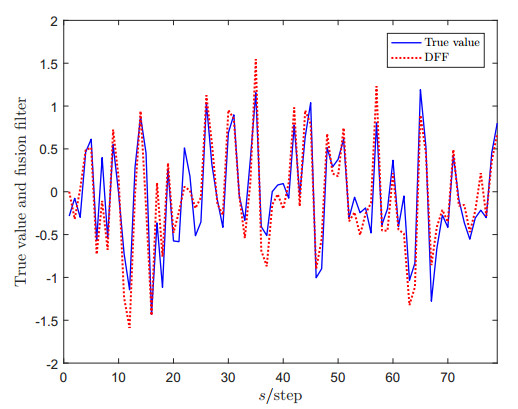
In this paper, the problem of optimized distributed fusion filtering is considered for a class of multi-sensor singular systems in the presence of fading measurements and stochastic nonlinearity. By utilizing the standard singular value decomposition, the multi-sensor stochastic singular systems are simplified to two reduced-order nonsingular subsystems (RONSs). The local filters (LFs) with corresponding error covariance matrices are proposed for RONSs via the innovation analysis approach. Then, on the basis of the matrix-weighted fusion estimation algorithm, the distributed fusion filters (DFFs) are designed for RONSs with multiple sensors in the linear minimum variance sense. Moreover, the DFFs are obtained by utilizing the state transformation for original singular systems. It can be observed that the DFFs have better accuracy in contrast with the LFs. Finally, an illustrate example is put forward to verify the feasibility of the proposed fusion filtering scheme.
Citation: Chen Wang, Jun Hu, Hui Yu, Dongyan Chen. Optimized distributed fusion filtering for singular systems with fading measurements and stochastic nonlinearity[J]. AIMS Mathematics, 2022, 7(2): 2543-2567. doi: 10.3934/math.2022143
[1] | Qian Li, Zhenghong Jin, Linyan Qiao, Aichun Du, Gang Liu . Distributed optimization of nonlinear singularly perturbed multi-agent systems via a small-gain approach and sliding mode control. AIMS Mathematics, 2024, 9(8): 20865-20886. doi: 10.3934/math.20241015 |
[2] | Weiwei Sun, Mengyang Qiu, Xinyu Lv . H∞ filter design for a class of delayed Hamiltonian systems with fading channel and sensor saturation. AIMS Mathematics, 2020, 5(4): 2909-2922. doi: 10.3934/math.2020188 |
[3] | Jiawen Li, Yi Zhang, Heung-wing Joseph Lee, Yingying Wang . Fuzzy tracking control of singular multi-agent systems under switching topology. AIMS Mathematics, 2024, 9(11): 29718-29735. doi: 10.3934/math.20241440 |
[4] | Andrey Borisov . Filtering of hidden Markov renewal processes by continuous and counting observations. AIMS Mathematics, 2024, 9(11): 30073-30099. doi: 10.3934/math.20241453 |
[5] | Biao Liu, Meiling Zhao . Synchronization and fluctuation of a stochastic coupled systems with additive noise. AIMS Mathematics, 2023, 8(4): 9352-9364. doi: 10.3934/math.2023470 |
[6] | Hamood Ur Rehman, Aziz Ullah Awan, Sayed M. Eldin, Ifrah Iqbal . Study of optical stochastic solitons of Biswas-Arshed equation with multiplicative noise. AIMS Mathematics, 2023, 8(9): 21606-21621. doi: 10.3934/math.20231101 |
[7] | Yonghong Duan, Ruiping Wen . An alternating direction power-method for computing the largest singular value and singular vectors of a matrix. AIMS Mathematics, 2023, 8(1): 1127-1138. doi: 10.3934/math.2023056 |
[8] | Nafissa T. Trouba, Huiying Xu, Mohamed E. M. Alngar, Reham M. A. Shohib, Haitham A. Mahmoud, Xinzhong Zhu . Soliton solutions and stability analysis of the stochastic nonlinear reaction-diffusion equation with multiplicative white noise in soliton dynamics and optical physics. AIMS Mathematics, 2025, 10(1): 1859-1881. doi: 10.3934/math.2025086 |
[9] | Yidan Wang, Li Xiao, Yanfeng Guo . Finite-time stability of singular switched systems with a time-varying delay based on an event-triggered mechanism. AIMS Mathematics, 2023, 8(1): 1901-1924. doi: 10.3934/math.2023098 |
[10] | Zhaohui Chen, Jie Tan, Yong He, Zhong Cao . Decentralized observer-based event-triggered control for an interconnected fractional-order system with stochastic Cyber-attacks. AIMS Mathematics, 2024, 9(1): 1861-1876. doi: 10.3934/math.2024091 |
In this paper, the problem of optimized distributed fusion filtering is considered for a class of multi-sensor singular systems in the presence of fading measurements and stochastic nonlinearity. By utilizing the standard singular value decomposition, the multi-sensor stochastic singular systems are simplified to two reduced-order nonsingular subsystems (RONSs). The local filters (LFs) with corresponding error covariance matrices are proposed for RONSs via the innovation analysis approach. Then, on the basis of the matrix-weighted fusion estimation algorithm, the distributed fusion filters (DFFs) are designed for RONSs with multiple sensors in the linear minimum variance sense. Moreover, the DFFs are obtained by utilizing the state transformation for original singular systems. It can be observed that the DFFs have better accuracy in contrast with the LFs. Finally, an illustrate example is put forward to verify the feasibility of the proposed fusion filtering scheme.
In recent years, increasing research attention has been devoted to the filtering or state estimation problems for the singular systems. It should be noted that the singular systems have more extensive description forms and widespread application domains compared with the normal systems, such as the electronic networks, chemical industry, biomedical sciences, robots and economic systems, and so on [1,2,3,4,5,6]. For example, the estimation problem for singular systems with single sensor has been studied in [7,8], including the filtering and smoothing problem. However, the estimation accuracy of the systems with single sensor would be affected by external interference and self-influence. Accordingly, the fusion estimation for singular systems with multiple sensors has long been one of the mainstream topics and a large number of literatures have been published to investigate the multi-sensor singular systems (MSSSs) [9,10].
At present, great efforts have been made to discuss the estimation problem for MSSSs. Generally, most of the studies rely on the generalization of classical system theory. For example, the issues of reduced-order fusion estimation for MSSSs have been studied in [11,12], where the singular systems have been converted into two subsystems with lower dimension than the original systems by means of the standard singular value decomposition methods [13,14]. In [15], the full-order fusion estimation problem has also been investigated for stochastic singular systems by transforming the singular systems into nonsingular systems. Furthermore, there are mainly two methods to estimate the system state, including the Kalman filtering method and the modern time series analysis method. For example, in [16,17,18,19], by utilizing the projection theory (PT) in [20], the optimal linear estimators have been presented in the sense of linear minimum variance (LMV). In [21], the optimal state estimation problem has been investigated for finite-field networks with stochastic disturbances in the sense of minimum mean square error. In [22], the local optimal estimators for the singular systems have been given by means of the autoregressive moving average innovation model method. For the sake of improving the accuracy of estimation, it is quite common that we need to fuse all measurement data coming from all sensors by employing the information fusion methods. In general, the information fusion methods have been presented in [23], including the state fusion and measurement fusion. The schemes of state fusion have the distributed fusion [24,25,26] and the centralized fusion [27]. In particular, the weighted measurement fusion scheme [31] has fused and weighted directly all measurement information to a lower-dimensional fusion measurement, then an individual fusion measurement information has been used to receive the fusion estimator. In addition, the distributed fusion approach has the advantages of expanding the communication and storage space requirements of the fusion center, and the input data rates can be improved due to the parallel structure. Moreover, when one of the sensors fails, this structure is convenient for detection and isolation. In view of the above advantages of distributed fusion structures, the problem of distributed fusion filtering is investigated in this study.
In practical networked systems, sensor aging or imperfect communication channels usually lead to fading measurements, which can lead to various distortions and information constraints [28,29,30,32]. Generally, the measurement signals may fade in a probabilistic way [33,34]. Obviously, the missing measurements may be considered as a special shape of fading measurements. In [35], a random variable obeying the Bernoulli distribution has been introduced to characterize the phenomenon of missing measurements. The missing measurements considered in [36] have different forms in [35], where the missing measurements have been modeled as a diagonal matrix that can be extensively applied to describe the multi-channel systems. In [37], the recursive filter has been designed for a stochastic system subject to multiple fading measurements, random parameter matrix and stochastic nonlinearity, where a diagonal matrix has been used to describe the phenomenon of multi-channel fading measurements. According to the methods in [35,36,37], a set of Bernoulli distributed random variables has been used to model the missing/fading measurements. However, it should be noted that only the case of single sensor has been considered. Recently, in [38], the issue of multi-sensor information fusion state estimation has been considered for random uncertain systems in the presence of unknown measurement disturbance and missing measurements, but it is only applicable for handling the nonsingular case. So far, the filtering problem for multi-sensor systems subject to fading measurements has not gained adequate attention, not to mention the singular systems with multiple sensors.
In addition, the nonlinearity inevitably exists in many practical systems, such as the telecommunication, neural networks and economics systems [39,40]. If not properly handled, the nonlinearity would influence the performance of the systems. Hence, a great number of results have been given to handle the nonlinear systems. For example, the problem of prescribed finite-time H∞ has been investigated in [41] for nonlinear singular systems with and without actuator saturation. In order to achieve prescribed finite-time stable for the closed-loop system, where the saturation nonlinearity has been decomposed into a linear form consisting of the control signal and its constant constraints. In addition, as a special kind of nonlinearity, the stochastic nonlinearity has received considerable attention. In [42], the issue of estimation with regard to the multi-step delays (MSDs) and packet dropouts (PDs) has been investigated for nonlinear stochastic systems. Then, the optimal linear estimators have been obtained by employing the innovation analysis approach (IAA). In [43], a distributed fusion filter (DFF), on the basis of the distributed matrix-weighted fusion algorithm (MWFA), has been proposed for stochastic nonlinear systems in the presence of MSDs and PDs, where the stochastic nonlinear effects come from the state equation and measurement equation. Unfortunately, up to now, very little research effort has been made on the fusion filtering problem in the presence of stochastic nonlinearity catering for multi-sensor circumstances, not to mention the case where multiple sensors may undergo the fading measurements phenomenon in singular systems.
Motivated by the above discussion, in this paper, we aim to deal with the fusion filtering problem for singular systems with fading measurements and stochastic nonlinearity. The problem seems to be significant owing to the following substantial challenges: (1) how to characterize the phenomenon of fading measurements with multiple channels; (2) how to select an appropriate way to convert the original singular systems into nonsingular systems; (3) how to obtain the local filters (LFs) and corresponding estimation error cross-covariances dependent on fading probability; (4) how to develop an effective distributed fusion algorithm so as to improve the accuracy of LFs. Accordingly, the main work of this paper is summarized as follows: (1) the fusion filtering issue is, for the first time, addressed for MSSSs in the presence of both fading measurements and stochastic nonlinearity; (2) the proposed LFs are optimal and unbiased in the sense of LMV; (3) the presented DFF weighted by matrices, which has the property of robustness and flexibility due to the parallel structure, has better precision than the LFs.
Notation In this paper, Is represents the unit matrix with dimension s. The superscript T represents the transpose of a matrix. tr(A) is used to describe the trace of matrix A. ⊥ denotes orthogonality. diag{A1,A2,⋯,AN} stands for a diagonal matrix with diagonal element Ai. δsh represents the Kronecker delta function. ⊙ is the Hadamard product.
In this paper, we consider the following class of nonlinear singular systems with N sensors:
MXs+1=ΦXs+f(Xs,ξs)+Υϖs, | (2.1) |
Yi,s=Ωi,sHiXs+ϑi,s,i=1,2,⋯,N | (2.2) |
where Xs∈Rn represents the state vector of the system, Yi,s∈Rmi (i=1,2,⋯,N) denote the measurement outputs, ϖs∈Rr is the zero-mean process noise, ϑi,s∈Rmi (i=1,2,⋯,N) are the zero-mean measurement noises. The subscript i represents the ith sensor. Ωi,s=diag{αi1,s,⋅⋅⋅,αimi,s} (i=1,2,⋯,N) characterize the phenomenon of fading measurements with multiple channels, where αik,s (k=1,2,⋯,mi) are mi random variables, which are uncorrelated in s as well as k. The random variable αik,s represents the fading situation of the kth measurement channel, and the probability density function pik,s(l) is on the interval [0, 1] with known mathematical expectation μik,s and variance σ2ik,s. The function f(Xs,ξs) is the stochastic nonlinearity, where ξs is a zero-mean Gaussian white noise. M, Φ, Υ and Hi are constant matrices with proper dimensions.
To begin, the following assumptions are introduced.
Assumption 1. M is a singular square matrix, i.e., rank(M)=n1<n.
Assumption 2. System (2.1) is regular, i.e., det(κM−Φ)≠0, where κ is an arbitrary complex number.
Assumption 3. rank(Φ)≥n2 and n1+n2=n.
Assumption 4. ϖs and ϑi,s (i=1,2,⋯,N) have the following statistical properties:
E{[ϖsϑi,s][ϖThϑTi,h]}=[Qϖ,s00Qϑi,s]δsh,E{ϑi,sϑTj,h}=0,(i≠j,∀s,h), |
where δsh is Kronecker function, that is to say
δsh={1s=h0s≠h. |
Assumption 5. The initial state X0, ϖs, ϑi,s, ξs and αik,s (i=1,2,⋯,N;k=1,2,⋯,mi) are mutually independent, moreover, E{X0}=π0 and E{(X0−π0)(X0−π0)T}=P0.
Assumption 6. The function f(Xs,ξs) satisfies
E{f(Xs,ξs)∣Xs}=0,E{f(Xs,ξs)fT(Xh,ξh)∣Xs}=m∑l=1ΠlXTsΓlXsδsh, |
where m is a known positive integer, Πl and Γl (l=1,2,⋯,m) are given matrices with appropriate dimensions.
Next, the key idea is to transform the original singular systems into two reduced-order nonsingular subsystems (RONSs) by using the singular value decomposition method. Then, the LFs for each sensor are presented in the sense of LMV via the PT. In the fusion center, the DFFs are presented for RONSs by utilizing the MWFA. Finally, we get the DFF for original singular systems.
For the convenience of subsequent developments, we introduce the following Lemma.
Lemma 1. [44] Let A=[aij]p×p be a real matrix and Q=diag{ϱ1,ϱ2,⋯,ϱp} be a diagonal random matrix. Then
E{QAQT}=[E{ϱ21}E{ϱ1ϱ2}⋯E{ϱ1ϱp}E{ϱ2ϱ1}E{ϱ22}⋯E{ϱ2ϱp}⋮⋮⋱⋮E{ϱpϱ1}E{ϱpϱ2}⋯E{ϱ2p}]⊙A, |
where ⊙ is the Hadamard product.
In this section, our goal is to provide an innovative scheme to deal with the fusion filtering problem for singular systems with fading measurements and stochastic nonlinearity, that is to say, we need to find the DFF ˆX0,s|s on the basis of the measurements (Yi,s,⋯,Yi,0) (i=1,⋯,N).
For systems (2.1) and (2.2) under Assumptions 1-3, according to [14], there exists nonsingular matrices U and R such that
UMR=[M10M20],UΦR=[Φ10Φ2Φ3],UΥ=[Υ1Υ2],HiR=[H(1)iH(2)i],U=[U1U2], |
where M1∈Rn1×n1 is nonsingular lower-triangular, Φ1∈Rn1×n1 is quasi-lower-triangular, Φ3∈Rn2×n2 is nonsingular lower-triangular, other matrix blocks have corresponding dimensions. By using the transformation Xs=R[XT1,sXT2,s]T with X1,s∈Rn1 and X2,s∈Rn2, the singular systems (2.1) and (2.2) are converted into the following systems:
X1,s+1=ˉΦX1,s+ˉUf(Xs,ξs)+ˉΓϖs, | (3.1) |
X2,s=BX1,s+Cf(Xs,ξs)+Dϖs, | (3.2) |
Yi,s=Ωi,s¯HiX1,s+Ωi,sEif(Xs,ξs)+ηi,s,i=1,2,⋯,N | (3.3) |
with
ˉΦ=M−11Φ1,ˉU=M−11U1,ˉΓ=M−11Υ1,B=Φ−13M2M−11Φ1−Φ−13Φ2,C=Φ−13M2M−11U1−Φ−13U2,D=Φ−13M2M−11Υ1−Φ−13Υ2,¯Hi=H(1)i+H(2)i(Φ−13M2M−11Φ1−Φ−13Φ2),Ei=H(2)i(Φ−13M2M−11U1−Φ−13U2),Fi=H(2)i(Φ−13M2M−11Υ1−Φ−13Υ2),ηi,s=Ωi,sFiϖs+ϑi,s. |
The noise sequences ϖs and ηi,s obey
E{[ϖsηi,s][ϖThηTj,h]}=[Qϖ,sSj,sSTi,sQηij,s]δsh, |
especially when i=j, we define Qηii,s=Qηi,s, where
Sj,s=E{ϖsηTj,s}=Qϖ,sFTjˉΩTj,s,Qηi,s=E{ηi,sηTi,s}=˜Ωi,s⊙(FiQϖ,sFTi)+Qϑi,s,Qηij,s=E{ηi,sηTj,s}=→Ωij,s⊙(FiQϖ,sFTj),(i≠j) |
with
ˉΩi,s=E{Ωi,s}=diag{μi1,s,μi2,s,⋯,μimi,s},˜Ωi,s=[σ2i1,s+μ2i1,sμi1,sμi2,s⋯μi1,sμimi,s∗σ2i2,s+μ2i2,s⋯μi2,sμimi,s⋮⋮⋱⋮∗∗⋯σ2imi,s+μ2imi,s],→Ωij,s=[μi1,sμj1,sμi1,sμj2,s⋯μi1,sμjmj,s∗μi2,sμj2,s⋯μi2,sμjmj,s⋮⋮⋱⋮∗∗⋯μimi,sμjmj,s]. |
Remark 1. For the filtering problem of singular systems, the usual way is to convert the singular systems into nonsingular systems under the different assumptions. In [9], the full-order nonsingular systems have been introduced under the assumption that the systems are completely observable. Furthermore, a singular value decomposition method has been presented in [13], where the RONS have been obtained under the causal assumption. In addition, it should be noted that a fast-slow subsystem decomposition approach has been employed in [45] when the system is not causal. Accordingly, similar to [14] without the causal assumption and observability assumption, the singular systems (2.1) and (2.2) in this paper are converted into RONSs (3.1), (3.2) and (3.3). Moreover, it should be noted that it is not easy to estimate the system state due to the existence of the stochastic nonlinear effects.
To proceed, we introduce the following assumption.
Assumption 7. Matrices Γi, Πj(i,j=1,2,⋯,m), C and R satisfy det(Ψ−Im)≠0, where
Ψ=[tr(ΓiΘj)]m×m,Θj=R[000CΠjCT]RT. |
Lemma 2. For the original systems (2.1) and (2.2) under the conditions of Assumptions 1-6, the second-order moment matrix qXs+1=E{Xs+1XTs+1} of state Xs+1 can be calculated by:
qXs+1=R[E{X1,s+1XT1,s+1}E{X1,s+1XT2,s+1}E{X2,s+1XT1,s+1}E{X2,s+1XT2,s+1}]RT, | (3.4) |
where
qX1,s+1=E{X1,s+1XT1,s+1}=ˉΦqX1,sˉΦT+ˉUm∑l=1Πltr(qXsΓl)ˉUT+ˉΓQϖ,sˉΓT, | (3.5) |
qX12,s+1=E{X1,s+1XT2,s+1}=qX1,s+1BT, | (3.6) |
qX2,s+1=E{X2,s+1XT2,s+1}=BqX1,s+1BT+Cm∑l=1Πltr(qXs+1Γl)CT+DQϖ,s+1DT. | (3.7) |
The initial value is qX0=π0πT0+P0.
Proof. Substituting Xs+1=R[XT1,s+1XT2,s+1]T into the qXs+1=E{Xs+1XTs+1}, we can obtain (3.4). Then, substituting (3.1) into the qX1,s+1=E{X1,s+1XT1,s+1} and using Assumptions 4-6 and X1,s⊥ϖs, we can obtain
qX1,s+1=ˉΦE{X1,sXT1,s}ˉΦT+ˉUE{f(Xs,ξs)fT(Xs,ξs)}ˉUT+ˉΓE{ϖsϖTs}ˉΓT+{ˉΦE{X1,sfT(Xs,ξs)}ˉUT+ˉΦE{X1,sϖTs}ˉΓT+ˉUE{f(Xs,ξs)ϖTs}ˉΓT}+{∗}T=ˉΦqX1,sˉΦT+ˉUm∑l=1Πltr(qXsΓl)ˉUT+ˉΓQϖ,sˉΓT. | (3.8) |
In the equation (3.8), the term {∗} represents the same item as the front neighboring term. Similarly, substituting (3.1) and (3.2) into qX12,s+1=E{X1,s+1XT2,s+1} and substituting (3.2) into qX2,s+1=E{X2,s+1XT2,s+1}, we can obtain (3.6) and (3.7).
Remark 2. From Lemma 2, we can see that the second-order moment matrix qXs can not computed recursively due to the fact that qX2,s contains ∑ml=1Πltr(qXsΓl). But in the subsequent calculations, it only needs to iterate ∑ml=1Πltr(qXsΓl), therefore, our objective is to solve it from equation (3.4). The calculation process is shown in the Appendix.
Lemma 3. For the ith RONSs (3.1), (3.2) and (3.3) under the conditions of Assumptions 4-6, the innovation ei,s=Yi,s−ˆYi,s|s−1 is calculated by
ei,s=(Ωi,s−ˉΩi,s)ˉHiX1,s+Ωi,sEif(Xs,ξs)+ηi,s+ˉΩi,sˉHi˜X(1)i,s|s−1. | (3.9) |
The covariance Qei,s=E{ei,seTi,s} of the innovation ei,s is calculated by
Qei,s=˘Ωi,s⊙(ˉHiqX1,sˉHTi)+˜Ωi,s⊙(Eim∑l=1Πltr(qXsΓl)ETi)+Qηi,s+ˉΩi,sˉHiP(1)i,s|s−1ˉHTiˉΩTi,s, | (3.10) |
where ˘Ωi,s=diag{σ2i1,s,⋯,σ2imi,s}. The cross-covariance Qeij,s=E{ei,seTj,s}(i≠j) of the innovation ei,s and ej,s is computed by
Qeij,s=→Ωij,s⊙(Eim∑l=1Πltr(qXsΓl)ETj)+Qηij,s+ˉΩi,sˉHiP(1)ij,s|s−1ˉHTjˉΩTj,s. | (3.11) |
Proof. According to the PT, we can easily obtain ˆηi,s|s−1=0. Then, we have
ei,s=Yi,s−ˆYi,s|s−1=Ωi,sˉHiX1,s+Ωi,sEif(Xs,ξs)+ηi,s−ˉΩi,sˉHiˆX(1)i,s|s−1=(Ωi,s−ˉΩi,s)ˉHiX1,s+Ωi,sEif(Xs,ξs)+ηi,s+ˉΩi,sˉHi˜X(1)i,s|s−1. | (3.12) |
Substituting (3.12) into Qei,s=E{ei,seTi,s} yields
Qei,s=E{(Ωi,s−ˉΩi,s)ˉHiX1,sXT1,sˉHTi(Ωi,s−ˉΩi,s)T}+E{Ωi,sEif(Xs,ξs)fT(Xs,ξs)ETiΩTi,s}+E{ηi,sηTi,s}+E{ˉΩi,sˉHi˜X(1)i,s|s−1˜X(1)Ti,s|s−1ˉHTiˉΩTi,s}+{E{(Ωi,s−ˉΩi,s)ˉHiX1,sfT(Xs,ξs)ETiΩTi,s}+E{(Ωi,s−ˉΩi,s)ˉHiX1,sηTi,s}+E{(Ωi,s−ˉΩi,s)ˉHiX1,s˜X(1)Ti,s|s−1ˉHTiˉΩTi,s}+E{Ωi,sEif(Xs,ξs)ηTi,s}+E{Ωi,sEif(Xs,ξs)˜X(1)Ti,s|s−1ˉHTiˉΩTi,s}+E{ηi,s˜X(1)Ti,s|s−1ˉHTiˉΩTi,s}}+{∗}T. | (3.13) |
By utilizing E{Ωi,s−ˉΩi,s}=0, Assumptions 4-6 and E{˜Xi,s|s−1ηTi,s}=0, we can obtain (3.10). Similarly, we also obtain (3.11).
Remark 3. Based on the above discussion, we see that the Hadamard product has been mainly used in Lemma 3. Note that the covariance Qei,s and the cross-covariance Qeij,s of innovation can not be derived directly since the innovation ei,s contains a diagonal-matrix with random variable that is different from the single random variable. Before the derivation of the LFs, we give the Lemma 3 firstly by the following Lemma 1, where the Hadamard product makes the expression more concise in taking the expectations on the products of some random matrices.
Lemma 4. For the convenience of subsequent computations, we get
E{X1,seTi,s}=P(1)i,s|s−1ˉHTiˉΩTi,s, | (3.14) |
E{ϖseTi,s}=Si,s, | (3.15) |
E{f(Xs,ξs)eTi,s}=m∑l=1Πltr(qXsΓl)ETiˉΩTi,s. | (3.16) |
Proof. Substituting (3.9) into E{X1,seTi,s} and using E{Ωi,s−ˉΩi,s}=0, X1,s⊥ηi,s and ˆX(1)i,s|s−1⊥˜X(1)i,s|s−1, we can obtain
E{X1,seTi,s}=E{X1,sXT1,sˉHTi(Ωi,s−ˉΩi,s)T}+E{X1,sfT(Xs,ξs)ETiΩTi,s}+E{X1,sηTi,s}+E{X1,s˜X(1)Ti,s|s−1ˉHTiˉΩTi,s}=E{˜X(1)i,s|s−1˜X(1)Ti,s|s−1}ˉHTiˉΩTi,s=P(1)i,s|s−1ˉHTiˉΩTi,s. |
Substituting (3.9) into E{ϖseTi,s} and using ϖs⊥˜X(1)i,s|s−1, we can easily get (3.15). Similarly, we can prove (3.16).
Lemma 5. For the RONSs (3.1), (3.2) and (3.3) under the conditions of Assumptions 4-6, the covariance matrices P(1)iXϖ,s|s, P(1)ijXϖ,s|s,(i≠j), Piϖ,s|s and Pijϖ,s|s,(i≠j) between the noise and the state are, respectively, computed by
P(1)iXϖ,s|s=E{˜X(1)i,s|s˜ϖTi,s|s}=−K(1)i,s|sQei,sKTiϖ,s|s, | (3.17) |
P(1)ijXϖ,s|s=E{˜X(1)i,s|s˜ϖTj,s|s}=K(1)i,s|sQeij,sKTjϖ,s|s−K(1)j,s|sQej,sKTjϖ,s|s−K(1)i,s|sQei,sKTiϖ,s|s, | (3.18) |
Piϖ,s|s=E{˜ϖi,s|s˜ϖTi,s|s}=Qϖ,s−Kiϖ,s|sQei,sKTiϖ,s|s, | (3.19) |
Pijϖ,s|s=E{˜ϖi,s|s˜ϖTj,s|s}=Qϖ,s−Kiϖ,s|sQei,sKTiϖ,s|s−Kjϖ,s|sQej,sKTjϖ,s|s+Kiϖ,s|sQeij,sKTjϖ,s|s, | (3.20) |
where the noise estimator ˆϖi,s|s is calculated by
ˆϖi,s|s=Kiϖ,s|sei,s, | (3.21) |
the gain matrix is expressed as follows:
Kiϖ,s|s=Si,sQ−1ei,s. | (3.22) |
Proof. Let PXY,∘|∙ be the covariance among errors ˜X∘|∙ and ˜Y∘|∙. Based on the PT, we obtain ˆX∘|∙⊥˜Y∘|∙ and ˆY∘|∙⊥˜X∘|∙. We have PXY,∘|∙=E{˜X∘|∙˜YT∘|∙}=E{X∘˜YT∘|∙}=E{˜X∘|∙YT∘}. So, by using X1,s⊥ϖs, we have
P(1)iXϖ,s|s=E{˜X(1)i,s|s˜ϖTi,s|s}=E{X1,s˜ϖTi,s|s}=−E{X1,sˆϖTi,s|s}. | (3.23) |
Then, we obtain (3.21) easily, where Kiϖ,s|s=E{ϖseTi,s}Q−1ei,s. Substituting (3.21) into (3.23), and using K(1)i,s|s=E{X1,seTi,s}Q−1ei,s, we obtain (3.17). Similarly, we can prove (3.18), (3.19) and (3.20).
Theorem 1. For the RONSs (3.1), (3.2) and (3.3) under the conditions of Assumptions 4-6, the LFs and one-step predictors for the states X1,s and X2,s are given by
ˆX(1)i,s|s=ˆX(1)i,s|s−1+K(1)i,s|sei,s, | (3.24) |
ˆX(1)i,s+1|s=ˉΦˆX(1)i,s|s+ˉUKif,s|sei,s+ˉΓˆϖi,s|s, | (3.25) |
ˆX(2)i,s|s=ˆX(2)i,s|s−1+K(2)i,s|sei,s, | (3.26) |
ˆX(2)i,s+1|s=BˆX(1)i,s+1|s, | (3.27) |
where the gain matrices K(1)i,s|s, K(2)i,s|s and Kif,s|s are, respectively, expressed by
K(1)i,s|s=P(1)i,s|s−1ˉHTiˉΩTi,sQ−1ei,s, | (3.28) |
Kif,s|s=m∑l=1Πltr(qXsΓl)ETiˉΩTi,sQ−1ei,s, | (3.29) |
K(2)i,s|s=[BP(1)i,s|s−1ˉHTiˉΩTi,s+Cm∑l=1Πltr(qXsΓl)ETiˉΩTi,s+DSi,s]Q−1ei,s. | (3.30) |
The filtering error covariance (FEC) P(1)i,s|s and the prediction error covariance (PEC) P(1)i,s+1|s for state X1,s are computed as
P(1)i,s|s=P(1)i,s|s−1−K(1)i,s|sQei,sK(1)Ti,s|s, | (3.31) |
P(1)i,s+1|s=ˉΦP(1)i,s|sˉΦT+ˉUm∑l=1Πltr(qXsΓl)ˉUT+ˉΓPiϖ,s|sˉΓT+ˉUKif,s|sQei,sKTif,s|sˉUT+{−ˉΦK(1)i,s|sˉΩi,sEim∑l=1Πltr(qXsΓl)ˉUT+ˉΦP(1)iXϖ,s|sˉΓT−ˉUm∑l=1Πltr(qXsΓl)ETiˉΩTi,sKTiϖ,s|sˉΓT−ˉUm∑l=1Πltr(qXsΓl)ETiˉΩTi,sKTif,s|sˉUT}+{∗}T. | (3.32) |
The filtering error cross-covariance (FECC) P(1)ij,s|s and the prediction error cross-covariance (PECC) P(1)ij,s+1|s among the ith and the jth sensor (i≠j) for state X1,s are computed as
P(1)ij,s|s=P(1)ij,s|s−1−P(1)ij,s|s−1ˉHTjˉΩTj,sK(1)Tj,s|s−K(1)i,s|sˉΩi,sˉHiP(1)ij,s|s−1+K(1)i,s|sQeij,sK(1)Tj,s|s, | (3.33) |
P(1)ij,s+1|s=ˉΦP(1)ij,s|sˉΦT+ˉUm∑l=1Πltr(qXsΓl)ˉUT+ˉΓPijϖ,s|sˉΓT+ˉUKif,s|sQeij,sKTjf,s|sˉUT−ˉΦK(1)i,s|sˉΩi,sEim∑l=1Πltr(qXsΓl)ˉUT+ˉΦP(1)ijXϖ,s|sˉΓT−ˉΦP(1)ij,s|s−1ˉHTjˉΩTj,sKTjf,s|sˉUT+ˉΦK(1)i,s|sQeij,sKTjf,s|sˉUT−ˉUm∑l=1Πltr(qXsΓl)ETjˉΩTj,sKTjϖ,s|sˉΓT−ˉUm∑l=1Πltr(qXsΓl)ETjˉΩTj,sKTjf,s|sˉUT−ˉΓKjϖ,s|sQej,sKTjf,s|sˉUT+ˉΓKiϖ,s|sQeij,sKTjf,s|sˉUT−ˉUm∑l=1Πltr(qXsΓl)ETjˉΩTj,sK(1)Tj,s|sˉΦT+ˉΓP(1)ijϖX,s|sˉΦT−ˉUKif,s|sˉΩi,s|sˉHiP(1)ij,s|s−1ˉΦT+ˉUKif,s|sQeij,sK(1)Tj,s|sˉΦT−ˉΓKiϖ,s|sˉΩi,sEim∑l=1Πltr(qXsΓl)ˉUT−ˉUKif,s|sˉΩi,sEim∑l=1Πltr(qXsΓl)ˉUT−ˉUKif,s|sQei,sKTiϖ,s|sˉΓT+ˉUKif,s|sQeij,sKTjϖ,s|sˉΓT. | (3.34) |
The FEC P(2)i,s|s and the FECC P(2)ij,s|s among the ith and the jth sensor (i≠j) for state X2,s are computed as
P(2)i,s|s=BP(1)i,s|s−1BT+Cm∑l=1Πltr(qXsΓl)CT+DQϖ,sDT+K(2)i,s|sQei,sK(2)Ti,s|s+{−BK(1)i,s|sQei,sK(2)Ti,s|s−Cm∑l=1Πltr(qXsΓl)ETiˉΩTi,sK(2)Ti,s|s−DSi,sK(2)Ti,s|s}+{∗}T, | (3.35) |
P(2)ij,s|s=BP(1)ij,s|s−1BT+Cm∑l=1Πltr(qXsΓl)CT+DQϖ,sDT+K(2)i,s|sQeij,sK(2)Tj,s|s−BP(1)ij,s|s−1ˉHTjˉΩTj,sK(2)Tj,s|s−Cm∑l=1Πltr(qXsΓl)ETjˉΩTj,sK(2)Tj,s|s−DSj,sK(2)Tj,s|s−K(2)i,s|sˉΩi,sˉHiP(1)ij,s|s−1BT−K(2)i,s|sˉΩi,sEim∑l=1Πltr(qXsΓl)CT−K(2)i,s|sSTi,sDT. | (3.36) |
The correlation matrices P(1,2)i,s|s and P(1,2)ij,s|s (i≠j) between the ˜X(1)i,s|s and the ˜X(2)j,s|s are computed as
P(1,2)i,s|s=P(1)i,s|s−1BT−K(1)i,s|sQei,sK(1)Ti,s|sBT+P(1)iXϖ,s|sDT−K(1)i,s|sˉΩi,sEim∑l=1Πltr(qXsΓl)CT, | (3.37) |
P(1,2)ij,s|s=P(1)ij,s|s−1BT−K(1)i,s|sˉΩi,sˉHiP(1)ij,s|s−1BT+P(1)iXϖ,s|sDT−K(1)i,s|sˉΩi,sEim∑l=1Πltr(qXsΓl)CT−P(1)ij,s|s−1ˉHTjˉΩTj,sK(2)Tj,s|s+K(1)i,s|sQeij,sK(2)Tj,s|s. | (3.38) |
The initial values are ˆX(1)i,0|−1=[In10]R−1π0 and P(1)i,0|−1=P(1)ij,0|−1=[In10]R−1P0(RT)−1[In10]T.
Proof. According to the PT, we obtain (3.24), (3.25), (3.26) and (3.27), where K(1)i,s|s, Kif,s|s, K(2)i,s|s are gain matrices. Substituting (3.14) and (3.16) into the gain matrices K(1)i,s|s=E{X1,seTi,s}Q−1ei,s and Kif,s|s=E{f(Xs,ξs)eTi,s}Q−1ei,s, respectively, we obtain (3.28) and (3.29) immediately. Substituting (3.2) into K(2)i,s|s=E{X2,seTi,s}Q−1ei,s, we can obtain
K(2)i,s|s=[BE{X1,seTi,s}+CE{f(Xs,ξs)eTi,s}+DE{ϖseTi,s}]Q−1ei,s, | (3.39) |
then, substituting (3.14), (3.15) and (3.16) into (3.39), we can easily obtain (3.30).
From (3.25), the prediction error can be obtained for the state X1,s as follows:
˜X(1)i,s+1|s=ˉΦ˜X(1)i,s|s+ˉUf(Xs,ξs)+ˉΓ˜ϖi,s|s−ˉUKif,s|sei,s. | (3.40) |
Substituting (3.40) into the PEC P(1)i,s+1|s=E{˜X(1)i,s+1|s˜X(1)Ti,s+1|s} for state X1,s, we get
P(1)i,s+1|s=ˉΦP(1)i,s|sˉΦT+ˉUE{f(Xs,ξs)fT(Xs,ξs)}ˉUT+ˉΓPiϖ,s|sˉΓT+ˉUKif,s|sQei,sKTif,s|sˉUT+{ˉΦE{˜X(1)i,s|sfT(Xs,ξs)}ˉUT+ˉΦP(1)iXϖ,s|sˉΓT−ˉΦE{˜X(1)i,s|seTi,s}KTif,s|sˉUT+ˉUE{f(Xs,ξs)˜ϖTi,s|s}ˉΓT−ˉUE{f(Xs,ξs)eTi,s}KTif,s|sˉUT−ˉΓE{˜ϖi,s|seTi,s}KTif,s|sˉUT}+{∗}T. | (3.41) |
From (3.24), we have the local filtering error equation for state X1,s as follows:
˜X(1)i,s|s=X1,s−ˆX(1)i,s|s=˜X(1)i,s|s−1−K(1)i,s|sei,s. | (3.42) |
Therefore, by using (3.16) and (3.42), we obtain
E{˜X(1)i,s|sfT(Xs,ξs)}=−K(1)i,s|sˉΩi,sEim∑l=1Πltr(qXsΓl). | (3.43) |
Moreover, by using (3.21), we have
E{f(Xs,ξs)˜ϖTi,s|s}=−m∑l=1Πltr(qXsΓl)ETiˉΩTi,sKTiϖ,s|s. | (3.44) |
Then, by using (3.14), ˆX(1)i,s|s−1⊥ei,s and K(1)i,s|s=E{X1,seTi,s}Q−1ei,s, we obtain
E{˜X(1)i,s|seTi,s}=0. | (3.45) |
Similarly, by using (3.15) and (3.22), we arrive at
E{˜ϖi,s|seTi,s}=0. | (3.46) |
Subsequently, substituting (3.16), (3.43), (3.44), (3.45) and (3.46) into (3.41), we get (3.32).
Substituting (3.42) into the FEC P(1)i,s|s=E{˜X(1)i,s|s˜X(1)Ti,s|s}, we have
P(1)i,s|s=P(1)i,s|s−1−E{˜X(1)i,s|s−1eTi,s}K(1)Ti,s|s−K(1)i,s|sE{ei,s˜X(1)Ti,s|s−1}+K(1)i,s|sQei,sK(1)Ti,s|s. | (3.47) |
Next, by using ˆX(1)i,s|s−1⊥ei,s and K(1)i,s|s=E{X1,seTi,s}Q−1ei,s, we obtain (3.31) from (3.47). Similarly, we can prove (3.33) and (3.34).
From (3.2) and (3.26), we get the local filtering error of state X2,s as follows:
˜X(2)i,s|s=B˜X(1)i,s|s−1+Cf(Xs,ξs)+Dϖs−K(2)i,s|sei,s. | (3.48) |
Then, substituting (3.48) into the FEC P(2)i,s|s=E{˜X(2)i,s|s˜X(2)Ti,s|s}, and utilizing E{˜X(1)i,s|s−1eTj,s}=P(1)ij,s|s−1ˉHTjˉΩTj,s, we get (3.35) easily. Similarly, we can prove (3.36).
Substituting (3.48) into P(1,2)i,s|s=E{˜X(1)i,s|s˜X(2)Ti,s|s}, we can obtain
P(1,2)i,s|s=E{˜X(1)i,s|s˜X(1)Ti,s|s−1}BT+E{˜X(1)i,s|sfT(Xs,ξs)}CT+E{˜X(1)i,s|sϖTs}DT−E{˜X(1)i,s|seTi,s}K(2)Ti,s|s. | (3.49) |
Moreover, by using (3.42), ˆX(1)i,s|s−1⊥ei,s and ˜X(1)i,s|s⊥ˆϖi,s|s, we can easily get
E{˜X(1)i,s|s˜X(1)Ti,s|s−1}=P(1)i,s|s−1−K(1)i,s|sQei,sK(1)Ti,s|s, | (3.50) |
E{˜X(1)i,s|sϖs}=P(1)iXϖ,s|s. | (3.51) |
Then, substituting (3.43), (3.45), (3.50) and (3.51) into (3.49), we get (3.37).
Substituting (3.48) into P(1,2)ij,s|s=E{˜X(1)i,s|s˜X(2)Tj,s|s}, we can get
(1,2)ij,s|s=E{˜X(1)i,s|s˜X(1)Tj,s|s−1}BT+E{˜X(1)i,s|sfT(Xs,ξs)}CT+E{˜X(1)i,s|sϖTs}DT−E{˜X(1)i,s|seTj,s}K(2)Tj,s|s. | (3.52) |
Then, we have
E{˜X(1)i,s|s˜X(1)Tj,s|s−1}=P(1)ij,s|s−1−K(1)i,s|sˉΩi,sˉHiP(1)ij,s|s−1, | (3.53) |
E{˜X(1)i,s|seTj,s}=P(1)ij,s|s−1ˉHTjˉΩTj,s−K(1)i,s|sQeij,s. | (3.54) |
Therefore, substituting (3.43), (3.51), (3.53) and (3.54) into (3.52), we can prove (3.38).
Remark 4. From Theorem 1, we have derived the optimal LFs on the basis of the IAA, which is a common method to obtain the optimal linear estimate in the sense of LMV. The existing results in [37], [43] and [46] with stochastic nonlinearity and fading measurements have been generalized in this paper. We can see that the proposed LFs are dependent on the noise filters, which is different from the suboptimal Kalman-type recursive filter in [37]. Moreover, it should be noted that the filters in [43] and [46] also have been presented by utilizing the IAA. However, the above literatures only take normal systems into consideration. Hence, in this paper, we have considered the fusion filtering problem for MSSSs with stochastic nonlinearity and fading measurements by employing the IAA.
In the fusion center, based on the LFs and covariance matrices proposed above, we have the following reduced-order DFFs for two RONSs (3.1), (3.2) and (3.3) by using the MWFA.
Theorem 2. For RONSs (3.1), (3.2) and (3.3), we have the reduced-order DFFs as follows:
ˆX(k)0,s|s=Λk,sˆℵk,s|s,k=1,2 |
where Λk,s=(ϵTnkΣ−1k,s|sϵnk)−1ϵTnkΣ−1k,s|s, ˆℵk,s|s=[ˆX(k)T1,s|sˆX(k)T2,s|s⋯ˆX(k)TN,s|s]T ϵnk=[Ink⋯Ink]T, Σk,s|s=[P(k)ij,s|s]Nnk×Nnk, whose nk×nk sub-block in the (i,j) place is P(k)ij,s|s. The covariance of ˆX(k)0,s|s are computed by
P(k)0,s|s=(ϵTnkΣ−1k,s|sϵnk)−1, |
and we have P(k)0,s|s≤P(k)i,s|s(i=1,2,⋯,N).
Proof. From the optimal fusion estimation algorithm [26], the proof is complete.
Theorem 3. For the original systems (2.1) and (2.2), the DFF has the following form:
ˆX0,s|s=R[ˆX(1)T0,s|sˆX(2)T0,s|s]T. |
The covariance of ˆX0,s|s is calculated by
P0,s|s=R[P(1)0,s|sP(1,2)0,s|sP(2,1)0,s|sP(2)0,s|s]RT, |
where the FEC P(1,2)0,s|s between ˜X(1)0,s|s and ˜X(2)0,s|s is calculated by
P(1,2)0,s|s=(ϵTn1Σ−11,s|sϵn1)−1ϵTn1Σ−11,s|sΣ12,s|sΣ−12,s|sϵn2(ϵTn2Σ−12,s|sϵn2)−1, |
where P(1,2)0,s|s=P(2,1)T0,s|s, Σ12,s|s=[P(1,2)ij,s|s]Nn1×Nn2.
Proof. From the transformation Xs=R[XT1,sXT2,s]T, we can prove it easily.
Remark 5. So far, we have encountered some obstacles in deriving the distributed matrix-weighted fusion filter. For example, (1) how to handle the diagonal-matrix with random variable exists in the measurement equations; (2) how to derive the FECC between any two LFs by using the IAA. In order to overcome these difficulties, the Hadamard product has been introduced to make the calculation more convenient in taking the expectations on the products of some random matrices. In addition, the FECCs are obtained by solving the cross-covariance matrices between state and noises, innovation and state.
The distributed fusion filtering algorithm for the singular systems can be given by the following steps:
Step 1. Set the initial values ˆX(1)i,0|−1=[In10]R−1π0 and P(1)i,0|−1=P(1)ij,0|−1=[In10]R−1P0(RT)−1[In10]T, where i,j=1,2,⋯,N;i≠j, qX0=π0πT0+P0.
Step 2. Compute the second-order moment matrix qXs by Lemma 2, and the innovation ei,s by (3.9).
Step 3. Compute the innovation covariance matrices Qei,s and Qeij,s by Lemma 3, the gain matrices K(1)i,s|s by (3.28), Kif,s|s by (3.29), K(2)i,s|s by (3.30), the covariance matrices P(1)iXϖ,s|s, P(1)ijXϖ,s|s, Piϖ,s|s and Pijϖ,s|s by Lemma 5.
Step 4. Compute the FECs P(1)i,s|s by (3.31), P(2)i,s|s by (3.35), the FECCs P(1)ij,s|s by (3.33), P(2)ij,s|s by (3.36), the PEC P(1)i,s+1|s by (3.32), the PECC P(1)ij,s+1|s by (3.34), the correlation matrices P(1,2)i,s|s by (3.37), P(1,2)ij,s|s by (3.38).
Step 5. Compute the LFs ˆX(1)i,s|s by (3.24), ˆX(2)i,s|s by (3.26), the local predictors ˆX(1)i,s+1|s by (3.25), ˆX(2)i,s+1|s by (3.27).
Step 6. Compute the reduced-order fusion filters ˆX(k)0,s|s (k=1,2) by Theorem 2.
Step 7. Compute the DFF ˆX0,s|s for original singular systems by Theorem 3.
Step 8. Let s=s+1, return step 2.
In this section, we provide a simulation example to illustrate the validity of the proposed distributed fusion filtering algorithm.
As we all know, the nonlinear RLC circuits can be molded by the nonlinear singular systems as in [47]. we consider the class of singular systems (2.1) and (2.2) with three sensors, where the related parameters are
M=[−1.80001.2020−2.380−10−1.4401.40],Φ=[0.60−0.50−2.80105.22−2−1.450−3.36−10.22],Υ=[−4−10.83.20.08−2.380.96−4.66], |
H1=[110.500011],H2=[10010110],H3=[110110.111]. |
We choose the nonsingular matrices
U=[−200010.5000.50.9100−1.201],R=[1000001001000001]. |
Then, we have the standard form as follows:
[M10M20][X1,s+1X2,s+1]=[Φ10Φ2Φ3][X1,sX2,s]+[U1U2]f(Xs,ξs)+[Υ1Υ2]ϖs, |
Yi,s=Ωi,s[H(1)iH(2)i][X1,sX2,s]+ϑi,s,i=1,2,3 |
where
M1=[3.60−1.21],M2=[−2.20.80−1],Φ1=[−1.21−0.80],Φ2=[3−0.80−1],Φ3=[−20−12], |
Υ1=[0.8200.6],Υ2=[0.600−0.82],H(1)1=[10.501],H(2)1=[1001],H(1)2=[1001],H(2)2=[0110], |
H(1)3=[1011],H(2)3=[110.11],U1=[−200010.500],U2=[0.50.9100−1.201]. |
We set Qϖ,s=I2, Qϑ1,s=5I2, Qϑ2,s=10I2, Qϑ3,s=15I2. The function f(Xs,ξs) is given as follows:
f(Xs,ξs)=[0.10.20.30.5][0.2sign(ˉX1,s)ˉX1,sξ1,s+0.3sign(ˉX2,s)ˉX2,sξ2,s+0.4sign(ˉX3,s)ˉX3,sξ3,s+0.5sign(ˉX4,s)ˉX4,sξ4,s], |
where ˉXi,s and ξi,s (i=1,2,3,4) represent the ith component of Xs and ξs, respectively. ξi,s are uncorrelated Gaussian white noises with zero-mean and unity variances. We can easily get
Π1=[0.10.20.30.5][0.10.20.30.5]T,Γ1=diag{0.04,0.09,0.16,0.25}. |
Let Ω1,s=diag{α11,s,α12,s}, Ω2,s=diag{α21,s,α22,s}, Ω3,s=diag{α31,s,α32,s}, where the probability density functions pik,s(l) (i=1,2,3;k=1,2) in the interval [0,1] for αik,s (i=1,2,3) satisfy
p1k,s(l)={0.05,l=00.1,l=0.50.85,l=1,p2k,s(l)={0.05,l=00.1,l=0.20.35,l=0.60.5,l=1,p3k,s(l)={0.05,l=00.1,l=0.20.2,l=0.50.3,l=0.80.35,l=1, |
with the mathematical expectations and variances μ1=0.9, μ2=0.73, μ3=0.71, σ21=0.065, σ22=0.0971 and σ23=0.0919. In the simulation, we chose the collection of 80 data points, the initial value is a zero-mean Gaussian variable with P0=0.1I4. For every RONS, applying Theorem 1, we have the LFs ˆX(1)i,s|s and ˆX(2)i,s|s, where i=1,2,3. Then applying Theorem 2, we obtain the DFFs weighted by matrices as follows: ˆX(1)0,s|s and ˆX(2)0,s|s. Subsequently, by applying Theorem 3, we can obtain DFF ˆX0,s|s of the original systems. The simulation results are given in Figures 1–9.
The proposed DFF is shown in Figures 1–4. As we can see, it has the effective estimation performance, where the true value is represented by solid curves, and the dashed curves denote the DFF. To show the performance of DFF algorithm, in Figures 5–8, the precision is compared by plotting the mean square errors (MSEs), where the MSEs of 1000 times (i.e., 11000∑1000l=1(Xls−ˆXli,s|s)2 with i=0,1,2,3) are for LFs when i=1,2,3 and DFF when i=0. From Figures 5–8, we can easily obtain that the DFF has better precision than the LFs. Thereby, it is easy to see that the proposed fusion algorithm in this paper has better estimation performance from the simulation results.
In addition, in order to illustrate the impacts from the fading measurements, Figure 9 shows the comparison of MSEs (i.e., 11000∑1000l=1∑4k=1(Xl,ks−ˆXl,k0,s|s)2) for DFF under different fading cases, where Xl,ks and ˆXl,k0,s|s represent the kth component of Xls and ˆXl0,s|s, respectively. Then, we consider the following different cases: Case 1: μ1=0.2, μ2=0.4, μ3=0.5; Case 2: μ1=0.5, μ2=0.5, μ3=0.5; Case 3: μ1=0.5, μ2=0.5, μ3=0.97. It is obviously observed that Case 3 is better than Case 2, while Case 1 is the worst. Owing to the dependence between the filtering error and fading probabilities, we can derive that the better estimations are obtained when the values μi (i=1,2,3) increase. Hence, we further illustrate the effectiveness of the proposed fusion filtering scheme.
In this paper, we have investigated the fusion filtering problem for MSSSs in the presence of fading measurements and stochastic nonlinearity. The phenomenon of fading measurements has been described by a diagonal matrix with a set of random variables. By using the nonsingular transformations, the singular systems have been converted into two RONSs. For subsystems, the distributed optimal fusion filters have been presented on the basis of the matrices weighted fusion criterion. It is worth pointing out that the matrices weighted fusion filter is unbiased and optimal, and the computation burden of fusion center is reduced by using the parallel structure. In simulation, by comparing the DFF with LFs, we can easily obtain the DFF with better precision. In some special fields, the nonsingular matrix M is a non-square matrix. In future work, we will consider the research of non-square networked singular systems.
From (3.7), we can see that qX2,s+1 contains ∑ml=1Πltr(qXs+1Γl), then substituting qX2,s+1 into (3.4), we get
qXs+1=R[qX1,s+1qX12,s+1qX21,s+1BqX1,s+1BT+DQϖ,s+1DT]RT+R[000Cm∑l=1Πltr(qXs+1Γl)CT]RT=Δs+1+tr(qXs+1Γ1)Θ1+tr(qXs+1Γ2)Θ2+⋯+tr(qXs+1Γm)Θm, | (5.1) |
where
Δs+1=R[qX1,s+1qX12,s+1qX21,s+1BqX1,s+1BT+DQϖ,s+1DT]RT,Θl=R[000CΠlCT]RT,l=1,2,⋯,m. |
Then, multiplying the right side of Γl (l=1,2,⋯,m) to (5.1), we obtain m equations as follows:
{qXs+1Γ1=Δs+1Γ1+tr(qXs+1Γ1)Θ1Γ1+⋯+tr(qXs+1Γm)ΘmΓ1⋮qXs+1Γm=Δs+1Γm+tr(qXs+1Γ1)Θ1Γm+⋯+tr(qXs+1Γm)ΘmΓm. | (5.2) |
Calculating the trace of equations (5.2), we get
[tr(Γ1Θ1)−1tr(Γ1Θ2)⋯tr(Γ1Θm)tr(Γ2Θ1)tr(Γ2Θ2)−1⋯tr(Γ2Θm)⋮⋮⋱⋮tr(ΓmΘ1)tr(ΓmΘ2)⋯tr(ΓmΘm)−1][tr(qXs+1Γ1)tr(qXs+1Γ2)⋮tr(qXs+1Γm)]=[−tr(Δs+1Γ1)−tr(Δs+1Γ2)⋮−tr(Δs+1Γm)]. | (5.3) |
According to Assumption 7, we obtain that the coefficient matrix of (5.3) is invertible, so we get
[tr(qXs+1Γ1)tr(qXs+1Γ2)⋮tr(qXs+1Γm)]=(Ψ−Im)−1[−tr(Δs+1Γ1)−tr(Δs+1Γ2)⋮−tr(Δs+1Γm)], | (5.4) |
then, multiplying the left side of [Π1Π2⋯Πm] to (5.4), we obtain
m∑l=1Πltr(qXs+1Γl)=[Π1Π2⋯Πm](Ψ−Im)−1[−tr(Δs+1Γ1)−tr(Δs+1Γ2)⋮−tr(Δs+1Γm)]. | (5.5) |
From the above discussion, we can see that the right-hand side of Eq (5.5) contains ∑ml=1Πltr(qXsΓl), and the Eq (5.5) can be computed recursively.
This work was supported in part by the National Natural Science Foundation of China under Grant 12171124 and 72001059, the Talent Training Project of Reform and Development Foundation for Local Universities from Central Government of China-Youth Talent Project, the Fundamental Research Foundation for Universities of Heilongjiang Province of China under Grant 2019-KYYWF-0215, the University Nursing Program for Young Scholars with Creative Talents in Heilongjiang Province of China under Grant UNPYSCT-2020186, and the Alexander von Humboldt Foundation of Germany.
The authors declare that they have no conflict of interest.
[1] | L. Dai, Singular control systems, Springer-Verlag, 1989. doi: 10.1007/BFb0002475. |
[2] |
I. Dassios, Optimal solutions for non-consistent singular linear systems of fractional nabla difference equations, Circuits Syst. Signal Process., 34 (2015), 1969–1797. doi: 10.1007/s00034-014-9930-2. doi: 10.1007/s00034-014-9930-2
![]() |
[3] |
I. Dassios, G. Kalogeropoulos, On a non-homogeneous singular linear discrete time system with a singular matrix pencil, Circuits Syst. Signal Process., 32 (2013), 1615–1635. doi: 10.1007/s00034-012-9541-8. doi: 10.1007/s00034-012-9541-8
![]() |
[4] |
I. Dassios, On non homogeneous linear generalized linear discrete time systems, Circuits Syst. Signal Process., 31 (2012), 1699–1712. doi: 10.1007/s00034-012-9400-7. doi: 10.1007/s00034-012-9400-7
![]() |
[5] |
Z. Feng, Z. Jiang, W. Zheng, Reachable set synthesis of singular Markovian jump systems, J. Frankl. Inst. Eng. Appl. Math., 357 (2020), 13785–13799. doi: 10.1016/j.jfranklin.2020.09.042. doi: 10.1016/j.jfranklin.2020.09.042
![]() |
[6] |
Q. Wu, Q. Song, Z. Zhao, Y. Liu, F. E. Alsaadi, Stabilization of T-S fuzzy fractional rectangular descriptor time-delay system, Int. J. Syst. Sci., 52 (2021), 2268–2282. doi: 10.1080/00207721.2021.1882613. doi: 10.1080/00207721.2021.1882613
![]() |
[7] |
S. Sun, J. Ma, Optimal filtering and smoothing for discrete-time stochastic singular systems, Signal Process., 87 (2007), 189–201. doi: 10.1016/j.sigpro.2006.05.007. doi: 10.1016/j.sigpro.2006.05.007
![]() |
[8] |
X. Yu, J. Li, Optimal filtering and a smoothing algorithm for a singular system with a complex stochastic uncertain parameter matrix, IEEE Trans. Circuits Syst. Ⅱ-Express Briefs, 67 (2020), 780–784. doi: 10.1109/TCSII.2019.2922010. doi: 10.1109/TCSII.2019.2922010
![]() |
[9] |
S. Sun, J. Ma, Distributed reduced-order optimal fusion Kalman filters for stochastic singular systems, ACTA Automatica Sinica, 32 (2006), 286–290. doi: 10.16383/j.aas.2006.02.017. doi: 10.16383/j.aas.2006.02.017
![]() |
[10] |
S. Sun, Y. Shen, J. Ma, Optimal fusion reduced-order Kalman estimators for discrete-time stochastic singular systems, Control Intell. Syst., 36 (2008), 1–9. doi: 10.2316/Journal.201.2008.1.201-1614. doi: 10.2316/Journal.201.2008.1.201-1614
![]() |
[11] |
J. Feng, M. Zeng, Descriptor recursive estimation for multiple sensors with different delay rates, Int. J. Control, 84 (2011), 584–596. doi: 10.1080/00207179.2011.563321. doi: 10.1080/00207179.2011.563321
![]() |
[12] |
Y. Dou, C. Ran, Y. Gao, Weighted measurement fusion Kalman estimator for multisensor descriptor system, Int. J. Syst. Sci., 47 (2016), 2722–2732. doi: 10.1080/00207721.2015.1018368. doi: 10.1080/00207721.2015.1018368
![]() |
[13] |
M. M. Fahmy, J. O'Reilly, Observers for descriptor systems, Int. J. Control, 49 (1989), 2013–2028. doi: 10.1080/00207178908559759. doi: 10.1080/00207178908559759
![]() |
[14] |
V. L. Syrmos, F. L. Lewis, Robust eigenvalue assignment for generalized systems, Automatica, 28 (1992), 1223–1228. doi: 10.1016/0005-1098(92)90064-M. doi: 10.1016/0005-1098(92)90064-M
![]() |
[15] |
J. Sun, C. Zhang, J. Gu, Decentralized optimal fusion filtering for multi-sensor multi-delay singular systems, Circuits Syst. Signal Process., 31 (2012), 163–176. doi: 10.1007/s00034-011-9313-x. doi: 10.1007/s00034-011-9313-x
![]() |
[16] |
C. Zhu, Y. Xia, L. Xie, L. Yan, Optimal linear estimation for systems with transmission delays and packet dropouts, IET Signal Process., 7 (2013), 814–823. doi: 10.1049/iet-spr.2012.0348. doi: 10.1049/iet-spr.2012.0348
![]() |
[17] |
S. Sun, J. Ma, Linear estimation for networked control systems with random transmission delays and packet dropouts, Inf. Sci., 269 (2014), 349–365. doi: 10.1016/j.ins.2013.12.055. doi: 10.1016/j.ins.2013.12.055
![]() |
[18] |
J. Linares-Pérez, R. Caballero-Águila, I. García-Garrido, Optimal linear filter design for systems with correlation in the measurement matrices and noises: recursive algorithm and applications, Int. J. Syst. Sci., 45 (2014), 1548–1562. doi: 10.1080/00207721.2014.909093. doi: 10.1080/00207721.2014.909093
![]() |
[19] |
S. Wang, Z. Wang, H. Dong, F. E. Alsaadi, Recursive state estimation for linear systems with lossy measurements under time-correlated multiplicative noises, J. Frankl. Inst. Eng. Appl. Math., 357 (2020), 1887–1908. doi: 10.1016/j.jfranklin.2019.11.031. doi: 10.1016/j.jfranklin.2019.11.031
![]() |
[20] | B. D. O. Anderson, J. B. Moore, Optimal filtering, New Jersey: Prentice-Hall, 1979. doi: 10.1007/978-94-011-4691-3. |
[21] |
Y. Li, H. Li, Optimal state estimation for finite-field networks with stochastic disturbances, Neurocomputing, 414 (2020), 238–244. doi: 10.1016/j.neucom.2020.07.065. doi: 10.1016/j.neucom.2020.07.065
![]() |
[22] |
Y. Dou, C. Ran, WMF self-tuning Kalman estimators for multisensor singular system, Int. J. Syst. Sci., 50 (2019), 1873–1888. doi: 10.1080/00207721.2019.1645234. doi: 10.1080/00207721.2019.1645234
![]() |
[23] |
Q. Gan, C. J. Harris, Comparison of two measurement fusion methods for Kalman-filter-based multisensor data fusion, IEEE Trans. Aerosp. Electron. Syst., 37 (2001), 273–279. doi: 10.1109/7.913685. doi: 10.1109/7.913685
![]() |
[24] |
S. Sun, Multi-sensor optimal information fusion Kalman filter with application, Aerosp. Sci. Technol., 8 (2003), 57–62. doi: 10.1016/j.ast.2003.08.003. doi: 10.1016/j.ast.2003.08.003
![]() |
[25] |
S. Sun, Multi-sensor information fusion white noise filter weighted by scalars based on Kalman predictor, Automatica, 40 (2004), 1447–1453. doi: 10.1016/j.automatica.2004.03.012. doi: 10.1016/j.automatica.2004.03.012
![]() |
[26] |
S. Sun, Z. Deng, Multi-sensor optimal information fusion Kalman filter, Automatica, 40 (2004), 1017–1023. doi: 10.1016/j.automatica.2004.01.014. doi: 10.1016/j.automatica.2004.01.014
![]() |
[27] |
J. Ma, S. Sun, Information fusion estimators for systems with multiple sensors of different packet dropout rates, Inf. Fusion, 12 (2011), 213–222. doi: 10.1016/j.inffus.2010.11.003. doi: 10.1016/j.inffus.2010.11.003
![]() |
[28] |
H. Tan, B. Shen, K. Peng, H. Liu, Robust recursive filtering for uncertain stochastic systems with amplify-and-forward relays, Int. J. Syst. Sci., 51 (2020), 1188–1199. doi: 10.1080/00207721.2020.1754960. doi: 10.1080/00207721.2020.1754960
![]() |
[29] |
L. Liu, L. Ma, J. Zhang, Y. Bo, Distributed non-fragile set-membership filtering for nonlinear systems under fading channels and bias injection attacks, Int. J. Syst. Sci., 52 (2021), 1192–1205. doi: 10.1080/00207721.2021.1872118. doi: 10.1080/00207721.2021.1872118
![]() |
[30] |
Y. Liu, F. Han, N. Hou, H. Dong, H. Liu, Set-membership filtering for piecewise linear systems with censored measurements under Round-Robin protocol, Int. J. Syst. Sci., 51 (2020), 1578–1588. doi: 10.1080/00207721.2020.1768453. doi: 10.1080/00207721.2020.1768453
![]() |
[31] |
C. Ran, S. Sun, Y. Dou, WMF reduced-order robust estimators for multisensor descriptor systems, IET Contr. Theory Appl., 12 (2018), 2232–2244. doi: 10.1049/iet-cta.2018.5498. doi: 10.1049/iet-cta.2018.5498
![]() |
[32] |
Y. Tang, W. Sun, D. Liu, X. Li, Finite-time simultaneous stabilization for stochastic port-controlled Hamiltonian systems over delayed and fading channels, Complexity, 2020 (2020), 1–12. doi: 10.1155/2020/6387025. doi: 10.1155/2020/6387025
![]() |
[33] |
J. Hu, Z. Wang, D. Chen, F. E. Alsaadi, Estimation, filtering and fusion for networked systems with network-induced phenomena: New progress and prospects, Inf. Fusion, 31 (2016), 65–75. doi: 10.1016/j.inffus.2016.01.001. doi: 10.1016/j.inffus.2016.01.001
![]() |
[34] |
S. Sun, H. Lin, J. Ma, X. Li, Multi-sensor distributed fusion estimation with applications in networked systems: a review paper, Inf. Fusion, 38 (2017), 122–134. doi: 10.1016/j.inffus.2017.03.006. doi: 10.1016/j.inffus.2017.03.006
![]() |
[35] |
Z. Wang, F. Yang, D. Ho, X. Liu, Robust finite-horizon filtering for stochastic systems with missing measurements, IEEE Signal Process. Lett., 12 (2005), 437–440. doi: 10.1109/LSP.2005.847890. doi: 10.1109/LSP.2005.847890
![]() |
[36] |
J. Hu, Z. Wang, S. Liu, H. Gao, A variance-constrained approach to recursive state estimation for time-varying complex networks with missing measurements, Automatica, 64 (2016), 155–162. doi: 10.1016/j.automatica.2015.11.008. doi: 10.1016/j.automatica.2015.11.008
![]() |
[37] |
J. Hu, Z. Wang, H. Gao, Recursive filtering with random parameter matrices, multiple fading measurements and correlated noises, Automatica, 49 (2013), 3440–3448. doi: 10.1016/j.automatica.2013.08.021. doi: 10.1016/j.automatica.2013.08.021
![]() |
[38] |
C. Pang, S. Sun, Fusion predictors for multisensor stochastic uncertain systems with missing measurements and unknown measurement disturbances, IEEE Sens. J., 15 (2015), 4346–4354. doi: 10.1109/JSEN.2015.2416511. doi: 10.1109/JSEN.2015.2416511
![]() |
[39] |
X. Li, X. Fu, R. Rakkiyappan, Delay-dependent stability analysis for a class of dynamical systems with leakage delay and nonlinear perturbations, Appl. Math. Comput., 226 (2014), 10–19. doi: 10.1016/j.amc.2013.10.004. doi: 10.1016/j.amc.2013.10.004
![]() |
[40] |
X. Lu, H. Li, An improved stability theorem for nonlinear systems on time scales with application to multi-agent systems, IEEE Trans. Circuits Syst. Ⅱ-Express Briefs, 67 (2020), 3277–3281. doi: 10.1109/TCSII.2020.2983180. doi: 10.1109/TCSII.2020.2983180
![]() |
[41] |
X. Lu, H. Li, Prescribed finite-time H∞ control for nonlinear descriptor systems, IEEE Trans. Circuits Syst. Ⅱ-Express Briefs, 68 (2021), 2917–2921. doi: 10.1109/TCSII.2021.3060550. doi: 10.1109/TCSII.2021.3060550
![]() |
[42] |
S. Wang, H. Fang, X. Tian, Recursive estimation for nonlinear stochastic systems with multi-step transmission delays, multiple packet dropouts and correlated noises, Signal Process., 115 (2015), 164–175. doi: 10.1016/j.sigpro.2015.03.022. doi: 10.1016/j.sigpro.2015.03.022
![]() |
[43] |
S. Wang, H. Fang, X. Liu, Distributed state estimation for stochastic non-linear systems with random delays and packet dropouts, IET Contr. Theory Appl., 9 (2015), 2657–2665. doi: 10.1049/iet-cta.2015.0257. doi: 10.1049/iet-cta.2015.0257
![]() |
[44] | R. A. Horn, C. R. Johnson, Topics in matrix analysis, New York: Cambridge University Press, 1991. doi: 10.1017/CBO9780511840371. |
[45] |
L. Dai, Observers for discrete singular systems, IEEE Trans. Autom. Control, 33 (1988), 187–191. doi: 10.1109/9.387. doi: 10.1109/9.387
![]() |
[46] |
S. Sun, T. Tian, H. Lin, State estimators for systems with random parameter matrices, stochastic nonlinearities, fading measurements and correlated noises, Inf. Sci., 397 (2017), 118–136. doi: 10.1016/j.ins.2017.02.048. doi: 10.1016/j.ins.2017.02.048
![]() |
[47] |
P. C. Müller, Some aspects of the optimal control of non-linear descriptor systems, J. Appl. Math. Mech., 65 (2001), 769–776. doi: 10.1016/S0021-8928(01)00082-X. doi: 10.1016/S0021-8928(01)00082-X
![]() |
1. | Jing Ma, Liling Xu, Globally Optimal Distributed Fusion Filter for Descriptor Systems with Time-Correlated Measurement Noises, 2022, 22, 1424-8220, 7469, 10.3390/s22197469 |