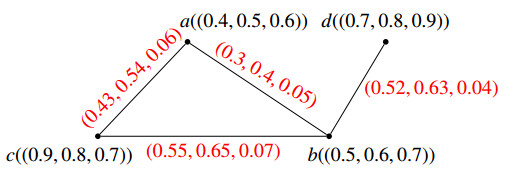
Utilizing two ideas of neutrosophic subsets (NS) and triangular norms, we introduce a new type of graph as valued-inverse Dombi neutrosophic graphs. The valued-inverse Dombi neutrosophic graphs are a generalization of inverse neutrosophic graphs and are dual to Dombi neutrosophic graphs. We present the concepts of (complete) strong valued-inverse Dombi neutrosophic graphs and analyze the complement of (complete) strong valued-inverse Dombi neutrosophic graphs and self-valued complemented valued-inverse Dombi neutrosophic graphs. Since the valued-inverse Dombi neutrosophic graphs depend on real values, solving the non-equation and the concept of homomorphism play a prominent role in determining the complete, strong, complementarity and self-complementarity of valued-inverse Dombi neutrosophic graphs. We introduce the truth membership order, indeterminacy membership order, falsity membership order, truth membership size, indeterminacy membership size and indeterminacy membership size of any given valued-inverse Dombi neutrosophic graph, which play a major role in the application of valued inverse Dombi neutrosophic graphs in complex networks. An application of a valued-inverse Dombi neutrosophic graph is also described in this study.
Citation: Mohammad Hamidi, Florentin Smarandache. Valued-inverse Dombi neutrosophic graph and application[J]. AIMS Mathematics, 2023, 8(11): 26614-26631. doi: 10.3934/math.20231361
[1] | Talha Midrar, Saifullah Khan, Saleem Abdullah, Thongchai Botmart . Entropy based extended TOPOSIS method for MCDM problem with fuzzy credibility numbers. AIMS Mathematics, 2022, 7(9): 17286-17312. doi: 10.3934/math.2022952 |
[2] | Muhammad Qiyas, Neelam Khan, Muhammad Naeem, Saleem Abdullah . Intuitionistic fuzzy credibility Dombi aggregation operators and their application of railway train selection in Pakistan. AIMS Mathematics, 2023, 8(3): 6520-6542. doi: 10.3934/math.2023329 |
[3] | Shichao Li, Zeeshan Ali, Peide Liu . Prioritized Hamy mean operators based on Dombi t-norm and t-conorm for the complex interval-valued Atanassov-Intuitionistic fuzzy sets and their applications in strategic decision-making problems. AIMS Mathematics, 2025, 10(3): 6589-6635. doi: 10.3934/math.2025302 |
[4] | Muhammad Qiyas, Talha Madrar, Saifullah Khan, Saleem Abdullah, Thongchai Botmart, Anuwat Jirawattanapaint . Decision support system based on fuzzy credibility Dombi aggregation operators and modified TOPSIS method. AIMS Mathematics, 2022, 7(10): 19057-19082. doi: 10.3934/math.20221047 |
[5] | Tahir Mahmood, Ahmad Idrees, Majed Albaity, Ubaid ur Rehman . Selection of artificial intelligence provider via multi-attribute decision-making technique under the model of complex intuitionistic fuzzy rough sets. AIMS Mathematics, 2024, 9(11): 33087-33138. doi: 10.3934/math.20241581 |
[6] | D. Ajay, P. Chellamani, G. Rajchakit, N. Boonsatit, P. Hammachukiattikul . Regularity of Pythagorean neutrosophic graphs with an illustration in MCDM. AIMS Mathematics, 2022, 7(5): 9424-9442. doi: 10.3934/math.2022523 |
[7] | Shama Liaqat, Zeeshan Saleem Mufti, Yilun Shang . Newly defined fuzzy Misbalance Prodeg Index with application in multi-criteria decision-making. AIMS Mathematics, 2024, 9(8): 20193-20220. doi: 10.3934/math.2024984 |
[8] | Muhammad Zeeshan Hanif, Naveed Yaqoob, Muhammad Riaz, Muhammad Aslam . Linear Diophantine fuzzy graphs with new decision-making approach. AIMS Mathematics, 2022, 7(8): 14532-14556. doi: 10.3934/math.2022801 |
[9] | Ali Al Khabyah, Haseeb Ahmad, Ali Ahmad, Ali N. A. Koam . A uniform interval-valued intuitionistic fuzzy environment: topological descriptors and their application in neural networks. AIMS Mathematics, 2024, 9(10): 28792-28812. doi: 10.3934/math.20241397 |
[10] | Zeeshan Saleem Mufti, Ali Tabraiz, Qin Xin, Bander Almutairi, Rukhshanda Anjum . Fuzzy topological analysis of pizza graph. AIMS Mathematics, 2023, 8(6): 12841-12856. doi: 10.3934/math.2023647 |
Utilizing two ideas of neutrosophic subsets (NS) and triangular norms, we introduce a new type of graph as valued-inverse Dombi neutrosophic graphs. The valued-inverse Dombi neutrosophic graphs are a generalization of inverse neutrosophic graphs and are dual to Dombi neutrosophic graphs. We present the concepts of (complete) strong valued-inverse Dombi neutrosophic graphs and analyze the complement of (complete) strong valued-inverse Dombi neutrosophic graphs and self-valued complemented valued-inverse Dombi neutrosophic graphs. Since the valued-inverse Dombi neutrosophic graphs depend on real values, solving the non-equation and the concept of homomorphism play a prominent role in determining the complete, strong, complementarity and self-complementarity of valued-inverse Dombi neutrosophic graphs. We introduce the truth membership order, indeterminacy membership order, falsity membership order, truth membership size, indeterminacy membership size and indeterminacy membership size of any given valued-inverse Dombi neutrosophic graph, which play a major role in the application of valued inverse Dombi neutrosophic graphs in complex networks. An application of a valued-inverse Dombi neutrosophic graph is also described in this study.
The fuzzy graph theory, as a generalization of graph theory, is a symmetric and binary fuzzy relation on the fuzzy subset and is introduced and extended by Kaufmann and Rosenfeld [13,18]. Later on, some researchers extended this concept, such as the complement of fuzzy graphs, the notions of regular fuzzy graphs, the notation of strong fuzzy graphs, totally regular fuzzy graphs and degree and total degree of a vertex in some fuzzy graphs, the novel concepts of M-strong fuzzy graphs and some types on domination number based on fuzzy graphs. Smarandache has presented the central concept idea of the neutrosophic set by extending the idea of fuzzy subsets [21]. A neutrosophic subset considers vague, indeterminate and inconsistent information of any real-life problem. A neutrosophic subset has three independent membership grades: truth, indeterminate and false. The bipolar fuzzy graph that is introduced by Ghorai and Pal is based on two ideas of fuzzy subset and graph structure and is used to design real-life problems [9]. The theory of single-valued neutrosophic graphs is a generalization of fuzzy graphs and has some essential applications in the real world. M. Akram has collected and has introduced a book on the scope of single-valued neutrosophic graphs that presents readers with fundamental concepts, including single-valued neutrosophic, neutrosophic graph structures, bipolar neutrosophic graphs, domination in bipolar neutrosophic graphs, bipolar neutrosophic planar graphs, interval-valued neutrosophic graphs, interval-valued neutrosophic graph structures, rough neutrosophic digraphs, neutrosophic rough digraphs, neutrosophic soft graphs, and intuitionistic neutrosophic soft graphs [2]. Recently, many researchers have more actively worked on neutrosophic graph theory, for instance, certain properties of the single-valued neutrosophic graph with application in food and agriculture organization [22], bipolar neutrosophic graph structures [6], a note on different types of product of neutrosophic graphs [15], generalized neutrosophic planar graphs and its application [16] and regularity of Pythagorean neutrosophic graphs with an illustration in MCDM [5]. The theory of single-valued neutrosophic hypergraphs as a generalization of single-valued neutrosophic graphs is a novel concept in the scope of single-valued neutrosophics and hypergraphs, which is introduced by M. Akram [1]. There is some applied research in the scope of single-valued neutrosophic hypergraphs, which are valuable in the real world, such as the implementation of single-valued neutrosophic soft hypergraphs on the human nervous system [3], and algorithms for the computation of regular single-valued neutrosophic soft hypergraphs applied to supranational Asian bodies [4]. A study on generalized graphs representations of complex neutrosophic information [19] and a novel decision-making method based on bipolar neutrosophic information [23] was conducted. The combination of triangular norms and fuzzy graphs is a novel concept for the generalization of fuzzy graphs and has applications of triangular norms in the real world. Recently, Ashraf et. al introduced the concept of Dombi fuzzy graphs and investigated some of their properties [7]. Later, Borzooei et al. presented the concept of inverse fuzzy graphs with application and analyzed the difference between fuzzy graphs and inverse fuzzy graphs [8]. Hamidi et al. applied the notation of topological spaces and fuzzy metrizable as the class of metrizable-topological spaces and introduced the concept of KM-fuzzy metric graph and KM-fuzzy metric hypergraph [11,12]. Pal et al. introduced the combination of triangular norms and neutrosophic graphs as a generalization of the combination of triangular norms and fuzzy graphs, as some operations on Dombi neutrosophic graph, such as union, intersection composition, cartesian product, box dot product, homomorphic product, and modular product [14].
Motivation and advantage: One of the most essential applications of mathematics in the real world is to model a real problem based on the concepts of mathematics to analyze it according to the logic governing the problem. Undoubtedly, graph theory is one of the essential branches of mathematics in this modeling, which makes it easy to solve problems. Due to the fact that real problems are based on weight and real labels, weighted graphs can be essential. One of the essential areas of mathematics is fuzzy graphs, which can be effective in this field. Due to the strong connections of the elements in the real world to each other and the various properties and characteristics of objects, fuzzy graphs alone cannot analyze all the problems, and therefore, this limitation is considered in this field. Therefore, the concept of inverse single-valued neutrosophic graphs can overcome this limitation and cover different characteristics. As an example, consider a social network whose members are humans. In this network, every human being has a different temperament, and therefore, good, bad, kindness, anger, and similar (the truth value of each person represents his positive influence on others, falsity value represents his negative influence on others and indeterminacy value represents uncertainty in his influence) traits can have a significant impact on this network. Our most essential motivation in this research is based on the fact that in a mixed network that has several essential characteristics and some properties that act opposite to other properties, we model them based on inverse single-valued neutrosophic graphs. In this modeling, we deal with the reverse effect of each characteristic and examine its effect. Regarding these points, we try to combine the notations of triangular norms and neutrosophic subsets to construct an extension of graphs. Thus, we apply the notation of the Dombi triangular norm and inverse fuzzy graph to introduce the Dombi inverse neutrosophic graph. The prominent motivation for this work is a type of generalization of crisp graphs to weighted graphs, which are essential in the real world. Indeed, for any given greater than or equal to one nonnegative real number, a valued Dombi inverse neutrosophic graph is introduced. Additionally, we investigated the properties of valued Dombi inverse neutrosophic graphs and an application of the Dombi neutrosophic graph is also described in this manuscript.
In this section, we recall some definitions and results, which we need use in what follows.
Definition 2.1. [17] A binary operation T:[0,1]×[0,1]→[0,1] is a t-norm, if for all x,y,z,w∈[0,1]:
(i)T(1,x)=x,
(ii)T(x,y)=T(y,x),
(iii)T(T(x,y),z)=T(x,T(y,z)),
(iii) if w≤x and y≤z, then T(w,y)≤T(x,z).
For any x,y∈[0,1], the t-norm TDo(x,y)=xyx+y−xy is called the Dombi t-norm as an especial of Hamacher family of t-norms.
Definition 2.2. [20] Let V be a universal set. A neutrosophic subset (NS)X in V is defined by X={(x,TX(x),IX(x),FX(x))|x∈V,0≤TX(x)+IX(x)+FX(x)≤3}, which X:V→[0,1]×[0,1]×[0,1] is characterized by a truth-membership function TX, an indeterminacy-membership function IX and a falsity-membership function FX.
Definition 2.3. [2] A single-valued neutrosophic graph on a nonempty X is a pair G=(A,B), where A is single-valued neutrosophic set in X and B single-valued neutrosophic relation on X, such that TB(xy)≤min{TA(x),TA(y)},IB(xy)≤min{IA(x),IA(y)},FB(xy)≤max{FA(x),FA(y)} for all x,y∈X. A is called single-valued neutrosophic vertex set of G and B is called single-valued neutrosophic edge set of G, respectively.
Definition 2.4. [10] Let k∈R>0,G∗=(V,E) be a simple graph, σ:V→[0,1] and μ:V×V→[0,1] be fuzzy subsets. Then G=(σ,μ,TDo) is called a k-Dombi inverse fuzzy graph, if for any xy∈E, get kμ(xy)≥TD(σ(x),σ(y)). If k=1, then G=(σ,μ,TDo) is called a Dombi inverse fuzzy graph.
Definition 2.5. [14] Let G∗=(V,E) be a simple graph, σ={(x,TV(x),IV(x),FV(x))|x∈V} and μ={(xy,TE(xy),IE(xy),FE(xy))|xy∈E} be two NS. Then G=(σ,μ,TDo) is called a Dombi neutrosophic graph (DNG), if for any xy∈E, get TE(xy)≤TDo(TV(x),TV(y)),IE(xy)≤TDo(IV(x),IV(y)) and FE(xy)≥TDo(FV(x),FV(y)).
In this section, for any k,l,m∈R>0, introduce the concept of (k,l,m)-Dombi inverse neutrosophic graph, via Dombi triangular norm and inverse neutrosophic graph.
Definition 3.1. Let k,l,m∈R>0,G∗=(V,E) be a simple graph, σ={(x,TV(x),IX(x),FV(x))|x∈V} and μ={(xy,TE(xy),IE(xy),FE(xy))|xy∈E} be two NS. Then
(i)G=(σ,μ,TDo) is called a (k,l,m)-Dombi inverse neutrosophic graph ((k,l,m)-DING), if for any xy∈E, get kTE(xy)≥TDo(TV(x),TV(y)),lIE(xy)≥TDo(IV(x),IV(y)) and mFE(xy)≤TDo(FV(x),FV(y)),
(ii)G=(σ,μ,TDo) is called a strong (k,l,m)-Dombi inverse neutrosophic graph (strong (k,l,m)-DING), if for any xy∈E, kTE(xy)=TDo(TV(x),TV(y)),lIE(xy)=TDo(IV(x),IV(y)) and mFE(xy)=TDo(FV(x),FV(y)),
(iii)G=(σ,μ,TDo) is called a complete (k,l,m)-Dombi neutrosophic graph (complete (k,l,m)-DING), if for any x,y∈V, kTE(xy)=TDo(TV(x),TV(y)),lIE(xy)=TDo(IV(x),IV(y)) and mFE(xy)=TDo(FV(x),FV(y)).
In the above definition, if (k,l,m)=(1,1,1), we will call G=(σ,μ,TDo) is a (complete) strong Dombi inverse neutrosophic graph.
Example 3.2. Let V={a,b,c,d}. Then G=(σ,μ,TDo) is a (4,3,2)-DING as shown in Figure 1.
Theorem 3.3. Let G=(σ,μ,TDo) be a (k,l,m)-DING and k,k′,l,l′,m,m′∈R>0. If k′≥k,l′≥l,m≥m′, then G=(σ,μ,TDo) is a (k′,l′,m′)-DING.
Proof. Let k,k,′l,l′,m,m′∈R>0. Since G=(σ,μ,TDo) is a (k,l,m)-DING and k′≥k,l′≥l,m≥m′, we get that
k′TE(xy)≥kTE(xy)≥TDo(TV(x),TV(y)),l′IE(xy)≥lIE(xy)≥IDo(IV(x),IV(y))andm′FE(xy)≤mFE(xy)≤TDo(FV(x),FV(y)), |
and so G=(σ,μ,TDo) is a (k′,l′,m′)-DING.
Proposition 3.4. Let G=(σ,μ,TDo) be a DNG and a (k,l,m)-DING. Then
(i)k≥1,l≥1 and 0<m≤1.
(ii)G=(σ,μ,TDo) is strong DNG and strong (k,l,m)-DING if and only if (k,l,m)=(1,1,1).
Proof. (i) Let x,y∈V. Then
1kTDo(TV(x),TV(y))≤TE(xy)≤TDo(TV(x),TV(x)),1lTDo(IV(x),IV(y))≤IE(xy)≤TDo(IV(x),IV(x)),and1mTDo(FV(x),FV(y))≥FE(xy)≥TDo(FV(x),FV(x)). |
It follows that
1kTDo(TV(x),TV(y))≤TDo(TV(x),TV(x)),1lTDo(IV(x),IV(y))≤TDo(IV(x),IV(x)),and1mTDo(FV(x),FV(y))≥TDo(IV(x),IV(x)). |
Hence k≥1,l≥1 and m≤1, because of 0<x,y≤1.
(ii) It is clear by (i).
Definition 3.5. Let G=(σ,μ,TDo) be a (k,l,m)-DING and k,k,′l,l′,m,m′∈R>0. Then G(c,(k′,l′,m′))=(σc,μc,TDo) is called a (k′,l′,m′)-complement of (k,l,m)-DING of G, if σc=σ and for all x,y∈V,
TcE(xy)={k′TE(xy)−TDo(TV(x),TV(y))ifxy∈ETDo(TV(x),TV(y))ifxy∉E,, |
IcE(xy)={l′IE(xy)−TDo(IV(x),IV(y))ifxy∈ETDo(IV(x),IV(y))ifxy∉E, |
and FcE(xy)={FE(xy)−m′TDo(FV(x),FV(y))ifxy∈ETDo(FV(x),FV(y))ifxy∉E,, where μc=(TcE,IcE,FcE) and σc=(TcV,IcV,FcV).
Theorem 3.6. Let G=(σ,μ,TDo) be a (k,l,m)-DING and k,l,m,k′,l′,m′∈R>0. Then
(i)G(c,(k′,l′,m′))=(σc,μc,TDo) is a (kk′−k,ll′−l,m(1−mm′))-DING.
(ii)G(c,(2k,2l,1−mm))=(σc,μc,TDo) is a DING.
(iii) If G=(σ,μ,TDo) is strong, then G(c,(2k,2l,1−mm))=(σc,μc,TDo) is strong.
Proof. (i) Let xy∈E. Then
TcE(xy)=k′TE(xy)−TDo(TV(x),TV(y))≥k′kTDo(TV(x),TV(y))−TDo(TV(x),TV(y))≥(k′k−1)TDo(TV(x),TV(y)),IcE(xy)=l′IE(xy)−TDo(IV(x),IV(y))≥l′lTDo(IV(x),IV(y))−TDo(IV(x),IV(y))≥(l′l−1)TDo(IV(x),IV(y))andFcE(xy)=FE(xy)−m′TDo(FV(x),FV(y))≤(1−mm′)FE(xy)≤(1−mm′)mTDo(FV(x),FV(y)). |
It follows that
kk′−kTcE(xy)≥TDo(TV(x),TV(y)),ll′−lIcE(xy)≥TDo(IV(x),IV(y)),m(1−mm′)FcE(xy)≤TDo(FV(x),FV(y)), |
and so G(c,(k′,l′,m′))=(σc,μc,TDo) is a (kk′−k,ll′−l,m(1−mm′))-DING.
(ii) Since 2k=k′,2l=l′,m′=1−mm, by (i), G(c,(2k,2l,1−mm))=(σc,μc,TDo) is a DING.
(iii) It is clear by (i),(ii).
Corollary 3.7. Let G=(σ,μ,TDo) be a (k,l,m)-DING and k,l,m∈R>0. Then
(i)G(c,(k,l,m))=(σc,μc,TDo) is't a DING.
(ii)G(c,(k′,l′,m′))=(σc,μc,TDo) is't a DING, which k′<k,l′<l,m′>1m.
Example 3.8. Let V={a,b,c,d}. Then G=(σ,μ,TDo) is a (2,3,4)-DING as shown in Figure 2 (A). Then G(c,(4,5,1100))=(σc,μc,TDo) is the (4,5,1100)-complement of (2,3,4)-DING G in Figure 2 (B).
Definition 3.9. Let k,k′,k″,m,m′,m″,l,l′,l″∈R>0,G=(σ,μ,TDo) and G′=(σ′,μ′,TDo) be (k,l,m)-DING and (k′,l′,m′)-DING on simple graph G∗=(V,E) and G′∗=(V′,E′), respectively.
(i) A homomorphism h:G→G′ is a map h:V→V′, which for all x∈V, TV(x)≥T′V(h(x)),IV(x)≥I′V(h(x)),FV(x)≥F′V(h(x)) and for all xy∈E, kTE(xy)≥k′T′E(h(x)h(y)),lIE(xy)≥l′T′E(h(x)h(y)),mFE(xy)≥m′F′(h(x)h(y)).
(ii) A bijective mapping h:V→V′ is called an isomorphism, if for any xy∈E and x∈V, TV(x)=T′V(h(x)),IV(x)=I′V(h(x)),FV(x)=F′V(h(x)) and for all xy∈E, kTE(xy)=k′T′E(h(x)h(y)),lIE(xy)=l′I′E(h(x)h(y)) and mFE(xy)=m′F′(h(x)h(y)). We will say that G=(σ,μ,TDo) and G′=(σ′,μ′,TDo) are isomorphic and will denote it by G≅G′.
(iii)G is called a self (k″,l″,m″)-complemented (k,l,m)-DING, if G≅G(c,(k″,l″,m″)).
Example 3.10. (i) Let V={a,b,c}. Then G1=(σ1,μ1,TDo)≅G2=(σ2,μ2,TDo), where G1 is a (2,3,4)-DING and G2 is a (4,6,8)-DING as shown in Figure 3.
(ii)(i) Consider the (4,5,1)-DING G=(σ,μ,TDo) and (8,9,0.5)-DING Gc=(σc,μc,TDo) in Figures 4(A) and (B), respectively. Then G is a self (8,9,0.5)-complemented (4,5,1)-DING.
Theorem 3.11. Let k1,l1,m1,k2,l2,m2,k′1,l′,m′1,k′2,l′2,m′2∈R>0,G1=(σ1,μ1,TDo)≅G2=(σ2,μ2,TDo), where G1 and G2 are (k1,l1,m1)-DING and (k2,l2,m2)-DING, respectively. Then
(i) If k′1k2=k1k′2,l′1l2=l1l′2 and m1m′1=m2m′2, then G(c,(k′1,l′1,m′1))1≅G(c,(k′2,l′2,m′2))2.
(ii)G(c,(k1,l1,m1))1≅G(c,(k1,l1,m1))2.
(iii)G(c,(k1k′2k1,l1l′2k1,m1m′1m′2))1≅G(c,(k2,l2,m2))2.
Proof. (i) Let G1≅G2, so there exists h:V1→V2, such that for any x1∈V1 and for all x1y1∈E1, T1V(x1)=T2V(h(x1)),I1V(x1)=I2V(h(x1)),F1V(x1)=F2V(h(x1)) and k1T1E(x1y1)=k2T2E(h(x1)h(y1)),l1I1E(x1y1)=l2I2E(h(x1)h(y1)),m1F1E(x1y1)=m2F2E(h(x1)h(y1)). It's clear that Tc1V(x1)=Tc12V(h(x1)),Ic1V(x1)=Ic12V(h(x1)),Fc1V(x1)=Fc12V(h(x1)) for all x1∈V1. Let x1y1∈E1,T1E(x1y1)≠0,I1E(x1y1)≠0 and F1E(x1y1)≠0. By Theorem 3.6, G(c,(k′,l′,m′))=(σc,μc,TDo) is a (kk′−k,ll′−l,m(1−mm′))-DING. Thus,
k1k′1−k1Tc1E(x1y1)=(k1k′1−k1)k′1T1E(x1y1)−(k1k′1−k1)TDo(T1V(x1),T1V(y1))=(k1k′1−k1)k′1k2k1T2E(h(x1)h(y1))−(k1k′1−k1)TDo(T2V(h(x1)),T2V(h(y1))). |
Thus,
(k1k′1−k1)Tc1E(x1y1)=(k2k′2−k2)Tc2E(h(x1)h(y1))⇔(k1k′1−k1)k′1k2k1T2E(h(x1)h(y1))−(k1k′1−k1)TDo(T2V(h(x1)),T2V(h(y1)))=(k2k′2−k2)Tc2E(h(x1)h(y1))⇔(k1k′1−k1)k′1k2k1T2E(h(x1)h(y1))−(k1k′1−k1)TDo(T2V(h(x1)),T2V(h(y1)))=((k2k′2−k2)k′2T2E(h(x1)h(y1))−(k2k′2−k2)TDo(T2V(h(x1)),T2V(h(y1)))⇔k′1k2=k1k′2. |
Let x1y1∈E and T1E(x1y1)=0. Thus,
Tc1E(x1y1)=TDo(T1V(x1),T1V(y1))=TDo(T2V(h(x1)),T2V(h(y1)))=Tc2E(h(x1)h(y1)). |
In a similar way, (l1l′1−l1)Ic1E(x1y1)=(l2l′2−l2)Ic2E(h(x1)h(y1)) if and only if l′1l2=l1l′2. In addition,
m11−m1m′1Fc1E(x1y1)=m11−m1m′1F1E(x1y1)−(m11−m1m′1)m′1TDo(F1V(x1),F1V(y1))=(m11−m1m′1)m2m1F2E(h(x1)h(y1))−(m11−m1m′1)m′1TDo(F2V(h(x1)),F2V(h(y1))). |
Thus,
(m11−m1m′1)Fc1E(x1y1)=(m21−m2m′2)Fc2E(h(x1)h(y1))⇔(m11−m1m′1)m2m1F2E(h(x1)h(y1))−(m11−m1m′1)m′1TDo(F2V(h(x1)),F2V(h(y1)))=((m21−m2m′2)F2E(h(x1)h(y1))−(m21−m2m′2)m′2TDo(F2V(h(x1)),F2V(h(y1)))⇔m1m′1=m2m′2. |
Let x1y1∈E and F1E(x1y1)=0. Thus,
Fc1E(x1y1)=TDo(F1V(x1),F1V(y1))=TDo(F2V(h(x1)),F2V(h(y1)))=Fc2E(h(x1)h(y1)). |
(ii),(iii) are obtained from (i).
Theorem 3.12. Let G=(σ,μ,TDo) be a self (k′,l′,m′)-complemented of strong (k,l,m)-DING on G∗=(V,E). Then
(i)∑x≠yxy∈ETE(xy)=(k′2−kk′k2)∑x≠yTDo(TV(x),TV(y)).
(ii)∑x≠yxy∈EIE(xy)=(l′2−ll′k2)∑x≠yTDo(IV(x),IV(y)).
(iii)∑x≠yxy∈EFE(xy)=(m′−mm′2m2)∑x≠yTDo(FV(x),FV(y)).
(iv)k′>k,l′>l and mm′<1.
Proof. (i),(ii),(iii) Let G=(σ,μ,TDo) be a self (k′,l′,m′)-complemented (k,l,m)-DING on G∗=(V,E). Since G≅G(c,(k′,l′,m′)), there exists an isomorphism f:V→V such that for any xy∈E,
k′TcE(f(x)f(y))=kTE(xy),l′IcE(f(x)f(y))=lIE(xy),m′FcE(f(x)f(y))=mFE(xy), |
and for any x∈V,
TcV(f(x))=TV(x),IcV(f(x))=IV(x),FcV(f(x))=FV(x). |
It follows that
kTE(xy)=k′TcE(f(x)f(y))=k′(k′TE(f(x)f(y))−TDo(TV(f(x)),TV(f(y)))=k′(k′kTDo(TV(f(x)),TV(f(y))−TDo(TV(f(x)),TV(f(y)))=k′(k′k−1)TDo(TV(f(x)),TV(f(y)). |
Hence TE(xy)=(k′2−kk′k2)TDo(TV(f(x)),TV(f(y)). In a similar way, one can see that IE(xy)=(k′2−kk′k2)TDo(IV(f(x)),IV(f(y)) and so for any xy∈E, we have
∑x≠yxy∈ETE(xy)=(k′2−kk′k2)∑x≠yTDo(TV(x),TV(y))and∑x≠yxy∈EIE(xy)=(l′2−ll′k2)∑x≠yTDo(IV(x),IV(y)). |
In addition,
mFE(xy)=m′FcE(f(x)f(y))=m′(FE(f(x)f(y))−m′TDo(FV(f(x)),FV(f(y)))=m′(1mTDo(FV(f(x)),FV(f(y))−m′TDo(FV(f(x)),FV(f(y)))=m′(1−mm′m)TDo(FV(f(x)),FV(f(y)). |
Thus, FE(xy)=(m′−mm′2m2)TDo(FV(f(x)),FV(f(y)) and so
∑x≠yxy∈EFE(xy)=(m′−mm′2m2)∑x≠yTDo(FV(x),FV(y)). |
(iv) Since k′2−kk′k2>0,l′2−ll′l2>0 and m′−mm′2m2>0, we get that k′>k,l′>l and mm′<1.
Let G=(σ,μ,TDo) be a (k,l,m)-DING on G∗=(V,E),k′,l′,m′∈R>0,α=k′k′2−k,β=l′l′2−l,γ=m′−mm′2m2,α′=1k−1,β′=1l−1 and γ′=1−m2m. Thus, we have the following theorem.
Theorem 3.13. Let G=(σ,μ,TDo) be a (k,l,m)-DING on G∗=(V,E),k′,l′,m′∈R>0.
(i) If μ(xy)=(α(TDo(TV(x),TV(y))),β(TDo(IV(x),IV(y))),γ(TDo(FV(x),FV(y)))), then k′>√k,l′>√l,m′<1m and G is a self (k′,l′,m′)-complemented (k,l,m)-DING.
(ii) If μ(xy)=(α′(TDo(TV(x),TV(y))),β′(TDo(IV(x),IV(y))),γ′(TDo(FV(x),FV(y)))), then k>1,l>1,m<1 and G is a self (k,l,m)-complemented (k,l,m)-DING.
Proof. (i) Let TE(xy)=(k′k′2−k)(TDo(TV(x),TV(y))) and f:V→Vx↦x. Then
k′TcE(xy)=k′(k′TE(xy)−TDo(TV(x),TV(y)))=k′(k′(k′k′2−k)(TDo(TV(x),TV(y)))−TDo(TV(x),TV(y))))=(kk′k′2−k)TDo(TV(x),TV(y)))=kTE(xy). |
It follows that G is a self k′-complement DING. It is similar to equation l′IcE(xy)=lTIE(xy) if and only if IE(xy)=l′l′2−lTDo(IV(x),IV(y))). In addition, FE(xy)=(k′k′2−k)(TDo(FV(x),FV(y))), implies that
m′FcE(xy)=m′(FE(xy)−m′TDo(FV(x),FV(y)))=m′((1m)(TDo(FV(x),FV(y)))−m′TDo(FV(x),FV(y))))=(m′−mm′2m2)TDo(FV(x),FV(y)))=mFE(xy). |
(ii) Consider (k′,l′,m′)=(k,l,m), then by (i), G is a self (k,l,m)-complemented (k,l,m)-DING.
Theorem 3.14. Let G=(σ,μ,TDo) be a (1,1,14)-DING on G∗=(V,E). Then G=(σ,μ,TDo) is a self (1+√52,1+√52,2+√3)-complemented DING.
Proof. Let G=(σ,μ,TDo) be a DING on G∗=(V,E). If G=(σ,μ,TD) is a self (k,l,m)-complemented Dombi inverse fuzzy graph, then
kTcE(xy)=k(kTE(xy)−TE(xy))=k2TE(xy)−kTE(xy)=TE(xy),kIcE(xy)=k(kIE(xy)−IE(xy))=k2IE(xy)−kIE(xy)=IE(xy),kFcE(xy)=k(FE(xy)−kTDo(FV(x),FV(y)))=kFE(xy)−k2TDo(FV(x),FV(y))=14FE(xy), |
because of, TE(xy)=TDo(TV(x),TV(y)),IE(xy)=TDo(IV(x),IV(y)) and 14FE(xy)=TDo(FV(x),FV(y)). It follows that, k=l=1+√52 and m=2+√3.
Corollary 3.15. Let G=(σ,μ,TDo) be a DING on G∗=(V,E). Then G=(σ,μ,TDo) is't a self complement DING.
Proof. Let G=(σ,μ,TDo) be a self complemented DING on G∗=(V,E) and TE(xy)≠0,IE(xy)≠0 and FE(xy)≠0 for any x,y∈V. By Theorem 3.14, k=l=1+√52. In addition, kFcE(xy)=FE(xy), implies that m=1+√3i2∉R>0, which is a contradiction.
Theorem 3.16. Let G=(σ,μ,TDo) be a (k,l,m)-DING. Then G(c,(c,(k′,l′,m′)))≅G if and only if μ(xy)=(δTDo(TV(x),TV(y)),θTDo(IV(x),IV(y)),ϑTDo(FV(x),FV(y)), where δ=k′2+k′k′3−k,θ=l′2+l′l′3−l and ϑ=2m′2m′−m.
Proof. Let xy∈E and x∈V. Then (TcV)c(x)=TV(x),(IcV)c(x)=IV(x),(FcV)c(x)=FV(x). In addition, k′((TcE)c(xy))=kTE(xy) if and only if
k′(k′(TcE(xy))−TDo(TV(x),TV(y)))=kTE(xy)⟺k′(k′(k′TE(xy)−TDo(TV(x),TV(y))))−TDo(TV(x),TV(y)))=kTE(xy)⟺TE(xy)=(k′2+k′k′3−k)(TDo(TV(x),TV(y))). |
In a similar way, l′((IcE)c(xy))=lIE(xy) if and only if IE(xy)=(l′2+l′l′3−l)(TDo(IV(x),IV(y))). Moreover, m′((FcE)c(xy))=mFE(xy) if and only if
m′(FcE(xy))−m′TDo(FV(x),FV(y)))=mFE(xy)⟺m′((FE(xy)−m′TDo(FV(x),FV(y))))−m′TDo(FV(x),FV(y)))=mFE(xy)⟺FE(xy)=(2m′2m′−m)(TDo(FV(x),FV(y))). |
It follows that G(c,(c,(k′,l′,m′)))≅G if and only if μ(xy)=(δTDo(TV(x),TV(y)),θTDo(IV(x),IV(y)),ϑTDo(FV(x),FV(y)), where δ=k′2+k′k′3−k,θ=l′2+l′l′3−l and ϑ=2m′2m′−m.
Let n∈N. Then from now on, will denote G(c,(c,(k′,l′,m′))) by G(c2,(k′,l′,m′)), G(c,c,(c,(k′,l′,m′))) by G(c3,(k′,l′,m′)) and G(c,c,…,c⏟(n−1)−times,(c,(k′,l′,m′))) by G(cn,(k′,l′,m′)). Additionally consider δn=k′(k′n−1)((k′)n+1−k)(k′−1)),θn=l′(l′n−1)((l′)n+1−l)(l′−1)) and ϑn=nm′2m′−m.
Corollary 3.17. Let G=(σ,μ,TDo) be a (k,l,m)-DING and k∈R>0. Then
(i)G(cn,(k′,l′,m′))≅G⇔μ(xy)=(δn(TDo(TV(x),TV(y)),θn(TDo(IV(x),IV(y)),ϑn(TDo(FV(x),FV(y))).
(ii)G(cn,(k,l,2m))≅G⇔μ(xy)=(a(TDo(TV(x),TV(y))),b(TDo(IV(x),IV(y))),(c(TDo(FV(x),FV(y)))), where a=1k−1,b=1l−1 and c=4nm.
(ii)G(cn,(k,l,m))≇G
Theorem 3.18. Let G=(σ,μ,TDo) be a complete (k,l,m)-DING and k,l,m∈R>0. Then
(i)G(c,(k′,l′,m′))=(σc,μc,TDo) is a complete (kk′−k,ll′−l,1−mm′m)-DING.
(ii)G(cn,(k′,l′,m′)) is a complete (kk′n−k(∑0≤i≤n−1k′i),jj′n−j(∑0≤i≤n−1j′i),1−nmm′m)-DING.
Proof. (i) Let xy∈E. Then
TcE(xy)=k′TE(xy)−TDo(TV(x),TV(y))=k′kTDo(TV(x),TV(y))−TDo(TV(x),TV(y))=(k′−kk)TDo(TV(x),TV(y)). |
In a similar way, IcE(xy)=(l′−ll)TDo(IV(x),IV(y)). Moreover,
FcE(xy)=FE(xy)−m′TDo(FV(x),FV(y))=1mTDo(FV(x),FV(y))−m′TDo(FV(x),FV(y))=(1−mm′m)TDo(TV(x),TV(y)). |
(ii) It is obtained from (i) and by induction.
Corollary 3.19. Let n∈N,G=(σ,μ,TDo) be a complete (k,l,m)-DING and k,l,m∈R>0. Then G(cn,(k,l,m)) can't be a complete (k,l,m)-DING.
Definition 3.20. Let G=(σ,μ,TDo) be a (k,l,m)-DING on a simple graph G∗=(V,E). Then OT(G)=∑x∈VTV(x)(as truth membership order of G), OI(G)=∑x∈VIV(x)(as indeterminacy membership order of G), OF(G)=∑x∈VFV(x)(as falsity membership order of G), ST(G)=k∑xy∈Ex≠yTE(xy)(as truth membership size of G), SI(G)=l∑xy∈Ex≠yIE(xy)(as indeterminacy membership size of G) and SF(G)=m∑xy∈Ex≠yFE(xy)(as indeterminacy membership size of G).
Theorem 3.21. Let G=(σ,μ,TDo) be a (k,l,m)-DING and G′=(σ′,μ′,TDo) be a (k′,l′,m′) on G∗=(V,E) and G′∗=(V′,E′), respectively. Then
(i)OT(G)=OT(G′), OI(G)=OT(G′) and OF(G)=OF(G′).
(ii)ST(G)=ST(G′), SI(G)=SI(G′) and SF(G)=SF(G′).
Proof. It is clear by definition.
Example 3.22. Let V={a,b,c}. Then G1=(σ1,μ1,TDo)≅G2=(σ2,μ2,TDo) are (5,5,0.2)-DING and G2 and (4,6,0.4)-DING, respectively as shown in Figure 5. Computations show that OT(G1)=OT(G2), OI(G1)=OT(G2),OF(G1)=OF(G2),ST(G1)=ST(G2), SI(G1)=SI(G2) and SF(G1)=SF(G2), while G1≆G2.
Example 3.22, shows that the converse of the Theorem 3.21, is not necessarily true.
Definition 3.23. Let G=(σ,μ,TDo) be a (k,l,m)-DING on a simple graph G∗=(V,E) and x∈V. Then dT(x,G)=∑xy∈ETE(xy)(as truth membership value of x), dI(x,G)=∑xy∈EIE(xy)(as indeterminacy membership value of x), dF(x,G)=∑xy∈EFE(xy)(as falsity membership value of x), dT(xy,G)=TV(x)+TV(y)(as truth membership value of xy), dI(xy,G)=IV(x)+IV(y)(as indeterminacy membership value of xy) and dF(xy,G)=FV(x)+FV(y)(as falsity membership value of xy).
Theorem 3.24. Let G=(σ,μ,TDo) be a (k,l,m)-DING and G′=(σ′,μ′,TDo) be a (k′,l′,m′) on G∗=(V,E) and G′∗=(V′,E′), respectively. Then
(i)dT(x,G)=k′kdT(x,G′), dT(x,G)=l′ldT(x,G′) and dT(x,G)=m′mdT(x,G′).
(ii)ST(G)=2kdT(x,G), SI(G)=2ldI(x,G) and SF(G)=2mdF(x,G).
Proof. It is clear by definition.
In this section, we apply the concept of valued inverse Dombi neutrosophic graph in the real world. In mathematics, a network is called a graph, objects are called vertices (or nodes), and the connections are called edges. For example, when we represent the social network of a collection of people as a graph, the vertices are the people under consideration, while the edges list all the friendships between them. Social network: Establishing social relations between people can have an impactive on a person's life. In today's developing world, social relations are one of the basic principles for the durability and sustainability of individual life. According to the experts in sociology, social relations can be defined as communication between two people or several groups, that is, social relations are the communication and interdependence of people towards each other. These relationships can be positive and constructive, like friendship, or negative and destructive, like enmity. In the unique sense of social communication, it refers to communication that leads to the transfer of meaning or message among a group of people. Therefore, social communication requires several elements such as intelligence (as a truth membership degree), incuriosity (as an indeterministic membership degree) and deception (as a falsity membership degree). Now, we consider a complex network, which we investigated as the following algorithm:
step1: In Table 1, we introduce a group of people with some characteristics of intelligence, incuriosity, and deception in social personality that are supposed to work in a social network(for instance, Benjamin(0.6,0.7,0.1) means that, based on psychological and scientific research, Benjamin uses 60 percent of his talent, 70 percent is curious and 10 percent is deceitful).
Intelligence | Incuriosity | Deception | |
Benjamin | 0.6 | 0.7 | 0.1 |
Oliver | 0.7 | 0.8 | 0.2 |
James | 0.8 | 0.9 | 0.3 |
William | 0.4 | 0.5 | 0.4 |
Lucas | 0.5 | 0.6 | 0.5 |
step2: In Figure 6 (the vertex set represents the people and the edge set represents the communication between them), we there are essential factors that can create communication between people in the group such as mutual respect (as a truth membership degree), responsibility (as an indeterministic membership degree) and insincere (as a falsity membership degree). In this step, we compute the relation between of vertices with impact (1,1,10). For instance, TE(BO)=0.6×0.70.6+0.7−0.42=2144,IE(BO)=0.7×0.80.7+0.8−0.56=2847,FE(BO)=1100.1×0.20.1+0.2−0.02=1140.
step 3: By definition 3.23, we want to identify the best one in terms of the most mutual respect, the most responsibility and the least insincere and to identify the best co-worker in terms of the most intelligence and mutual respect, incuriosity, and responsibility, and the least deception and Insincere. Thus, computations show these aims in Tables 2 and 3. For instance, dT(W,G)=411+619≃0.7,dT(B,G)=2144+619≃0.8 and so dT(BW)=0.7+0.8=1.5.
dT(x,G) | dI(x,G) | dF(x,G) |
(O, 1.5) | (O, 1.8) | (B, 0.02) |
(J, 1) | (J, 1.2) | (L, 0.02) |
(B, 0.8) | (B, 1) | (J, 0.03) |
(W, 0.7) | (W, 0.9) | (W, 0.03) |
(L, 0.4) | (L, 0.5) | (O, 0.04) |
dT(xy,G) | dI(xy,G) | dF(xy,G) |
(OJ, 2.5) | (OJ, 3) | (WB, 0.05) |
(BO, 2.3) | (BO, 2.8) | (BO, 0.06) |
(LO, 1.9) | (LO, 2.3) | (JW, 0.06) |
(WJ, 1.7) | (JW, 2.1) | (LO, 0.06) |
(BW, 1.5) | (BW, 1.9) | (JO, 0.07) |
step 4: From Table 2, we find that the best candidate In term of mutual respect and responsibility is Oliver, and the best candidate In term of insincere is Benjamin. Additionally for any x,y,dT(JO)≥dT(xy),dI(JO)≥dI(xy), while dF(JO)≤dT(xy).
The current paper has introduced a novel concept of valued-inverse Dombi neutrosophic graph and (complete) strong valued-inverse Dombi neutrosophic graph as a generalization of the inverse neutrosophic graph. This study investigates some conditions such that neutrosophic subsets be valued-inverse Dombi neutrosophic graphs and Dombi neutrosophic graphs.The complement of valued-inverse Dombi neutrosophic graphs is introduced and concerning the notation of isomorphism theorems, we can prove that the complement of a valued-inverse Dombi neutrosophic graph is a valued-inverse Dombi neutrosophic graph. The complement of valued-inverse Dombi neutrosophic graphs is extended by induction and the isomorphic complement of valued-inverse Dombi neutrosophic graphs is analyzed. Finally, we apply these concepts to the real world and design a real problem in the social network. Also
(i) we show that for any given (k,l,m)-DING, with what changes of parameters is a valued-inverse Dombi neutrosophic graph.
(ii) We show that for any given (k,l,m)-DING, conditions in parameters, is a strong valued-inverse Dombi neutrosophic graph and strong (k,l,m)-DING.
(iii) The solving of non-equations plays a prominent role in finding the ordered triple for a value of complement of valued-inverse Dombi neutrosophic graphs.
(iv) We find the conditions on ordered triples and how a complement of strong valued-inverse Dombi neutrosophic graph is strong.
(v) It is introduced as the notation of homomorphism (isomorphism), and it proves how the complement of two isomorphic valued-inverse Dombi neutrosophic graphs are isomorphic.
(vi) It introduces the concept of self-valued-inverse Dombi of strong (k,l,m)-DING and investigates the relation between the components of its neutrosophic vertices and neutrosophic edges.
(vii) It presents the truth membership order, indeterminacy membership order, falsity membership order, truth membership size, indeterminacy membership size, and indeterminacy membership size of any given (k,l,m)-DING.
(viii) The truth membership order, indeterminacy membership order, falsity membership order, truth membership size, indeterminacy membership size, and indeterminacy membership size of any given (k,l,m)-DING play the prominent role in the application of valued-inverse Dombi neutrosophic graphs in complex networks.
The authors declare they have not used Artificial Intelligence (AI) tools in the creation of this article.
The authors declare that they have no conflict of interest.
[1] | M. Akram, S. Shahzadi, A. B. Saeid, Single valued neutrosophic Hypergraphs, TWMS J. Appl. Eng. Math., 8 (2018), 122–135. |
[2] | M. Akram, Single-Valued Neutrosophic Graphs, Singapore: Springer, 2018. https://doi.org/10.1007/978-981-13-3522-8 |
[3] | M. Akram, H. Saba Nawaz, Implementation of single-valued neutrosophic soft hypergraphs on human nervous system, Artif. Intell. Rev., 56 (2022), 1387–1425. |
[4] | M. Akram, H. Saba Nawaz, Algorithms for the computation of regular single-valued neutrosophic soft hypergraphs applied to supranational asian bodies, J. Appl. Math. Comput., 68 (2022), 4479–4506. |
[5] |
D. Ajay, P. Chellamani, G. Rajchakit, N. Boonsatit, P. Hammachukiattikul, Regularity of Pythagorean neutrosophic graphs with an illustration in MCDM, AIMS Mathematics, 7 (2022), 9424–9442. https://doi.org/10.3934/math.2022523 doi: 10.3934/math.2022523
![]() |
[6] | M. Akram, M. H Sarwar, W. A. Dudek, Bipolar Neutrosophic Graph Structures, In: Graphs for the Analysis of Bipolar Fuzzy Information, Singapore: Springer, 2020,393–446. https://doi.org/10.1007/978-981-15-8756-6_10 |
[7] | S. Ashraf, S. Naz, E. E. Kerre, Dombi Fuzzy Graphs, Fuzzy Inf. Eng., 10 (2018), 58–79. https://doi.org/10.1080/16168658.2018.1509520 |
[8] | R. A. Borzooei, R. Almallah, Y. B. Jun, H. Ghaznavi, Inverse Fuzzy Graphs with Applications, New Math. Nat. Comput., 16 (2020), 397–418. https://doi.org/10.1142/S1793005720500246 |
[9] | G. Ghorai, M. Pal, Certain types of product bipolar fuzzy graphs, Int. J. Appl. Comput. Math., 3 (2017), 605–619. |
[10] | S. Jahanpanah, M. Hamidi, Valued-Inverse Dombi Fuzzy Graph, 2023, Submitted. |
[11] | M. Hamidi, S. Jahanpanah, A. Radfar, Extended graphs based on KM-fuzzy metric spaces, Iran. J. Fuzzy Syst., 17 (2020), 81–95. |
[12] | M. Hamidi, S. Jahanpanah, A. Radfar, On KM-Fuzzy Metric Hypergraphs, Fuzzy Inf. Eng., 12 (2020), 300–321. https://doi.org/10.1080/16168658.2020.1867419 |
[13] | A. Kaufmann, Introduction to the theory of fuzzy subsets, New York: Academic Press, 1975. |
[14] | T. S. Lakhwani, K. Mohanta, A. Dey, S. P. Mondal, A. Pal, Some operations on Dombi neutrosophic graph, J. Ambient Intell. Human. Comput., 13 (2022), 425–443. https://doi.org/10.1007/s12652-021-02909-3 |
[15] | K. Mohanta, A. Dey, A. Pal, A note on different types of product of neutrosophic graphs, Complex. Intell. Syst., 7 (2021), 857–871. https://doi.org/10.1007/s40747-020-00238-0 |
[16] | R. Mahapatra, S. Samanta, M. Pal, Generalized neutrosophic planar graphs and its application, J. Appl. Math. Comput., 65 (2021), 693–712. https://doi.org/10.1007/s12190-020-01411-x |
[17] | H. T. Nguyen, C. L. Walker, E. A. Walker, A First Course in Fuzzy Logic, 4 Eds., Boca Raton: CRC Press, 2018. |
[18] | A. Rosenfeld, Fuzzy graphs, In: Fuzzy sets and their applications to cognitive and decision processes, New York: Academic Press, 1975, 77–95. https://doi.org/10.1016/B978-0-12-775260-0.50008-6 |
[19] | S. Siddique, U. Ahmad, M. Akram, A study on generalized graphs representations of complex neutrosophic information, J. Appl. Math. Comput., 65 (2021), 481–514. https://doi.org/10.1007/s12190-020-01400-0 |
[20] | S. Sahin, A. Kargın, M. Yucel, Hausdorff Measures on Generalized Set Valued Neutrosophic Quadruple Numbers and Decision Making Applications for Adequacy of Online Education, Neutrosophic Sets Syst., 40 (2021), 86–116. |
[21] | F. Smarandache, Neutrosophic set a generalization of the intuitionistic fuzzy set, Int. J. Pure. Appl. Math., 24 (2005), 287–297. |
[22] |
S. Zeng, M. Shoaib, S. Ali, F. Smarandache, H. Rashmanlou, F. Mofidnakhaei, Certain Properties of Single-Valued Neutrosophic Graph With Application in Food and Agriculture Organization, Int. J. Comput. Intell., 14 (2021), 1516–1540. https://doi.org/10.2991/ijcis.d.210413.001 doi: 10.2991/ijcis.d.210413.001
![]() |
[23] | J. Zhan, M. Akram, M. Sitara, Novel decision-making method based on bipolar neutrosophic information, Soft Comput., 23 (2019), 9955–9977. |
Intelligence | Incuriosity | Deception | |
Benjamin | 0.6 | 0.7 | 0.1 |
Oliver | 0.7 | 0.8 | 0.2 |
James | 0.8 | 0.9 | 0.3 |
William | 0.4 | 0.5 | 0.4 |
Lucas | 0.5 | 0.6 | 0.5 |
dT(x,G) | dI(x,G) | dF(x,G) |
(O, 1.5) | (O, 1.8) | (B, 0.02) |
(J, 1) | (J, 1.2) | (L, 0.02) |
(B, 0.8) | (B, 1) | (J, 0.03) |
(W, 0.7) | (W, 0.9) | (W, 0.03) |
(L, 0.4) | (L, 0.5) | (O, 0.04) |
dT(xy,G) | dI(xy,G) | dF(xy,G) |
(OJ, 2.5) | (OJ, 3) | (WB, 0.05) |
(BO, 2.3) | (BO, 2.8) | (BO, 0.06) |
(LO, 1.9) | (LO, 2.3) | (JW, 0.06) |
(WJ, 1.7) | (JW, 2.1) | (LO, 0.06) |
(BW, 1.5) | (BW, 1.9) | (JO, 0.07) |
Intelligence | Incuriosity | Deception | |
Benjamin | 0.6 | 0.7 | 0.1 |
Oliver | 0.7 | 0.8 | 0.2 |
James | 0.8 | 0.9 | 0.3 |
William | 0.4 | 0.5 | 0.4 |
Lucas | 0.5 | 0.6 | 0.5 |
dT(x,G) | dI(x,G) | dF(x,G) |
(O, 1.5) | (O, 1.8) | (B, 0.02) |
(J, 1) | (J, 1.2) | (L, 0.02) |
(B, 0.8) | (B, 1) | (J, 0.03) |
(W, 0.7) | (W, 0.9) | (W, 0.03) |
(L, 0.4) | (L, 0.5) | (O, 0.04) |
dT(xy,G) | dI(xy,G) | dF(xy,G) |
(OJ, 2.5) | (OJ, 3) | (WB, 0.05) |
(BO, 2.3) | (BO, 2.8) | (BO, 0.06) |
(LO, 1.9) | (LO, 2.3) | (JW, 0.06) |
(WJ, 1.7) | (JW, 2.1) | (LO, 0.06) |
(BW, 1.5) | (BW, 1.9) | (JO, 0.07) |