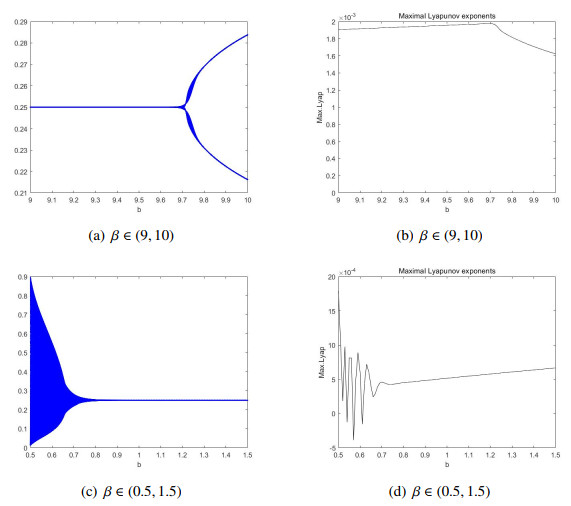
In this paper, we use a semi-discretization method to explore a predator-prey model with Michaelis-Menten functional response. Firstly, we investigate the local stability of fixed points. Then, by using the center manifold theorem and bifurcation theory, we demonstrate that the system experiences a flip bifurcation and a Neimark-Sacker bifurcation at a fixed point when one of the parameters goes through its critical value. To illustrate our results, numerical simulations, which include maximum Lyapunov exponents, fractal dimensions and phase portraits, are also presented.
Citation: Xianyi Li, Xingming Shao. Flip bifurcation and Neimark-Sacker bifurcation in a discrete predator-prey model with Michaelis-Menten functional response[J]. Electronic Research Archive, 2023, 31(1): 37-57. doi: 10.3934/era.2023003
[1] | Zhuo Ba, Xianyi Li . Period-doubling bifurcation and Neimark-Sacker bifurcation of a discrete predator-prey model with Allee effect and cannibalism. Electronic Research Archive, 2023, 31(3): 1405-1438. doi: 10.3934/era.2023072 |
[2] | Jie Xia, Xianyi Li . Bifurcation analysis in a discrete predator–prey model with herd behaviour and group defense. Electronic Research Archive, 2023, 31(8): 4484-4506. doi: 10.3934/era.2023229 |
[3] | Jiange Dong, Xianyi Li . Bifurcation of a discrete predator-prey model with increasing functional response and constant-yield prey harvesting. Electronic Research Archive, 2022, 30(10): 3930-3948. doi: 10.3934/era.2022200 |
[4] | Yujia Xiang, Yuqi Jiao, Xin Wang, Ruizhi Yang . Dynamics of a delayed diffusive predator-prey model with Allee effect and nonlocal competition in prey and hunting cooperation in predator. Electronic Research Archive, 2023, 31(4): 2120-2138. doi: 10.3934/era.2023109 |
[5] | Miao Peng, Rui Lin, Zhengdi Zhang, Lei Huang . The dynamics of a delayed predator-prey model with square root functional response and stage structure. Electronic Research Archive, 2024, 32(5): 3275-3298. doi: 10.3934/era.2024150 |
[6] | Xiaowen Zhang, Wufei Huang, Jiaxin Ma, Ruizhi Yang . Hopf bifurcation analysis in a delayed diffusive predator-prey system with nonlocal competition and schooling behavior. Electronic Research Archive, 2022, 30(7): 2510-2523. doi: 10.3934/era.2022128 |
[7] | Mengting Sui, Yanfei Du . Bifurcations, stability switches and chaos in a diffusive predator-prey model with fear response delay. Electronic Research Archive, 2023, 31(9): 5124-5150. doi: 10.3934/era.2023262 |
[8] | San-Xing Wu, Xin-You Meng . Hopf bifurcation analysis of a multiple delays stage-structure predator-prey model with refuge and cooperation. Electronic Research Archive, 2025, 33(2): 995-1036. doi: 10.3934/era.2025045 |
[9] | Fengrong Zhang, Ruining Chen . Spatiotemporal patterns of a delayed diffusive prey-predator model with prey-taxis. Electronic Research Archive, 2024, 32(7): 4723-4740. doi: 10.3934/era.2024215 |
[10] | Yichao Shao, Hengguo Yu, Chenglei Jin, Jingzhe Fang, Min Zhao . Dynamics analysis of a predator-prey model with Allee effect and harvesting effort. Electronic Research Archive, 2024, 32(10): 5682-5716. doi: 10.3934/era.2024263 |
In this paper, we use a semi-discretization method to explore a predator-prey model with Michaelis-Menten functional response. Firstly, we investigate the local stability of fixed points. Then, by using the center manifold theorem and bifurcation theory, we demonstrate that the system experiences a flip bifurcation and a Neimark-Sacker bifurcation at a fixed point when one of the parameters goes through its critical value. To illustrate our results, numerical simulations, which include maximum Lyapunov exponents, fractal dimensions and phase portraits, are also presented.
In the past several decades, predator-prey interaction has become a hot point of study in biomathematics [1,2,3,4,5,6,7,8,9,10,11,12]. The differential equation is the main tool to be used in modeling predator-prey interaction when the populations have generation overlap or the numbers (densities) of populations are regarded as varying continuously in time. It can help us understand the interactions of different species within a fluctuating natural environment. Generally speaking, the classical predator-prey model may be written as
{dxdt=f(x)x−g(x,y)y,dydt=h(x,y)y−dy, | (1.1) |
where x and y can be expressed as prey and predator population sizes respectively. The function f(x) denotes the growth rate of prey with the absence of predator. g(x,y) represents the amount of prey consumed by per predator per unit time (also called functional response). h(x,y) is on behalf of predator production per capita, and d is the intrinsic death rate of predator [1].
Due to the realistic meaning of f(x), one can assume the prey grows logistically with carrying capacity k and growth rate r in the absence of predator, i.e., f(x)=r(1−xk). Besides a good approximation, many scholars [2,3] reduce the system (1.1) as
{dxdt=rx(1−xk)−g(x,y)y,dydt=ag(x,y)y−dy, | (1.2) |
where a is the conversion effciency.
As for the functional response g(x,y), there are many different types. The dynamical complexity of predator-prey system depends on functional response. Liu and Cheng [4] proposed a system with square-root functional response, while Bian et al. [5] proposed a system with Beddington-DeAngelis funcional response, and so on. In this paper, we discuss the following system
{dxdt=rx(1−xk)−cxymy+x,dydt=y(fxmy+x−d), | (1.3) |
which is called Michaelis-Menten type predator-prey system (or ratio-dependent predator-prey system). The funcional response cxmy+x can be expressed as the function of the ratio of prey to predator, where m is the number of prey necessary to achieve one-half of the maximum rate c. In this system all of the parameters are positive.
Now nondimensionalize the system (1.3). Let xk→x,rt→t,myk→y,cmr→α,fr→β,df→γ. Then, we can derive a simplier form of the system (1.3) as follows
{dxdt=x(1−x)−αxyx+y,dydt=βy(xx+y−γ). | (1.4) |
This continuous system has been discussed in [6,7,8,9,10,11,12].
To be honest, although many methods for continuous systems are mature and have been used to get some interesting results [13,14], it is very difficult to solve a complicated differential equation (system) without using a computer. So, we try to use discretization method to derive and study the discrete model of a complicate differential equation (system) so that we can understand the properties of corresponding continuous systems [15,16,17,18,19,20]. The discrete system related to the system (1.4) has not been investigated yet. In this paper, we select the semi-discretization method, which has better accuracy, to get the discrete version of the system (1.4). To this end, let [t] represent the greatest integer not exceeding t. Now, we explore the average change rate of the system (1.4) at integer number points
{1x(t)dx(t)dt=(1−x([t]))−αy([t])x([t])+y([t]),1y(t)dy(t)dt=β(x([t])x([t])+y([t])−γ). | (1.5) |
We know that the system (1.5) has piecewise constant arguments, and that a solution (x(t),y(t)) of the system (1.5) for t∈[0,+∞) has two characteristics as follows:
1) x(t) and y(t) are continuous on the interval [0,+∞);
2) dx(t)dt and dy(t)dt exist when t∈[0,+∞) except for the points {0,1,2,3,⋯}.
One can derive the following system by integrating the system (1.5) over the interval [n, t] for any t∈[n,n+1) and n=0,1,2,⋯
{x(t)=xne(1−xn)−αynxn+yn(t−n),y(t)=yneβ(xnxn+yn−γ)(t−n), | (1.6) |
where xn=x(n) and yn=y(n). Assuming t→(n+1)− in the system (1.6) produces
{xn+1=xne1−xn−αynxn+yn,yn+1=yneβ(xnxn+yn−γ), | (1.7) |
where α,β,γ>0.
In the sequel, one considers the dynamical properties of the system (1.7). The rest of the paper is organized as follows: in Section 2, one investigates in detail the existence and local stability of the nonnegative fixed points of the system (1.7). Then, the sufficient conditions are formulated for the occurences of flip bifurcation and Neimark-Sacker bifurcation of the system (1.7) in Section 3. Next, numerical simulations are shown to illustrate the results obtained above in Section 4. In the end, some brief conclusions and discussions are stated in Section 5.
Because of the biological meaning of the system (1.7), we discuss the local stability of its nonnegative fixed points in this section. By letting
x=xe1−x−αyx+y,y=yeβ(xx+y−γ), |
it's easy to find that there are two nonnegative fixed points E1=(1,0) and E2=(x0,y0) for max{α−1α,0}<γ<1 where
x0=1−α(1−γ),y0=(1−γ)[1−α(1−γ)]γ. |
The Jacobian matrix of the system (1.7) at a fixed point E(x,y) is
J(E)=([1+x(−1+αy(x+y)2)]e1−x−αyx+y−αx2(x+y)2e1−x−αyx+yβy2(x+y)2eβ(xx+y−γ)(1−βxy(x+y)2)eβ(xx+y−γ)), |
whose charactertistic polynomial reads as
F(λ)=λ2−Tr(J(E))λ+Det(J(E)). |
In order to analyze the prosperties of the fixed points of the system (1.7), one needs the following lemma and definition [21,22].
Definition 2.1. Let E(x,y) be a fixed piont of a 2D discrete system with multipliers λ1 and λ2.
(i) E(x,y) is called sink if |λ1|<1 and |λ2|<1, so, a sink is locally asymptotically stable.
(ii) E(x,y) is called source if |λ1|>1 and |λ2|>1, so, a source is locally asymptotically unstable.
(iii) E(x,y) is called saddle if |λ1|<1 and |λ2|>1 (or |λ1|>1 and |λ2|<1), .
(iv) E(x,y) is called to be non-hyperbolic if either |λ1|=1 or |λ2|=1.
Lemma 2.2. Let F(λ)=λ2+Mλ+N, where M and N are two real constants. Suppose λ1 and λ2 are two roots of F(λ)=0. Then, the following statements hold.
(i) If F(1)>0, then
(i.1) |λ1|<1 and |λ2|<1 if and only if F(−1)>0 and N<1;
(i.2) λ1=−1 and λ2≠−1 if and only if F(−1)=0 and M≠2;
(i.3) |λ1|<1 and |λ2|>1 if and only if F(−1)<0;
(i.4) |λ1|>1 and |λ2|>1 if and only if F(−1)>0 and N>1;
(i.5) λ1 and λ2 are a pair of conjugate complex roots and, |λ1|=|λ2|=1 \setlength\parindent{4em}if and only if −2<M<2 and N=1;
(i.6) λ1=λ2=−1 if and only if F(−1)=0 and M=2.
(ii) If F(1)=0, in other words 1 is one root of F(λ)=0, then, another root λ satisfies |λ|=(<,>)1 if and only if |N|=(<,>)1.
(iii) If F(1)<0, then F(λ)=0 has one root lying in (1,∞). Moreover,
(iii.1) the other root λ satisfies λ<(=)−1 if and only if F(−1)<(=)0;
(iii.2) the other root −1<λ<1 if and only if F(−1)>0.
By using Definition 2.1 and Lemma 2.2, the following conclusions can be obtained.
Theorem 2.3. The fixed point E1=(1,0) of the system (1.7) is a saddle.
The proof for Theorem 2.3 is easy and omitted here. Now consider the fixed point E2. For max{α−1α,0}<γ<1, denote β0=2+2α(1−γ2)γ(1−γ)[1+α(1−γ)] and β1=α(1−γ2)−1αγ(1−γ)2. Obviously, β0>β1.
Theorem 2.4. Assume max{α−1α,0}<γ<1, then, E2=(x0,y0) is a positive fixed point of the system (1.7). Furthermore, the following statements about the fixed point E2 are true.
1) When β<β0,
(a) if 0<α≤1 or α>1 and γ≤√α−1α, then, E2 is a stable node;
(b) if α>1 and γ>√α−1α, then, for 0<β<β1, E2 is an unstable node; for β=β1, E2 is nonhyperbolic; for β1<β<β0, E2 is a stable node.
2) When β=β0, then, E2 is non-hyperbolic.
3) When β>β0, then, E2 is a saddle.
Proof. The Jacobian matrix J(E2) of the system (1.7) at E2 is
J(E2)=(α(1−γ2)−αγ2β(1−γ)21−βγ(1−γ)), |
whose characteristic polynomial can be written as
F(λ)=λ2−pλ+q, | (2.1) |
where
p=Tr(J(E2))=1+α(1−γ2)−βγ(1−γ), |
q=Det(J(E2))=α(1−γ2)−αβγ(1−γ)2. |
Obviously,
F(1)=βγ(1−γ)[1−α(1−γ)]>0,F(−1)=2+2α(1−γ2)−βγ(1−γ)[1+α(1−γ)]=γ(1−γ)[1+α(1−γ)](β0−β), |
F(−1)>(=,<)0⟺β<(=,>)β0andq>(=,<)1⟺β<(=,>)β1. |
So, when β<β0, F(−1)>0. If 0<α≤1 or α>1 and γ≤√α−1α, then β1≤0<β, so q<1, which reads |λ1|<1 and |λ2|<1 by Lemma 2.2(i.1), therefore, E2 is a stable node.
If α>1 and γ>√α−1α, then, for 0<β<β1, q>1, which reads |λ1|>1 and |λ2|>1 by Lemma 2.2(i.4), therefore, E2 is an unstable node; for β=β1, q=1, so, Lemma 2.2(i.5) says E2 is nonhyperbolic; for β1<β<β0, q<1, which indicates |λ1,2|<1 by Lemma 2.2(i.1), and so E2 is a stable node.
When β=β0, F(−1)=0. Namely, −1 is a root of the characteristic polynomial, namely, E2 is non-hyperbolic.
When β>β0, then, F(−1)<0. Lemma 2.2(i.3) says that |λ1|<1 and |λ2|>1, so E2 is a saddle. The proof is over.
In this section, by using the bifurcation theory and center manifold theorem in [23,24,25,26,27], we must pay attention to the flip bifurcation and Neimark-Sacker bifurcation of the system (1.7) at the fixed point E2.
Theorem 2.4 shows that when β=β0 or β=β1, the fixed point E2 is non-hyperbolic. Moreover, the dimensional numbers for the stable manifold and the unstable manifold of the fixed point E2 vary when the parament β goes through these values, which indicates a bifurcation may occur at each case. In the following analyzation, the paraments comply with (α,β,γ)∈SE+={(α,β,γ)∈R3+|α>0,β>0,max{α−1α,0}<γ<1}.
When β=β0, F(−1)=0, which is an indispensable condition for a flip bifurcation to occur. One now explores whether it really exists at the fixed point E2. In fact, the answer is positive.
Theorem 3.1. Suppose the parameters (α,β,γ)∈SE+. If the parameter β varies in a small neighborhood of β0, then, the system (1.7) experiences a flip bifurcation at the fixed point E2.
Proof. Firstly, let un=xn−x0,vn=yn−y0, which transform the fixed point E2 to the origin, and the system (1.7) to
{un+1=(un+x0)e1−(un+x0)−α(vn+y0)un+x0+vn+y0−x0,vn+1=(vn+y0)eβ(un+x0un+x0+vn+y0−γ)−y0. | (3.1) |
Secondly, setting a perturbation β∗ of the parameter β around β0, i.e., β∗=β−β0 with 0<|β∗|≪1. And letting β∗n+1=β∗n=β∗, the system (3.1) is perturbed into
{un+1=(un+x0)e1−(un+x0)−α(vn+y0)un+x0+vn+y0−x0,vn+1=(vn+y0)e(β∗n+β0)(un+x0un+x0+vn+y0−γ)−y0,β∗n+1=β∗n. | (3.2) |
By Taylor expansion, the system (3.2) at (un,vn,β∗n)=(0,0,0) can be expaned into
{un+1=a100un+a010vn+a200u2n+a020v2n+a110unvn+a300u3n+a030v3n+a210u2nvn+a120unv2n+o(ρ31),vn+1=b100un+b010vn+b001β∗n+b200u2n+b020v2n+b002β∗n2+b110unvn+b101unβ∗n+b011vnβ∗n+b300u3n+b030v3n+b003β∗n3+b210u2nvn+b120unv2n+b021v2nβ∗n+b201u2nβ∗n+b102unβ∗n2+b012vnβ∗n2+b111unvnβ∗n+o(ρ31),β∗n+1=β∗n, | (3.3) |
where ρ1=√u2n+v2n+β∗n2,
a100=α(1−γ2),a010=−αγ2,a200=α(1−γ){γ−2γ2+(1+γ)[−1+αγ(1−γ)+α(1−γ)]}2[1−α(1−γ)],a020=αγ3(1+αγ)1−α(1−γ),a110=αγ2(−1+2γ+2α−2αγ)1−α(1−γ),a300=1−α−αγ+2αγ2+4αγ3−2αγ4−2αγ5−α2+α2γ−5α2γ26[1−α(1−γ)]2+7α2γ3+2α2γ4−4α2γ5+α3−3α3γ2+3α3γ4−α3γ66[1−α(1−γ)]2,a210=−αγ2+6αγ3−6αγ4+α2γ−2α2γ2+4α2γ32[1−α(1−γ)]2+−α2γ4−2α2γ5−α3γ+2α3γ3−α3γ52[1−α(1−γ)]2,a120=2αγ3−6αγ4+2α2γ3+α2γ4−6α2γ5+2α3γ4−2α3γ62[1−α(1−γ)]2,a030=−6αγ4+6α2γ5+α3γ66[1−α(1−γ)]2,b102=b001=b002=b003=b102=b012=0,b100=β(1−γ)2,b010=1−βγ(1−γ),b101=(1−γ)22,b011=−γ(1−γ)2,b200=2(1−γ)[1+α(1−γ2)][1−γ+αγ(1−γ)](1−γ)[1+α(1−γ)]2[1−α(1−γ)],b020=−[γ+αγ(1−γ2)][−2+2γ−2α(1−γ)](1−γ)[1+α(1−γ)]2[1−α(1−γ)],b110=[2γ+2αγ(1−γ2)]{−2−2α(1−γ2)+2γ[1+α(1−γ)]2}γ[1+α(1−γ)]3[1−α(1−γ)],b300=2(1−γ)[1+α(1−γ2)]{γ[1+α(1−γ)]+2[1+α(1−γ2)]}[1+α(1−γ)]2[1−α(1−γ)]2,b030=2γ3+2αγ3(1−γ2)(1−γ)[1+α(1−γ)][1−α(1−γ)]2−γ2[2γ+2αγ(1−γ2)]2[−1+2γ−α(1−γ)]6(1−γ)2[1+α(1−γ)]3[1−α(1−γ)]2,b210=[γ+αγ(1−γ2)]{(2−6γ)γ2[1+α(1−γ)]2−[2+2α(1−γ2)]2}xγ2[1+α(1−γ)]3[1−α(1−γ)]2+[γ+αγ(1−γ2)][10−8(1−γ)+2α(1−γ2)+8αγ(1−γ2)]γ[1+α(1−γ)]2[1−α(1−γ)]2,b120=[2γ2+2αγ2(1−γ2)](−2+3γ)(1−γ)[1+α(1−γ)][1−α(1−γ)]2+γ[2+2α(1−γ2)]2(1−3γ+2γ2)(1−γ)2[1+α(1−γ)]3[1−α(1−γ)]2−[γ+αγ(1−γ2)][−2+2γ−2α(1−γ)](1−γ)[1+α(1−γ)]3[1−alpha(1−γ)]2,b201=(1−γ)2{2+2α(1−γ2)−γ[1+α(1−γ)]}[1+α(1−γ)][1−α(1−γ)],b021=γ2{2+2α(1−γ2)+γ[1+α(1−γ)]}[1+α(1−γ)][1−α(1−γ)],b111=(1−γ){−2−2α(1−γ2)+γ2[1+α(1−γ)]}[1+α(1−γ)][1−α(1−γ)]. |
Let
J(E2)=(a100a0100b100b0100001), |
i.e.,
J(E2)=(α(1−γ2)−αγ202(1−γ)[1+α(1−γ2)]γ[1+α(1−γ)]1−2+2α(1−γ2)1+α(1−γ)0001), |
whose three eigenvalues are
λ1=α2(1−γ)(1−γ2)−αγ(1−γ)−1−μ2[1+α(1−γ)]=−1, |
λ2=α2(1−γ)(1−γ2)−αγ(1−γ)−1+μ2[1+α(1−γ)],λ3=1, |
with corresponding eigenvectors
ξ1=(2αγ2[1+α(1−γ)]K+μ0),ξ2=(2αγ2[1+α(1−γ)]K−μ0),ξ3=(001), |
where
K=1+αγ(1−γ)+α2(1−γ)(1−γ2)+2α(1−γ2),μ=α2(1−γ)(1−γ2)+α(2−γ)(1−γ)+1. |
Set T1=(ξ1,ξ2,ξ3), i.e.,
T1=(2αγ2[1+α(1−γ)]2αγ2[1+α(1−γ)]0K+μK−μ0001), |
then,
T−11=(μ−K4αμγ2[1+α(1−γ)]12μ0μ+K4αμγ2[1+α(1−γ)]−12μ0001). |
Let
(un,vn,β∗n)T=T1(Xn,Yn,ωn)T, |
the system (3.2) is changed as follows
{Xn+1=−Xn+F(Xn,Yn,ωn)+o(ρ32),Yn+1=λ2Yn+G(Xn,Yn,ωn)+o(ρ32),ωn+1=ωn, | (3.4) |
where ρ2=√X2n+Y2n+ω2n,
F(Xn,Yn,ωn)=m200X2n+m020Y2n+m002ωn2+m110XnYn+m101Xnωn+m011Ynωn+m300X3n+m030Y3n+m003ωn3+m210X2nYn+m120XnY2n+m201X2nωn+m102Xnωn2+m021Y2nωn+m012Ynωn2+m111XnYnωn,G(Xn,Yn,ωn)=l200X2n+l020Y2n+l002ωn2+l110XnYn+l101Xnωn+l011Ynωn+l300X3n+l030Y3n+l003ωn3+l210X2nYn+l120XnY2n+l201X2nωn+l102Xnωn2+l021Y2nωn+l012Ynωn2+l111XnYnωn, |
m102=m012=m002=m003=0,m200=4(Aa200+b2002μ)α2γ4[1+α(1−γ)]2+2(Aa110+b1102μ)αγ2[1+α(1−γ)](K+μ)+(Aa020+b0202μ)(K+μ)2,m110=8(Aa200+b2002μ)α2γ4[1+α(1−γ2)]+4(Aa110+b1102μ)αγ2[1+α(1−γ)]K+2(Aa020+b0202μ)(K2−μ2),m020=4(Aa200+b2002μ)α2γ4[1+α(1−γ)]2+2(Aa110+b1102μ)αγ2[1+α(1−γ)](K−μ)+(Aa020+b0202μ)(K−μ)2,m101=b0112μ(K+μ)+b101μαγ2[1+α(1−γ)],m011=b0112μ(K−μ)+b101μαγ2[1+α(1−γ)],m300=8(Aa300+b3002μ)α3γ6[1+α(1−γ)]3+(Aa030+b0302μ)(K+μ)3+4(Aa210+b2102μ)α2γ4[1+α(1−γ)]2(K+μ)+2(Aa120+b1202μ))αγ2[1+α(1−γ)](K+μ)2,m030=8(Aa300+b3002μ)α3γ6[1+α(1−γ)]3+(Aa030+b0302μ)(K−μ)3+4(Aa210+b2102μ)α2γ4[1+α(1−γ)]2(K−μ)+2(Aa120+b1202μ))αγ2[1+α(1−γ)](K−μ)2,m210=24(Aa300+b3002μ)α3γ6[1+α(1−γ)]3+3(Aa030+b0302μ)(K−μ)(K+μ)2+4(Aa210+b2102μ)α2γ4[1+α(1−γ)]2(3K+μ)+2(Aa120+b1202μ))αγ2[1+α(1−γ)][(K+μ)2+2(K2−μ2)],m120=24(Aa300+b3002μ)α3γ6[1+α(1−γ)]3+3(Aa030+b0302μ)(K−μ)2(K+μ)+4(Aa210+b2102μ)α2γ4[1+α(1−γ)]2(3K−μ)+2(Aa120+b1202μ))αγ2[1+α(1−γ)][(K−μ)2+2(K2−μ2)],m201=2b201μα2γ4[1+α(1−γ)]2+b0212μ(K+μ)2+b111μαγ2[1+α(1−γ)](K+μ),m021=2b201μα2γ4[1+α(1−γ)]2+b0212μ(K−μ)2+b111μαγ2[1+α(1−γ)](K−μ),m111=2b201μα2γ4[1+α(1−γ)]2+b0212μ(K2−μ2)+2b111μαγ2[1+α(1−γ)]K,l102=l012=l002=l003=0,l200=4(Ba200−b2002μ)α2γ4[1+α(1−γ)]2+2(Ba110−b1102μ)αγ2[1+α(1−γ)](K+μ)+(Ba020−b0202μ)(K+μ)2,l110=8(Ba200−b2002μ)α2γ4[1+α(1−γ)]2+4(Ba110−b1102μ)αγ2[1+α(1−γ)]K+2(Ba020−b0202μ)(K2−μ2),l020=4(Ba200−b2002μ)α2γ4[1+α(1−γ)]2+2(Ba110−b1102μ)αγ2[1+α(1−γ)](K−μ)+(Ba020−b0202μ)(K−μ)2,l101=−b0112μ(K+μ)−b101μαγ2[1+α(1−γ)],l011=−b0112μ(K−μ)−b101μαγ2[1+α(1−γ)],l300=8(Ba300−b3002μ)α3γ6[1+α(1−γ)]3+(Ba030−b0302μ)(K+μ)3+4(Ba210−b2102μ)α2γ4[1+α(1−γ)]2(K+μ)+2(Ba120−b1202μ)αγ2[1+α(1−γ)](K+μ)2,l030=8(Ba300−b3002μ)α3γ6[1+α(1−γ)]3+(Ba030−b0302μ)(K−μ)3+4(Ba210−b2102μ)α2γ4[1+α(1−γ)]2(K−μ)+2(Ba120−b1202μ)αγ2[1+α(1−γ)](K−μ)2,l210=24(Ba300−b3002μ)α3γ6[1+α(1−γ)]3+3(Ba030−b0302μ)(K−μ)(K+μ)2+4(Ba210−b2102μ)α2γ4[1+α(1−γ)]2(3K+μ)+2(Ba120−b1202μ)αγ2[1+α(1−γ)][(K−μ)2+2(K2−μ2)],l120=24(Ba300−b3002μ)α3γ6[1+α(1−γ)]3+3(Ba030−b0302μ)(K−μ)2(K+μ)+4(Ba210−b2102μ)α2γ4[1+α(1−γ)]2(3K−μ)+2(Ba120−b1202μ)αγ2[1+α(1−γ)][(K+μ)2+2(K2−μ2)],l201=−2b201μαγ2[1+α(1−γ)]2−b0212μ(K+μ)2−b111μαγ2[1+α(1−γ)](K+μ),l021=−2b201μα2γ4[1+α(1−γ)]2−b0212μ(K−μ)2−b111μαγ2[1+α(1−γ)](K−μ),l111=−2b201μα2γ4[1+α(1−γ)]2−b0212μ(K2−μ2)−2b111μαγ2[1+α(1−γ)]K, |
where A=μ−K4αμγ2[1+α(1−γ)],B=μ+K4αμγ2[1+α(1−γ)]. Next, suppose on the center manifold
Yn=h(Xn,ωn)=h20X2n+h11Xnωn+h02ω2n+o(ρ23), |
where ρ3=√X2n+ω2n. According to
Yn+1=h(Xn+1,ωn+1)=λ2h(Xn,ωn)+G(Xn,h(Xn,ωn),ωn)+o(ρ33),h(Xn+1,ωn+1)=h20(−Xn+F(Xn,h(Xn,ωn),ωn))2+h11(−Xn+F(Xn,h(Xn,ωn),ωn))ωn+h02ω2n+o(ρ33), |
one has
λ2h(Xn,ωn)+G(Xn,h(Xn,ωn),ωn)=h20(−Xn+F(Xn,h(Xn,ωn),ωn))2+h11(−Xn+F(Xn,h(Xn,ωn),ωn))ωn+h02ω2n+o(ρ33). |
By comparing the corresponding coefficients of terms in the above equation, we get
h20=0,h11=0,h02=0. |
That's to say the system (3.4) which is restricted to the center manifold can be written as
Xn+1=f(Xn,ωn)=−Xn+F(Xn,h(Xn,ωn),ωn)+o(ρ33)=−Xn+l200X2n+l101Xnωn+l300X3n+l201X2nωn+o(ρ33), |
and
f2(Xn,ωn)=f(f(Xn,ωn),ωn)=Xn−2l101Xnωn−(2l300+2l2200)X3n−l200l101X2nωn+l2101Xnω2n+o(ρ33). |
Therefore, we have
f(Xn,ωn)|(0,0)=0,∂f∂Xn|(0,0)=−1,∂f2∂ωn|(0,0)=0,∂2f2∂X2n|(0,0)=0,∂2f2∂Xn∂ωn|(0,0)=−2l101=b011μ(K+μ)+2b101μαγ2[1+α(1−γ)]=−γ(1−γ)[1+α(1−γ2)+α(1−γ)2+α2γ(1−γ)2]μ<0(≠0),−∂3f22∂X3n|(0,0)=l300+l2200>2γ3+2αγ3(1−γ2)(1−γ)[1+α(1−γ)][1−α(1−γ)]2+γ2[2γ+2αγ(1−γ2)]26(1−γ)2[1+α(1−γ)]3[1−α(1−γ)]2+[γ+αγ(1−γ2)][8γ+2α(1−γ2)+8αγ(1−γ2)+2]γ[1+α(1−γ)]2[1−α(1−γ)]2+[γ+αγ(1−γ2)][2+2α(1−γ2)]2+γ2[1+α(1−γ)]2γ2[1+α(1−γ)]3[1−α(1−γ)]2+γ+αγ(1−γ2)[2+2γ−2α(1−γ)](1−γ)[1+α(1−γ)]3[1−α(1−γ)]2+γ[1+2α(1−γ)]2(1+3γ+2γ2)(1−γ)2[1+α(1−γ)]3[1−α(1−γ)]2+[α3γ6+6α2γ5+6αγ4][2+2α2(1−γ)(1−γ2)+α(1−γ)(2−γ)]24α3γ2(1−γ)(1−γ2)[1+α(1−γ)]3[1−α(1−γ)]2+2α2(1−γ)(1−γ2)+α(1−γ)(1−γ2)+23α2γ2(1−γ)(1−γ2)[1+α(1−γ)][1−α(1−γ)]2+1>0(≠0), |
i.e.,
∂3f2∂X3n|(0,0)<0(≠0). |
According to (21.1.42)–(21.1.46) in [24], all of the conditions for the occurrence of a flip bifurcation are satisfied. The proof is over.
When β=β1, a pair of imaginary roots with |λ1|=|λ2|=1 occur, which is the necessary condition for a Neimark-Sacker bifurcation to occur. That is to say, there may be an occurrence of a Neimark-Sacker bifurcation at the fixed point E2. In fact, one has the following results.
Theorem 3.2. Suppose the parameters (α,β,δ)∈SE+ and α>11−γ2. Let β1=α(1−γ2)−1αγ(1−γ)2. Then, the system (1.7) undergoes a Neimark-Sacker bifurcation at the fixed point E2 if the parament β varies in a small neighborhood of the critical value β1. Moreover, if L<(>)0 in (3.9), then an attracting (repelling) invariant closed curve bifurcates from the fixed point E2 for β>(<)β1.
Proof. Firstly, set a perturbation β∗∗ of the parameter β around β1 in the system (3.1), i.e., β∗∗=β−β1 with 0<|β∗∗|≪1, the perturbation of the system (3.1) reads
{un+1=(un+x0)e1−(un+x0)−α(vn+y0)un+x0+vn+y0−x0,vn+1=(vn+y0)e(β∗∗+β1)(un+x0un+x0+vn+y0−γ)−y0. | (3.5) |
The characteristic equation of the linearized eqution of the system (3.5) at the fixed point (0, 0) is
F(λ)=λ2−p(β∗∗)λ+q(β∗∗)=0, | (3.6) |
where
p(β∗∗)=1−αγ(1−γ)+α2(1−γ2)(1−γ)α(1−γ)−β∗∗γ(1−γ), |
q(β∗∗)=1−αβ∗∗γ(1−γ2). |
α>11−γ2 implies p2(0)−4q(0)<0, so, the two roots of F(λ)=0 are
λ1,2(β∗∗)=p(β∗∗)±i√4q(β∗∗)−p2(β∗∗)2. |
The Neimark-Sacker bifurcation needs to satisfy the following two conditions to occur:
1) (d|λ1,2(β∗∗)|dβ∗∗)|β∗∗=0≠0;
2) λi1,2(0)≠1,i=1,2,3,4.
Due to
|λ1,2(β∗∗)|=√q(δ∗∗),(d|λ1,2(β∗∗)|dβ∗∗)|β∗∗=0=−αγ(1−γ)22<0(≠0), |
and obviously λi1,2(0)≠1,i=1,2,3,4, so the two conditions are satisfied.
Secondly, one expands (3.5) into power series up to the third-order term around the origin to get the normal form of the system (3.5) as follows:
{un+1=c10un+c01vn+c20u2n+c11unvn+c02v2n+c30u3n+c21u2nvn+c12unv2n+c03v3n+o(ρ34),vn+1=d10un+d01vn+d20u2n+d11unvn+d02v2n+d30u3n+d21u2nvn+d12unv2n+d03v3n+o(ρ34), | (3.7) |
where ρ4=√u2n+v2n,
c10=a100,c01=a010,c20=a200,c11=a110,c02=a020,c30=a300,c21=a210,c12=a120,c03=a030,d10=α(1−γ2)−1αγ,d01=1−αγ(1−γ)α(1−γ),d20=[α(1−γ2)−1][−1−2αγ(1−γ)+α(1−γ2)]2α2γ(1−γ)[1−α(1−γ)],d02=γ[α(1−γ2)−1][−1−2α(1−γ)+α(1−γ2)+2α(1−γ)2]2α2(1−γ)3[1−α(1−γ)],d11=[α(1−γ2)−1][1+2α(1−γ)−α(1−γ2)−2α(1−γ)2]α2(1−γ)2[1−α(1−γ)],d30=6α3γ2(1−γ)2[α(1−γ2)−1]−6αγ(1−γ)[α(1−γ2)−1]26α3γ(1−γ)2[1−α(1−γ)]2+[α(1−γ2)−1]36α3γ(1−γ)2[1−α(1−γ)]2,d03=[α(1−γ2)−1][6α2γ2(1−γ)3−6α2γ2(1−γ)4]6α3(1−γ)5[1−α(1−γ)]2−[α(1−γ2)−1]2{αγ2(1−γ)+γ2[−1−2α(1−γ)+α(1−γ2)+2α(1−γ)2]}6α3(1−γ)5[1−α(1−γ)]2,d21=[α(1−γ2)−1][6α2γ(1−γ)3−4α2(1−γ)2−4α(1−γ)2]2α3(1−γ)3[1−α(1−γ)]2+[α(1−γ2)−1]2[3α(1−γ)+2αγ2(1−γ)]−[α(1−γ2)−1]32α3(1−γ)5[1−α(1−γ)]2,d12=[α(1−γ2)−1][4α2γ2(1−γ)2−2α2γ(1−γ)2+2α2γ(1−γ)3−4α2γ2(1−γ)3]2α3(1−γ)4[1−α(1−γ)]2+γ2[α(1−γ2)−1]2[−1+2α(1−γ)+α(1−γ2)+2α(1−γ)2]2α3(1−γ)4[1−α(1−γ)]2. |
Then, we can obtain the two roots (eigenvalues) of Eq (3.6) are a pair of conjugate complex as follows:
λ1,2(β∗∗)=1−αγ(1−γ)+α2(1−γ2)(1−γ)∓iθ2α(1−γ), |
where θ=√4α2(1−γ)2−[1−αγ(1−γ)+α2(1−γ2)(1−γ)]2.
Their corresponding eigenvectors are
v1,2=(2α2γ2(1−γ)R)∓i(0θ), |
where R=−1+αγ(1−γ)+α2(1−γ2)(1−γ).
Let
T2=(02α2γ2(1−γ)θR),thenT2−1=(−R2α2γ2θ(1−γ)1θ12α2γ2(1−γ)0). |
Transform the variables
(u,v)T=T2(X,Y)T, |
then, the system (3.7) is changed to the form as follows
{X→2α2γ2(1−γ)−R2α(1−γ)X+θ2α(1−γ)Y+¯F(X,Y)+o(ρ35),Y→−θ2α(1−γ)X+2α2γ2(1−γ)−R2α(1−γ)Y+¯G(X,Y)+o(ρ35), | (3.8) |
where ρ5=√X2+Y2,
¯F(X,Y)=e20X2+e11XY+e02Y2+e30X3+e21X2Y+e12XY2+e03Y3, |
¯G(X,Y)=f20X2+f11XY+f02Y2+f30X3+f21X2Y+f12XY2+f03Y3, |
e20=(Cc02+d02θ)θ2,e30=(Cc03+d03θ)θ3,e02=(Cc02+d02θ)R2+4(Cc20+d20θ)α4γ4(1−γ)2+2(Cc11+d11θ)Rα2γ2(1−γ),e11=2(Cc02+d02θ)Rθ+2(Cc11+d11θ)Rα2γ2(1−γ),e03=(Cc03+d03θ)R3+8(Cc30+d30θ)α6γ6(1−γ)3+2(Cc12+d12θ)R2α2γ2(1−γ)+4(Cc21+d21θ)Rα4γ4(1−γ)2,e21=3(Cc03+d03θ)Rθ2+2(Cc12+d12θ)R2α2γ2(1−γ),e12=3(Cc03+d03θ)R2θ+4(Cc12+d12θ)Rθα2γ2(1−γ)+4(Cc21+d21θ)Rα4γ4(1−γ)2,f20=Dc02θ2,f30=Dc03θ3,f02=D{4α4γ4(1−γ)2c20+R2c02−2Rα4γ4(1−γ)c11},f11=D{2Rθc02+2θα2γ2(1−γ)c11},f03=D{8α6γ6(1−γ)3c30+R3c03}+D{2R2α2γ2(1−γ)c12+4α4γ4(1−γ)2c21},f21=D{3DR2θc03+2θ2α2γ2(1−γ)c12},f12=D{4θα4γ4(1−γ)2c21+4Rθα2γ2(1−γ)c12+3R2θc03}, |
where C=−R2θα2γ2(1−γ), D=12α2γ2(1−γ).
One can easily calculate that
¯FXX=2(Cc02+d02θ)θ2,¯FXXX=6(Cc03+d03θ)θ3,¯FXY=2(Cc02+d02θ)Rθ+2(Cc11+d11θ)Rα2γ2(1−γ),¯FYY=2(Cc02+d02θ)R2+8(Cc20+d20θ)α4γ4(1−γ)2+4(Cc11+d11θ)Rα2γ2(1−γ),¯FXXY=6(Cc03+d03θ)Rθ2+4(Cc12+d12θ)R2α2γ2(1−γ),¯FXYY=6(Cc03+d03θ)R2θ+8(Cc12+d12θ)Rθα2γ2(1−γ)+8(Cc21+d21θ)Rα4γ4(1−γ)2,¯FYYY=6(Cc03+d03θ)R3+48(Cc30+d30θ)α6γ6(1−γ)3+12(Cc12+d12θ)R2α2γ2(1−γ)+24(Cc21+d21θ)Rα4γ4(1−γ)2,¯GXX=2Dc02θ2,¯GXY=D{2Rθc02+2θα2γ2(1−γ)c11},¯GYY=2D{4α4γ4(1−γ)2c20+R2c02−2Rα4γ4(1−γ)c11},¯GXXX=6Dc03θ3,¯GXXY=2D{3DR2θc03+2θ2α2γ2(1−γ)c12},¯GXYY=2D{4θα4γ4(1−γ)2c21+4Rθα2γ2(1−γ)c12+3R2θc03},¯GYYY=6D{8α6γ6(1−γ)3c30+R3c03}+6D{2R2α2γ2(1−γ)c12+4α4γ4(1−γ)2c21}. |
To determine the local stability of the closed orbit bifurcated from the Neimark-Sacker bifurcation of the system (3.8), the discriminating quantity L should be calculated and not to be zero [25,26,27], where
L=−Re((1−2λ1)λ221−λ1ζ20ζ11)−12|ζ11|2−|ζ02|2+Re(λ2ζ21), | (3.9) |
ζ20=18[¯FXX−¯FYY+2¯GXY+i(¯GXX−¯GYY−2¯FXY)],ζ11=14[¯FXX+¯FYY+i(¯GXX+¯GYY)],ζ02=18[¯FXX−¯FYY−2¯GXY+i(¯GXX−¯GYY+2¯FXY)],ζ21=116[¯FXXX+¯FXYY+¯GXXY+¯GYYY+i(¯GXXX+¯GXYY−¯FXXY−¯FYYY)]. |
By calculation we get
ζ20=18{2(Cc02+d02θ)θ2−2(Cc02+d02θ)R2−8(Cc20+d20θ)α4γ4(1−γ)2+2D{2Rθc02+2θα2γ2(1−γ)c11}}+18i{2Dc02θ2−2D{4(1−γ)2α4γ4c20+R2c02}−24(Cc02+d02θ)Rθ+4(Cc11+d11θ)Rα2γ2(1−γ)},ζ11=14[2(Cc02+d02θ)θ2γ2+2(Cc02+d02θ)R2γ2]+14i[2Dc02θ2+2D{4α4γ4(1−γ)2c20+R2c02}],ζ02=18{2(Cc02+d02θ)θ2−2(Cc02+d02θ)R2−8(Cc20+d20θ)α4γ4(1−γ)2−2D{2Rθc02+2θα2γ2(1−γ)c11}}+18i{2Dc02θ2−2D{4α4γ4(1−γ)2c20+R2c02}+24(Cc02+d02θ)Rθ+4(Cc11+d11θ)Rα2γ2(1−γ)},ζ21=116[6(Cc03+d03θ)θ3+8(Cc21+d21θ)Rα4γ4(1−γ)+6(Cc03+d03θ)R2θ+8(Cc12+d12θ)Rθα2γ2(1−γ)+2D{3DR2θc03+2θ2α2γ2(1−γ)c12}+6D{8α6γ6(1−γ)3c30+R3c03}+6D{2R2α2γ2(1−γ)c12+4α4γ4(1−γ)2c21}]+116i{6Dc03θ3+6DR2θc03−12(Cc12+d12θ)R2α2γ2(1−γ)+2D{4θα4γ4(1−γ)2c21+4Rθα2γ2(1−γ)c12}−6(Cc03+d03θ)Rθ2+4(Cc12+d12θ)R2α2γ2(1−γ)−6(Cc03+d03θ)R3+48(Cc30+d30θα6γ6)(1−γ)3−24(Cc21+d21θ)Rα4γ4(1−γ)2}. |
Based on the above analysis, it is clear that a Neimark-Sacker bifurcation of the system (1.7) occurs at the fixed point E2 and that the stability of the invariant closed curve bifurcated from the fixed point E2 is determined by the value of L. Up to here, the proof for Theorem 3.2 is complete.
In this section, numerical simulations are performed to validate above theoretical analysis, including bifurcation diagrams, phase portraits, maximum Lyapunov exponents and fractal dimensions of system (1.7) at the fixed point E2.
Firstly, vary β in the range (9,10), and fix α=1.5,γ=0.5 with the initial value (x0,y0)=(0.3,0.3). Figure 1(a) shows that the existence of a flip bifurcation at the fixed point E2=(0.25,0.25) when β=β0=9.7, which is in accordance with the result in Theorem 3.1. Figure 1(b) means the spectrum of the maximum Lyapunov exponent. Flip bifurcation may lead to chaos, which makes the system more complex.
Then, let β∈(0.5,1.5). The bifurcation diagram is depicted in Figure 1(c), which illustrates that the fixed point E2 is stable for β>β1=0.67, and unstable when β<β1. Hence, a Neimark-Sacker bifurcation occurs at E2 when β=β1, whose multipliers are λ1,2=47±√95i48 with |λ1,2|=1. The maximum Lyapunov exponents related to Figure 1(c) are disposed of in Figure 1(d), which exhibits the existence of periodic orbits and chaos as the parameter β decreases.
Take the initial values (x0,y0)=(0.3,0.3) in Figure 2. These phase portraits illustrate that the dynamical properties of the fixed point E2 have big changes with the parameter β increasing. They change from unstable to stable. What's more, an invariant closed curve around E2 occurs when β=β1. These phenomenons verify the result of Theorem 3.2.
The occurrence of a Neimark-Sacker bifurcation causes the system to jump from stable window to chaotic states through periodic and quasi-periodic states, and trigger a route to chaos.
This work is concerned with a Michaelis-Menten predator-prey model. Using the semi-discretization method, the system (1.4) is transformed into the system (1.7). Comparing the corresponding continuous system in [9], the discrete model has more rich dynamical behaviors. With the given parametric conditions, one demonstrates the existence and local stability of two nonnegative fixed points E1=(1,0) and E2=(1−α(1−γ),(1−γ)[1−α(1−γ)]γ). Utilizing the center manifold theorem, one determines the existence conditions of the flip bifurcation and Neimark-Sacker bifurcation of the system (1.7) around the fixed point E2. Especially, E2 is asymptotically stable when β>β1=α(1−γ2)−1αγ(1−γ)2 and unstable when β<β1. So, it is clear that the system (1.7) undergoes a Neimark-Sacker bifurcation when the parameter β goes through the critical value β1. This phenomenon indicates that the coexistence of prey and predator when the parameter β=β1. Our results clearly display that the system (1.7) is very sensitive to its parameters: different parameter perturbations will lead to different bifurcations.
This work is partly supported by the National Natural Science Foundation of China (grant: 61473340), the Distinguished Professor Foundation of Qianjiang Scholar in Zhejiang Province (grant: F703108L02), and the Natural Science Foundation of Zhejiang University of Science and Technology (grant: F701108G14).
The authors declare that they have no competing interests.
[1] |
R. Arditi, L. R. Ginzburg, Coupling in predator-prey dynamics: ratio-dependence, J. Theoret. Biol., 139 (1989), 311–326. https://doi.org/10.1016/S0022-5193(89)80211-5 doi: 10.1016/S0022-5193(89)80211-5
![]() |
[2] |
L. B. Slobodkin, The role of minimalism in art and science, Am. Nat., 127 (1986), 257–265. https://doi.org/10.1086/284484 doi: 10.1086/284484
![]() |
[3] |
M. J. Coe, D. H. Cumming, J. Phillipson, Biomass and production of large African herbivores in relation to rainfall and primary production, Oecologia, 22 (1976), 341–354. https://doi.org/10.1007/BF00345312 doi: 10.1007/BF00345312
![]() |
[4] |
H. Liu, H. Cheng, Dynamic analysis of a prey-predator model with state-dependent control strategy and square root response function, Adv. Differ. Equations, 1 (2018). https://doi.org/10.1186/s13662-022-03729-1 doi: 10.1186/s13662-022-03729-1
![]() |
[5] |
F. Bian, W. Zhao, Y. Song, R. Yue, Dynamical analysis of a class of prey-predator model with Beddington-Deangelis functional response, stochastic perturbation, and impulsive toxicant input, Complexity, 3 (2017), 1–18. https://doi.org/10.1155/2017/3742197 doi: 10.1155/2017/3742197
![]() |
[6] |
P. A. Abrams, L. R. Ginzburg, The nature of predation: prey dependent, ratio dependent or neither?, Trends Ecol. Evol., 15 (2000), 337–341. https://doi.org/10.1016/S0169-5347(00)01908-X doi: 10.1016/S0169-5347(00)01908-X
![]() |
[7] |
H. R. Akcakaya, R. Arditi, L. R. Ginzburg, Ratio-dependent predation: an abstraction that works, Ecology, 76 (1995), 995–1004. https://doi.org/10.2307/1939362 doi: 10.2307/1939362
![]() |
[8] |
L. Wang, G. Feng, Stability and Hopf bifurcation for a ratio-dependent predator-prey system with stage structure and time delay, Adv. Differ. Equations, 255 (2015). https://doi.org/10.1186/s13662-015-0548-x. doi: 10.1186/s13662-015-0548-x
![]() |
[9] |
Y. Kuang, E. Beretta, Global qualitative analysis of a ratio-dependent predator-prey system, J. Math. Biol., 36 (1998), 389–406. https://doi.org/10.1007/s002850050105 doi: 10.1007/s002850050105
![]() |
[10] |
R. Shi, L. Chen, The study of a ratio-dependent predator-prey model with stage structure in the prey, Nonlinear Dyn., 58 (2009), 443–451. https://doi.org/10.1007/s11071-009-9491-2 doi: 10.1007/s11071-009-9491-2
![]() |
[11] |
R. Xu, Z. Ma, Stability and Hopf bifurcation in a ratio-dependent predator-prey system with stage structure, Chaos, Solitons Fractals, 38 (2008), 669–684. https://doi.org/10.1016/j.chaos.2007.01.019 doi: 10.1016/j.chaos.2007.01.019
![]() |
[12] |
R. Xu, Q. Gan, Z. Ma, Stability and bifurcation analysis on a ratio-dependent predator-prey model with time delay, J. Comput. Appl. Math., 230 (2009), 187–203. https://doi.org/10.1016/j.cam.2008.11.009 doi: 10.1016/j.cam.2008.11.009
![]() |
[13] |
W. Li, J. Ji, L. Huang, Z. Guo, Global dynamics of a controlled discontinuous diffusive SIR epidemic system, Appl. Math. Lett., 121 (2021). https://doi.org/10.1016/j.aml.2021.107420 doi: 10.1016/j.aml.2021.107420
![]() |
[14] |
W. Li, J. Ji, L. Huang, Dynamic of a controlled discountinous computer worm system, P. Am. Math. Soc., 148 (2020), 4389–4403. https://doi.org/10.1090/proc/15095 doi: 10.1090/proc/15095
![]() |
[15] |
Q. Din, Complexity and chaos control in a discrete-time prey-predator model, Commun. Nonlinear Sci., 49 (2017), 113–134. https://doi.org/10.1016/j.cnsns.2017.01.025 doi: 10.1016/j.cnsns.2017.01.025
![]() |
[16] |
J. Huang, S. Liu, S. Ruan, D. Xiao, Bifurcations in a discrete predator-prey model with nonmonotonic functional response, J. Math. Anal. Appl., 464 (2018), 201–230. https://doi.org/10.1016/j.jmaa.2018.03.074 doi: 10.1016/j.jmaa.2018.03.074
![]() |
[17] |
A. Singh, P. Deolia, Dynamical analysis and chaos control in discrete-time prey-predator model, Commun. Nonlinear Sci., 90 (2020). https://doi.org/10.1016/j.cnsns.2020.105313. doi: 10.1016/j.cnsns.2020.105313
![]() |
[18] |
H. Singh, J. Dhar, H. Bhatti, Discrete-time bifurcation behavior of a prey-predator system with generalized predator, Adv. Differ. Equations, 206 (2015), 1–15. https://doi.org/10.1186/s13662-015-0546-z doi: 10.1186/s13662-015-0546-z
![]() |
[19] |
X. Jiang, C. Chen, X. Zhang, M. Chi, H. Yan, Bifurcation and chaos analysis for a discrete ecological developmental system, Nonlinear Dyn., 104 (2021), 4671–4680. https://doi.org/10.1007/s11071-021-06474-4 doi: 10.1007/s11071-021-06474-4
![]() |
[20] |
X. Jiang, X. Chen, Bifurcation and control for a predator-prey system with two delays, IEEE T. Circuits-Ⅱ., 68 (2021), 376–380. https://doi.org/10.1109/TCSII.2020.2987392 doi: 10.1109/TCSII.2020.2987392
![]() |
[21] |
W. Li, X. Li, Neimark-Sacker bifurcation of a semi-discrete hematopoiesis model, J. Appl. Anal. Comput., 8 (2018), 1679–1693. https://doi.org/10.11948/2018.1679 doi: 10.11948/2018.1679
![]() |
[22] |
C. Wang, X. Li, Stability and Neimark-Sacker bifurcation of a semi-discrete population model, J. Appl. Anal. Comput., 4 (2014), 419–435. https://doi.org/10.11948/2014024 doi: 10.11948/2014024
![]() |
[23] | Y. Kuzenetsov, Elements of Apllied Bifurcation Theory, 3rd edition, Springer-Verlag, New York, 2004. https://doi.org/10.1007/978-1-4757-3978-7nosfx=y |
[24] | C. Robinson, Dynamical Systems: Stability, Symbolic Dynamics and Chaos, 2nd edition, Boca Raton, New York, 1999. https://doi.org/10.1112/S0024609397343616 |
[25] | S. Wiggins, Introduction to Applied Nonlinear Dynamical Systems and Chaos, Springer-Verlag, New York, 2003. https://doi.org/10.1007/b97481 |
[26] | J. Carr, Application of Center Manifold Theory, Springer-Verlag, New York, 1981. https://doi.org/10.1007/978-1-4612-5929-9 |
[27] | J. Guckenheimer, P. Holmes, Nonlinear Oscillations, Dynamical Systems, and Bifurcation of Vector Fields, Springer-Verlag, New York, 1983. https://doi.org/10.1007/978-1-4612-1140-2 |
1. | Binhao Hong, Chunrui Zhang, Neimark–Sacker Bifurcation of a Discrete-Time Predator–Prey Model with Prey Refuge Effect, 2023, 11, 2227-7390, 1399, 10.3390/math11061399 | |
2. | Binhao Hong, Chunrui Zhang, Bifurcations and chaotic behavior of a predator-prey model with discrete time, 2023, 8, 2473-6988, 13390, 10.3934/math.2023678 | |
3. | Danyang Li, Xianyi Li, Transcritical bifurcation and Neimark-Sacker bifurcation of a discrete predator-prey model with herd behaviour and square root functional response, 2024, 30, 1387-3954, 31, 10.1080/13873954.2024.2304798 | |
4. | Luyao Lv, Xianyi Li, Stability and Bifurcation Analysis in a Discrete Predator–Prey System of Leslie Type with Radio-Dependent Simplified Holling Type IV Functional Response, 2024, 12, 2227-7390, 1803, 10.3390/math12121803 | |
5. | Jie Liu, Qinglong Wang, Xuyang Cao, Ting Yu, Bifurcation and optimal harvesting analysis of a discrete-time predator–prey model with fear and prey refuge effects, 2024, 9, 2473-6988, 26283, 10.3934/math.20241281 | |
6. | Jie Xia, Xianyi Li, Bifurcation analysis in a discrete predator–prey model with herd behaviour and group defense, 2023, 31, 2688-1594, 4484, 10.3934/era.2023229 | |
7. | Linxia Hu, Yonghong Shen, Xiumei Jia, Global behavior of a discrete population model, 2024, 9, 2473-6988, 12128, 10.3934/math.2024592 | |
8. | Dongmei Chen, Xianyi Li, Complex bifurcation phenomena seized in a discrete ratio-dependent Holling-Tanner predator-prey system, 2024, 2024, 2731-4235, 10.1186/s13662-024-03855-y | |
9. | 秀叶 王, Bifurcation Analysis of Discrete Predator-Prey Model with Michaelis-Menten Type, 2025, 14, 2324-7991, 360, 10.12677/aam.2025.141036 |