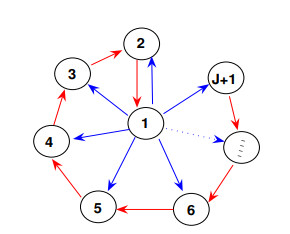
In this paper, we present a hybrid algorithm based on parareal and Schwarz waveform relaxation (SWR) for solving time dependent partial differential equations. The parallelism can be simultaneously realized in the time direction by using a parareal and in the space direction via SWR. We give a convergence analysis for the hybrid algorithm for a 1D model problem, the reaction-diffusion equation. Weak scaling of the algorithm in terms of both the number of space subdomains and the number of paralleled time intervals were investigated via theoretical analysis and numerical experiments.
Citation: Liping Yang, Hu Li. A hybrid algorithm based on parareal and Schwarz waveform relaxation[J]. Electronic Research Archive, 2022, 30(11): 4086-4107. doi: 10.3934/era.2022207
[1] | Chunhua Chu, Yijun Chen, Qun Zhang, Ying Luo . Joint linear array structure and waveform design for MIMO radar under practical constraints. Electronic Research Archive, 2022, 30(9): 3249-3265. doi: 10.3934/era.2022165 |
[2] | Arun Kumar, J Venkatesh, Nishant Gaur, Mohammed H. Alsharif, Peerapong Uthansakul, Monthippa Uthansakul . Cyclostationary and energy detection spectrum sensing beyond 5G waveforms. Electronic Research Archive, 2023, 31(6): 3400-3416. doi: 10.3934/era.2023172 |
[3] | Zhencheng Fan . Zero-stability of waveform relaxation methods for ordinary differential equations. Electronic Research Archive, 2022, 30(3): 1126-1141. doi: 10.3934/era.2022060 |
[4] | Yu Chen, Qingyang Meng, Zhibo Liu, Zhuanzhe Zhao, Yongming Liu, Zhijian Tu, Haoran Zhu . Research on filtering method of rolling bearing vibration signal based on improved Morlet wavelet. Electronic Research Archive, 2024, 32(1): 241-262. doi: 10.3934/era.2024012 |
[5] | Yujia Chang, Yi Jiang, Rongliang Chen . A parallel domain decomposition algorithm for fluid-structure interaction simulations of the left ventricle with patient-specific shape. Electronic Research Archive, 2022, 30(9): 3377-3396. doi: 10.3934/era.2022172 |
[6] | Zhenzhong Xu, Xu Chen, Linchao Yang, Jiangtao Xu, Shenghan Zhou . Multi-modal adaptive feature extraction for early-stage weak fault diagnosis in bearings. Electronic Research Archive, 2024, 32(6): 4074-4095. doi: 10.3934/era.2024183 |
[7] | Jie Wang . A Schwarz lemma of harmonic maps into metric spaces. Electronic Research Archive, 2024, 32(11): 5966-5974. doi: 10.3934/era.2024276 |
[8] | Ruiping Wen, Liang Zhang, Yalei Pei . A hybrid singular value thresholding algorithm with diagonal-modify for low-rank matrix recovery. Electronic Research Archive, 2024, 32(11): 5926-5942. doi: 10.3934/era.2024274 |
[9] | Hongze Yao, Yingting Xu, Weitao WU, Huabin He, Wen Ren, Zhiming Cai . Audio2DiffuGesture: Generating a diverse co-speech gesture based on a diffusion model. Electronic Research Archive, 2024, 32(9): 5392-5408. doi: 10.3934/era.2024250 |
[10] | Cui-Xia Li . A generalization of the AOR iteration method for solving absolute value equations. Electronic Research Archive, 2022, 30(3): 1062-1074. doi: 10.3934/era.2022056 |
In this paper, we present a hybrid algorithm based on parareal and Schwarz waveform relaxation (SWR) for solving time dependent partial differential equations. The parallelism can be simultaneously realized in the time direction by using a parareal and in the space direction via SWR. We give a convergence analysis for the hybrid algorithm for a 1D model problem, the reaction-diffusion equation. Weak scaling of the algorithm in terms of both the number of space subdomains and the number of paralleled time intervals were investigated via theoretical analysis and numerical experiments.
For time dependent partial differential equations (PDEs), Schwarz waveform relaxation (SWR) is a powerful technique for realizing fast computation. It belongs to the widely used domain decomposition (DD) methods [1,2] but with a completely new implementation strategy: the classical strategy employs the alternating or parallel Schwarz method to the elliptic PDEs which result from semi-discretization of the original PDEs in time [3,4,5]; but, in the SWR framework one directly decomposes the space-time domain and solve each subproblem simultaneously or alternately. The main features (or say merits) of the SWR algorithms are able to treat different subproblems numerically differently with an adapted procedure for each subdomain. One can refer to [6,7,8,9,10,11,12,13] for more details about the SWR algorithm.
The aforementioned SWR algorithm realizes parallelism across space. Next, we introduce another efficient parallel algorithm, namely 'parareal', which realizes parallelism across time steps. This method was invented by Lions, Maday and Turinici in [14] as a numerical method to solve evolution problems in parallel. The name was chosen to indicate that the algorithm is well suited for parallel real time computations of evolution problems whose solution cannot be obtained in real time using one processor only. The method approximates successfully the solution later in time before having fully accurate approximations from earlier times. Successful applications of this method include power system simulations [15,16], differential algebraic equations [17], complex chemical reactions [18,19], optimal control problems [20,21,22,23] and Volterra integro-differential equations [24,25,26]. Convergence analysis of the parareal algorithm can be found in [27,28,29,30].
It is natural to combine a parareal with other algorithms to formulate a hybrid algorithm with higher parallelism. For example, in [31,32,33,34,35,36,37,38,39] the classical waveform relaxation (WR) algorithm [40] is embedded into the parareal framework to reduce the computational cost. The authors proposed the "parareal-WR" algorithm and defined it by using the k0-iteration WR algorithm as the fine propagator for the parareal algorithm. The algorithm is analyzed at a continuous level and superlinear and linear convergence were proved on short and long time intervals, respectively. In this paper we combine the SWR technique and the parareal algorithm to construct a hybrid algorithm, namely, Para-SWR, for time dependent PDEs, which can realize parallelism both in space and time directions. Combining a parareal with SWR has already been addressed in recent years; see, e.g., [41,42,43]. In those previous works, the SWR algorithm was used as the so-called fine propagator for the parareal algorithm and this leads to inner-outer iterations. This strategy is different from ours, since in our Para-SWR algorithm we use a single iteration of the parareal as a time integrator for the SWR algorithm. This actually leads to a special discrete SWR algorithm which can be used in parallel in terms of both space and time.
Here, we focus on analyzing the Para-SWR algorithm at the discrete level and deriving suitable conditions for convergence. We also investigate how the convergence rate of the Para-SWR algorithm behaves with respect to the number of subdomains and the number of parallelled time intervals. It is inspiring to report that the proposed Para-SWR algorithm posses the weak scaling property, which implies that increasing the number of subdomains and the number of parallelled time intervals has no (or slight) influence on the convergence rate. An algorithm scales weakly if it can solve a larger problem in reasonable time by increasing the number of processors.
The remainder of this paper is organized as follows. In Section 2, we describe the Para-SWR algorithm investigated in this paper. In Section 3, we analyze the Para-SWR algorithm and present suitable convergence conditions. Weak scaling of the algorithm is also investigated. Section 4 provides some numerical results to validate our theoretical predictions. We finish this paper in Section 5 with some conclusion remarks.
We consider the following 1-D linear reaction diffusion equation as the model problem
{∂tu−ν2∂xxu+au=f(x,t),(x,t)∈(0,L)×R+,u(0,t)=b1(t),t≥0,u(L,t)=b2(t),t≥0,u(x,0)=u0(x),x∈[0,L], | (2.1) |
where a and ν(≠0) are constants. Without loss of generality, we assume a>0 since otherwise we can make a change of variables v=ue−τt with τ+a>0 which leads to (2.1) with a positive reaction coefficient. We first introduce the SWR algorithm at a continuous level to (2.1). We let Δx be a suitable small parameter and L=[N×(m−1)+2]×Δx with some integers N(≥2) and m(≥2). We then decompose the space domain [0,L] into N subdomains
Ωj:=[Lj,Rj],Lj=(j−1)(m−1)Δx,Rj=(j(m−1)+2)Δx,j=1,2,…,N. | (2.2) |
In this situation, the overlap size between every two adjacent subdomains is 2Δx. Then, the N-subdomain SWR algorithm for (2.1) can be written as
x∈Ω1:{∂tuk1−ν2∂xxuk1+auk1=f(x,t),uk1(0,t)=b1(t),(∂x+p)uk1(R1,t)=(∂x+p)uk−12(R1,t),uk1(x,0)=u0(x),x∈Ωj:{∂tukj−ν2∂xxukj+aukj=f(x,t),(∂x−p)ukj(Lj,t)=(∂x−p)uk−1j−1(Lj,t),(∂x+p)ukj(Rj,t)=(∂x+p)uk−1j+1(Rj,t),ukj(x,0)=u0(x),j=2,3,…,N−1,x∈ΩN:{∂tukN−ν2∂xxukN+aukN=f(x,t),(∂x−p)ukN(LN,t)=(∂x−p)uk−1N−1(LN,t),ukN(0,t)=b2(t),ukN(x,0)=u0(x), | (2.3) |
where p is a free parameter. Note that, the transmission condition
(∂x−p)ukj(Lj,t)=(∂x−p)uk−1j−1(Lj,t),(∂x+p)ukj(Rj,t)=(∂x+p)uk−1j+1(Rj,t) |
can be equivalently rewritten as
(∂xp−1)ukj(Lj,t)=(∂xp−1)uk−1j−1(Lj,t),(∂xp+1)ukj(Rj,t)=(∂xp+1)uk−1j+1(Rj,t). |
Hence, by letting p→+∞ we get
ukj(Lj,t)=uk−1j−1(Lj,t),ukj(Rj,t)=uk−1j+1(Rj,t). |
This is the so-called Dirichlet transmission condition and the corresponding algorithm is called classical SWR algorithm (see [9]). We next use the central finite difference method with mesh size Δx to discretize the SWR algorithm (2.3) and this leads to the following semi-discrete SWR algorithm:
{∂tvkj(i,t)−ν2vkj(i−1,t)−2vkj(i,t)+vkj(i+1,t)Δx2+avkj(i,t)=f(xi,t),vkj((j−1)(m−1)+1,t)−vkj((j−1)(m−1),t)Δx−pvkj((j−1)(m−1),t)=vk−1j−1((j−1)(m−1)+1,t)−vk−1j−1((j−1)(m−1),t)Δx−pvk−1j−1((j−1)(m−1),t),vkj(j(m−1)+2,t)−vkj(j(m−1)+1,t)Δx+pvkj(j(m−1)+2,t)=vk−1j+1(j(m−1)+2,t)−vk−1j+1(j(m−1)+1,t)Δx+pvk−1j+1(j(m−1)+2,t),vkj(i,0)=u0(iΔx), | (2.4a) |
where i varies from (j−1)(m−1)+1 to j(m−1)+1 and j=2,3,…,N−1. Similarly, for the left subdomain Ω1 and the right subdomain ΩN
i from 1 to m:{∂tvk1(i,t)−ν2vk1(i−1,t)−2vk1(i,t)+vk1(i+1,t)Δx2+avk1(i,t)=f(xi,t),vk1(0,t)=b0(t),vk1(m+1,t)−vk1(m,t)Δx+pvk1(m+1,t)=vk−12(m+1,t)−vk−12(m,t)Δx+pvk−12(m+1,t),vk1(i,0)=u0(iΔx),i from (N−1)(m−1)+1 to N(m−1)+1:{∂tvkN(i,t)−ν2vkN(i−1,t)−2vkN(i,t)+vkN(i+1,t)Δx2+avkN(i,t)=f(xi,t),vkN((N−1)(m−1)+1,t)−vkN((N−1)(m−1),t)Δx−pvkN((N−1)(m−1),t)=vk−1N−1((N−1)(m−1)+1,t)−vk−1N−1((N−1)(m−1),t)Δx−pvk−1N−1((N−1)(m−1),t),vkN(N(m−1)+2,t)=b1(t),vkN(i,0)=u0(iΔx). | (2.4b) |
Note that, vkj(i,t)=ukj(iΔx,t)+O(Δx2) if iΔx∈(Lj,Rj) and vkj(i,t)=ukj(iΔx,t)+O(Δx) if iΔx=Lj or iΔx=Rj. However, upon convergence it holds that v∞j(i,t)=u∞j(iΔx,t)+O(Δx2)=uj(iΔx,t)+O(Δx2) for iΔx∈[Lj,Rj], that is the discretization of the artificial boundary conditions does not effect the accuracy of the converged solutions [44]. Define
f+(t)=(f(x1,t)+ν2Δx2b0(t)f(x2,t)⋮f(xm,t)),fj(t)=(f(x(j−1)(m−1)+1,t)f(x(j−1)(m−1)+2,t)⋮f(xj(m−1)+1,t)),f−(t)=(f(x(N−1)(m−1)+1,t)⋮f(xN(m−1),t)f(xN(m−1)+1,t)+ν2Δx2b1(t)),q=1Δxp+1,A=ν2Δx2(−2+q11−21⋱⋱⋱1−211−2+q)−aI,Vkj(t)=(vkj((j−1)(m−1)+1,t)vkj((j−1)(m−1)+2,t)⋮vkj(j(m−1)+1,t)),A+=ν2Δx2(−211−21⋱⋱⋱1−211−2+q)−aI,A−=ν2Δx2(−2+q11−21⋱⋱⋱1−211−2)−aI,B+=ν2Δx2(000…0000…0⋮⋮⋮⋮⋮000…0−q10⋯0),B−=ν2Δx2(0⋯01−q0⋯000⋮⋮⋮⋮⋮0⋯0000⋯000). |
Then the semi-discrete SWR algorithm (2.4) can be equivalently rewritten as
∂tVkj(t)=AVkj(t)+B−Vk−1j−1(t)+B+Vk−1j+1(t)+fj(t),j=2,3,…,N−1,∂tVk1(t)=A+Vk1(t)+B+Vk−12(t)+f+(t),∂tVkN(t)=A−VkN(t)+B−Vk−1N−1(t)+f−(t). | (2.5) |
Clearly, this can be written in a more concise form
∂tVk(t)=AVk(t)+BVk−1(t)+˜f(t), | (2.6) |
where
˜f(t)=(f+(t)f2(t)⋮fN−1(t)f−(t)),Vk(t)=(Vk1(t)Vk2(t)⋮VkN−1(t)VkN(t)),A=(A+A⋱AA−),B=(OB+B−OB+⋱⋱⋱B−OB+B−O). | (2.7) |
We next introduce the parareal algorithm. For a general system of ordinary differential equations
{v′(t)=F(t,v(t)),t∈[0,T],v(0)=v0,t=0, | (2.8) |
the parareal algorithm can be described as follows. First, the whole time interval [0,T] is divided into Nt time slices [Tn,Tn+1], n=0,1,…,Nt−1. We suppose that all time slices are of uniform size, i.e., Tn+1−Tn=ΔT=TNt. Second, each large time slice [Tn,Tn+1] is divided into J(≥2) small time slices. Then, two numerical propagators G and F are assigned to the coarse and fine time grids, respectively. We designate by the symbol ⊖ the time-sequential implementation, and by the symbol ⊕ the time parallel implementation. With the aforementioned introduction, the concrete parareal algorithm (for Problem (2.8)) proposed by Lions, Maday and Turinici [14] is given in Algorithm 2.1.
Algorithm 2.1 Parareal Algorithm. |
⊖ Initialization: Perform sequential computation v0n+1=G(Tn,v0n,ΔT) for n=0,1,…,Nt−1; |
For k=0,1,… |
⊕ Step 1 In each subinterval [Tn,Tn+1], compute vn+j+1J=F(Tn+jJ,vn+jJ,ΔTJ) with the initial value vn=vkn, where Tn+jJ=Tn+jΔTJ and j=0,1,…,J−1. |
⊖ Step 2 Perform sequential corrections |
vk+1n+1=G(Tn,vk+1n,ΔT)+vn+1−G(Tn,vkn,ΔT),vk+10=v0,n=0,1,…,Nt−1. |
⊖ Step 3 If {vk+1n}Ntn=1 satisfy the stopping criteria, terminate the iteration; otherwise go to Step 1. |
Clearly, the argument ˜vn+1 can be written as ˜vn+1=FJ(Tn,vkn,Δt) and therefore Algorithm 2.1 can be written compactly as
vk+1n+1=G(Tn,vk+1n,ΔT)+FJ(Tn,vkn,Δt)−G(Tn,vkn,ΔT), | (2.9) |
where Δt=ΔTJ and FJ(Tn,vkn,Δt) denotes a value obtained by running J steps of the fine propagator F with the initial value vkn and the fine step size Δt.
Our Para-SWR algorithm consists of applying the one-iteration parareal algorithm to System (2.6), which is derived from the semi-discrete SWR algorithm. We use the backward Euler method as both the coarse and the fine propagators. On each time interval [Tn,Tn+1], we need to provide a seed value to start up the parallel computation and it is natural to use the Vk−1n obtained in the previous iteration as the seed. Then, the parallel computation in each subinterval [Tn,Tn+1] is
G−propagator:Vkn+1−Vk−1nΔT=AVkn+1+BVk−1n+1+˜f(tn+1),F−propagator:Vkn,jJ−Vkn,j−1JΔt=AVkn,jJ+BVk−1n,jJ+˜f(tn+jΔt),j=1,2,…,J, | (2.10) |
where Vkn,0=Vk−1n and ΔT=JΔt. From (2.10) we get
Vkn+1=(I−ΔTA)−1(Vk−1n+ΔTBVk−1n+1+ΔT˜f(tn+1)),Vkn,jJ=(I−ΔtA)−1(Vkn,j−1J+ΔtBVk−1n,jJ+Δt˜f(tn+jΔt)),j=1,2,…,J. | (2.11) |
With the values Vkn+1 and Vkn,1, the serial correction is
Vkn+1=Vkn+1−G(Tn,Vkn,ΔT)+Vkn,1, | (2.12) |
where the argument G(Tn,Vkn,ΔT) denotes the value generated by the backward Euler method with the starting value Vkn, that is
G(Tn,Vkn,ΔT)=(I−ΔTA)−1(Vkn+ΔTBVk−1n+1+ΔT˜f(tn+1)). | (2.13) |
It then follows by substituting (2.11) and (2.13) into (2.12) that
Vkn+1=(I−ΔTA)−1(Vk−1n−Vkn)+Vkn,1. | (2.14) |
Remark 1. The uniform formula (2.6) of the semi-discrete SWR algorithm leads to (2.14) which will greatly facilitate the theoretical analysis done in the next section. In practical computation, it is convenient to directly apply the parareal algorithm on each subdomain Ωj×[0,T], j=1,2,…,N.
In this section, we focus on investigating the theoretical property of the Para-SWR algorithm. The analysis is divided into two parts, i.e., the convergence of the algorithm and the weak scaling of the algorithm. In the sequel, we assume Δxp≥1 and this implies q≥0.
To analyze the convergence of the Para-SWR iteration (2.14), for simplicity and without loss of generality we set ˜f(t)=0. Let
ˉAc=(I−ΔTA)−1,ˉAf=(I−ΔtA)−1, | (3.1) |
and by using the relation Vkn,0=Vk−1n we know from the second equality of (2.11) that
Vkn,jJ=ˉAjfVk−1n+j∑i=1ΔtˉAifBVk−1n,j−i+1J,j=1,2,…,J−1. | (3.2) |
It then follows by substituting (3.2) into (2.14) that
Vkn+1=ˉAc(Vkn−Vk−1n)+ˉAJfVk−1n+ΔtJ∑j=1ˉAjfBVk−1n,J−j+1J⏟Vkn,1. | (3.3) |
Define
λc=‖(I−ΔTA)−1‖2,λf=‖(I−ΔtA)−1‖2,μ=Δt‖B‖2,γ=‖ˉAJf−ˉAc‖2,D=(λfλ2fλ3f⋮λJf),E=(λfλ2fλfλ3fλ2fλf⋮⋱⋱⋱λJfλJ−1fλJ−2f⋯λf),ϵkn=(‖Vkn,1J‖2‖Vkn,2J‖2⋮‖Vkn,JJ‖2). | (3.4) |
Under the assumptions q≥0 and a>0, it is easy to know that λc<λf<1. From the second equality of (3.2) we get
ϵkn≤D‖Vk−1n‖2+μEϵk−1n. | (3.5) |
From (3.3) we have
‖Vkn+1‖2≤λc‖Vkn‖2+γ‖Vk−1n‖2+μE1ϵk−1n, | (3.6) |
where E1 denotes the last row of the matrix E. Define
Vk=maxn≥1‖Vkn‖2,ϵkj=maxn≥0ϵkn,j,ϵk=(ϵk1,ϵk2,…,ϵkJ)T. | (3.7) |
Then from (3.5) and (3.6) it is easy to get
Vk≤11−λc(γVk−1+μE1ϵk−1),ϵk≤DVk−1+μEϵk−1. | (3.8) |
Define
β=γ1−λc,η=μ1−λc,M=(βηE1DμE)=(βηλJfηλJ−1fηλJ−2f⋯ηλfλfμλfλ2fμλ2fμλfλ3fμλ3fμλ2fμλf⋮⋮⋱⋱⋱λJfμλJfμλJ−1fμλJ−2f⋯μλf). | (3.9) |
Let the vectors {zk} be generated by the following recurrence relation
zk=Mzk−1, | (3.10) |
with the initial vector z0=(V0ϵ0). Since the matrix M is nonnegative, it is clear that (Vkϵk)≤zk in the component sense for all k≥1. Thus, it is sufficient to investigate the convergence of the iteration (3.10). It is well known that the iteration converges to zero if and only if the spectral radius of M is less than one, i.e., ρ(M)<1. To analyze the spectral radius of M, we need the following definitions and lemmas concerning nonnegative matrices.
Definiton 1. [45,page 95] For n×n real matrices P, P1 and P2, the relation P=P1−P2 is called a regular splitting of P if P1 is nonsingular with P−11≥0 and P2≥0.
Definiton 2. [45,pages 53-54] Let P be a n×n real matrix and G(PB) be a directed graph associated with the matrix P, which has n vertices and an edge from the vertex i to vertex j precisely when Pij>0. Then the matrix P is irreducible if and only if its associated graph G(P) is strongly connected, that is for any two vertices i and j of G(P), G(P) contains a path from i to j.
Lemma 1. [45,page 90] Let P=(pij) be a n×n real matrix with pij≤0 for all i≠j; then, the following statements are equivalent:
1) P is nonsingular and P−1≥0;
2) the diagonal entries of P are positive real numbers and letting PD be the diagonal matrix whose diagonal entries dii are defined as
dii:=pii,1≤i≤n, |
then the matrix PB:=I−P−1DP is nonnegative and ρ(PB)<1.
Lemma 2. [45,page 51] Let P∈Rn×n and P=P1−P2 be a regular splitting of P. Then P is nonsingular with P−1≥0 if and only if ρ(P−11P2)<1.
Lemma 3. [45,page 50] Let P∈Rn×n be an irreducible non-negative matrix with the spectral radius ρ(P). Then, the number ρ(P) is a positive real number and an eigenvalue of the matrix P, called the Perron-Frobenius eigenvalue.
Theorem 1. Let q≥0, a>0, μλf<1 and β<1. Then the Para-SWR algorithm based on the backward Euler method is convergent and the convergence rate can be bounded by ρ(M), provided the following condition is satisfied
ηλJf(1−[1−μλf]J)μ(1−β)(1−μλf)J<1, | (3.11) |
where λf and μ are defined by (3.4) and β and η are defined by (3.9).
Proof. It is sufficient to prove that Condition (3.11) implies ρ(M)<1. In fact, this is an "if and only if" condition. Let
D=(010⋱⋱1010)J×J,Q=(1λf1λ2fλf1⋮⋱⋱⋱λJ−1f⋯λ2fλf1)J×J. | (3.12) |
It is easy to check that Q=(I−λfD)−1. Let X1=ηλfET1 and X2=λf(I−λfD)D and we have
M=P−11P2,P1=(1I−λfD),P2=(βXT1X2μλfI). | (3.13) |
Define
P:=P1−P2=(1−β−ηλJf−ηλJ−1f⋯−ηλf−λf1−μλf0−λf1−μλf⋮⋱⋱0−λf1−μλf),PD:=(1−β1−μλf⋱1−μλf). |
Then we have
PB:=I−P−1DP=(0ηλJf1−βηλJ−1f1−β⋯ηλf1−βλf1−μλf0λf1−μλf0⋱⋱λf1−μλf0). | (3.14) |
From Definition 1, we know that P=P1−P2 is a regular splitting of the matrix P. Since μλf<1 and β<1, it is clear that the matrix PB is nonnegative. Hence, we have
ρ(PB)<1⟺ Lem.1 P−1≥0⟺ Lem.2 ρ(P−11P2)=ρ(M)<1. | (3.15) |
Our ultimate goal is to prove that ρ(M)<1, and that due to (3.15) it is sufficient to prove that ρ(PB)<1. Our proof toward ρ(PB)<1 is divided into two parts.
Part 1: irreducibility of the matrix PB. The directed graph G(PB) associated with the matrix PB defined by (3.14) is shown in Figure 1. For the arbitrarily chosen vertices i and j, the path from i to j can be chosen as
● if i>j: the path is (i→i−1→i−2→⋯→j);
● if i<j: the path is (i→i−1→i−2→⋯→2→1→j).
Hence, G(PB) is strongly connected. From Definition 2, we know that the matrix PB is irreducible.
Part 2: the characteristic polynomial of the matrix PB. Let R(λ)=Δ(PB−λI) be the characteristic polynomial of the matrix PB, where λ is a scale number and for any square matrix A, Δ(A) denotes the determinant of A. To derive an explicit expression of R(λ), we recall a well known result which states that
Δ(PB−λI)=−λΔ(R1,1)−ηλJf1−βΔ(R1,2)+ηλJ−1f1−βΔ(R1,3)+⋯+(−1)1+Jηλf1−βΔ(R1,J+1), | (3.16) |
where R1,j is a J×J matrix obtained by removing the first row and the j-th column from the matrix PB−λI. Clearly, Δ(R1,1)=(−λ)J and Δ(R1,2)=λf1−μλf(−λ)J−1 since both R1,1 and R1,2 are lower triangular matrices. But the matrices R1,j with 3≤j≤J+1 are not lower triangular. For any j∈{3,4,…,J,J+1}, we have
R1,j=(σ−λ⋱⋱σ−λσ00−λσ−λ⋱⋱σ−λ),σ=λf1−μλf, |
where the entries "σ,0" corresponds to the (j−1)-th row of the matrix R1,j. It follows by applying the expansion like (3.16) to R1,j that Δ(R1,j)=σj−1(−λ)J−j+1. Hence, substituting these expressions into (3.16) gives
Δ(PB−λI)=(−λ)J+1+J+1∑j=2(−1)j+1ηλJ+1f(1−μλf)j−1(1−β)(−λ)J−j+1=λJ(−1)J+1(λ−ηλJ+1f1−βJ+1∑j=21[(1−μλf)λ]j−1)=λJ(−1)J+1(λ−ηλJ+1f1−βJ∑j=11[(1−μλf)λ]j). | (3.17) |
From (3.17), we know that the nonzero root of Δ(PB−λI)=0 satisfies
λ=ηλJ+1f1−βJ∑j=11[(1−μλf)λ]j. | (3.18) |
Now, by combining Parts 1 and 2 and by using Lemma 3 we get
ρ(PB)<1⇔ηλJ+1f1−βJ∑j=11(1−μλf)j<1(⇔ηλJf(1−[1−μλf]J)μ(1−β)(1−μλf)J<1), | (3.19) |
since ∑Jj=11[(1−μλf)λ]j is a decreasing function of λ for λ>0.
Remark 2. (speed-up analysis). On each time grid, let Te and te be the CPU time spent for applying the backward Euler method directly to the original PDE and the PDE on a subdomain, respectively. Since the number of subdomains is N and the overlap size is small, it holds that te≈TeN. Now, assume we have Nt×N processors at our disposal, where Nt processors are assigned to the parareal method and N processors are assigned to the SWR method. The overall cost of the Para-SWR algorithm for per iteration is given by the cost of applying the fine and the coarse propagators simultaneously on each processor|(J+1)te, the cost of applying the coarse propagator G sequentially|Ntte and some necessary communication cost|Tc; in total
TPara-SWR:=Jte+Ntte+Tc. | (3.20) |
At the current iteration, say the k-th iteration, the communication cost Tc spent on each coarse time grid Tn mainly comes from transmission of solutions on fine time grids between adjacent subdomains. For example, the processor Pn,i|assigned to the n-th coarse time grid Tn and the i-th subdomain, needs to get fine solutions VTn,1,2,…,J(i−1) and VTn,1,2,…,J(i+1) from its neighboring processor Pn,i−1 and Pn,i+1, respectively. Also, it needs to broadcast the solutions VTn,1,2,…,J(i) to its two neighboring processors. Clearly, this process can be done simultaneously in all parallelled time intervals. In the longitudinal direction, this can be done sequentially by two steps: first the N processors simultaneously transmit the fine solutions along the upward direction and then along the downward direction (see Figure 2 for illustration). Let δ be the unit cost spent to communicate a float number between two processors. Then, the total communication time Tc can be written as
Tc=2×J×m×δ, | (3.21) |
where m is the number of space mesh points on a spatial subdomain. Substituting Tc into (3.20) gives
TPara-SWR=(J+Nt)te+2Jmδ≈(J+Nt)TeN+2Jmδ | (3.22) |
The cost of applying the fine propagator sequentially and directly to the large circuit is
TSequent:=Nt×J×Te. | (3.23) |
We therefore obtain a speed-up of using the Para-SWR algorithm as
TSequentTPara-SWR=Nt×J×TeKmax((J+Nt)TeN+2Jmδ)≈NNtJKmax(J+Nt)(if Te≫2Jmδ), | (3.24) |
where Kmax is the number of iterations performed to meet the stopping criterion. The condition Te≫2Jmδ occurs naturally if the space domain of the original PDE is large and the discretization mesh Δx is small.
The analysis given above is performed on sufficiently long time domains and therefore ρ(M) is independent of Nt, the number of paralleled time intervals. We now focus on proving that ρ(M) is robust with respect to N, the number of subdomains. We assume a>0 and consider the case q=0. Let Δt, J, Δx and L (the length of the space domain) be fixed constants, and then we claim that the arguments λf and λc decrease as N increases. Indeed, since q=0 we have A+=A−=A and the eigenvalues are given by
λi(A)=−(2ν2Δx2+a)+2ν2Δx2cos(iπm+1)=−a−4ν2Δx2sin2(jπ2(m+1)),j=1,2,…,m, | (3.25) |
where m=L/Δx−2N+1. From (3.25), we have
λf=11+Δt(a+4ν2Δx2sin2(π2(m+1))),λc=11+ΔT(a+4ν2Δx2sin2(π2(m+1))). | (3.26) |
Clearly, both λf and λc increase as m increases, i.e., λf and λc decrease as N increases, since L=[N×(m−1)+2]×Δx is constant. For the matrix B defined by (2.7) it is easy to know that the quantity ‖B‖2 is independent of the number m and equal to ν2Δx2 since q=0. Hence, we get μ=Δtν2Δx2 for all possible N.
Now, for the iteration matrix M defined by (3.9) the aforementioned analysis implies that, except for β, all of the other elements decrease as N increases. From (3.25), we also have
‖ˉAJf−ˉAc‖2=max1≤i≤m|1(1+Δt(a+4ν2Δx2sin2(iπ2(m+1))))J−11+ΔT(a+4ν2Δx2sin2(iπ2(m+1)))|≤maxz≥0Γ(J), | (3.27) |
where Γ(J)=maxz≥0|11+Jz−1(1+z)J|. The function Γ(J) is shown in Figure 3, and it holds that Γ(J)≤0.205 for J∈{2,3,…,500}. We therefore can bound β as β≤Γ(J)1−λc for all possible N.
Corollary 1 (weak scaling). Let q=0 and a>0. Then, for a fixed Δt, J, Δx and L, the Para-SWR algorithm converges linearly on sufficiently long time intervals and the convergence rate can be bounded by ρ(M∗), which is independent of the number of subdomains, provided Condition (3.11) is satisfied with
λ∗f=11+Δt(a+4ν2Δx2sin2(π2(mmax+1))),λ∗c=11+ΔT(a+4ν2Δx2sin2(π2(mmax+1))),β∗=Γ(J)1−λ∗c,μ∗=ν2ΔtΔx2,η∗=μ∗1−λ∗c, | (3.28) |
where the matrix M∗ is derived from M by letting λf=λ∗f, λc=λ∗c, η=η∗, β=β∗ and μ=μ∗, and mmax=1+[L2Δx] and [L2Δx] denote the integer part of L2Δx.
Proof. Since N≥2 and the length of the space domain L=[N×(m−1)+2]Δx is fixed, it is easy to know that the maximal integer m in (3.26) is mmax=1+[L2Δx]. Hence, for any possible integer N it holds that M≤M∗. Since both M and M∗ are nonnegative matrices, we know from [45,Theorem 2.21,p. 51] that ρ(M)≤ρ(M∗).
Note that, the choice q=0 corresponds to p=∞ and in this case the transmission condition used in (2.3) reduces to the Dirichlet transmission condition. For the general Robin transmission condition, i.e., q∈(0,+∞), it is difficult to prove a similar conclusion like Corollary 1.
We next investigate how the convergence rate of the Para-SWR algorithm behaves with respect to the quantities ν2Δx2 and a. It is difficult to make a theoretical analysis, and we have to rely on numerical investigation. Since ρ(M∗) is a suitable upper bound of ρ(M) and independent of the number of subdomains, we plotted it in Figure 4 on the top of the quantity ρ(M∗) as a function of ν2Δx2 and a, where J=50 and ΔT=0.1 are considered. We see that ρ(M∗) decreases as a increases or ν2Δx2 decreases. This provides us with two possible acceleration strategies for the Para-SWR algorithm. The first one is the change of variables that we have described at the beginning of Section 2. For the model problem (2.1), by letting u(x,t)=v(x,t)eτt we get
{∂tv−ν2∂xxv+(a+τ)v=e−τtf(x,t),(x,t)∈(0,L)×R+,v(0,t)=e−τtb1(t),t≥0,v(L,t)=e−τtb2(t),t≥0,v(x,0)=u0(x),x∈[0,L], | (3.29) |
and this is essentially the same PDE as (2.1), but with a new reaction parameter a+τ. Due to the plot shown in Figure 4 on the top, it seems that the Para-SWR algorithm for (3.29) converges very fast if we choose a very large τ. However, this is a false prediction. Briefly speaking, for a large τ, we find numerically that even though maxj,n‖Vk(xj,Tn)−V(xj,Tn)‖2 is already very small, maxj,n‖Uk(xj,Tn)−U(xj,Tn)‖2 is still a huge number, where Uk(xj,Tn) is calculated by Uk(xj,Tn)=eτTnVk(xj,Tn); we denote Vk as the numerical solutions generated by the Para-SWR algorithm after k iterations for the transformed PDE (3.29). To illustrate this, by using the example given in (4.1) with a=1,ν=0.01, T=5, ΔT=0.1,J=50 and N=8, we plotted the diminution history of maxj,n‖Vk(xj,Tn)−V(xj,Tn)‖2 and maxj,n‖Uk(xj,Tn)−U(xj,Tn)‖2 in Figure 4 on the bottom for two choices of τ.
The second possibility is the change of space coordinates. Note that, if we let x=ω˜x the PDE problem (2.1) is transformed to
∂tu(˜x,t)−(ων)2∂2˜xu(˜x,t)+au(˜x,t)=f(˜x,t),(˜x,t)∈(0,˜L)×R+, | (3.30) |
where ˜L=Lω. The new diffusion coefficient ων can be sufficiently small by choosing a small positive ω, which implies that the Para-SWR algorithm converges significantly fast. However, in the meantime the computational domain (0,Lω) will become large and to maintain a required spatial accuracy, a uniform step size Δx should be used for all ω. Hence, for a fixed N|the number of subdomains, the computational cost at each iteration will also significantly increase. To reduce the computational cost at each iteration, we can increase the number of subdomains to ˜N=N[1ω]. Since the convergence rate of the Para-SWR algorithm is robust with respect to the number of subdomains, we know that the total computational cost for the Para-SWR algorithm will be evidently reduced by using a small ω. In practical computation, we can choose ω=12,14,18,…, since in this way it is convenient to increase the subdomains multiplicatively, as ˜N=2N,4N,8N,….
In this section, we show several numerical experiments to test the efficiency of the Para-SWR algorithm proposed in this paper. We focus on observing how the convergence rate of this algorithm behaves with respect to N|the number of subdomains, Nt|the number of paralleled time intervals and J|the multiplication ratio between ΔT and Δt. We consider the following PDE problem
{∂tu−ν2∂xxu+au=5sin(3[√(t−0.5T)2+(10(x−0.5))2])√(t−0.5T)2+(10(x−0.5))2,(x,t)∈(0,1)×(0,T),u(0,t)=u(1,t)=0,t≥0,u(x,0)=0,x∈[0,1], | (4.1) |
where ν=0.01 and a=6. The space discretization parameter Δx is fixed as Δx=1128 and the initial solution for the Para-SWR algorithm is chosen randomly in the interval [0,T]. For all experiments the iteration stops when the maximal error between the current iteration and the converged solution arrives at a prescribed tolerance.
Example 4.1 (Dirichlet transmission condition). We first consider the Dirichlet transmission condition, i.e., q=0. Let J=50 and ΔT=0.1, and then it is easy to get ρ(M∗)=0.8465. Hence, Corollary 1 implies that the convergence rate of the Para-SWR algorithm is robust with respect to N, the number of subdomains. The results shown in Figure 5 on the top indicate that this theoretical predication is correct. The other two panels of Figure 5 are concerned with the dependence of the convergence rate of the algorithm on the multiplication ratio J (bottom left) and the number of parallelled time intervals (bottom right). We see that the convergence rate is also robust with respect to J and Nt. The results shown in Figure 5 imply that the Para-SWR algorithm really scales weakly, and that this strong robustness indicates that the strategy of change of space coordinates mentioned in Subsection 3.2 is viable.
Example 4.2 (Robin transmission condition). We next consider the Robin transmission condition. For the SWR algorithm, it is well known that the Robin transmission condition is more powerful than the Dirichlet transmission condition; see, e.g., [8,9]. For T=9, J=50 and ΔT=0.1, we show in Figure 6, on the top, the argument ρ(M) as a function of the parameter q (=1Δxp−1). We see that ρ(M) attains its minimum at q=0, i.e., p=∞, which corresponds to the Dirichlet transmission condition. Does this predict the practical computation well? In Figure 6 on the bottom, we show the errors obtained by running the Para-SWR algorithms with Robin transmission conditions after k iterations and various choices of the free parameters q. We see that, indeed, q=0 is the best choice; therefore, these numerical results confirm our theoretical predication shown on the top.
The plot shown in Figure 6 on the top also predicts that increasing q has a negative effect on the convergence rate of the Para-SWR algorithm, and that, for a very large q the algorithm will not converge. This is verified in Figures 7 and 8. In Figure 7, we show on the top row the third, the fourth and the fifth iterations of the 8-subdomain Para-SWR algorithm with q=0, and below we show the same iterations for the algorithm with q=4. This clearly shows that the choice q=0 is more advisable than q>0, which confirms the prediction shown in Figure 6 on the top.
In Figure 8, we show the measured convergence rate of the Para-SWR algorithm in the case of two subdomains and 32 subdomains. The results shown in this figure imply that a large q really slows down the convergence rate; note how the iteration stagnates for q=100.
In this paper, we have presented a hybrid algorithm based on the well understood parareal algorithm and the SWR algorithm. The algorithm, namely, Para-SWR, has been defined by first applying the SWR algorithm to time-dependent PDEs in the case of general N subdomains and then applying the one-iteration parareal algorithm to each subproblem. Convergence of the algorithm was analyzed, and it has been shown theoretically and numerically that the convergence rate is robust with respect to the number of subdomains and the number of parallelled time intervals. This implies that, compared to the parareal algorithm and the SWR algorithm, the proposed hybrid algorithm really brings more parallelism.
There are still some important problems that need to be answered in further work. First of all, the spectral radius ρ(M) is not a good bound of the practical convergence rate. It is conservative and even invalid in some cases. Briefly speaking, for the case ν2Δx2/a≫1 we found that ρ(M)>1, while the Para-SWR algorithm still converges. For example, by letting a=0 in (4.1) (i.e., the heat equation), ν=0.01, T=10,Δx=1128, ΔT=0.1 and J=50, we have ρ(M)>1 for all q≥0 and ν2Δx2>0, but the numerical results indicate that the Para-SWR algorithm is actually convergent.
The other problem is how to generalize current work to other numerical methods, such as the Runge-Kutta (RK) methods. Such a generalization is not straightforward: if we choose an s-stage RK method with ˜s off-steps (the intermediary nodes located in the fine time slice(Tn+jΔtJ,Tn+(j+1)ΔtJ)), the iteration matrix M would be a (1+˜s+J)×(1+˜s+J) matrix and its structure would be completely different from the one given by (3.9). Therefore, the current approach for proving ρ(M)<1 is not applicable. However, one can check that the proof can be straightforwardly generalized to the linear-θ method, which can be regarded as a family of simple RK methods with ˜s=0.
This work was supported by the National Natural Science Foundation of China (12101089) and the Natural Science Foundation of Sichuan Province (2022NSFSC1844).
The authors declare that there is no conflicts of interest.
[1] | A. Toselli, O. Widlund, Domain Decomposition Methods: Algorithms and Theory, Springer, Berlin, 2005. |
[2] | T. P. A. Mathew, Domain Decomposition Methods for the Numerical Solution of Partial Differential Equations, Springer-Verlag, Berlin, 2008. |
[3] |
X. C. Cai, Additive Schwarz algorithms for parabolic convection-diffusion equations, Numer. Math., 60 (1991), 41-61. https://doi.org/10.1007/BF01385713 doi: 10.1007/BF01385713
![]() |
[4] |
X. C. Cai, Multiplicative Schwarz methods for parabolic problems, SIAM J. Sci. Comput., 15 (1994), 587-603. https://doi.org/10.1137/0915039 doi: 10.1137/0915039
![]() |
[5] |
L. Qin, X. J. Xu, Optimized Schwarz methods with Robin transmission conditions for parabolic problems, SIAM J. Sci. Comput., 31 (2008), 608-623. https://doi.org/10.1137/070682149 doi: 10.1137/070682149
![]() |
[6] |
M. Al-Khaleel, S. L. Wu, Quasi-overlapping semi-discrete Schwarz waveform relaxation algorithms: The hyperbolic problem, Comput. Methods Appl. Math., 20 (2020), 397-417. https://doi.org/10.1515/cmam-2018-0188 doi: 10.1515/cmam-2018-0188
![]() |
[7] |
D. Bennequin, M. J. Gander, L. Gouarin, L. Halpern, Optimized Schwarz waveform relaxation for advection reaction diffusion equations in two dimensions, Numer. Math., 134 (2016), 513-567. https://doi.org/10.1007/s00211-015-0784-8 doi: 10.1007/s00211-015-0784-8
![]() |
[8] |
D. Bennequin, M. J. Gander, L. Halpern, A homographic best approximation problem with application to optimized Schwarz waveform relaxation, Math. Comput., 78 (2009), 185-223. https://doi.org/10.1090/S0025-5718-08-02145-5 doi: 10.1090/S0025-5718-08-02145-5
![]() |
[9] |
M. J. Gander, L. Halpern, Optimized Schwarz waveform relaxation for advection reaction diffusion problems, SIAM J. Numer. Anal., 45 (2007), 666-697. https://doi.org/10.1007/s00211-015-0784-8 doi: 10.1007/s00211-015-0784-8
![]() |
[10] |
M. J. Gander, A. M. Stuart, Space-time continuous analysis of waveform relaxation for the heat equation, SIAM J. Sci. Comput., 19 (1998), 2014-2031. https://doi.org/10.1137/S1064827596305337 doi: 10.1137/S1064827596305337
![]() |
[11] |
E. Giladi, H. B. Keller, Space-time domain decomposition for parabolic problems, Numer. Math., 93 (2002), 279-313. https://doi.org/10.1007/s002110100345 doi: 10.1007/s002110100345
![]() |
[12] |
S. L. Wu, M. D. Al-Khaleel, Semi-discrete Schwarz waveform relaxation algorithms for reaction diffusion equations, BIT Numer. Math., 54 (2014), 831-866. https://doi.org/10.1007/s10543-014-0475-3 doi: 10.1007/s10543-014-0475-3
![]() |
[13] |
S. L. Wu, M. D. Al-Khaleel, Convergence analysis of the Neumann-Neumann waveform relaxation method for time-fractional RC circuits, Simul. Model. Pract. Theory, 64 (2016), 43-56. https://doi.org/10.1016/j.simpat.2016.01.002 doi: 10.1016/j.simpat.2016.01.002
![]() |
[14] |
J. L. Lions, Y. Maday, G. Turinici, Résolution d'EDP par un schéma en temps pararéel, C. R. Acad. Sci. Paris Sér. I Math., 332 (2001), 661-668. https://doi.org/10.1016/S0764-4442(00)01793-6 doi: 10.1016/S0764-4442(00)01793-6
![]() |
[15] |
M. Cai, J. Mahseredjian, I. Kocar, X. Fu, A. Haddadi, A parallelization-in-time approach for accelerating EMT simulations, Electr. Power Syst. Res., 197 (2021), 107346. https://doi.org/10.1016/j.epsr.2021.107346 doi: 10.1016/j.epsr.2021.107346
![]() |
[16] | T. Cheng, N. Lin, V. Dinavahi, Hybrid parallel-in-time-and-space transient stability simulation of large-ccale AC/DC grids, IEEE Trans. Power Syst., in press, 2022. https://doi.org/10.1109/TPWRS.2022.3153450 |
[17] |
I. C. Garcia, I. Kulchytska-Ruchka, S. Schops, Parareal for index two differential algebraic equations, Numer. Alg., 91 (2022), 389-412. https://doi.org/10.1007/s11075-022-01267-1 doi: 10.1007/s11075-022-01267-1
![]() |
[18] |
E. Celledoni, T. Kvamsdal, Parallelization in time for thermo-viscoplastic problems in extrusion of aluminium, Int. J. Numer. Methods Eng., 79 (2009), 576-598. https://doi.org/10.1002/nme.2585 doi: 10.1002/nme.2585
![]() |
[19] |
D. Max, M. Marc, D. Stéphane, Parareal operator splitting techniques for multi-scale reaction waves: numerical analysis and strategies, ESAIM Math. Model. Numer. Anal., 45 (2011), 825-852. https://doi.org/10.1051/m2an/2010104 doi: 10.1051/m2an/2010104
![]() |
[20] |
L. Fang, S. Vandewalle, J. Meyers, A parallel-in-time multiple shooting algorithm for large-scale PDE-constrained optimal control problems, J. Comput. Phys., 452 (2021), 110926. https://doi.org/10.1016/j.jcp.2021.110926 doi: 10.1016/j.jcp.2021.110926
![]() |
[21] |
M. J. Gander, F. Kwok, J. Salomon, PARAOPT: a parareal algorithm for optimality systems, SIAM J. Sci. Comput., 42 (2020), A2773-A2802. https://doi.org/10.1137/19M1292291 doi: 10.1137/19M1292291
![]() |
[22] | Y. Maday, M. K. Riahi, J. Salomon, Parareal in time intermediate targets methods for optimal control problems, in Control and Optimization with PDE Constraints, Birkhäuser, Basel, 164 (2013), 79-92. https://doi.org/10.1007/978-3-0348-0631-2_5 |
[23] |
M. K. Riahi, J. Salomon, S. J. Glaser, D. Sugny, Fully efficient time-parallelized quantum optimal control algorithm, Phys. Rev. A, 93 (2016), 043410. https://doi.org/10.1103/PhysRevA.93.043410 doi: 10.1103/PhysRevA.93.043410
![]() |
[24] |
X. M. Gu, S. L. Wu, A parallel-in-time iterative algorithm for Volterra partial integro-differential problems with weakly singular kernel, J. Comput. Phys., 417 (2020), 109576. https://doi.org/10.1016/j.jcp.2020.109576 doi: 10.1016/j.jcp.2020.109576
![]() |
[25] |
X. M. Gu, Y. L. Zhao, X. L. Zhao, B. Carpentieri, Y. Y. Huang, A note on parallel preconditioning for the all-at-once solution of Riesz fractional diffusion equations, Numer. Math. Theor. Meth. Appl., 14 (2021), 893-919. https://doi.org/10.4208/nmtma.OA-2020-0020 doi: 10.4208/nmtma.OA-2020-0020
![]() |
[26] |
Y. L. Zhao, P. Y. Zhu, X. M. Gu, X. L. Zhao, H. Y. Jian, A preconditioning technique for all-at-once system from the nonlinear tempered fractional diffusion equation, J. Sci. Comput., 83 (2020), 10. https://doi.org/10.1007/s10915-020-01193-1 doi: 10.1007/s10915-020-01193-1
![]() |
[27] |
M. J. Gander, S. Vandewalle, Analysis of the parareal time-parallel time-integration method, SIAM J. Sci. Comput., 29 (2007), 556-578. https://doi.org/10.1137/05064607X doi: 10.1137/05064607X
![]() |
[28] |
M. J. Gander, S. L. Wu, A diagonalization-based parareal algorithm for dissipative and wave propagation problems, SIAM J. Numer. Anal., 58 (2020), 2981-3009. https://doi.org/10.1137/19M1271683 doi: 10.1137/19M1271683
![]() |
[29] |
S. L. Wu, Toward parallel coarse grid correction for the parareal algorithm, SIAM J. Sci. Comput., 40 (2018), A1446-A1472. https://doi.org/10.1137/17M1141102 doi: 10.1137/17M1141102
![]() |
[30] |
S. L. Wu, T. Zhou, Convergence analysis for three parareal solvers, SIAM J. Sci. Comput., 37 (2015), A970-A992. https://doi.org/10.1137/140970756 doi: 10.1137/140970756
![]() |
[31] | T. Cadeau, F. Mafoules, Coupling the parareal algorithm with the waveform relaxation method for the solution of differential algebraic equations, in 10th International Symposium on Distributed Computing and Applications to Business, Engineering and Science, (2011), 15-19. https://doi.org/10.1109/DCABES.2011.34 |
[32] | Y. L. Jiang, B. Song, Coupling parareal and Dirichlet-Neumann/Neumann-Neumann waveform relaxation methods for the heat equation, in International Conference on Domain Decomposition Methods, Springer, Cham, 125 (2018), 405-413. https://doi.org/10.1007/978-3-319-93873-8_38 |
[33] | T. Cadeau, F. Magoulès, Coupling parareal and waveform relaxation methods for power systems, in IEEE International Conference on Electrical and Control Engineering, (2011), 2947-2950. https://doi.org/10.1109/ICECENG.2011.6057305 |
[34] |
J. Li, Y. L. Jiang, Z. Miao, A parareal approach of semi-linear parabolic equations based on general waveform relaxation, Numer. Methods Partial Differ. Equations, 35 (2019), 2017-2043. https://doi.org/10.1002/num.22390 doi: 10.1002/num.22390
![]() |
[35] | J. Liu, Y. L. Jiang, A parareal algorithm based on waveform relaxation, Math. Comput. Simul. 82 (2012), 2167-2181. https://doi.org/10.1016/j.matcom.2012.05.017 |
[36] |
J. Liu, Y. L. Jiang, A parareal waveform relaxation algorithm for semi-linear parabolic partial differential equations, J. Comput. Appl. Math., 236 (2012), 4245-4263. https://doi.org/10.1016/j.cam.2012.05.014 doi: 10.1016/j.cam.2012.05.014
![]() |
[37] |
B. Song, Y. L. Jiang, Analysis of a new parareal algorithm based on waveform relaxation method for time-periodic problems, Numer. Algorithms, 67 (2014), 599-622. https://doi.org/10.1007/s11075-013-9810-z doi: 10.1007/s11075-013-9810-z
![]() |
[38] |
B. Song, Y. L. Jiang, A new parareal waveform relaxation algorithm for time-periodic problems, Int. J. Comput. Math., 92 (2015), 377-393. https://doi.org/10.1080/00207160.2014.891734 doi: 10.1080/00207160.2014.891734
![]() |
[39] |
B. Song, Y. L. Jiang, X. Wang, Analysis of two new parareal algorithms based on the Dirichlet-Neumann/Neumann-Neumann waveform relaxation method for the heat equation, Numer. Algorithms, 6 (2021), 1685-1703. https://doi.org/10.1007/s11075-020-00949-y doi: 10.1007/s11075-020-00949-y
![]() |
[40] |
E. Lelarasmee, A. E. Ruehli, A. L. Sangiovanni-Vincentelli, The waveform relaxation methods for time-domain analysis of large scale integrated circuits, IEEE Trans. Comput. Aided Des. Integr. Circuits Syst., 1 (1982), 131-145. https://doi.org/10.1109/TCAD.1982.1270004 doi: 10.1109/TCAD.1982.1270004
![]() |
[41] |
D. Q. Bui, C. Japhet, Y. Maday, P. Omnes, Coupling parareal with optimized Schwarz waveform relaxation for parabolic problems, SIAM J. Numer. Anal., 60 (2022), 913-9399. https://doi.org/10.1137/21M1419428 doi: 10.1137/21M1419428
![]() |
[42] | M. J. Gander, Y. Jiang, R. Li, Parareal Schwarz waveform relaxation methods, in Domain Decomposition Methods in Science and Engineering XX, Springer, Berlin, Heidelberg, 91 (2012), 451-458. https://doi.org/10.1007/978-3-642-35275-1_53 |
[43] |
M. Gander, Y. Jiang, B. Song, A superlinear convergence estimate for the parareal Schwarz waveform relaxation algorithm, SIAM J. Sci. Comput., 41 (2019), A1148-A1169. https://doi.org/10.1137/18M1177226 doi: 10.1137/18M1177226
![]() |
[44] |
M. J. Gander, M. Al-Khaleel, A. Ruehli, Optimized waveform relaxation methods for the longitudinal partitioning of transmission lines, IEEE Trans. Circuits Syst. I Regul. Pap., 56 (2009), 1732-1743. https://doi.org/10.1109/TCSI.2008.2008286 doi: 10.1109/TCSI.2008.2008286
![]() |
[45] | R. S. Varga, Matrix Iterative Analysis, 2nd edition, Spring-Verlag, Berlin Heidelberg, 2000. |