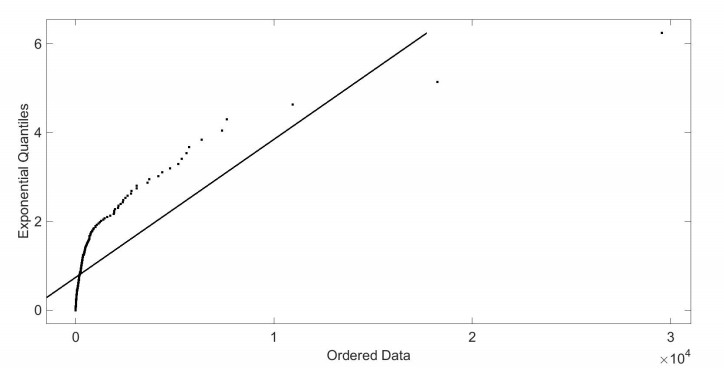
Catastrophe reinsurance is an important way to prevent and resolve catastrophe risks. As a consequence, the pricing of catastrophe reinsurance becomes a core problem in catastrophic risk management field. Due to the severity of catastrophe loss, the Peak Over Threshold (POT) model in extreme value theory (EVT) is extensively applied to capture the tail characteristics of catastrophic loss distribution. However, there is little research available on the pricing formula of catastrophe excess of loss (Cat XL) reinsurance when the catastrophe loss is modeled by POT. In the context of POT model, we distinguish three different relations between retention and threshold, and then prove the explicit pricing formula respectively under the standard deviation premium principle. Furthermore, we fit POT model to the earthquake loss data in China during 1990–2016. Finally, we give the prices of earthquake reinsurance for different retention cases. The computational results illustrate that the pricing formulas obtained in this paper are valid and can provide basis for the pricing of Cat XL reinsurance contracts.
Citation: Wen Chao. Pricing catastrophe reinsurance under the standard deviation premium principle[J]. AIMS Mathematics, 2022, 7(3): 4472-4484. doi: 10.3934/math.2022249
[1] | Ying Fang, Guo Cheng, Zhongfeng Qu . Optimal reinsurance for both an insurer and a reinsurer under general premium principles. AIMS Mathematics, 2020, 5(4): 3231-3255. doi: 10.3934/math.2020208 |
[2] | Ling Chen, Xiang Hu, Mi Chen . Optimal investment and reinsurance for the insurer and reinsurer with the joint exponential utility under the CEV model. AIMS Mathematics, 2023, 8(7): 15383-15410. doi: 10.3934/math.2023786 |
[3] | Wanlu Zhang, Hui Meng . Robust optimal investment-reinsurance strategies with the preferred reinsurance level of reinsurer. AIMS Mathematics, 2022, 7(6): 10024-10051. doi: 10.3934/math.2022559 |
[4] | Wuyuan Jiang, Zechao Miao, Jun Liu . Optimal investment and reinsurance for the insurer and reinsurer with the joint exponential utility. AIMS Mathematics, 2024, 9(12): 35181-35217. doi: 10.3934/math.20241672 |
[5] | Peng Yang . Robust optimal reinsurance-investment problem for n competitive and cooperative insurers under ambiguity aversion. AIMS Mathematics, 2023, 8(10): 25131-25163. doi: 10.3934/math.20231283 |
[6] | Peng Yang . Robust optimal reinsurance strategy with correlated claims and competition. AIMS Mathematics, 2023, 8(7): 15689-15711. doi: 10.3934/math.2023801 |
[7] | Hua Zhao, Yue Xin, Jinwu Gao, Yin Gao . Power-barrier option pricing formulas in uncertain financial market with floating interest rate. AIMS Mathematics, 2023, 8(9): 20395-20414. doi: 10.3934/math.20231040 |
[8] | Xiaoyi Tang, Wei Liu, Wanyin Wu, Yijun Hu . An optimal investment strategy for DC pension plans with costs and the return of premium clauses under the CEV model. AIMS Mathematics, 2024, 9(8): 21731-21754. doi: 10.3934/math.20241057 |
[9] | Shuang Guo, Yong Zhang . Moderate deviation principle for m-dependent random variables under the sub-linear expectation. AIMS Mathematics, 2022, 7(4): 5943-5956. doi: 10.3934/math.2022331 |
[10] | Yao Fu, Sisi Zhou, Xin Li, Feng Rao . Multi-assets Asian rainbow options pricing with stochastic interest rates obeying the Vasicek model. AIMS Mathematics, 2023, 8(5): 10685-10710. doi: 10.3934/math.2023542 |
Catastrophe reinsurance is an important way to prevent and resolve catastrophe risks. As a consequence, the pricing of catastrophe reinsurance becomes a core problem in catastrophic risk management field. Due to the severity of catastrophe loss, the Peak Over Threshold (POT) model in extreme value theory (EVT) is extensively applied to capture the tail characteristics of catastrophic loss distribution. However, there is little research available on the pricing formula of catastrophe excess of loss (Cat XL) reinsurance when the catastrophe loss is modeled by POT. In the context of POT model, we distinguish three different relations between retention and threshold, and then prove the explicit pricing formula respectively under the standard deviation premium principle. Furthermore, we fit POT model to the earthquake loss data in China during 1990–2016. Finally, we give the prices of earthquake reinsurance for different retention cases. The computational results illustrate that the pricing formulas obtained in this paper are valid and can provide basis for the pricing of Cat XL reinsurance contracts.
In the past few decades, natural disasters such as earthquakes, floods, and typhoons occurred more frequently, which usually lead to serious casualties and property damage. In order to disperse the risk of a possible large payment caused by these catastrophe events, insurance companies may purchase catastrophe excess of loss (Cat XL) contracts from reinsurers. It is thus natural and important to ask: What is the correct price of a Cat XL contract?
The early pricing model of catastrophe reinsurance is introduced by Strickler [23] in 1960. In [23] (see also [12]), the author supposed a constant deterministic rate of catastrophes, which not only brings an elegant pricing formula but also causes no statistical approach to update the model in accordance to new data. Combing the compound Poisson process and the POT model, Ekheden and Hössjer [8] suggested a new pricing model to overcome the drawbacks of [12,23]. Under the standard deviation premium principle, they got the approximate price of a Cat XL contract using Monte Carlo simulation techniques. Subsequently, Leppisaari [15] further improved the pricing model established in [8] by providing a micro-simulation approach to catastrophe reinsurance pricing. Considering the influence of interest rate fluctuation, Chao [3] priced a new type of multi-risk catastrophe reinsurance contract with interest rate dynamics described by the Cox-Ingersoll-Ross model. However, we have to emphasize that the above works [3,8,15] cannot give accurate price of Cat XL contract due to the lack of explicit pricing formulas. For more related results, we refer the interested readers to [1,6,21] and the references therein.
We also remark that catastrophe bond is an innovative financial instrument, by which the insurers can transfer the risk of a possible large payment caused by catastrophic events to the capital market. As pointed in the pioneer work of Cox and Pedersen [5], the catastrophe risk cannot be replicated by a portfolio of the traditional securities such as stock and bond, which means that the pricing of a catastrophe bond has to be done in an incomplete market framework. In [5], the authors derived the theoretically pricing of the catastrophe bond via a time-repeatable representative agent utility, which is based on an interest rate term structure model and the probability of catastrophe risk model. Under the same framework as [5], Zimbidis [27] calculated the price of a Greek risk bond for earthquakes by applying the equilibrium pricing theory together with extreme value theory. Another common technique employed for pricing in an incomplete market is indifference pricing via expected utility, see e.g. [7,14,26]. Among others, the paper by Young [26] is the first to calculate the indifference price of a contingent claim in a stochastic interest rate environment for an exponential utility function. In [14], by using the utility indifference pricing method, Karagiannis et al. further obtained the price of an agricultural catastrophe bond, which can be described as an over-the-counter insurance contract on catastrophe risk between an insurance company and a hedge-fund.
The relationship between the catastrophe bond and reinsurance has attracted the attention of some researchers. For example, Finken et al. [10] showed that catastrophe bonds involving parametric or index triggers can provide low-risk insurers with an alternative to reinsurance contracts when basis risk is not too high. Subsequently, Härdle and Cabrera [13] performed the calibration of a parametric catastrophe bond that was sponsored by the Mexican government, and found that combining reinsurance with catastrophe bond is optimal. Trottier and Lai [24] further developed a contingent claims framework and illustrated that it is optimal to cover small losses using reinsurance and hedge higher losses by using a CAT bond.
However, as far as we know, there is no study found in the literature that focused on explicit pricing formula of catastrophe reinsurance through POT modelling except the one by Xiao and Meng [25]. Based on the POT model, [25] proved explicit Cat XL contract formulas in term of three different retention cases under the assumption that catastrophe reinsurance premium is calculated by the expectation principle. However, in the practice of (re)insurance, the reinsurers usually charge for their capital costs apart from the expected claims. It follows, then that a percentage of the standard deviation is often added to the pure premium. In view of this, the object of this paper is to prove explicit pricing formula for Cat XL contract under the standard deviation premium principle.
The reminder of this paper is organized as follows. Section 2 briefly describes the Extreme Value Theory. In Section 3, we prove the catastrophe reinsurance pricing formulas in three different relations between the retention and the threshold. In Section 4, we present an empirical analysis according to the earthquake loss data in China during 1990–2016. Finally, it ends with conclusions in Section 5.
The frequency of catastrophe risk is low, but the losses are very serious, which has obvious characteristics of heavy tail. Specifically, Extreme Value Theory is mainly employed to research the extreme value distribution and its characteristics of random variables.
Extreme Value Theory mainly provides two characterization methods based on the extreme value behavior of the observation data, namely Block Maximum Method (BMM) and Peak Over Threshold (POT). The former one divides the data into several groups, and then takes the maximum (or minimum) in these groups as a sample for fitting. The latter one firstly selects a threshold, and then fit the data that exceeds the threshold. For the BMM, it only takes into account the maximum value (or minimum value) during the modeling process, but extreme value data actually appear in series. This may cause a large amount of effective data information to be lost. In order to make full use of data, we apply in this paper the POT model first introduced by Pickands [20] to fit the earthquake catastrophe loss. This model is to study the asymptotic distribution of observation samples greater than the threshold.
Let X1,X2,⋯,Xn be a sequence of independent and identically distributed random variable with distribution function F. Denote by u(u>0) the threshold. Additionally, the Y=X−u is called excess. Correspondingly, the distribution function of Y is called as conditional excess distribution function
Fu(y)=P(X−u≤y|X>u)=F(u+y)−F(u)1−F(u),y≥0, |
which yields
F(x)=(1−F(u))Fu(x−u)+F(u),x≥u>0. | (2.1) |
Let x∗=sup{x∈R:F(x)<1}. According to Balkema and Haan [2] and Pickands [19], for large enough u>0, there holds
limu→x∗sup0≤y<x∗−u|Fu(y)−G(y;u,β,ξ)|=0 |
where G is a generalized Pareto distribution (GPD). Thence, for large u>0, Fu(y) can be well approximated by G, namely
Fu(y)≈Gξ,σ(y)={1−(1+ξyσ)−1/ξ,ξ>0,y≥0;1−exp(−yσ),ξ=0,y≥0;1−(1+ξyσ)−1/ξ,ξ<0,0≤y≤−β/ξ, | (2.2) |
where ξ and β are the shape parameter and the scale parameter, respectively. Note that the variance of GPD does not exist if ξ>1/2. To ensure the price of Cat XL contract can be calculated by the standard deviation premium principle, we assume that ξ≤1/2 from now on.
Substituting (2.2) into (2.1), we have the following result,
F(x)={(1−F(u))(1−(1+ξx−uσ))+F(u),ξ≠0,x≥u>0;(1−F(u))(1−exp(−x−uσ))+F(u),ξ=0,x≥u>0. | (2.3) |
Since catastrophic risks possesses obvious characteristics of heavy tails, many scholars (e.g. [4,16,18]) adopted heavy-tailed distributions to fit catastrophe loss data, e.g. Gamma distribution, log-normal distribution, etc. However, these distributions cannot well reflect the large losses of catastrophe risk. Therefore, these general heavy-tailed distributions are not suitable for fitting the high over claims of catastrophe reinsurance. Meanwhile the extreme value distribution is able to make up for this defect, see [11,15,17,22,27].
Let N(t) be a Poisson process with a constant arrival rate λ and let D be the cedant's retention. Xi denotes the economic loss of the ith catastrophe event, and Xi is independent of the N. The catastrophe excess of loss (Cat XL) contract is defined as follows: If the aggregate claim of the ceding insurance company as a result of a single catastrophic event exceeds the retention D, the excess Xi−D will be paid by the reinsurer. Therefore, the total amount of claims paid by the reinsurer can be expressed by
Z=N(t)∑i=1(Xi−D)+=:N(t)∑i=1Ci |
where (Xi−D)+=max{Xi−D,0}.
In the light of the standard deviation premium principle, the Cat XL contract price V is given by
V=E(Z)+ρ×SD(Z) | (3.1) |
where E(Z) is the pure premium, ρ×SD(Z) is an additional loading with typically ρ∈[0.1,0.5].
It is well known that
E(Z)=E(N)E(Ci)=λE(Ci) |
and
Var(Z)=E2(Ci)Var(N)+E(N)Var(Ci)=λE(C2i). | (3.2) |
Moreover, by the total expectation formula, one has
E(C2i)=E[(Xi−D)2+|Xi>D]P(Xi>D)+E[(Xi−D)2+|Xi≤D]P(Xi≤D)=E[(Xi−D)2+|Xi>D]P(Xi>D). |
This together with (3.2) imply that
Var(Z)=λE[(Xi−D)2+|Xi>D]P(Xi>D). | (3.3) |
Similarly,
E(Z)=λE[(Xi−D)+|Xi>D]P(Xi>D). | (3.4) |
Combining (3.1), (3.3) and (3.4), we have that
V=λE[(Xi−D)+|Xi>D]P(Xi>D)+ρ√λE[(Xi−D)2+|Xi>D]P(Xi>D). | (3.5) |
To proceed, we need the following proposition.
Proposition 3.1. If a random variable X obeys the generalized Pareto distribution G(x;β,ξ), then there holds
E(Xk)=βkΓ(ξ−1−k)k!ξk+1Γ(1+ξ−1) |
where k is a positive integer, ξ<1/k and Γ(⋅) is the Gamma function.
In practical applications, the relationship between the retention D and the threshold u is uncertain. Motivated by the idea of [25], we next prove the pricing formula of the Cat XL contract under three different cases between D and u.
Case (Ⅰ). D=u.
For this case, by Proposition 3.1, one has
E[(Xi−D)2+|Xi>D]=∫+∞D(x−D)2f(x)ˉF(D)dx=∫+∞0t2fu(t)dt=E(X2)=2β2(1−ξ)(1−2ξ) | (3.6) |
where ˉF(D)=1−F(D). Similarly, we also have
E[Xi−D|Xi>D]=β1−ξ. | (3.7) |
Let n be the sample size, and let nu be the number of the sample exceeding the threshold u. According to the historical simulation method, ˉF(D) can be estimated by nu/n. It then follows from (3.5)–(3.7) that
V=nuλβn(1−ξ)+ρβ√2λnu√n(1−ξ)(1−2ξ). |
Case (Ⅱ). D>u.
In this case, we have
E[(Xi−D)2+|Xi>D]=∫+∞D(x−D)2f(x)ˉF(D)dx=∫+∞0t2f(t+D)ˉF(D)dtdt=∫+∞0t2dFD(t). |
Since FD(x)=Gξ;β+ξ(D−u)(x) and the fact that the generalized Pareto distribution excess distribution function is still a generalized Pareto distribution, it follows that
E[(Xi−D)2+|Xi>D]=∫+∞0t2dGξ;β+ξ(D−u)(t)=2(β+ξ(D−u))2(1−ξ)(1−2ξ). | (3.8) |
Similarly,
E[Xi−D|Xi>D]=β+ξ(D−u)1−ξ. | (3.9) |
On the other hand, there holds
P(X>D)=(1−F(D)−F(u)1−F(u))(1−F(u))=(1−Gξ;β(D−u))(1−F(u)). | (3.10) |
Thus, we can use (3.5) and (3.8)–(3.10) to get
V=λ(β+ξ(D−u))(1−Gξ;β(D−u))(1−F(u))1−ξ+(β+ξ(D−u))√2λ(1−Gξ;β(D−u))(1−F(u))√(1−ξ)(1−2ξ). |
Case (Ⅲ). D<u.
In this case, the POT model discards the data information that the catastrophe loss is below the threshold, therefore, the loss data lie in the range of (D,u) cannot be captured by the POT model. For this sake, we need to find other distribution to describe the information below the threshold. Considering that this article uses China earthquake data for empirical analysis, and it has been proved in [16] that the log-normal distribution is the best fitting for China earthquake loss data among the 12 common heavy tail distributions. Therefore, we choose the log-normal distribution as the fitting distribution of the main data. Namely, the part of the loss data above the threshold is fitted by the generalized Pareto distribution Gξ;β(x), the rest part is fitted by a log-normal distribution F(x;μ,σ2) with the probability density function given by
f(x;μ,σ2)=1σ√2πexp(−(lnx−μ)22σ2),x>0. |
Then the mixed fitting distribution function can be expressed as follows:
F(x)=F(x;μ,σ2)I{x≤u}+[F(u;μ,σ2)+(1−F(u;μ,σ2))Gξ;β(x−u)]I{x>u}, |
where I is a indicator function.
Set J1=∫uD(x−D)2f(x)dx and J2=∫+∞u((x−u)+(u−D)2)f(x)dx. For J1, we easily see that
J1=∫uDx2f(x)dx−2D∫uDxf(x)dx+D2∫uDf(x)dx=e2μ+2σ2[Φ(lnu−μ−2σ2σ)−Φ(lnD−μ−2σ2σ)]−2Deμ+σ22[Φ(lnu−μ−2σ2σ)−Φ(lnD−μ−2σ2σ)]+D2[Φ(lnu−μσ)−Φ(lnD−μσ)], | (3.11) |
where Φ(⋅) is the standard normal distribution.
For J2, one has
J2=∫+∞u(x−u)2f(x)dx+2(u−D)∫+∞u(x−u)f(x)dx+(u−D)2∫+∞uf(x)dx=ˉF(u)∫+∞0t2f(t+u)ˉF(u)dt+2(u−D)ˉF(u)∫+∞0tf(t+u)ˉF(u)dt+(u−D)2ˉF(u)=ˉF(u)2β2(1−ξ)(1−2ξ)+2(u−D)ˉF(u)β1−ξ+(u−D)2ˉF(u). |
This and (3.11) give that
E[(Xi−D)2+|Xi>D]=1ˉF(D)[J1+J2]=1ˉF(D){e2μ+2σ2[Φ(lnu−μ−2σ2σ)−Φ(lnD−μ−2σ2σ)]−2Deμ+σ22[Φ(lnu−μ−2σ2σ)−Φ(lnD−μ−2σ2σ)]+D2[Φ(lnu−μσ)−Φ(lnD−μσ)]+ˉF(u)2β2(1−ξ)(1−2ξ)+2(u−D)ˉF(u)β1−ξ+(u−D)2ˉF(u)}. | (3.12) |
Similar to the proof of (3.12), we also have
E[Xi−D|Xi>D]=1ˉF(D){eμ+σ2/2[Φ(lnu−μ−σ2σ)−Φ(lnD−μ−σ2σ)]−D[Φ(lnu−μσ)−Φ(lnD−μσ)]+β1−ξˉF(u)+(u−D)ˉF(u)}. | (3.13) |
Combining (3.5), (3.12), (3.13), P(X>D)=1−F(D;μ,σ2) and ˉF(u)=nu/n, the pricing formula of Case (Ⅲ) can arrive. The concrete result of formula is omitted for brevity.
This study uses the earthquake direct economic loss (million Chinese Yuan, MCY) data, consisting of 257 observations, provided by China earthquake information network*. In order to ensure the comparability of the loss data in each year, all actual direct economic losses caused by the earthquake were adjusted to the year of 2016's level via the Gross Domestic Product (GDP) provided by the China Statistical Yearbook†. More precisely,
†http://www.stats.gov.cn/english/Statisticaldata/AnnualData/.
Adjusted loss=Actual loss×GDP of 2016GDP of base year. |
Since the form of the GPD function depends on the characteristics of the tail of sample data, it is necessary to test the heavy tail of the data before constructing the POT model. There are two commonly inspection methods including exponential quantile-quantile (QQ) diagram and kurtosis comparison. This paper makes a comprehensive application of the above two methods to judge the characteristics of the tail. As can be seen from Figure 1, the tail behavior of the exponential QQ graph is upward convex, which suggests a heavy-tailed underlying distribution. In addition, the value of kurtosis is 72.7865, which is significantly larger than 3. Thus, we can determined that the earthquake economic loss data posses the characteristic of heavy tail.
One of the main challenges for fitting the POT model is the selection of a suitable threshold. To this end, we draw the Hill plot of economic loss data, see Figure 2. The Hill plot is constructed such that the tail index α is plotted as a function of the number k of exceedances, where α=1/ξ. For a more detailed description, see [9]. Looking at Figure 2, we see that the curve begins to show a steady trend at k=40, which is equivalent to u=906. This means that we can select 906 as the threshold. Moreover, Figure 3 shows the GPD fitted to exceedances of u=906. It is clear to see that the fit for the selected threshold is fairly good.
In the case of u=906, we can further compute the rest parameter estimates in the established model, see Table 1.
GPD | Log-normal distribution | Poisson process | |
parameters | ˆξ=0.3303 | ˆμ=5.0350 | ˆλ=9.5185 |
ˆβ=2260.5798 | ˆσ=1.8817 |
Let ρ=0.3 and recall that the threshold u=906. According to the three pricing formulas obtained in Section 3, we are able to give the prices of earthquake reinsurance for different retentions, see Table 2. Table 2 shows that, the price of Cat XL contract decreases as the retention increases, which is consistent with reality. In addition, the price is higher under the standard deviation premium principle than under the net premium principle. Higher premiums can enhance reinsurers' willingness to cover catastrophe risks, and then raise the market capacity of catastrophe reinsurance. Therefore, the standard deviation premium principle is widely applied in pricing property and casualty (re)insurance, which is conducive to the diversification of catastrophe risks.
Price (MCY) | D<u | D=u | D>u | ||||
D=506 | D=706 | D=906 | D=1106 | D=1306 | |||
Net premium | 5742 | 5329 | 5001 | 4717 | 4457 | ||
Additional premium | 2526 | 2486 | 2448 | 2413 | 2378 | ||
Gross premium | 8268 | 7815 | 7449 | 7130 | 6835 |
Moreover, we study how the Cat XL contract prices are affected by the claim distribution. Table 3 shows that the differences between Cat XL contract prices in the GPD and lognormal distribution vary from 39.64% to 68.61%. This difference is especially marked in the tail (higer retention D), therefore the heavy-tailed distribution is a more suitable choice for modelling catastrophe claim.
D | 906 | 1306 | 2306 | 3306 |
Differences (MCY) | 2953 | 2963 | 3131 | 3305 |
Differences (%) | 39.64 | 43.34 | 55.38 | 68.61 |
In Figure 4, we illustrate how the Cat XL contract prices are affected by loading parameter ρ. It is obvious to observe that the price V is linear in ρ. When ρ increases by a factor of 5 (i.e., from 0.1 to 0.5), V increases by an approximate factor 1.5. This indicates that the choice of ρ has a major impact on the Cat XL contract prices. Namely, the standard deviation SD(Z) heavily influences the pricing results.
Due to the heavy-tailed characteristic of catastrophe losses, reinsurance companies covering Cat XL contracts face great risks, which leads to low willingness of reinsurance companies to underwrite catastrophe contracts. In order to improve the underwriting willingness, the price of a Cat XL contract is usually determinated by the standard deviation premium principle instead of net premium principle.
To capture the heavy tail characteristics of catastrophe risk, in this paper, we use the POT model to fit the catastrophic loss data. Based on this, we obtain explicit pricing formulas in term of three different relations between the retention and the threshold. Compared with the traditional catastrophe reinsurance pricing method, the standard deviation premium principle includes two aspects: the mathematical expectation of the catastrophe excess loss and the standard deviation. Computational results show that the choice of the fitted claim distribution has a major impact on the Cat XL contract prices. Also, the additional standard deviation is a significant factor when using the standard deviation premium principle, thereby giving higher and fairer Cat XL contract prices. Thus, we can conclude that the pricing method presented in this paper is effective and feasible.
Although this article only discusses the price of a Cat XL contract under the standard deviation premium principle, the results obtained here are also applicable to other type of catastrophe reinsurance contracts. For example, in the case of Cat XL contract with both the retention D1 and limit D2 (i.e., D1 xs D2 Cat XL contract), we can give the price of such Cat XL using the pricing formula of retention D1 minus the one of retention D2. In the other case of a mixed catastrophe reinsurance contract of proportional reinsurance and excess-of-loss, it is clear that the price can also be calculated with some slight modifications of the arguments presented in this paper. Therefore, our results have certain reference value in the pricing field of catastrophe reinsurance, which can be regarded as the extension and complement of the existed literatures.
The author would like to thank the unknown referees for their valuable suggestions. This work was supported by Fujian Social Science Foundation (No. FJ2021C084).
The author declares that there are no conflicts of interests.
[1] |
K. Antonio, R. Plat, Micro-level stochastic loss reserving for general insurance, Scand. Actuar. J., 2014 (2014), 113–145. http://dx.doi.org/10.2139/ssrn.1620446 doi: 10.2139/ssrn.1620446
![]() |
[2] |
A. A. Balkema, L. de Haan, Residual life time at great age, Ann. Probab., 2 (1974), 792–804. http://dx.doi.org/10.1214/aop/1176996548 doi: 10.1214/aop/1176996548
![]() |
[3] |
W. Chao, Valuing multirisks catastrophe reinsurance based on the Cox-Ingersoll-Ross (CIR) model, Discrete Dyn. Nat. Soc., 2021 (2021), 1–8. http://dx.doi.org/10.1155/2021/8818486 doi: 10.1155/2021/8818486
![]() |
[4] |
W. Chao, H. W. Zhou, Multiple-event catastrophe bond pricing based on Cox-Ingersoll-Ross model, Discrete Dyn. Nat. Soc., 2018 (2018), 1–9. http://dx.doi.org/10.1155/2018/5068480 doi: 10.1155/2018/5068480
![]() |
[5] |
S. H. Cox, H. W. Pedersen, Catastrophe risk bonds, N. Am. Actuar. J., 4 (2000), 56–82. http://dx.doi.org/10.1080/10920277.2000.10595938 doi: 10.1080/10920277.2000.10595938
![]() |
[6] | C. D. Daykin, T. Pentikäinen, M. Pesonen, Practical risk theory for actuaries, London: Chapman and Hall, 1994. |
[7] |
M. Egami, V. R. Young, Indifference prices of structured catastrophe (CAT) bonds, Insur. Math. Econ., 42 (2008), 771–778. http://dx.doi.org/10.1016/j.insmatheco.2007.08.004 doi: 10.1016/j.insmatheco.2007.08.004
![]() |
[8] |
E. Ekheden, O. Hössjer, Pricing catastrophe risk in life (re)insurance, Scand. Actuar. J., 4 (2014), 352–367. http://dx.doi.org/10.1080/03461238.2012.695747 doi: 10.1080/03461238.2012.695747
![]() |
[9] | P. Embrechts, C. Klüppelberg, T. Mikosch, Modeling extremal events for insurance and finance, Berlin: Springer, 1997. http://dx.doi.org/10.1007/978-3-642-33483-2 |
[10] |
S. Finken, C. Laux, Catastrophe bonds and reinsurance: The competitive effect of information-insensitive triggers, J. Risk Insur., 76 (2009), 579–605. http://dx.doi.org/10.1111/j.1539-6975.2009.01317.x doi: 10.1111/j.1539-6975.2009.01317.x
![]() |
[11] |
M. N. Giuricich, K. Burnecki, Modelling of left-truncated heavy-tailed data with application to catastrophe bond pricing, Phys. A: Stat. Mech. Appl., 525 (2019), 498–513. http://dx.doi.org/10.1016/j.physa.2019.03.073 doi: 10.1016/j.physa.2019.03.073
![]() |
[12] | M. Harbitz, Catastrophe covers in life assurance, In: Transactions of international congress of actuaries, 3 (1992), 109–119. http://dx.doi.org/10.1016/0167-6687(94)90441-3 |
[13] |
W. K. Härdle, B. L. Cabrera, Calibrating CAT bonds for Mexican earthquakes, J. Risk Insur., 77 (2010), 625–650. http://dx.doi.org/10.1111/j.1539-6975.2010.01355.x doi: 10.1111/j.1539-6975.2010.01355.x
![]() |
[14] |
N. Karagiannis, H. Assa, A. A. Pantelous, C. G. Turvey, Modelling and pricing of catastrophe risk bonds with a temperature-based agricultural application, Quant. Finance, 16 (2016), 1949–1959. http://dx.doi.org/10.1080/14697688.2016.1211791 doi: 10.1080/14697688.2016.1211791
![]() |
[15] |
M. Leppisaari, Modeling catastrophic deaths using EVT with a microsimulation approach to reinsurance pricing, Scand. Actuar. J., 2016 (2016), 113–145. http://dx.doi.org/10.1080/03461238.2014.910833 doi: 10.1080/03461238.2014.910833
![]() |
[16] | J. Liu, Y. Li, Empirical study on earthquake losses distribution and CAT bond pricing in China (in Chinese), Finance Trade Res., 20 (2009), 82–88. |
[17] |
Z. G. Ma, C. Q. Ma, S. T. Xiao, Pricing zero-coupon catastrophe bonds using EVT with doubly stochastic poisson arrivals, Discrete Dyn. Nat. Soc., 2017 (2017), 1–14. http://dx.doi.org/10.1155/2017/3279647 doi: 10.1155/2017/3279647
![]() |
[18] |
P. Nowak, M. Romaniuk, Pricing and simulations of catastrophe bonds, Insur. Math. Econ., 52 (2013), 18–28. http://dx.doi.org/10.1016/j.insmatheco.2012.10.006 doi: 10.1016/j.insmatheco.2012.10.006
![]() |
[19] |
J. Pickands, The two-dimensional possion process and external process, J. Appl. Probab., 8 (1971), 745–756. http://dx.doi.org/10.1017/s0021900200114640 doi: 10.1017/s0021900200114640
![]() |
[20] |
J. Pickands, Statistical inference using extreme order statistics, Ann. Stat., 3 (1975), 119–131. http://dx.doi.org/10.1214/aos/1176343003 doi: 10.1214/aos/1176343003
![]() |
[21] |
M. Pigeon, K. Antonio, M. Denuit, Individual loss reserving with the multivariate skew normal framework, Astin Bull., 43 (2013), 399–428. http://dx.doi.org/10.1017/asb.2013.20 doi: 10.1017/asb.2013.20
![]() |
[22] |
J. Shao, A. Papaioannou, A. Pantelous, Pricing and simulating catastrophe risk bonds in a Markov-dependent environment, Appl. Math. Comput., 309 (2017), 68–84. http://dx.doi.org/10.1016/j.amc.2017.03.041 doi: 10.1016/j.amc.2017.03.041
![]() |
[23] | P. Strickler, Rückversicherung des kumulrisikos in der lebensversicherung, In: XVI International Congress of Actuaries in Brussels, 1 (1960), 666–679. |
[24] |
D. A. Trottier, V. S. Lai, Reinsurance or CAT bond? How to optimally combine both, J. Fixed Income Fall, 27 (2017), 65–87. http://dx.doi.org/10.3905/jfi.2017.27.2.065 doi: 10.3905/jfi.2017.27.2.065
![]() |
[25] |
H. Q. Xiao, S. W. Meng, EVT and its application to pricing of catastrophe reinsurance (in Chinese), J. Appl. Stat. Manage., 32 (2013), 240–246. http://dx.doi.org/10.13860/j.cnki.sltj.2013.02.003 doi: 10.13860/j.cnki.sltj.2013.02.003
![]() |
[26] |
V. R. Young, Pricing in an incomplete market with an affine term stucture, Math. Finance, 14 (2004), 359–381. http://dx.doi.org/10.1111/j.0960-1627.2004.00195.x doi: 10.1111/j.0960-1627.2004.00195.x
![]() |
[27] |
A. A. Zimbidis, N. E. Frangos, A. A. Pantelous, Modeling earthquake risk via extreme value theory and pricing the respective catastrophe bonds, ASTIN Bull.: J. IAA, 37 (2007), 163–183. http://dx.doi.org/10.2143/AST.37.1.2020804 doi: 10.2143/AST.37.1.2020804
![]() |
1. | Hilda Azkiyah Surya, Herlina Napitupulu, , Double Risk Catastrophe Reinsurance Premium Based on Houses Damaged and Deaths, 2023, 11, 2227-7390, 810, 10.3390/math11040810 | |
2. | Hilda Azkiyah Surya, Herlina Napitupulu, , 2024, 2867, 0094-243X, 020027, 10.1063/5.0228856 |
GPD | Log-normal distribution | Poisson process | |
parameters | ˆξ=0.3303 | ˆμ=5.0350 | ˆλ=9.5185 |
ˆβ=2260.5798 | ˆσ=1.8817 |
Price (MCY) | D<u | D=u | D>u | ||||
D=506 | D=706 | D=906 | D=1106 | D=1306 | |||
Net premium | 5742 | 5329 | 5001 | 4717 | 4457 | ||
Additional premium | 2526 | 2486 | 2448 | 2413 | 2378 | ||
Gross premium | 8268 | 7815 | 7449 | 7130 | 6835 |
D | 906 | 1306 | 2306 | 3306 |
Differences (MCY) | 2953 | 2963 | 3131 | 3305 |
Differences (%) | 39.64 | 43.34 | 55.38 | 68.61 |
GPD | Log-normal distribution | Poisson process | |
parameters | ˆξ=0.3303 | ˆμ=5.0350 | ˆλ=9.5185 |
ˆβ=2260.5798 | ˆσ=1.8817 |
Price (MCY) | D<u | D=u | D>u | ||||
D=506 | D=706 | D=906 | D=1106 | D=1306 | |||
Net premium | 5742 | 5329 | 5001 | 4717 | 4457 | ||
Additional premium | 2526 | 2486 | 2448 | 2413 | 2378 | ||
Gross premium | 8268 | 7815 | 7449 | 7130 | 6835 |
D | 906 | 1306 | 2306 | 3306 |
Differences (MCY) | 2953 | 2963 | 3131 | 3305 |
Differences (%) | 39.64 | 43.34 | 55.38 | 68.61 |