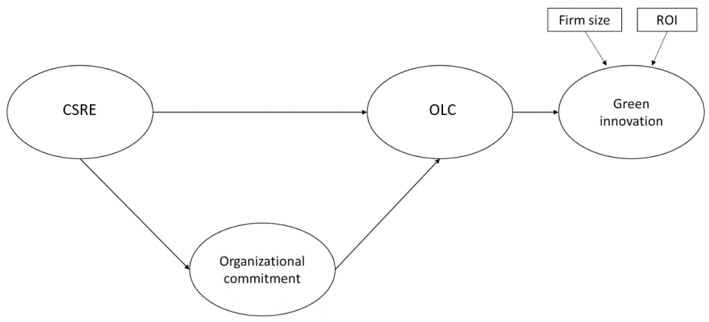
This paper investigates a robust portfolio selection problem with the agent's limited attention. The agent has access to a risk-free asset and a stock in a financial market. But she does not observe perfectly the expected return rate of the stock so she has to estimate this key parameter before making decisions. Besides the general observable financial information, the agent can also acquire a news signal process whose accuracy depends on the agent's attention. We assume that the agent pays limited attention on the signal and she does not trust her estimation model. So it is necessary to consider model ambiguity in this paper as well. The agent maximizes the expected utility of her terminal wealth under the worst-case scenario. Under this setting, we derive the robust optimal strategy explicitly. In the presence of the attention and ambiguity aversion, the myopic term of the strategy, the hedging term of the strategy and the worst-case scenario are all changed. We find that more attention makes the variance of the estimated return smaller. The numerical examples also show that a more attentive agent has a better estimation of the unobservable parameter and is more confident on her estimation. Consequently, the worst-case scenario deviates less from the reference model, which implies a higher expected return rate under the worst-case scenario, thus invests more in the stock.
Citation: Yue Ma, Zhongfei Li. Robust portfolio choice with limited attention[J]. Electronic Research Archive, 2023, 31(7): 3666-3687. doi: 10.3934/era.2023186
[1] | Yun Xin, Hao Wang . Positive periodic solution for third-order singular neutral differential equation with time-dependent delay. AIMS Mathematics, 2020, 5(6): 7234-7251. doi: 10.3934/math.2020462 |
[2] | Emad R. Attia . On the upper bounds for the distance between zeros of solutions of a first-order linear neutral differential equation with several delays. AIMS Mathematics, 2024, 9(9): 23564-23583. doi: 10.3934/math.20241145 |
[3] | Axiu Shu, Xiaoliang Li, Bo Du, Tao Wang . Positive periodic stability for a neutral-type host-macroparasite equation. AIMS Mathematics, 2025, 10(3): 7449-7462. doi: 10.3934/math.2025342 |
[4] | Wentao Wang . Positive almost periodic solution for competitive and cooperative Nicholson's blowflies system. AIMS Mathematics, 2024, 9(5): 10638-10658. doi: 10.3934/math.2024519 |
[5] | Yongxiang Zhu, Min Zhu . Well-posedness and order preservation for neutral type stochastic differential equations of infinite delay with jumps. AIMS Mathematics, 2024, 9(5): 11537-11559. doi: 10.3934/math.2024566 |
[6] | Manal Elzain Mohamed Abdalla, Hasanen A. Hammad . Solving functional integrodifferential equations with Liouville-Caputo fractional derivatives by fixed point techniques. AIMS Mathematics, 2025, 10(3): 6168-6194. doi: 10.3934/math.2025281 |
[7] | Xiulin Hu, Lei Wang . Positive solutions to integral boundary value problems for singular delay fractional differential equations. AIMS Mathematics, 2023, 8(11): 25550-25563. doi: 10.3934/math.20231304 |
[8] | Yan Yan . Multiplicity of positive periodic solutions for a discrete impulsive blood cell production model. AIMS Mathematics, 2023, 8(11): 26515-26531. doi: 10.3934/math.20231354 |
[9] | Mohammed Ahmed Alomair, Ali Muhib . On the oscillation of fourth-order canonical differential equation with several delays. AIMS Mathematics, 2024, 9(8): 19997-20013. doi: 10.3934/math.2024975 |
[10] | H. H. G. Hashem, Hessah O. Alrashidi . Qualitative analysis of nonlinear implicit neutral differential equation of fractional order. AIMS Mathematics, 2021, 6(4): 3703-3719. doi: 10.3934/math.2021220 |
This paper investigates a robust portfolio selection problem with the agent's limited attention. The agent has access to a risk-free asset and a stock in a financial market. But she does not observe perfectly the expected return rate of the stock so she has to estimate this key parameter before making decisions. Besides the general observable financial information, the agent can also acquire a news signal process whose accuracy depends on the agent's attention. We assume that the agent pays limited attention on the signal and she does not trust her estimation model. So it is necessary to consider model ambiguity in this paper as well. The agent maximizes the expected utility of her terminal wealth under the worst-case scenario. Under this setting, we derive the robust optimal strategy explicitly. In the presence of the attention and ambiguity aversion, the myopic term of the strategy, the hedging term of the strategy and the worst-case scenario are all changed. We find that more attention makes the variance of the estimated return smaller. The numerical examples also show that a more attentive agent has a better estimation of the unobservable parameter and is more confident on her estimation. Consequently, the worst-case scenario deviates less from the reference model, which implies a higher expected return rate under the worst-case scenario, thus invests more in the stock.
Over the last decades the concept of corporate social responsibility (CSR) has emerged as a strategic tool aimed at benefiting company stakeholders, including consumers, suppliers, employees, suppliers, owners, communities, governments and investors (Aguinis and Glavas, 2012; Turker, 2009). CSR can be understood as a set of firm initiatives that go beyond the notion of profit-making or compliance with the law, entailing the promotion of good causes, instituting good practices and implementing philanthropic programs, all of which highlight a firm's ethical position (Hameed et al., 2016). In recent years, the CSR dimension of employee satisfaction, or CSR toward employees (CSRE), has attracted growing scholarly attention. However, this field of study is still in its infancy, as some authors point out (Shen and Zhang, 2019). CSRE involves treating employees fairly to improve their well-being through practices related to employee health and safety, compensation issues, work-life balance, career opportunities, employee training, work dignity, equal opportunity or diversity policies (Hameed et al., 2016). These CSRE policies or practices can help to improve satisfaction levels, retention and attraction of workers or job performance or to avoid negative consequences such as burnout, organizational employee disaffection or organizational neglect (Farooq et al., 2014; Rupp and Mallory, 2015; Shen and Zhang, 2019).
Although in recent years significant advances have been made in discovering the effects of CSR for organizations, this analysis has generally focused on macroeconomic and financial issues, leaving aside other individual processes and organizational issues (Rupp and Mallory, 2015). While a direct link has been established between CSR and both organizational innovation (e.g., Bocquet et al., 2019; Ratajczak and Szutowski, 2016; Wu et al., 2018) and green innovation (e.g., Kraus et al., 2020; Shahzad et al., 2020), CSRE is associated with specific attitudes and behaviors that may help to explain this linkage through unobserved mechanisms. Still, the impact that some CSR strategies such as CSRE can have on organizational behavior and employee attitudes is largely unknown (Bouraoui et al., 2019; Shen and Zhang, 2019). Likewise, previous studies of the tourism industry have specifically analyzed CSR issues related to the environmental dimension (Rhou and Singal, 2020), excluding other CSR dimensions such as CSR towards employees (CSRE). The CSRE dimension includes those HR policies or practices aimed at improving the quality of life at work and employee well-being (Santana and López-Cabrales, 2019).
Drawing on stewardship theory (Davis et al., 1997), we contend that caring for employees might have beneficial effects on important organizational aspects. The stewardship theory of management recognizes the needs for balance between one's own goals and those of the larger entity (Hernandez, 2008). This theory has a moral basis and centers on a feeling of obligation, responsibility and accountability toward a bigger entity that is reflected in the willingness to balance one's own self- or group interests with the long-term interests of this bigger entity, even if this requires some personal sacrifice (Avent et al., 2016). Davis et al. (1997) upheld the important relationship between this theory and commitment. Organizational commitment is an affective bond that workers maintain with their firms, making them want to stay with them (Allen and Meyer, 1996). Some research suggests that CSR increases organizational commitment (e.g., Bouraoui et al., 2019; Mueller et al., 2012). In turn, previous literature also indicates that CSRE and organizational commitment would be linked to organizational learning capability (OLC) (Fortis et al., 2018), that is, with the ability of a company to change and adapt (Chiva and Alegre, 2007). At the same time, this ability has been demonstrated to increase organization innovation (Alegre and Chiva, 2008). However, the relationship between OLC and specific dimensions of organizational innovation, such as green innovation (GI), has not been established. GI involves the development of techniques, processes or technologies that make it possible to manufacture products or provide services in a more environmentally friendly way (Chen et al., 2012).
Following these premises, we propose a research model in which the relationship between CSRE and OLC is mediated by organizational commitment, which will eventually lead to higher GI. Finding solutions to encourage green innovation can be particularly relevant in a sector such as tourism since this industry is responsible for a considerable proportion of environmental damage (Dogan and Aslan, 2017; Yue et al., 2021). However, the tourism industry can also be instrumental in reducing climate change and becoming a sustainable sector through the development of green technology innovation (Asadi et al., 2020, Razzaq et al., 2021). In fact, several recent studies (e.g., Higgins-Desbiolles, 2020; Forés et al., 2022; Nepal, 2020; Niewiadomski, 2020) argue that in the new tourism paradigm, businesses must be built on the principles of sustainability, considering the implications of their activity on economic, social and environmental performance. As such, the inclusion of environmental sustainability in the management of tourism companies is nowadays crucial, given the significant harmful effects that tourism activity can have on the environment (Forés et al., 2022; Hall, 2019; Lenzen et al., 2018). This study aims to advance in this field by uncovering the effects and relationships between CSR and environmental sustainability. The discovery of these patterns of relationships would help provide a deeper insight into the consequences of implementing welfare policies in tourism companies and provide novel knowledge with new explanatory models.
The structure of the paper is as follows: The next section presents a theoretical review of the literature. The hypotheses are then developed, followed by an explanation of the methodology used. Next, the results are presented, and finally, we draw a series of conclusions.
Traditionally, strategic human resource (HR) management (HRM) has focused on the search for better organizational results linked to economic performance. This approach has primarily examined the value that these practices offer in improving organizational performance and not so much the value they generate for employees (Lengnick-Hall et al., 2009; Carroll and Shabana, 2010). Recently, however, a new approach to HRM has emerged, which explicitly recognizes the impact that HR policies have on both the economic performance of the firm and on people (Freeman and Hasnaoui, 2011; Aguinis and Glavas, 2012). This approach to HRM is known as corporate social responsibility toward employees (CSRE) (Rodríguez-Sanchez et al., 2021). CSRE consists of respecting human and labor rights through different investment projects to improve employees' quality of life or well-being (Austin, 2008; Wang et al., 2017).
These HR practices have emerged in response to the recent growing interest in the impact of HR practices on ethical, social and human aspects (e.g., Greenwood, 2013; Morgeson et al., 2013). Thus, the term CSRE has been used to refer to HR practices that contribute positively to social or human outcomes (Santana and López-Cabrales, 2019; López-Gamero et al., 2023). These human outcomes include community and family well-being or employee health levels (Shen and Zhang, 2019). The goal of these HR practices is therefore to satisfy the greatest number of workers, reducing, for example, job dissatisfaction, work-related illness or social fracture by improving living and working conditions (Rodríguez-Sanchez et al., 2021).
Specifically, organizations that apply CSR practices treat workers as their main stakeholder group because, among other reasons, human capital is the fundamental pillar of their operations and is responsible for all other resources, be they financial, economic, technical or other (Bao and Yu, 2019). These organizations take care of the working conditions in their company, provide generous maternity and paternity leave, offer flexible working hours, promote occupational health and safety practices and adopt training plans focused on the long term, among other practices (Benn et al., 2011; López-Gamero et al., 2023). These practices aim to achieve greater well-being and health among employees, foster higher quality work relationships and boost positive attitudes and behaviors such as organizational commitment and identification (Rodríguez-Sanchez et al., 2021). Therefore, companies that successfully implement CSRE policies could achieve two things: first, improve internal management by having happier and more motivated employees in their jobs, who are therefore more confident and committed to the company's objectives; and second, promote the company's value creation by improving society's perception of the way it acts and its commitment to its employees.
CSRE includes a set of "best" HR management practices designed to address employees' needs and concerns. In short, CSRE is postulated as an emerging set of HR practices that also positively impact employee health and satisfaction, which would also improve financial and social outcomes (López-Cabrales and Valle-Cabrera, 2020). Therefore, the application of CSRE practices generates a win-win situation in which all parties benefit, both the company and the employees.
Organizational commitment refers to the connection that employees have with the company they are part of, as well as the degree to which these individuals are engaged with and attached to their organizations and willing to continue working in them (Allen and Meyer, 1996; Mercurio, 2015). Organizational commitment occurs when individuals identify with the values and objectives of the organization and strive to achieve them (Porter et al., 1976; Yahaya and Ebrahim, 2016). Identification with organizational values and goals leads to the employee accepting the requirements and principles of the company, making a considerable effort to achieve good performance and wanting to remain in the organization (Modway et al., 1982). Numerous studies reveal the benefits of employee commitment to the organization, as it reduces absenteeism and turnover, encourages organizational citizenship behaviors (behaviors beyond those established on the job, such as helping colleagues or customers) and improves performance (Wright and Bonett, 2002; Mercurio, 2015).
In recent years, many companies have shown interest in the degree of commitment their employees have to the organization. One reason for this interest is the growing recognition that in order to achieve competitive advantages in a global economy, human resources that identify with the objectives, values and culture of the company are essential. In addition, employee commitment is one of the most effective ways of achieving organizational goals, fostering a good working environment, ensuring effective, efficient and continuous communication with the team and promoting teamwork, among others (Allen and Meyer, 1996; Nikpour, 2017). Furthermore, employees' commitment to their organization has been shown to be closely linked to their behavior in the workplace. However, the nature and direction of such relationships are complex and depend on the context and variables under study (Meyer et al., 2002).
Organizational learning capability (OLC) is the tendency of an organization to learn and adapt to change (Chiva and Alegre, 2007). In order to develop this learning capability, it is necessary for the organization to develop in a number of ways: experimentation, risk acceptance, interaction with the environment, dialogue and participatory decision-making (Chiva and Alegre, 2007; Camps et al., 2016). When the organization seeks to test new ideas, make changes in the management of the company and implement new initiatives and alternative solutions, and an interest in change and innovations is awakened, the experimentation dimension is considered to be deployed (Alegre and Chiva, 2013; Odor, 2018). We refer to risk acceptance when there is a working environment that tolerates ambiguity, accepts uncertainty as a non-threatening condition and learns from both mistakes and successes, thus opening up to new business opportunities (Kouzes and Posner, 1987; Le and Lei, 2019). The dimension of interaction with the external environment entails the degree of relations with factors outside the organization's direct control, including the effects of the economy, political/legal system and competitors, among others. Moreover, the exchange of views and ideas brought about by communication and dialogue within companies is another determining factor in OLC (Oswick et al., 2000). Finally, by facilitating the exchange of information, the quality of information and decision outcomes, participatory decision-making is another fundamental condition for OLC (Scott-Ladd and Chan, 2004). Numerous studies on OLC corroborate the effectiveness of this variable in fostering an organization's productivity and performance (e.g., Alegre and Chiva, 2013; Guinot et al., 2013; Tippins and Sohi, 2003). Likewise, authors such as Jiménez-Jimenez et al. (2008) consider organizational learning as a mechanism that helps companies to adapt to turbulent changes in the environment and to be more proactive.
The increasing emergence of environmental problems arising from climate change, the destruction of the biosphere and the loss of biodiversity has led governments, companies and other institutions to devote special attention to tackling this uncertain scenario. Part of the solution to these problems requires the development of techniques, processes and technologies that respect the natural environment (Leal-Rodríguez et al., 2018). This scenario has meant that the agenda of many governments and companies is now dominated by the development of these environmental solutions (Henriksen et al., 2012). In particular, environmentally committed companies are concerned that their product designs should have as little environmental impact as possible. To achieve this commitment, it is essential that the products or services offered by companies use reusable raw materials, save energy and generate as little waste as possible (Chen et al., 2012; Xie et al., 2019). This implies establishing production and service delivery systems that take into account the entire life cycle of the products offered (MacDonald and She, 2015; Liu et al., 2020). To this end, ecological sustainability criteria must be applied from the initial design throughout the entire life cycle of the products or covering the entire phase of service provision (Jabbour et al., 2015; Tang et al., 2018). The development of these products or services, therefore, involves the application of innovative solutions that allow for greener production and consumption. This type of innovation that seeks to respond to environmental sustainability problems is known as green innovation (Albort-Morant et al., 2016). Specifically, green innovation can be defined as the development of innovations that seek to develop environmentally sustainable business models through technologies and processes that save energy and reduce pollution and the use of raw materials. The application of green innovation can help to improve the value of products or services and create a competitive advantage for the company due to the growing interest of consumers in sustainable consumption (Borsatto and Bazani, 2021).
CSRE focuses on organizational actions to improve the working conditions of the organization's members. These actions include an organization's policies and practices aimed at improving the psychological and physiological well-being of its employees, including equal opportunity, work diversity, employee health and safety, work-life balance or employee training (Hameed et al., 2016). Previous research suggests that such engagement practices directed at workers influence their attitudes and behaviors, which impact organizational functioning (Shen and Zhang, 2019). Thus, when the company is willing to support its employees with responsible HR practices, some psychological or behavioral processes may be triggered on an individual basis. These, in turn, could have an impact on the organizational climate. Indeed, as has been shown, HR practices have effects through certain social or psychological processes (Guest 2011; Jiang et al., 2012).
Employee engagement in attitudes and behaviors such as organizational commitment, helping behavior or striving for higher job performance depends to a large extent on their interpretation and evaluation of HRM practices (Guest 2011; Wright and Boswell 2002). This foundation stems from attribution theory (Weiner 1986), according to which people analyze the intentions of others' behaviors and then react by behaving accordingly. Therefore, a person's future behavior will be determined in part by their appraisal of others' behaviors (Nishii et al., 2008). In this way, since employees tend to personalize their companies (Bowen and Ostroff 2004; Nishii et al., 2008), following this attribution theory, company decisions will be interpreted by their employees and will ultimately affect their behaviors and attitudes.
Therefore, a company committed to its employees could trigger a work climate characterized by certain behaviors and attitudes. Specifically, by avoiding layoffs, encouraging job autonomy, providing life insurance, enabling a work-life balance and maintaining equitable remuneration, these CSRE practices could foster positive driving forces among employees. These more positive behaviors and attitudes could include some linked to OLC. More specifically, the company's commitment to its employees may lead them to reciprocate by showing a greater willingness to take risks, experiment, interact, dialogue, and participate. A learning climate could thus be fostered through CSRE practices. In fact, previous research suggests that when organizations engage in the development of CSR actions and policies, they characteristically face several challenges that are related to organizational learning (Fortis et al., 2018). Accordingly, it seems that CSR could help to facilitate organizational learning processes. However, this field of study is still limited, and the role that CSRE can play in OLC in particular is not known. Hence, the following hypothesis is put forward:
Hypothesis 1: CSR toward employees is positively related to OLC.
Previous research argues that CSR contributes to improved employee commitment (e.g., Brammer et al., 2007; Mueller et al., 2012). However, while much of the literature has focused on addressing the relationship between CSR and macroeconomic or financial variables, few research initiatives have attempted to assess its consequences at the more behavioral, individual or work climate levels (Bouraoui et al., 2019). To address this research constraint, in recent years a new approach to the implications of CSR has emerged, known as the micro-CSR approach (Aguinis and Glavas, 2012; Rupp and Mallory, 2015). This new approach aims to explain more implications of CSR, by focusing on testing the effects of CSR on individuals' behavior and attitudes (Bouraoui et al., 2019). We rely on this alternative approach to consider the role that CSR initiatives play in variables such as commitment, which in turn could affect other directly related variables.
Organizational commitment is defined as a psychological state capturing the extent to which an individual is attached to their organization (Meyer and Herscovitch, 2001). More precisely, affective commitment, which is the subject of analysis in this study, is conceived as the emotional identification and personal attachment that the employee feels towards his or her organization (Bouraoui et al., 2019). It considers the employee's desire to remain attached to and identified with his or her organization (Meyer and Allen, 2001). There is a broad consensus in the literature that employees' attitudes and reactions are conditioned by their perceptions of the organization's CSR activities (Aguinis and Glavas, 2013; Bouraoui et al., 2019). In this way, if workers are treated with dignity and fairness, they will react by showing a greater sense of belonging to the company. This can be explained by the perception that their organization supports them, as explained by social exchange theory (Blau, 1964). Social exchange suggests that individuals will respond behaviorally according to how they are treated and valued by their organization (Bouraoui et al., 2019). Thus, when individuals are treated favorably, they are likely to reciprocate this favorable treatment by responding positively (Gouldner, 1960). This positive response could consist not only in them working harder to achieve organizational goals but also showing higher levels of affective organizational commitment (Bouraoui et al., 2019; Farooq et al., 2014).
Moreover, previous research seems to indicate that organizational commitment and organizational learning are interrelated constructs. In particular, these studies suggest that affective organizational commitment is positively related to organizational learning (Bhatnagar, 2007; Milić et al., 2017). However, this body of work has focused on organizational learning as a predictor of affective commitment (e.g., Joo and Park, 2010; Naim and Lenka, 2020; ), while very few studies have examined whether commitment might enhance learning. Moreover, studies examining the relationship between OLC and affective organizational commitment are very scarce, and it is yet to be studied further in different geographical areas (Islam et al., 2015). In any case, the literature suggests that workers who are affectively committed to their organization will show a willingness to share knowledge and experiences with others (Bhatnagar, 2007) and consequently may enhance OLC. Accordingly, we propose that when workers feel emotionally attached to their organization, they will behave with more positive attitudes and responses which might include a willingness to take risks, experiment, interact, dialogue and participate. Hence, we propose that affective organizational commitment may increase OLC. Therefore, since CSRE could positively influence organizational commitment, which in turn could favor OLC, we propose the following mediation hypothesis:
Hypothesis 2: Organizational commitment mediates the relationship between CSR and OLC.
Organizational learning is the process by which organizations learn, considering learning as any change in the organization's knowledge that might also affect other organizational processes or capabilities. The capacity to learn or OLC has been revealed as a key indicator of an organization's potential to innovate (e.g., Alegre and Chiva, 2008). In fact, several studies show the positive relationship between OLC and innovation, innovation performance and radical innovation (Alegre and Chiva, 2008; Fernández-Mesa et al., 2013; Dominguez-Escrig et al., 2016). Accordingly, due to the growing importance of innovation in the current business environment, organizational learning has emerged as an essential issue in organizational settings (Chiva and Alegre, 2009).
OLC has been shown to have a positive effect on employees' well-being in aspects such as job satisfaction (Chiva and Alegre, 2009), which simultaneously is affected by concepts such as altruism within organizations and altruistic leaders (Dominguez-Escrig et al., 2016; Guinot et al., 2016), compassion (Guinot et al., 2020) and trust within organizations (Guinot et al., 2013). Thus, we can assume that OLC implies a certain recognition of the significance of reconciling personal goals with those of a larger entity, as put forward in stewardship theory (Davis et al., 1997; Hensen et al., 2016). Davis et al. (2017) argued that the existence of trust in organizations is strongly linked to the assumptions of this theory. The stewardship theory is built on the principle that "responsible actions bring about long-term benefits for multiple stakeholders and that these must be balanced against self-serving, shorter term economic goals" (Hensen et al., 2016). Therefore, green innovation implies a more responsible approach to innovation, and OLC seems to support stewardship theory. Hence, we might therefore expect OLC to have a positive effect on any type of innovation, including green innovation. We might assume the following:
Hypothesis 3: OLC is positively related to green innovation.
This study is based on a sample of 300 employees of companies in the tourism sector. The companies were located in the Comunidad Valenciana region of Spain and included tourist accommodation companies such as hotels, flat rentals, rural houses and campsites and tour operators. According to data from the Spanish Ministry of Industry, Trade and Tourism, in the first quarter of 2022, 2.4 million people were employed in this sector, which represents 12.1% of total employment in the Spanish economy. This figure reflects an increase of 16.87% on the previous year. Tourism activity in Spain accounted for 5.5% of GDP in 2020, far below pre-Covid pandemic levels of 12.4% of GDP. The Comunidad Valenciana is also among the Spanish regions with the highest number of tourists in Spain. In total, in 2021 this region received more than 4 million tourists and 4,341 million euros in tourism expenditure, according to the Spanish National Institute of Statistics. Even so, these figures fall well below the record highs of 2019, before the pandemic, when over 9.5 million international tourist arrivals were reported, and spending reached 9,620.7 million euros.
Fieldwork was initiated by telephone calls to these companies asking them to complete the survey. Respondents were guaranteed anonymity in order to encourage participation and stimulate more honest responses, thereby reinforcing the reliability of the data. The companies were randomly drawn from the Spanish SABI (Sistema de Análisis de Balances Ibéricos) database, which allows the filtering of companies belonging to the tourism sector in this region of Spain. One employee was surveyed in each company. The final sample was 300 cases. Company sizes ranged from 1 to 607 employees, with a mean value of 22 employees (SD = 22.09) and a mean age of 40 years old (SD = 39. 68). The sample population consisted of more male employees (28.7%) than female employees (71.3%). More than half (56.3%) of the employees had completed higher education, and percentages were very similar for women and men. Employees surveyed were receptionists (46.4%), administration staff (21.2%), managers (19.3%), tourist agents (5.3%), customer service staff (2.4%) and others such as accounting, technical, commercial or maintenance staff (5.4%).
To measure the study variables, we used scales previously validated in the literature. Respondents indicated their level of agreement with the scale items on a 7-point Likert-type scale. We measured CSR toward employees using Turker's (2009) scale, where 1 is the lowest level of compliance, and 7 the highest. This scale is composed of five items such as "our company policies encourage employees to develop their skills and careers" or "our company implements flexible policies to provide a good work-life balance for its employees." This scale has been adopted in other studies to measure the CSR variable (e.g., Farooq et al., 2014; Holcomb and Smith, 2017) and has shown high reliability. Moreover, the confirmatory factor analysis (CFA) with these five items (α = 0.91) fell within the commonly accepted values (χ2SB = 6.38, d.f. = 4, p = 0.17, CFI = 0.99, Bentler Bonnet Nonnormed Fit Index = 0.98, RMSEA = 0.05).
To measure OLC, we employed the scale developed by Chiva and Alegre (2009), who suggested measuring this variable as a multidimensional construct that brings together propositions from the social perspective, the individual perspective and the learning organization. This scale was composed of five dimensions and a total of 14 items. These dimensions are experimentation, risk-taking, interaction with the external environment, dialogue and participation (Chiva et al., 2007). Examples of such items are "people in this company are supported when they come up with new ideas" and "people in this company are encouraged to communicate with each other." Given the complexity of this scale and in order to increase the model's parsimony, we used aggregated variables to measure the five dimensions of OLC (α = 0.85). The CFA corresponding to this scale shows poor fit indexes (χ2SB = 48.72, d.f. = 5, p = 0.00, CFI = 0.88, BBNNFI = 0.76, RMSEA = 0.16); however, previous studies have used this measurement scale, confirming its appropriateness and reliability (e.g., Alegre and Chiva, 2008, 2013; Camps et al., 2016; Fernandez-Mesa and Alegre, 2015; Guinot et al., 2013). Moreover, the scale has proven to be appropriate for measuring perceptions about OLC based on a single informant from each company (Camps et al., 2016; Guinot et al., 2013).
The organizational commitment variable was measured using the scale originally proposed by Meyer et al. (1993) and later adapted by Bulut and Culha (2010). The scale includes a total of six items such as "I would be very happy to spend the rest of my career in this organization, " "I feel like 'part of the family' at this organization" or "I feel a strong sense of belonging to this organization." These items, therefore, measure the level of affective organizational commitment, that is, the degree to which the employee identifies with and is involved with their organization (Bulut and Culha, 2010). This scale has been extensively used in previous studies to measure the variable organizational commitment, showing a good level of reliability (e.g., Erdheim et al., 2006; Kim et al., 2015). We also conducted a CFA with the six scale items (α = 0.91), which showed acceptable fit indexes (χ2SB = 16.89, d.f. = 9, p = 0.05, CFI = 0.99, BBNNFI = 0.98, RMSEA = 0.05) with factor loadings ranging from 0.71 to 0.92.
Finally, the green innovation variable was measured with the scale designed and validated by Chen et al. (2006). This scale uses eight items to measure the level of development and involvement of products or services associated with green innovation. Examples of these items are "the company chooses the least pollution-producing product materials to carry out product development or design, " "the company chooses product materials that consume the least amount of energy and resources to carry out product development or design, " "the company recycles waste and emissions that can be treated and reused in an effective way" and "the company reduces consumption of water, electricity, coal or oil in an effective way." Numerous studies analyzing the green innovation variable have used this measurement scale, confirming its validity and appropriateness (e.g., Albort-Morant et al., 2016; Chang and Chen, 2013). In line with these previous studies, to measure green innovation, we used only one informant from each company. The CFA corresponding to this scale (α = 0.95) shows acceptable fit indexes (χ2SB = 84.89, d.f. = 20, p = 0.00, CFI = 0.93, BBNNFI = 0.90, RMSEA = 0.1).
Moreover, we included as control variables some external variables that could explain part of the results. Thus, variables such as firm size and ROI were included to control for external effects that could condition the results of the model. Previous research has frequently suggested that these two control variables affect organizational innovation (e.g., Dominguez-Escrig et al., 2022; Sanz-Valle et al., 2011). We therefore also included them in the research model (Figure 1).
Three indicators were used to confirm the reliability of the scales: Cronbach's alpha coefficient, the Kaiser-Meyer-Olkin (KMO) test and Bartlett's test (see Table 1). Cronbach's alpha coefficient allows us to analyze the reliability of measurement scales. More specifically, it indicates the consistency between items. As can be seen in Table 2, the Cronbach's alpha coefficient value is acceptable for all the variables in the model (CSRE α = 0.907; OLC α = 0.935; green innovation α = 0.954; commitment α = 0.940). Moreover, to demonstrate the complete reliability of the model, the Kaiser-Meyer-Olkin test (KMO) and Bartlett's test of sphericity were performed. The value obtained for the KMO test measures the suitability of the data for factor analysis by comparing the values of the observed correlation coefficients with the partial correlation coefficients. The test returns values between 0 and 1, and an acceptable value is considered when KMO > = 0.75. Therefore, the model demonstrates the adequacy of the variables and of the complete model because the KMO test for the four variables is higher than the minimum threshold. On the other hand, the chi-square of Bartlett's test of sphericity is statistically significant in the four variables (p < 0.05), which indicates that the correlation matrix does not correspond to an identity. Therefore, the items of the variables are correlated, verifying the adequacy of the factorial model used for the analysis.
Cronbach's α | KMO Test | Bartlett's Test | |
CSRE | 0.907 | 0.870 | 0.001 |
OLC | 0.935 | 0.906 | 0.000 |
Green innovation | 0.954 | 0.917 | 0.000 |
Commitment | 0.940 | 0.911 | 0.000 |
CSRE | COMMITMENT | OLC | |
COMMITMENT | Δχ2 = 81.03 (205.73–124.70) Δd.f. = 1 (44–43) p-value = 0.00 |
||
OLC | Δχ2 = 36.78 (307.04–270.26) Δd.f. = 1 (35–34) p-value = 0.00 |
Δχ2 = 74.46 (350.38–275.92) Δd.f. = 1 (44–43) p-value = 0.00 |
|
GREEN INNOVATION | Δχ2 = 54.90 (546.88–491.98) Δd.f. = 1 (65–64) p-value = 0.00 |
Δχ2 = 97.3 (639.85–542.55) Δd.f. = 1 (77–76) p-value = 0.00 |
Δχ2 = 70.00 (699–629) Δd.f. = 1 (65–64) p-value = 0.00 |
Our study used a single informant to assess all variables, which led us to consider possible common method variance. To address this issue, we implemented preventive measures during the study design phase. Following the proposals of Conway and Lance (2010) and Sheel and Vohra (2016), we ensured participant confidentiality when administering the questionnaire to participants. This common practice reduces the risk of common method bias by decreasing the likelihood that respondents will alter their responses to meet the expectations of others. In addition, we felt that self-reports from participants were particularly appropriate for our research. We also conducted a discriminant validity test between variables using the approach proposed by Bagozzi and Phillips (1982). This test allowed us to compare a two-factor correlated model with a nested model in which the correlation was fixed at one, equivalent to a single-factor model. Table 2 summarizes the results of this analysis. The chi-square difference values (Δχ2) for the six pairs of variables were found to be statistically significant at the 5% level, indicating adequate discriminant validity between variables.
Finally, we also conducted Harman's single factor test (Podsakoff et al., 2003; Podsakoff and Organ, 1986) to assess the presence of common method bias. According to this test, if common method variance were present, a single factor would manifest itself in a confirmatory factor analysis (CFA) that included all items measured by the same informant. Therefore, we conducted a CFA that incorporated all items from the different constructs assessed in our study, with the goal of determining whether most of the variance could be explained by a single factor (Podsakoff et al., 2003: 890). The results of this CFA (χ2SB = 1253.18, d.f. = 252, p = 0.00, BBNNFI = 0.72, CFI = 0.74, RMSEA = 0.12) suggest that there is no common method bias in our research, as these indices are below acceptable values.
The descriptive statistics of the indicators considered in the study (means and standard deviations) and the correlation factors are presented in Table 3.
Mean | S.D. | Green innovation | OLC | CSRE | Commitment | |
Green innovation | 5.866 | 0.905 | 1 | 0.479** | 0.572** | 0.517** |
OLC | 5.775 | 0.839 | 0.479** | 1 | 0.708** | 0.630** |
CSRE | 5.870 | 0.841 | 0.572** | 0.708** | 1 | 0.582** |
Commitment | 6.015 | 0.771 | 0.517** | 0.630** | 0.582** | 1 |
Notes: *Significant correlation (p < 0.10) (1) For the standard deviations and correlations between factors, we worked with the mean of the items that make up each variable. |
We used structural equation modeling (SEM) to empirically validate the proposed research model. SEM allows us to simultaneously estimate all the proposed relationships in a single model. We calculated composite measures of the variables in order to reduce the complexity of these models. Composite measures in SEM provide several advantages, such as increasing the stability of the parameter estimates or reducing the impact of sampling error on the estimation process (Bandalos and Finney, 2001). A composite measure for each variable (CSRE, commitment, OLC, green innovation) was calculated as the average value of the items corresponding to each variable (Landis et al., 2000). Thus, the hypothesized relationships were tested by examining a set of path analyses. Regarding hypothesis 1, we estimated a path analysis including the direct effect of CSRE on OLC. To evaluate hypothesis 2, we examined the mediating relationship by estimating a fully mediated baseline model. In this model, two pathways were established: 1) from CSRE to commitment and 2) from commitment to OLC. No indirect pathways were specified in this model (James et al., 2006). Subsequently, we compared this baseline model with a nested model that included the direct pathway from CSRE to OLC. To make the comparison, we considered both the fit indices and the significance of the routes in both models (Binyamin and Carmeli, 2010). In addition, following the suggestion of James et al. (2006), we examined the significance of the indirect effect of social and environmental responsibility (SEER) on green innovation through engagement. Finally, to evaluate hypothesis 3, we incorporated all the proposed relationships into a single comprehensive model that also included the control variables.
Table 4 shows the results of the estimation of the models. Model 1 corresponds to a saturated model without degrees of freedom. A statistically significant coefficient was observed for CSRE to OLC (0.71, p < 0.01), thus supporting hypothesis 1. This is a relationship not previously demonstrated in the literature. In Model 2, statistically significant coefficients were found for CSRE to Commitment (0.58, p < 0.01) and Commitment to CLO (0.63, p < 0.01) paths. However, this model presented a poor fit according to chi-square values and fit indices (χ2SB = 20.11, d.f. = 1, p = 0.00, BBNNFI = 0.63, CFI = 0.88, RMSEA = 0.25). Next, a nested model was tested in which the direct path between CSRE and OLC was added. The results of the fit improvement (LM) test indicate that the inclusion of the relationship between CSRE and OLC would improve the fit of the model, leading us to the nested model. This nested model, which is a saturated model with no degrees of freedom, revealed statistically significant coefficients for the paths of CSRE to Commitment (0.58, p < 0.01), Commitment to OLC (0.33, p < 0.01) and CSRE to OLC (0.52, p < 0.01). In addition, the indirect effect through Commitment was found to be statistically significant, with a value of 0.19 (p < 0.01). These results support Hypothesis 2 by indicating the presence of a partial mediation effect of Commitment on the relationship between CSRE and OLC. Finally, Model 3 incorporated all the relationships proposed in hypothesis 3. This model showed a good fit to the data (χ2SB = 1.58, d.f. = 5, p = 0.82, BBNNFI = 1, CFI = 1, RMSEA = 0). Statistically significant coefficients were found for the trajectories of CSRE to commitment (0.61, p < 0.01), Commitment to OLC (0.37, p < 0.01), CSRE to OLC (0.48, p < 0.01) and OLC to Green Innovation (0.82, p < 0.01), supporting hypothesis 3.
Model 1 | Model 2 (baseline model) | Model 2 (nested model) | Model 3 | |
CSRE → OLC | 0.71*** | |||
CSRE →Commitment | 0.58*** | 0.58*** | 0.61*** | |
Commitment → OLC | 0.63*** | 0.33*** | 0.37*** | |
CSRE → OLC | 0.52*** | 0.48*** | ||
OLC → Green innovation | 0.82*** | |||
Firm size → Green innovation | 0.01 | |||
ROI → Green innovation | 0.03 | |||
Note: Standardized parameter estimates. *p < 0.10. **p < 0.05. ***p < 0.01 |
Previous literature had suggested that CSRE might favor certain more positive behaviors or attitudes such as performance, extra-role behavior or organizational identity. However, its effect on other variables, and particularly on the learning climate in organizations, was unknown until now. This study therefore succeeds in taking a step forward in the CSR literature by discovering the effects of CSRE and OLC. In turn, although previous CSR literature has identified CSR practices as predictors of innovativeness, in this article we advance the study of this link by discovering mechanisms that would expose this relationship. These results indicates that professional training and career development opportunities, work flexibility and work-life balance policies, attention to employee priorities and fair decision-making foster a work climate in which learning is more likely to take place. Therefore, the beneficiaries of these policies are not only the employees but also the companies themselves. In an industry as globalized and competitive as the tourism sector, which requires companies to learn to adapt continuously, these results can be particularly relevant for managers and human resource practitioners in tourism industry.
On the other hand, in a tourism sector with increasingly demanding environmental regulations, with customers who increasingly demand greater environmental commitment and in which environmental policies are a factor in attracting customers, these findings show strategies for converting tourism companies into more sustainable, functional and attractive business models. As demonstrated by the results found, CSRE policies not only have positive repercussions on employee well-being but also contribute to generating more environmentally friendly business models. While previous literature on CSRE had linked these practices to the facilitation of certain positive behaviors or behaviors, it was unknown how these policies could be simultaneously linked to more complex capabilities, such as learning capability or green innovation performance. This research brings to light the benefits of implementing CSRE policies by charting unexplored avenues. We provide empirical evidence about the link between CSRE and green innovation and the routes of relationship, which means taking a step forward in this emerging research field.
All these findings underline the importance of focusing on employee well-being, not only as a matter of social commitment to employees, but also because of the positive results it has for organizational performance. In addition to enhancing a company's reputation, engaging in CSRE strategies can help improve its competitive position. This study demonstrates that CSRE can be a tool to boost a company's ability to respond to environmental challenges in the tourism sector. As shown, companies that implement CSRE actions and policies also have a greater capacity to offer more environmentally friendly products or services through green innovation. On the other hand, in the tourism industry, environmental sustainability is becoming recognized as a rising value for companies and one their customers increasingly value. Accordingly, tourism companies that are concerned with career development, work-life balance, employee training or equal opportunity issues may end up not only improving employee satisfaction and reputation but also their organizational performance.
The company's commitment to these human resources practices can therefore help to raise the company's reputation, customer loyalty, market share and profits. In this way, a win-win scenario is created in which all of the company's stakeholders—customers, employees, shareholders, the community and the environment—benefit. Hence, the implementation of HRM policies linked to employee satisfaction can therefore become a tool for tourism companies to adapt to changing times while providing greater value to society and responding to the challenges of the environmental crisis. In turn, the scientific validation of the value of CSRE practices can encourage managers to adopt a more humanistic and responsible tourism business model. As such, the findings obtained can serve as inspiration for managers of tourism companies wishing to redirect their businesses towards social and environmental commitment. The tourism sector is essential in most economies, accounting for an important part of the GDP of many countries and involving a large number of companies globally. Therefore, the findings found here may have relevance for an international audience. This study has provided empirical evidence of the consequences of CSRE, but it is not without limitations. One limitation of this study is the cross-sectional nature of the sample, which prevents us from drawing definitive conclusions about causality in the observed associations. Long-term longitudinal studies would therefore be needed to confirm causes and consequences. In addition, the people surveyed were the managers or heads of reception or administration. Future studies could usefully examine these variables from a multi-level approach, taking into account not only managers' perceptions of these variables but also those of the workers themselves. A further line for future research would be to explore the effects of CSRE on other organizational variables such as trust, organizational citizenship behavior or burnout, all of which are highly important for the effective functioning of organizations. Moreover, since this study focuses on a very specific sample of companies, it might be interesting to know whether these results apply to all types of tourism organizations or to those belonging to other sectors of activity or different geographical regions. This study has highlighted the value of CSRE strategies, but this research field is still in its infancy, particularly in the area of tourism management. Therefore, and given the promising results achieved, we particularly encourage future research to further advance knowledge on the value of CSRE in the management of organizations.
The tourism sector is a highly human resource intensive sector, hence the importance of knowing how to manage all this human capital correctly. We note that despite the importance of human capital for the performance of tourism companies, levels of precariousness in the sector are high. The results of this study show that it is worth worrying about the human factor at work, as a strategy not only to attract and retain talent, but also to adapt organizationally to the new challenges of the current business environment. The results serve to advance the literature on tourism management, first by exploring the relationships between CSR and other variables related to the work climate and psychological aspects, therefore leaving behind the traditionally economistic view of CSR. Second, the study approaches the analysis of CSR from the perspective of employees, a research approach that has so far been very little addressed in the field of tourism management. We believe that the findings presented here are of interest not only for the academic literature on tourism management but also for tourism managers to discover HRM practices that make their companies more competitive.
The authors declare they have not used Artificial Intelligence (AI) tools in the creation of this article.
This work was supported by the Generalitat Valenciana under Grant GV/2021/088, Universitat Jaume I under Grant UJI-A2022-07 and Ministerio de Ciencia e Innovación de España under Grant PID2020-116299GB-I00.
All authors declare no conflicts of interest in this paper.
[1] |
A. Lusardi, O. S. Mitchell, The economic importance of financial literacy: Theory and evidence, J. Econ. lit., 52 (2014), 5–44. https://doi.org/10.1257/jel.52.1.5 doi: 10.1257/jel.52.1.5
![]() |
[2] |
F. Huang, J. Song, N. J. Taylor, The impact of business conditions and commodity market on us stock returns: An asset pricing modelling experiment, Quant. Finance Econ., 6 (2022), 433–458. https://doi.org/10.3934/QFE.2022019 doi: 10.3934/QFE.2022019
![]() |
[3] |
A. Yin, Equity premium prediction: keep it sophisticatedly simple, Quant. Finance Econ., 5 (2021), 264–286. https://doi.org/10.3934/QFE.2021012 doi: 10.3934/QFE.2021012
![]() |
[4] |
Z. Li, J. Zhu, J. He, The effects of digital financial inclusion on innovation and entrepreneurship: A network perspective, Electron. Res. Arch., 30 (2022), 4697–4715. https://doi.org/10.3934/era.2022238 doi: 10.3934/era.2022238
![]() |
[5] |
Y. Liu, Z. Li, M. Xu, The influential factors of financial cycle spillover: evidence from china, Emerging Mark. Finance Trade, 56 (2020), 1336–1350. https://doi.org/10.1080/1540496X.2019.1658076 doi: 10.1080/1540496X.2019.1658076
![]() |
[6] |
Z. Li, C. Yang, Z. Huang, How does the fintech sector react to signals from central bank digital currencies, Finance Res. Lett., 50 (2022), 103308. https://doi.org/10.1016/j.frl.2022.103308 doi: 10.1016/j.frl.2022.103308
![]() |
[7] |
Z. Li, B. Mo, H. Nie, Time and frequency dynamic connectedness between cryptocurrencies and financial assets in China, Int. Rev. Econ. Finance, 86 (2023), 46–57. https://doi.org/10.1016/j.iref.2023.01.015 doi: 10.1016/j.iref.2023.01.015
![]() |
[8] |
Y. Luo, Robustly strategic consumption-portfolio rules with informational frictions, Manage. Sci., 63 (2017), 4158–4174. https://doi.org/10.1287/mnsc.2016.2553 doi: 10.1287/mnsc.2016.2553
![]() |
[9] | X. Lei, Information and inequality, J. Econ. Theory, 184 (2019), 104937. https://doi.org/10.1016/j.jet.2019.08.007 |
[10] |
D. Andrei, M. Hasler, Dynamic attention behavior under return predictability, Manage. Sci., 66 (2020), 2906–2928. https://doi.org/10.1287/mnsc.2019.3328 doi: 10.1287/mnsc.2019.3328
![]() |
[11] |
Y. Zhang, Y. Niu, T. Wu, Stochastic interest rates under rational inattention, North Am. J. Econ. Finance, 54 (2020), 101258. https://doi.org/10.1016/j.najef.2020.101258 doi: 10.1016/j.najef.2020.101258
![]() |
[12] |
K. Kasa, Robustness and information processing, Rev. Econ. Dyn., 9 (2006), 1–33. https://doi.org/10.1016/j.red.2005.05.003 doi: 10.1016/j.red.2005.05.003
![]() |
[13] |
L. Peng, Learning with information capacity constraints, J. Financ. Quant. Anal., 40 (2005), 307–329. https://doi.org/10.1017/S0022109000002325 doi: 10.1017/S0022109000002325
![]() |
[14] | R. Reis, When Should Policymakers Make Announcements, Columbia University, 2010. |
[15] |
Z. Chen, L. Epstein, Ambiguity, risk, and asset returns in continuous time, Econometrica, 70 (2002), 1403–1443. https://doi.org/10.1111/1468-0262.00337 doi: 10.1111/1468-0262.00337
![]() |
[16] |
E. W. Anderson, L. P. Hansen, T. J. Sargent, A quartet of semigroups for model specification, robustness, prices of risk, and model detection, J. Eur. Econ. Assoc., 1 (2003), 68–123. https://doi.org/10.1162/154247603322256774 doi: 10.1162/154247603322256774
![]() |
[17] |
I. Gilboa, D. Schmeidler, Maxmin expected utility with non-unique prior, J. Math. Econ., 18 (1989), 141–153. https://doi.org/10.1016/0304-4068(89)90018-9 doi: 10.1016/0304-4068(89)90018-9
![]() |
[18] |
P. Klibanoff, M. Marinacci, S. Mukerji, A smooth model of decision making under ambiguity, Econometrica, 73 (2005), 1849–1892. https://doi.org/10.1111/j.1468-0262.2005.00640.x doi: 10.1111/j.1468-0262.2005.00640.x
![]() |
[19] |
Z. Li, J. Zhong, Impact of economic policy uncertainty shocks on china's financial conditions, Finance Res. Lett., 35 (2020), 101303. https://doi.org/10.1016/j.frl.2019.101303 doi: 10.1016/j.frl.2019.101303
![]() |
[20] |
Y. Liu, P. Failler, Y. Ding, Enterprise financialization and technological innovation: Mechanism and heterogeneity, PloS One, 17 (2022), e0275461. https://doi.org/10.1371/journal.pone.0275461 doi: 10.1371/journal.pone.0275461
![]() |
[21] |
F. Corradin, M. Billio, R. Casarin, Forecasting economic indicators with robust factor models, Natl. Accounting Rev., 4 (2022), 167–190. https://doi.org/10.3934/NAR.2022010 doi: 10.3934/NAR.2022010
![]() |
[22] |
B. Yi, Z. Li, F. G. Viens, Y. Zeng, Robust optimal control for an insurer with reinsurance and investment under Heston's stochastic volatility model, Insur. Math. Econ., 53 (2013), 601–614. https://doi.org/10.1016/j.insmatheco.2013.08.011 doi: 10.1016/j.insmatheco.2013.08.011
![]() |
[23] |
B. Yi, F. Viens, Z. Li, Y. Zeng, Robust optimal strategies for an insurer with reinsurance and investment under benchmark and mean-variance criteria, Scand. Actuarial J., 2015 (2015), 725–751. https://doi.org/10.1080/03461238.2014.883085 doi: 10.1080/03461238.2014.883085
![]() |
[24] |
L. Mao, Y. Zhang, Robust optimal excess-of-loss reinsurance and investment problem with p-thinning dependent risks under CEV model, Quant. Finance Econ., 5 (2021), 134–162. https://doi.org/10.3934/QFE.2021007 doi: 10.3934/QFE.2021007
![]() |
[25] |
P. Wang, Z. Li, Robust optimal investment strategy for an AAM of DC pension plans with stochastic interest rate and stochastic volatility, Insur. Math. Econ., 80 (2018), 67–83. https://doi.org/10.1016/j.insmatheco.2018.03.003 doi: 10.1016/j.insmatheco.2018.03.003
![]() |
[26] |
Y. Zeng, D. Li, Z. Chen, Z. Yang, Ambiguity aversion and optimal derivative-based pension investment with stochastic income and volatility, J. Econ. Dyn. Control, 88 (2018), 70–103. https://doi.org/10.1016/j.jedc.2018.01.023 doi: 10.1016/j.jedc.2018.01.023
![]() |
[27] |
P. Wang, Z. Li, J. Sun, Robust portfolio choice for a DC pension plan with inflation risk and mean-reverting risk premium under ambiguity, Optimization, 70 (2021), 191–224. https://doi.org/10.1080/02331934.2019.1679812 doi: 10.1080/02331934.2019.1679812
![]() |
[28] |
Q. Lin, Y. Luo, X. Sun, Robust investment strategies with two risky assets, J. Econ. Dyn. Control, 134 (2022), 104275. https://doi.org/10.1016/j.jedc.2021.104275 doi: 10.1016/j.jedc.2021.104275
![]() |
[29] |
N. Branger, L. S. Larsen, C. Munk, Robust portfolio choice with ambiguity and learning about return predictability, J. Banking Finance, 37 (2013), 1397–1411. https://doi.org/10.1016/j.jbankfin.2012.05.009 doi: 10.1016/j.jbankfin.2012.05.009
![]() |
[30] |
P. Wang, Y. Shen, L. Zhang, Y. Kang, Equilibrium investment strategy for a dc pension plan with learning about stock return predictability, Insur. Math. Econ., 100 (2021), 384–407. https://doi.org/10.1016/j.insmatheco.2021.07.001 doi: 10.1016/j.insmatheco.2021.07.001
![]() |
[31] | R. S. Lipster, A. N. Shiryaev, Statistics of Random Processes II, Springer Science, New York, 2001. |
[32] |
N. Branger, L. S. Larsen, Robust portfolio choice with uncertainty about jump and diffusion risk, J. Banking Finance, 37 (2013), 5036–5047. https://doi.org/10.1016/j.jbankfin.2013.08.023 doi: 10.1016/j.jbankfin.2013.08.023
![]() |
[33] |
P. J. Maenhout, Robust portfolio rules and asset pricing, Rev. Financ. Stud., 17 (2004), 951–983. https://doi.org/10.1093/rfs/hhh003 doi: 10.1093/rfs/hhh003
![]() |
[34] |
P. J. Maenhout, Robust portfolio rules and detection-error probabilities for a mean-reverting risk premium, J. Econ. Theory, 128 (2006), 136–163. https://doi.org/10.1016/j.jet.2005.12.012 doi: 10.1016/j.jet.2005.12.012
![]() |
[35] |
G. C. dos Santos, P. Zambra, J. A. P. Lopez, Hedge accounting: results and opportunities for future studies, Natl. Accounting Rev., 4 (2022), 74–94. https://doi.org/10.3934/NAR.2022005 doi: 10.3934/NAR.2022005
![]() |
[36] |
Y. Liu, P. Failler, Z. Liu, Impact of environmental regulations on energy efficiency: A case study of china air pollution prevention and control action plan, Sustainability, 14 (2022), 3168. https://doi.org/10.3390/su14063168 doi: 10.3390/su14063168
![]() |
[37] |
T. Li, J. Wen, D. Zeng, K. Liu, Has enterprise digital transformation improved the efficiency of enterprise technological innovation? a case study on chinese listed companies, Math. Biosci. Eng., 19 (2022), 12632–12654. https://doi.org/10.3934/mbe.2022590 doi: 10.3934/mbe.2022590
![]() |
[38] | Z. Li, Z. Huang, Y. Su, New media environment, environmental regulation and corporate green technology innovation: Evidence from China, Energy Econ., 106545. https://doi.org/10.1016/j.eneco.2023.106545 |
[39] |
Y. Liu, C. Ma, Z. Huang, Can the digital economy improve green total factor productivity? an empirical study based on chinese urban data, Math. Biosci. Eng., 20 (2023), 6866–6893. https://doi.org/10.3934/mbe.2023296 doi: 10.3934/mbe.2023296
![]() |
[40] |
Y. Liu, J. Liu, L. Zhang, Enterprise financialization and R & D innovation: A case study of listed companies in China, Electron. Res. Arch., 31 (2023), 2447–2471. https://doi.org/10.3934/era.2023124 doi: 10.3934/era.2023124
![]() |
[41] |
Z. Kang, X. Li, Z. Li, Mean-CVaR portfolio selection model with ambiguity in distribution and attitude, J. Ind. Manage. Optim., 16 (2020), 3065. https://doi.org/10.3934/jimo.2019094 doi: 10.3934/jimo.2019094
![]() |
[42] |
X. Feng, The role of esg in acquirers' performance change after M & A deals, Green Finance, 3 (2021), 287–318. https://doi.org/10.3934/GF.2021015 doi: 10.3934/GF.2021015
![]() |
[43] |
H. Tanaka, C. Tanaka, Sustainable investment strategies and a theoretical approach of multi-stakeholder communities, Green Finance, 4 (2022), 329–346. https://doi.org/10.3934/GF.2022016 doi: 10.3934/GF.2022016
![]() |
[44] |
R. Bhattacharyya, Green finance for energy transition, climate action and sustainable development: overview of concepts, applications, implementation and challenges, Green Finance, 4 (2022), 1–35. https://doi.org/10.3934/GF.2022001 doi: 10.3934/GF.2022001
![]() |
[45] |
G. Desalegn, A. Tangl, Forecasting green financial innovation and its implications for financial performance in ethiopian financial institutions: Evidence from arima and ardl model, Natl. Accounting Rev., 4 (2022), 95–111. https://doi.org/10.3934/NAR.2022006 doi: 10.3934/NAR.2022006
![]() |
1. | Mouataz Billah Mesmouli, Adel A. Attiya, A. A. Elmandouha, Ayékotan M. J. Tchalla, Taher S. Hassan, Serena Matucci, Dichotomy Condition and Periodic Solutions for Two Nonlinear Neutral Systems, 2022, 2022, 2314-8888, 1, 10.1155/2022/6319312 |
Cronbach's α | KMO Test | Bartlett's Test | |
CSRE | 0.907 | 0.870 | 0.001 |
OLC | 0.935 | 0.906 | 0.000 |
Green innovation | 0.954 | 0.917 | 0.000 |
Commitment | 0.940 | 0.911 | 0.000 |
CSRE | COMMITMENT | OLC | |
COMMITMENT | Δχ2 = 81.03 (205.73–124.70) Δd.f. = 1 (44–43) p-value = 0.00 |
||
OLC | Δχ2 = 36.78 (307.04–270.26) Δd.f. = 1 (35–34) p-value = 0.00 |
Δχ2 = 74.46 (350.38–275.92) Δd.f. = 1 (44–43) p-value = 0.00 |
|
GREEN INNOVATION | Δχ2 = 54.90 (546.88–491.98) Δd.f. = 1 (65–64) p-value = 0.00 |
Δχ2 = 97.3 (639.85–542.55) Δd.f. = 1 (77–76) p-value = 0.00 |
Δχ2 = 70.00 (699–629) Δd.f. = 1 (65–64) p-value = 0.00 |
Mean | S.D. | Green innovation | OLC | CSRE | Commitment | |
Green innovation | 5.866 | 0.905 | 1 | 0.479** | 0.572** | 0.517** |
OLC | 5.775 | 0.839 | 0.479** | 1 | 0.708** | 0.630** |
CSRE | 5.870 | 0.841 | 0.572** | 0.708** | 1 | 0.582** |
Commitment | 6.015 | 0.771 | 0.517** | 0.630** | 0.582** | 1 |
Notes: *Significant correlation (p < 0.10) (1) For the standard deviations and correlations between factors, we worked with the mean of the items that make up each variable. |
Model 1 | Model 2 (baseline model) | Model 2 (nested model) | Model 3 | |
CSRE → OLC | 0.71*** | |||
CSRE →Commitment | 0.58*** | 0.58*** | 0.61*** | |
Commitment → OLC | 0.63*** | 0.33*** | 0.37*** | |
CSRE → OLC | 0.52*** | 0.48*** | ||
OLC → Green innovation | 0.82*** | |||
Firm size → Green innovation | 0.01 | |||
ROI → Green innovation | 0.03 | |||
Note: Standardized parameter estimates. *p < 0.10. **p < 0.05. ***p < 0.01 |
Cronbach's α | KMO Test | Bartlett's Test | |
CSRE | 0.907 | 0.870 | 0.001 |
OLC | 0.935 | 0.906 | 0.000 |
Green innovation | 0.954 | 0.917 | 0.000 |
Commitment | 0.940 | 0.911 | 0.000 |
CSRE | COMMITMENT | OLC | |
COMMITMENT | Δχ2 = 81.03 (205.73–124.70) Δd.f. = 1 (44–43) p-value = 0.00 |
||
OLC | Δχ2 = 36.78 (307.04–270.26) Δd.f. = 1 (35–34) p-value = 0.00 |
Δχ2 = 74.46 (350.38–275.92) Δd.f. = 1 (44–43) p-value = 0.00 |
|
GREEN INNOVATION | Δχ2 = 54.90 (546.88–491.98) Δd.f. = 1 (65–64) p-value = 0.00 |
Δχ2 = 97.3 (639.85–542.55) Δd.f. = 1 (77–76) p-value = 0.00 |
Δχ2 = 70.00 (699–629) Δd.f. = 1 (65–64) p-value = 0.00 |
Mean | S.D. | Green innovation | OLC | CSRE | Commitment | |
Green innovation | 5.866 | 0.905 | 1 | 0.479** | 0.572** | 0.517** |
OLC | 5.775 | 0.839 | 0.479** | 1 | 0.708** | 0.630** |
CSRE | 5.870 | 0.841 | 0.572** | 0.708** | 1 | 0.582** |
Commitment | 6.015 | 0.771 | 0.517** | 0.630** | 0.582** | 1 |
Notes: *Significant correlation (p < 0.10) (1) For the standard deviations and correlations between factors, we worked with the mean of the items that make up each variable. |
Model 1 | Model 2 (baseline model) | Model 2 (nested model) | Model 3 | |
CSRE → OLC | 0.71*** | |||
CSRE →Commitment | 0.58*** | 0.58*** | 0.61*** | |
Commitment → OLC | 0.63*** | 0.33*** | 0.37*** | |
CSRE → OLC | 0.52*** | 0.48*** | ||
OLC → Green innovation | 0.82*** | |||
Firm size → Green innovation | 0.01 | |||
ROI → Green innovation | 0.03 | |||
Note: Standardized parameter estimates. *p < 0.10. **p < 0.05. ***p < 0.01 |