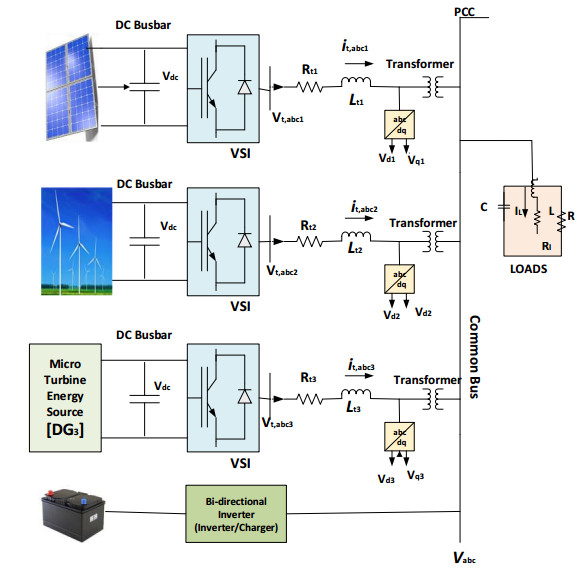
The advancement in communication technology and the availability of intelligent electronic devices (IEDs) have impacted positively on the penetration of renewable energy sources (RES) into the main electricity grid. High penetration of RES also come along with greater demand for more effective control approaches, congestion management techniques, and microgrids optimal dispatch. Most of the secondary control methods of microgrid systems in the autonomous mode require communication links between the distributed generators (DGs) for sharing power information and data for control purposes. This article gives ample review on the communication induced impairments in islanded microgrids. In the review, attention is given to communication induced delay, data packet loss, and cyber-attack that degrades optimal operations of islanded microgrids. The review also considered impairments modelling, the impact of impairments on microgrids operation and management, and the control methods employed in mitigating some of their negative impacts. The paper revealed that innovative control solutions for impairment mitigation rather than the development of new high-speed communication infrastructure should be implemented for microgrid control. It was also pointed out that a sparse communication graph is the basis for communication topology design for distributed secondary control in the microgrid.
Citation: Olayanju Sunday Akinwale, Dahunsi Folasade Mojisola, Ponnle Akinlolu Adediran. Mitigation strategies for communication networks induced impairments in autonomous microgrids control: A review[J]. AIMS Electronics and Electrical Engineering, 2021, 5(4): 342-375. doi: 10.3934/electreng.2021018
[1] | Cherechi Ndukwe, M. Tariq Iqbal, Xiaodong Liang, Jahangir Khan, Lawrence Aghenta . LoRa-based communication system for data transfer in microgrids. AIMS Electronics and Electrical Engineering, 2020, 4(3): 303-325. doi: 10.3934/ElectrEng.2020.3.303 |
[2] | Desh Deepak Sharma, Ramesh C Bansal . LSTM-SAC reinforcement learning based resilient energy trading for networked microgrid system. AIMS Electronics and Electrical Engineering, 2025, 9(2): 165-191. doi: 10.3934/electreng.2025009 |
[3] | Shahzad Ashraf, Arshad Ahmad, Adnan Yahya, Tauqeer Ahmed . Underwater routing protocols: Analysis of link selection challenges. AIMS Electronics and Electrical Engineering, 2020, 4(3): 234-248. doi: 10.3934/ElectrEng.2020.3.234 |
[4] | Dipak R. Swain, Sunita S. Biswal, Pravat Kumar Rout, P. K. Ray, R. K. Jena . Optimal fractional sliding mode control for the frequency stability of a hybrid industrial microgrid. AIMS Electronics and Electrical Engineering, 2023, 7(1): 14-37. doi: 10.3934/electreng.2023002 |
[5] | Yipeng Yang . Stability analysis of networked control systems with bounded random delay and state compensation: How large is the actual system scale?. AIMS Electronics and Electrical Engineering, 2019, 3(1): 16-32. doi: 10.3934/ElectrEng.2019.1.16 |
[6] | Lidong Wang, Cheryl Ann Alexander . Cyber security during the COVID-19 pandemic. AIMS Electronics and Electrical Engineering, 2021, 5(2): 146-157. doi: 10.3934/electreng.2021008 |
[7] | Muamer M. Shebani, M. Tariq Iqbal, John E. Quaicoe . Comparison between alternative droop control strategy, modified droop method and control algorithm technique for parallel-connected converters. AIMS Electronics and Electrical Engineering, 2021, 5(1): 1-23. doi: 10.3934/electreng.2021001 |
[8] | Yanming Wu, Zelun Wang, Guanglei Meng, Jinguo Liu . Neural networks-based event-triggered consensus control for nonlinear multiagent systems with communication link faults and DoS attacks. AIMS Electronics and Electrical Engineering, 2024, 8(3): 332-349. doi: 10.3934/electreng.2024015 |
[9] | Dharmesh Faquir, Nestoras Chouliaras, Vlachou Sofia, Kalopoulou Olga, Leandros Maglaras . Cybersecurity in smart grids, challenges and solutions. AIMS Electronics and Electrical Engineering, 2021, 5(1): 24-37. doi: 10.3934/electreng.2021002 |
[10] | Efthymios N. Lallas . A survey on key roles of optical switching and labeling technologies on big data traffic of Data Centers and HPC environments. AIMS Electronics and Electrical Engineering, 2019, 3(3): 233-256. doi: 10.3934/ElectrEng.2019.3.233 |
The advancement in communication technology and the availability of intelligent electronic devices (IEDs) have impacted positively on the penetration of renewable energy sources (RES) into the main electricity grid. High penetration of RES also come along with greater demand for more effective control approaches, congestion management techniques, and microgrids optimal dispatch. Most of the secondary control methods of microgrid systems in the autonomous mode require communication links between the distributed generators (DGs) for sharing power information and data for control purposes. This article gives ample review on the communication induced impairments in islanded microgrids. In the review, attention is given to communication induced delay, data packet loss, and cyber-attack that degrades optimal operations of islanded microgrids. The review also considered impairments modelling, the impact of impairments on microgrids operation and management, and the control methods employed in mitigating some of their negative impacts. The paper revealed that innovative control solutions for impairment mitigation rather than the development of new high-speed communication infrastructure should be implemented for microgrid control. It was also pointed out that a sparse communication graph is the basis for communication topology design for distributed secondary control in the microgrid.
The increasing penetration of renewable energy sources (RES) into the main electricity grid is a profitable development. With the high penetration of RES, dependence on fossil fuel-based energy sources is reduced significantly. Thus, the concern for the impacts of greenhouse gas emission on the environment could be reduced by the large scale implementation of RES-based microgrids [1,2,3,4]. A microgrid (MG) which is a fraction of the main electric grid, is a small electrical network that can independently generate electricity when it is disconnected from the main electricity grid owing to faults, disturbance of the power flow of the main grid or when the main grid is non-existent [5,6,7,8]. Two modes of operations are possible with microgrids; the grid-connected or autonomous mode of operation. When operating in the grid-connected mode, the microgrid is directly connected to and is controlled by the main grid. While in autonomous or islanded mode, the frequency and voltage of the microgrid are controlled locally and independent of the main grid. Shown in Figure 1 are three DGs of an islanded system of microgrids with heterogeneous RES: the solar PV system, the wind generator and the microturbine. The RES serves as the prime mover for the microgrid system, without which there will be no output from the microgrid. A three-phase voltage source inverter (VSI) is connected to change the energy generated to its AC equivalent. The output of the VSI is connected to the microgrid system's main bus via a line impedance and a transformer. The energy storage system serves to store generated energy for later use. The load to be powered is connected to the main bus of the microgrid.
A microgrid has one or more DG sources which could be non-renewable energy-based or renewable energy-based. Common renewable energy sources are solar photovoltaic systems, small hydro turbines, wind turbines, and fuel cells etc. Unlike synchronous generator rich in inertial, RES-based DG microgrids have very low inertial and random output values. Therefore, microgrid uses intelligent electronic devices (IEDs) and communication networks for its smooth operation. Although, some methods, such as the droop-based techniques, used control methods that are not dependent on the communication network. Power converters and electronic control units are essential for the interconnected DG systems for appropriate working. Communication networks are indispensable for the exchange of information in the MG. System operation and control information upon which the power inverters' smooth operation must be shared via the installed communication networks [9].
Distributed systems in the microgrid are interconnected with communication networks. The electrical energy produced flows along the microgrid, while energy management data, billing and control data are transmitted through the communications networks which could be wireline or wireless [10,11,12]. The use of communications networks for information sharing gives rise to delays in the delivery of system operation information and power control information or even a complete loss of vital information during the signal transmission. Signal interference is likewise a common problem because communication channel is shared with other users. Some previous work considered information delay a constant value to simplify the design. In other studies, the communication delay is regarded as negligible. It is vital to give attention to communications delays and packet loss during transmission while designing a load sharing control system for the DGs to have appropriate and synchronized output values from all the inverters [13,14]. Most communication networks are prone to delay. Wireless communication networks such as ZigBee (802.15.4), Wi-Fi (802.11), and WiMAX (802.16) proposed for microgrid communication experience time-varying delay. The observations mentioned above make the study of time-varying communication delay very crucial for power inverters control in MG.
Several methods have been proposed to mitigate communication network-induced impairments in microgrids. In [15], an investigation on the effects of wireless communication on MG control was carried out. A constant delay was maintained for all transfer routes using a unified Smith predictor approach. The approach was unsuccessful in mitigating the time-varying delay, which generally results when different routes are involved. Y. Xu et al. [16] studied smart grid wireless network, communication delay analysis was carried out. Results provided from the work set-theoretical delay bounds that could be employed in designing wireless networks for smart grids. Results also suggest the applicability of wireless mesh networks for microgrids. However, the possibility of communication failure when packet loss occurs was not considered. An optimal design scheme was proposed by [17] for directed network topology. A small signal dynamic model of an MG was derived to investigate the effects of delay on MG stability. Real-time hardware-in loop simulations were used to verify the proposed method's effectiveness.
In [18] master-slave communication model between generating sources in the MG was used. Lyapunov-Krasovskii was employed in the calculation of allowable delay that ensures system stability. The system was modelled as a time delay system that uses wireless communication between inverters in the MG. Although master-slave depicts good performance in load sharing among inverters, it suffers from a single point of failure. If the master cluster fails to function, the whole system will collapse. G. Chen et al. [19] proposed Lyapunov-Krasovskii functions for the analysis of the stability of a distributed secondary control of an MG under communication delays. The control protocol is implemented using a sparse communication network. The performance of the method was not tested under varying load characteristics. G. Lou et al. [14] worked on a distributed cooperative scheme to achieve frequency synchronization from different distributed generations in the MG. Time-varying communication delays were taken into consideration in the design. Delay boundary that ensures stable system operation of the system was presented. However, signal failure was not accounted for in this design.
A series of research conducted by [20,21,22] on MG control use different approaches for DC and AC MG control. Distributed adaptive droop control and distributed cooperative control of DC MG was investigated. Also, a droop-free distributed control for AC MGs was investigated. In all these works, neighbouring inverters share control information using wireless communication. A sparse communication graph that connected the inverters in the MG was used. The ability of the methods to handle disturbances originating from communication networks was presented. However, delay caused by communication network was treated as a constant value. Q. Shafiee et al. [23] defined packet loss and packet latency as important communication network parameters to analyze control effects in microgrids. In this article, the review of communication induced impairments in microgrids and how they are modelled is carried out. The impacts of communication impairments on microgrids operation, the mitigation methods employed for communication impairments reduction and findings from the work are analyzed.
Secondary control in microgrids requires communication networks for smooth operation [24]. The impairments induced by the use of communication networks in secondary control of microgrids should be mitigated. Efforts to achieve optimum secondary control have been reviewed, some of the related review articles are presented in this section. In [13], the impact of communication failure on distributed control methods in AC microgrids was the review's focus. A comparison of methods used to reduce communication latency, data packet dropout, and channel noise on distributed controllers was presented. Also discussed is the distinctiveness of distributed control systems and their challenges. An analysis of the influence of wireline and wireless communications on microgrid performance was carried out in [25], the review aimed at determining the network requirements for a successful operation of microgrids. The review in [26] is focused on hierarchical control in microgrids. The discussion is on the optimum active and reactive power-sharing, and eliminating the effects of communication delays using hierarchical control of microgrids. The review article by [27] highlights the control strategies and techniques used to stabilise DC microgrids. Stability analysis tools for smooth system operation was also presented. A survey of primary controllers used for distributed generation synchronization was carried out by [28], the control scheme for centralized architecture and master/slave control technique was presented. To avoid communication link challenges, droop control was intended for use where the system's stability is considered critical. The review however does not include secondary control methods. The review's focus carried out by [29,30] is to describe various techniques employed for autonomous AC microgrid control. Attention was given to the level of communication required by the secondary control methods discussed. [31,32] reviewed control methods used in DC microgrids, while [32] carried out a general review of the methods, [31] presented a review describing hierarchical control issues such as power-sharing, voltage regulation and stabilization methods. [33] review operation of smart inverters with a specific interest in plug and play, self-awareness, cooperativeness and adaptability of the inverters as reasons for categorizing them as smart. The work also discusses the possibility of inverters communicating over a long-range to empower various control schemes. The survey in [34] discussed the cyber security of smart microgrids. Attacks on data integrity, availability and confidentiality were discussed. Also reviewed are the economic impacts of cyber-attacks on microgrids installations and the detection and defensive strategies employed against false data injection attacks. Adaptive protection of microgrids was the focus of the review in [35]. It was pointed out that the reliability of the communication network and protection against cyber-attacks remains a big challenge in the implementation of reliable schemes based on adaptive protection.
In all these review articles, no attention was given to how communication impairments are modelled, and none has considered cyber-attacks on microgrids installations as impairment induced because of the increasing use of wireless communication networks.
From the viewpoint of the previously mentioned research gaps, this review investigates communication induced impairments in autonomous microgrids. The study contributions are described as follows:
(1) Impairment models and mitigation approaches were described for data packet dropouts and communication network induced delay in autonomous microgrids.
(2) Cyber-attacks resulting from using communication networks in the microgrid network, especially wireless communications, were discussed.
(3) The review presented and discussed cyber-attack detection techniques, impacts of attacks on system operations, and attack mitigation techniques.
The paper is arranged thus: Section Ⅰ presents the introduction to the review, related reviews are presented in section Ⅱ. Communication technologies employed in microgrid operations were discussed in section Ⅲ, while the focus of section Ⅳ is the specific communication induced impairments studied in the literature, their impacts on microgrid operations and how they are modelled for further investigation of their nature. Section Ⅴ deals with mitigation approaches and control methods used in islanded microgrid control. Ⅵ and Ⅶ discuss mitigation approaches and control methods used in islanded microgrids control. In section Ⅷ, a conclusion to the review was drawn.
For wireless technologies, the advantages of lower installation cost, suitability for deployment in rural areas and flexibility future expansion make its applications more desirable than their wired counterparts. Wired communication technologies can give higher bandwidth, and are more reliable, but the installation cost is high, and they are not as flexible when there is a need for expansion [25]. Suitable wired communication technologies for microgrids applications include power-line communication (PLC), Ethernet and Fibre Optics, while Bluetooth, Wi-Fi, ZigBee, Cellular, LoRa, and WiMax are commonly used wireless communication technologies.
All wired transmission media have a physical connection from the signal source to the sink where the transmitted signal is received. Power Line Communication (PLC) technology enables the transmission of data over existing power cables. This implies that, with this technology, power cables that initially can only carry power to devices can now simultaneously control the device and even retrieve data from it. In Narrow Band Power Line Communication (NB-PLC) technology, data transmission is achieved through a narrow frequency band and at a low bit rate. The technologies emanating from NB-PLC had the focus on enhancing smart grid applications and building automation. A data transfer speed of between 10-500kbps is achievable with this technology. The Broad Band Power Line Communication (BB-PLC) can operate at a higher speed, reaching hundreds of Mbps. It was designed to have the capability for applications that require internet access [36].
Ethernet communication provides the means of connecting various electronic devices and computers in a physical network often referred to as the local area network (LAN). The idea makes efficient sharing of data, files, and information possible between computers and electronic devices. The most common deployment of Ethernet cables is connecting a Wi-Fi modem or router to the entry port of the internet. Ethernet connections are faster and more reliable than its wireless counterpart. Fibre optics technology involves using thin strands of glass drawn carefully to a diameter similar to that of human hair. Bundles of the glass strands are put together in optical cables. Data transmission is by light. At the source, the data information is used to encode the light signals which is effectively decoded to retrieve the data at the receiving end. The optical fibre is essentially a transmission medium.
Bluetooth technology is widespread for wireless voice and data communications within a short range. Bluetooth devices employ short-range radio waves for pairing with devices within range. Each Bluetooth device is equipped with a tiny computer chip that broadcast its signal to detect and connect. Wi-Fi technology is comparable to Bluetooth since it also utilizes radio waves for conveying data at a high-speed over short ranges wirelessly. By breaking the entire signal into small units, transmitting the pieces over multiple radio frequencies. Wi-Fi can achieve transmission at lower power per individual frequencies. This technique also makes possible the use of multiple devices on the same Wi-Fi transmitter. Another technology for the connection of smart devices over the wireless link is the ZigBee, which was built upon the IEEE 802.15.4 standard. The technology promises to be cost-effective and energy efficient compared to Bluetooth and Wi-Fi technologies. Unlike Bluetooth, which operate on point-to-point communication, ZigBee operates in a mesh network. Its connections are spread among the nodes of the wireless network. The nodes are capable of communicating with one another and sharing networks over a large area [25]. The operation of WiMAX is close to Wi-Fi except that the speed of operation is higher and the range and numbers of users are much larger. LoRa (Long Range), a wireless technology, combines two desirable characteristics; ultra-low power consumption and effective long-range. Long Range Wide Area Network (LoRaWAN) represents the communication protocol and system architecture for LoRa network. However, the range is dependent on the topology of the environment. A typical LoRa operates within a range of 13 Km and 15 Km. Lora radios are equipped with chirp spread spectrum (CSS) modulation method to realize an advantageous long range of communication while sustaining a low power consumption capability.
Global System for Mobile Communications (GSM) come with a combination of Time Division Multiple Access (TDMA) and Frequency Division Multiple Access (FDMA). In FDMA GSM, a 25 MHz frequency band is divided into 124 carrier frequencies with a 200 KHz space between each carrier. The same frequency channel is allotted to different subscribers; this is done by dividing the frequency band into multiple time slots. This arrangement makes sharing the same transmission space by multiple stations possible. While GSM is still in use, upgrades of the basic system are already available in Universal Mobile Telecommunications System (UTMS), the Long Term Evolution (LTE) and the emerging 5G networks wireless standards.
Table 1 compares microgrids communication technologies, giving attention to coverage, data rate, frequency and specific usage of the technologies considered in microgrids applications with given references.
Technology | Coverage [m] | Data Rate | Frequency | Applications in Microgrids | ||
Wired | PLC | NB | 150,000 | 10-500 Kbps | 1.8-250 MHz | Data communication for freq. control Islanding control in DC MGs. [43], [44] |
Ethernet | IEEE 802.3 | 100-300 | 10 Mbps | 2.4 GHz | Information communication between DGs. [37], Monitoring and control of energy meters [38] |
|
Fibre Optics | PON | 10,000-60,000 | 100 Mbps-2.5Gbps | 3 x 1011 - 4 x 1014 Hz | Metering communication [39] | |
Wireless | Bluetooth | IEEE 802.15 | 15-30 | 1-2 Mb/s | 2.4 GHz | Data communication [40] |
Wi-Fi | IEEE 802.11 | 100 | 54 Mb/s | 2.4/5.9 GHz | Information sensing and data communication [41] | |
ZigBee/6LoWPAN | IEEE 802.15.4 | 30-50 | 250 Kbps | 868 MHz, 915 MHz, 2.4 GHz | Data communications. [42] | |
Cellular | GSM | 1000-10,000 | 14.4 Kbps | 0.9, 1.8, 2.1, 2.4 GHz | Data communications. [40] | |
WiMAX | 10,000-50,000 | 75 Mb/s | 2-11 GHz | Protection relay communications [45]. Monitoring and control of energy meters [38]. |
||
LPWAN | LoRa | 10,000-15,000 | 0.3-37.5 Kbps | 433/868/915 MHz | Data Transfer. [46] | |
SIGFOX | 30,000-50,000 | 0.1 Kbps | 902–928 MHz | Data Transfer. [47] |
Optical fibre and Ethernet are suitable for primary control purposes in the microgrids. They have the required data rate, which ensures low latency for optimal operation of the primary controllers. They have been applied for information communication between DGs in [37], and monitoring and control of energy meters in [38]. In [39], optical fibre was used for metering communication. PLC may not be suitable for primary control purposes given its low data rate. GSM networks are primarily designed for machine to human communications and operate in the licensed radio frequencies. Operating such networks for microgrid will be expensive and can reduce the gains derived from microgrid installations. Bluetooth, Wi-Fi, and ZigBee/6LoWPAN technologies are appropriate and cost-effective in applying microgrids. They have been applied for information sensing and data communications [40,41,42]. Bluetooth technology, however, is limited in coverage range. While the likes of WiMAX, LoRa and SIGFOX technologies offer a wider coverage range. They are essentially designed for communication over a long distance.
The inclusion of communication networks in the microgrid network can lead to several challenges that must be addressed to avoid degrading the performance of the microgrid operation. In this section, the impacts of communication networks induced impairments are discussed. Communication induced impairments in microgrids mostly investigated in pieces of literature are packet latency or delay, packet loss, noise, jitter and cyberattacks.
A data packet is considered lost if it is not delivered at the expected receiving end after transmission due to communication link failure or expiration of the delay limit while queuing at the point of reception [48]. Time-varying delays naturally result because of the asymmetry of interactions, congestion, bandwidth, and transmission speed. Packet latency or delay can be estimated by the total time taken from the time the last bit is transmitted at the transmitting end, and the time the last bit is received at the targeted receiving end [49,50,51]. Communication noise is another practical impairment that plagues the microgrid operation. It is stochastic and dependent on the characteristics of the medium of communication. Channel noise in communication among several agents was considered in [52], while investigating the impact of communication noise among multi-agent systems using consensus protocol was the focus in [53,54,55]. Cyber-attack is not inherent impairment, but the network is exposed to such attacks that come mainly external to the microgrid system because the wireless communication network is integrated in the system operation.
For microgrid operations and management, data packet losses, information packet delay and cyber-attacks have the most devastating effects and are primarily investigated. The effects of communication network delay and packet loss are inherent in a wireless communication network; they generally reduce the performance of the control algorithm in microgrids. In [24] it was shown that latency affects the speed and stability of the system control. [56] used Smith Predictor based on information of delayed packets. The results show that time-varying delay affects both the amplitude and frequency of the entire system. It also shows that power loss also increased when there is no mitigation of packet latency. In [57], the algorithm for state estimation of microgrids was presented. The least mean square was used for the implementation of the algorithm. In the simulation, predefined values were set for the Gaussian noise and packet loss. The results show that the proposed state estimation converges rapidly without packet loss. In a similar work, [9] made a simulation with four sensing stations sending information in a broadcast fashion and using predefined, time-invariant parameters for the entire system. The results also showed that packet loss increase system instability.
Communication delay was calculated in [42], [58] based on the number of DGs, controller location and wireless network used. The work sought to improve load sharing performance, regulate the frequency and voltage of microgrid containing multiple DGs. Results show that voltages of the system are subjected to substantial oscillations when communication delay is lower than that required for processing by the converter. It also shows that the lower the communication bandwidth, the more significant the delay becomes. Varying communication latencies were tested by [23,59,60], in attempts to regulate voltage, stabilize frequency and share power in islanded microgrids. Close-loop system response tends to oscillate also; results indicate oscillations increases with delays and convergence speed is proportional to communication delay.
Distributed secondary control rely on communication networks for operation. Although many advantages could be derived from the application of distributed systems, it is, however, vulnerable to malicious attacks. In [61], individual DERs were the subjects of cyber-attacks while the link between DERs was targeted in [62] to corrupt data transfer. When false data injection is capable of corrupting data transfer, and as a result, the integrity of the data can be compromised. Using a centralized control structure, [63] proposed detection and mitigation schemes to correct cyber-attacks in DC power systems.
Data packet transmission between two agents or nodes is illustrated in Figure 2. The problem of packet loss is most significant when wireless communication networks such as Wi-Fi, Bluetooth, LoRa, ZigBee and WiMAX are used for data communication. The mathematical model for the description of data packet loss is explained thus: Given two nodes m and n as shown in Figure 2; the information packet Jk arrives at node m, packet waits in the queue at node m after which it is transmitted to node n, via link (m, n). Following the requirement of power inverters for optimal performance in the microgrid system, a delay limit τlimit is set for each packet. A packet arriving outside this τlimit is discarded and considered lost. However, a packet lost during transmission within τlimit will be retransmitted as long as the τlimit is not exceeded until it is successfully delivered or eventually lost due to exceeding τlimit. The total packet loss probability G(m,n)l by packet Jk is dependent on two factors:
1. the probability of packet drop G(m)d due to expiration of delay limit while queueing at node m, and
2. the probability of packet loss G(m)l over the link (m, n), which is a function of packet error probability G(m)e due to signal fading over the link (m, n).
Determining packet loss probability over communication link affected by signal fading requires the knowledge of the packet error probability G(m)e which depends on the specific channel conditions of the link (m, n) and the applied transmission schemes. The packet error probability G(m)e for packet Jk can be modelled by the sigmoid function as [64]:
G(m)e=11+e−ζ(γ−δ) | (1) |
where γ is the channel status information parameters in terms of the detected link SINR, ζ and δ are constants corresponding to the used modulation and coding schemes for a given packet of length L. In a wireless network such as Wi-Fi, the packet collision probability G(m)c due to contention access to the medium is also included in the estimation of the link's packet loss probability. Packet collision probability G(m)c can be determined from:
G(m)c=1−(1−τ)x−1 | (2) |
where τ is the probability that node m transmits in a randomly chosen slot time, and x is the number of contending nodes. Therefore, packet loss probability over the link (m, n) can be written as [15,64]:
G(m)l=1−(1−G(m)e)(1−G(m,n)c) | (3) |
However, Bernoulli distribution was used to represent the packet loss model in [9,65], the probability representation used for packet loss is given in Eq 4 as:
154αik={10withProbabilityofλawithProbabilityof1−λa | (4) |
where λa is the average packet arrival rate at node m. The model shown in Eq 4 is simple yet effective, therein packet loss is measured based on whether the signal is successfully delivered at the receiving point or not.
Packet loss due to expiration of delay limit is affected by the bandwidth, available throughput and transmission rate. If b(m,n) is the bandwidth of link (m, n). Then, the available throughput over the link (m, n) is directly determined by the transmission rate r(m,n)(b(m,n)). The effective transmission rate is given by:
R(m,n)=r(m.n)(b(m,n))1+e−ζ(γ−δ) | (5) |
To derive the packet drop probability, G(m)d due to the packet delay limit expiration during the wait in the queue of node m, it is important to analyze the queueing model of the packet Jk at that node. Let τcrt be the current delay incurred by packet Jk when the packet arrives at node m and enters the queue of that node. The maximum retransmission limit τ(m,n)max for packet Jk over the link (m, n) based on the delayed deadline τlimit can be expressed as [66]:
τ(m,n)max=[R(m,n)i,j(τlimit−τcrt)L] | (6) |
Considering service time Xt(m,n) for packet Jk over the link (m, n), by queuing analysis, the mean time spent waiting for packet Jk at node m can be shown as in [15,64]:
E[q(m,n)i]=λaE(Xt(m,n))22(1−λaE(Xt(m,n))) | (7) |
where λa is the average packet arrival rate at node m.
Research work on microgrid communication that investigates mitigation techniques used to reduce the impacts of packet loss is discussed here. Frequency and voltage restoration in an islanded microgrid is the focus of the research conducted in [23]. Each agent in the network calculates a new average of data after the reception of information from other agents in the network. Bernoulli random process was used to model packet losses to give a probability of 0 or 1 when investigating the impact of data packet dropout on the system. The control method uses the previously measured value at intervals when the information packet is lost to prevent performance degradation. Thus, the distributed secondary control method restored frequency and voltage to normal values for optimum operation of the microgrid. Estimation of packet loss on the microgrid was carried out by [9,65], Bernoulli distribution was used for modelling packet loss. Information data were transmitted over a sparse communication graph as reported in [67,68]; the graph was designed with a minimum redundancy to prevent a breakdown of operation when data packets are not delivered as a result of link breakdown. Results demonstrated the capability of a well-designed sparse communication graph in mitigating link failure. Distributed cooperative control method was used in [48] for microgrid voltage control. Communication link failure causes a time-varying communication graph which could degrade system performance. The DG that lost communication operates in the network based on the droop controller and the latest control command received. Results from work show that the output of the DG with communication link failure is not synchronized with the other DGs in the network. Presented in Table 2 are the description of packet loss and the mitigation approaches employed in pieces of literature.
References | Packet Loss Description | Mitigation Approaches |
[23] | Bernoulli random process was used to model packet losses to produce a probability of 0 or 1 | The control method uses the previously measured value at intervals when the information packet is lost. |
[67], [68] | A single point failure was used to test the redundancy of the sparse com graph used. | Distributed communication topology: The communication graph was designed with minimum redundancy. |
[48] | Communication link failure between DGs in the microgrid network. | The DG that lost communication operates based on the droop controller and the latest control command received. |
[9], [65] | Bernoulli random process | Loss estimation was carried out |
Latency or delay is inherent in the communication systems. In some applications, the communication delay is negligible when the delay is within an acceptable range for the normal operation of that system. A comprehensive link delay includes four components: the processing delay, the time interval from the time packet is received, and the time it is assigned for transmission. The propagation delay occurs between the time the last bit is transmitted and received from the head node and the link's tail node, respectively [49,50,51]. Other components are the queueing delay which signifies the time a packet is transmitted and the actual time reception. Delay as a result of transmission is the time interval between the transmission of the first and last bits of the packet.
The inherent communication delay of the channels of each DG can be measured using the packet round trip time (RTT) method [14]. Suppose τ(t) represent RTT when node i received acknowledgement, for the corresponding link i, let: dp,i(t) be processing delay, dg,i(t) the propagation delay, dq,i(t) stand for the delay due to queueing and dt,i(t) be the transmission delay. Given that the network end-to-end connection has K nodes, then [69]:
τ(t)=∑Ki=1(dp,i(t)+dg,i(t)+dq,i(t)+dt,i(t)) | (8) |
The microgrids communication network connection is expected to be constant or fixed during a session. The propagation and processing delay can be safely treated as a constant value, denoted here as X. Delay dynamics will then be limited to the evolving state of transmission and queueing along the path. Thus, total delay due to packet transmission via link (m, n) when the acknowledgement is received at node n can be calculated using:
τ(t)=∑Ki=1(q(m,n)i(t)+Xt(m,n)+dt,i(t)) | (9) |
where q(m,n)i(t) dynamics of queue length, and it is given by Eq 7.
In [70] voltage regulation was the main focus of the research. Distributed controller, which mimics harmonic voltage sources together with virtual impedance, thus lower voltage distortion in the network. Communication delay was quantified as time addition of time set for point generation and the inverter to receive set point from neighbours. Results from the work indicate that current sharing errors increase with communication delay. The method employed reduced communication congestion and established current sharing performance is highly dependent on delay. To regulate voltage and achieve accurate power-sharing in DC MGs, [71], a secondary control technique handles power-sharing via a distributed strategy, while dc bus voltage restoration was achieved using a decentralized technique. Communication delay was represented by e−τds, transient response damping becomes lower under delay, however, it becomes stable and oscillating around consensus values with the application of the control method. Disturbance-observer based method for frequency regulation in a MG with low inertia was carried out by researchers in [72]. Communication delay was taken to vary randomly from 0.05 to 0.15 sec. Uniform distribution was assumed. The delay led to high-frequency transients in the system frequency. The magnitude of oscillations was significantly reduced using the method proposed.
Using a fixed communication delay of 0.01 to 1 sec [68] investigated the performance of a consensus-based cooperative droop control to achieve enhanced reactive power-sharing, in the technique used, the droop slope gain was adaptively adjusted. Laboratory results show that the system remains stable as the eigenvalues remain in the left half complex plane. Also, simulated power-sharing at a delay of 1 second is moderate. In the research by [73], precise load sharing is affected through the droop control technique. A low-speed communication; control area network (CAN) was used to obtain voltage regulation. A time delay of 0, 20, 60 and 100μs were used to test the impacts of communication delay. Stability analysis revealed that communication delay forces the closed-loop system poles to draw closer to the imaginary axis.
Microgrid installations become prone to cyber-attacks, especially when a wireless communication network is used for information sharing. Such attacks seek to gain unauthorized access to user data to deny intended users access to the network, change consumer data for economic reasons, or disturb the smooth operation of the entire system. Common attacks experienced because of the use of wireless communication networks in MGs are discussed in this section.
This type of malicious attack is carried out to prevent authorized user access to the network. In synchronization floods DOS attack, the attacker transmits spoofed synchronization requests continuously to the IED. This attack can distort the connection between the intended user and the network. In buffer overflow DOS, a malicious code is transmitted to initiate a large data size, resulting in buffer overflow [35,74]. The focus of the research in [75] is to investigate the impacts of denial of service attacks on the security of cyber-physical MGs and analyze the system stability while under such an attack. Simulation results revealed DoS attacks on MGs can result in communication delay and eventual system instability. In [76] mitigation of DoS attacks was carried out by splitting the data plane from the network control plane. Then, an exchange of control plane overpower bus was implemented. In this way, the configuration of the data plane was made possible. Each DER are treated as either active or passive agent in the network. The operating mode of each agent is determined by software-defined MG control. Using a distributed secondary control framework, researchers in [77] were able to detect DoS attacks on energy storage systems of an MG. The detection mechanism was based on an acknowledgement-based strategy. For paralyzed communication graphs, a communication recovery technique was proposed. Experimental results show the applicability of the approach.
An attacker intends to compromise the password and manipulate data to gain undue advantage and access to a system's network or IEDs. Upon gaining such unauthorized access, a false signal could be sent across the network, resulting in system collapse and disengagement from the targeted part, thereby disrupting regular system operation. The approach used in [78], [79] relies on information theory precisely Kullback-Liebler divergence-based criterion in detecting and mitigating data manipulation attacks on secondary control of frequency and voltage in an AC microgrid distributed system. Individual DER can detect compromise in the integrity of the information received from its neighbour in the distributed network and discard such malicious information. The approach was operated on communication graph connectivity.
In a false data injection attack, an attacker alters the system mode by injecting malicious data into each of the network agents [80]. [81] investigate false data injection attacks aimed at depriving users of MG network services paid for. The study results suggest that an attack signal may be optimized to balance energy demand with supply. Such false data injection attacks could be detected when differences in earnings are calculated for similar energy trading. In similar research, researchers in [80] used Hilbert-Huang transform and blockchain-based approach to secure data exchange to detect false data injection attack. Simulation results showed that the approach could detect and prevent attackers from penetrating the network of DC MGs. To detect and quantify the impact of false data injection attacks of DC MG network, [82] use signal temporal logic-based detection approach. The effectiveness of the approach was verified using a hardware-in-loop experiment. Preliminary results indicate the method can be applied for detection of both DoS and false data injection attacks on MG networks. Researchers in [83] use the mitigation approach relies on a weighted mean subsequence reduced algorithm for a multi-microgrid system. Individual MG acting as agents is programmed to reject unusual traffic coming from an agent under attack in the network, and thus system desired performance could be maintained. In Table 3, a description of cyber-attack featuring; attack detection techniques, impacts on microgrid installations and mitigation approaches is presented.
Cyber Attack Description | Attack Detection Technique | Impacts of Cyber Attacks | Mitigation Approach |
Data Manipulation | Kullback-Liebler (KL) divergence-based criterion [78], [79] | Degrades Frequency and voltage control of islanded MGs [78], [79] | Calculated KL divergence factors to determine compromise [78], [79] |
False data injection | *Calculates Earnings based on energy [81] purchased and supplied. * Hilbert-Huang transform [80] *Signal temporal logic. [82] |
*Financial Loss [81] * consensus protocols interruptions [80] *Power sharing compromise. [82] * Voltage regulation compromise. [82] |
* Blockchain-based data exchange layout. [81]. * weighted mean subsequence reduced algorithm. [83] |
Denial of service | *ACK-based detection [77] * Entropy-based. [84] *Signal temporal logic. [82] |
*Communication delay and system oscillations. [75] *Blockage of communication channel [76], [77] *Variation in communication graph. [74]. |
*Separation of the data plane from the network control plane. [76] *Distributed averaging proportional-integral control. [74] *Communication recovery mechanism. [77]. |
Communication induced impairments in microgrids could be handled using two methods, which are:
ⅰ. the use of high-speed communication networks such as fibre optics, 5G cellular network, or non-industrial, scientific, and medical (ISM) frequency bands (Non-ISM bands).
ⅱ. development of smart control solutions and mechanisms incorporated into the microgrids controllers that make the microgrids resilient against communication impairments, thus reducing the resultant effects of communication impairments on the general operation of the system [25].
In this review, attention will be given to secondary control solutions employed to mitigate communication impairments in microgrids. Apart from the lack of interoperability of specialized high-speed communication networks, the cost of operating one could render the microgrid itself cost-ineffective. Therefore, researchers tend to use inexpensive but effective control methods for impairments mitigation. However, new communication technologies are emerging that can bridge the gap between the two alternatives.
The hierarchical control structure is the bedrock for the microgrid control framework. The primary, secondary and tertiary control structures are the three levels of hierarchical control in microgrid [25]. Voltage and frequency stability maintenance after the islanding process is handled by the primary control. The autonomous operation of MG using only the primary control layer results in deviations in operating frequency and voltage. The corresponding adverse effects on the general performance and operations of the MG demands a secondary control that will effectively mitigate the anomaly introduced by the primary control method [59]. As a follow-up of primary control, the secondary control compensates for the inadequacies created by the primary control. Voltage and frequency synchronization are restored at this level of control. The tertiary control manages the power flow between the main grid and the microgrid. The economical and optimal operation of the grid is a responsibility of the tertiary control level. Microgrids hierarchical control structure and the function of each control stage is shown in Figure 3. In Primary control, instantaneous control of frequency and voltage is a requirement to ensure system stability. This implies the operation of the controllers are time-critical and, thus, should not rely on the communication network. The effects of communication impairments are chiefly felt in the secondary control. Communication induced impairments such as latency, data packet dropout and noise could degrade the performance of the microgrids. Information shared in the tertiary control layer are not time critical and cannot affect system performance, since data shared at this level are related to business aspect of the microgrid. An exception to this is when the system comes under malicious attacks seeking to compromise the integrity, authenticity and intension of the data shared.
Mitigation strategies that rely on the CCDC schemes exchange information such as control commands, operation and sensors' information between a centralized controller and the local controllers in the DERs. The centralized controller is responsible for processing the information after which the control commands are related to the individual local controllers. The drawback of the CCDC is related to its dependence on the communication network used. It also has reliability concerns because it is subject to a single point of failure. Once there is a problem with the central control, the entire system collapses. In Figure 4, all the local controllers take information from the central controller for their operation. The performance of the local controllers is directly dependent on the central controller.
Distributed communication dependent control scheme uses a framework where each DG can communicate directly with each other [85]. Local controllers achieve control but status information is shared so that synchronization can be ensured at the common bus of the microgrid. DCDC scheme is more reliable than centralized control since there is no single point of failure that can result in a complete system collapse in a situation where one link of the system failed. However, communication latency and packet dropout between the links could degrade the system's performance. Local controllers must operate at a faster rate compared to the secondary controller to prevent system instability. The architecture of a distributed communication dependent controller is shown in Figure 5. Each controller shares information with its neighbour via the communication network installed. The individual controller can operate independently. However, under normal operating conditions, the neighbour's reference signals are used by the local controller to update its output so that synchronization can be achieved at the common bus where the output of the microgrid is connected to the load.
The droop control method is used at the primary level of control because at this level, the operation of the controllers is time-critical. Long latency will result in system instability since instantaneous control of frequency and voltage is required to ensure system instability. Hence, control at this level is not reliant on communication networks whose major impairment is a delay. However, the anomaly that results from primary control methods is corrected by the secondary control methods.
Imbalance line impedance degrades the performance of the conventional droop method. Poor load sharing and voltage regulation is the drawback of the method [86,87]. Voltage regulation via secondary control techniques is a common approach used in combatting the menace of voltage deviations or mismatch among distributed inverters in islanded microgrids. The conventional voltage and frequency droop control is given as [88]:
E=E∗−nqQ | (10) |
ω=ω∗−mpP | (11) |
where E is the amplitude of DG output voltage (RMS value), E∗ is the rated RMS voltage of the inverter, ω∗ is the rated system line frequency, ω is the measured system line frequency, nq and mp are the droop coefficients. Artificial droop is introduced in the inverter frequency to share real power among the multi-inverters, [32]. If ω is inverter frequency which is set by mp and the phase is set by integrating the frequency. Droop characteristics introduced in the voltage magnitude is responsible for reactive power sharing among multiple inverters. A voltage set point say δe2i and frequency set point of δω2i is assigned to the inverters attached to primary controllers in order to reach a global voltage regulation. The set point is responsible for adjusting the voltage to the nominal value. The droop equation then takes the form:
E=E∗−nqQ+δe2i | (12) |
ω=ω∗−mpP+δω2i | (13) |
For simplicity and ease of understanding, the diagram shown in Figure 6 depicts an equivalent circuit representing islanded microgrid with n DGs connected in parallel. Although the DGs are of similar power ratings, disparities in the impedances results in varying voltage magnitude and frequency at the common AC bus. Considering DG1 and DG2, given that Z1>Z2, the E-Q droop characteristics are shown in Figure 7, while the P-ω droop characteristics are represented in Figure 8.
(a) Voltage restoration: The E-Q characteristics representing voltage restoration is depicted in Figure 7a, E1 and Q1 gives the voltage magnitude and reactive power due to DG1, respectively, while DG2 injected E2 and Q2 into the microgrid network. The voltage and reactive power responses with the conventional droop control are represented by K (E1, Q1) and M (E2, Q2); the solid black line. The different values of reactive power is an indication that it is not being shared accurately. ΔQ1 gives the reactive power deviation at this point. The point L (E*, Q'1) and N (E*, Q'2) represent the points when the secondary method is used to restore the voltage to the rated value E* at which point the reactive power deviation is larger and it is given by ΔQ2.
(b) Reactive power-sharing: A similar scenario is depicted in Figure 7b, which represents reactive power-sharing. Points M (E'1, Q') and N (E'2, Q') which represent the new values of voltages from the secondary reactive power sharing control, from the initial values of K (E1, Q1) and L(E2, Q2) when the conventional droop was applied. With the improvement in reactive power-sharing at Q', the magnitude of voltage deviation increases from ΔE1 to ΔE2. Obviously, achieving voltage restoration comes at the cost of degrading reactive power-sharing. So, there is a trade-off between the two objectives [26].
(a) Frequency restoration: The ω-P characteristics representing frequency restoration is shown in Figure 8a, ω1 and P1 gives the magnitude of frequency and active power due to DG1, respectively, while DG2 injected ω2 and P2 into the microgrid network. The frequency and active power curves with the conventional droop control are represented by K (ω1, P1) and M (ω2, P2); the solid black line. The active power is shared according to the power ratings of each DGs. The active power deviation at this point is given by ΔP1. The point L (ω*, P'1) and N (E*, Q'2) represent the points when the secondary method is used to restore the frequency to the rated value ω* at which point the active power deviation is larger and it is given by ΔP2.
(b) Active power-sharing: Following the same narration, depicted in Figure 8b is active power sharing characteristics. Points M (ω'1, P') and N (ω'2, P') which are the new values of frequency from the secondary active power sharing control, from the initial values of K (ω1, P1) and L (ω2, Q2) when the conventional droop control was employed. The active power sharing improvement can be seen in the fact that the two DG sources shared a common value P'. However, the magnitude of frequency deviation increases from ΔP1 to ΔP2. The analysis shows that a trade-off must be made between frequency stability and active power-sharing while using the secondary droop control technique for islanded microgrids.
To enhance the stability of voltage and frequency using droop control, voltage and frequency are drooped based on the active (P) and reactive (Q) power as the control level demanded. [86] proposed an improved droop method for reactive power-sharing, low-bandwidth synchronization signals is used for error reduction and voltage restoration operations. The technique used improved reactive power-sharing and general performance of the microgrid system. Also, in [89], the analysis of the line impedance difference between various inverters on power-sharing with the traditional droop control method was presented. [90] proposed a control strategy for harmonic power-sharing in an islanded microgrid in another droop-based method. In work by [91], a strategy that employs an adaptive voltage droop control to achieve accurate reactive power-sharing was investigated. Droop-based methods are usually low cost, take measurement locally and apply to both grid-connected and islanded mode. A drawback of the control method in the islanded mode is that microgrid voltage and frequency vary with load change. Therefore, a mechanism must restore the system frequency and voltage to nominal values after a load change. The performance is also poor in MG with high penetration of RES.
Various modifications of the conventional droop control method have been used to reduce the disadvantages of the method. In the adjustable load sharing method, the active and reactive power control can be achieved without impacting the frequency and voltage of the DG source. The voltage active power droop/ frequency reactive power boost (VPD/FQB) approach, alternate consideration of VPD/FQB characteristics for improvement in the control was employed. Another modification is the virtual frame transformation technique. This method transfers the active and reactive powers to a different reference frame where the effects of line impedance do not impact power generated. The adjustable load sharing control and adaptive voltage droop methods are the most effective for voltage control. The droop control is generally weak in handling nonlinear loads and most times accurate voltage regulation is not guaranteed. The droop techniques offer local controls independent of any communication infrastructures. The pros and cons of the conventional droop control technique and its modifications indicate the need for the secondary control level for improved control and operation of the microgrid system [30].
The droop control technique is a conventional method used for primary control and power-sharing in islanded microgrids. However, the secondary control methods mitigate the anomalies introduced by using the droop control. The restoration of voltage and frequency synchronization takes place at this level. The need for information sharing to achieve system stability makes secondary control communication dependent. The impacts of communication impairments are chiefly felt in the secondary control. Communication induced impairments such as latency, data packet dropout and noise could degrade the performance of the microgrids. Secondary control in AC MGs is essential for frequency and voltage regulation, active and reactive load power-sharing, islanded microgrid synchronization, and power quality matters.
Agent-based methods have appeared recently in distributed control of islanded MG. In a multi-agent system, each essential component is represented by an intelligent, independent agent. Control is achieved with a sparse communication graph that is considered a basic component that gives a rational and computationally feasible solution. Multi-agent systems control can give a mechanism that is efficient for coordination and communication of protocol for the individual component in the system. Control schemes are rested on the output characteristics of individual power sources. The technique also includes control approaches to optimize the trade-off between performance of the system and cost of operation [14], [92], [93].
A directed graph (digraph) can be used for DGs topology representation to achieve distributed control. It describes the topology between agents in a microgrid. Given G=(v,ε,A) is a weighted digraph of order n, with the set of nodes v={v1,….vn}, set of edges ε⊆v×v, and a weighted adjacency matrix A=[aij] having nonnegative adjacency elements aij. The nodes of the digraph represent each DG agent in a microgrid. The edges denote communication links between DGs. Each edge eij=(vi,vj) implies that the agent DGi receives information from agent DGj [94], [95]. For a digraph, an agent DGi only receives information from its neighbours Ni. aij is the weight of edge eij=(vi,vj) and aij = 1 if (Vi,Vj)ϵε, otherwise aij = 0. The set of neighbours of node viis denoted by [96], [97]:
Ni={vjϵv:(vi,vj)ϵε} | (14) |
Smart control issue for an islanded microgrid for secure voltages and maximum economic and environmental benefits [98]. [99] worked on decentralized multi-agent system-based cooperative frequency control for autonomous MGs with communication constraints. In [93], an agent-based secondary control strategy for an islanded MG consisting of a two-layer control structure was proposed. [100] presented a distributed control structure for implementing the frequency control of an islanded MG. Multi-agent based methods rely on communication networks and are directly affected by the performance of the installed communication system. Although a very advantageous distributed secondary control technique, the design of multi-agent control must be built with resilience for communication link failure in mind.
Consensus-based methods seek to converge values from different RES in a MG to a single value. Using the communication links between adjacent RES, an optimal global value for all the distributed agents can be attained for voltage and frequency control in AC MGs [13], [29]. Consensus problems in a network of continuous-time integrator agents, when the topology is switching, and communication delay is negligible, can be solved using the consensus protocol given as:
ui=∑uj∈Niaij(xj−xi) | (15) |
where the set of neighbours Ni=Ni(G) of the node viis variable in networks with switching topology. However, a fixed topology G=(v,ε,A) and communication time-delay τij>0corresponding to the edge eij∈ε the following linear time-delayed consensus protocol is used:
ui(t)=∑vj∈Niaij[xj(t−τij)−xi(t−τij)] | (16) |
The consensus-based method was used in [101] to investigate reactive power-sharing among DGs. The proposed method can improve system reliability and flexibility. In [94], to achieve accurate reactive power sharing, not being bothered by the effects of line impedance mismatched. A method that employs both consensus and control was used to determine the reactive power mismatch among DG units. Also, in related research by [102], a containment and consensus-based distributed coordination control was presented to achieve both bounded voltage and accurate reactive power sharing regulation in islanded AC MG'. [103] presented a paper to address distributed noise in the communication links between DGs in MGs. The consensus-based control methods, just like its counterpart, the multi-agent-based methods, require a communication network for operation. Its performance is closely related to the performance of the communication network used for the sharing of its coordination and control information.
MPC is a control method used in the process industries. It has also found applications in error tracking based on dynamic models in power systems. The MPC approach can take future prediction and an effective control action. When current plant measurement, process variables, and system dynamic state are available, the MPC method can be used to determine future events. The cost function that has been predetermined is used to calculate optimal values for future control objectives [104], [105]. For example, to enhance operation efficiency, RES implementation and reducing energy storage system depletion. The optimization problem can be implemented using an objective cost function C given as:
C=∑k+Ni=k[Jbt(i)+Jpv(i)+Jw(i)+Jdpg(i)] | (17) |
where Jbt(i), Jpv(i), Jw(i), and Jdpg(i) represent the cost term relating to battery, PV generation system, wind generation, and amount of dispatchable generation used, respectively.
A model predictive control method used in secondary control of microgrid frequency by [87] output signals from the control unit is transmitted via the communication channel to the generation sources to achieve frequency control. The performance of the MPC method is better when compared with the smith predictor based method. [105], [106] used MPC to assess energy management in an autonomous microgrid, which relies on PV generation and a hybrid energy storage system composed of a fuel cell, battery and supercapacitor. In [106] MPC approach was applied for operations optimization in microgrids to improve economic efficiency. The performance of MPC was compared with the heuristic-based method, and results indicate the MPC method improves the performance of islanded microgrid. Model Predictive Control (MPC) was proposed by [107] for frequency stabilization in a microgrid under solar penetration and load fluctuation. In addition, [108] designed a robust model predictive controller for grid voltage control of an islanded microgrid. [109] used MPC for distributed secondary control for both voltage and frequency regulation in islanded microgrids. MPC method for MG control was robust, but it could be very complex to implement.
LQG controllers can track the performance of islanded microgrids. It gives large gain at low frequency. Considering the state pace representation of system (microgrids) dynamics and noise as:
˙x=A1x+B1u+v | (18) |
y=C1x+w | (19) |
where x, is the state vector, u, represent the system input, and y measured output. v and w, are the input and output Gaussian white noise, respectively. A1, is the matrix of the system state while B1, and C1 are the input and output matrix, respectively. Finding a control input u that will optimize the cost function is the objective of the LQG controller. The cost function for the LQG controller can be represented as [110], [111]:
J∞=E{xT(T)Fx(T)+∞∫0[xT(t)Qx(t)+uT(t)Ru(t)]dt} | (20) |
when the time horizon becomes infinity, the term xT(T)Fx(T) becomes negligible. E is the expected value, the term xTQx represent the state minimization, while uTRu describe control input minimization. Though this controller has a significant gain at low frequency, it suffers from the lack of robustness against the changes in plant dynamics [111], [112].
The voltage oscillation controller designed for microgrids control in [110] is based on integral LQG, in the approach used, the system output is augmented to attain integral action. The LQG controller can also track grid voltage when the microgrid is operating in grid-connected mode. A comparison with LQR and LQG controllers shows that the integral LQG performs better in damping voltage oscillations in microgrid networks. The method used in [113] utilizes an H-infinity controller to modify the droop control method. MG voltage control using linear quadratic regulator (LQR) method based on linearization techniques designed to attain fast and accurate response was proposed [111].
Proportional-Integral-Derivative (PID) takes the leading role among many other controllers being implemented in industrial applications today [104]. The transfer function of PID controller consisting of Proportional, Integral and Derivative structures in a single package is represented by:
U(s)=E(s)[Kp+KIs+KDs] | (21) |
where U(s) is the control variable, E(s) the error, and Kp, KI, and KD are proportional, integral, and differential gains. The P-term is proportional to the error, the Ⅰ-term is proportional to the integral of the error, and the D-term is proportional to the derivative of the error. The system's transient response is enhanced via proportional gain; the integral gain minimises the steady-state error, while the derivative gain of the PID controller degrades the overshoot of the system.
The benefits of using PID controllers for voltage control in islanded microgrids are investigated in [114]. In the analysis, both theoretical and experimental approaches were employed. The analysis shows cascade control is a possibility in islanded microgrid control, and employing measured current in the control information enhances the system performance. Frequency regulation in AC microgrids was the focus of [115], multi-verse optimized fractional order PID controller, an adaptation of the PID controller. Also, in similar research, [116] used fractional order PID with parameter optimization done by the multi-verse optimizer for frequency regulation in AC microgrids. PID and Combined Proportional-Integral (PI) controllers have been proposed to improve the limitation of H-infinity controller by [117]. Furthermore, [7] designed a modified adaptive PID controller for voltage and current control of islanded microgrid.
The general problem of the H∞ is to realize compensator K such that the close-loop controller is stabilized and minimized following the H∞ norm. H-infinity controller was developed by [118] to achieve a robust microgrid performance against unmolded load and uncertainties. [119], in their work use H∞ and μ-synthesis approach to developing the secondary frequency control in islanded MG. The work by [120] presents a robust virtual inertial control of an islanded MG with high penetration of RESs. The design of H-infinity depends on the norms minimization and order of the system. As a result, a high order system needs a high order controller that may require advanced digital signal processing to control the microgrid's performance.
Presented in Table 4 is the summary of some of the control methods used for mitigating impairments induced by communication network on the smooth operation of the microgrid. As discussed under section 6.3, the droop based methods are independent of communication network and are not affected by the impairments they introduced. It is conventionally use at the primary control level of the microgrids. The weaknesses and strengths of some of the secondary control methods used are presented in the table.
Control Method | Strengths | Weaknesses |
Droop-Based [86], [89], [90], and [91] |
Take local measurement | Poor performance under Nonlinear loads |
Low cost | Weak in handling high RES penetration | |
Suitable for both grid and island mode connection | Poor control of power-sharing under uncertain loads | |
A Simple controller and easy to use | ||
Multi Agent-Based [93], [98], [99] and [100] |
Suitable for both grid and island mode connection | Dependent on communication network for smooth operation. |
Suitable for MG with large penetration of RES | Can be impacted by communication link failure | |
Very effective in islanded MG control | ||
Cost-effective | ||
Consensus-Based [94], [101], [102], and [103]. |
Suitable for both grid and island mode connection | Dependent on communication network for smooth operation. |
Good scalability Properties | It's sensitive to communication failure | |
If the algorithm is not optimized, it could be time-consuming | ||
MPC-Based [87], [105], [106], [107], [108], and [109] |
Suitable for both grid and island mode connection | It cannot be used in uncertain systems |
Easy to tune | It could be complex to implement | |
Good at handling multivariable control problems | ||
LQG-Based [110], [113], and [111]. |
Suitable for both grid and island mode connection | Surfers from lack of robustness against changing plant dynamics |
PID-Based [7], [114]. [115], [116], and [117], |
Easy to design | Poor control of power sharing |
Fast transient response | Cannot effectively handle high RES penetration | |
Robust performance in the presence of un-modelled loads, dynamic loads, nonlinear loads and harmonic loads. | ||
H-infinity based [118]. [119], and [120] |
Could offer precise reference frequency tracking. | Depends on the norms minimization and order of the system |
Suitable for both grid and island mode connection | May require advanced digital signal processing to control the performance of MG |
The need for reliable, sustainable and clean energy coupled with the demand for optimal operation of renewable energy-based autonomous microgrids requires developing its control system. The mitigation of the communication induced impairments in islanded microgrids will improve the overall performance of the control system and the microgrid at large. Exchanging information between microgrid components is faced with challenges, as highlighted in this paper. literature does not fully address communication-induced impairments such as latency, packets dropouts, and cyber security challenges. Smart control techniques are emerging that can reduce the impacts of the impairments on the performance of the microgrid system. Smart control techniques are preferable to developing new communication technology because of the cost and difficulties new communication infrastructure would require before eventual deployment.
The primary control units of microgrids do not require communication infrastructures, and as a result, does not suffer severe degradation because of malfunction induced by the presence of a communication network. Mitigation efforts for communication induced impairments are not required at this level. But the secondary layer depends on communication networks and are directly affected by their performance. Control techniques must be smart enough to guarantee the microgrid operation's performance. Consensus protocol and multi-agent-based control techniques are being applied to microgrid control. The results in [121], [122] have shown robustness against communication failure and cyber-attacks.
Existing wireless communication technology such as Wi-Fi, WiMAX, LoRa, and the emerging 5G cellular network can be deployed for microgrid control applications. Wireless communication is flexible, meaning future expansion will not be problematic. Deployment in rural communities will also be comparatively easy compared to its wired counterpart. The comparison of both wired and wireless networks given in this paper could guide while making choices. Depending on the specific application targeted by the user and the range required, choices could be made for the most suitable technology.
GSM technology was originally designed for machine to human communication. Its application to a microgrid system, a machine to machine communication, is still undergoing development. Fibre optics communication will be most appropriate in time-critical applications where large delay cannot be tolerated. However, if flexibility becomes an issue, the emerging 5G network could be considered for deployment. Some inverters available for installation come with Wi-Fi technology for information sharing between individual inverters in the microgrid network. However, using Ethernet cables for connection rather than the wireless Wi-Fi connection proved more effective for most of the products. Future products should come with more reliable wireless infrastructure in order to enjoy all the benefits derivable from wireless connection. Most microgrid controllers follow the IEEE 2030.7-2017 standard for microgrid controllers. It provides microgrid operation based on the microgrid internal controller and SCADA. The products enhance the integration of renewable energy to the main grid and can autonomous control of microgrid when operating in islanded mode. Most of the available controllers operate with the droop controller in the primary level of control.
This paper presents a comprehensive review of mitigation strategies for communication induced impairments in autonomous microgrid control. Three major communication network induced impairments were mostly researched in literature: data packet dropouts, information packet delay/latency and cyber-attacks induced by using communication networks, especially the wireless networks for information sharing among DGs in the microgrid networks. The approach used in the literature rely mainly on smart control solutions rather than using high-speed communication networks for impairment mitigation. High-speed communication network could be costly to use, and incorporating one in the microgrid system can render the system too expensive to operate. It can therefore be seen that smart control solutions integrated with microgrid control are the way to go in the mitigation of impairments induced as a result of using communication networks in microgrid operation. The review also shows the following:
(ⅰ) Bernoulli random process is very effective for modelling data packet dropouts; it yields an output of 1 or 0 for successful data delivery or communication failure, respectively. The probability of data packet drops when queuing time exceeds the delay limit can also be explored to measure and quantify data packet dropout and delay.
(ⅱ) If propagation and processing delay are treated as a constant value, delay dynamics can be limited to the evolving transmission state and queueing along the signal path.
(ⅲ) Common cyber-attacks directed against microgrid installations are denial of service, false data injection, and data manipulation attacks. Therefore, advanced effort is required to effectively combat these evolving attacks on microgrid installations.
(ⅳ) A sparse communication graph is a basis for communication topology design for distributed secondary control in the microgrid. The fixed and shifting topology should be further investigated to engender optimal topology design.
The need for further investigation on effective integration of communication networks in the microgrid is presented in this paper. The use of communication networks in the microgrids network is indispensable. Current trends in the development of the smart grid suggest that the incorporation of communication networks will continue to increase.
The authors declare that there is no conflict of interest.
[1] |
Kahrobaeian A, Mohamed YARI (2015) Networked-based hybrid distributed power sharing and control for islanded microgrid systems. IEEE T Power Electr 30: 603-617. doi: 10.1109/TPEL.2014.2312425. doi: 10.1109/TPEL.2014.2312425
![]() |
[2] | Bidram A, Nasirian V, Davoudi A, et al. (2017) Cooperative Synchronization in Distributed Microgrid Control. Advances in Industrial Control, vol. 1. |
[3] |
Alzahrani A, Ferdowsi M, Shamsi P, et al. (2017) Modeling and Simulation of Microgrid. Procedia Comput Sci 114: 392-400. doi: 10.1016/j.procs.2017.09.053. doi: 10.1016/j.procs.2017.09.053
![]() |
[4] |
Hossain MA, Pota HR, Issa W, et al. (2017) Overview of AC microgrid controls with inverter-interfaced generations. Energies 10: 1-27. doi: 10.3390/en10091300. doi: 10.3390/en10091300
![]() |
[5] |
Hossain E, Kabalci E, Bayindir R, et al. (2014) A comprehensive study on microgrid technology. Int J Renew Energy Res 4: 1094-1104. doi: 10.20508/ijrer.20561. doi: 10.20508/ijrer.20561
![]() |
[6] |
Porsinger T, Janik P, Leonowicz Z, et al. (2017) Modelling and optimization in microgrids. Energies 10: 1-22. doi: 10.3390/en10040523. doi: 10.3390/en10040523
![]() |
[7] |
Siddique AB, Munsi S, Sarkar SK, et al. (2019) Model reference modified adaptive PID controller design for voltage and current control of islanded microgrid. 4th Int Conf Electr Eng Inf Commun Technol iCEEiCT 2018, 130-135. doi: 10.1109/CEEICT.2018.8628074. doi: 10.1109/CEEICT.2018.8628074
![]() |
[8] | Shayeghi H, Sobhany B, Moradzadeh M (2017) Management of Autonomous Microgrids Using Multi-Agent Based Online Optimized NF-PID Controller. J Energy Manag Technol 1: 79-87. |
[9] |
Rana MM, Li L, Su SW (2017) Distributed State Estimation of Smart Grids with Packet Losses. Asian J Control 19: 1306-1315. doi: 10.1002/asjc.1578. doi: 10.1002/asjc.1578
![]() |
[10] |
Parisio A, Wiezorek C, Kyntäjä T, et al. (2017) Cooperative MPC-Based Energy Management for Networked Microgrids. IEEE T Smart Grid 8: 3066-3074. doi: 10.1109/TSG.2017.2726941. doi: 10.1109/TSG.2017.2726941
![]() |
[11] | Sun Y, Hu J, Zhang Y, et al. (2018) Distributed Secondary Voltage Control of Microgrids with Nonuniform Time-Varying Delays. in Chinese Control Conference, CCC, 2018, 8809-8814. doi: 10.23919/ChiCC.2018.8483983. |
[12] |
Sarkar SK, Roni MHK, Datta D, et al. (2019) Improved Design of High-Performance Controller for Voltage Control of Islanded Microgrid. IEEE Syst J 13: 1786-1795. doi: 10.1109/JSYST.2018.2830504. doi: 10.1109/JSYST.2018.2830504
![]() |
[13] |
Aghaee F, Dehkordi NM, Bayati N, et al. (2019) Distributed control methods and impact of communication failure in AC microgrids: A comparative review. Electronics 8: 1265. doi: 10.3390/electronics8111265. doi: 10.3390/electronics8111265
![]() |
[14] |
Lou G, Gu W, Lu X, et al. (2020) Distributed Secondary Voltage Control in Islanded Microgrids with Consideration of Communication Network and Time Delays. IEEE T Smart Grid 11: 3702-3715. doi: 10.1109/TSG.2020.2979503. doi: 10.1109/TSG.2020.2979503
![]() |
[15] |
Ci S, Qian J, Wu D, et al. (2012) Impact of wireless communication delay on load sharing among distributed generation systems through smart microgrids. IEEE Wirel Commun 19: 24-29. doi: 10.1109/MWC.2012.6231156. doi: 10.1109/MWC.2012.6231156
![]() |
[16] |
Xu Y, Wang W (2013) Wireless mesh network in smart grid: Modeling and analysis for time critical communications. IEEE T Wirel Commun 12: 3360-3371. doi: 10.1109/TWC.2013.061713.121545. doi: 10.1109/TWC.2013.061713.121545
![]() |
[17] |
Lai J, Zhou H, Hu W, et al. (2015) Synchronization of Hybrid Microgrids with Communication Latency. Math Probl Eng 2015: 1-10. doi: 10.1155/2015/586260. doi: 10.1155/2015/586260
![]() |
[18] |
Alfergani A, Khalil A (2017) Modeling and control of master-slave microgrid with communication delay. 2017 8th Int Renew Energy Congr IREC, 1-6. doi: 10.1109/IREC.2017.7926049. doi: 10.1109/IREC.2017.7926049
![]() |
[19] |
Chen G, Guo Z (2019) Distributed secondary and optimal active power sharing control for islanded microgrids with communication delays. IEEE T Smart Grid 10: 2002-2014. doi: 10.1109/TSG.2017.2785811. doi: 10.1109/TSG.2017.2785811
![]() |
[20] |
Nasirian V, Davoudi A, Lewis FL, et al. (2014) Distributed adaptive droop control for DC distribution systems. IEEE T Energy Conver 29: 944-956. doi: 10.1109/TEC.2014.2350458. doi: 10.1109/TEC.2014.2350458
![]() |
[21] |
Nasirian V, Moayedi S, Davoudi A, et al. (2015) Distributed cooperative control of dc microgrids. IEEE T Power Electr 30: 2288-2303. doi: 10.1109/TPEL.2014.2324579. doi: 10.1109/TPEL.2014.2324579
![]() |
[22] |
Nasirian V, Shafiee Q, Guerrero JM, et al. (2016) Droop-Free Distributed Control for AC Microgrids. IEEE T Power Electr 31: 1600-1617. doi: 10.1109/TPEL.2015.2414457. doi: 10.1109/TPEL.2015.2414457
![]() |
[23] |
Shafiee Q, Stefanovic C, Dragicevic T, et al. (2014) Robust networked control scheme for distributed secondary control of islanded microgrids. IEEE T Ind Electron 61: 5363-5374. doi: 10.1109/TIE.2013.2293711. doi: 10.1109/TIE.2013.2293711
![]() |
[24] |
Sun Y, Zhong C, Hou X, et al. (2017) Distributed cooperative synchronization strategy for multi-bus microgrids. Int J Electr Power 86: 18-28. doi: 10.1016/j.ijepes.2016.09.002. doi: 10.1016/j.ijepes.2016.09.002
![]() |
[25] |
Serban I, Cespedes S, Marinescu C, et al. (2020) Communication requirements in microgrids: A practical survey. IEEE Access 8: 47694-47712. doi: 10.1109/ACCESS.2020.2977928. doi: 10.1109/ACCESS.2020.2977928
![]() |
[26] |
Han, Y, Li, H, Shen P, et al. (2017) Review of Active and Reactive Power Sharing Strategies in Hierarchical Controlled Microgrids. IEEE T Power Electr 32: 2427-2451. doi: 10.1109/TPEL.2016.2569597 doi: 10.1109/TPEL.2016.2569597
![]() |
[27] |
Dragicevic T, Lu X, Vasquez JC, et al. (2016) DC Microgrids - Part Ⅰ: A Review of Control Strategies and Stabilization Techniques. IEEE T Power Electron 31: 4876-4891. doi: 10.1109/TPEL.2015.2478859. doi: 10.1109/TPEL.2015.2478859
![]() |
[28] |
Vandoorn TL, De Kooning JDM, Meersman B, et al. (2013) Review of primary control strategies for islanded microgrids with power-electronic interfaces. Renewable and Sustainable Energy Reviews 19: 613-628. doi: 10.1016/j.rser.2012.11.062. doi: 10.1016/j.rser.2012.11.062
![]() |
[29] |
Ekanayake UN, Navaratne US (2020) A Survey on Microgrid Control Techniques in Islanded Mode. Electr Comput Eng 2020: 1-8. doi: 10.1155/2020/6275460. doi: 10.1155/2020/6275460
![]() |
[30] |
Rajesh KS, Dash SS, Rajagopal R, et al. (2016) A review on control of ac microgrid. Renew Sustain Energy Rev 71: 814-819. doi: 10.1016/j.rser.2016.12.106. doi: 10.1016/j.rser.2016.12.106
![]() |
[31] |
Han Y, Ning X, Yang P, et al. (2019) Review of Power Sharing, Voltage Restoration and Stabilization Techniques in Hierarchical Controlled DC Microgrids. IEEE Access 7: 149202-149223. doi: 10.1109/ACCESS.2019.2946706. doi: 10.1109/ACCESS.2019.2946706
![]() |
[32] |
Meng L, Shafiee Q, Trecate GF, et al. (2017) Review on Control of DC Microgrids and Multiple Microgrid Clusters. IEEE J Emerg Sel Top Power Electron 5: 928-948. doi: 10.1109/JESTPE.2017.2690219. doi: 10.1109/JESTPE.2017.2690219
![]() |
[33] |
Arbab-Zavar B, Palacios-Garcia EJ, Vasquez JC, et al. (2019) Smart inverters for microgrid applications: A review. Energies 12: 840. doi: 10.3390/en12050840. doi: 10.3390/en12050840
![]() |
[34] |
Nejabatkhah F, Li YW, Liang H, et al. (2021) Cyber-security of smart microgrids: A survey. Energies 14: 27. doi: 10.3390/en14010027. doi: 10.3390/en14010027
![]() |
[35] |
Habib HF, Lashway CR, Mohammed OA (2017) On the adaptive protection of microgrids: A review on how to mitigate cyber attacks and communication failures. 2017 IEEE Ind Appl Soc Annu Meet IAS, 1-8. doi: 10.1109/IAS.2017.8101886. doi: 10.1109/IAS.2017.8101886
![]() |
[36] |
Dahunsi F, Olayanju S, Ponle A, et al. (2021) Communication Network Simulation for Smart Metering Applications: A Review. J Innov Sci Eng 5: 101-128. doi: 10.38088/jise.835725. doi: 10.38088/jise.835725
![]() |
[37] |
O'Raw J, Laverty DM, Morrow DJ (2016) Software defined networking as a mitigation strategy for data communications in power systems critical infrastructure. IEEE Power and Energy Society General Meeting, 1-5. doi: 10.1109/PESGM.2016.7741417. doi: 10.1109/PESGM.2016.7741417
![]() |
[38] |
Sivaneasan B, So PL, Gooi HB, et al. (2013) Performance measurement and analysis of WiMAX-LAN communication operating at 5.8 GHz. IEEE T Ind Inform 9: 1497-1506. doi: 10.1109/TⅡ.2013.2258163. doi: 10.1109/TⅡ.2013.2258163
![]() |
[39] |
Sevilla AP, Ortega EI, Hincapie R (2015) FiWi network planning for smart metering based on multistage stochastic programming. IEEE Lat Am Trans 13: 3838-3843. doi: 10.1109/TLA.2015.7404917. doi: 10.1109/TLA.2015.7404917
![]() |
[40] |
Llaria A, Terrasson G, Curea O, et al. (2016) Application of wireless sensor and actuator networks to achieve intelligent microgrids: A promising approach towards a global smart grid deployment. Appl Sci 6: 61. doi: 10.3390/app6030061. doi: 10.3390/app6030061
![]() |
[41] |
Siow LK, So PL, Gooi HB, et al. (2009) Wi-Fi based server in microgrid energy management system. IEEE Reg 10 Annu Int Conf Proceedings/TENCON, 1-5. doi: 10.1109/TENCON.2009.5395995. doi: 10.1109/TENCON.2009.5395995
![]() |
[42] |
Setiawan MA, Shahnia F, Rajakaruna S, et al. (2015) ZigBee-Based Communication System for Data Transfer Within Future Microgrids. IEEE T Smart Grid 6: 2343-2355. doi: 10.1109/TSG.2015.2402678. doi: 10.1109/TSG.2015.2402678
![]() |
[43] |
Sharma D, Dubey A, Mishra S, et al. (2019) A Frequency Control Strategy Using Power Line Communication in a Smart Microgrid. IEEE Access 7: 21712-21721. doi: 10.1109/ACCESS.2019.2897051. doi: 10.1109/ACCESS.2019.2897051
![]() |
[44] |
Jeong DK, Kim HS, Baek JW, et al. (2018) Autonomous control strategy of DC microgrid for islanding mode using power line communication. Energies 11: 1-22. doi: 10.3390/en11040924. doi: 10.3390/en11040924
![]() |
[45] |
Ustun TS, Khan RH (2015) Multiterminal Hybrid Protection of Microgrids over Wireless Communications Network. IEEE T Smart Grid 6: 2493-2500. doi: 10.1109/TSG.2015.2406886. doi: 10.1109/TSG.2015.2406886
![]() |
[46] |
Ndukwe C, Iqbal MT, Liang X, et al. (2020) LoRa-based communication system for data transfer in microgrids. AIMS Electron Electr Eng 4: 303-325. doi: 10.3934/ElectrEng.2020.3.303. doi: 10.3934/ElectrEng.2020.3.303
![]() |
[47] |
Khatua PK, Ramachandaramurthy VK, Kasinathan P, et al. (2020) Application and assessment of internet of things toward the sustainability of energy systems: Challenges and issues. Sustain Cities Soc 53: 101957. doi: 10.1016/j.scs.2019.101957. doi: 10.1016/j.scs.2019.101957
![]() |
[48] | Nojavanzadeh D, Lotfifard S, Liu Z, et al. (2021) Scale-free Distributed Cooperative Voltage Control of Inverter-based Microgrids with General Time-varying Communication Graphs. IEEE T Power Syst, 1-8. |
[49] |
Cardwell N, Savage S, Anderson T (2000) Modeling TCP latency. Proc IEEE INFOCOM 3: 1742-1751. doi: 10.1109/infcom.2000.832574. doi: 10.1109/infcom.2000.832574
![]() |
[50] | Jacobsson K, Hjalmarsson H, Möller N, et al. (2004) Round trip time estimation in communication networks using adpative Kalman filtering. Regl Conf. |
[51] |
Jiang L, Yao W, Wu QH, et al. (2012) Delay-dependent stability for load frequency control with constant and time-varying delays. IEEE T Power Syst 27: 932-941. doi: 10.1109/TPWRS.2011.2172821. doi: 10.1109/TPWRS.2011.2172821
![]() |
[52] |
Cheng L, Hou ZG, Tan M (2014) A mean square consensus protocol for linear multi-agent systems with communication noises and fixed topologies. IEEE T Automat Contr 59: 261-267. doi: 10.1109/TAC.2013.2270873. doi: 10.1109/TAC.2013.2270873
![]() |
[53] |
Wang Y, Cheng L, Hou ZG, et al. (2015) Consensus seeking in a network of discrete-time linear agents with communication noises. Int J Syst Sci 46: 1874-1888. doi: 10.3182/20140824-6-za-1003.00344. doi: 10.3182/20140824-6-za-1003.00344
![]() |
[54] |
Morita R, Wada T, Masubuchi I, et al. (2016) Multiagent consensus with noisy communication: Stopping rules based on network graphs. IEEE T Control Netw 3: 358-365. doi: 10.1109/TCNS.2015.2481119. doi: 10.1109/TCNS.2015.2481119
![]() |
[55] |
Liu J, Ming P, Li S (2016) Consensus gain conditions of stochastic multi-agent system with communication noise. Int J Control Autom 14: 1223-1230. doi: 10.1007/s12555-014-0360-5. doi: 10.1007/s12555-014-0360-5
![]() |
[56] |
Chaudhuri B, Majumder R, Pal BC (2004) Wide-area measurement-based stabilizing control of power system considering signal transmission delay. IEEE T Power Syst 19: 1971-1979. doi: 10.1109/TPWRS.2004.835669. doi: 10.1109/TPWRS.2004.835669
![]() |
[57] |
Rana MM (2017) Least mean square fourth based microgrid state estimation algorithm using the internet of things technology. PLoS One 12: 1-13. doi: 10.1371/journal.pone.0176099. doi: 10.1371/journal.pone.0176099
![]() |
[58] |
Setiawan MA, Abu-Siada A, Shahnia F (2018) A New Technique for Simultaneous Load Current Sharing and Voltage Regulation in DC Microgrids. IEEE T Ind Inform 14: 1403-1414. doi: 10.1109/TⅡ.2017.2761914. doi: 10.1109/TⅡ.2017.2761914
![]() |
[59] |
Ullah S, Khan L, Sami I, et al. (2021) Consensus-Based Delay-Tolerant Distributed Secondary Control Strategy for Droop Controlled AC Microgrids. IEEE Access 9: 6033-6049. doi: 10.1109/ACCESS.2020.3048723. doi: 10.1109/ACCESS.2020.3048723
![]() |
[60] |
Shuai Z, Huang W, Shen X, et al. (2019) A Maximum Power Loading Factor (MPLF) Control Strategy for Distributed Secondary Frequency Regulation of Islanded Microgrid. IEEE T Power Electron 34: 2275-2291. doi: 10.1109/TPEL.2018.2837125. doi: 10.1109/TPEL.2018.2837125
![]() |
[61] |
Jin D, Li Z, Hannon C, et al. (2017) Toward a Cyber Resilient and Secure Microgrid Using Software-Defined Networking. IEEE T Smart Grid 8: 2494-2504. doi: 10.1109/TSG.2017.2703911. doi: 10.1109/TSG.2017.2703911
![]() |
[62] |
Liu X, Li Z (2017) False Data Attacks Against AC State Estimation with Incomplete Network Information. IEEE T Smart Grid 8: 2239-2248. doi: 10.1109/TSG.2016.2521178. doi: 10.1109/TSG.2016.2521178
![]() |
[63] |
Saha S, Roy TK, Mahmud MA, et al. (2018) Electrical Power and Energy Systems Sensor fault and cyber attack resilient operation of DC microgrids. Int J Elec Power 99: 540-554. doi: 10.1016/j.ijepes.2018.01.007. doi: 10.1016/j.ijepes.2018.01.007
![]() |
[64] | Wu D, Member S, Ci S, et al. (2010) Application-Centric Routing for Video Streaming Over MultiHop Wireless Networks. IEEE T Circ Syst Vid 20: 1721-1734. |
[65] |
Rana MM, Li L, Su SW (2016) Distributed condition monitoring of renewable microgrids using adaptive-then-combine algorith. IEEE Power and Energy Society General Meeting, 1-6. doi: 10.1109/PESGM.2016.7741544. doi: 10.1109/PESGM.2016.7741544
![]() |
[66] |
Zheng L, Lu N, Cai L (2013) Reliable wireless communication networks for demand response control. IEEE T Smart Grid 4: 133-140. doi: 10.1109/TSG.2012.2224892. doi: 10.1109/TSG.2012.2224892
![]() |
[67] |
Zhang R, Hredzak B (2019) Distributed finite-time multiagent control for DC microgrids with time delays. IEEE T Smart Grid 10: 2692-2701. doi: 10.1109/TSG.2018.2808467. doi: 10.1109/TSG.2018.2808467
![]() |
[68] |
Zhou J, Tsai MJ, Cheng PT (2020) Consensus-Based Cooperative Droop Control for Accurate Reactive Power Sharing in Islanded AC Microgrid. IEEE J Em Sel Top P 8: 1108-1116. doi: 10.1109/JESTPE.2019.2946658. doi: 10.1109/JESTPE.2019.2946658
![]() |
[69] | Jacobsson K, Hjalmarsson H, Möller N, et al. (2004) Round trip time estimation in communication networks using adpative Kalman filtering. Reglermöte Conference, 1-5. |
[70] |
Das A, Shukla A, Shyam AB, et al. (2021) A Distributed-Controlled Harmonic Virtual Impedance Loop for AC Microgrids. IEEE T Ind Electron 68: 3949-3961. doi: 10.1109/TIE.2020.2987290. doi: 10.1109/TIE.2020.2987290
![]() |
[71] |
Da Silva WWAG, Oliveira TR, Donoso-Garcia PF (2020) Hybrid Distributed and Decentralized Secondary Control Strategy to Attain Accurate Power Sharing and Improved Voltage Restoration in DC Microgrids. IEEE T Power Electron 35: 6458-6469. doi: 10.1109/TPEL.2019.2951012. doi: 10.1109/TPEL.2019.2951012
![]() |
[72] |
Sharma D, Mishra S (2020) Disturbance-Observer-Based Frequency Regulation Scheme for Low-Inertia Microgrid Systems. IEEE Syst J 14: 782-792. doi: 10.1109/JSYST.2019.2901749. doi: 10.1109/JSYST.2019.2901749
![]() |
[73] |
Prabhakaran P, Goyal Y, Agarwal V (2019) A novel communication-based average voltage regulation scheme for a droop controlled DC microgrid. IEEE T Smart Grid 10: 1250-1258. doi: 10.1109/TSG.2017.2761864. doi: 10.1109/TSG.2017.2761864
![]() |
[74] |
Liu J, Du Y, Yim S, et al. (2020) Steady-State Analysis of Microgrid Distributed Control under Denial of Service Attacks. IEEE J Em Sel Top P 9: 5311-5325. doi: 10.1109/JESTPE.2020.2990879. doi: 10.1109/JESTPE.2020.2990879
![]() |
[75] |
Fu R, Huang X, Sun J, et al. (2017) Stability analysis of the cyber physical microgrid system under the intermittent DoS attacks. Energies 10: 1-15. doi: 10.3390/en10050680. doi: 10.3390/en10050680
![]() |
[76] |
Danzi P, Angjelichinoski M, Stefanovic C, et al. (2018) Software-Defined Microgrid Control for Resilience Against Denial-of-Service Attacks. IEEE T Smart Grid 10: 5258-5268. doi: 10.1109/TSG.2018.2879727. doi: 10.1109/TSG.2018.2879727
![]() |
[77] |
Ding L, Han QL, Ning B, et al. (2020) Distributed Resilient Finite-Time Secondary Control for Heterogeneous Battery Energy Storage Systems under Denial-of-Service Attacks. IEEE T Ind Inform 16: 4909-4919. doi: 10.1109/TII.2019.2955739. doi: 10.1109/TII.2019.2955739
![]() |
[78] |
Mustafa A, Poudel B, Bidram A, et al. (2020) Detection and Mitigation of Data Manipulation Attacks in AC Microgrids. IEEE T Smart Grid 11: 2588-2603. doi: 10.1109/TSG.2019.2958014. doi: 10.1109/TSG.2019.2958014
![]() |
[79] |
Poudel BP, Mustafa A, Bidram A, et al. (2020) Detection and mitigation of cyber-threats in the DC microgrid distributed control system. Int J Elec Power 120: 105968. doi: 10.1016/j.ijepes.2020.105968. doi: 10.1016/j.ijepes.2020.105968
![]() |
[80] |
Ghiasi M, Dehghani M, Niknam T, et al. (2021) Cyber-Attack Detection and Cyber-Security Enhancement in Smart DC-Microgrid Based on Blockchain Technology and Hilbert Huang Transform. IEEE Access 9: 29429-29440. doi: 10.1109/ACCESS.2021.3059042. doi: 10.1109/ACCESS.2021.3059042
![]() |
[81] |
Islam SN, Mahmud MA, Oo AMT (2018) Impact of optimal false data injection attacks on local energy trading in a residential microgrid. ICT Express 4: 30-34. doi: 10.1016/j.icte.2018.01.015. doi: 10.1016/j.icte.2018.01.015
![]() |
[82] |
Beg OA, Nguyen LV, Johnson TT, et al. (2019) Signal Temporal Logic-Based Attack Detection in DC Microgrids. IEEE T Smart Grid 10: 3585-3595. doi: 10.1109/TSG.2018.2832544. doi: 10.1109/TSG.2018.2832544
![]() |
[83] |
Yassaie N, Hallajiyan M, Sharifi I, et al. (2021) Resilient control of multi-microgrids against false data injection attack. ISA T 110: 238-246. doi: 10.1016/j.isatra.2020.10.030. doi: 10.1016/j.isatra.2020.10.030
![]() |
[84] |
Mahmood H, Mahmood D, Shaheen Q, et al. (2021) S-DPs: An SDN-based DDoS protection system for smart grids. Secur Commun Netw. doi: 10.1155/2021/6629098. doi: 10.1155/2021/6629098
![]() |
[85] |
Rokrok E, Shafie-khah M, Catalão JPS (2018) Review of primary voltage and frequency control methods for inverter-based islanded microgrids with distributed generation. Renewable and Sustainable Energy Reviews 82: 3225-3235. doi: 10.1016/j.rser.2017.10.022. doi: 10.1016/j.rser.2017.10.022
![]() |
[86] |
Han H, Liu Y, Sun Y, et al. (2015) An Improved Droop Control Strategy for Reactive Power Sharing in Islanded Microgrid. IEEE T Power Electr 30: 3133-3141. doi: 10.1109/TPEL.2014.2332181. doi: 10.1109/TPEL.2014.2332181
![]() |
[87] |
Ahumada C, Cárdenas R, Sáez D, et al. (2016) Secondary Control Strategies for Frequency Restoration in Islanded Microgrids With Consideration of Communication Delays. IEEE T Smart Grid 7: 1430-1441. doi: 10.1109/TSG.2015.2461190. doi: 10.1109/TSG.2015.2461190
![]() |
[88] |
Sheng W, Hong Y, Wu M, et al. (2020) A cooperative control scheme for AC/DC hybrid autonomous microgrids. Processes 8: 1-15. doi: 10.3390/pr8030311. doi: 10.3390/pr8030311
![]() |
[89] |
Ma J, Wang X, Liu J, et al. (2019) An improved droop control method for voltage-source inverter parallel systems considering line impedance differences. Energies 12: 1158. doi: 10.3390/en12061158. doi: 10.3390/en12061158
![]() |
[90] |
Sreekumar P, Khadkikar V (2015) A New Virtual Harmonic Impedance Scheme for Harmonic Power Sharing in an Islanded Microgrid. IEEE T Power Deliver 31: 936-945. doi: 10.1109/TPWRD.2015.2402434. doi: 10.1109/TPWRD.2015.2402434
![]() |
[91] |
Mahmood H, Michaelson D, Jiang J (2015) Reactive Power Sharing in Islanded Microgrids Using Adaptive Voltage Droop Control. IEEE T Smart Grid 6: 3052-3060. doi: 10.1109/TSG.2015.2399232. doi: 10.1109/TSG.2015.2399232
![]() |
[92] |
Khan MRB, Jidin R, Pasupuleti J (2016) Multi-agent based distributed control architecture for microgrid energy management and optimization. Energy Convers Manag 112: 288-307. doi: 10.1016/j.enconman.2016.01.011. doi: 10.1016/j.enconman.2016.01.011
![]() |
[93] |
Li Q, Chen F, Chen M, et al. (2016) Agent-Based Decentralized Control Method for Islanded Microgrids. IEEE T Smart Grid 7: 637-649. doi: 10.1109/TSG.2015.2422732. doi: 10.1109/TSG.2015.2422732
![]() |
[94] |
Zhang H, Kim S, Sun Q, et al. (2017) Distributed Adaptive Virtual Impedance Control for Accurate Reactive Power Sharing Based on Consensus Control in Microgrids. IEEE T Smart Grid 8: 1749-1761. doi: 10.1109/TSG.2015.2506760. doi: 10.1109/TSG.2015.2506760
![]() |
[95] | Olfati-Saber R, Murray RM (2004) Consensus problems in networks of agents with switching topology and time-delays. IEEE T Automat Contr 49: 1520-1533. |
[96] |
Sugie T, Anderson BDO, Sun Z, et al. (2018) On a hierarchical control strategy for multi-agent formation without reflection. Proceedings of the IEEE Conference on Decision and Control, 2023-2028. doi: 10.1109/CDC.2018.8619404. doi: 10.1109/CDC.2018.8619404
![]() |
[97] |
Ajorlou A, Aghdam AG (2013) Connectivity preservation in nonholonomic multi-agent systems: A bounded distributed control strategy. IEEE T Automat Contr 58: 2366-2371. doi: 10.1109/TAC.2013.2251792. doi: 10.1109/TAC.2013.2251792
![]() |
[98] |
Dou CX, Liu B (2013) Multi-agent based hierarchical hybrid control for smart microgrid. IEEE T Smart Grid 4: 771-778. doi: 10.1109/TSG.2012.2230197. doi: 10.1109/TSG.2012.2230197
![]() |
[99] |
Liu W, Gu W, Sheng W, et al. (2014) Decentralized multi-agent system-based cooperative frequency control for autonomous microgrids with communication constraints. IEEE T Sustain Energy 5: 446-456. doi: 10.1109/TSTE.2013.2293148. doi: 10.1109/TSTE.2013.2293148
![]() |
[100] |
Nguyen TL, Tran QT, Caire R, et al. (2017) Agent based distributed control of islanded microgrid-Real-time cyber-physical implementation. 2017 IEEE PES Innovative Smart Grid Technologies Conference Europe, ISGT-Europe 2017 - Proceedings, 1-6. doi: 10.1109/ISGTEurope.2017.8260275. doi: 10.1109/ISGTEurope.2017.8260275
![]() |
[101] | Yao J, Yang S, Wang K, et al. (2014) Framework for Future Smart Grid Operation and Control with Source-Grid-Load Interaction. IFAC Proceedings Volumes 47: 2788-2793. |
[102] |
Han R, Meng L, Ferrari-Trecate G, et al. (2017) Containment and Consensus-Based Distributed Coordination Control to Achieve Bounded Voltage and Precise Reactive Power Sharing in Islanded AC Microgrids. IEEE T Ind Appl 53: 5187-5199. doi: 10.1109/TIA.2017.2733457. doi: 10.1109/TIA.2017.2733457
![]() |
[103] |
Dehkordi NM, Baghaee HR, Sadati N, et al. (2019) Distributed Noise-Resilient Secondary Voltage and Frequency Control for Islanded Microgrids. IEEE T Smart Grid 10: 3780-3790. doi: 10.1109/TSG.2018.2834951. doi: 10.1109/TSG.2018.2834951
![]() |
[104] |
Badal FR, Das P, Sarker SK, et al. (2019) A survey on control issues in renewable energy integration and microgrid. Prot Control Mod Power Syst 4: 1-27. doi: 10.1186/s41601-019-0122-8. doi: 10.1186/s41601-019-0122-8
![]() |
[105] |
Nair UR (2020) A Model Predictive Control-Based Energy Management Scheme for Hybrid Storage System in Islanded Microgrids. IEEE Access 8: 97809-97822. doi: 10.1109/ACCESS.2020.2996434. doi: 10.1109/ACCESS.2020.2996434
![]() |
[106] |
Parisio A, Rikos E, Tzamalis G, et al. (2014) Use of model predictive control for experimental microgrid optimization. Appl Energy 115: 37-46. doi: 10.1016/j.apenergy.2013.10.027. doi: 10.1016/j.apenergy.2013.10.027
![]() |
[107] |
Verma AK, Gooi HB, Ukil A, et al. (2017) Microgrid frequency stabilization using model predictive controller. 2016 IEEE PES Transm Distrib Conf Expo Am PES T D-LA, 1-6. doi: 10.1109/TDC-LA.2016.7805637. doi: 10.1109/TDC-LA.2016.7805637
![]() |
[108] |
Sarkar SK, Badal FR, Das SK, et al. (2017) Discrete time model predictive controller design for voltage control of an islanded microgrid. 3rd Int Conf Electr Inf Commun Technol EICT, 1-6. doi: 10.1109/EICT.2017.8275162. doi: 10.1109/EICT.2017.8275162
![]() |
[109] |
Lou G, Gu W, Sheng W, et al. (2018) Distributed model predictive secondary voltage control of islanded microgrids with feedback linearization. IEEE Access 6: 50169-50178. doi: 10.1109/ACCESS.2018.2869280. doi: 10.1109/ACCESS.2018.2869280
![]() |
[110] |
Sarker SK, Badal FR, Das P, et al. (2019) Multivariable integral linear quadratic Gaussian robust control of islanded microgrid to mitigate voltage oscillation for improving transient response. Asian J Control 21: 2114-2125. doi: 10.1002/asjc.2215. doi: 10.1002/asjc.2215
![]() |
[111] | Vandoorn T, Renders B, Degroote L, et al. (2010) Voltage control in islanded microgrids by means of a linear-quadratic regulator. Proc IEEE Benelux Young Researchers Symposium in Electrical Power Engineering (YRS10). |
[112] |
Rahman M, Sarkar SK, Das SK, et al. (2018) A comparative study of LQR, LQG, and integral LQG controller for frequency control of interconnected smart grid. 3rd Int Conf Electr Inf Commun Technol EICT, 1-6. doi: 10.1109/EICT.2017.8275216. doi: 10.1109/EICT.2017.8275216
![]() |
[113] |
Sedhom BE, Hatata AY, El-Saadawi MM, et al. (2019) Robust adaptive H-infinity based controller for islanded microgrid supplying non-linear and unbalanced loads. IET Smart Grid 2: 420-435. doi: 10.1049/iet-stg.2019.0024. doi: 10.1049/iet-stg.2019.0024
![]() |
[114] |
Vandoorn TL, Ionescu CM, De Kooning JDM, et al. (2013) Theoretical analysis and experimental validation of single-phase direct versus cascade voltage control in islanded microgrids. IEEE T Ind Electron 60: 789-798. doi: 10.1109/TIE.2012.2205362. doi: 10.1109/TIE.2012.2205362
![]() |
[115] |
Singh A, Suhag S (2020) Frequency regulation in an AC microgrid interconnected with thermal system employing multiverse-optimised fractional order-PID controller. Int J Sustain Energy 39: 250-262. doi: 10.1080/14786451.2019.1684286. doi: 10.1080/14786451.2019.1684286
![]() |
[116] |
Singh A, Suhag S (2019) Frequency Regulation in AC Microgrid with and without Electric Vehicle Using Multiverse-Optimized Fractional Order- PID controller. Int J Comput Digit Syst 8: 375-385. doi: 10.12785/ijcds/080406. doi: 10.12785/ijcds/080406
![]() |
[117] |
Sarkar SK, Badal FR, Das SK (2018) A comparative study of high performance robust PID controller for grid voltage control of islanded microgrid. Int J Dyn Control 6: 1207-1217. doi: 10.1007/s40435-017-0364-0. doi: 10.1007/s40435-017-0364-0
![]() |
[118] |
Mongkoltanatas J, Riu D, Lepivert X (2013) H infinity controller design for primary frequency control of energy storage in islanding MicroGrid. 2013 15th Eur Conf Power Electron Appl (EPE), 1-11. doi: 10.1109/EPE.2013.6634714. doi: 10.1109/EPE.2013.6634714
![]() |
[119] |
Bevrani H, Feizi MR, Ataee S (2016) Robust Frequency Control in an Islanded Microgrid: H∞ and μ-Synthesis Approaches. IEEE T Smart Grid 7: 706-717. doi: 10.1109/TSG.2015.2446984. doi: 10.1109/TSG.2015.2446984
![]() |
[120] |
Kerdphol T, Rahman FS, Mitani Y, et al. (2018) Robust Virtual Inertia Control of an Islanded Microgrid Considering High Penetration of Renewable Energy. IEEE Access 6: 625-636. doi: 10.1109/ACCESS.2017.2773486. doi: 10.1109/ACCESS.2017.2773486
![]() |
[121] |
Krishna Metihalli B, Narayana Sabhahit J (2021) Disturbance Observer Based Distributed Consensus Control Strategy of Multi-Agent System with External Disturbance in a Standalone DC Microgrid. Asian J Control 23: 920-936. doi: 10.1002/asjc.2287. doi: 10.1002/asjc.2287
![]() |
[122] |
Keshta HE, Ali AA, Saied EM, et al. (2019) Real-time operation of multi-micro-grids using a multi-agent system. Energy 174: 576-590. doi: 10.1016/j.energy.2019.02.145. doi: 10.1016/j.energy.2019.02.145
![]() |
1. | Hamdi Abdi, A Brief Review of Microgrid Surveys, by Focusing on Energy Management System, 2022, 15, 2071-1050, 284, 10.3390/su15010284 |
Technology | Coverage [m] | Data Rate | Frequency | Applications in Microgrids | ||
Wired | PLC | NB | 150,000 | 10-500 Kbps | 1.8-250 MHz | Data communication for freq. control Islanding control in DC MGs. [43], [44] |
Ethernet | IEEE 802.3 | 100-300 | 10 Mbps | 2.4 GHz | Information communication between DGs. [37], Monitoring and control of energy meters [38] |
|
Fibre Optics | PON | 10,000-60,000 | 100 Mbps-2.5Gbps | 3 x 1011 - 4 x 1014 Hz | Metering communication [39] | |
Wireless | Bluetooth | IEEE 802.15 | 15-30 | 1-2 Mb/s | 2.4 GHz | Data communication [40] |
Wi-Fi | IEEE 802.11 | 100 | 54 Mb/s | 2.4/5.9 GHz | Information sensing and data communication [41] | |
ZigBee/6LoWPAN | IEEE 802.15.4 | 30-50 | 250 Kbps | 868 MHz, 915 MHz, 2.4 GHz | Data communications. [42] | |
Cellular | GSM | 1000-10,000 | 14.4 Kbps | 0.9, 1.8, 2.1, 2.4 GHz | Data communications. [40] | |
WiMAX | 10,000-50,000 | 75 Mb/s | 2-11 GHz | Protection relay communications [45]. Monitoring and control of energy meters [38]. |
||
LPWAN | LoRa | 10,000-15,000 | 0.3-37.5 Kbps | 433/868/915 MHz | Data Transfer. [46] | |
SIGFOX | 30,000-50,000 | 0.1 Kbps | 902–928 MHz | Data Transfer. [47] |
References | Packet Loss Description | Mitigation Approaches |
[23] | Bernoulli random process was used to model packet losses to produce a probability of 0 or 1 | The control method uses the previously measured value at intervals when the information packet is lost. |
[67], [68] | A single point failure was used to test the redundancy of the sparse com graph used. | Distributed communication topology: The communication graph was designed with minimum redundancy. |
[48] | Communication link failure between DGs in the microgrid network. | The DG that lost communication operates based on the droop controller and the latest control command received. |
[9], [65] | Bernoulli random process | Loss estimation was carried out |
Cyber Attack Description | Attack Detection Technique | Impacts of Cyber Attacks | Mitigation Approach |
Data Manipulation | Kullback-Liebler (KL) divergence-based criterion [78], [79] | Degrades Frequency and voltage control of islanded MGs [78], [79] | Calculated KL divergence factors to determine compromise [78], [79] |
False data injection | *Calculates Earnings based on energy [81] purchased and supplied. * Hilbert-Huang transform [80] *Signal temporal logic. [82] |
*Financial Loss [81] * consensus protocols interruptions [80] *Power sharing compromise. [82] * Voltage regulation compromise. [82] |
* Blockchain-based data exchange layout. [81]. * weighted mean subsequence reduced algorithm. [83] |
Denial of service | *ACK-based detection [77] * Entropy-based. [84] *Signal temporal logic. [82] |
*Communication delay and system oscillations. [75] *Blockage of communication channel [76], [77] *Variation in communication graph. [74]. |
*Separation of the data plane from the network control plane. [76] *Distributed averaging proportional-integral control. [74] *Communication recovery mechanism. [77]. |
Control Method | Strengths | Weaknesses |
Droop-Based [86], [89], [90], and [91] |
Take local measurement | Poor performance under Nonlinear loads |
Low cost | Weak in handling high RES penetration | |
Suitable for both grid and island mode connection | Poor control of power-sharing under uncertain loads | |
A Simple controller and easy to use | ||
Multi Agent-Based [93], [98], [99] and [100] |
Suitable for both grid and island mode connection | Dependent on communication network for smooth operation. |
Suitable for MG with large penetration of RES | Can be impacted by communication link failure | |
Very effective in islanded MG control | ||
Cost-effective | ||
Consensus-Based [94], [101], [102], and [103]. |
Suitable for both grid and island mode connection | Dependent on communication network for smooth operation. |
Good scalability Properties | It's sensitive to communication failure | |
If the algorithm is not optimized, it could be time-consuming | ||
MPC-Based [87], [105], [106], [107], [108], and [109] |
Suitable for both grid and island mode connection | It cannot be used in uncertain systems |
Easy to tune | It could be complex to implement | |
Good at handling multivariable control problems | ||
LQG-Based [110], [113], and [111]. |
Suitable for both grid and island mode connection | Surfers from lack of robustness against changing plant dynamics |
PID-Based [7], [114]. [115], [116], and [117], |
Easy to design | Poor control of power sharing |
Fast transient response | Cannot effectively handle high RES penetration | |
Robust performance in the presence of un-modelled loads, dynamic loads, nonlinear loads and harmonic loads. | ||
H-infinity based [118]. [119], and [120] |
Could offer precise reference frequency tracking. | Depends on the norms minimization and order of the system |
Suitable for both grid and island mode connection | May require advanced digital signal processing to control the performance of MG |
Technology | Coverage [m] | Data Rate | Frequency | Applications in Microgrids | ||
Wired | PLC | NB | 150,000 | 10-500 Kbps | 1.8-250 MHz | Data communication for freq. control Islanding control in DC MGs. [43], [44] |
Ethernet | IEEE 802.3 | 100-300 | 10 Mbps | 2.4 GHz | Information communication between DGs. [37], Monitoring and control of energy meters [38] |
|
Fibre Optics | PON | 10,000-60,000 | 100 Mbps-2.5Gbps | 3 x 1011 - 4 x 1014 Hz | Metering communication [39] | |
Wireless | Bluetooth | IEEE 802.15 | 15-30 | 1-2 Mb/s | 2.4 GHz | Data communication [40] |
Wi-Fi | IEEE 802.11 | 100 | 54 Mb/s | 2.4/5.9 GHz | Information sensing and data communication [41] | |
ZigBee/6LoWPAN | IEEE 802.15.4 | 30-50 | 250 Kbps | 868 MHz, 915 MHz, 2.4 GHz | Data communications. [42] | |
Cellular | GSM | 1000-10,000 | 14.4 Kbps | 0.9, 1.8, 2.1, 2.4 GHz | Data communications. [40] | |
WiMAX | 10,000-50,000 | 75 Mb/s | 2-11 GHz | Protection relay communications [45]. Monitoring and control of energy meters [38]. |
||
LPWAN | LoRa | 10,000-15,000 | 0.3-37.5 Kbps | 433/868/915 MHz | Data Transfer. [46] | |
SIGFOX | 30,000-50,000 | 0.1 Kbps | 902–928 MHz | Data Transfer. [47] |
References | Packet Loss Description | Mitigation Approaches |
[23] | Bernoulli random process was used to model packet losses to produce a probability of 0 or 1 | The control method uses the previously measured value at intervals when the information packet is lost. |
[67], [68] | A single point failure was used to test the redundancy of the sparse com graph used. | Distributed communication topology: The communication graph was designed with minimum redundancy. |
[48] | Communication link failure between DGs in the microgrid network. | The DG that lost communication operates based on the droop controller and the latest control command received. |
[9], [65] | Bernoulli random process | Loss estimation was carried out |
Cyber Attack Description | Attack Detection Technique | Impacts of Cyber Attacks | Mitigation Approach |
Data Manipulation | Kullback-Liebler (KL) divergence-based criterion [78], [79] | Degrades Frequency and voltage control of islanded MGs [78], [79] | Calculated KL divergence factors to determine compromise [78], [79] |
False data injection | *Calculates Earnings based on energy [81] purchased and supplied. * Hilbert-Huang transform [80] *Signal temporal logic. [82] |
*Financial Loss [81] * consensus protocols interruptions [80] *Power sharing compromise. [82] * Voltage regulation compromise. [82] |
* Blockchain-based data exchange layout. [81]. * weighted mean subsequence reduced algorithm. [83] |
Denial of service | *ACK-based detection [77] * Entropy-based. [84] *Signal temporal logic. [82] |
*Communication delay and system oscillations. [75] *Blockage of communication channel [76], [77] *Variation in communication graph. [74]. |
*Separation of the data plane from the network control plane. [76] *Distributed averaging proportional-integral control. [74] *Communication recovery mechanism. [77]. |
Control Method | Strengths | Weaknesses |
Droop-Based [86], [89], [90], and [91] |
Take local measurement | Poor performance under Nonlinear loads |
Low cost | Weak in handling high RES penetration | |
Suitable for both grid and island mode connection | Poor control of power-sharing under uncertain loads | |
A Simple controller and easy to use | ||
Multi Agent-Based [93], [98], [99] and [100] |
Suitable for both grid and island mode connection | Dependent on communication network for smooth operation. |
Suitable for MG with large penetration of RES | Can be impacted by communication link failure | |
Very effective in islanded MG control | ||
Cost-effective | ||
Consensus-Based [94], [101], [102], and [103]. |
Suitable for both grid and island mode connection | Dependent on communication network for smooth operation. |
Good scalability Properties | It's sensitive to communication failure | |
If the algorithm is not optimized, it could be time-consuming | ||
MPC-Based [87], [105], [106], [107], [108], and [109] |
Suitable for both grid and island mode connection | It cannot be used in uncertain systems |
Easy to tune | It could be complex to implement | |
Good at handling multivariable control problems | ||
LQG-Based [110], [113], and [111]. |
Suitable for both grid and island mode connection | Surfers from lack of robustness against changing plant dynamics |
PID-Based [7], [114]. [115], [116], and [117], |
Easy to design | Poor control of power sharing |
Fast transient response | Cannot effectively handle high RES penetration | |
Robust performance in the presence of un-modelled loads, dynamic loads, nonlinear loads and harmonic loads. | ||
H-infinity based [118]. [119], and [120] |
Could offer precise reference frequency tracking. | Depends on the norms minimization and order of the system |
Suitable for both grid and island mode connection | May require advanced digital signal processing to control the performance of MG |