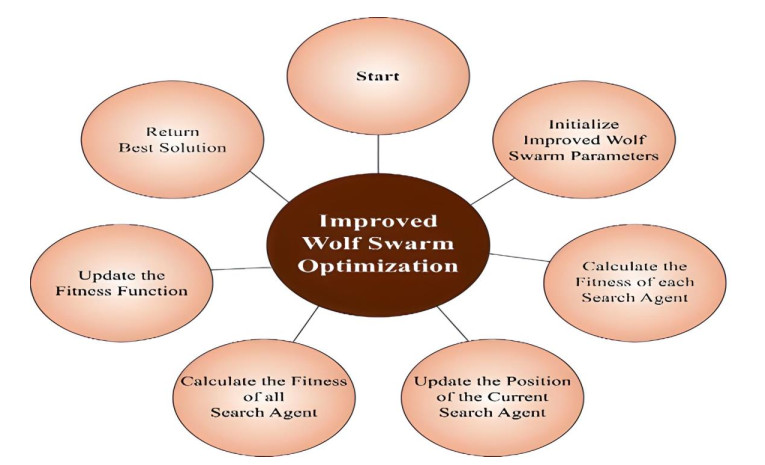
A wide variety of applications like patient monitoring, rehabilitation sensing, sports and senior surveillance require a considerable amount of knowledge in recognizing physical activities of a person captured using sensors. The goal of human activity recognition is to identify human activities from a collection of observations based on the behavior of subjects and the surrounding circumstances. Movement is examined in psychology, biomechanics, artificial intelligence and neuroscience. To be specific, the availability of pervasive devices and the low cost to record movements with machine learning (ML) techniques for the automatic and quantitative analysis of movement have resulted in the growth of systems for rehabilitation monitoring, user authentication and medical diagnosis. The self-regulated detection of human activities from time-series smartphone sensor datasets is a growing study area in intelligent and smart healthcare. Deep learning (DL) techniques have shown enhancements compared to conventional ML methods in many fields, which include human activity recognition (HAR). This paper presents an improved wolf swarm optimization with deep learning based movement analysis and self-regulated human activity recognition (IWSODL-MAHAR) technique. The IWSODL-MAHAR method aimed to recognize various kinds of human activities. Since high dimensionality poses a major issue in HAR, the IWSO algorithm is applied as a dimensionality reduction technique. In addition, the IWSODL-MAHAR technique uses a hybrid DL model for activity recognition. To further improve the recognition performance, a Nadam optimizer is applied as a hyperparameter tuning technique. The experimental evaluation of the IWSODL-MAHAR approach is assessed on benchmark activity recognition data. The experimental outcomes outlined the supremacy of the IWSODL-MAHAR algorithm compared to recent models.
Citation: Tamilvizhi Thanarajan, Youseef Alotaibi, Surendran Rajendran, Krishnaraj Nagappan. Improved wolf swarm optimization with deep-learning-based movement analysis and self-regulated human activity recognition[J]. AIMS Mathematics, 2023, 8(5): 12520-12539. doi: 10.3934/math.2023629
[1] | Hanan T. Halawani, Aisha M. Mashraqi, Yousef Asiri, Adwan A. Alanazi, Salem Alkhalaf, Gyanendra Prasad Joshi . Nature-Inspired Metaheuristic Algorithm with deep learning for Healthcare Data Analysis. AIMS Mathematics, 2024, 9(5): 12630-12649. doi: 10.3934/math.2024618 |
[2] | Mashael Maashi, Mohammed Abdullah Al-Hagery, Mohammed Rizwanullah, Azza Elneil Osman . Deep convolutional neural network-based Leveraging Lion Swarm Optimizer for gesture recognition and classification. AIMS Mathematics, 2024, 9(4): 9380-9393. doi: 10.3934/math.2024457 |
[3] | Youseef Alotaibi, Veera Ankalu. Vuyyuru . Electroencephalogram based face emotion recognition using multimodal fusion and 1-D convolution neural network (ID-CNN) classifier. AIMS Mathematics, 2023, 8(10): 22984-23002. doi: 10.3934/math.20231169 |
[4] | Mashael M Asiri, Abdelwahed Motwakel, Suhanda Drar . Robust sign language detection for hearing disabled persons by Improved Coyote Optimization Algorithm with deep learning. AIMS Mathematics, 2024, 9(6): 15911-15927. doi: 10.3934/math.2024769 |
[5] | Thavavel Vaiyapuri, M. Sivakumar, Shridevi S, Velmurugan Subbiah Parvathy, Janjhyam Venkata Naga Ramesh, Khasim Syed, Sachi Nandan Mohanty . An intelligent water drop algorithm with deep learning driven vehicle detection and classification. AIMS Mathematics, 2024, 9(5): 11352-11371. doi: 10.3934/math.2024557 |
[6] | Zhencheng Fan, Zheng Yan, Yuting Cao, Yin Yang, Shiping Wen . Enhancing skeleton-based human motion recognition with Lie algebra and memristor-augmented LSTM and CNN. AIMS Mathematics, 2024, 9(7): 17901-17916. doi: 10.3934/math.2024871 |
[7] | Abdelwahed Motwake, Aisha Hassan Abdalla Hashim, Marwa Obayya, Majdy M. Eltahir . Enhancing land cover classification in remote sensing imagery using an optimal deep learning model. AIMS Mathematics, 2024, 9(1): 140-159. doi: 10.3934/math.2024009 |
[8] | Thavavel Vaiyapuri, Prasanalakshmi Balaji, S. Shridevi, Santhi Muttipoll Dharmarajlu, Nourah Ali AlAseem . An attention-based bidirectional long short-term memory based optimal deep learning technique for bone cancer detection and classifications. AIMS Mathematics, 2024, 9(6): 16704-16720. doi: 10.3934/math.2024810 |
[9] | Chenmin Ni, Muhammad Fadhil Marsani, Fam Pei Shan, Xiaopeng Zou . Flood prediction with optimized gated recurrent unit-temporal convolutional network and improved KDE error estimation. AIMS Mathematics, 2024, 9(6): 14681-14696. doi: 10.3934/math.2024714 |
[10] | Manal Abdullah Alohali, Fuad Al-Mutiri, Kamal M. Othman, Ayman Yafoz, Raed Alsini, Ahmed S. Salama . An enhanced tunicate swarm algorithm with deep-learning based rice seedling classification for sustainable computing based smart agriculture. AIMS Mathematics, 2024, 9(4): 10185-10207. doi: 10.3934/math.2024498 |
A wide variety of applications like patient monitoring, rehabilitation sensing, sports and senior surveillance require a considerable amount of knowledge in recognizing physical activities of a person captured using sensors. The goal of human activity recognition is to identify human activities from a collection of observations based on the behavior of subjects and the surrounding circumstances. Movement is examined in psychology, biomechanics, artificial intelligence and neuroscience. To be specific, the availability of pervasive devices and the low cost to record movements with machine learning (ML) techniques for the automatic and quantitative analysis of movement have resulted in the growth of systems for rehabilitation monitoring, user authentication and medical diagnosis. The self-regulated detection of human activities from time-series smartphone sensor datasets is a growing study area in intelligent and smart healthcare. Deep learning (DL) techniques have shown enhancements compared to conventional ML methods in many fields, which include human activity recognition (HAR). This paper presents an improved wolf swarm optimization with deep learning based movement analysis and self-regulated human activity recognition (IWSODL-MAHAR) technique. The IWSODL-MAHAR method aimed to recognize various kinds of human activities. Since high dimensionality poses a major issue in HAR, the IWSO algorithm is applied as a dimensionality reduction technique. In addition, the IWSODL-MAHAR technique uses a hybrid DL model for activity recognition. To further improve the recognition performance, a Nadam optimizer is applied as a hyperparameter tuning technique. The experimental evaluation of the IWSODL-MAHAR approach is assessed on benchmark activity recognition data. The experimental outcomes outlined the supremacy of the IWSODL-MAHAR algorithm compared to recent models.
The goal of human activity recognition (HAR) is to identify activities from a collection of data on subject behavior and environmental factors. Computer vision and human-computer interaction experts have been working on HAR for the last three decades, proposing several methods and techniques for improving the process. The earliest HAR-related work was done in the late 1990s. A lot of recent research has been devoted to testing out methods for distinguishing activities of daily life (ADLs) from inertial signals. The widespread use of mobile devices with inertial sensors and the falling cost of hardware are the key causes of this. Smart homes, surveillance, healthcare and other application contexts can all benefit from the use of smartphones that can acquire and process signals. The advancement of new systems with assistive and medical techniques to create an appropriate environment (provide living conditions via the environment) or provide long-term care shows that research workers are exploring the living standard of elder people and their individuality [1]. HAR is an innovative technology that could identify human activities via computer systems and sensors. A HAR system is complicated and could monitor individual situations and provide effective devices in case of an emergency [2]. Activity represents a behavior that comprises a set of activities done by interacting with one another or one individual. Providing appropriate and accurate data regarding the activity is the major computation task in the HAR technique [3]. With the increased development of neural networks, computing and machine learning algorithms, HAR based on wearable sensors becomes widespread in different areas involving medical services, smart homes, healthcare for old age people [4], mechanization in industry, enlightening human interface with computers, security systems, robot monitoring system, monitoring athlete training and rehabilitation systems. It is categorized into 3 classes in data acquisition: wearable sensors, external sensors (non-wearable) and an incorporation of the 2 abovementioned classes [5].
Due to the advancement in machine learning and context-aware technologies, researchers have employed distinct techniques for HAR using data gathered from smartphones [6]. Smartphones have become more popular for HAR because of three reasons. The first is the ubiquitous nature of this smaller device that is utilized by almost everybody. The next is the efficiency and reliability of the data procurement, and finally, less restriction is considered with respect to privacy concerns. Thus, many kinds of research were introduced through distinct artificial intelligence (AI) technologies [7]. Lately, deep learning (DL) has revolutionized conventional machine learning (ML) and brought about enhanced performance in various domains involving object detection, image recognition, natural language processing and speech recognition.
DL has enhanced the robustness and performance of HAR, which speeds up its application and adoption to a wider variety of wearable sensor-based applications [8]. There exist two major reasons why DL is robust for a variety of applications. First, the DL method is skilled at directly learning strong features from raw information for certain uses, while the feature needs to be manually engineered or extracted in a conventional ML technique that generally needs a large amount of human effort and proficient domain knowledge [9]. Deep neural networks (DNN) could effectively learn representative features from raw signals with smaller domain knowledge. Any neural network architecture, mathematically speaking, seeks to identify any mathematical function y = f(x) that can map attributes(x) to output (y) [10]. DL has witnessed considerable development in HAR-based applications due to this expressive power.
This paper presents an improved wolf swarm optimization with deep learning based movement analysis and self-regulated human activity recognition (IWSODL-MAHAR) method. The IWSODL-MAHAR approach aimed to recognize various kinds of human activities. The IWSO algorithm is applied as a dimensionality reduction technique. In addition, the IWSODL-MAHAR technique uses a hybrid DL model for activity recognition. To further improve the recognition performance, a Nadam optimizer is applied as a hyperparameter tuning technique. The experimental evaluation of the IWSODL-MAHAR technique is performed with benchmark activity recognition datasets.
Hassan et al. [11] introduced a mobile device inertial sensor-related methodology for HAR. In this study, efficient features will be first mined from the raw datasets. The features involve autoregressive coefficients, mean, median and so on. To make them more robust, the features are processed by linear discriminant analysis (LDA) and kernel principal component analysis (KPCA). At last, the features will be trained with deep belief networks (DBN) for effective activity recognition. In [12], a method for HAR utilizing DL was modeled related to stepped frequency continuous wave (SFCW) radar. To be specific, SFCW radar was mainly employed for generating 2 types of characteristic representation domains, as they are range maps in the range domain and multiple frequencies of spectrograms in the time–frequency domain. After that, a particular DL network, which includes a sparse auto encoders (AE) and multiple parallel deep convolutional neural network (DCNN), was modeled for extracting and fusing such features linked with human actions from range maps and multifrequency spectrograms.
In [13], the authors focused on the DL-enhanced HAR in Internet of healthcare things (IoHT) atmospheres. A semi-supervised DL structure was devised and framed for precise HAR which effectively employs and examines the weakly labeled sensor datasets for training the classifier learning method. An intellectual autolabeling technique related to deep Q-network (DQN) can be formulated with new modeled distance-oriented reward rules, to better solve the issue of inadequately labeled samples that enhances the learning efficiency in Internet of things (IoT) platforms. Gumaei et al. [14] introduced a potential multi-sensor-oriented structure for HAR utilizing a hybrid DL method, which integrates the gated recurrent unit (GRU) and a simple recurrent unit (SRU) of NN. The authors employ deep SRUs for processing a series of multimodal input datasets by utilizing the ability of their internal memory states. Chen et al. [15] introduced an innovative DL related method, that is, attention oriented BLSTM (ABLSTM), for passive HAR utilizing WiFi channel state information (CSI) signals. From raw sequential CSI readings, representative features in two directions were learned using the BLSTM. The authors use an attention system for assigning different weights for every learned feature.
Thakur et al. [16] proposed a DL-related method for activity recognition with smartphone sensor dataset, that is, gyroscope dataset and accelerometer. Long short-term memory networks (LSTM), convolutional neural network (CNN), and auto encoders (AE) possess complementary modeling capabilities, as LSTMs are adept at temporal modeling, CNNs are suitable for automated feature extraction and AEs were employed for dimensionality reduction. Wan et al. [17] presented a smartphone inertial accelerometer-oriented structure for HAR. Whenever the participants do day-to-day activities, the smartphone will collect the sensory data series, derive the high-efficiency features from the original datasets and acquire physical behavior data of users by utilizing multiple three-axis accelerometers. Moreover, a real-time human activity classifier technique relies upon a CNN that was modeled, which leverages CNNs for local feature extracting purposes.
In this paper, an automated IWSODL-MAHAR algorithm has been modeled for movement and action detection. The major intention of the IWSODL-MAHAR technique is to recognize various kinds of human activities. At the primary level, the activity data is preprocessed to transform it into a meaningful format. Next, the dimensionality reduction process is carried out using the IWSO algorithm. Finally, the hybrid DL model is applied to the activity recognition process.
In this study, the activity data is primarily preprocessed to transform it into a meaningful format. The standard scalar approach is used to remove the mean and scale the data into unit variance. The major concept behind the standard scalar approach is that it converts the data so that the distribution holds an average value of 0 and a standard deviation of 1. For multivariate data, the preprocessing occurs feature-wise (in other words, independently for every column of data). For a provided data distribution, every individual value in the dataset has the mean value deducted and is divided by the standard deviation of the entire dataset.
To reduce the high dimensionality of data, the IWSO algorithm is utilized in this work. The IWSO technique is an optimum search method developed for simulating prey allocation, wolves' division of labor and cooperative hunting [18,19]. It has the features of fast convergence and global search. In comparison to WSO, the improvement of IWSO has been demonstrated in the following. In roaming behavior, we present the wolf detection updating rule and design a "coarse to fine" roaming model. The fundamental concept is shown in the following: In the h direction around wolf detective i, if the function value in the h direction is small when compared to the main function values of wolf detective i, then it is likely that there will be a maximum value near the wolf detective i, and the tour was reduced.
xpid=xid+(1−kkmax+η)× sin(2π×pli+Θ)×stepda, | (1) |
In Eq (1), p refers to the direction, d denotes the dimension, stepa represent the walking step length, k shows the iteration count, and η∈(−0.1,0.1), Θ∈(0,p/li), h∈[4,7] are integers. In comparison to the conventional WSO, the IWSO has the following features: IWSO technique has strong global search capability and escapes from local optima; the proposal of siege radius improves the local development capability and quantifies the algorithm to escape from the local optima; in the solution of 2D functions, the calculation accurateness and convergence speed of IWSO were high; in the solution of calculation speed, the multidimensional complex function, the convergence speed and calculation accuracy of IWSO were more efficient. Figure 1 illustrates the steps involved in IWSO technique.
The computation step was shown below:
Step 1: Initialization.
As per the reverse bidirectional chaotic update approach, the maximal count of iterations kmax, number N of artificial wolves, distance determination factors ω, maximal number of walks Tmax, wolf group spatial position X, update scale factor β and step factor S are initialized.
Step 2: Wandering behavior.
The artificial wolf with large main function value was chosen as the head wolf, and the remaining artificial wolves were considered detective wolves and walk based on Eq. (1) unless the Yi objective function value of i - th wolf detective was larger than YLead main function value of leading wolves
Step 3: Summoning behavior.
The Alpha Wolf was named, and Detective Wolves quickly rushed to Alpha Wolf as follows:
xk+1id=xk+1id+Stepdb×gkd−xkid|gkd−xkid| | (2) |
When the Yi function values of ith wolf are greater than the Ylead function value of the head wolf, return to the end of Step 2; when Yi function values of i - th wolve is lesser than function value Ylead of head wolve, the i - th wolf detective continue to attack unless it enters siege region, viz., distance Li among i - th wolf detective and head, wolf is lesser than or equivalent to Llead, and return to Step 4.
Step 4: Siege behaviour.
The location of the head wolves is considered the prey location, and wolves contributing in the siege would besiege the prey.
Step 5: Wolves update.
The objective function value of the optimum wolf produced in these iterations is compared to the main function values of head wolves in the preceding iteration. Define the number R of artificial wolves with a small value of the main function to be removed based on the updating scale factor β. When t is lesser than tmax, the wolf group would be upgraded based on the subsequent equation and integrated with reverse learning; then, the wolf group would be upgraded based on the reverse double chaos strategy:
xid=gd. [ sin (γ)+1], | (3) |
where ∈(−0.1,0.1).
Step 6: Judgment ended.
Decide whether the head wolf's objective function has fulfilled the requirement of computational performance, or the process obtains the maximal amount of iterations kmax. Then, the output is the location of the head wolf, that is, the objective function value, or else, return to Step 2.
The fitness function (FF) employed in the presented technique was modeled to maintain a balance between the classifier accuracy (max) gained through these selected features and the number of selected features in all solutions (min). Eq (4) signifies the FF to assess solutions.
Fitness=αγR(D)+β|R||C| | (4) |
where γR(D) signifies the classifier error rate of a given classifier was exploited here. α and β were 2 parameters equivalent to the significance of classification quality and subset length , |C| denotes total number of features in the dataset ,|R| indicates cardinality of the selected subset β=1−α.
The IWSO algorithm involves three types of wolves: alpha, beta and omega. The alpha wolf is the best solution found so far, the beta wolf is the second-best solution, and the omega wolf is the third-best solution. The algorithm starts with a random population of wolves and iteratively improves the solutions by following the hunting behavior of wolves.
Algorithm 1: IWSO algorithm Pseudo-code
Initialize population of wolves
Calculate fitness of each wolf
Set global best wolf as the wolf with the highest fitness
repeat until termination criteria are met:
for each wolf:
Determine the three neighboring wolves: alpha, beta and delta
Generate a new position for the wolf using the formula:
new_position = current_position + (alpha_position - (A * D)) * r1
+ (beta_position - (B * D)) * r2
+ (delta_position - (C * D)) * r3
Compute the new position's suitability.
If the new position is better than the current position, update the wolf's position and
fitness
If the new position is the best among all wolves, update the global best position
Update the values of A, B, C and D for the next iteration
Return the best wolf and its fitness
For activity recognition, the IWSODL-MAHAR technique makes use of a hybrid DL model. In this work, a hybrid form of bidirectional long short-term memory (BLSTM) and enhanced recurrent neural network (ERNN) is developed to correctly label the features of the input datasets [20,21,22]. In the presented method, the features were passed initially to the BLSTM layer and later to the ERNN layer for precisely mapping the features to the accurate label. The BLSTM network makes use of forward and backward passes for learning the feature, while the ERNN makes use of feedback from context layer for accurately predicting labels. BLSTM is a kind of recurrent NN (RNN) with 2 LSTM units collectively compiled in distinct directions. The forward and backward layers of LSTM process the feature in forward and backward directions. These features are an enhancement of the conventional LSTM for preserving the previous and upcoming datasets. As well, this might assist in better understanding the context of classifying brain tumors. The LSTM unit encompasses forget, input, output and hidden gates in cell state, and the computation of LSTM forward units is defined as follows:
ζt=σ(→ωζ[→ћt−1→xxt]+→βζ) | (5) |
ηt=σ(→ωn[→ћt−1→xt]+→βη) | (6) |
→ot=σ(→ωo[→ћt−1→xt]+→βo) | (7) |
→ξt=tanh(→ωξ[→ћt−1,→x]+→βξ) | (8) |
→Θt=→ζt⊗→Θt−1♁→ηt⊗→ξt | (9) |
→ћt=→ot⊗tanh(→Θt) | (10) |
Now, ζt represents forget gate outcome; ηt specifies input gate outcome; 0t shows the total resultant of output gate; ξt refers to the candidate cell states output; Θt signifies the cell state output; ћt characterizes the hidden state outcome; σ means the sigmoid activation function and tanh activation functions; ћt−1 stands for the prior hidden state outcome; χt denotes the input; ωζ, ωη, ωo and ωξ symbolize the weight vectors of forgetting, input, output and cell state gates; βζ,βη,β0 and βξ indicate the bias vectors of corresponding gates; and Θt−1 is represents the outcome of earlier cell state. The computation is also considered for LSTM units in the backward direction. The total resultant of the hidden state in a backward direction is provided in the following expression:
←ћt=f(χtΘt−1←LSTM) | (11) |
The output of Bi‐LSTM layer was transferred to the ERNN layer for appropriately labeling the feature. The major reason for the hybridization of BLSTM with the ERNN was to improve the classification performance. This can be accomplished by increasing the amount of data available for the NN in the training stage. The ERNN layer encompasses output, input and hidden components for classification and feature extraction. The input layer directly sends the input towards the hidden layer (HL), and the HL is accountable to derive features from input. One of the main advantages of the hybrid method is that the discriminatory nature of the method will be increased. Thus, the model can recognize the differences among the features and label them accordingly. The HL of ERNN is defined by Eq (12):
At=fh(ϖhxt+vhAt−1+βh) | (12) |
Now, At represents the output of HL, fλ indicates activation function, χt denotes the input to ERNN, ϖλ and vλ represent the weight matrix, and βλ represents bias vector. The ERNN method encompasses a context layer including self‐connected feedback for providing previous data. The context layer is defined below:
clt−1=Ajt | (13) |
In Eq (13), c1t−1 indicates the output of l - th context layer. Ajt represents output of the jth HL, and it is evaluated as follows:
Ot=f0(ϖO[λt]+β0) | (14) |
In Eq (14), f0 denotes activation function of the output layer, ϖO indicates weight values of the output layer, and β0 indicates bias vector of the output layer. The output from the FC layer was given to the softmax layer in which probability is allocated to all classes. The probability dominance might assist in deciding the accurate class for the input feature.
To adjust the hyperparameter values of the hybrid DL model, the Nadam optimizer is employed in this work. The Nadam optimizer tries to join Nesterov accelerated adaptive moment prediction with the Adam optimizer [23,24]. The advantage of this technique is that the used adaptive moment prediction helps to accomplish a very accurate stage in the gradient direction by updating module variables including the momentum stage before the gradient computation [25,26]. The upgrade rule of Nadam is formulated as
wt=wt−1−αׯmt√ˆvt+ϵ, | (15) |
where
¯mt=(1−β1,t)ˆgt+β1,t+1ˆmt, |
ˆmt=mt1−∏t+1i=1β1i, | (16) |
ˆgt=gt1−∏t+1i=1 β1i. |
In this section, the activity recognition of the IWSODL-MAHAR method is tested by utilizing the UCI HAR dataset [27]. It is a balanced dataset which comprises 10299 samples with six class labels as portrayed in Table 1. The sample images are shown in Figure 2.
UCI HAR | ||
Label | Class | No. of Instances |
0 | Walking | 1722 |
1 | Moving upward | 1444 |
2 | Moving downward | 1506 |
3 | Sitting | 1877 |
4 | Standing | 1808 |
5 | Lying | 1942 |
Total No. of Instances | 10299 |
The confusion matrices of the IWSODL-MAHAR model are shown in Figure 3. The figure represents that the IWSODL-MAHAR method has gained effectual recognition of human activities under all aspects.
Table 2 exhibits the overall activity recognition outcome of the IWSODL-MAHAR model with 60% of Training (TR) databases and 40% of Testing (TS) databases. In Figure 4, the overall HAR outcome of the IWSODL-MAHAR method on 60% of TR database is given. The experimental values demonstrated that the IWSODL-MAHAR model identified all activities. It is observable that the IWSODL-MAHAR model attained average accuy of 99.47%, sensy of 98.40%, specy of 99.68%, Fscore of 98.39%, receiver operating characteristic (ROC) ROCscore of 99.04% and Matthews correlation coefficient (MCC) of 98.07%.
Labels | Accuracy | Sensitivity | Specificity | F-Score | ROC Score | MCC |
Training Phase (60%) | ||||||
0 | 99.43 | 98.27 | 99.67 | 98.32 | 98.97 | 97.98 |
1 | 99.51 | 98.18 | 99.75 | 98.39 | 98.97 | 98.11 |
2 | 99.56 | 98.58 | 99.72 | 98.40 | 99.15 | 98.15 |
3 | 99.40 | 98.64 | 99.55 | 98.21 | 99.10 | 97.86 |
4 | 99.24 | 97.60 | 99.62 | 97.98 | 98.61 | 97.51 |
5 | 99.64 | 99.14 | 99.76 | 99.05 | 99.45 | 98.83 |
Average | 99.47 | 98.40 | 99.68 | 98.39 | 99.04 | 98.07 |
Testing Phase (40%) | ||||||
0 | 99.15 | 96.61 | 99.65 | 97.40 | 98.13 | 96.90 |
1 | 99.42 | 97.70 | 99.72 | 98.02 | 98.71 | 97.68 |
2 | 99.64 | 98.40 | 99.83 | 98.66 | 99.11 | 98.45 |
3 | 99.49 | 99.33 | 99.53 | 98.60 | 99.43 | 98.30 |
4 | 99.25 | 97.69 | 99.59 | 97.89 | 98.64 | 97.43 |
5 | 99.42 | 99.11 | 99.49 | 98.48 | 99.30 | 98.13 |
Average | 99.39 | 98.14 | 99.63 | 98.18 | 98.89 | 97.81 |
In Figure 5, an overall HAR outcome of the IWSODL-MAHAR approach on 40% of TS databases is given. The experimental values exhibited that the IWSODL-MAHAR system has identified all activities. It can be apparent that the IWSODL-MAHAR system has reached average accuy of 99.39%, sensy of 98.14%, specy of 99.63%, Fscore of 98.18%, ROCscore of 98.89% and MCC of 97.81%.
Table 3 displays the overall activity recognition outcome of the IWSODL-MAHAR approach on 70% of TR and 30% of TS databases. In Figure 6, an overall HAR outcome of the IWSODL-MAHAR approach on 70% of TR databases is given. It can be obvious that the IWSODL-MAHAR system has accomplished average accuy of 99.55%, sensy of 98.59%, specy of 99.73%, Fscore of 98.60%, ROCscore of 99.16% and MCC of 98.33%.
Labels | Accuracy | Sensitivity | Specificity | F-Score | ROC Score | MCC |
Training Phase (70%) | ||||||
0 | 99.54 | 98.75 | 99.70 | 98.63 | 99.23 | 98.35 |
1 | 99.40 | 98.32 | 99.59 | 98.00 | 98.96 | 97.65 |
2 | 99.49 | 97.82 | 99.74 | 98.07 | 98.78 | 97.78 |
3 | 99.68 | 98.71 | 99.88 | 99.07 | 99.30 | 98.88 |
4 | 99.64 | 98.87 | 99.81 | 99.02 | 99.34 | 98.80 |
5 | 99.53 | 99.07 | 99.64 | 98.79 | 99.36 | 98.50 |
Average | 99.55 | 98.59 | 99.73 | 98.60 | 99.16 | 98.33 |
Testing Phase (30%) | ||||||
0 | 99.68 | 98.85 | 99.84 | 99.04 | 99.35 | 98.85 |
1 | 99.61 | 98.72 | 99.77 | 98.72 | 99.25 | 98.49 |
2 | 99.42 | 97.07 | 99.81 | 97.95 | 98.44 | 97.62 |
3 | 99.39 | 98.70 | 99.53 | 98.25 | 99.12 | 97.87 |
4 | 99.71 | 99.30 | 99.80 | 99.22 | 99.55 | 99.04 |
5 | 99.74 | 99.63 | 99.76 | 99.26 | 99.70 | 99.11 |
Average | 99.59 | 98.71 | 99.75 | 98.74 | 99.23 | 98.50 |
In Figure 7, an overall HAR outcome of the IWSODL-MAHAR system on 30% of TS database is given. The experimental values exhibited that IWSODL-MAHAR algorithm has identified all activities. It can be apparent that the IWSODL-MAHAR algorithm has achieved average accuy of 99.59%, sensy of 98.71%, specy of 99.75%, Fscore of 98.74%, ROCscore of 99.23% and MCC of 98.50%.
The IWSODL-MAHAR algorithm's training accuracy (TACC) and validation accuracy (VACC) are assessed using HAR performance in Figure 8. The figure shows that the IWSODL-MAHAR algorithm performed better at maximum TACC and VACC values. The IWSODL-MAHAR algorithm has evidently produced better VACC results.
In Figure 9, the IWSODL-MAHAR system's training loss (TLS) and validation loss (VLS) are evaluated in relation to HAR performance. The graphic shows that the IWSODL-MAHAR algorithm performed better with low TLS and VLS values. The IWSODL-MAHAR system has produced less favorable VLS outcomes, as may be seen.
Figure 10 details an evident precision-recall analysis of the IWSODL-MAHAR system in the test database. The IWSODL-MAHAR approach produced higher precision recall values in many classes, as shown by the figure. Finally, the detailed comparative analysis of the IWSODL-MAHAR with recent methods is given in Table 4. Figure 11 offers a comparative accuy study of the IWSODL-MAHAR. The results implied the enhanced performance of the IWSODL-MAHAR model. Based on accuy, the IWSODL-MAHAR has reached an improved value of 99.59%. Contrastingly, the CNN [28], ensemble AE [29], Support Vector Machine (SVM) [30], ConvAE-LSTM [31], RelieF and hybrid gradient based optimizer- grey wolf optimizer feature selection (GBO-GWO) models have reported reduced accuy of 96.88%, 80.80%, 91.12%, and 98.67%. 96.71%, and 98.52%, respectively.
Methods | Accuracy | Sensitivity | Specificity |
IWSODL-MAHAR | 99.59 | 98.71 | 99.75 |
CNN Model | 96.88 | 97.64 | 98.19 |
Ensemble of AE Model | 80.80 | 81.36 | 82.00 |
SVM Model | 91.12 | 91.75 | 92.38 |
ConvAE-LSTM | 98.67 | 98.27 | 98.91 |
ReliefF Model | 96.71 | 97.49 | 98.14 |
Hybrid GBO-GWO | 98.52 | 97.90 | 98.11 |
Figure 12 provides a comparative sensy and specy analysis of the IWSODL-MAHAR technique. The outcomes showed the higher performance of the IWSODL-MAHAR approach. With respect to sensy, the IWSODL-MAHAR system has gained an enhanced value of 98.71%. Contrastingly, the CNN, ensemble AE, SVM, ConvAE-LSTM, RelieF and hybrid GBO-GWO methods have reported minimal sensy of 97.64%, 81.36%, 91.75%, 98.27%, 97.49%, and 97.90%, respectively.
Along with that, in terms of specy, the IWSODL-MAHAR has reached an improved value of 99.75%. Contrastingly, the CNN, ensemble AE, SVM, ConvAE-LSTM, RelieF and hybrid GBO-GWO approaches have reported minimal specy of 98.19%, 82%, 92.38%, 98.91%, 98.14% and 98.11%, respectively. The improved performance of the IWSODL-MAHAR technique over other current models was highlighted by these results.
In this paper, an automated IWSODL-MAHAR method has been presented for movement and action detection. The major intention of the IWSODL-MAHAR algorithm is to recognize various kinds of human activities. Initially, data preprocessing is performed to make the input activity data a compatible format for the activity recognition process. In addition, the IWSO algorithm is applied as a dimensionality reduction technique. For activity recognition, the IWSODL-MAHAR technique has employed a Nadam optimizer with a hybrid DL model. The Nadam optimizer is applied as a hyperparameter tuning technique to boost the classifier results. The experimental evaluation of the IWSODL-MAHAR approach is assessed on benchmark activity recognition datasets. The experimental outcomes outlined the supremacy of the IWSODL-MAHAR methodology compared to recent models. In the future, an ensemble of DL oriented fusion methods will be designed to boost the recognition performance.
This research has been funded by the Deanship for Research & Innovation, Ministry of Education in Saudi Arabia, funding this research work through project number IFP22UQU4281768DSR122.
Conceptualization, YA, SR. Methodology, TT, KN. Software, validation and formal analysis, YA, SR. Investigation, YA, KN. Resources, TT, SR. Data curation, YA. Writing—original draft preparation, YA, SR. Writing—review and editing, TT, KN. Visualization, KN, SR. Supervision, YA. Project administration, TT.
All authors declare no conflicts of interest in this paper
[1] |
Y. Wang, S. Cang, H. Yu, A survey on wearable sensor modality centred human activity recognition in health care, Expert Syst. Appl., 137 (2019), 167–190. https://doi.org/10.1016/j.eswa.2019.04.057 doi: 10.1016/j.eswa.2019.04.057
![]() |
[2] |
L. M. Dang, K. Min, H. Wang, M. J. Piran, C. H. Lee, H. Moon, Sensor-based and vision-based human activity recognition: A comprehensive survey, Pattern Recogn., 108 (2020), 107561. https://doi.org/10.1016/j.patcog.2020.107561 doi: 10.1016/j.patcog.2020.107561
![]() |
[3] |
K. A. Ogudo, R. Surendran, O. I. Khalaf, Optimal artificial intelligence based automated skin lesion detection and classification model, Comput. Syst. Sci. Eng., 44 (2023), 693–707. https://doi.org/10.32604/csse.2023.024154 doi: 10.32604/csse.2023.024154
![]() |
[4] | A. Subasi, M. Radhwan, R. Kurdi, K. Khateeb, IoT based mobile healthcare system for human activity recognition, Proceedings of the 15th learning and technology conference (L & T), Jeddah, Saudi Arabia, (2018), 29–34. https://doi.org/10.1109/LT.2018.8368507 |
[5] |
N. Ahmed, J. I. Rafiq, M. R. Islam, Enhanced human activity recognition based on smartphone sensor data using hybrid feature selection model, Sensors, 20 (2020), 317. https://doi.org/10.3390/s20010317 doi: 10.3390/s20010317
![]() |
[6] |
W. Taylor, S. A. Shah, K. Dashtipour, A. Zahid, Q. H. Abbasi, M. A. Imran, An intelligent non-invasive real-time human activity recognition system for next-generation healthcare, Sensors, 20 (2020), 2653. https://doi.org/10.3390/s20092653 doi: 10.3390/s20092653
![]() |
[7] |
S. Mekruksavanich, A. Jitpattanakul, Biometric user identification based on human activity recognition using wearable sensors: An experiment using deep learning models, Electronics, 10 (2021), 308. https://doi.org/10.3390/electronics10030308 doi: 10.3390/electronics10030308
![]() |
[8] |
V. Bianchi, M. Bassoli, G. Lombardo, P. Fornacciari, M. Mordonini, I. De Munari, IoT wearable sensor and deep learning: An integrated approach for personalized human activity recognition in a smart home environment, IEEE Internet Things, 6 (2019), 8553–8562. https://doi.org/10.1109/JIOT.2019.2920283 doi: 10.1109/JIOT.2019.2920283
![]() |
[9] |
N. Golestani, M. Moghaddam, Human activity recognition using magnetic induction-based motion signals and deep recurrent neural networks, Nat. Commun., 11 (2020), 1–11. https://doi.org/10.1038/s41467-020-15086-2 doi: 10.1038/s41467-020-15086-2
![]() |
[10] |
B. Vidya, P. Sasikumar, Wearable multisensor data fusion approach for human activity recognition using machine learning algorithms, Sensor. Actuat. A-Phys., 341 (2022), 113557. https://doi.org/10.1016/j.sna.2022.113557 doi: 10.1016/j.sna.2022.113557
![]() |
[11] |
M. M. Hassan, M. Z. Uddin, A. Mohamed, A. Almogren, A robust human activity recognition system using smartphone sensors and deep learning, Future Gener. Comp. Sy., 81 (2018), 307–313. https://doi.org/10.1016/j.future.2017.11.029 doi: 10.1016/j.future.2017.11.029
![]() |
[12] |
Y. Jia, Y. Guo, G. Wang, R. Song, G. Cui, X. Zhong, Multi-frequency and multidomain human activity recognition based on SFCW radar using deep learning, Neurocomputing, 444 (2021), 274–287. https://doi.org/10.1016/j.neucom.2020.07.136 doi: 10.1016/j.neucom.2020.07.136
![]() |
[13] |
X. Zhou, W. Liang, I. Kevin, K. Wang, H. Wang, L. T. Yang, et al., Deep-learning-enhanced human activity recognition for Internet of healthcare things, IEEE Internet Things, 7 (2020), 6429–6438. https://doi.org/10.1109/JIOT.2020.2985082 doi: 10.1109/JIOT.2020.2985082
![]() |
[14] |
A. Gumaei, M. M. Hassan, A. Alelaiwi, H. Alsalman, A hybrid deep learning model for human activity recognition using multimodal body sensing data, IEEE Access, 7 (2019), 99152–99160. https://doi.org/10.1109/ACCESS.2019.2927134 doi: 10.1109/ACCESS.2019.2927134
![]() |
[15] |
Z. Chen, L. Zhang, C. Jiang, Z. Cao, W. Cui, WiFi CSI based passive human activity recognition using attention-based BLSTM, IEEE T. Mobile Comput., 18 (2018), 2714–2724. https://doi.org/10.1109/TMC.2018.2878233 doi: 10.1109/TMC.2018.2878233
![]() |
[16] |
D. Thakur, S. Biswas, E. S. Ho, S. Chattopadhyay, Convae-lstm: Convolutional autoencoder long short-term memory network for smartphone-based human activity recognition, IEEE Access, 10 (2022), 4137–4156. https://doi.org/10.1109/ACCESS.2022.3140373 doi: 10.1109/ACCESS.2022.3140373
![]() |
[17] |
S. Wan, L. Qi, X. Xu, C. Tong, Z. Gu, Deep learning models for real-time human activity recognition on smartphones, Mobile Netw. Appl., 25 (2020), 743–755. https://doi.org/10.1007/s11036-019-01445-x doi: 10.1007/s11036-019-01445-x
![]() |
[18] | H. Guan, B. Tang, X. Zhou, H. Tan, Z. Liang, Y. Li, et al., Reliability analysis of boom of new lifting equipment based on improved wolf swarm algorithm, J. Eng., 2023 (2023). https://doi.org/10.1049/tje2.12202 |
[19] |
A. A. Malibari, S. S. Alotaibi, R. Alshahrani, S. Dhahbi, R. Alabdan, F. N. Al-wesabi, et al., A novel metaheuristic with deep learning enabled intrusion detection system for secured smart environment, Sustain. Energy Techn., 52 (2022), 102312. https://doi.org/10.1016/j.seta.2022.102312 doi: 10.1016/j.seta.2022.102312
![]() |
[20] |
M. H. Alharbi, A. N. Alqefari, Y. A. Alhawday, A. F. Alghammas, A. Hershan, Association of menstrual and reproductive factors with thyroid cancer in Saudi female patients, J. Umm Al-Qura University Medical Sci., 7 (2021), 11–13. https://doi.org/10.54940/ms81150310 doi: 10.54940/ms81150310
![]() |
[21] |
F. Alrowais, S. Althahabi, S. Alotaibi, A. Mohamed, M. A. Hamza, Automated machine learning enabled cyber security threat detection in Internet of things environment, Comput. Syst. Sci. Eng., 45 (2023), 687–700. https://doi.org/10.32604/csse.2023.030188 doi: 10.32604/csse.2023.030188
![]() |
[22] |
S. Rajagopal, T. Thanarajan, Y. Alotaibi, S. Alghamdi, Brain tumor: Hybrid feature extraction based on UNET and 3DCNN, Comput. Syst. Sci. Eng., 45 (2023), 2093–2109. https://doi.org/10.32604/csse.2023.032488 doi: 10.32604/csse.2023.032488
![]() |
[23] |
K. Nagappan, S. Rajendran, Y. Alotaibi, Trust aware Multi-Objective metaheuristic Optimization-Based secure route planning technique for Cluster-Based IIoT environment, IEEE Access, 10 (2022), 112686–112694. https://doi.org/10.1109/ACCESS.2022.3211971 doi: 10.1109/ACCESS.2022.3211971
![]() |
[24] |
M. A. Duhayyim, A. A. Malibari, S. Dhahbi, M. K. Nour, I. Al-Turaiki, Sailfish optimization with deep learning based oral cancer classification model, Comput. Syst. Sci. Eng., 45 (2023), 753–767. https://doi.org/10.32604/csse.2023.030556 doi: 10.32604/csse.2023.030556
![]() |
[25] |
R. Edwards, M. Wood, Branch prioritization motifs in biochemical networks with sharp activation. AIMS Math., 7 (2022), 1115–1146. https://doi.org/10.3934/math.2022066 doi: 10.3934/math.2022066
![]() |
[26] |
A. Q. Khan, Z. Saleem, T. F. Ibrahim, K. Osman, F. M. Alshehri, M. A. El-Moneam, Bifurcation and chaos in a discrete activator-inhibitor system, AIMS Math., 8 (2023), 4551–4574. https://doi.org/10.3934/math.2023225 doi: 10.3934/math.2023225
![]() |
[27] | https://archive.ics.uci.edu/ml/datasets/Smartphone+Dataset+for+Human+Activity+Recognition+%28HAR%29+in+Ambient+Assisted+Living+%28AAL%29 |
[28] |
Y. Tang, L. Zhang, F. Min, J. He, Multiscale deep feature learning for human activity recognition using wearable sensors, IEEE T. Ind. Electron., 70 (2023), 2106–2116. https://doi.org/10.1109/TIE.2022.3161812 doi: 10.1109/TIE.2022.3161812
![]() |
[29] |
T. Tamilvizhi, R. Surendran, K. Anbazhagan, K. Rajkumar, Quantum behaved particle swarm Optimization-Based deep transfer learning model for sugarcane leaf disease detection and classification, Math. Probl. Eng., 2022 (2022), 3452413. https://doi.org/10.1155/2022/3452413 doi: 10.1155/2022/3452413
![]() |
[30] |
C. Han, L. Zhang, Y. Tang, W. Huang, F. Min, J. He, Human activity recognition using wearable sensors by heterogeneous convolutional neural networks, Expert Syst. Appl., 198 (2022), 116764. https://doi.org/10.1016/j.eswa.2022.116764 doi: 10.1016/j.eswa.2022.116764
![]() |
[31] |
K. Wang, J. He, L. Zhang, Sequential weakly labeled multiactivity localization and recognition on wearable sensors using recurrent attention networks, IEEE T. Hum-Mach. Syst., 51 (2021), 355–364. https://doi.org/10.48550/arXiv.2004.05768 doi: 10.48550/arXiv.2004.05768
![]() |
1. | Logapriya E, Surendran R, 2023, Knowledge Based Health Nutrition Recommendations for During Menstrual Cycle, 979-8-3503-0085-7, 639, 10.1109/ICSSAS57918.2023.10331838 | |
2. | Majdi Khalid, Sugitha Deivasigamani, Sathiya V, Surendran Rajendran, An efficient colorectal cancer detection network using atrous convolution with coordinate attention transformer and histopathological images, 2024, 14, 2045-2322, 10.1038/s41598-024-70117-y | |
3. | Saranya G, Tamilvizhi T, Tharun S V, Surendran R, 2023, Speech Emotion Recognition with High Accuracy and Large Datasets using Convolutional Neural Networks, 979-8-3503-9663-8, 859, 10.1109/ICCES57224.2023.10192891 | |
4. | D. P. Surya, K. Ishwarya, K. G. Rani, N. Leema, R. Surendran, S. Raveena, C. Y. Lau, 2024, 3161, 0094-243X, 020302, 10.1063/5.0229272 | |
5. | Venkatraman M, Surendran R, 2023, Industrial 5.0 Aquaponics System Using Machine Learning Techniques, 979-8-3503-4060-0, 835, 10.1109/ICECA58529.2023.10395500 | |
6. | Youseef Alotaibi, Veera Ankalu. Vuyyuru, Electroencephalogram based face emotion recognition using multimodal fusion and 1-D convolution neural network (ID-CNN) classifier, 2023, 8, 2473-6988, 22984, 10.3934/math.20231169 | |
7. | S. V. Tharun, G. Saranya, T. Tamilvizhi, R. Surendran, 2023, Chapter 10, 978-3-031-44083-0, 95, 10.1007/978-3-031-44084-7_10 | |
8. | Youseef Alotaibi, Arun Mozhi Selvi Sundarapandi, Subhashini P, Surendran Rajendran, Computational linguistics based text emotion analysis using enhanced beetle antenna search with deep learning during COVID-19 pandemic, 2023, 9, 2376-5992, e1714, 10.7717/peerj-cs.1714 | |
9. | S Raveena, R Surendran, 2023, Recommending the Right Biofertilizer Using Deep Collaborative Matrix Factorization in the Coffee Plantation, 979-8-3503-4060-0, 476, 10.1109/ICECA58529.2023.10395096 | |
10. | Logapriya E, Surendran R, 2024, Combination of Food Nutrition Recommend for Women Healthcare at Menstrual Bleeding, 979-8-3503-7999-0, 1438, 10.1109/ICSCSS60660.2024.10624744 | |
11. | Joypriyanka Mariselvam, Surendran Rajendran, Youseef Alotaibi, Reinforcement learning-based AI assistant and VR play therapy game for children with Down syndrome bound to wheelchairs, 2023, 8, 2473-6988, 16989, 10.3934/math.2023867 | |
12. | Weihao Zhang, Bojian Chen, Zhuolei Chen, Zhezhou Li, Tengfei Han, Wenbin Wu, Xiaoqiang Zhang, Optimal path planning for unmanned aerial vehicles in power line inspection in rechargeable scenario, 2024, 0954-4054, 10.1177/09544054241289460 | |
13. | Raveena Selvanarayanan, Surendran Rajendran, Sameer Algburi, Osamah Ibrahim Khalaf, Habib Hamam, Empowering coffee farming using counterfactual recommendation based RNN driven IoT integrated soil quality command system, 2024, 14, 2045-2322, 10.1038/s41598-024-56954-x | |
14. | Madhusundar Nelson, Surendran Rajendran, Youseef Alotaibi, Vision graph neural network-based neonatal identification to avoid swapping and abduction, 2023, 8, 2473-6988, 21554, 10.3934/math.20231098 | |
15. | Joypriyanka M, Surendran R, 2023, Reinforcement Learning in Supermarket Tycoon to Enhance Multitasking and Problem-Solving in Autistic Children, 979-8-3503-0777-1, 234, 10.1109/3ICT60104.2023.10391564 | |
16. | Venkatraman M, Surendran R, 2023, Design and Implementation of Smart Hydroponics Farming for Manufacture using Novel Genetic Algorithm, 979-8-3503-9663-8, 1367, 10.1109/ICCES57224.2023.10192762 | |
17. | Sakorn Mekruksavanich, Wikanda Phaphan, Narit Hnoohom, Anuchit Jitpattanakul, Attention-Based Hybrid Deep Learning Network for Human Activity Recognition Using WiFi Channel State Information, 2023, 13, 2076-3417, 8884, 10.3390/app13158884 | |
18. | Sridharan S, Sugitha Deivasigamani, Rajesh R, Surendran R, 2024, Enhancing Healthcare Through AI Deep Learning: A Human-Centric IoT Advisory System Cloud, 979-8-3503-7999-0, 346, 10.1109/ICSCSS60660.2024.10625451 | |
19. | Raveena Selvanarayanan, Surendran Rajendran, 2023, Roaming the Coffee Plantations Using Grey Wolves Optimisation and the Restricted Boltzmann Machine to Predict Coffee Berry Disease, 979-8-3503-0085-7, 681, 10.1109/ICSSAS57918.2023.10331629 | |
20. | Madhusundar Nelson, Surendran Rajendran, Osamah Ibrahim Khalaf, Habib Hamam, Deep-learning-based intelligent neonatal seizure identification using spatial and spectral GNN optimized with the Aquila algorithm, 2024, 9, 2473-6988, 19645, 10.3934/math.2024958 | |
21. | Youseef Alotaibi, R Deepa, K Shankar, Surendran Rajendran, Inverse chi-square-based flamingo search optimization with machine learning-based security solution for Internet of Things edge devices, 2024, 9, 2473-6988, 22, 10.3934/math.2024002 | |
22. | Madhusundar Nelson, Surendran Rajendran, 2023, Deep Learning-Based Intelligent Model for Neonatal Cyanosis Identification Using SSGNN, 979-8-3503-0777-1, 282, 10.1109/3ICT60104.2023.10391661 | |
23. | Ajay Kumar CH, Surendran R, Madhusundar N, 2023, Fake News Classification in Twitter Data Using Innovative K Nearest Neighbor Comparing Logistics Regression, 979-8-3503-9458-0, 1, 10.1109/ICCEBS58601.2023.10449101 | |
24. | Logapriya E, Surendran R, 2023, Hybrid Recommendations System for Women Health Nutrition at Menstruation Cycle, 979-8-3503-0777-1, 357, 10.1109/3ICT60104.2023.10391518 | |
25. | S Madhan, S Sridharan, Sugitha Deivasigamani, R Rajesh, R Surendran, 2024, Facial Expression Analysis using K-Nearest Neighbor Classification Method: Enhancing Emotion Detection and Stress Monitoring in an Interactive Music Player, 979-8-3315-0440-3, 1316, 10.1109/ICOSEC61587.2024.10722685 | |
26. | Khushi Bawistale, Surendran R, 2024, Macqueens Based Yoga Recommendation System for First Trimester Pregnancy, 979-8-3503-8435-2, 552, 10.1109/ICACCS60874.2024.10717010 | |
27. | Ajitha P, Sadish Sendi M, Sundara Rajulu Navaneethakrishnan, Sivasangari A, Surendran R, 2024, Multibio Authentication of Online Users in Proctoring and E-Learning, 979-8-3503-6908-3, 1, 10.1109/ICEEICT61591.2024.10718417 | |
28. | R Naveen Kumar, R Surendran, N Madhusundar, 2024, An Intelligent Human Pose Detection Comparing with Random forest and Recurrent Neural Network, 979-8-3503-6790-4, 915, 10.1109/ICECA63461.2024.10800936 | |
29. | Deepa R, Jayalakshmi V, Thilakavathy P, Manikandan G, Surendran R, 2024, Custom Transformer-Based Approach for Enhanced Bengali Automatic Speech Recognition, 979-8-3503-7651-7, 1, 10.1109/ICICEC62498.2024.10808418 | |
30. | Mahaveerakannan R, Sreerengan V R Nair, Rohini M. S, Vinoth Kumar M, B. Rajakumar, 2024, A Deep Learning Application for Identifying Cavities in Dentistry: Utilizing Smartphone-Taken Intraoral Photos, 979-8-3315-3001-3, 718, 10.1109/ICSCNA63714.2024.10863875 | |
31. | Logapriya E, Surendran R, Poornima D, Kartheesan L, 2024, Optimized Deep Learning Framework for Personalized Nutritional Recommendations Across the Menstrual Cycle, 979-8-3315-3001-3, 1078, 10.1109/ICSCNA63714.2024.10864015 | |
32. | E. Logapriya, Surendran Rajendran, Mohammad Zakariah, Hybrid Greylag Goose deep learning with layered sparse network for women nutrition recommendation during menstrual cycle, 2025, 15, 2045-2322, 10.1038/s41598-025-88728-4 | |
33. | Youseef Alotaibi, B. Rajasekar, R. Jayalakshmi, Surendran Rajendran, Falcon Optimization Algorithm-Based Energy Efficient Communication Protocol for Cluster-Based Vehicular Networks, 2024, 78, 1546-2226, 4243, 10.32604/cmc.2024.047608 | |
34. | Tamilvizhi Thanarajan, Youseef Alotaibi, Surendran Rajendran, Krishnaraj Nagappan, Eye-Tracking Based Autism Spectrum Disorder Diagnosis Using Chaotic Butterfly Optimization with Deep Learning Model, 2023, 76, 1546-2226, 1995, 10.32604/cmc.2023.039644 | |
35. | Raveena Selvanarayanan, Surendran Rajendran, Youseef Alotaibi, Early Detection of Colletotrichum Kahawae Disease in Coffee Cherry Based on Computer Vision Techniques, 2024, 139, 1526-1506, 759, 10.32604/cmes.2023.044084 |
UCI HAR | ||
Label | Class | No. of Instances |
0 | Walking | 1722 |
1 | Moving upward | 1444 |
2 | Moving downward | 1506 |
3 | Sitting | 1877 |
4 | Standing | 1808 |
5 | Lying | 1942 |
Total No. of Instances | 10299 |
Labels | Accuracy | Sensitivity | Specificity | F-Score | ROC Score | MCC |
Training Phase (60%) | ||||||
0 | 99.43 | 98.27 | 99.67 | 98.32 | 98.97 | 97.98 |
1 | 99.51 | 98.18 | 99.75 | 98.39 | 98.97 | 98.11 |
2 | 99.56 | 98.58 | 99.72 | 98.40 | 99.15 | 98.15 |
3 | 99.40 | 98.64 | 99.55 | 98.21 | 99.10 | 97.86 |
4 | 99.24 | 97.60 | 99.62 | 97.98 | 98.61 | 97.51 |
5 | 99.64 | 99.14 | 99.76 | 99.05 | 99.45 | 98.83 |
Average | 99.47 | 98.40 | 99.68 | 98.39 | 99.04 | 98.07 |
Testing Phase (40%) | ||||||
0 | 99.15 | 96.61 | 99.65 | 97.40 | 98.13 | 96.90 |
1 | 99.42 | 97.70 | 99.72 | 98.02 | 98.71 | 97.68 |
2 | 99.64 | 98.40 | 99.83 | 98.66 | 99.11 | 98.45 |
3 | 99.49 | 99.33 | 99.53 | 98.60 | 99.43 | 98.30 |
4 | 99.25 | 97.69 | 99.59 | 97.89 | 98.64 | 97.43 |
5 | 99.42 | 99.11 | 99.49 | 98.48 | 99.30 | 98.13 |
Average | 99.39 | 98.14 | 99.63 | 98.18 | 98.89 | 97.81 |
Labels | Accuracy | Sensitivity | Specificity | F-Score | ROC Score | MCC |
Training Phase (70%) | ||||||
0 | 99.54 | 98.75 | 99.70 | 98.63 | 99.23 | 98.35 |
1 | 99.40 | 98.32 | 99.59 | 98.00 | 98.96 | 97.65 |
2 | 99.49 | 97.82 | 99.74 | 98.07 | 98.78 | 97.78 |
3 | 99.68 | 98.71 | 99.88 | 99.07 | 99.30 | 98.88 |
4 | 99.64 | 98.87 | 99.81 | 99.02 | 99.34 | 98.80 |
5 | 99.53 | 99.07 | 99.64 | 98.79 | 99.36 | 98.50 |
Average | 99.55 | 98.59 | 99.73 | 98.60 | 99.16 | 98.33 |
Testing Phase (30%) | ||||||
0 | 99.68 | 98.85 | 99.84 | 99.04 | 99.35 | 98.85 |
1 | 99.61 | 98.72 | 99.77 | 98.72 | 99.25 | 98.49 |
2 | 99.42 | 97.07 | 99.81 | 97.95 | 98.44 | 97.62 |
3 | 99.39 | 98.70 | 99.53 | 98.25 | 99.12 | 97.87 |
4 | 99.71 | 99.30 | 99.80 | 99.22 | 99.55 | 99.04 |
5 | 99.74 | 99.63 | 99.76 | 99.26 | 99.70 | 99.11 |
Average | 99.59 | 98.71 | 99.75 | 98.74 | 99.23 | 98.50 |
Methods | Accuracy | Sensitivity | Specificity |
IWSODL-MAHAR | 99.59 | 98.71 | 99.75 |
CNN Model | 96.88 | 97.64 | 98.19 |
Ensemble of AE Model | 80.80 | 81.36 | 82.00 |
SVM Model | 91.12 | 91.75 | 92.38 |
ConvAE-LSTM | 98.67 | 98.27 | 98.91 |
ReliefF Model | 96.71 | 97.49 | 98.14 |
Hybrid GBO-GWO | 98.52 | 97.90 | 98.11 |
UCI HAR | ||
Label | Class | No. of Instances |
0 | Walking | 1722 |
1 | Moving upward | 1444 |
2 | Moving downward | 1506 |
3 | Sitting | 1877 |
4 | Standing | 1808 |
5 | Lying | 1942 |
Total No. of Instances | 10299 |
Labels | Accuracy | Sensitivity | Specificity | F-Score | ROC Score | MCC |
Training Phase (60%) | ||||||
0 | 99.43 | 98.27 | 99.67 | 98.32 | 98.97 | 97.98 |
1 | 99.51 | 98.18 | 99.75 | 98.39 | 98.97 | 98.11 |
2 | 99.56 | 98.58 | 99.72 | 98.40 | 99.15 | 98.15 |
3 | 99.40 | 98.64 | 99.55 | 98.21 | 99.10 | 97.86 |
4 | 99.24 | 97.60 | 99.62 | 97.98 | 98.61 | 97.51 |
5 | 99.64 | 99.14 | 99.76 | 99.05 | 99.45 | 98.83 |
Average | 99.47 | 98.40 | 99.68 | 98.39 | 99.04 | 98.07 |
Testing Phase (40%) | ||||||
0 | 99.15 | 96.61 | 99.65 | 97.40 | 98.13 | 96.90 |
1 | 99.42 | 97.70 | 99.72 | 98.02 | 98.71 | 97.68 |
2 | 99.64 | 98.40 | 99.83 | 98.66 | 99.11 | 98.45 |
3 | 99.49 | 99.33 | 99.53 | 98.60 | 99.43 | 98.30 |
4 | 99.25 | 97.69 | 99.59 | 97.89 | 98.64 | 97.43 |
5 | 99.42 | 99.11 | 99.49 | 98.48 | 99.30 | 98.13 |
Average | 99.39 | 98.14 | 99.63 | 98.18 | 98.89 | 97.81 |
Labels | Accuracy | Sensitivity | Specificity | F-Score | ROC Score | MCC |
Training Phase (70%) | ||||||
0 | 99.54 | 98.75 | 99.70 | 98.63 | 99.23 | 98.35 |
1 | 99.40 | 98.32 | 99.59 | 98.00 | 98.96 | 97.65 |
2 | 99.49 | 97.82 | 99.74 | 98.07 | 98.78 | 97.78 |
3 | 99.68 | 98.71 | 99.88 | 99.07 | 99.30 | 98.88 |
4 | 99.64 | 98.87 | 99.81 | 99.02 | 99.34 | 98.80 |
5 | 99.53 | 99.07 | 99.64 | 98.79 | 99.36 | 98.50 |
Average | 99.55 | 98.59 | 99.73 | 98.60 | 99.16 | 98.33 |
Testing Phase (30%) | ||||||
0 | 99.68 | 98.85 | 99.84 | 99.04 | 99.35 | 98.85 |
1 | 99.61 | 98.72 | 99.77 | 98.72 | 99.25 | 98.49 |
2 | 99.42 | 97.07 | 99.81 | 97.95 | 98.44 | 97.62 |
3 | 99.39 | 98.70 | 99.53 | 98.25 | 99.12 | 97.87 |
4 | 99.71 | 99.30 | 99.80 | 99.22 | 99.55 | 99.04 |
5 | 99.74 | 99.63 | 99.76 | 99.26 | 99.70 | 99.11 |
Average | 99.59 | 98.71 | 99.75 | 98.74 | 99.23 | 98.50 |
Methods | Accuracy | Sensitivity | Specificity |
IWSODL-MAHAR | 99.59 | 98.71 | 99.75 |
CNN Model | 96.88 | 97.64 | 98.19 |
Ensemble of AE Model | 80.80 | 81.36 | 82.00 |
SVM Model | 91.12 | 91.75 | 92.38 |
ConvAE-LSTM | 98.67 | 98.27 | 98.91 |
ReliefF Model | 96.71 | 97.49 | 98.14 |
Hybrid GBO-GWO | 98.52 | 97.90 | 98.11 |