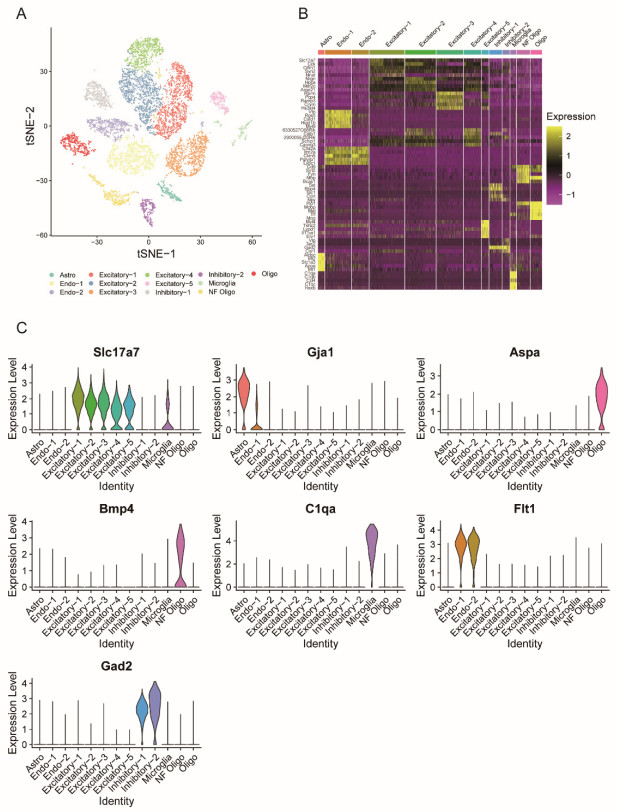
Citation: Shota Tobaru, Ryuto Shigenobu, Foday Conteh, Naomitsu Urasaki, Abdul Motin Howlader, Tomonobu Senjyu, Toshihisa Funabashi. Optimal operation method coping with uncertainty in multi-area small power systems[J]. AIMS Energy, 2017, 5(4): 718-734. doi: 10.3934/energy.2017.4.718
[1] | Jiaxin Luo, Lin Wu, Dinghui Liu, Zhaojun Xiong, Linli Wang, Xiaoxian Qian, Xiaoqiang Sun . Gene regulatory network analysis identifies key genes and regulatory mechanisms involved in acute myocardial infarction using bulk and single cell RNA-seq data. Mathematical Biosciences and Engineering, 2021, 18(6): 7774-7789. doi: 10.3934/mbe.2021386 |
[2] | Linqian Guo, Qingrong Meng, Wenqi Lin, Kaiyuan Weng . Identification of immune subtypes of melanoma based on single-cell and bulk RNA sequencing data. Mathematical Biosciences and Engineering, 2023, 20(2): 2920-2936. doi: 10.3934/mbe.2023138 |
[3] | Kaiyu Shen, Shuaiyi Ke, Binyu Chen, Tiantian Zhang, Hongtai Wang, Jianhui Lv, Wencang Gao . Identification and validation of biomarkers for epithelial-mesenchymal transition-related cells to estimate the prognosis and immune microenvironment in primary gastric cancer by the integrated analysis of single-cell and bulk RNA sequencing data. Mathematical Biosciences and Engineering, 2023, 20(8): 13798-13823. doi: 10.3934/mbe.2023614 |
[4] | Shaoyu Li, Su Xu, Xue Wang, Nilüfer Ertekin-Taner, Duan Chen . An augmented GSNMF model for complete deconvolution of bulk RNA-seq data. Mathematical Biosciences and Engineering, 2025, 22(4): 988-1018. doi: 10.3934/mbe.2025036 |
[5] | Yi Shi, Xiaoqian Huang, Zhaolan Du, Jianjun Tan . Analysis of single-cell RNA-sequencing data identifies a hypoxic tumor subpopulation associated with poor prognosis in triple-negative breast cancer. Mathematical Biosciences and Engineering, 2022, 19(6): 5793-5812. doi: 10.3934/mbe.2022271 |
[6] | Xiao Tu, Qinran Zhang, Wei Zhang, Xiufen Zou . Single-cell data-driven mathematical model reveals possible molecular mechanisms of embryonic stem-cell differentiation. Mathematical Biosciences and Engineering, 2019, 16(5): 5877-5896. doi: 10.3934/mbe.2019294 |
[7] | Changxiang Huan, Jiaxin Gao . Insight into the potential pathogenesis of human osteoarthritis via single-cell RNA sequencing data on osteoblasts. Mathematical Biosciences and Engineering, 2022, 19(6): 6344-6361. doi: 10.3934/mbe.2022297 |
[8] | Haiqiang Liu, Feifei Shen, Hewei Zhang, Weikai Zhang . Expression and role of cystatin C in hyperthermia-induced brain injury in rats. Mathematical Biosciences and Engineering, 2023, 20(2): 2716-2731. doi: 10.3934/mbe.2023127 |
[9] | Heyrim Cho, Ya-Huei Kuo, Russell C. Rockne . Comparison of cell state models derived from single-cell RNA sequencing data: graph versus multi-dimensional space. Mathematical Biosciences and Engineering, 2022, 19(8): 8505-8536. doi: 10.3934/mbe.2022395 |
[10] | Jingxi Xu, Jiangtao Li . Construction of a three commitment points for S phase entry cell cycle model and immune-related ceRNA network to explore novel therapeutic options for psoriasis. Mathematical Biosciences and Engineering, 2022, 19(12): 13483-13525. doi: 10.3934/mbe.2022630 |
Traumatic brain injury (TBI) results from external forces such as a blow to the head or an object penetrating the skull. TBI is regarded as a common cause of death and disability and a major healthcare issue worldwide [1,2]. It often leads to various central nervous system (CNS) symptoms and greatly increases the risk of developing long-lasting psychiatric disorders. Since TBI can be highly heterogeneous, the prevention of post-TBI complications is one of the greatest challenges for researchers and therapeutists.
Neuroinflammation is considered as an important post-TBI secondary injury mechanism, with hallmarks such as neurovascular unit (NVU) alterations [3]. The NVU is mainly composed of neurons, astrocytes, microglia, endothelial cells and smooth muscle cells [4], and it has been demonstrated that the activation of microglia and astrocytes could promote neuroinflammation via secreting more proinflammatory cytokines, such as TNF-α and IL-1β [1,5,6]. Certain microglia receptors, namely CX3CR1, P2Y12R, TLR4, IL1R1, RAGE and TNFR1, have been highlighted, and their associated downstream pathways include the PI3K/AKT, MAPK, cAMP, and NF-κB signaling pathway, which activate the expression of a series of inflammatory genes [7]. Previous studies have also suggested an association between neuroinflammatory responses and excitatory-to-inhibitory synaptic imbalance in patients and animal models of TBI. Synaptic reorganization has been observed in mice after TBI, which is possibly caused by disturbed neurotransmitter release [8,9]. In addition, TBI could lead to endothelial dysfunction and cardiovascular diseases, where the nitric oxide (NO) and endothelial-dependent hyperpolarization (EDH) pathways played a critical role [5]. Meanwhile, vascular endothelial growth factor (VEGF) has been found to participate in neuroprotection after TBI [10].
The advances in single-cell RNA sequencing technology have enabled the exploration of complex and rare cell populations and helped uncover regulatory mechanisms between genes and cells involved in the pathogenic processes of several diseases [11,12,13,14]. However, a detailed landscape of molecular mechanisms and pathways involved in post-TBI pathology still remains absent. In the present study, we have integrated single-cell and bulk RNA-seq data to identify TBI-related cell types and regulators, hopefully providing some novel therapeutic targets for TBI.
The single-cell RNA-seq data from cortex of mice was collected from Gene Expression Omnibus (GEO) with accession number GSE124952 [15]. The RNA-seq data of cortex tissues in mice with TBI and normal controls and the RNA-seq data of Dap12 (Tyrobp)-deficient mouse microglial cells were downloaded from GEO with accession numbers GSE79441 [16] and GSE9043, respectively. Data from GEO under accession numbers GSE68207 [17] and GSE155063 [18] were used for TYROBP validation. All these data were pre-normalized in GEO database, and were used for downstream analysis.
The cell clustering analysis was implemented in R Seurat [15] package. Specifically, the count-based expression data was first imported into R using CreateSeuraObject, and the top 2000 highly variable features were selected using FindVariableFeatures with vst method. We selected different clustering resolutions at 0.1, 0.3, 0.5 and 0.7, manually compared those clustering results, and observed that the clustering with a resolution at 0.3 achieved the optimal partitions. Thus, the clusters were identified at a resolution of 0.3 using FindClusters, and T-distributed Stochastic Neighbor Embedding (t-SNE) was applied to reduce the dimensionality. The cell-type specific genes were detected using FindAllMarkers function at adjusted P-value < 0.05 and minimal percentage > 0.25. Reported marker genes of those cell clusters were collected from an earlier study [16]. Visualization tools for the scRNA-seq data such as t-SNE, heat-map, and vioplot were also implemented in R Seurat package.
Two enrichment algorithms, gene set enrichment analysis (GSEA) and gene set overrepresentation enrichment analysis (ORA) were employed in this study. These two algorithms were applied to identify cell types involved in TBI. Also, GSEA was applied to identify pathways enriched in the gene sets of interest, which was implemented in R clusterProfiler [17] package.
The ligand-receptor pairs were collected from a public dataset provided by an earlier study [18]. The cell-type specificity of differentially expressed genes encoding ligands or receptors in TBI were then tested in identified cell populations. Subsequently, these cell-type specific ligands or receptors involved in TBI were mapped to known ligand-receptor pairs, and those successfully mapped pairs were considered as links between corresponding cell types.
Differential expression analysis of bulk RNA-seq was conducted using R DESeq2 package. Specifically, disease status (TBI or control) was regarded as a variable in the meta-data. Genes with an FPKM value above 1 in at least 20% of samples were retained for this analysis. The count-based gene expression was normalized using the median of ratios method. The thresholds of adjusted P-value and log2 (fold change) were selected at 0.05 and 0.5, respectively. Differential expression analysis of single-cell RNA-seq data based on Wilcoxon rank sum test was performed, which was implemented in R Seurat package with function FindAllMarkers.
To define cell types in mouse cortex, we collected a total of 10,538 single cells in cortex without any treatments from a previous study [16], and conducted T-distributed Stochastic Neighbor Embedding (t-SNE) analysis to identify cell clusters. Totally, we identified 13 cell clusters and characterized cell types by known markers [16] of astrocytes (Astro), oligodendrocyte, newly formed oligodendrocytes (NF Oligo), oligodendrocyte precursors (Oligo), microglia (Microglia), endothelial cells (Endo), excitatory and inhibitory neurons (Excitatory and Inhibitory) (Figure 1A). Particularly, excitatory and inhibitory neurons and endothelial cells could be further divided into 5, 2 and 2 sub-clusters in this clustering (Figure 1B), and these cell populations were denoted as Excitatory-1/2/3/4/5, Inhibitory-1/2 and Endo-1/2. Specifically, marker genes including Ctla2a, Ly6c1, Pglyrp1, Cldn5 and Itm2a were shared by these two types of endothelial cells, while Myl9, Higd1b, Cald1, Rgs5 and Vtn were specifically expressed in Endo-1. Notably, Myl9 and Cald1 were involved in vascular smooth muscle contraction. Comparison of the representative genes in the excitatory neurons revealed that gene expressions in Excitatory-1, 2 and 4 shared a high similarity, and Excitatory-3 and 5 had more unique expression patterns. Specific expressions of certain marker genes further confirmed that this characterization of cell types makes biological sense (Figure 1C), including Slc17a7, Gja1, Aspa, Bmp4, C1qa, Flt1 and Gad2, which were detected respectively in excitatory neurons, astrocytes, oligodendrocyte, newly formed oligodendrocytes, microglia, endothelial cells, and inhibitory neurons.
To identify cell types involved in traumatic brain injury (TBI), we conducted a systematic data analysis by integrating scRNA-seq and bulk RNA-seq data. The bulk RNA-seq data was collected from a previous study [19]. Specifically, differential expression analysis of mouse cortex RNA-seq data revealed 2977 upregulated and 1726 downregulated genes in TBI as compared with controls (TBI (n = 3) vs. control (n = 3), adjusted P-value < 0.05 and log2 (fold change) > 0.5). Gene set overrepresentation enrichment analysis (ORA) of the upregulated and downregulated genes against the cell-type specific marker genes by scRNA-seq data revealed that the upregulated genes were highly enriched in microglia and endothelial cells, while the downregulated genes were enriched in excitatory neurons (Figure 2A, FDR < 0.05). Consistently, gene set enrichment analysis (GSEA) further confirmed that these cell types were either highly activated or inactivated in TBI (Figures 2B-2H, FDR < 0.05). These results indicated that the systematic analysis of scRNA-seq and bulk RNA-seq data could accurately detect various cell types involved in TBI.
To predict cell-cell communications between those activated cell types, we linked those cell populations by ligand-receptor interactions. We first identified those upregulated genes encoding ligands or receptors in each cell type, and then mapped the receptor-ligand pairs according to the curated ligand-receptor interactions from an earlier study [18]. As shown in Figure 3A, autocrine ligand-receptor interaction was observed in microglia and endothelial cell (Endo-1) via Icam1-Itgam and Col4a1/2-Cd93 pairs. Moreover, microglia could also communicate with endothelial cells via Icam1-Il2rg and C1qa-Cd93.
Furthermore, functional enrichment analysis of the upregulated marker genes in microglia revealed that autocrine ligand-receptor interaction in microglia via Icam1-Itgam might result in activation of TYROBP causal network (Figure 3B, FDR < 0.05, C1qc, Tyrobp, Plek, Apbb1ip, Itgam, Hcls1, Cd84, Ncf2 and Pycard). The intercellular communication between microglia and endothelial cells via C1qa-Cd93 might activate the integrin signaling (Figure 3B, FDR < 0.05, Fn1, Vwf and Tln1).
As chronically activated microglia could release pro-inflammatory molecules, resulting in further tissue damage and contributing potentially to neurodegeneration in TBI, we investigated whether the microglia activation could be attenuated by inhibiting TYROBP causal network, especially through targeting the key regulator, Tyrobp, in this network. Fortunately, we found a gene expression dataset of three wild type and three Tyrobp/Dap12-deficient mouse microglia. As expected, downregulated genes in Tyrobp/Dap12-deficient microglia were highly enriched in microglia activation (Figure 4A). Specifically, 42 genes involved in microglia activation were upregulated in TBI, but were downregulated in Tyrobp-deficient microglia as compared with the controls (Figure 4B). To further confirm the upregulation of Tyrobp in TBI, we collected two gene expression datasets from TBI-affected and control animal models. Consistently, Tyrobp was confirmed to be upregulated in TBI-affected samples of the two datasets (Figure S1). These results indicated that Tyrobp deficiency could significantly reduce the microglia activity, and Tyrobp might be a candidate therapeutic target for TBI so as to inhibit pro-inflammatory molecules and reduce tissue damage.
As the excitatory neurons were inactivated in TBI, we then investigated the cell-cell communications between those inactivated cell types. In accordance with the communications between activated cell types, the autocrine ligand-receptor pairs were also observed in type-3 excitatory neurons (Excitatory-3, Figure 5A, FDR < 0.05). Furthermore, functional enrichment analysis of those downregulated marker genes in excitatory neurons revealed that terms including PDZs pathway, neurexins and neuroligins, disruption of postsynaptic signaling by CNV, protein-protein interaction at synapses, and neuron system were significantly downregulated in Excitatory-3 (Figure 5B). Particularly, the receptor Nrxn3 was involved in these pathways (Figure 5C), suggesting that downregulation of these ligands and receptors might cause dysfunction of nervous system-related pathways, thereby impairing the normal brain function after TBI.
In this study, we aimed to identify key cell types and regulators involved in traumatic brain injury. Specifically, we have successfully identified and characterized 13 cell populations, including astrocytes, oligodendrocyte, newly formed oligodendrocytes, microglia, two types of endothelial cells, five types of excitatory and two types of inhibitory neurons (Figure 1A). The cell-type specific expression of marker genes, including Slc17a7, Gja1, Aspa, Bmp4, C1qa, Flt1 and Gad2, which were found respectively in excitatory neurons [20], astrocytes [21], oligodendrocyte [22], newly formed oligodendrocytes [23], microglia [24], endothelial cells [25], and inhibitory neurons [26], confirmed the validity of proposed characterization of cell types.
Differential expression analysis and GSEA revealed that marker genes of microglia and endothelial cells were among the upregulated genes, while those of excitatory neurons were among the downregulated genes in TBI. Microglia activation has been observed in both animal models [27] and TBI patients [28], and can persist for several years [29]. When TBI occurs, the microglia can migrate into the sites of injury, and establish a protective environment mitigating the damage of TBI [30]. However, long-term activation of microglia can release pro-inflammatory cytokines and cause tissue damage [31]. These results indicated that microglia activation in TBI may mitigate the damage of TBI in the short term, but may cause tissue damage by releasing pro-inflammatory cytokines in the long term.
The cell-cell communication analysis revealed that microglia and endothelial cell (Endo-1) might interact via Icam1-Il2rg and C1qa-Cd93, and microglia might also communicate with each other via Icam1-Itagm. Particularly, autocrine ligand-receptor interaction in microglia via Icam1-Itgam might result in activation of TYROBP causal network (Figure 3B, FDR < 0.05, C1qc, Tyrobp, Plek, Apbb1ip, Itgam, Hcls1, Cd84, Ncf2 and Pycard). The receptor ITGAM is required, along with TYROBP, in microglia to control the pro-inflammatory cytokines [32]. Furthermore, as a ligand of C1q, C1QA has been widely reported to be bounded to CD93 in endothelial cells [33,34]. CD93 could promote β1 integrin activation during tumor angiogenesis [35], indicating that the ligand-receptor interaction between C1QA-CD93 could promote integrin signaling activation in endothelial cells. As chronically activated microglia could release pro-inflammatory molecules [31], resulting in further tissue damage and potentially contributing to neurodegeneration in TBI, we further investigated those downregulated genes in Tyrobp/Dap12-deficient microglia, and found that those genes were highly enriched in microglia activation, suggesting the upregulation of Tyrobp and TYROBP causal network in microglia might be a candidate therapeutic target in TBI [36,37]. In contrast, the excitatory neurons might help maintain the normal brain function [38], and their inactivation might cause dysfunction of nervous system in TBI patients.
In conclusion, the present study has identified key cell types such as microglia, endothelial cells and excitatory neurons, and revealed key regulator in TBI such as TYROBP, C1QA and CD93, which shall improve our understanding of the pathogenesis of TBI.
This work was supported by The National Natural Science Foundation of China (No. 81871458), Talents Training Program of Pudong Hospital affiliated to Fudan University (Project no. PX202003), and the main subject of the discipline construction of the Pudong New Area Health and Family Planning Commission (PWZzk2017-16).
The authors declare that they have no conflicts of interest.
[1] |
Amenedo JLR, Arnalte S, Burgos JC, et al. (2002) Automatic generation control of a wind farm with variable speed wind turbines. IEEE T Energy Conver 17: 279–284. doi: 10.1109/TEC.2002.1009481
![]() |
[2] | Senjyu T, Hayashi D, Sakamoto Y, et al. (2005) Generating power leveling of renewable energy for small power system in isolated island. IEE Japan 125: 1209–1215. |
[3] | Senjyu T, Tokudome M, Yona A, et al. (2009) A Frequency Control Approach by Decentralized Controllable Loads in Small Power Systems. IEE Japan 129: 1074–1080. |
[4] | Senjyu T, Kikunaga T, Tokudome M, et al. (2009) Coordinate Control ofWind Turbine and Battery in Wind Turbine Generator System. IEE Japan 129: 653–660. |
[5] | Kurohane K, Senjyu T, Yona A, et al. (2010) A High Quality Power Supply System with DC Smart Grid. 2010 IEEE PES Transmission and Distribution Conference and Exposition. |
[6] | Ogimi K, Kamiyama S, Palmer M, et al. (2013) Optimal OperationPlanning ofWind Farm Installed BESS Using Wind Power Forecast Data of Wind Turbine Generators Considering Forecast Error. Int J Emerg Electric Power Syst 14: 207–218. |
[7] | Bracale A, Carpinelli G, Fazio AD, et al. (2015) Advanced, Cost-Based Indices for Forecasting the Generation of Photovoltaic Power. Int J Emerg Electric Power Syst 16: 195–206. |
[8] | Shimoji T, Tahara H, Matayoshi H, et al. (2015) Comparison and Validation of Operational Cost in Smart Houses with the Introduction of a Heat Pump or a Gas Engine. Int J Emerg Electric Power Syst 16: 59–74. |
[9] | MengyanW, HigaW, Yona A, et al. (2012) Optimal Operation of Power Systems with Power Players. 2012 International Conference on Renewable Energy Research and Applications (ICRERA). |
[10] | Higa S, Mengyan W, Yona A, et al. (2013) Optimal Operation Method Considering Uncertainly of Renewable Energy and Load Demand in Micro-grids. The 5th International Conference on Advanced Power System Automation and Protection. |
[11] | Higa S, Howlader AM, Shiroma Y, et al. (2014) Optimal Operation Method Considering Replanning and Uncertainly in Power Systems. The International Conference on Electrical Engineering 2014. |
[12] |
Zheng Y, Dong ZY, Luo FJ, et al. (2014) Optimal Allocation of Energy Storage System for Risk Mitigation of DISCOs With High Renewable Penetrations. IEEE T Power Syst 29: 212–220. doi: 10.1109/TPWRS.2013.2278850
![]() |
[13] |
Zhang L, Li Y (2013) Optimal Energy Management of Wind-Battery Hybrid Power System With Two-Scale Dynamic Programming. IEEE T Sust Energy 4: 765–773. doi: 10.1109/TSTE.2013.2246875
![]() |
[14] |
Ahn SJ, Nam SR, Choi JH, et al. (2013) Power Scheduling of Distributed Generators Economic and Stable Operation of a Microtrid. IEEE T Smart Grid 4: 398–405. doi: 10.1109/TSG.2012.2233773
![]() |
[15] |
Zhao B, Zhang X, Chen J, et al. (2013) Operation Optimization of Standalone Microgrids Considering Lifetime Characteristics of Battery Energy StorageSystem. IEEE T Sust Energy 4: 934–943. doi: 10.1109/TSTE.2013.2248400
![]() |
[16] | He D, Tan Z, Harley RG, et al. (2012) Chance Constrained Unit Commitment With Wind Generation and Superconducting Magnetic Energy Storages. 2012 IEEE Power and Energy Society General Meeting. |
[17] | Reddy SS, Abhyankar AR, Bijwe PR, et al. (2014) Co-optimization of Energy and Demand-Side Reserves in Day-Ahead Electricity Markets. Int J Emerg Electric Power Syst 15: 77–91. |
[18] | Nguyen MY, Nguyen DM (2015) A Generalized Formulation of Demand Response under Market Environments. Int J Emerg Electric Power Syst 16: 217–224. |
[19] |
Aalami HA, Moghaddam MP, Yousefi GR, et al. (2010) Demand response modeling considering Interruptible/Curtailable loads and capacity market programs. Appl Energy 87: 243–250. doi: 10.1016/j.apenergy.2009.05.041
![]() |
[20] | Andebili MR, Shen H (2017) Energy Management of End Users Modeling their Reaction from a GENCOs Point of View. 2017 International Conference on Computing, Networking and Communications (ICNC). |
[21] |
Andebili MR (2016) Modeling nonlinear incentive-based and price-based demand response programs and implementing on real power markets. Electr Pow Syst Res 132: 115–124. doi: 10.1016/j.epsr.2015.11.006
![]() |
[22] | Andebili MR, Shen H (2017) Price-Controlled Energy Management of Smart Homes for Maximizing Profit of a GENCO. IEEE T Syst Man Cy A. |
[23] |
Andebili MR (2015) Risk-Cost Based Generation Scheduling Smartly Mixed with Reliability and Market-Driven Demand Response Measures. Int T Electr Energy Syst 25: 994–1007. doi: 10.1002/etep.1884
![]() |
[24] | AndebiliMR(2016) Nonlinear demand response programs for residential customers with nonlinear behavioral models. Energ Buildings 119: 352–362. |
[25] |
Andebili MR (2013) Investigating effects of responsive loads models on unit commitment collaborated with demand-side resources. IET Gener Transm D 7: 420–430. doi: 10.1049/iet-gtd.2012.0552
![]() |
[26] | Japan Electric Power Exchange, JEPX, 2017. Available from: http://www.jepx.org/index.html. |
[27] |
Nguyen MY, Yoon YT (2014) Optimal Scheduling and Operation of Battery/Wind Generation System in Response to Real-time Market Prices. IEE T Electr Electr Eng 9: 129–135. doi: 10.1002/tee.21947
![]() |
[28] |
Havel P (2014) Utilization of Real-Time Balancing Market for Transmission System Control Under Uncertainty. IEEE T Power Syst 29: 450–457. doi: 10.1109/TPWRS.2012.2228676
![]() |
[29] | Kiviluoma J, O'Malley M, Tuohy A, et al. (2011) Impact ofWind Power on the Unit Commitment, Operating Reserves, and Market Design. 2011 IEEE Power and Energy Society General Meeting. |
[30] | Vlachos AG, Biskas PN, et al. (2011) Balancing Supply and Demand Under Mixed Pricing Rules in Multi-Area Electricity Markets. IEEE T Power Syst 26: 1444–1453. |
[31] | Ma J, Silva V, Belhomme R, et al. (2013) Evaluating and Planning Flexibility in Sustainable Power Systems. 2013 IEEE Power and Energy Society General Meeting (PES). |
1. | Muyu Situ, Ali Francisco Citalan-Madrid, Svetlana M. Stamatovic, Richard F. Keep, Anuska V. Andjelkovic, Transcriptomic Profile of Blood–Brain Barrier Remodeling in Cerebral Amyloid Angiopathy, 2022, 16, 1662-5102, 10.3389/fncel.2022.931247 | |
2. | Rui-zhe Zheng, Kuin-yu Lee, Zeng-xin Qi, Zhe Wang, Ze-yu Xu, Xue-hai Wu, Ying Mao, Neuroinflammation Following Traumatic Brain Injury: Take It Seriously or Not, 2022, 13, 1664-3224, 10.3389/fimmu.2022.855701 | |
3. | Oandy Naranjo, Olivia M Osborne, Silvia Torices, Sarah Schmidlin, Destiny Tiburcio, Minseon Park, Michal Toborek, Microvessel isolation protocol for RNA visualization and profiling, 2024, 14, 2045-2322, 10.1038/s41598-024-77501-8 | |
4. | Lindsay D. Strehle, Lauren D. Otto‐Dobos, Corena V. Grant, Erica R. Glasper, Leah M. Pyter, Microglia contribute to mammary tumor‐induced neuroinflammation in a female mouse model, 2024, 38, 0892-6638, 10.1096/fj.202301580RR | |
5. | John Leonard, Xiaoran Wei, Jack Browning, Erwin Kristobal Gudenschwager-Basso, Jiangtao Li, Elizabeth A. Harris, Michelle L. Olsen, Michelle H. Theus, Transcriptomic alterations in cortical astrocytes following the development of post-traumatic epilepsy, 2024, 14, 2045-2322, 10.1038/s41598-024-58904-z | |
6. | Ian G. Gober, Ashley L. Russell, Tyler J. Shick, Vincent A. Vagni, Jenna C. Carlson, Patrick M. Kochanek, Amy K. Wagner, Exploratory assessment of the effect of systemic administration of soluble glycoprotein 130 on cognitive performance and chemokine levels in a mouse model of experimental traumatic brain injury, 2024, 21, 1742-2094, 10.1186/s12974-024-03129-0 | |
7. | Ruchira M. Jha, Dhivyaa Rajasundaram, Chaim Sneiderman, Brent T. Schlegel, Casey O’Brien, Zujian Xiong, Keri Janesko-Feldman, Ria Trivedi, Vincent Vagni, Benjamin E. Zusman, Joshua S. Catapano, Adam Eberle, Shashvat M. Desai, Ashutosh P. Jadhav, Sandra Mihaljevic, Margaux Miller, Sudhanshu Raikwar, Anupama Rani, Jarrod Rulney, Shima Shahjouie, Itay Raphael, Aditya Kumar, Chia-Ling Phuah, Ethan A. Winkler, Dennis W. Simon, Patrick M. Kochanek, Gary Kohanbash, A single-cell atlas deconstructs heterogeneity across multiple models in murine traumatic brain injury and identifies novel cell-specific targets, 2024, 112, 08966273, 3069, 10.1016/j.neuron.2024.06.021 | |
8. | Mi Zhang, Yuhao Duan, Hui Gan, Ning Jiang, Le Qin, Yujia Luo, Ailiyaer Palahati, Yaying He, Chenyang Li, Xuan Zhai, TYROBP serve as potential immune-related signature genes in the acute phase of intracerebral hemorrhage, 2024, 14, 2045-2322, 10.1038/s41598-024-71132-9 | |
9. | Kaiping Bai, Yanxi Long, Fei Yuan, Xiaoling Huang, Pengtao Liu, Yanping Hou, Xiangyu Zou, Tao Jiang, Jie Sun, Hedyotis diffusa injection modulates the ferroptosis in bladder cancer via CAV1/JUN/VEGFA, 2025, 147, 15675769, 113925, 10.1016/j.intimp.2024.113925 | |
10. | Peng Zou, Tianjing Li, Zixuan Cao, Erwan Yang, Mingdong Bao, Haofuzi Zhang, Zhuoyuan Zhang, Dan Liu, Min Zhang, Xiangyu Gao, Junmiao Ge, Xiaofan Jiang, Zhicheng Tian, Peng Luo, High-altitude hypoxia aggravated neurological deficits in mice induced by traumatic brain injury via BACH1 mediating astrocytic ferroptosis, 2025, 11, 2058-7716, 10.1038/s41420-025-02337-8 | |
11. | Liping Shi, Shuyi Liu, Jialing Chen, Hong Wang, Zhengbo Wang, Microglial polarization pathways and therapeutic drugs targeting activated microglia in traumatic brain injury, 2026, 21, 1673-5374, 39, 10.4103/NRR.NRR-D-24-00810 |