,333.15,347.80,363.43,397.06,403.46,425.79, 400.65,405.52,433.35,468.06,465.40,488.27,487.03,516.71,532.23,432.55,371.54,384.04,411.75, 428.13,460.63,459.14,412.43,422.83,407.27,427.24,405.65, 423.91,444.75,426.54,373.43,225.98,148.81,216.04,279.61, 294.83,301.01,276.45,267.42,279.53,318.90,346.71, 390.55,415.50,410.38,427.01,461.28, 474.47,446.01,470.01,526.12,510.69.
For easily carrying out the numerical computation, we transformed the oil prices data using X=Y10, where Y represents the data set. Some summary measures (SMs) of the transformed data are given by: is is given by minimum = 1.488, 1st Quartile (Q1) = 3.473, Median (Q2) = 4.056, Mean = 3.912, 3rd Quartile (Q1) = 4.391, maximum = 5.322, skewness = -0.6735137, kurtosis = 3.442013, variance = 0.6276279, and range = 3.8342. In line with the oil price data, some key plots are obtained in Figure 9.
Corresponding to the oil prices data, the values of the maximum likelihood estimators (MLE) (ˆλMLE,ˆηMLE,ˆφMLE,^α1MLE) of the fitted models are reported in Table 5. For the NRE-Fréchet distribution, the profiles of the log-likelihood function of the MLES are displayed visually in Figure 10.
Model | ˆλMLE | ˆηMLE | ˆφMLE | ^α1MLE |
NRE-Fréchet | 0.00317 | 0.97677 | 25.00396 | - |
Fréchet | - | 3.14566 | 44.87837 | - |
APT-Fréchet | - | 4.20771 | 41.89421 | 139.89786 |
Furthermore, the values of AD, CVM, KS and p-value are obtained in Table 6. From the given results in Table 6, we can see that (i) for the NRE-Fréchet model, the values of the selection criteria are CVM = 0.27746, AD = 1.59522, KS = 0.11565, p-value = 0.38000, (ii) for the Fréchet model, the values of the selection criteria are CVM = 0.70603, AD = 4.02970, KS = 0.20811, p-value = 0.60170, and (iii) for the APT-Fréchet model, the values of the selection criteria are CVM = 0.50369, AD = 2.873401, KS = 0.16504, p-value = 0.07141. From the above discussion, it is obvious that the NRE-Fréchet distribution provides a close fit to the oil prices data.
Model | CVM | AD | KS | p-value |
NRE-Fréchet | 0.27746 | 1.59522 | 0.11565 | 0.38000 |
Fréchet | 0.70603 | 4.02970 | 0.20811 | 0.06170 |
APT-Fréchet | 0.50369 | 2.873401 | 0.16504 | 0.07141 |
Besides the numerical illustration, visual proof of the best fitting of the NRE-Fréchet distribution is also provided. For the visual illustration, the plots of fitted PDF, CDF, SF, QQ (quantile-quantile), and PP (probability-probability) are considered; see Figure 11. Based on the visual illustration provided in Figure 11, it is clear that the NRE-Fréchet distribution provides a close fit to the oil prices data.
This subsection is devoted to apply the NRE-logistic distribution for analyzing the prices returns of an oil company. The prices returns (log-returns) of the oil company, rt, is obtained as rt=log(Pt)−log(Pt−1), where Pt represents the oil price at time t. The SMs of this is given by minimum = -0.502284, 1st Quartile (Q1) = -0.036423, Median (Q2) = 0.028893, Mean = 0.005572, 3rd Quartile (Q3) = 0.053652, maximum = 0.372793, skewness = -1.396718, kurtosis = 9.199987, variance = 0.01558175, and range = 0.8750773. Corresponding to the log-returns of the oil prices data, some basic plots are presented in Figure 12.
In the link to the prices returns data, the values ˆθMLE,ˆσMLE,ˆλMLE, and ˆαMLE of the logistic, Exp-logisitc, and NRE-logistic distributions are presented in Table 7. For the NRE-logistic distribution, the profiles of the log-likelihood function of the MLEs based on the prices returns data are displayed visually in Figure 13.
Model | ˆλMLE | ˆθMLE | ˆσMLE | ˆαMLE | ˆβMLE |
NRE-logistic | 1.11894 | 0.08833 | 0.06210 | - | - |
Logistic | - | 0.01619 | 0.05521 | - | - |
Exp-logistic | - | 0.05203 | 0.03959 | 0.55644 | - |
Kum-logistic | - | 0.09952 | 0.07700 | 1.06195 | 2.81753 |
Furthermore, the values of AD, CVM, KS and p-value are reported in Table 8. From the obtained results in Table 8, we can see that (i) for the NRE-logistict model, the values of the selection criteria are CVM = 0.28420, AD = 1.68629, KS = 0.11902, p-value = 0.35560, (ii) for the Logistic model, the values of the selection criteria are CVM = 0.33210, AD = 1.91355, KS = 0.12817, p-value = 0.27250, (iii) for the Exp-logistic model, the values of the selection criteria are CVM = 0.30118, AD = 1.84708, KS = 0.12076, p-value = 0.31480, and (iv) for the Kum-logistic model, the values of the selection criteria are CVM = 0.32518, AD = 1.96717, KS = 0.13960, p-value = 0.18960. From the above discussion, it is obvious that the NRE-Fréchet distribution provides a close fit to the oil prices data. Based on the obtained results in Table 8, it is clear that the NRE-logistic distribution provides a close fit to the prices returns data of an oil company.
Model | CVM | AD | KS | p-value |
NRE-logistic | 0.28244 | 1.68355 | 0.12088 | 0.33750 |
Logistic | 0.33210 | 1.91355 | 0.12817 | 0.27250 |
Exp-logistic | 0.30118 | 1.84708 | 0.12096 | 0.31480 |
Kum-logistic | 0.32518 | 1.96717 | 0.13960 | 0.18960 |
After the proof of the best fitting capability of the NRE-logistic distribution, a visual illustration of the performances of the NREi-logistic model is also provided. For this purpose, the plots of the fitted PDF, CDF, PP, QQ, and SF are again considered; see Figure 14. The plots, in Figure 14, show that the NRE-logistic distribution provides the best fit for the price returns data.
In the classical linear regression model, it is assumed that there is no severe correlation among the set of explanatory variables, and such an ideal situation usually does not exist in practice. The violation of such an assumption induces a problem that refers to multicollinearity. In the presence of severe multicollinearity, the estimates computed from the ordinary least squares (OLS) approach are not reliable. In other words, the estimates of the unknown parameters have a large standard error with the false signs, which adversely affect estimation as well as forecasting. In such circumstances, the most popular way of estimating the unknown parameters is the penalization family of tools.
Generally, penalized regression methods are modified versions of ordinary least squares regression methods (OLS) and are undeniably deemed an essential part of Machine Learning (ML) techniques. It has already been demonstrated in the literature that ML methods are effective for analyzing large datasets [27].
This study adopts the dual most well-known tools of penalized regression, including the least absolute shrinkage and selection operator (Lasso) and elastic net (Enet) for variable selection [28]. Penalized the least square regression performs variable selection and coefficients estimation by minimizing
12∥ϑ−zθ∥22+τ[ρm∑k=1|θk|+(1−ρ)m∑k=1|θk|2], |
where ϑ=(ϑ1,ϑ2,...,ϑn), z=(z1,z2,...,zn), and θ is the coefficient matrix with θ=(θ1,θ2,...,θn). Here, m and n represent the number of explanatory variables and data points, respectively. The second term indicates a penalty function, which plays a crucial role in variable selection. The penalty function puts some coefficients exactly equal to zero in order to reduce the dimensionality. The component τ is so-called the hyperparameter that controls the amount of shrinkage, and its range lies between zero and infinity [29,30]. The different values of parameter ρ are responsible for providing different models, given as follows:
● Ridge regression if ρ=0.
● Lasso regression if ρ=1.
● Elastic net if 0<ρ<1.
The selection of a hyperparameter, also known as a tuning parameter is very crucial and therefore several approaches have been followed in the literature while selecting its optimum value for the model [31]. One of them is cross-validation (CV), that commonly used for obtaining the optimum model. Our study uses the CV approach for the selection of tuning parameters.
Data analysis
After performing univariate analysis, now we carry out multivariate analysis to investigate the impact of macroeconomic variables on oil prices (OP) in the case of China for the period January 2017 to November 2021. The macroeconomic variables include the gross domestic product (GDP), the real effective exchange rate (REER), consumer price index (CPI) and money supply (MS). Although the data is time series, it is more probable to face the problem of autocorrelation, because autocorrelation is a pure phenomenon of time series data. Therefore, we proceed with our analysis by incorporating the common factors in the models. This study takes into account five lags for each variable (response and explanatory variables), which in turn induce a total of 29 variables. Before modeling, it is more appropriate to determine the correlation pattern among the set of 29 variables. For that purpose, we use the graphical approach.
In Figure 15, red and blue colors manifest the negative and positive association, respectively. The circle area and severity are positively related to the correlation coefficients. There are numerous dark colors in Figure 15, which ensures the problem of multicollinearity in the data.
We observed that the length of explanatory variables is large, and they are highly correlated to each other, and thereby it is plausible to apply the traditional models like vector autoregression, etc., to the underlying dataset [32]. Therefore, this study adopts dual advanced machine learning techniques, namely Lasso and Enet, to resolve these problems and obtain reliable results.
The feature selection plots are presented in Figures 16 and 17. The Lasso holds 22 features out of 29 and the rival approach Enet selects 18 features. The RMSE, MAE and R-squared values are given in Table 9. The Lasso produces the RMSE, MAE and R-squared values of 44.77, 33.84 and 0.75. In contrast, the Enet produces the RMSE, MAE and R-squared values of 41.71, 31.30 and 0.78, respectively. We can observe that the error metrics associated with Enet are smaller than the error metrics attached with Lasso, and similarly the value of Enet R-squared exceeds the Lasso R-squared value. These results clearly reveal that Enet fitted the data more accurately as compared to the competitor counterpart. Figures 18 and 19 are based on the findings given in Table 9. It can be concluded that Lasso holds more irrelevant features and thereby over-specify the model, which in turn adversely affects the performance of Lasso.
Method | RMSE | MAE | R-squared |
Lasso | 44.77 | 33.84 | 0.75 |
Enet | 41.71 | 31.30 | 0.78 |
The COVID-19 pandemic has highly damaged the healthcare and business sectors. To have enough knowledge and description of the events related to the COVID-19 epidemic, numerous research studies have been performed. Most of these research studies have been carried out by introducing new statistical methodologies. This paper further contributed to the development of the statistical methodologies by introducing a novel statistical approach for modeling the oil price data. The proposed method was named the NRE-X approach. Based on the NRE-X approach, two new statistical models were introduced. The first model was named the NRE-Fréchet distribution. The proposed NRE-Fréchet distribution was applied for analyzing the oil price data, whereas, the second model was named the NRE-logistic distribution. The suggested NRE-logistic distribution was applied for modeling the log-returns of the oil price data. By considering three different analytical measures (with p-value) as decisive tools, it is observed that the proposed probability distributions were the best competitors for the oil price and log-returns of the oil price data set. Complementing the univariate analysis, we performed the multivariate analysis to examine the influence of macroeconomic variables on oil price in the case of China. For this purpose, our study implemented dual penalization techniques, including Lasso and Enet. The empirical analysis reveals, that Lasso keeps 22 covariates out of 29 and Enet holds 18 covariates. On the basis of three popular statistical accuracy measures, namely, the RMSE, MAE, and R-squared, Enet and Lasso were compared, and it can be inferred that the forecasting ability of Enet is superior to Lasso. It clearly demonstrates that Lasso over-specified the model. In other words, Lasso holds more irrelevant variables in contrast to Enet, which adversely affects its forecasting performance.
In the future, we are motivated to introduce the bivariate and multivariate modifications of the NRE-logistic and NRE-Fréchet distributions for bivariate and multivariate analyses.
The authors declare they have not used Artificial Intelligence (AI) tools in the creation of this article.
Princess Nourah bint Abdulrahman University Researchers Supporting Project number (PNURSP2023R 299), Princess Nourah bint Abdulrahman University, Riyadh, Saudi Arabia.
The authors declare no conflict of interest.
[1] |
B. B. Ahundjanov, S. B. Akhundjanov, B. B. Okhunjanov, Risk perception and oil and gasoline markets under COVID-19, J. Econ. Bus., 115 (2021), 105979. https://doi.org/10.1016/j.jeconbus.2020.105979 doi: 10.1016/j.jeconbus.2020.105979
![]() |
[2] |
C. T. Vidya, K. P. Prabheesh, Implications of COVID-19 pandemic on the global trade networks, Emerg. Mark. Financ. Tr., 56 (2020), 2408–2421. https://doi.org/10.1080/1540496X.2020.1785426 doi: 10.1080/1540496X.2020.1785426
![]() |
[3] |
M. Ali, N. Alam, S. A. R. Rizvi, Coronavirus (COVID-19). An epidemic or pandemic for financial markets, J. Behav. Expe. Financ., 27 (2020), 100341. https://doi.org/10.1016/j.jbef.2020.100341 doi: 10.1016/j.jbef.2020.100341
![]() |
[4] |
W. Zhao, S. K. Khosa, Z. Ahmad, M. Aslam, A. Z. Afify, Type-Ⅰ heavy tailed family with applications in medicine, engineering and insurance, Plos One, 15 (2020), e0237462. https://doi.org/10.1371/journal.pone.0237462 doi: 10.1371/journal.pone.0237462
![]() |
[5] |
R. Gerlagh, R. J. Heijmans, K. E. Rosendahl, COVID-19 tests the market stability reserve, Environ. Resour. Econ., 76 (2020), 855–865. https://doi.org/10.1007/s10640-020-00441-0 doi: 10.1007/s10640-020-00441-0
![]() |
[6] |
C. Gharib, S. Mefteh-Wali, S. B. Jabeur, The bubble contagion effect of COVID-19 outbreak: Evidence from crude oil and gold markets, Financ. Res. Lett., 38 (2021), 101703. https://doi.org/10.1016/j.frl.2020.101703 doi: 10.1016/j.frl.2020.101703
![]() |
[7] |
Z. Ahmad, E. Mahmoudi, S. Dey, S. K. Khosa, Modeling vehicle insurance loss data using a new member of TX family of distributions, J. Stat. Theory Appl., 19 (2020), 133–147. https://doi.org/10.2991/jsta.d.200421.001 doi: 10.2991/jsta.d.200421.001
![]() |
[8] |
D. Zhang, M. Hu, Q. Ji, Financial markets under the global pandemic of COVID-19, Financ. Res. Lett., 36 (2020), 101528. https://doi.org/10.1016/j.frl.2020.101528 doi: 10.1016/j.frl.2020.101528
![]() |
[9] |
E. Arrigo, C. Liberati, P. Mariani, Social media data and users' preferences: A statistical analysis to support marketing communication, Big Data Res., 24 (2021), 100189. https://doi.org/10.1016/j.bdr.2021.100189 doi: 10.1016/j.bdr.2021.100189
![]() |
[10] |
M. Z. Rashid, A. S. Akhter, Survival weighted power function distribution with applications to medical, oceanology and metrology data, Adv. Appl. Stat., 67 (2021), 117–253. https://doi.org/10.17654/AS067020133 doi: 10.17654/AS067020133
![]() |
[11] |
W. Wang, Z. Ahmad, O. Kharazmi, C. B. Ampadu, E. H. Hafez, M. M. M. El-Din, New generalized-X family: Modeling the reliability engineering applications, Plos One, 16 (2021), e0248312. https://doi.org/10.1371/journal.pone.0248312 doi: 10.1371/journal.pone.0248312
![]() |
[12] |
C. S. Kumar, S. R. Nair, A generalized Log-Weibull distribution with bio-medical applications, Int. J. Stat. Med. Res., 10 (2021), 10–21. https://doi.org/10.6000/1929-6029.2021.10.02 doi: 10.6000/1929-6029.2021.10.02
![]() |
[13] |
H. S. Bakouch, C. Chesneau, O. A. Elsamadony, The Gumbel kernel for estimating the probability density function with application to hydrology data, J. Data Inform. Manag., 3 (2021), 261–269. https://doi.org/10.1007/s42488-021-00058-y doi: 10.1007/s42488-021-00058-y
![]() |
[14] |
D. Bhati, S. Ravi, On generalized log-Moyal distribution: A new heavy tailed size distribution, Insur. Math. Econ., 79 (2018), 247–259. https://doi.org/10.1016/j.insmatheco.2018.02.002 doi: 10.1016/j.insmatheco.2018.02.002
![]() |
[15] |
Z. Ahmad, E. Mahmoudi, G. G. Hamedani, O. Kharazmi, New methods to define heavy-tailed distributions with applications to insurance data, J. Taibah Univ. Sci., 14 (2020), 359–382. https://doi.org/10.1080/16583655.2020.1741942 doi: 10.1080/16583655.2020.1741942
![]() |
[16] |
Z. Li, J. Beirlant, S. Meng, Generalizing the log-Moyal distribution and regression models for heavy-tailed loss data, ASTIN Bull. J. IAA, 51 (2021), 57–99. https://doi.org/10.1017/asb.2020.35 doi: 10.1017/asb.2020.35
![]() |
[17] |
Ł. Bielak, A. Grzesiek, J. Janczura, A. Wyłomańska, Market risk factors analysis for an international mining company. Multi-dimensional, heavy-tailed-based modelling, Resour. Policy, 74 (2021), 102308. https://doi.org/10.1016/j.resourpol.2021.102308 doi: 10.1016/j.resourpol.2021.102308
![]() |
[18] |
D. B. Madan, Multivariate distributions for financial returns, Int. J. Theor. Appl. Fin., 23 (2020), 20500417. https://doi.org/10.1142/S0219024920500417 doi: 10.1142/S0219024920500417
![]() |
[19] |
C. O. Cepoi, Asymmetric dependence between stock market returns and news during COVID-19 financial turmoil, Financ. Res. Lett., 36 (2020), 101658. https://doi.org/10.1016/j.frl.2020.101658 doi: 10.1016/j.frl.2020.101658
![]() |
[20] |
W. Wang, W. Li, N. Zhang, K. Liu, Portfolio formation with preselection using deep learning from long-term financial data, Expert Syst. Appl., 143 (2020), 113042. https://doi.org/10.1016/j.eswa.2019.113042 doi: 10.1016/j.eswa.2019.113042
![]() |
[21] |
S. M. Carta, S. Consoli, A. S. Podda, D. R. Recupero, M. M. Stanciu, Ensembling and dynamic asset selection for risk-controlled statistical arbitrage, IEEE Access, 9 (2021), 29942–29959. https://doi.org/10.1109/ACCESS.2021.3059187 doi: 10.1109/ACCESS.2021.3059187
![]() |
[22] |
L. W. Cong, K. Tang, J. Wang, Y. Zhang, Deep sequence modeling: Development and applications in asset pricing, J. Financ. Data Sci., 3 (2021), 28–42. https://doi.org/10.3905/jfds.2020.1.053 doi: 10.3905/jfds.2020.1.053
![]() |
[23] |
F. Schuhmacher, H. Kohrs, B. R. Auer, Justifying mean-variance portfolio selection when asset returns are skewed, Manag. Sci., 67 (2021), 7812–7824. https://doi.org/10.1287/mnsc.2020.3846 doi: 10.1287/mnsc.2020.3846
![]() |
[24] |
S. Karim, M. U. Akhtar, R. Tashfeen, M. R. Rabbani, A. A. A. Rahman, A. AlAbbas, Sustainable banking regulations pre and during coronavirus outbreak: The moderating role of financial stability, Econ. Res.-Ekon. Istraž., 35 (2022), 3360–3377. https://doi.org/10.1080/1331677X.2021.1993951 doi: 10.1080/1331677X.2021.1993951
![]() |
[25] |
Z. Ahmad, G. G. Hamedani, N. S. Butt, Recent developments in distribution theory: A brief survey and some new generalized classes of distributions, Pak. J. Stat. Oper. Res., 15 (2019), 87–110. https://doi.org/10.18187/pjsor.v15i1.2803 doi: 10.18187/pjsor.v15i1.2803
![]() |
[26] |
A. Alzaatreh, C. Lee, F. Famoye, A new method for generating families of continuous distributions, Metron, 71 (2013), 63–79. https://doi.org/10.1007/s40300-013-0007-y doi: 10.1007/s40300-013-0007-y
![]() |
[27] |
J. L. Castle, J. A. Doornik, D. F. Hendry, Modelling non-stationary 'big data', Int. J. Forecasting, 37 (2021), 1556–1575. https://doi.org/10.1016/j.ijforecast.2020.08.002 doi: 10.1016/j.ijforecast.2020.08.002
![]() |
[28] |
F. Khan, A. Urooj, K. Ullah, B. Alnssyan, Z. Almaspoor, A comparison of Autometrics and penalization techniques under various error distributions: Evidence from Monte Carlo simulation, Complexity, 2021. https://doi.org/10.1155/2021/9223763 doi: 10.1155/2021/9223763
![]() |
[29] |
R. Tibshirani, Regression shrinkage and selection via the lasso, J. R. Stat. Soc. B, 58 (1996), 267–288. https://doi.org/10.1111/j.2517-6161.1996.tb02080.x doi: 10.1111/j.2517-6161.1996.tb02080.x
![]() |
[30] |
F. Khan, A. Urooj, S. A. Khan, S. K. Khosa, S. Muhammadullah, Z. Almaspoor, Evaluating the performance of feature selection methods using huge big data: A Monte Carlo simulation approach, Math. Prob. Eng., 2022. https://doi.org/10.1155/2022/6607330 doi: 10.1155/2022/6607330
![]() |
[31] |
S. Smeekes, E. Wijler, Macroeconomic forecasting using penalized regression methods, Int. J. Forecasting, 34 (2018), 408–430. https://doi.org/10.1016/j.ijforecast.2018.01.001 doi: 10.1016/j.ijforecast.2018.01.001
![]() |
[32] |
J. H. Stock, M. W. Watson, Macroeconomic forecasting using diffusion indexes, J. Bus. Econ. Stat., 20 (2002), 147–162. https://doi.org/10.1198/073500102317351921 doi: 10.1198/073500102317351921
![]() |
1. | Xiangming Tang, Jin-Taek Seong, Randa Alharbi, Aned Al Mutairi, Said G. Nasr, A new probabilistic model: Theory, simulation and applications to sports and failure times data, 2024, 10, 24058440, e25651, 10.1016/j.heliyon.2024.e25651 | |
2. | Jianping Zhu, Xuxun Cai, Eslam Hussam, Jin-Taek Seong, Fatimah A. Almulhima, Afaf Alrashidi, A novel cosine-derived probability distribution: Theory and data modeling with computer knowledge graph, 2024, 103, 11100168, 1, 10.1016/j.aej.2024.05.114 | |
3. | Omalsad Hamood Odhah, Huda M. Alshanbari, Zubair Ahmad, Faridoon Khan, Abd Al-Aziz Hosni El-Bagoury, A Novel Probabilistic Approach Based on Trigonometric Function: Model, Theory with Practical Applications, 2023, 15, 2073-8994, 1528, 10.3390/sym15081528 | |
4. | Li Jiang, Jin-Taek Seong, Marwan H. Alhelali, Basim S.O. Alsaedi, Fatimah M. Alghamdi, Ramy Aldallal, A new cosine-based approach for modelling the time-to-event phenomena in sports and engineering sectors, 2024, 98, 11100168, 19, 10.1016/j.aej.2024.04.037 | |
5. | Zhidong Liang, A new statistical approach with simulation study: Its implementations in management sciences and reliability, 2025, 119, 11100168, 531, 10.1016/j.aej.2025.01.113 | |
6. | Zhidong Liang, A new statistical model with optimal fitting performance: Its assessments in management sciences and reliability, 2025, 119, 11100168, 545, 10.1016/j.aej.2025.01.094 |
n | Parameters | MLEs | MSEs | Biases |
θ | 1.5306540 | 0.0845499 | 0.03065429 | |
25 | σ | 1.6616360 | 1.6801157 | 0.76163600 |
λ | 2.1413169 | 6.5317397 | 1.64131690 | |
θ | 1.4878190 | 0.0405659 | -0.01218063 | |
50 | σ | 1.3621325 | 0.8178465 | 0.46213251 |
λ | 1.7129257 | 4.8094673 | 1.21292570 | |
θ | 1.4833020 | 0.0266625 | -0.01669770 | |
75 | σ | 1.2395189 | 0.5670010 | 0.33951893 |
λ | 1.3942232 | 3.2730729 | 0.89422320 | |
θ | 1.4907440 | 0.0200039 | -0.00925587 | |
100 | σ | 1.1644847 | 0.4874031 | 0.26448468 |
λ | 1.2066578 | 2.6246481 | 0.70665780 | |
θ | 1.4939300 | 0.0128720 | -0.00606980 | |
200 | σ | 1.0687798 | 0.3153811 | 0.16877977 |
λ | 0.9406648 | 1.4242122 | 0.44066480 | |
θ | 1.4850080 | 0.0079217 | -0.01499184 | |
300 | σ | 1.0421690 | 0.2105968 | 0.14216899 |
λ | 0.7456199 | 0.7993679 | 0.30561990 | |
θ | 1.4895150 | 0.0067249 | -0.01048548 | |
400 | σ | 1.0060013 | 0.1530510 | 0.10600131 |
λ | 0.5966013 | 0.3541581 | 0.19660130 | |
θ | 1.4963270 | 0.0048694 | -0.00367308 | |
500 | σ | 0.9351764 | 0.1209646 | 0.07517640 |
λ | 0.5406189 | 0.2645338 | 0.14061890 |
n | Parameters | MLEs | MSEs | Biases |
θ | 1.3409810 | 0.06089320 | 4.0981e-02 | |
25 | σ | 0.9684732 | 0.32269754 | 0.26847323 |
λ | 2.4804880 | 6.35740400 | 1.58048760 | |
θ | 1.3207820 | 0.03098274 | 2.0781e-02 | |
50 | σ | 0.8257357 | 0.18517215 | 0.12573567 |
λ | 2.0018830 | 4.78013720 | 1.10188330 | |
θ | 1.3131030 | 0.02263240 | 1.3103e-02 | |
75 | σ | 0.8056960 | 0.16232288 | 0.10569596 |
λ | 1.8454520 | 3.95911760 | 0.94545150 | |
θ | 1.2969670 | 0.01678957 | -3.0325e-03 | |
100 | σ | 0.8074029 | 0.13781325 | 0.10740290 |
λ | 1.8371310 | 3.76135120 | 0.93713060 | |
θ | 1.3000190 | 0.01000074 | 1.9164e-05 | |
200 | σ | 0.7502970 | 0.09277251 | 0.05029699 |
λ | 1.4371740 | 2.04954990 | 0.53717410 | |
θ | 1.2876490 | 0.00735997 | -1.2350e-02 | |
300 | σ | 0.7879704 | 0.07972821 | 0.08797043 |
λ | 1.4682940 | 1.76350150 | 0.56829450 | |
θ | 1.2992960 | 0.00527875 | -7.0367e-04 | |
400 | σ | 0.7273118 | 0.05311106 | 0.02731182 |
λ | 1.1906500 | 0.97227000 | 0.29065020 | |
θ | 1.3000400 | 0.00404874 | 3.9596e-05 | |
500 | σ | 0.7113346 | 0.04542952 | 0.01133456 |
λ | 1.1060500 | 0.72525460 | 0.20605010 |
n | Parameters | MLEs | MSEs | Biases |
η | 0.8295898 | 0.08826282 | 0.12958978 | |
25 | ϕ | 1.6435660 | 0.20131711 | 0.04356598 |
λ | 1.8830760 | 1.75815404 | 0.58307614 | |
η | 0.7636202 | 0.03381550 | 0.06362024 | |
500 | ϕ | 1.6144310 | 0.11071804 | 0.01443136 |
λ | 1.5688950 | 0.60710519 | 0.26889488 | |
η | 0.7413941 | 0.02058671 | 0.04139414 | |
75 | ϕ | 1.6032170 | 0.07300298 | 0.00321683 |
λ | 1.4934430 | 0.38750257 | 0.19344311 | |
η | 0.7308403 | 0.01473447 | 0.03084032 | |
100 | ϕ | 1.6124770 | 0.05724885 | 0.01247658 |
λ | 1.4101360 | 0.15857094 | 0.11013555 | |
η | 0.7159275 | 0.00628118 | 0.01592747 | |
200 | ϕ | 1.5983640 | 0.02611875 | -0.00163616 |
λ | 1.3440490 | 0.02956159 | 0.04404876 | |
η | 0.7083867 | 0.00356276 | 0.00838667 | |
300 | ϕ | 1.6127650 | 0.01877270 | 0.01276520 |
λ | 1.3214120 | 0.01317318 | 0.02141173 | |
η | 0.7037929 | 0.00282884 | 0.00379289 | |
400 | ϕ | 1.6012280 | 0.01354377 | 0.00122802 |
λ | 1.3194250 | 0.01055359 | 0.01942524 | |
η | 0.7062376 | 0.00234377 | 0.00623763 | |
500 | ϕ | 1.5949220 | 0.01018247 | -0.00507846 |
λ | 1.3100780 | 0.00762077 | 0.01907786 |
n | Parameters | MLEs | MSEs | Biases |
η | 1.0040981 | 0.083711686 | 0.10409810 | |
25 | ϕ | 1.2140710 | 0.098602278 | 0.11407104 |
λ | 1.8282900 | 0.975918910 | 0.3282901 | |
η | 0.9628862 | 0.037615081 | 0.06288619 | |
500 | ϕ | 1.1532450 | 0.036819558 | 0.05324535 |
λ | 1.6785700 | 0.420855040 | 0.17856980 | |
η | 0.9416555 | 0.026231108 | 0.04165548 | |
75 | ϕ | 1.1467750 | 0.027392599 | 0.04677475 |
λ | 1.5794770 | 0.139978210 | 0.07947676 | |
η | 0.9243457 | 0.015663488 | 0.02434573 | |
100 | ϕ | 1.1333150 | 0.021317467 | 0.03331549 |
λ | 1.5717710 | 0.105736930 | 0.07177117 | |
η | 0.9111239 | 0.007281454 | 0.01112393 | |
200 | ϕ | 1.1173830 | 0.012048905 | 0.01738310 |
λ | 1.5347830 | 0.045606720 | 0.03478277 | |
η | 0.9082706 | 0.005246932 | 0.00827062 | |
300 | ϕ | 1.1059050 | 0.007748683 | 0.00590497 |
λ | 1.5355600 | 0.032318420 | 0.03556033 | |
η | 0.9051241 | 0.003407605 | 0.00512410 | |
400 | ϕ | 1.1116230 | 0.006835205 | 0.01162262 |
λ | 1.5194740 | 0.024970670 | 0.01947445 | |
η | 0.9068855 | 0.003215347 | 0.00688549 | |
500 | ϕ | 1.1049340 | 0.005313549 | 0.00493448 |
λ | 1.5219570 | 0.020792440 | 0.02195670 |
Model | ˆλMLE | ˆηMLE | ˆφMLE | ^α1MLE |
NRE-Fréchet | 0.00317 | 0.97677 | 25.00396 | - |
Fréchet | - | 3.14566 | 44.87837 | - |
APT-Fréchet | - | 4.20771 | 41.89421 | 139.89786 |
Model | CVM | AD | KS | p-value |
NRE-Fréchet | 0.27746 | 1.59522 | 0.11565 | 0.38000 |
Fréchet | 0.70603 | 4.02970 | 0.20811 | 0.06170 |
APT-Fréchet | 0.50369 | 2.873401 | 0.16504 | 0.07141 |
Model | ˆλMLE | ˆθMLE | ˆσMLE | ˆαMLE | ˆβMLE |
NRE-logistic | 1.11894 | 0.08833 | 0.06210 | - | - |
Logistic | - | 0.01619 | 0.05521 | - | - |
Exp-logistic | - | 0.05203 | 0.03959 | 0.55644 | - |
Kum-logistic | - | 0.09952 | 0.07700 | 1.06195 | 2.81753 |
Model | CVM | AD | KS | p-value |
NRE-logistic | 0.28244 | 1.68355 | 0.12088 | 0.33750 |
Logistic | 0.33210 | 1.91355 | 0.12817 | 0.27250 |
Exp-logistic | 0.30118 | 1.84708 | 0.12096 | 0.31480 |
Kum-logistic | 0.32518 | 1.96717 | 0.13960 | 0.18960 |
Method | RMSE | MAE | R-squared |
Lasso | 44.77 | 33.84 | 0.75 |
Enet | 41.71 | 31.30 | 0.78 |
n | Parameters | MLEs | MSEs | Biases |
θ | 1.5306540 | 0.0845499 | 0.03065429 | |
25 | σ | 1.6616360 | 1.6801157 | 0.76163600 |
λ | 2.1413169 | 6.5317397 | 1.64131690 | |
θ | 1.4878190 | 0.0405659 | -0.01218063 | |
50 | σ | 1.3621325 | 0.8178465 | 0.46213251 |
λ | 1.7129257 | 4.8094673 | 1.21292570 | |
θ | 1.4833020 | 0.0266625 | -0.01669770 | |
75 | σ | 1.2395189 | 0.5670010 | 0.33951893 |
λ | 1.3942232 | 3.2730729 | 0.89422320 | |
θ | 1.4907440 | 0.0200039 | -0.00925587 | |
100 | σ | 1.1644847 | 0.4874031 | 0.26448468 |
λ | 1.2066578 | 2.6246481 | 0.70665780 | |
θ | 1.4939300 | 0.0128720 | -0.00606980 | |
200 | σ | 1.0687798 | 0.3153811 | 0.16877977 |
λ | 0.9406648 | 1.4242122 | 0.44066480 | |
θ | 1.4850080 | 0.0079217 | -0.01499184 | |
300 | σ | 1.0421690 | 0.2105968 | 0.14216899 |
λ | 0.7456199 | 0.7993679 | 0.30561990 | |
θ | 1.4895150 | 0.0067249 | -0.01048548 | |
400 | σ | 1.0060013 | 0.1530510 | 0.10600131 |
λ | 0.5966013 | 0.3541581 | 0.19660130 | |
θ | 1.4963270 | 0.0048694 | -0.00367308 | |
500 | σ | 0.9351764 | 0.1209646 | 0.07517640 |
λ | 0.5406189 | 0.2645338 | 0.14061890 |
n | Parameters | MLEs | MSEs | Biases |
θ | 1.3409810 | 0.06089320 | 4.0981e-02 | |
25 | σ | 0.9684732 | 0.32269754 | 0.26847323 |
λ | 2.4804880 | 6.35740400 | 1.58048760 | |
θ | 1.3207820 | 0.03098274 | 2.0781e-02 | |
50 | σ | 0.8257357 | 0.18517215 | 0.12573567 |
λ | 2.0018830 | 4.78013720 | 1.10188330 | |
θ | 1.3131030 | 0.02263240 | 1.3103e-02 | |
75 | σ | 0.8056960 | 0.16232288 | 0.10569596 |
λ | 1.8454520 | 3.95911760 | 0.94545150 | |
θ | 1.2969670 | 0.01678957 | -3.0325e-03 | |
100 | σ | 0.8074029 | 0.13781325 | 0.10740290 |
λ | 1.8371310 | 3.76135120 | 0.93713060 | |
θ | 1.3000190 | 0.01000074 | 1.9164e-05 | |
200 | σ | 0.7502970 | 0.09277251 | 0.05029699 |
λ | 1.4371740 | 2.04954990 | 0.53717410 | |
θ | 1.2876490 | 0.00735997 | -1.2350e-02 | |
300 | σ | 0.7879704 | 0.07972821 | 0.08797043 |
λ | 1.4682940 | 1.76350150 | 0.56829450 | |
θ | 1.2992960 | 0.00527875 | -7.0367e-04 | |
400 | σ | 0.7273118 | 0.05311106 | 0.02731182 |
λ | 1.1906500 | 0.97227000 | 0.29065020 | |
θ | 1.3000400 | 0.00404874 | 3.9596e-05 | |
500 | σ | 0.7113346 | 0.04542952 | 0.01133456 |
λ | 1.1060500 | 0.72525460 | 0.20605010 |
n | Parameters | MLEs | MSEs | Biases |
η | 0.8295898 | 0.08826282 | 0.12958978 | |
25 | ϕ | 1.6435660 | 0.20131711 | 0.04356598 |
λ | 1.8830760 | 1.75815404 | 0.58307614 | |
η | 0.7636202 | 0.03381550 | 0.06362024 | |
500 | ϕ | 1.6144310 | 0.11071804 | 0.01443136 |
λ | 1.5688950 | 0.60710519 | 0.26889488 | |
η | 0.7413941 | 0.02058671 | 0.04139414 | |
75 | ϕ | 1.6032170 | 0.07300298 | 0.00321683 |
λ | 1.4934430 | 0.38750257 | 0.19344311 | |
η | 0.7308403 | 0.01473447 | 0.03084032 | |
100 | ϕ | 1.6124770 | 0.05724885 | 0.01247658 |
λ | 1.4101360 | 0.15857094 | 0.11013555 | |
η | 0.7159275 | 0.00628118 | 0.01592747 | |
200 | ϕ | 1.5983640 | 0.02611875 | -0.00163616 |
λ | 1.3440490 | 0.02956159 | 0.04404876 | |
η | 0.7083867 | 0.00356276 | 0.00838667 | |
300 | ϕ | 1.6127650 | 0.01877270 | 0.01276520 |
λ | 1.3214120 | 0.01317318 | 0.02141173 | |
η | 0.7037929 | 0.00282884 | 0.00379289 | |
400 | ϕ | 1.6012280 | 0.01354377 | 0.00122802 |
λ | 1.3194250 | 0.01055359 | 0.01942524 | |
η | 0.7062376 | 0.00234377 | 0.00623763 | |
500 | ϕ | 1.5949220 | 0.01018247 | -0.00507846 |
λ | 1.3100780 | 0.00762077 | 0.01907786 |
n | Parameters | MLEs | MSEs | Biases |
η | 1.0040981 | 0.083711686 | 0.10409810 | |
25 | ϕ | 1.2140710 | 0.098602278 | 0.11407104 |
λ | 1.8282900 | 0.975918910 | 0.3282901 | |
η | 0.9628862 | 0.037615081 | 0.06288619 | |
500 | ϕ | 1.1532450 | 0.036819558 | 0.05324535 |
λ | 1.6785700 | 0.420855040 | 0.17856980 | |
η | 0.9416555 | 0.026231108 | 0.04165548 | |
75 | ϕ | 1.1467750 | 0.027392599 | 0.04677475 |
λ | 1.5794770 | 0.139978210 | 0.07947676 | |
η | 0.9243457 | 0.015663488 | 0.02434573 | |
100 | ϕ | 1.1333150 | 0.021317467 | 0.03331549 |
λ | 1.5717710 | 0.105736930 | 0.07177117 | |
η | 0.9111239 | 0.007281454 | 0.01112393 | |
200 | ϕ | 1.1173830 | 0.012048905 | 0.01738310 |
λ | 1.5347830 | 0.045606720 | 0.03478277 | |
η | 0.9082706 | 0.005246932 | 0.00827062 | |
300 | ϕ | 1.1059050 | 0.007748683 | 0.00590497 |
λ | 1.5355600 | 0.032318420 | 0.03556033 | |
η | 0.9051241 | 0.003407605 | 0.00512410 | |
400 | ϕ | 1.1116230 | 0.006835205 | 0.01162262 |
λ | 1.5194740 | 0.024970670 | 0.01947445 | |
η | 0.9068855 | 0.003215347 | 0.00688549 | |
500 | ϕ | 1.1049340 | 0.005313549 | 0.00493448 |
λ | 1.5219570 | 0.020792440 | 0.02195670 |
Model | ˆλMLE | ˆηMLE | ˆφMLE | ^α1MLE |
NRE-Fréchet | 0.00317 | 0.97677 | 25.00396 | - |
Fréchet | - | 3.14566 | 44.87837 | - |
APT-Fréchet | - | 4.20771 | 41.89421 | 139.89786 |
Model | CVM | AD | KS | p-value |
NRE-Fréchet | 0.27746 | 1.59522 | 0.11565 | 0.38000 |
Fréchet | 0.70603 | 4.02970 | 0.20811 | 0.06170 |
APT-Fréchet | 0.50369 | 2.873401 | 0.16504 | 0.07141 |
Model | ˆλMLE | ˆθMLE | ˆσMLE | ˆαMLE | ˆβMLE |
NRE-logistic | 1.11894 | 0.08833 | 0.06210 | - | - |
Logistic | - | 0.01619 | 0.05521 | - | - |
Exp-logistic | - | 0.05203 | 0.03959 | 0.55644 | - |
Kum-logistic | - | 0.09952 | 0.07700 | 1.06195 | 2.81753 |
Model | CVM | AD | KS | p-value |
NRE-logistic | 0.28244 | 1.68355 | 0.12088 | 0.33750 |
Logistic | 0.33210 | 1.91355 | 0.12817 | 0.27250 |
Exp-logistic | 0.30118 | 1.84708 | 0.12096 | 0.31480 |
Kum-logistic | 0.32518 | 1.96717 | 0.13960 | 0.18960 |
Method | RMSE | MAE | R-squared |
Lasso | 44.77 | 33.84 | 0.75 |
Enet | 41.71 | 31.30 | 0.78 |