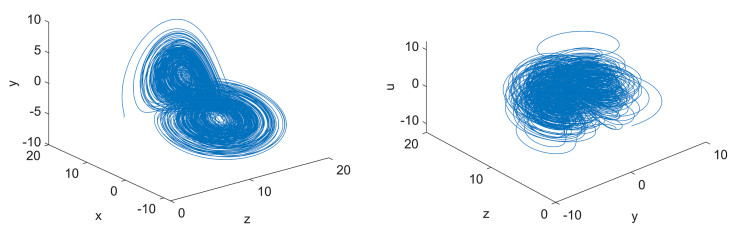
Traffic surveillance systems are utilized to collect and monitor the traffic condition data of the road networks. This data plays a crucial role in a variety of applications of the Intelligent Transportation Systems (ITSs). In traffic surveillance, it is challenging to achieve accurate vehicle detection and count the vehicles from traffic videos. The most notable difficulties include real-time system operations for precise classification, identification of the vehicles' location in traffic flows and functioning around total occlusions that hamper the vehicle tracking process. Conventional video-related vehicle detection techniques such as optical flow, background subtraction and frame difference have certain limitations in terms of efficiency or accuracy. Therefore, the current study proposes to design the spotted hyena optimizer with deep learning-enabled vehicle counting and classification (SHODL-VCC) model for the ITSs. The aim of the proposed SHODL-VCC technique lies in accurate counting and classification of the vehicles in traffic surveillance. To achieve this, the proposed SHODL-VCC technique follows a two-stage process that includes vehicle detection and vehicle classification. Primarily, the presented SHODL-VCC technique employs the RetinaNet object detector to identify the vehicles. Next, the detected vehicles are classified into different class labels using the deep wavelet auto-encoder model. To enhance the vehicle detection performance, the spotted hyena optimizer algorithm is exploited as a hyperparameter optimizer, which considerably enhances the vehicle detection rate. The proposed SHODL-VCC technique was experimentally validated using different databases. The comparative outcomes demonstrate the promising vehicle classification performance of the SHODL-VCC technique in comparison with recent deep learning approaches.
Citation: Manal Abdullah Alohali, Mashael Maashi, Raji Faqih, Hany Mahgoub, Abdullah Mohamed, Mohammed Assiri, Suhanda Drar. Spotted hyena optimizer with deep learning enabled vehicle counting and classification model for intelligent transportation systems[J]. Electronic Research Archive, 2023, 31(7): 3704-3721. doi: 10.3934/era.2023188
[1] | Ning Cui, Junhong Li . A new 4D hyperchaotic system and its control. AIMS Mathematics, 2023, 8(1): 905-923. doi: 10.3934/math.2023044 |
[2] | Junhong Li, Ning Cui . Dynamical behavior and control of a new hyperchaotic Hamiltonian system. AIMS Mathematics, 2022, 7(4): 5117-5132. doi: 10.3934/math.2022285 |
[3] | Figen Kangalgil, Seval Isșık . Effect of immigration in a predator-prey system: Stability, bifurcation and chaos. AIMS Mathematics, 2022, 7(8): 14354-14375. doi: 10.3934/math.2022791 |
[4] | Sukono, Siti Hadiaty Yuningsih, Endang Rusyaman, Sundarapandian Vaidyanathan, Aceng Sambas . Investigation of chaos behavior and integral sliding mode control on financial risk model. AIMS Mathematics, 2022, 7(10): 18377-18392. doi: 10.3934/math.20221012 |
[5] | Minghung Lin, Yiyou Hou, Maryam A. Al-Towailb, Hassan Saberi-Nik . The global attractive sets and synchronization of a fractional-order complex dynamical system. AIMS Mathematics, 2023, 8(2): 3523-3541. doi: 10.3934/math.2023179 |
[6] | Binhao Hong, Chunrui Zhang . Bifurcations and chaotic behavior of a predator-prey model with discrete time. AIMS Mathematics, 2023, 8(6): 13390-13410. doi: 10.3934/math.2023678 |
[7] | Wei Li, Qingkai Xu, Xingjian Wang, Chunrui Zhang . Dynamics analysis of spatiotemporal discrete predator-prey model based on coupled map lattices. AIMS Mathematics, 2025, 10(1): 1248-1299. doi: 10.3934/math.2025059 |
[8] | A. E. Matouk . Chaos and hidden chaos in a 4D dynamical system using the fractal-fractional operators. AIMS Mathematics, 2025, 10(3): 6233-6257. doi: 10.3934/math.2025284 |
[9] | Xiaoyan Hu, Bo Sang, Ning Wang . The chaotic mechanisms in some jerk systems. AIMS Mathematics, 2022, 7(9): 15714-15740. doi: 10.3934/math.2022861 |
[10] | Zhao Li, Shan Zhao . Bifurcation, chaotic behavior and solitary wave solutions for the Akbota equation. AIMS Mathematics, 2024, 9(8): 22590-22601. doi: 10.3934/math.20241100 |
Traffic surveillance systems are utilized to collect and monitor the traffic condition data of the road networks. This data plays a crucial role in a variety of applications of the Intelligent Transportation Systems (ITSs). In traffic surveillance, it is challenging to achieve accurate vehicle detection and count the vehicles from traffic videos. The most notable difficulties include real-time system operations for precise classification, identification of the vehicles' location in traffic flows and functioning around total occlusions that hamper the vehicle tracking process. Conventional video-related vehicle detection techniques such as optical flow, background subtraction and frame difference have certain limitations in terms of efficiency or accuracy. Therefore, the current study proposes to design the spotted hyena optimizer with deep learning-enabled vehicle counting and classification (SHODL-VCC) model for the ITSs. The aim of the proposed SHODL-VCC technique lies in accurate counting and classification of the vehicles in traffic surveillance. To achieve this, the proposed SHODL-VCC technique follows a two-stage process that includes vehicle detection and vehicle classification. Primarily, the presented SHODL-VCC technique employs the RetinaNet object detector to identify the vehicles. Next, the detected vehicles are classified into different class labels using the deep wavelet auto-encoder model. To enhance the vehicle detection performance, the spotted hyena optimizer algorithm is exploited as a hyperparameter optimizer, which considerably enhances the vehicle detection rate. The proposed SHODL-VCC technique was experimentally validated using different databases. The comparative outcomes demonstrate the promising vehicle classification performance of the SHODL-VCC technique in comparison with recent deep learning approaches.
The concept of the hyperchaos was first put forward by Rössler [1] in 1979. Any system with at least two positive Lyapunov exponents is defined as hyperchaotic. Compared to chaotic attractors, hyperchaotic attractors have more complicated dynamical phenomena and stronger randomness and unpredictability. Hyperchaotic systems have aroused wide interest from more and more researchers in the last decades. A number of papers have investigated various aspects of hyperchaos and many valuable results have been obtained. For instance, in applications, in order to improve the security of the cellular neural network system, the chaotic degree of the system can be enhanced by designing 5D memristive hyperchaotic system [2]. For higher computational security, a new 4D hyperchaotic cryptosystem was constructed by adding a new state to the Lorenz system and well used in the AMr-WB G.722.2 codec to fully and partially encrypt the speech codec [3]. In fact, hyperchaos has a wide range applications such as image encryption [4], Hopfield neural network [5] and secure communication [6] and other fields [7,8]. Meanwhile, there are many hyperchaotic systems have been presented so far. Aimin Chen and his cooperators constructed a 4D hyperchaotic system by adding a state feedback controller to Lü system [9]. Based on Chen system, Z. Yan presented a new 4D hyperchaotic system by introducing a state feedback controller [10]. By adding a controlled variable, Gao et al. introduced a new 4D hyperchaotic Lorenz system [11]. Likewise, researchers also formulated 5D Shimizu-Morioka-type hyperchaotic system [12], 5D hyperjerk hypercaotic system [13] and 4D T hyperchaotic system [14] and so on.
In [15], a chaotic Rabinovich system was introduced
{˙x=hy−ax+yz,˙y=hx−by−xz,˙z=−cz+xy, | (1.1) |
where (x,y,z)T∈R3 is the state vector. When (h,a,b,c)=(0.04,1.5,−0.3,1.67), (1.1) has chaotic attractor[15,16]. System (1.1) has similar properties to Lorenz system, the two systems can be considered as special cases of generalized Lorenz system in [17]. Liu and his cooperators formulated a new 4D hyperchaotic Rabinovich system by adding a linear controller to the 3D Rabinovich system [18]. The circuit implementation and the finite-time synchronization for the 4D hyperchaotic Rabinovich system was also studied in [19]. Reference [20] formulated a 4D hyperchaotic Rabinovich system and the dynamical behaviors were studied such as the hidden attractors, multiple limit cycles and boundedness. Based on the 3D chaotic Rabinovich system, Tong et al. presented a new 4D hyperchaotic system by introducing new state variable [21]. The hyperchaos can be generated by adding variables to a chaotic system, which has been verificated by scholars [3,9,10,11,14]. In [18,19,20,21], the hyperchaotic systems were presented by adding a variable to the second equation of system (1.1). In fact, hyperchaos can also be generated by adding a linear controller to the first equation and second equation of system (1.1). Based on it, the following hyperchaotic system is obtained
{˙x=hy−ax+yz+k0u,˙y=hx−by−xz+mu,˙z=−dz+xy,˙u=−kx−ky, | (1.2) |
where k0,h,a,b,d,k,k0,m are positive parameters. Like most hyperchaotic studies (see [14,22,23,24] and so on), the abundant dynamical properties of system (1.2) are investigated by divergence, phase diagrams, equilibrium points, Lyapunov exponents, bifurcation diagram and Poincaré maps. The results show that the new 4D Rabinovich system not only exhibit hyperchaotic and Hopf bifurcation behaviors, but also has the rich dynamical phenomena including periodic, chaotic and static bifurcation. In addition, the 4D projection figures are also given for providing more dynamical information.
The rest of this paper is organized as follows: In the next section, boundedness, dissipativity and invariance, equilibria and their stability of (1.2) are discussed. In the third section, the complex dynamical behaviors such as chaos and hyperchaos are numerically verified by Lyapunov exponents, bifurcation and Poincaré section. In the fourth section, the Hopf bifurcation at the zero equilibrium point of the 4D Rabinovich system is investigated. In addition, an example is given to test and verify the theoretical results. Finally, the conclusions are summarized in the last section.
Theorem 2.1. If k0>m, system (1.2) has an ellipsoidal ultimate bound and positively invariant set
Ω={(x,y,z,u)|mx2+k0y2+(k0−m)[z−h(k0+m)k0−m]2+k0mu2k≤M}, |
where
M={14h2d2(m+k0)2(k0−m)a(d−a),(k0>m,d>a),14h2d2(m+k0)2(k0−m)b(−b+d),(k0>m,d>b),(m+k0)2h2k0−m,(k0>m). | (2.1) |
Proof. V(x,y,z,u)=mx2+k0y2+(k0−m)[z−h(k0+m)k0−m]2+k0mu2k. Differentiating V with respect to time along a trajectory of (1.2), we obtain
˙V(x,y,z,u)2=−amx2−by2k0+dhmz+dhzk0+dmz2−dz2k0. |
When ˙V(x,y,z,u)2=0, we have the following ellipsoidal surface:
Σ={(x,y,z,u)|amx2+bk0y2+d(k0−m)(z−hm+hk02k0−2m)2=dh2(k0+m)24(k0−m)}. |
Outside Σ that is,
amx2+bk0y2+d(k0−m)(z−hm+hk02k0−2m)2<dh2(k0+m)24(k0−m), |
˙V<0, while inside Σ, that is,
amx2+bk0y2+d(k0−m)(z−hm+hk02k0−2m)2>dh2(k0+m)24(k0−m), |
˙V>0. Thus, the ultimate bound for system (1.2) can only be reached on Σ. According to calculation, the maximum value of V on Σ is Vmax=14h2d2(m+k0)2(k0−m)a(d−a),(k0>m,d>a) and
Vmax=14h2d2(m+k0)2(k0−m)b(−b+d),(k0>m,d>b);Vmax=(m+k0)2h2k0−m,(k0>m). |
In addition, Σ⊂Ω, when a trajectory X(t)=(x(t),y(t),z(t),u(t)) of (1.2) is outside Ω, we get ˙V(X(t))<0. Then, limt→+∞ρ(X(t,t0,X0),Ω)=0. When X(t)∈Ω, we also get ˙V(X(t))<0. Thus, any trajectory X(t) (X(t)≠X0) will go into Ω. Therefore, the conclusions of theorem is obtained.
We can see that system (1.2) is invariant for the coordinate transformation
(x,y,z,u)→(−x,−y,z,−u). |
Then, the nonzero equilibria of (1.2) is symmetric with respect to z axis. The divergence of (1.2) is
∇W=∂˙x∂x+∂˙y∂y+∂˙z∂z+∂˙u∂u=−(a+b+d), |
system (1.2) is dissipative if and only if a+b+d>0. It shows that each volume containing the system trajectories shrinks to zero as t→∞ at an exponential rate −(a+b+d).
For m≤k0, system (1.2) only has one equilibrium point O(0,0,0,0) and the Jacobian matrix at O is
J=[−ah0k0h−b0m00−d0−k−k00]. |
Then, the characteristic equation is
(d+λ)(λ3+s2λ2+s1λ+s0), | (2.2) |
where
s2=a+b,s1=ab−h2+km+kk0,s0=akm+bkk0+hkm+hkk0. |
According to Routh-Hurwitz criterion [25], the real parts of eigenvalues are negative if and only if
d>0,a+b>0,(a+b)(ab−h2)+k(ak0+bm−hm−hk0)>0. | (2.3) |
When m>k0, system (1.2) has other two nonzero equilibrium points E1(x∗1,y∗1,z∗1,u∗1) and E2(−x∗1,−y∗1,z∗1,−u∗1), where
x∗1=√d(am+bk0+hm+hk0)m−k0,y∗1=−√d(am+bk0+hm+hk0)m−k0, |
z∗1=−am+bk0+hm+hk0m−k0,u∗1=−a+b+2hm−k0√d(am+bk0+hm+hk0)m−k0. |
The characteristic equation of Jacobi matrix at E1 and E2 is
λ4+δ3λ3+δ2λ2+δ1λ+δ0=0, |
where
δ3=a+b+d,δ2=x∗14d2+ab+ad+bd−h2+km+kk0, |
δ1=1d[x∗12(3x∗12+ad−bd+kk0−km)+k(m+k0)(d+h)]+abd+akm+bkk0−dh2, |
δ0=3kk0x∗12−3kmx∗12+akmd+bkk0d+hkk0d+hkmd. |
Based on Routh-Hurwitz criterion [25], the real parts of eigenvalues are negative if and only if
δ0>0,δ3>0,δ3δ2−δ1>0,δ3δ2δ1−δ21−δ0δ23>0. | (2.4) |
Therefore, we have:
Theorem 2.2. (I) When m≤k0, system (1.2) only has one equilibrium point O(0,0,0,0) and O is asymptotically stable if and only if (2.3) is satisfied.
(II) when m>k0, system (1.2) has two nonzero equilibria E1, E2, (2.4) is the necessary and sufficient condition for the asymptotically stable of E1 and E2.
When (b,d,h,a,k0,k,m)=(1,1,10,10,0.8,0.8,0.8), system (1.2) only has zero-equilibrium point E0(0,0,0,0,0), its corresponding characteristic roots are: −1,0.230,5.232,−16.462, E0 is unstable. The Lyapunov exponents are: LE1=0.199, LE2=0.083, LE3=0.000, LE4=−12.283, system (1.2) is hyperchaotic. Figure 1 shows the hyperchaotic attractors on z−x−y space and y−z−u space. The Poincaré mapping on the x−z plane and power spectrum of time series x(t) are depicted in Figure 2.
In the following, we fix b=1,d=1,h=10,a=10,k0=0.8,m=0.8, Figure 3 indicates the Lyapunov exponent spectrum of system (1.2) with respect to k∈[0.005,1.8] and the corresponding bifurcation diagram is given in Figure 4. These simulation results illustrate the complex dynamical phenomena of system (1.2). When k varies in [0.005,1.8], there are two positive Lyapunov exponents, system (1.2) is hyperchaoic as k varies.
Assume b=1,d=1,h=10,k=0.8,k0=0.8,m=0.8, the different Lyapunov exponents and dynamical properties with different values of parameter a are given in Table 1. It shows that system (1.2) has rich dynamical behaviors including periodic, chaos and hyperchaos with different parameters. The bifurcation diagram of system (1.2) with a∈[0.5,5] is given in Figure 5. Therefore, we can see that periodic orbits, chaotic orbits and hyperchaotic orbits can occur with increasing of parameter a. When a=1.08, Figure 6 indicates the (z,x,y,u) 4D surface of section and the location of the consequents is given in the (z,x,y) subspace and are colored according to their u value. The chaotic attractor and hyperchaotic attractor on y−x−z space and hyperchaotic attractor on y−z−u space are given in Figures 7 and 8, respectively. The Poincaré maps on x−z plane with a=2 and a=4 are depicted in Figure 9.
a | LE1 | LE2 | LE3 | LE4 | Dynamics |
1.08 | 0.000 | −0.075 | −4.661 | −2.538 | Periodic |
2 | 0.090 | 0.000 | −0.395 | −3.696 | Chaos |
4 | 0.325 | 0.047 | −0.000 | −6.373 | Hyperchaos |
Theorem 4.1. Suppose that ab>h2 and k0(a−h)+m(b−h)<0 are satisfied. Then, as m varies and passes through the critical value k=ah2+bh2−a2b−ab2ak0+bm−hm−hk0, system (1.2) undergoes a Hopf bifurcation at O(0,0,0,0).
Proof. Assume that system (1.2) has a pure imaginary root λ=iω,(ω∈R+). From (2.2), we get
s2ω2−s0=0,ω3−s1ω=0, |
then
ω=ω0=√ab−h2+k(k0+m), |
k=k∗=(a+b)(h2−ab)k0(a−h)+m(b−h). |
Substituting k=k∗ into (2.2), we have
λ1=iω,λ2=iω,λ3=−d,λ4=−(a+b). |
Therefore, when ab>h2, k0(a−h)+m(b−h)<0 and k=k∗, the first condition for Hopf bifurcation [26] is satisfied. From (2.2), we have
Re(λ′(k∗))|λ=iω0=h(k0+m)−ak0−bm2(s22+s1)<0, |
Thus, the second condition for a Hopf bifurcation [26] is also met. Hence, Hopf bifurcation exists.
Remark 4.1. When ab−h2+k(k0+m)≤0, system (1.2) has no Hopf bifurcation at the zero equilibrium point.
Theorem 4.2. When ab>h2 and k0(a−h)+m(b−h)<0, the periodic solutions of (1.2) from Hopf bifurcation at O(0,0,0,0) exist for sufficiently small
0<|k−k∗|=|k−(a+b)(h2−ab)k0(a−h)+m(b−h)|. |
And the periodic solutions have the following properties:
(I) if δ1g>0 (resp., δ1g<0), the hopf bifurcation of system (1.2) at (0,0,0,0) is non-degenerate and subcritical (resp. supercritical), and the bifurcating periodic solution exists for m>m∗ (resp., m<m∗) and is unstable (resp., stable), where
δ1g=14dδ(−k2δ01k02+ahδ01s1+kδ01k0s1−2dδ03δ05+2dδ04δ06),δ=ω0[(−ab+h2)(akk0+hkk0−hs1)+k(bm−hk0)(ha+h2+kk0)−k(ak−hm)(a2+ha−kk0+s1)],δ01=kω0(a2bm+a2hk−a2hk0+abhm+ah2k−ah2m−ah2k0+ak2k0−bkmk0−h3m−hkmk0+hkk02+bms1−hk0s1),δ03=ω0(a2bkm−2a2bkk0+a2hk2−a2hkk0+abhkm+2abhkk0+ah2k2−ah2km+ah2kk0−ak3k0+bk2mk0−h3km−2h3kk0+hk2mk0−hk2k02+2a2bs1−2ah2s1−bkms1+hkk0s1),δ04=(−ab+h2)s12+(a3b−a2h2+abh2−2abkm+2abkk0−ahk2+2ahkk0−bhkm−h4+h2km−h2kk0)s1−a2k3k0+abk2mk0−2abk2k02−ahk3k0+ahk2mk0−ahk2k02+bhk2mk0+h2k2mk0+h2k2k02,δ05=12d2+8s1[2ω0(−kk0aω0+hkk0ω0−hω0s1)+d(−hω02a−k2k02+kk0s1],δ06=12d2+8s1[d(−kk0aω0+hkk0ω0−hω0s1)−2ω0(k2k02+ahs1−kk0s1)]. |
(II) The period and characteristic exponent of the bifurcating periodic solution are:
T=2πω0(1+τ2∗ϵ2+O(ϵ4)),β=β2ϵ2+O(ϵ4), |
where ϵ=k−k∗μ2+O[(k−k∗)2] and
μ2=−ReC1(0)α′(0)=−(s22+s1)δ1gh(k0+m)−ak0−bm, |
τ2∗=δ2gω0−δ1g(ams2+bk0s2+hms2+hk0s2+ms1+k0s1)s1(h(k0+m)−ak0−bm), |
β2=δ1g, |
δ2g=14dδ(−k2δ02k02+ahδ02s1+kδ02k0s1−2δ03δ06d−2δ04δ05d). |
(III) The expression of the bifurcating periodic solution is
[xyzu]=[−kk0ϵcos(2πtT)−hω0ϵsin(2πtT)(kk0−s1)ϵcos(2πtT)−aω0ϵsin(2πtT)ϵ2[−kk0(kk0−s1)+hs1a2d+δ05−δ06sin(4πtT)]−k(a+h)ϵcos(2πtT)+kω0ϵsin(2πtT)]+O(ϵ3). |
Proof. Let k=k∗, by straightforward computations, we can obtain
t1=[ihω0−kk0kk0−s1+iaω00−(iω0+a+h)k],t3=[0010],t4=[ak0−hmbm−hk00h2−ab], |
which satisfy
Jt1=iω0t1,Jt3=−dt3,Jt4=−(a+b)t4. |
Now, we use transformation X=QX1, where X=(x,y,z,u)T, X1=(x1,y1,z1,u1)T, and
Q=[−kk0−hω00ak−hmkk0−s1−aω00bm−hk00010−k(a+h)kω00h2−ab], |
then, system (1.2) is transformed into
{˙x1=−ω0y1+F1(x1,y1,z1,u1),˙y1=ω0x1+F2(x1,y1,z1,u1),˙z1=−dz1+F3(x1,y1,z1,u1),˙u1=−(a+b)u1+F4(x1,y1,z1,u1), | (4.1) |
where
δ=ω0 [(−ab+h2)(akk0+hkk0−hs1)+k(bm−hk0)(ha+h2+kk0)−k(ak−hm) (a2+ha−kk0+s1)],
F1(x1,y1,z1,u1) =1δz1(f11x1+f12y1+f13u1),
f11=−kk0ω0(abh−ak2−h3+hkm)+ (kk0−s1)ω0(a2b−ah2−bkm+hkk0),
f12=−kk0ω0(abh−ak2−h3+hkm)+ (kk0−s1)ω0(a2b−ah2−bkm+hkk0),
f13=−kk0ω0(abh−ak2−h3+hkm)+ (kk0−s1)ω0(a2b−ah2−bkm+hkk0),
F2(x1,y1,z1,u1)= −1δz1(f21x1+f22y1+f23u1),
f21=(−ab+h2)(2k2k02−2kk0s1+s12) −k(a+h)(ak2k0−bkmk0−hkmk0+ hkk02+bms1−hk0s1),
f22=−aω0[abk(m−k0)−hk(ak0−bm)] +abs1−h2s1]−hω0[ak(ak+bk0+hk−hm)−h2k(m+k0)],
f23=(h2−ab)[hkk0(m−k0)−kk0(ak−bm)−s1(bm−hk0)]+k(a+h)[ak(ak−2hm) +bm(bm−2hk0)+h2(m2+k02)],
F3(x1,y1,z1,u1)= [−kk0x1−hω0y1+(ak−hm)u1][(kk0−s1)x1−aω0y1+(bm−hk0)u1],
F4(x1,y1,z1,u1)= 1δz1(f41x1+f42y1+f43u1),
f41=kk0 (hω0ka+h2ω0k+k2k0ω0)− (kk0−s1)kω0(a2+ah−kk0+s1),
f42= a3ω02k+a2ω02kh+h2ω02ka−ak2k0ω02+h3 ω02k+k2k0hω02+ akω02s1,
f43=−a2bkmω0−a2hk2ω0+ a2hkk0ω0−abhkmω0−ah2k2 ω0+ah2kmω0+ah2kk0ω0−ak3 k0ω0 +bk2mk0ω0+ h3kmω0+hk2mk0ω0−hk2k02ω0−bkm ω0s1+ hkk0ω0s1.
Furthermore,
g11=14[∂2F1∂x21+∂2F1∂y21+i(∂2F2∂x21+∂2F2∂y21)]=0, |
g02=14[∂2F1∂x21−∂2F1∂y21−2∂2F2∂x1∂y1+i(∂2F2∂x21−∂2F2∂y21+2∂2F1∂x1∂y1)]=0, |
g20=14[∂2F1∂x21−∂2F1∂y21+2∂2F2∂x1∂y1+i(∂2F2∂x21−∂2F2∂y21−2∂2F1∂x1∂y1)]=0, |
G21=18[∂3F1∂x31+∂3F2∂y31+∂3F1∂x1∂y21+∂3F2∂x21∂y1+i(∂3F2∂x31−∂3F2∂y31+∂3F2∂x1∂y21−∂3F1∂x21∂y1)]=0. |
By solving the following equations
[−d00−(a+b)][ω111ω211]=−[h111h211],[−d−2iω000−(a+b)−2iω0][ω120ω220]=−[h120h220], |
where
h111=12[(kk0+ah)s1−k2k02], |
h211=14(∂2F4∂x21+∂2F4∂y21)=0, |
h120=12[−k2k02−hs1a+kk0s1+(hkk0ω0−kk0aω0−hω0s1)i], |
h220=14(∂2F4∂x21−∂2F4∂y21−2i∂2F4∂x1∂y1)=0, |
one obtains
ω111=12d[hs1a−kk0(kk0−s1)],ω211=0,ω220=0, |
ω120=12d2+8s1{2ω0(−kk0aω0+hkk0ω0−hω0s1)+d(−hω02a−k2k02+kk0s1) |
+[d(−kk0aω0+hkk0ω0−hω0s1)−2ω0(k2k02+ahs1−kk0s1)]i}, |
G1110=12[(∂2F1∂x1∂z1+∂2F2∂y1∂z1)+i(∂2F2∂x1∂z1−∂2F1∂y1∂z1)]=12δ(δ01+δ02i), |
where
δ01=kω0(a2bm+a2hk−a2hk0+abhm+ah2k−ah2m−ah2k0+ak2k0−bkmk0−h3m−hkmk0+hkk02+bms1−hk0s1),δ02=(ab−h2)s12+(a3b−a2h2+abh2−2abkk0−ahk2+bhkm−h4+h2km+h2kk0)s1+k2k0(a2k−bma+2abk0+ahk−ahm+ahk0−bhm−h2m−h2k0),G2110=12[(∂2F1∂x1∂u1+∂2F2∂y1∂u1)+i(∂2F2∂x1∂u1−∂2F1∂y1∂u1)]=0,G1101=12[(∂2F1∂x1∂z1−∂2F2∂y1∂z1)+i(∂2F2∂x1∂z1+∂2F1∂y1∂z1)]=−12δ[δ03+δ04i], |
where
δ03=ω0(a2bkm−2a2bkk0+a2hk2−a2hkk0+abhkm+2abhkk0+ah2k2−ah2km+ah2kk0−ak3k0+bk2mk0−h3km−2h3kk0+hk2mk0−hk2k02+2a2bs1−2ah2s1−bkms1+hkk0s1),δ04=(−ab+h2)s12+(a3b−a2h2+abh2−2abkm+2abkk0−ahk2+2ahkk0−bhkm−h4+h2km−h2kk0)s1−a2k3k0+abk2mk0−2abk2k02−ahk3k0+ahk2mk0−ahk2k02+bhk2mk0+h2k2mk0+h2k2k02, |
G2101=12[(∂2F1∂x1∂u1−∂2F2∂y1∂u1)+i(∂2F2∂x1∂u1+∂2F1∂y1∂u1)]=0, |
g21=G21+2∑j=1(2Gj110ωj11+Gj101ωj20)=δ1g+δ2gi, |
where
δ1g=14dδ(−k2δ01k02+ahδ01s1+kδ01k0s1−2dδ03δ05+2dδ04δ06), |
δ2g=14dδ(−k2δ02k02+ahδ02s1+kδ02k0s1−2δ03δ06d−2δ04δ05d), |
δ05=12d2+8s1[2ω0(−kk0aω0+hkk0ω0−hω0s1)+d(−hω02a−k2k02+kk0s1)], |
δ06=12d2+8s1[d(−kk0aω0+hkk0ω0−hω0s1)−2ω0(k2k02+ahs1−kk0s1)]. |
Based on above calculation and analysis, we get
C1(0)=i2ω0(g20g11−2|g11|2−13|g02|2)+12g21=12g21, |
μ2=−ReC1(0)α′(0)=−(s22+s1)δ1gh(k0+m)−ak0−bm, |
τ2∗=δ2gω0−δ1g(ams2+bk0s2+hms2+hk0s2+ms1+k0s1)s1(h(k0+m)−ak0−bm), |
where
ω′(0)=ω0(ams2+bk0s2+hms2+hk0s2+ms1+k0s1)s1s22+s21, |
α′(0)=h(k0+m)−ak0−bm2(s22+s1),β2=2ReC1(0)=δ1g. |
Note α′(0)<0. From ab>h2 and k0(a−h)+m(b−h)<0, we obtain that if δ1g>0 (resp., δ1g<0), then μ2>0 (resp., μ2<0) and β2>0 (resp., β2<0), the hopf bifurcation of system (1.2) at (0,0,0,0) is non-degenerate and subcritical (resp. supercritical), and the bifurcating periodic solution exists for k>k∗ (resp., k<k∗) and is unstable (resp., stable).
Furthermore, the period and characteristic exponent are
T=2πω0(1+τ2∗ϵ2+O(ϵ4)),β=β2ϵ2+O(ϵ4), |
where ϵ=k−k∗μ2+O[(k−k∗)2]. And the expression of the bifurcating periodic solution is (except for an arbitrary phase angle)
X=(x,y,z,u)T=Q(¯y1,¯y2,¯y3,¯y4)T=QY, |
where
¯y1=Reμ,¯y1=Imμ,(¯y3,¯y4,¯y5)T=ω11|μ|2+Re(ω20μ2)+O(|μ|2), |
and
μ=ϵe2itπT+iϵ26ω0[g02e−4itπT−3g20e4itπT+6g11]+O(ϵ3)=ϵe2itπT+O(ϵ3). |
By computations, we can obtain
[xyzu]=[−kk0ϵcos(2πtT)−hω0ϵsin(2πtT)(kk0−s1)ϵcos(2πtT)−aω0ϵsin(2πtT)ϵ2[−kk0(kk0−s1)+hs1a2d+δ05−δ06sin(4πtT)]−k(a+h)ϵcos(2πtT)+kω0ϵsin(2πtT)]+O(ϵ3). |
Based on the above discussion, the conclusions of Theorem 4.2 are proved.
In order to verify the above theoretical analysis, we assume
d=2,h=1,k0=1,m=2,b=1.5,a=0.5. |
According to Theorem 4.1, we get k∗=1. Then from Theorem 4.2, μ2=−4.375 and β2=−0.074, which imply that the Hopf bifurcation of system (1.2) at (0,0,0,0) is nondegenerate and supercritical, a bifurcation periodic solution exists for k<k∗=1 and the bifurcating periodic solution is stable. Figure 10 shows the Hopf periodic solution occurs when k=0.999<k∗=1.
In this paper, we present a new 4D hyperchaotic system by introducing a linear controller to the first equation and second equation of the 3D Rabinovich system, respectively. If k0=0, m=1 and the fourth equation is changed to −ky, system (1.2) will be transformed to 4D hyperchaotic Rabinovich system in [18,19]. Compared with the system in [18], the new 4D system (1.1) has two nonzero equilibrium points which are symmetric about z axis when m>k0 and the dynamical characteristics are more abundant. The complex dynamical behaviors, including boundedness, dissipativity and invariance, equilibria and their stability, chaos and hyperchaos of (1.2) are investigated and analyzed. Furthermore, the existence of Hopf bifurcation, the stability and expression of the Hopf bifurcation at zero-equilibrium point are studied by using the normal form theory and symbolic computations. In order to analyze and verify the complex phenomena of the system, some numerical simulations are carried out including Lyapunov exponents, bifurcations and Poincaré maps, et al. The results show that the new 4D Rabinovich system can exhibit complex dynamical behaviors, such as periodic, chaotic and hyperchaotic. In the real practice, the hyperchaotic Rabinovich system can be applied to generate key stream for the entire encryption process in image encryption scheme [27]. In some cases, hyperchaos and chaos are usually harmful and need to be suppressed such as in biochemical oscillations [8] and flexible shaft rotating-lifting system [28]. Therefore, we will investigate hyperchaos control and chaos control in further research.
Project supported by the Doctoral Scientific Research Foundation of Hanshan Normal University (No. QD202130).
The authors declare that they have no conflicts of interest.
[1] | V. Kocur, M. Ftacnik, Multi-class multi-movement vehicle counting based on CenterTrack, in Proceedings of the IEEE/CVF Conference on Computer Vision and Pattern Recognition, 2021, 4009–4015. |
[2] | J. Mirthubashini, V. Santhi, Video based vehicle counting using deep learning algorithms, in 2020 6th International Conference on Advanced Computing and Communication Systems (ICACCS), IEEE, (2020), 142–147. |
[3] |
C. J. Lin, S. Y. Jeng, H. W. Lioa, A real-time vehicle counting, speed estimation, and classification system based on virtual detection zone and YOLO, Math. Probl. Eng., 2021 (2021), 1–10. https://doi.org/10.1155/2021/1577614 doi: 10.1155/2021/1577614
![]() |
[4] |
A. Glowacz, Thermographic fault diagnosis of shaft of BLDC motor, Sensors, 22 (2022), 8537. https://doi.org/10.3390/s22218537 doi: 10.3390/s22218537
![]() |
[5] |
H. Xu, Z. Cai, R. Li, W. Li, Efficient citycam-to-edge cooperative learning for vehicle counting in ITS, IEEE Trans. Intell. Transp. Syst., 23 (2022), 16600–16611. https://doi.org/10.1109/TITS.2022.3149657 doi: 10.1109/TITS.2022.3149657
![]() |
[6] | Y. Y. Tseng, T. C. Hsu, Y. F. Wu, J. J. Chen, Y. C. Tseng, Efficient vehicle counting based on time-spatial images by neural networks, in 2021 IEEE 18th International Conference on Mobile Ad Hoc and Smart Systems (MASS), IEEE, (2021), 383–391. https://doi.org/10.1109/MASS52906.2021.00055 |
[7] |
M. Haris, A. Glowacz, Lane line detection based on object feature distillation, Electronics, 10 (2021), 1102. https://doi.org/10.3390/electronics10091102 doi: 10.3390/electronics10091102
![]() |
[8] |
Z. Xie, R. Rajamani, Vehicle counting and maneuver classification with support vector machines using low-density flash lidar, IEEE Trans. Veh. Technol., 71 (2021), 86–97. https://doi.org/10.1109/TVT.2021.3125919 doi: 10.1109/TVT.2021.3125919
![]() |
[9] |
A. Glowacz, Ventilation diagnosis of angle grinder using thermal imaging, Sensors, 21 (2021), 2853. https://doi.org/10.3390/s21082853 doi: 10.3390/s21082853
![]() |
[10] | C. Liu, D. Q. Huynh, Y. Sun, M. Reynolds, S. Atkinson, A vision-based pipeline for vehicle counting, speed estimation, and classification, IEEE Trans. Intell. Transp. Syst., 22 (2020), 7547–7560. https://doi.org/10.1109/TITS.2020.3004066 |
[11] | A. M. Santos, C. J. Bastos-Filho, A. Maciel, Counting vehicle by axes with high-precision in brazilian roads with deep learning methods, in International Conference on Intelligent Systems Design and Applications, Springer, Cham, 418 (2021), 188–198. https://doi.org/10.1007/978-3-030-96308-8_17 |
[12] |
A. Glowacz, Thermographic fault diagnosis of ventilation in BLDC motors, Sensors, 21 (2021), 7245. https://doi.org/10.3390/s21217245 doi: 10.3390/s21217245
![]() |
[13] |
O. E. A. Agudelo, C. E. M. Marín, R. G. Crespo, Sound measurement and automatic vehicle classification and counting applied to road traffic noise characterization, Soft Comput., 25 (2021), 12075–12087. https://doi.org/10.1007/s00500-021-05766-6 doi: 10.1007/s00500-021-05766-6
![]() |
[14] | A. Alsanabani, A. Ahmed, A. M. Al Smadi, Vehicle counting using detecting-tracking combinations: A comparative analysis, in 2020 The 4th International Conference on Video and Image Processing, (2020), 48–54. https://doi.org/10.1145/3447450.3447458 |
[15] |
H. Lin, Z. Yuan, B. He, X. Kuai, X. Li, R. Guo, A deep learning framework for video-based vehicle counting, Front. Phys., 10 (2022), 32. https://doi.org/10.3389/fphy.2022.829734 doi: 10.3389/fphy.2022.829734
![]() |
[16] |
M. Fachrie, A simple vehicle counting system using deep learning with YOLOv3 model, Jurnal RESTI (Rekayasa Sistem Dan Teknologi Informasi), 4 (2020), 462–468. https://doi.org/10.29207/resti.v4i3.1871 doi: 10.29207/resti.v4i3.1871
![]() |
[17] |
K. Yin, L. Wang, J. Zhang, ST-CSNN: a novel method for vehicle counting, Mach. Vision Appl., 32 (2021), 1–13. https://doi.org/10.1007/s00138-021-01233-2 doi: 10.1007/s00138-021-01233-2
![]() |
[18] |
Y. Youssef, M. Elshenawy, Automatic vehicle counting and tracking in aerial video feeds using cascade region-based convolutional neural networks and feature pyramid networks, Trans. Res. Rec., 2675 (2021), 304–317. https://doi.org/10.1177/0361198121997833 doi: 10.1177/0361198121997833
![]() |
[19] | J. Navarro, D. S. Benítez, N. Pérez, D. Riofrío, R. F. Moyano, Towards a low-cost embedded vehicle counting system based on deep-learning for traffic management applications, in 2021 IEEE CHILEAN Conference on Electrical, Electronics Engineering, Information and Communication Technologies (CHILECON), 2021, 1–6. https://doi.org/10.1109/CHILECON54041.2021.9702914 |
[20] | S. Djukanović, Y. Patel, J. Matas, T. Virtanen, Neural network-based acoustic vehicle counting, in 2021 29th European Signal Processing Conference (EUSIPCO), 2021,561–565. https://doi.org/10.23919/EUSIPCO54536.2021.9615925 |
[21] | Z. Al-Ariny, M. A. Abdelwahab, M. Fakhry, E. S. Hasaneen, An efficient vehicle counting method using mask r-cnn, in 2020 International Conference on Innovative Trends in Communication and Computer Engineering (ITCE), 2020,232–237. https://doi.org/10.1109/ITCE48509.2020.9047800 |
[22] |
J. Liu, R. Jia, W. Li, F. Ma, H. M. Abdullah, H. Ma, et al., High precision detection algorithm based on improved RetinaNet for defect recognition of transmission lines, Energy Rep., 6 (2020), 2430–2440. https://doi.org/10.1016/j.egyr.2020.09.002 doi: 10.1016/j.egyr.2020.09.002
![]() |
[23] | M. Ahmad, M. Abdullah, D. Han, Small object detection in aerial imagery using RetinaNet with anchor optimization, in 2020 International Conference on Electronics, Information, and Communication (ICEIC), 2020, 1–3. https://doi.org/10.1109/ICEIC49074.2020.9051269 |
[24] |
G. Dhiman, V. Kumar, Spotted hyena optimizer: A novel bio-inspired based metaheuristic technique for engineering applications, Adv. Eng. Softw., 114 (2017), 48–70. https://doi.org/10.1016/j.advengsoft.2017.05.014 doi: 10.1016/j.advengsoft.2017.05.014
![]() |
[25] |
A. Saha, P. Dash, N. R. Babu, T. Chiranjeevi, M. Dhananjaya, L. Knypiński, Dynamic stability evaluation of an integrated biodiesel-geothermal power plant-based power system with spotted hyena optimized cascade controller, Sustainability, 14 (2022), 14842. https://doi.org/10.3390/su142214842 doi: 10.3390/su142214842
![]() |
[26] |
M. Gafar, R. A. El-Sehiemy, H. M. Hasanien, A. Abaza, Optimal parameter estimation of three solar cell models using modified spotted hyena optimization, J. Ambient Intell. Human. Comput., 2022 (2022), 1–12. https://doi.org/10.1007/s12652-022-03896-9 doi: 10.1007/s12652-022-03896-9
![]() |
[27] |
H. D. Shao, H. K. Jiang, X. Q. Li, S. P. Wu, Intelligent fault diagnosis of rolling bearing using deep wavelet auto-encoder with extreme learning machine, Knowl. Based Syst., 140 (2018), 1–14. https://doi.org/10.1016/j.knosys.2017.10.024 doi: 10.1016/j.knosys.2017.10.024
![]() |
[28] |
I. Abd El Kader, G. Xu, Z. Shuai, S. Saminu, I. Javaid, I. S. Ahmad, et al., Brain tumor detection and classification on MR images by a deep wavelet auto-encoder model, Diagnostics, 11 (2021), 1589. https://doi.org/10.3390/diagnostics11091589 doi: 10.3390/diagnostics11091589
![]() |
[29] |
H. D. Shao, H. K. Jiang, K. Zhao, D. D. Wei, Dongdong, X. Q. Li, A novel tracking deep wavelet auto-encoder method for intelligent fault diagnosis of electric locomotive bearings, Mech. Syst. Signal Process., 110 (2018), 193–209. https://doi.org/10.1016/j.ymssp.2018.03.011 doi: 10.1016/j.ymssp.2018.03.011
![]() |
[30] |
H. Song, H. Liang, H. Li, Z. Dai, X. Yun, Vision-based vehicle detection and counting system using deep learning in highway scenes, Eur. Transp. Res. Rev., 11 (2019), 1–16. https://doi.org/10.1186/s12544-019-0390-4 doi: 10.1186/s12544-019-0390-4
![]() |
1. | Xinna Mao, Hongwei Feng, Maryam A. Al-Towailb, Hassan Saberi-Nik, Dynamical analysis and boundedness for a generalized chaotic Lorenz model, 2023, 8, 2473-6988, 19719, 10.3934/math.20231005 |
a | LE1 | LE2 | LE3 | LE4 | Dynamics |
1.08 | 0.000 | −0.075 | −4.661 | −2.538 | Periodic |
2 | 0.090 | 0.000 | −0.395 | −3.696 | Chaos |
4 | 0.325 | 0.047 | −0.000 | −6.373 | Hyperchaos |