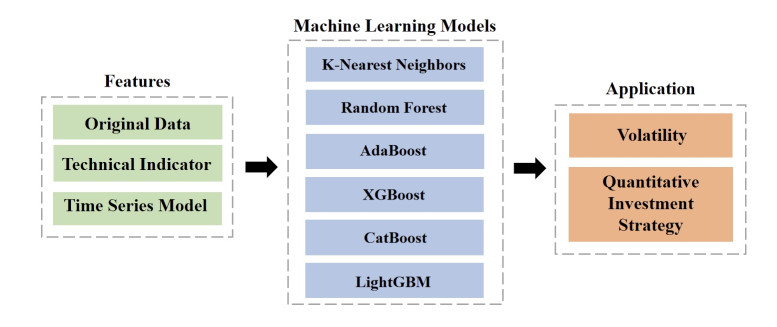
Citation: Raghvendra K Pandey, William A Stapleton, Mohammad Shamsuzzoha, Ivan Sutanto. Voltage biased Varistor-Transistor Hybrid Devices: Properties and Applications[J]. AIMS Materials Science, 2015, 2(3): 243-259. doi: 10.3934/matersci.2015.3.243
[1] | Jan Friedrich, Oliver Kolb, Simone Göttlich . A Godunov type scheme for a class of LWR traffic flow models with non-local flux. Networks and Heterogeneous Media, 2018, 13(4): 531-547. doi: 10.3934/nhm.2018024 |
[2] | Alexander Kurganov, Anthony Polizzi . Non-oscillatory central schemes for traffic flow models with Arrhenius look-ahead dynamics. Networks and Heterogeneous Media, 2009, 4(3): 431-451. doi: 10.3934/nhm.2009.4.431 |
[3] | Raimund Bürger, Christophe Chalons, Rafael Ordoñez, Luis Miguel Villada . A multiclass Lighthill-Whitham-Richards traffic model with a discontinuous velocity function. Networks and Heterogeneous Media, 2021, 16(2): 187-219. doi: 10.3934/nhm.2021004 |
[4] | Maya Briani, Emiliano Cristiani . An easy-to-use algorithm for simulating traffic flow on networks: Theoretical study. Networks and Heterogeneous Media, 2014, 9(3): 519-552. doi: 10.3934/nhm.2014.9.519 |
[5] | Raimund Bürger, Antonio García, Kenneth H. Karlsen, John D. Towers . Difference schemes, entropy solutions, and speedup impulse for an inhomogeneous kinematic traffic flow model. Networks and Heterogeneous Media, 2008, 3(1): 1-41. doi: 10.3934/nhm.2008.3.1 |
[6] | Raimund Bürger, Harold Deivi Contreras, Luis Miguel Villada . A Hilliges-Weidlich-type scheme for a one-dimensional scalar conservation law with nonlocal flux. Networks and Heterogeneous Media, 2023, 18(2): 664-693. doi: 10.3934/nhm.2023029 |
[7] | . Adimurthi, Siddhartha Mishra, G.D. Veerappa Gowda . Existence and stability of entropy solutions for a conservation law with discontinuous non-convex fluxes. Networks and Heterogeneous Media, 2007, 2(1): 127-157. doi: 10.3934/nhm.2007.2.127 |
[8] | Helge Holden, Nils Henrik Risebro . Follow-the-Leader models can be viewed as a numerical approximation to the Lighthill-Whitham-Richards model for traffic flow. Networks and Heterogeneous Media, 2018, 13(3): 409-421. doi: 10.3934/nhm.2018018 |
[9] | Paola Goatin, Chiara Daini, Maria Laura Delle Monache, Antonella Ferrara . Interacting moving bottlenecks in traffic flow. Networks and Heterogeneous Media, 2023, 18(2): 930-945. doi: 10.3934/nhm.2023040 |
[10] | Felisia Angela Chiarello, Paola Goatin . Non-local multi-class traffic flow models. Networks and Heterogeneous Media, 2019, 14(2): 371-387. doi: 10.3934/nhm.2019015 |
With the rapid development of technology, computing and the advancement of data processing capabilities, computer technology has become an integral part of quantitative finance and automated trading. Currently in the financial market, computer technology is mainly used for financial data analysis and mining, algorithmic trading, risk management, and quantitative investment strategies (Tatsat et al., 2020). Among them, the quantitative investment strategy is a process using mathematical or statistical models for investment practice and implementation of automated trading strategies. In this process, the quantitative investment strategy can strictly implement the trading logic initially designed by the investor and will never make the decision to buy and sell stocks due to emotions from the individual investor or the people in the financial trading market (Wang and Yan, 2023). Currently, there are a number of studies and research papers in finance that focus on the problem of how to construct quantitative trading strategies using computer technology. For example, some researchers used traditional time series models and machine learning models to construct quantitative trading strategies and achieved high returns in the backtesting of the cryptocurrency market (Attanasio et al., 2019). There is also a research paper using a combination of the random forest model and the traditional bollinger band strategy to construct a strategy that utilizes the stock price prediction results and bollinger bands for buying and selling operations (Yan et al., 2023). As for the ups and downs in stock trading, volatility is often used to measure the magnitude of the up and down fluctuations of stock prices in a certain time frame. Volatility is common in the field of financial mathematics and engineering and usually defined as the standard deviation of daily stock returns. Volatility is used as a statistical measure of the dispersion of stocks' return data and for measuring the value of options traded in financial markets (Li et al., 2023). The bigger the volatility, the bigger the dispersion of stock returns. Therefore, volatility is a concept that can be used to measure the risk and deviation of a stock (Schwert, 1990, and Brooks et al., 2003). In our research, we aim to forecast the future volatility of different American financial stocks using various variable construction methods, time series, and machine learning models in the first step. As a different method from other studies only focused on prediction, we not only focus on how to predict volatility, but also combine the predicted volatility with quantitative investment in real life. In the second step, we employ a quantitative investment strategy that integrates the predicted volatility with the historical volatility trend to inform our buy or sell decisions. To assess the performance of this strategy, we create a simulated trading environment for each American financial stock. Our experimental results indicate that employing a volatility quantitative investment strategy yields positive returns across a diverse range of sample stocks. Notably, some stocks have achieved returns exceeding 10%.
The rest of this research can be summarized as follows. Section 2 reviews some research works related to traditional time series models, machine learning, and deep learning models, and some quantitative investment strategies. Section 3 describes the logical-technical approach to time series, machine learning models, and volatility quantitative investment trading strategies used in detail. Section 4 presents the performance of the machine learning model metrics and the performance of the volatility quantitative investment strategy on a variety of American financial stocks. Finally, Section 5 summarizes this research and our future research plans.
At present, academic researchers and investors are keen on applying computer technology in finance and hope that statistical and computer technology models manage to obtain excess returns in investment. Before machine learning and deep learning were widely used, traditional statistical time series models occupied a very important position in financial forecasting. Kumbure et al. (2022) made a very detailed description of the financial stock price forecasting in recent years and summarized the relevant research. In general, traditional statistical time series models have been widely used for forecasting stock prices or risk prediction for a long period of time (Khanderwal et al., 2021, and Herwartz, 2017). Currently, the usage processes of traditional statistical time series models are widely used in both forecasting and risk management (Karasan, 2021).
In recent years, as artificial intelligence, big data, and other concepts continued to develop, many studies had been devoted to using machine learning classification models or regression models to predict the rise or fall of stock prices or the future value of different stocks (Rouf et al., 2021). In the field of traditional machine learning applications, Basak et al. (2019) used XGBoost and random forest models to predict future American stock prices under different trading windows, and reported that the future rise or fall of a stock would be significantly different under different labeling settings. Similarly, a research paper applied ensemble learning regression models to predict stock prices after moving average treatment and found that the prediction error was smaller for daily data using moving average as a label (Yan and Wang, 2023). Some scholars believed that machine learning models need some constructed variables related to the research problem, so models could show a better result after training. When faced with the stocks in the Saudi Stock Exchange, Alsulmi and Al-Shahrani (2022) constructed 31 variables of technical characteristics of the stock such as the exponential moving average, triple exponential moving average, weighted close price, williams' %R, on-balance volume, and so on. Patel et al. (2015) used some more classical technical features such as the simple moving average, momentum, moving average convergence/divergence, and relative strength index for stock trend prediction.
With the development of computer technology, deep learning algorithms and richer data sources have also been introduced to study the rise and fall of stocks (Wang and Yan, 2022). Vijh et al. (2020) applied the well-known artificial neural network model to predict the stock prices of four companies listed in America and found that the artificial neural network model had a smaller error by comparing it with the random forest model. Similarly, some research papers showed that deep learning models such as long short-term memory (LSTM) and neural network models had better results than traditional machine learning models such as support vector machine (SVM) and random forest by predicting the world's famous stock market indices (Yu et al., 2020, and Nikou et al., 2019). More novel methods have also been applied to feature construction. Rezaei et al. (2021) decomposed the original stock price data to reduce the noise in the original data, and found that the LSTM model could reorganize the decomposed data and make predictions. In addition, the gated recurrent unit (GRU) model, another deep learning model based on time series data, was used in many research projects to predict stock prices. Researchers could control the parameters of the model and change the data inputs to reduce errors (Shahi et al., 2020, and Gao et al., 2021). Except for using historical stock price data for prediction, some studies considered introducing richer data sources for analysis. Khan et al. (2020) collected social media and financial news data and conducted analysis to find investors' optimistic or pessimistic sentiment scores on the financial market. These scores facilitated a more accurate prediction of the future stock prices. The study also pointed out that the variables constructed using text data had different effects on the prediction of different types of stock. Although deep learning models can have excellent fitting effects, they also have some limitations on the specific object of prediction, which may affect their accuracy. Ayyildiz and Iskenderoglu (2024) found that machine learning models were better than neural networks in predicting the Nikkei Stock Average (NIKKEI 225), Cotation Assistée en Continu (CAC 40), and Toronto Stock Exchange (TSX) from the global financial markets. To make matters worse, the parameter selection of deep learning models can be more complex than machine learning models and the training process is very time-consuming.
Many research papers have concentrated on predicting the rise and fall of the stock price. However, some studies focused on predicting the stock volatility instead of price (Luong et al., 2018, and Diane et al., 2024). Volatility holds a significant position in the financial markets. Zhang et al. (2023) successfully predicted the risk in the crude oil market by combining the flexible Fourier form with the generalized autoregressive conditional heteroskedasticity (GARCH) model. Other studies, such as those by Bezerra and Albuquerque (2017) and Sun et al. (2020), have attempted to merge the GARCH model with the machine learning model SVM to create an SVM-GARCH model for market risk prediction. Nonetheless, these studies are limited as they focused on prediction and error comparison only. There has been little effort to utilize predicted volatility as a trading signal within quantitative investment strategies. In our prior research, we explored the application of double models in conjunction with volatility-based quantitative trading strategies within the Hong Kong stock market (Yan et al., 2024). In our current research, we aim to integrate a more precise volatility prediction model to implement a quantitative investment strategy. Our objective is to forecast the subsequent day's stock volatility using machine learning models. We integrate these predictions with historical volatility trends to guide our investment decisions regarding stock positions. The effectiveness of our volatility quantitative investment strategies is evaluated within a comprehensive simulated trading framework.
The framework completed in this research is depicted in Figure 1. Our research aims to utilize a blend of traditional time series and machine learning models to forecast the future volatility of twelve financial stocks in the American stock market. Additionally, we devise suitable quantitative investment strategies. Our modeling process builds upon the methodologies from our previous research (Yan et al., 2024). We maintain a hybrid approach that combines time series and machine learning models for volatility prediction. Nevertheless, we have implemented improvements in feature construction, model selection, the training process, and the quantitative investment process to enhance the alignment with real-world applications, thus improving the framework's overall effectiveness. The details of these enhancements are outlined in Sections 3.1, 3.2, 3.3, and 4.
Based on Figure 1, we download the stock datasets first and construct characteristic variables that facilitate the prediction of volatility based on common financial technical indicators. In addition, some better-known volatility forecasting time series models such as GARCH, glosten jagannathan runkle GARCH (GJR-GARCH), and exponential GARCH (EGARCH) are discussed. The sliding window is used dynamically during the time series modeling. The features that are constructed using financial technical indicators and the volatility predicted by the time series modeling are then combined to form brand new features. These features are used to predict future volatility using six well-known machine learning models. Similarly, we train these machine learning models using a sliding window approach. At last, financial simulation trading experiments based on the future volatility predicted by different machine learning models are conducted. These experiments compare the profitability of volatility quantitative investment strategies with different feature variables and different machine learning models.
America boasts the world's largest economy and consumer base, and its stock market plays a pivotal role in the global economy. The American stock market is recognized as one of the most mature capital markets globally, distinguished by its vast size, high liquidity, and wide array of investment options. In contrast to the Hong Kong stock market, which was the focus of our previous research (Yan et al., 2024), the American market is considerably larger and more active with a large number of market participants. Consequently, the insights derived from this research carry significant implications. Moreover, we have included a selection of globally renowned stocks for our analysis. The names of these twelve financial stocks selected from the American stock market are JPMorgan Chase & Co (JPM), Bank of America (BAC), Wells Fargo & Company (WFC), The Goldman Sachs Group (GS), Morgan Stanley (MS), PNC Financial Services Group (PNC), Bank of Montreal (BMO), State Street Corporation (STT), HSBC Group (HSBC), American Express (AXP), Fifth Third Bank (FITB), and M & T Bank Corporation (MTB). These stocks have made up a large share of the American financial market and comprise the country's bustling financial industry. We downloaded the day-trading datasets of the above stocks spanning from January 1, 2014 to December 31, 2023 (Yahoo Finance, 2024).
Our dataset comprises five original variables: open, high, low, close, and volume. Beyond these fundamental data points, we have developed volatility-related features derived from financial technical indicators cited in research literature. In our previous research (Yan et al., 2024), we limited our analysis to features associated with historical volatility. In our current research, we are incorporating additional financial technical indicators such as momentum, on-balance volume, moving average convergence/divergence, and stochastic oscillator to enhance the precision of our predictions. These indicators have been constructed using the Pandas and Talib packages within Python. The versions used are 1.3.4 for Pandas and 0.4.24 for Talib. Also, volatilities of the past few days are used to be the features of the machine learning models, while the volatility of the next day is considered to be the output of the machine learning models.
Relative strength index (RSI) is a common momentum indicator, used to calculate the average price rise or fall of a stock over a certain period in the investment trading market (Levy et al., 1967). The default period $ d $ is set to be 14 days. When the RSI value is above 70, the underlying investment is in an overbought state. When the RSI value is below 30, the underlying investment is in an oversold state.
$ RSI(d)t=100−1001+average gain over past d daysaverage loss over past d days. $
|
(1) |
Momentum indicator (MOM), a common technical analysis tool, is used to measure the rate of change in stock prices over a specific time period. The MOM calculates the momentum of price change by comparing the current stock price with the stock price at a specific point in the past, which can help investors identify the trend and strength of the market. A positive value of MOM indicates upward price momentum, while a negative value indicates downward price momentum. A continuous rising of the indicator suggests that buying momentum is increasing and the price may continue to rise. On the contrary, decreasing of the indicator implies that the selling momentum is increasing and the price may fall (Khand et al., 2019). In this research, $ d $ is set to 5 and the stock price is set to the close price.
$ MOM(d)t=Closet−Closet−d. $
|
(2) |
On-balance volume (OBV) is also a common momentum indicator. It centers on the idea that changes in volume can be indicative of future movements in stock prices. If the close price of an asset is higher than the previous day, the volume for that day is added to the OBV value, and conversely, if the close price is lower than the previous day, the volume for that day is subtracted from the OBV value. If the close price is the same as the previous day, the OBV value remains unchanged. In this way, the OBV indicator reflects the change in buying and selling power in the market (Nti et al., 2020).
$ OBVt={OBVt−1+Volumet,ifCloset>Closet−1,OBVt−1−Volumet,ifCloset<Closet−1,OBVt−1,ifCloset=Closet−1. $
|
(3) |
Moving average convergence/divergence (MACD) utilizes the relationship between two moving average lines to predict price movements. The MACD indicator used in our research consists of three parts: the MACD line, the MACD signal line, and the MACD histogram. The MACD line is the difference between the fast exponential moving average (EMA) of the last 12 days and the slow EMA of the last 26 days, which measures the trend and momentum of the price. The MACD signal line is a smooth version of the MACD line. The MACD histogram is the difference between the MACD line and the MACD signal line, which shows the convergence and divergence of the two lines. The difference between the MACD line and the MACD signal line, always represented by a bar graph in stock investing software (Wang and Kim, 2018), can help investors identify the strength and direction of the market. The specific formulas for these indicators are:
$ EMA(d)t=2d+1t−1∑i=0(d−1d+1)iCloset−i, $
|
(4) |
$ MACDt=EMA(12)t−EMA(26)t, $
|
(5) |
$ MACD Signalt=EMA(MACDt,9)t, $
|
(6) |
$ MACD Histogramt=MACDt−MACD Signalt. $
|
(7) |
Stochastic oscillator, also known as the KDJ indicator, has been originally used in predicting the futures trading market. The stochastic oscillator can be easily viewed in stock software and has a wide range of applications among the investor population. With the %K line and %D line, this indicator measures the momentum and direction of the price. It shows the relationship between the high and low levels of the stock price and the close price also reflects the strength of the price trend and the phenomenon of overbought and oversold (Lai et al., 2019). It can help investors identify the optimal entry and exit points for trading.
$ %K(d)t=100×Closet−i−LowestLow(d)tHighestHigh(d)t−LowestLow(d)t, $
|
(8) |
$ %D(n)t=100×K(d)t+K(d)t−1+…+K(d)t−(n−1)n. $
|
(9) |
Since we are forecasting future volatility, we also include historical volatility as features when constructing variables. First, we compute the daily return of a stock, denoted as $ R_t $. Volatility is calculated as the standard deviation of the daily return of a stock over a range of past periods of time. For the purpose of this research, we applied a past period of 30 days, which means that $ n $ is 30.
$ Rt=Closet−Closet−1Closet−1, $
|
(10) |
$ Volatilityt=√1n−1n−1∑i=0(Rt−i−μ)2. $
|
(11) |
In our research, we intricately weave the tapestry of volatility's past to predict its future, utilizing the features $ Volatility_{t-1} $, $ Volatility_{t-2} $, $ Volatility_{t-3} $, $ Volatility_{t-4} $, $ Volatility_{t-5} $, and $ Volatility_{t-6} $, which represent the volatility metrics from the preceding six days. These features serve as the independent variables in our machine learning models, providing a robust framework for discerning the nuanced trends and shifts in stock volatility. The dependent variable, $ Volatility_{t+1} $, embodies the projected volatility for the subsequent day, anchoring the predictive prowess of our models.
As elucidated in the Introduction and visually represented in Figure 1, our exploration commences with three venerable time series models: GARCH, GJR-GARCH, and EGARCH. These models are harnessed to forecast volatility, and their predictions are then integrated as feature input for the machine learning models one by one. The modeling process is executed using the Arch package, version 6.3.0, within the Python programming environment. We begin with the GARCH model, a time-honored stalwart in the realm of time series analysis. Renowned for its capacity to estimate the volatility of financial asset returns, the GARCH model adeptly captures the clustering and leptokurtic nature of volatility. The canonical GARCH($ p $, $ q $) model is articulated as follows:
$ σ2t=ω+p∑i=1αiϵ2t−i+q∑j=1βjσ2t−j, $
|
(12) |
where the predictor variable $ \sigma_t^2 $ is the conditional variance at time $ t $ and $ \epsilon_{t} $ is the independent identically distribution white noise series. $ e_t \sim N(0, 1) $. $ \epsilon_{t} = \sigma_{t} e_t $. The remaining $ \omega $, $ \alpha_i $, and $ \beta_j $ are the model parameters estimated by maximum likelihood.
The GJR-GARCH model represents an evolution of the traditional GARCH framework, adeptly capturing the asymmetric nature of financial asset return volatility. This is achieved through the incorporation of an innovative indicator function, which allows for a nuanced reflection of market dynamics. The indicator function is articulated as follows:
$ σ2t=ω+p∑i=1(αi+γiNt−i)ϵ2t−i+q∑j=1βjσ2t−j, $
|
(13) |
where $ N_{t-i} $ is the indicator function. When $ \epsilon_{t-i} $ is less than 0, $ N_{t-i} $ takes 1, otherwise it takes 0 (Monfared et al., 2014). $ \omega $, $ \alpha_i $, $ \beta_j $, and $ \gamma_i $ are the model parameters.
In parallel, the EGARCH model stands as another sophisticated iteration of the GARCH model. Its hallmark lies in the modeling of logarithmic volatility, a feature that liberates the model from the constraints typically imposed on parameter values (Epaphra, 2016). The EGARCH model is represented as:
$ ln(σ2t)=ω+p∑i=1αi(|ϵt−iσt−i|−√2π)+q∑j=1βjln(σ2t−j). $
|
(14) |
The distinction between these three models resides in their unique approaches to volatility modeling. Our research uses the methodologies outlined by Karasan (2021), enhanced with the application of a sliding window method. This dynamic update mechanism ensures that the predicted sigma values are not only current but also highly indicative of future volatility. As we weave these time series models into the fabric of machine learning, we adopt a hybridized approach. A time series model is meticulously paired with a machine learning model to form our final model, ensuring robustness and accuracy when other feature variables remain constant. This fusion of traditional and contemporary techniques yields a predictive model that is both grounded in proven theory and enhanced by modern computational intelligence.
Building upon our previous paper (Yan et al., 2024), we have selected a range of popular and extensively utilized machine learning models. Alongside the retained AdaBoost and random forest models, we have chosen four additional widely recognized models for volatility prediction regression modeling: K-nearest neighbors, CatBoost, LightGBM, and XGBoost. The K-nearest neighbors, AdaBoost, and random forest models were sourced from the Sklearn package, version 1.1.2, while the XGBoost model was from the Xgboost package, version 1.7.4. The CatBoost model was implemented using the Catboost package, version 1.0.6, and the LightGBM model was from the Lightgbm package, version 3.3.2. The grid search method was chose to select the best parameters of each machine learning model during the pre-training process. The five-fold validation method was used for the effect evaluation during the grid search for the best parameters (Müller et al., 2016). The ranges of parameters of each model are listed here:
1) For the K-nearest neighbors model, we selected the following parameters using the grid search method: n_neighbors (1, 3, 5, 7), weights ("uniform", "distance"), algorithm ("auto", "ball_tree", "kd_tree$ \ddot{, } $ "brute").
2) For the AdaBoost model, we selected the following parameters using the grid search method: n_estimators (50,100,150,200,250), learning_rate (0.1, 0.5, 1.0).
3) For the CatBoost model, we selected the following parameters using the grid search method: n_estimators (100,150,200), depth (2, 5, 8), learning_rate (0.1, 0.5, 1.0).
4) For the LightGBM model, we selected the following parameters using the grid search method: learning_rate (0.01, 0.1, 1), n_estimators (50,100,150,200,250), max_depth (1, 2, 4, 8), num_leaves (10, 20, 30, 40, 50).
5) For the XGBoost model, we selected the following parameters using the grid search method: n_estimators (50,100,150,200), max_depth (5, 8, 11), reg_alpha (0, 0.1, 0.5), reg_lambda (0, 0.1, 0.5).
6) For the random forest model, we selected the following parameters using the grid search method: n_estimators (50,100,150,200,250), max_depth (2, 5, 8, 11, 14, 17), max_features (2, 4, 6, 8, 10).
We forecasted future volatility using a combination of time series models and machine learning models, where the time series models are GARCH, GJR-GARCH, and EGARCH. The process of combining six machine learning models results in $ 3 \times 6 = 18 $ combinations, such as GARCH-K-nearest neighbors, GARCH-random forest, GJR-GARCH-AdaBoost, GJR-GARCH-XGBoost, EGARCH-CatBoost, EGARCH-LightGBM, and other models. In this research, we have adopted a new training methodology for our 18 machine learning model combinations. Unlike our previous research (Yan et al., 2024), where we trained models using a static training dataset and evaluated them on a testing dataset, we now employ a sliding window approach. This method allows the model's internal parameters to adapt over time, yielding more accurate results for time series data and enhancing decision-making in quantitative investment strategies. To evaluate the performance of our machine learning models, we utilize four standard regression metrics: R squared, root mean square error (RMSE), mean square error (MSE), and mean absolute error (MAE). These metrics gauge the discrepancy between the actual and predicted values. An R squared value closer to 1 indicates superior model interpretability and predictive reliability. Conversely, lower values of RMSE, MSE, and MAE, approaching 0, signify a better model fit. The formulas for these metrics are as follows:
$ R squared=1−∑nt=1(yt−ˆyt)2∑nt=1(yt−ˉyt)2, $
|
(15) |
$ RMSE=√1nn∑t=1(yt−ˆyt)2, $
|
(16) |
$ MSE=1nn∑t=1(yt−ˆyt)2, $
|
(17) |
$ MAE=1nn∑t=1|yt−ˆyt|, $
|
(18) |
where $ y_t $ is the actual value, $ \hat{y}_t $ is the predicted value, $ \bar{y}_t $ is the mean value, and $ n $ is the number of observations.
Quantitative stock investment represents a sophisticated trading paradigm that employs statistical models and advanced computer algorithms to execute stock transactions autonomously. This method capitalizes on historical market data and identified trading patterns to inform buying and selling decisions. The quintessential aim of this approach is to amplify efficiency and profitability by mitigating the influence of human emotions through the application of scientific techniques. This domain encompasses a diverse array of strategies, including but not limited to trend-following, arbitrage, and mean reverting strategies. The volatility quantitative investment strategy explored in our research draws inspiration from the foundational principles of mean reverting strategies. Such strategies gauge whether a stock is overbought or oversold by measuring the deviation of its price from the historical average. Our strategy involves initiating a buy or sell order when there is a significant disparity between the current trading price and the historical mean, thereby capitalizing on the anticipated reversion to the stock's intrinsic value to generate profits, as delineated by Lo et al. (2023). Our research substitutes historical stock prices with historical volatility as the metric of interest. We introduce two pivotal constructs: the upper track line $ Upper \text{ }Track_{t} $ and the lower track line $ Lower \text{ }Track_{t} $. The upper track line signifies the maximum of volatility in the past 60 days, while the lower track line represents the moving average of volatility over a trailing 60-day window. These parameters serve as critical thresholds in our refined mean reverting strategy, offering a fresh perspective on volatility quantitative investment strategy. In our previous research (Yan et al., 2024), we set the parameter $ n $ at 70 when defining these track lines. However, given that stocks in the American market exhibit greater stability compared to those in the Hong Kong market, we have adjusted $ n $ from 70 to 60. This modification renders the upper and lower track lines more indicative of significant market movements.
$ Upper Trackt=Max(Volatilityt,Volatilityt−1,Volatilityt−2,…,Volatilityt−59), $
|
(19) |
$ Lower Trackt=Volatilityt+Volatilityt−1+Volatilityt−2+…+Volatilityt−5960, $
|
(20) |
where $ Volatility_t $ is the historical volatility at time $ t $. The volatility of the coming day predicted by the machine learning model is used to guide the investment strategy. We buy the stock when predicted $ Volatility_{t+1} $ is greater than the $ Upper \text{ }Track_{t} $. At this time, the volatility of the trading market of the stock is huge, which indicates that the investors' moods are in a panicked or frenzied state. We sell the stock when predicted $ Volatility_{t+1} $ is smaller than the $ Lower \text{ }Track_{t} $. At this time, the volatility of the trading market of the stock returns to a smoother stage and implies that the investors' degree of panic or frenzy has dispersed. The Backtrader package, version 1.9.76.123, is used to simulate the quantitative investment strategy for twelve financial stocks. Some performance metrics can evaluate the return and risk of the volatility quantitative investment strategy. The backtest profit of an individual stock on the test dataset can be simply expressed as the total assets at the end of the backtest minus the initial investment amount, which is given by the following formula:
$ Profit=Assetend−Assetinitial. $
|
(21) |
To scientifically observe the returns, we can also use the annualized return to evaluate the effectiveness of the investment strategy. It indicates the expected return for one year at the time of investing and is calculated as a percentage. Its calculation formula is as follows, where $ n $ denotes the number of backtesting days on the test set.
$ Annualized Return=(AssetendAssetinitial)252n−1. $
|
(22) |
To assess risk, we use maximum drawdown, calculated as a percentage. It represents the maximum percentage of loss that an investment strategy can incur over a period of time. When the maximum drawdown is large, the risk of the strategy is also high and less effective.
In this section, we meticulously discuss the efficacy of volatility quantitative investment strategies as they are applied across a spectrum of machine learning predictive models and a selection of equities. We employ the statistical metrics of R squared, RMSE, MSE, and MAE to gauge the predictive models' precision, while the financial metrics of profit, annualized return, and maximum drawdown serve as our yardsticks for evaluating the economic viability of the volatility quantitative investment strategies. Our empirical analysis is anchored in backtesting conducted on a dozen stalwarts of the American financial stocks: JPM, BAC, WFC, GS, MS, PNC, BMO, STT, HSBC, AXP, FITB, and MTB. The temporal scope of our data spans from January of 2014 to December of 2023. A substantial portion, precisely 70% of the dataset, encapsulating day-trading data from 2014 through 2020, is harnessed to train the machine models. The residual 30%, encompassing data from 2021 to 2023, is utilized to put the models through their paces, testing their performance and the robustness of the quantitative investment strategies through backtesting.
In this analytical segment, we embark on a comparative evaluation of the forecasting prowess of a diverse group of 18 models. This ensemble includes three time series models and a series of machine learning models, meticulously applied to a curated selection of twelve financial stocks. The robustness of the regression predictions is quantitatively gauged through an aggregate metric of mean value ± standard deviation value (Mean ± SD), providing a holistic view of model performance. Figure 2 serves as a visual testament to the superior volatility fitting capabilities of the GARCH-XGBoost model when applied to HSBC stock, unequivocally demonstrating the machine learning model's ascendancy.
We categorize the time series models and compute the efficacy of an array of machine learning models. These models are scrutinized under the lens of GARCH, GJR-GARCH, and EGARCH time series frameworks, with a keen focus on the precision of fit and predictive error. Based on the financial trading dataset of twelve American stocks, we employ mean and standard deviation to critically assess model performance. Intriguingly, as Table 1 reveals, the machine learning models exhibit a uniformity in predictive capability across the GARCH, GJR-GARCH, and EGARCH classifications. Despite this parity in error metrics within the test dataset, we unearth a marked divergence in the performance of the trading strategies, a subject we delve into with more details in Section 4.2.
Time Series | R Squared | RMSE | MSE | MAE |
GARCH | 0.907 ± 0.051 | 0.122 ± 0.036 | 0.016 ± 0.010 | 0.083 ± 0.028 |
GJR-GARCH | 0.907 ± 0.051 | 0.122 ± 0.035 | 0.016 ± 0.009 | 0.083 ± 0.027 |
EGARCH | 0.907 ± 0.051 | 0.122 ± 0.035 | 0.016 ± 0.010 | 0.083 ± 0.027 |
In the machine learning model analysis, we discern notable disparities in the fitting results. Table 2 shows the predicted volatility of the K-nearest neighbors and AdaBoost models, which fluctuates more than the actual volatility. The R squared metric for these two models wavers between 0.8 and 0.9, a testament to their erratic predictive behavior. Moreover, the RMSE, MSE, and MAE errors are also the largest among several models. In stark contrast, the XGBoost and random forest models stand as paragons of precision, marrying stellar fitting performance with the most diminutive error rates in our research. As we proceed to invest through machine learning models in Section 4.2, it remains to be seen whether the XGBoost and random forest models can continue to hold their ground in this arena of algorithmic prowess.
Machine Learning | R Squared | RMSE | MSE | MAE |
K-Nearest Neighbors | 0.830 ± 0.049 | 0.168 ± 0.030 | 0.029 ± 0.011 | 0.121 ± 0.021 |
AdaBoost | 0.879 ± 0.035 | 0.141 ± 0.023 | 0.020 ± 0.007 | 0.105 ± 0.019 |
CatBoost | 0.920 ± 0.027 | 0.117 ± 0.029 | 0.014 ± 0.007 | 0.075 ± 0.015 |
LightGBM | 0.921 ± 0.027 | 0.114 ± 0.022 | 0.013 ± 0.005 | 0.078 ± 0.016 |
XGBoost | 0.943 ± 0.013 | 0.099 ± 0.020 | 0.010 ± 0.004 | 0.061 ± 0.009 |
Random Forest | 0.948 ± 0.016 | 0.095 ± 0.023 | 0.010 ± 0.005 | 0.059 ± 0.013 |
Upon the synthesis of each time series and machine learning model, we present a detailed exposition of the new model's predictive acumen regarding the volatility of a dozen financial stocks in Table 3, and more granularly in Figures 3 and 4. In a parallel vein to the findings of Table 1, Figures 3 and 4 elucidate the minimal variance in predictive efficacy among the machine learning models classified under GARCH, GJR-GARCH, and EGARCH, with all corresponding models exhibiting analogous distributions. A closer inspection of the machine learning models reveals that the K-nearest neighbors model is beleaguered by the least favorable fitting effect and the most substantial error magnitude. The box plots within Figures 3 and 4 further illustrate that this model's volatility range is broader than the other five counterparts, signaling a deficiency in stability and robustness. AdaBoost, albeit marginally superior to the K-nearest neighbors, still falls short when measured against the collective performance of the other three ensemble learning models. Conversely, the XGBoost and random forest models are lauded for their enhanced fitting effects. However, when appraised on error performance, the random forest model's prowess eclipses that of the XGBoost model. Furthermore, the incorporation of financial technical indicators has enhanced the precision of our machine learning outcomes. Compared to our past research (Yan et al., 2024), we can observe that models relying solely on historical volatility and time series features underperform in forecasting Hong Kong stocks. The overall R squared values are inferior to those of the eighteen models discussed in this section. Similarly, the RMSE and MSE values are approximately 0.1 higher than those of the models in the current section. In addition, the random forest model in the Hong Kong stock market prediction also got the best results compared with other machine learning models. Therefore, in the problem of volatility prediction, we can consider using Random forest, which provides excellent prediction results in different stock markets.
Time Series | Machine Learning | R Squared | RMSE | MSE | MAE |
GARCH | K-Nearest Neighbors | 0.831 ± 0.049 | 0.168 ± 0.031 | 0.029 ± 0.011 | 0.121±0.021 |
AdaBoost | 0.880 ± 0.033 | 0.141 ± 0.023 | 0.020 ± 0.007 | 0.105 ± 0.019 | |
CatBoost | 0.917 ± 0.030 | 0.119 ± 0.031 | 0.015 ± 0.008 | 0.075 ± 0.016 | |
LightGBM | 0.921 ± 0.028 | 0.115 ± 0.023 | 0.014 ± 0.005 | 0.079 ± 0.017 | |
XGBoost | 0.944 ± 0.013 | 0.098 ± 0.021 | 0.010 ± 0.004 | 0.061 ± 0.009 | |
Random Forest | 0.948 ± 0.014 | 0.094 ± 0.022 | 0.009 ± 0.004 | 0.059 ± 0.012 | |
GJR-GARCH | K-Nearest Neighbors | 0.829 ± 0.049 | 0.169 ± 0.030 | 0.029 ± 0.011 | 0.120 ± 0.022 |
AdaBoost | 0.877 ± 0.036 | 0.142 ± 0.022 | 0.021 ± 0.006 | 0.106 ± 0.019 | |
CatBoost | 0.922 ± 0.019 | 0.115 ± 0.025 | 0.014 ± 0.006 | 0.075 ± 0.013 | |
LightGBM | 0.923 ± 0.026 | 0.113 ± 0.021 | 0.013 ± 0.005 | 0.076 ± 0.015 | |
XGBoost | 0.942 ± 0.013 | 0.100 ± 0.020 | 0.010 ± 0.004 | 0.061 ± 0.009 | |
Random Forest | 0.946 ± 0.015 | 0.096 ± 0.023 | 0.010 ± 0.004 | 0.060 ± 0.014 | |
EGARCH | K-Nearest Neighbors | 0.829 ± 0.047 | 0.169 ± 0.029 | 0.029 ± 0.011 | 0.122 ± 0.019 |
AdaBoost | 0.881 ± 0.035 | 0.140 ± 0.022 | 0.020 ± 0.007 | 0.104 ± 0.018 | |
CatBoost | 0.921 ± 0.030 | 0.116 ± 0.031 | 0.014 ± 0.008 | 0.075 ± 0.015 | |
LightGBM | 0.920 ± 0.026 | 0.115 ± 0.021 | 0.014 ± 0.005 | 0.078 ± 0.015 | |
XGBoost | 0.942 ± 0.013 | 0.099 ± 0.019 | 0.010 ± 0.004 | 0.062 ± 0.008 | |
Random Forest | 0.948 ± 0.019 | 0.094 ± 0.024 | 0.009 ± 0.005 | 0.059 ± 0.014 |
Compared with the models presented in Table 3, the GARCH-random forest model exhibits superior performance across four key metrics: R squared, RMSE, MSE, and MAE. Consequently, we select the GARCH-random forest model for the interpretable analysis within this section. In the process of forecasting volatility for twelve American financial stocks using the GARCH-random forest model, we concurrently assess the significance of the model's independent variables using Gini impurity. Among the top 10 independent variables, $ Volatility_t $ stands out as the most critical, boasting an average Gini impurity score of 0.501 ± 0.076. Furthermore, the volatilities from previous days also significantly influence the forecast of the next day $ Volatility_{t+1} $. This is evidenced by the average Gini impurity scores of $ Volatility_{t-1} $ (0.252 ± 0.027), $ Volatility_{t-2} $ (0.095 ± 0.030), $ Volatility_{t-3} $ (0.061 ± 0.035), $ Volatility_{t-4} $ (0.012 ± 0.009), $ Volatility_{t-5} $ (0.034 ± 0.016), and $ Volatility_{t-6} $ (0.013 ± 0.005). Additionally, the technical indicators we developed, $ MACD_t $ (0.005 ± 0.006) and $ MACD \text{ } Signal_t $ (0.013 ± 0.009), contribute significantly to the model's predictive capability. Ultimately, the GARCH model's importance is determined to be 0.002 ± 0.002. Although it is numerically the least significant among the variables, the machine learning models incorporating the GARCH framework consistently demonstrate enhanced performance when all other independent variables remain constant.
We extend the results analysis in this section, offering a comprehensive exposition of the profitability performance of volatility quantitative investment strategies. These strategies are meticulously categorized based on their foundational time series models and machine learning models. Prior to embarking on the backtesting of these quantitative investments, we set a standard initial capital benchmark of $100,000. This serves as the financial bedrock upon which we assess the investment prowess of each model, scrutinizing their profitability and risk management capabilities. Figure 5 stands as a testament to the efficacy of our approach, graphically depicting the GARCH-XGBoost model's backtesting performance on HSBC stock. The model's acumen shines, demonstrating its ability to pinpoint opportune moments for stock acquisition during periods of undervaluation, all predicated on accurately forecasted volatility. Equally impressive is the model's proficiency in issuing sell signals, capitalizing on the stock's return to normalcy or peak valuation, thereby securing profits. The culmination of this strategic execution is a commendable profitability, with the model's capital swelling to $161,742.67 by the end of the backtesting period.
To provide an intuitive grasp of the quantitative investment performance across all models for each selected stock, we have distilled the return and risk metrics into Table 4. In our commitment to realism and pedagogical clarity for investors and researchers alike, we eschew the traditional Mean ± SD in favor of a 95% confidence interval. This statistical choice delineates the upper and lower bounds of our strategies' profitability and risk control performances more accurately. A panoramic view of the results reveals a spectrum of positive profits and annualized returns within the confidence interval for all stocks. Yet, the interval values exhibit significant divergence; for instance, stocks like BAC, GS, MS, and STT present modest returns, whereas BMO, HSBC, and FITB offer substantial gains to investors. These disparities underscore the autonomous nature of the companies behind each stock, where executive decisions—shaped by market forces, political climates, and economic conditions—directly influence the company's trajectory and, by extension, its stock price. Despite these variances, our strategy consistently delivers robust performance across the board, affirming the soundness and applicability of our strategic framework.
Stock Code | Profit | Annualized Return | Maximum Drawdown |
JPM | [11086, 22675] | [3.520, 6.967] | [16.061, 23.929] |
BAC | [9246, 21605] | [2.924, 6.647] | [10.921, 15.958] |
WFC | [11256, 24170] | [3.546, 7.391] | [10.246, 14.231] |
GS | [8511, 25243] | [2.668, 7.592] | [15.562, 22.351] |
MS | [3452, 12961] | [1.068, 4.104] | [20.540, 26.880] |
PNC | [13971, 24450] | [4.394, 7.528] | [14.106, 18.843] |
BMO | [32221, 51386] | [9.599, 14.722] | [11.160, 15.410] |
STT | [7741, 15916] | [2.476, 5.020] | [13.636, 18.315] |
HSBC | [24742, 39152] | [7.541, 11.592] | [6.347, 9.870] |
AXP | [22786, 28597] | [7.080, 8.755] | [12.200, 18.408] |
FITB | [42923, 52183] | [12.657, 15.019] | [14.920, 21.174] |
MTB | [15122, 33634] | [4.677, 9.955] | [13.955, 18.412] |
In our exploration of modeling, Table 5 shows the outcomes of quantitative investment strategies when categorized under time series-based models. Three distinct time series models exhibit a unique performance spectrum that stands in stark contrast to the findings presented in Table 2. Within this realm, machine learning models anchored in the GARCH framework flourish, boasting the most impressive span of returns. Conversely, the EGARCH model lags, finding itself at a return disadvantage. The absence of notable disparities in the aggregate predictive prowess of these time series models belies the pronounced variations observed during the quantitative investment phase. This phenomenon stems from the divergent predictions each model makes regarding volatility's apexes and nadirs, which in turn dictate disparate transactional junctures during the backtesting interval of quantitative trading, culminating in a spectrum of profitability scenarios. Shifting focus to risk management, the maximum drawdown for all models hovers between 14% and 17%, signaling an inconsequential variance in this metric.
Time Series | Profit | Annualized Return | Maximum Drawdown |
GARCH | [20219, 28328] | [6.162, 8.464] | [14.058, 17.235] |
GJR-GARCH | [19712, 28029] | [5.995, 8.369] | [14.530, 17.474] |
EGARCH | [17350, 25120] | [5.311, 7.560] | [14.654, 17.907] |
Advancing our analysis, we delve into the efficacy of quantitative investment strategies delineated by machine learning model classifications, with insights presented in Table 6. Similar to Table 2 in Section 4.1, the K-nearest neighbors and AdaBoost models continue to underwhelm, not only faltering in predictive accuracy but also delivering lackluster results in the realm of quantitative investing. Their profits and annualized returns languish at the lower end of the spectrum. The LightGBM model, with its superior forecasting abilities, only manages to eclipse the K-nearest neighbors in backtesting performance, while its edge over the AdaBoost model remains marginal. In contrast, the CatBoost, XGBoost, and random forest models distinguish themselves with more robust profit margins and elevated annualized returns. When appraising risk management, the K-nearest neighbors model emerges as the paragon of prudence, advocating a conservative investment approach. Its maximum drawdown is the most contained. Some ensemble learning models like CatBoost, XGBoost, and LightGBM exhibit maximum drawdown confidence intervals spanning from 12% to 21%. Notably, the random forest model stands out for its ability to confine loss risks within a tolerable 18% threshold.
Machine Learning | Profit | Annualized Return | Maximum Drawdown |
K-Nearest Neighbors | [9769, 19029] | [3.073, 5.817] | [7.587, 10.744] |
AdaBoost | [17871, 26243] | [5.568, 7.978] | [14.491, 19.115] |
CatBoost | [20131, 31349] | [6.128, 9.372] | [16.080, 19.265] |
LightGBM | [18403, 29710] | [5.678, 8.860] | [16.732, 20.215] |
XGBoost | [21708, 33333] | [6.597, 9.905] | [16.480, 20.461] |
Random Forest | [17384, 32584] | [5.217, 9.526] | [12.799, 17.747] |
We dissect the models further to illuminate the quantitative investment outcomes of the 18 fusion models, as delineated in Table 7. The visualization of profit, annualized return, and maximum drawdown is more vividly depicted in Figures 6, 7, and 8, offering an intuitive grasp of the financial metrics. Similar to the results in Tables 5 and 6, the sextet of machine learning models within the GARCH taxonomy surpasses its GJR-GARCH and EGARCH counterparts in Table 7. This superiority is further corroborated by Figures 6 and 7, where the GARCH-classified models are shown to seize greater profits. The profitability landscape of the machine learning models is not uniform across different time series classifications, revealing a nuanced performance matrix. The K-nearest neighbors model consistently trails behind within the GARCH, GJR-GARCH, and EGARCH groupings, delivering a performance that can best be described as standard. In a twist of outcomes, the CatBoost model emerges as a curious anomaly, outshining in the GJR-GARCH and EGARCH brackets with returns on par with the XGBoost and random forest models. However, its maximum drawdown remains within a normal range, and under the GARCH classification, CatBoost's performance noticeably lags behind XGBoost and random forest. Based on the results of the XGBoost and random forest models, we find that they outpace their counterparts in harnessing volatility-based trading signals. During the forecasting phase outlined in Section 4.1, these two models demonstrate commendable performance, with their volatility predictions holding substantial referential value. In the quantitative investment phase, they exhibit more consistent return performance and maximum drawdown metrics, particularly under the GARCH and GJR-GARCH classifications. When it comes to returns, XGBoost slightly outperforms random forest, yet in the arena of maximum drawdown control, random forest significantly outstrips XGBoost. For investors, the choice of a volatility quantitative investment strategy hinges on their return expectations and risk appetite. Those with an aggressive stance, willing to embrace certain losses, may find that the GARCH-XGBoost and GJR-GARCH-XGBoost models more fitting for their quantitative investment endeavors. Conversely, conservative investors, averse to high risk, may prefer the stability offered by the GARCH-random forest and GJR-GARCH-random forest models in their investment strategies. Upon comparing the outcomes of the strategies from our previous research (Yan et al., 2024), it is evident that the performance of the eighteen models in the American stock market surpasses that of similar models in the Hong Kong stock market. The quantitative investment models applied to the American market managed to maintain the maximum drawdown at approximately 20%, whereas in the Hong Kong market, the models exhibited a maximum drawdown of about 30%, which is notably 10% higher. Regarding profitability, the minimum annualized return for American stocks is marginally higher than that for Hong Kong stocks, by about 1 to 2%. This superior model fit translates into enhanced returns within the quantitative investment process. Finally, the application of volatility quantitative investment strategies provided in this research can realize more returns for the investors, and provide meaningful references for the investors and researchers to combine volatility, machine learning, and quantitative investment.
Time Series | Machine Learning | Profit | Annualized Return | Maximum Drawdown |
GARCH | K-Nearest Neighbors | [6764, 23861] | [2.211, 7.265] | [6.956, 12.600] |
AdaBoost | [12764, 29836] | [4.115, 8.965] | [12.057, 20.516] | |
CatBoost | [15203, 34345] | [4.781, 10.259] | [15.165, 20.838] | |
LightGBM | [16468, 35657] | [5.158, 10.625] | [14.408, 21.586] | |
XGBoost | [16621, 41346] | [5.127, 12.122] | [14.076, 22.399] | |
Random Forest | [13978, 44440] | [4.290, 12.834] | [8.108, 19.049] | |
GJR-GARCH | K-Nearest Neighbors | [4345, 23148] | [1.439, 7.018] | [5.811, 12.010] |
AdaBoost | [13889, 28065] | [4.385, 8.572] | [12.225, 21.674] | |
CatBoost | [16931, 40658] | [5.116, 12.041] | [14.648, 20.315] | |
LightGBM | [11334, 36605] | [3.681, 10.685] | [15.766, 21.783] | |
XGBoost | [18272, 41205] | [5.654, 12.097] | [14.185, 21.325] | |
Random Forest | [13007, 38988] | [4.089, 11.406] | [12.639, 19.639] | |
EGARCH | K-Nearest Neighbors | [5279, 22998] | [1.744, 6.992] | [5.625, 11.991] |
AdaBoost | [15454, 32338] | [4.885, 9.716] | [12.746, 21.598] | |
CatBoost | [12966, 34338] | [4.065, 10.239] | [14.025, 21.045] | |
LightGBM | [11966, 32312] | [3.842, 9.626] | [15.194, 22.106] | |
XGBoost | [14580, 33098] | [4.529, 9.975] | [15.709, 23.129] | |
Random Forest | [4681, 34812] | [1.483, 10.127] | [11.140, 21.061] |
This research employed cases from the American market to validate the integration of time series and machine learning models, offering practical insights for volatility quantitative investment strategies and introducing novel methodologies. We selected twelve financial stocks from the American stock market as our subjects and applied three statistical time series models: GARCH, GJR-GARCH, and EGARCH, alongside six machine learning models: K-nearest neighbors, AdaBoost, CatBoost, LightGBM, XGBoost, and random forest to predict next-day stock volatility. Based on these forecasts, we constructed a quantitative investment strategy to evaluate investment performance. The results indicate that the XGBoost and random forest models, among the newly integrated models, deliver optimal predictions for the twelve stocks, providing a solid foundation for developing quantitative investment strategies. By integrating the predicted $ Volatility_{t+1} $ with the investment logic established in this research, the volatility quantitative investment strategy achieves positive returns for all twelve stocks. Notably, the models utilizing XGBoost and random forest yield higher returns and exhibit superior risk management. Building on previous research (Yan et al., 2024), we conducted analysis on stocks within the more established stock trading market in America. We also significantly enhanced the entire modeling framework, including feature construction, model selection, the training process, and the quantitative investment process, leading to models with improved fitting, reduced errors, and increased returns with lower risks. These improvements provide valuable contributions to the broader investment community.
For future research, we plan to expand our sample beyond the American financial stock market to include markets from other regions such as Europe, South America, Africa, and mainland China. We will also explore additional models, training methods, and trading patterns to further enhance the efficacy and resilience of volatility quantitative investment strategies.
The authors declare they have not used Artificial Intelligence (AI) tools in the creation of this work.
The authors have received no financial assistance from any source in the preparation of this work.
All authors declare no conflicts of interest in this work.
[1] |
Ginley D, Butler M (1977) The photoelectrolysis of water using iron titanate anodes. J Appl Phys 48: 2019. doi: 10.1063/1.323911
![]() |
[2] |
Morison B, Baughman R, Ginley D, et al. (1978) The influence of crystal structure on the photoresponse of iron-titanium oxide electrodes. J Appl Cryst 11: 121-124. doi: 10.1107/S0021889878012868
![]() |
[3] |
Kazuka H, Kajimura M (2001) Sol-gel preparation and photoelectrochemical properties of Fe2TiO5 thin films. J Sol Gel Sci Tech 22: 125-132. doi: 10.1023/A:1011228706934
![]() |
[4] |
Min K, Park K, Lim A, et al. (2012) Synthesis of pseudobrookite-type Fe2TiO5 nanoparticles and their Li-ion electroactivity. Ceram Int 38: 6009-6013. doi: 10.1016/j.ceramint.2012.03.044
![]() |
[5] | Pauling L (1930) The crystal structure of pseudobrookite, Z. Krystallographie-Crystalline Materials 73: 97. |
[6] | Pandey R, Stapleton W, Sutanto I, et al. (2014) Processing properties of advanced ceramics and composites VI. Ceram Transactions, John Wiley and Sons; 249: 175. |
[7] | Mizusaki J, Tabuchi J, Matsuura T, et al. (1989) Electrical conductivity and Seebeck coefficient of nonstoichiometric La1.x Srx Co O 3-δ. J Electrochem Soc 136: 2082-2088. |
[8] |
Jin L, Zhou C (2013) Electronic structures and optic properties of Fe2TiO5 using LSDA+U approach. Prog Nat Sci Mater Int 23: 413-419. doi: 10.1016/j.pnsc.2013.06.012
![]() |
[9] |
Garten G, Smith S, Wanklyn B (1972) Crystal growth from the flux systems PbO·V2O5 and Bi2O3·V2O5. J Cryst Growth 13/14: 588-592. doi: 10.1016/0022-0248(72)90523-4
![]() |
[10] |
Pandey R, Stapleton W, Sutanto I, et al. (2015) Voltage-Biased Magnetic Sensors Based on Tuned Varistors. J Electron Mater 44: 1100-1109. doi: 10.1007/s11664-015-3632-9
![]() |
[11] | Pandey R, Padmini P, Deravi L, et al. (2006) Magnetic-semiconductors in Fe-Ti-oxide series and their potential applications. IEEE Proceedings of the 8th International Conference on Solid State and Integrated Circuit Technology 2: 992. |
[12] |
Pandey R, Padmini P, Schad R, et al. (2009) Novel magnetic-semiconductors in modified iron titanates for radhard electronics. J Electroceram 22: 334-341. doi: 10.1007/s10832-007-9390-1
![]() |
[13] | Lohn C, Geerts W, O’Brien C, et al. (2008) Functionalized Nanoscale Materials, Devices and Systems. NATO Science for Peace and Security Series B: Physics and Biophysics, 419. |
[14] |
Pandey R, Stapleton W, Sutanto Iv (2015) Nature and characteristics of a voltage-biased varistor and its embedded transistor. J Electron Dev Soc 3: 276-284. doi: 10.1109/JEDS.2015.2409023
![]() |
[15] | Schwing U, Hoffmann B (1980) ZnO single crystals with an intermediate layer of metal oxides—A macroscopic varistor model. J Appl Phys 51: 4558. |
1. | Ying Li, Keyue Yan, Prediction of bank credit customers churn based on machine learning and interpretability analysis, 2025, 5, 2769-2140, 19, 10.3934/DSFE.2025002 | |
2. | Claudia Cappello, Antonella Congedi, Sandra De Iaco, Leonardo Mariella, Traditional Prediction Techniques and Machine Learning Approaches for Financial Time Series Analysis, 2025, 13, 2227-7390, 537, 10.3390/math13030537 | |
3. | Ching-Hsue Cheng, Jun-He Yang, Jia-Pei Dai, Verifying Technical Indicator Effectiveness in Cryptocurrency Price Forecasting: a Deep-Learning Time Series Model Based on Sparrow Search Algorithm, 2025, 17, 1866-9956, 10.1007/s12559-025-10422-4 | |
4. | Keyue Yan, Chi-Fai Lam, Simon Fong, João Alexandre Lobo Marques, Richard Charles Millham, Sabah Mohammed, A Novel Improvement of Feature Selection for Dynamic Hand Gesture Identification Based on Double Machine Learning, 2025, 25, 1424-8220, 1126, 10.3390/s25041126 | |
5. | Ying Liu, Zengyu Wei, Long Chen, Cai Xu, Ziyu Guan, Multi-Modal Temporal Dynamic Graph Construction for Stock Rank Prediction, 2025, 13, 2227-7390, 845, 10.3390/math13050845 |
Time Series | R Squared | RMSE | MSE | MAE |
GARCH | 0.907 ± 0.051 | 0.122 ± 0.036 | 0.016 ± 0.010 | 0.083 ± 0.028 |
GJR-GARCH | 0.907 ± 0.051 | 0.122 ± 0.035 | 0.016 ± 0.009 | 0.083 ± 0.027 |
EGARCH | 0.907 ± 0.051 | 0.122 ± 0.035 | 0.016 ± 0.010 | 0.083 ± 0.027 |
Machine Learning | R Squared | RMSE | MSE | MAE |
K-Nearest Neighbors | 0.830 ± 0.049 | 0.168 ± 0.030 | 0.029 ± 0.011 | 0.121 ± 0.021 |
AdaBoost | 0.879 ± 0.035 | 0.141 ± 0.023 | 0.020 ± 0.007 | 0.105 ± 0.019 |
CatBoost | 0.920 ± 0.027 | 0.117 ± 0.029 | 0.014 ± 0.007 | 0.075 ± 0.015 |
LightGBM | 0.921 ± 0.027 | 0.114 ± 0.022 | 0.013 ± 0.005 | 0.078 ± 0.016 |
XGBoost | 0.943 ± 0.013 | 0.099 ± 0.020 | 0.010 ± 0.004 | 0.061 ± 0.009 |
Random Forest | 0.948 ± 0.016 | 0.095 ± 0.023 | 0.010 ± 0.005 | 0.059 ± 0.013 |
Time Series | Machine Learning | R Squared | RMSE | MSE | MAE |
GARCH | K-Nearest Neighbors | 0.831 ± 0.049 | 0.168 ± 0.031 | 0.029 ± 0.011 | 0.121±0.021 |
AdaBoost | 0.880 ± 0.033 | 0.141 ± 0.023 | 0.020 ± 0.007 | 0.105 ± 0.019 | |
CatBoost | 0.917 ± 0.030 | 0.119 ± 0.031 | 0.015 ± 0.008 | 0.075 ± 0.016 | |
LightGBM | 0.921 ± 0.028 | 0.115 ± 0.023 | 0.014 ± 0.005 | 0.079 ± 0.017 | |
XGBoost | 0.944 ± 0.013 | 0.098 ± 0.021 | 0.010 ± 0.004 | 0.061 ± 0.009 | |
Random Forest | 0.948 ± 0.014 | 0.094 ± 0.022 | 0.009 ± 0.004 | 0.059 ± 0.012 | |
GJR-GARCH | K-Nearest Neighbors | 0.829 ± 0.049 | 0.169 ± 0.030 | 0.029 ± 0.011 | 0.120 ± 0.022 |
AdaBoost | 0.877 ± 0.036 | 0.142 ± 0.022 | 0.021 ± 0.006 | 0.106 ± 0.019 | |
CatBoost | 0.922 ± 0.019 | 0.115 ± 0.025 | 0.014 ± 0.006 | 0.075 ± 0.013 | |
LightGBM | 0.923 ± 0.026 | 0.113 ± 0.021 | 0.013 ± 0.005 | 0.076 ± 0.015 | |
XGBoost | 0.942 ± 0.013 | 0.100 ± 0.020 | 0.010 ± 0.004 | 0.061 ± 0.009 | |
Random Forest | 0.946 ± 0.015 | 0.096 ± 0.023 | 0.010 ± 0.004 | 0.060 ± 0.014 | |
EGARCH | K-Nearest Neighbors | 0.829 ± 0.047 | 0.169 ± 0.029 | 0.029 ± 0.011 | 0.122 ± 0.019 |
AdaBoost | 0.881 ± 0.035 | 0.140 ± 0.022 | 0.020 ± 0.007 | 0.104 ± 0.018 | |
CatBoost | 0.921 ± 0.030 | 0.116 ± 0.031 | 0.014 ± 0.008 | 0.075 ± 0.015 | |
LightGBM | 0.920 ± 0.026 | 0.115 ± 0.021 | 0.014 ± 0.005 | 0.078 ± 0.015 | |
XGBoost | 0.942 ± 0.013 | 0.099 ± 0.019 | 0.010 ± 0.004 | 0.062 ± 0.008 | |
Random Forest | 0.948 ± 0.019 | 0.094 ± 0.024 | 0.009 ± 0.005 | 0.059 ± 0.014 |
Stock Code | Profit | Annualized Return | Maximum Drawdown |
JPM | [11086, 22675] | [3.520, 6.967] | [16.061, 23.929] |
BAC | [9246, 21605] | [2.924, 6.647] | [10.921, 15.958] |
WFC | [11256, 24170] | [3.546, 7.391] | [10.246, 14.231] |
GS | [8511, 25243] | [2.668, 7.592] | [15.562, 22.351] |
MS | [3452, 12961] | [1.068, 4.104] | [20.540, 26.880] |
PNC | [13971, 24450] | [4.394, 7.528] | [14.106, 18.843] |
BMO | [32221, 51386] | [9.599, 14.722] | [11.160, 15.410] |
STT | [7741, 15916] | [2.476, 5.020] | [13.636, 18.315] |
HSBC | [24742, 39152] | [7.541, 11.592] | [6.347, 9.870] |
AXP | [22786, 28597] | [7.080, 8.755] | [12.200, 18.408] |
FITB | [42923, 52183] | [12.657, 15.019] | [14.920, 21.174] |
MTB | [15122, 33634] | [4.677, 9.955] | [13.955, 18.412] |
Time Series | Profit | Annualized Return | Maximum Drawdown |
GARCH | [20219, 28328] | [6.162, 8.464] | [14.058, 17.235] |
GJR-GARCH | [19712, 28029] | [5.995, 8.369] | [14.530, 17.474] |
EGARCH | [17350, 25120] | [5.311, 7.560] | [14.654, 17.907] |
Machine Learning | Profit | Annualized Return | Maximum Drawdown |
K-Nearest Neighbors | [9769, 19029] | [3.073, 5.817] | [7.587, 10.744] |
AdaBoost | [17871, 26243] | [5.568, 7.978] | [14.491, 19.115] |
CatBoost | [20131, 31349] | [6.128, 9.372] | [16.080, 19.265] |
LightGBM | [18403, 29710] | [5.678, 8.860] | [16.732, 20.215] |
XGBoost | [21708, 33333] | [6.597, 9.905] | [16.480, 20.461] |
Random Forest | [17384, 32584] | [5.217, 9.526] | [12.799, 17.747] |
Time Series | Machine Learning | Profit | Annualized Return | Maximum Drawdown |
GARCH | K-Nearest Neighbors | [6764, 23861] | [2.211, 7.265] | [6.956, 12.600] |
AdaBoost | [12764, 29836] | [4.115, 8.965] | [12.057, 20.516] | |
CatBoost | [15203, 34345] | [4.781, 10.259] | [15.165, 20.838] | |
LightGBM | [16468, 35657] | [5.158, 10.625] | [14.408, 21.586] | |
XGBoost | [16621, 41346] | [5.127, 12.122] | [14.076, 22.399] | |
Random Forest | [13978, 44440] | [4.290, 12.834] | [8.108, 19.049] | |
GJR-GARCH | K-Nearest Neighbors | [4345, 23148] | [1.439, 7.018] | [5.811, 12.010] |
AdaBoost | [13889, 28065] | [4.385, 8.572] | [12.225, 21.674] | |
CatBoost | [16931, 40658] | [5.116, 12.041] | [14.648, 20.315] | |
LightGBM | [11334, 36605] | [3.681, 10.685] | [15.766, 21.783] | |
XGBoost | [18272, 41205] | [5.654, 12.097] | [14.185, 21.325] | |
Random Forest | [13007, 38988] | [4.089, 11.406] | [12.639, 19.639] | |
EGARCH | K-Nearest Neighbors | [5279, 22998] | [1.744, 6.992] | [5.625, 11.991] |
AdaBoost | [15454, 32338] | [4.885, 9.716] | [12.746, 21.598] | |
CatBoost | [12966, 34338] | [4.065, 10.239] | [14.025, 21.045] | |
LightGBM | [11966, 32312] | [3.842, 9.626] | [15.194, 22.106] | |
XGBoost | [14580, 33098] | [4.529, 9.975] | [15.709, 23.129] | |
Random Forest | [4681, 34812] | [1.483, 10.127] | [11.140, 21.061] |
Time Series | R Squared | RMSE | MSE | MAE |
GARCH | 0.907 ± 0.051 | 0.122 ± 0.036 | 0.016 ± 0.010 | 0.083 ± 0.028 |
GJR-GARCH | 0.907 ± 0.051 | 0.122 ± 0.035 | 0.016 ± 0.009 | 0.083 ± 0.027 |
EGARCH | 0.907 ± 0.051 | 0.122 ± 0.035 | 0.016 ± 0.010 | 0.083 ± 0.027 |
Machine Learning | R Squared | RMSE | MSE | MAE |
K-Nearest Neighbors | 0.830 ± 0.049 | 0.168 ± 0.030 | 0.029 ± 0.011 | 0.121 ± 0.021 |
AdaBoost | 0.879 ± 0.035 | 0.141 ± 0.023 | 0.020 ± 0.007 | 0.105 ± 0.019 |
CatBoost | 0.920 ± 0.027 | 0.117 ± 0.029 | 0.014 ± 0.007 | 0.075 ± 0.015 |
LightGBM | 0.921 ± 0.027 | 0.114 ± 0.022 | 0.013 ± 0.005 | 0.078 ± 0.016 |
XGBoost | 0.943 ± 0.013 | 0.099 ± 0.020 | 0.010 ± 0.004 | 0.061 ± 0.009 |
Random Forest | 0.948 ± 0.016 | 0.095 ± 0.023 | 0.010 ± 0.005 | 0.059 ± 0.013 |
Time Series | Machine Learning | R Squared | RMSE | MSE | MAE |
GARCH | K-Nearest Neighbors | 0.831 ± 0.049 | 0.168 ± 0.031 | 0.029 ± 0.011 | 0.121±0.021 |
AdaBoost | 0.880 ± 0.033 | 0.141 ± 0.023 | 0.020 ± 0.007 | 0.105 ± 0.019 | |
CatBoost | 0.917 ± 0.030 | 0.119 ± 0.031 | 0.015 ± 0.008 | 0.075 ± 0.016 | |
LightGBM | 0.921 ± 0.028 | 0.115 ± 0.023 | 0.014 ± 0.005 | 0.079 ± 0.017 | |
XGBoost | 0.944 ± 0.013 | 0.098 ± 0.021 | 0.010 ± 0.004 | 0.061 ± 0.009 | |
Random Forest | 0.948 ± 0.014 | 0.094 ± 0.022 | 0.009 ± 0.004 | 0.059 ± 0.012 | |
GJR-GARCH | K-Nearest Neighbors | 0.829 ± 0.049 | 0.169 ± 0.030 | 0.029 ± 0.011 | 0.120 ± 0.022 |
AdaBoost | 0.877 ± 0.036 | 0.142 ± 0.022 | 0.021 ± 0.006 | 0.106 ± 0.019 | |
CatBoost | 0.922 ± 0.019 | 0.115 ± 0.025 | 0.014 ± 0.006 | 0.075 ± 0.013 | |
LightGBM | 0.923 ± 0.026 | 0.113 ± 0.021 | 0.013 ± 0.005 | 0.076 ± 0.015 | |
XGBoost | 0.942 ± 0.013 | 0.100 ± 0.020 | 0.010 ± 0.004 | 0.061 ± 0.009 | |
Random Forest | 0.946 ± 0.015 | 0.096 ± 0.023 | 0.010 ± 0.004 | 0.060 ± 0.014 | |
EGARCH | K-Nearest Neighbors | 0.829 ± 0.047 | 0.169 ± 0.029 | 0.029 ± 0.011 | 0.122 ± 0.019 |
AdaBoost | 0.881 ± 0.035 | 0.140 ± 0.022 | 0.020 ± 0.007 | 0.104 ± 0.018 | |
CatBoost | 0.921 ± 0.030 | 0.116 ± 0.031 | 0.014 ± 0.008 | 0.075 ± 0.015 | |
LightGBM | 0.920 ± 0.026 | 0.115 ± 0.021 | 0.014 ± 0.005 | 0.078 ± 0.015 | |
XGBoost | 0.942 ± 0.013 | 0.099 ± 0.019 | 0.010 ± 0.004 | 0.062 ± 0.008 | |
Random Forest | 0.948 ± 0.019 | 0.094 ± 0.024 | 0.009 ± 0.005 | 0.059 ± 0.014 |
Stock Code | Profit | Annualized Return | Maximum Drawdown |
JPM | [11086, 22675] | [3.520, 6.967] | [16.061, 23.929] |
BAC | [9246, 21605] | [2.924, 6.647] | [10.921, 15.958] |
WFC | [11256, 24170] | [3.546, 7.391] | [10.246, 14.231] |
GS | [8511, 25243] | [2.668, 7.592] | [15.562, 22.351] |
MS | [3452, 12961] | [1.068, 4.104] | [20.540, 26.880] |
PNC | [13971, 24450] | [4.394, 7.528] | [14.106, 18.843] |
BMO | [32221, 51386] | [9.599, 14.722] | [11.160, 15.410] |
STT | [7741, 15916] | [2.476, 5.020] | [13.636, 18.315] |
HSBC | [24742, 39152] | [7.541, 11.592] | [6.347, 9.870] |
AXP | [22786, 28597] | [7.080, 8.755] | [12.200, 18.408] |
FITB | [42923, 52183] | [12.657, 15.019] | [14.920, 21.174] |
MTB | [15122, 33634] | [4.677, 9.955] | [13.955, 18.412] |
Time Series | Profit | Annualized Return | Maximum Drawdown |
GARCH | [20219, 28328] | [6.162, 8.464] | [14.058, 17.235] |
GJR-GARCH | [19712, 28029] | [5.995, 8.369] | [14.530, 17.474] |
EGARCH | [17350, 25120] | [5.311, 7.560] | [14.654, 17.907] |
Machine Learning | Profit | Annualized Return | Maximum Drawdown |
K-Nearest Neighbors | [9769, 19029] | [3.073, 5.817] | [7.587, 10.744] |
AdaBoost | [17871, 26243] | [5.568, 7.978] | [14.491, 19.115] |
CatBoost | [20131, 31349] | [6.128, 9.372] | [16.080, 19.265] |
LightGBM | [18403, 29710] | [5.678, 8.860] | [16.732, 20.215] |
XGBoost | [21708, 33333] | [6.597, 9.905] | [16.480, 20.461] |
Random Forest | [17384, 32584] | [5.217, 9.526] | [12.799, 17.747] |
Time Series | Machine Learning | Profit | Annualized Return | Maximum Drawdown |
GARCH | K-Nearest Neighbors | [6764, 23861] | [2.211, 7.265] | [6.956, 12.600] |
AdaBoost | [12764, 29836] | [4.115, 8.965] | [12.057, 20.516] | |
CatBoost | [15203, 34345] | [4.781, 10.259] | [15.165, 20.838] | |
LightGBM | [16468, 35657] | [5.158, 10.625] | [14.408, 21.586] | |
XGBoost | [16621, 41346] | [5.127, 12.122] | [14.076, 22.399] | |
Random Forest | [13978, 44440] | [4.290, 12.834] | [8.108, 19.049] | |
GJR-GARCH | K-Nearest Neighbors | [4345, 23148] | [1.439, 7.018] | [5.811, 12.010] |
AdaBoost | [13889, 28065] | [4.385, 8.572] | [12.225, 21.674] | |
CatBoost | [16931, 40658] | [5.116, 12.041] | [14.648, 20.315] | |
LightGBM | [11334, 36605] | [3.681, 10.685] | [15.766, 21.783] | |
XGBoost | [18272, 41205] | [5.654, 12.097] | [14.185, 21.325] | |
Random Forest | [13007, 38988] | [4.089, 11.406] | [12.639, 19.639] | |
EGARCH | K-Nearest Neighbors | [5279, 22998] | [1.744, 6.992] | [5.625, 11.991] |
AdaBoost | [15454, 32338] | [4.885, 9.716] | [12.746, 21.598] | |
CatBoost | [12966, 34338] | [4.065, 10.239] | [14.025, 21.045] | |
LightGBM | [11966, 32312] | [3.842, 9.626] | [15.194, 22.106] | |
XGBoost | [14580, 33098] | [4.529, 9.975] | [15.709, 23.129] | |
Random Forest | [4681, 34812] | [1.483, 10.127] | [11.140, 21.061] |