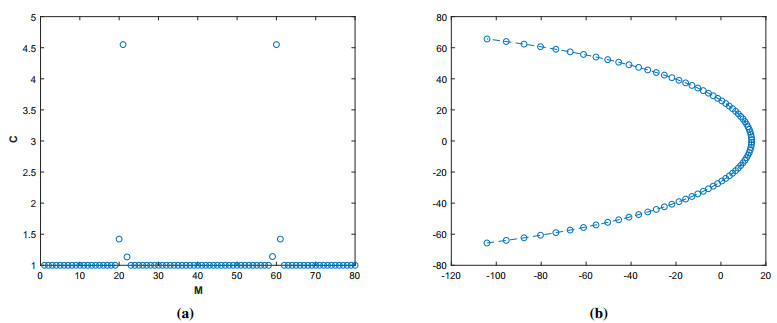
Researchers have proposed applying optimization techniques to improve performance of microstrip antennas (MSAs) in terms of bandwidth, radiation characteristics, polarization, directivity and size. The drawbacks of the conventional MSAs can be overcome by optimizing the antenna parameters while keeping a compact configuration. Applying a global optimizer is a better technique than using a local optimizer or a trial and error method for performance enhancement. This paper discusses genetic algorithm (GA) optimization of microstrip antennas presented by the antenna research community. The GA optimization procedure, antenna parameters optimized by using GA and the optimization objectives are presented by reviewing the literature. Further, evolution of GA in the field of MSAs and its significance are explored. Application of GA optimization to design broadband, multiband, high-directivity and miniature antennas is demonstrated with the support of several case studies giving an insight for further developments in the field.
Citation: Jeevani W. Jayasinghe. Application of Genetic Algorithm for Binary Optimization of Microstrip Antennas: A Review[J]. AIMS Electronics and Electrical Engineering, 2021, 5(4): 315-333. doi: 10.3934/electreng.2021016
[1] | Zihan Yue, Wei Jiang, Boying Wu, Biao Zhang . A meshless method based on the Laplace transform for multi-term time-space fractional diffusion equation. AIMS Mathematics, 2024, 9(3): 7040-7062. doi: 10.3934/math.2024343 |
[2] | Farman Ali Shah, Kamran, Zareen A Khan, Fatima Azmi, Nabil Mlaiki . A hybrid collocation method for the approximation of 2D time fractional diffusion-wave equation. AIMS Mathematics, 2024, 9(10): 27122-27149. doi: 10.3934/math.20241319 |
[3] | Xiangmei Li, Kamran, Absar Ul Haq, Xiujun Zhang . Numerical solution of the linear time fractional Klein-Gordon equation using transform based localized RBF method and quadrature. AIMS Mathematics, 2020, 5(5): 5287-5308. doi: 10.3934/math.2020339 |
[4] | Chao Wang, Fajie Wang, Yanpeng Gong . Analysis of 2D heat conduction in nonlinear functionally graded materials using a local semi-analytical meshless method. AIMS Mathematics, 2021, 6(11): 12599-12618. doi: 10.3934/math.2021726 |
[5] | Fouad Mohammad Salama, Nur Nadiah Abd Hamid, Norhashidah Hj. Mohd Ali, Umair Ali . An efficient modified hybrid explicit group iterative method for the time-fractional diffusion equation in two space dimensions. AIMS Mathematics, 2022, 7(2): 2370-2392. doi: 10.3934/math.2022134 |
[6] | Xiangyun Qiu, Xingxing Yue . Solving time fractional partial differential equations with variable coefficients using a spatio-temporal meshless method. AIMS Mathematics, 2024, 9(10): 27150-27166. doi: 10.3934/math.20241320 |
[7] | Bin Fan . Efficient numerical method for multi-term time-fractional diffusion equations with Caputo-Fabrizio derivatives. AIMS Mathematics, 2024, 9(3): 7293-7320. doi: 10.3934/math.2024354 |
[8] | Bin He . Developing a leap-frog meshless methods with radial basis functions for modeling of electromagnetic concentrator. AIMS Mathematics, 2022, 7(9): 17133-17149. doi: 10.3934/math.2022943 |
[9] | Hijaz Ahmad, Muhammad Nawaz Khan, Imtiaz Ahmad, Mohamed Omri, Maged F. Alotaibi . A meshless method for numerical solutions of linear and nonlinear time-fractional Black-Scholes models. AIMS Mathematics, 2023, 8(8): 19677-19698. doi: 10.3934/math.20231003 |
[10] | Sayed Saifullah, Amir Ali, Zareen A. Khan . Analysis of nonlinear time-fractional Klein-Gordon equation with power law kernel. AIMS Mathematics, 2022, 7(4): 5275-5290. doi: 10.3934/math.2022293 |
Researchers have proposed applying optimization techniques to improve performance of microstrip antennas (MSAs) in terms of bandwidth, radiation characteristics, polarization, directivity and size. The drawbacks of the conventional MSAs can be overcome by optimizing the antenna parameters while keeping a compact configuration. Applying a global optimizer is a better technique than using a local optimizer or a trial and error method for performance enhancement. This paper discusses genetic algorithm (GA) optimization of microstrip antennas presented by the antenna research community. The GA optimization procedure, antenna parameters optimized by using GA and the optimization objectives are presented by reviewing the literature. Further, evolution of GA in the field of MSAs and its significance are explored. Application of GA optimization to design broadband, multiband, high-directivity and miniature antennas is demonstrated with the support of several case studies giving an insight for further developments in the field.
Recently, partial differential equations (PDEs) with fractional derivatives have gained significant attention of the research community in applied sciences and engineering. Such equations are encountered in various applications (continuum mechanics, gas dynamics, hydrodynamics, heat and mass transfer, wave theory, acoustics, multiphase flows, chemical engineering, etc.). Numerous phenomenon in Chemistry, Physics, Biology, Finance, Economics and other relevant fields can be modelled using PDEs of fractional order [1,2,3,4,5]. In literature a significant theoretical work on the explicit solution of fractional order differential equations can be found [6,7] and there references. Since the explicit solutions can be obtained for special cases and most of the time the exact/analytical solutions are cumbersome for differential equations of non-integer order, therefore an alternative way is to find the solutions numerically. Various computational methods have been developed for approximation of differential equations of fractional order. The authors in [8], for example, have analyzed the implicit finite difference method and proved its unconditional convergence and stability. In [9] approximate solution of fractional diffusion equation is obtained via compact finite difference scheme. Liu et al.[10] studied the sub-diffusion equation having non-linear source term using analytical and numerical techniques. In [11] a numerical scheme for the solution of turbulent Riesz type diffusion equation is presented. The authors in [12] have solved diffusion-wave equations of fractional order using a compact finite difference method which is based on its equivalent integro-differential form. Garg et al.[13] utilized the matrix method for approximation of space-time wave-diffusion equation of non-integer order. In [14] the authors solved multi-term wave-diffusion equation of fractional order via Galerkin spectral method and a high order difference scheme. Two finite difference methods for approximating wave-diffusion equations are proposed in [15]. Bhrawy et al. [16] utilized Jacobi operational matrix based spectral tau algorithm for numerical solution of diffusion-wave equation of non-integer order. In [4] the authors proposed numerical schemes for approximating the multi-term wave-diffusion equation. The Legendre wavelets scheme for diffusion wave equations is proposed in [17]. The authors [18] presented a numerical scheme which is based on alternating direction implicit method and compact difference method for 2-D wave-diffusion equations. Similarly a compact difference scheme [19] is utilized for approximation of 1-D and 2-D diffusion-wave equations. Yang et al. [20] proposed a fractional multi-step method for the approximation of wave-diffusion equation of non-integer order. A spectral collocation method and its convergence analysis are presented in [21] for fractional wave-diffusion equation.
Since all these methods are mesh dependent and in modern problems these methods have been facing difficulties due to complicated geometries. Meshfree methods, as an alternative numerical method have attracted the researchers. Some meshless methods have been devoloped such as element-free Galerkin method(EFG)[22], reproducing kernel particle method (RKPM)[23], singular boundary method [24], the boundary particle method [25], Local radial point interpolation method (MLRPI) [26] and so on.
Numerous meshless methods have been developed for the approximation of fractional PDEs. Dehghan et al. [27] analyzed a meshless scheme for approximation of diffusion-wave equation of non-integer order and proved its stability and convergence. In [28] the authors presented an implicit meshless scheme for approximation of anomalous sub-diffusion equation. Diffusion equations of fractional orders are apprximated via RBF based implicit meshless method in [29]. Hosseini et al. [26] developed a local radial point interpolation meshless method based on the Galerkin weak form for numerical solution of wave-diffusion equation of non integer order. In [30] the authors approximated distributed order diffusion-wave equation of fractional order using meshless method. The authors in [31] proposed a meshless point collocation method for approximation of 2−D multi-term wave-diffusion equations. In [32] the authors proposed a local meshless method for time fractional diffusion-wave equation. Kansa method [33] is utilized for numerical solution of fractional diffusion equations. Zhuang et al. [34] proposed an implicit MLS meshless method for time fractional advection diffusion equation. The numerical solution of 2D wave-diffusion equation is studied in [35] using implicit MLS meshless method. The mentioned methods are meshfree time stepping methods and these methods faces stability restriction in time, and in these methods for convergence a very small step size is required. To overcome the issue of time instability some transformations may be used.
In literature some valuable work is available on resolving the problem of time instability. The researchers have coupled the Laplace transform with other well known numerical methods. For example the Laplace transform with Kansa method [33,36], finite element method [37,38], finite difference method [39], RBF method on unit sphere [40] and the references therein. In the present work we have coupled the Laplace transform with local meshless method for approximating the solution of the multi-term diffusion wave equation of fractional order.
In our numerical scheme we transform the multi-term time fractional wave-diffusion equation to a time independent problem with Laplace transformation. The reduced problem is then approximated using local meshless method in Laplace space. Finally the solution of the original problem is obtained using contour integration. We apply the proposed method to multi-term fractional wave-diffusion equation of the form [14]
Pα,α1,α2,...,αm(Dτ)U(χ,τ)=KLU(χ,τ)+f(χ,τ),forχ∈Ω,K∈Rτ>0, | (2.1) |
where
Pα,α1,α2,...,αm(Dτ)=Dατ+m∑j=1djDαjτ, |
1<αm<...<α1<α<2, and dj≥0,j=1,2,...,m,m∈N are constants. Dαjτ is a Caputo derivative of order αj defined by
Dαjτf(τ)=1Γ(n−αj)∫τaf(n)(ν)dν(τ−ν)αj+1−n,forn−1<αj<n,n∈N, | (2.2) |
also for n=2, we have
Dαjτf(τ)=1Γ(n−αj)∫τa∂2f(ν)∂ν2dν(τ−ν)αj−1,forαj∈(1,2). | (2.3) |
The initial conditions for the above Eq (2.1) are
U(χ,0)=U0(χ),∂U(χ,0)∂τ=U1(χ). | (2.4) |
and the boundary conditions are
BU(χ,τ)=q(χ,τ),χ∈∂Ω, | (2.5) |
where L is the governing linear differential operator, and B is the boundary differential operator. By applying the Laplace transformation to Eq (2.1), we get
ˆU(χ,s)=W(s;L)ˆg(χ,s), | (2.6) |
where
W(s;L)=(sαI+sα1I+...+sαmI−KL)−1, |
and
ˆg(χ,s)=sα−1U0(χ)+sα−2U1(χ)+sα1−1U0(χ)+sα1−2U1(χ)+...+sαm−1U0(χ)+sαm−2U1(χ)+ˆf(χ,s). |
Similarly applying the Laplace transform to (2.5), we get
BˆU(χ,s)=ˆq(χ,s), | (2.7) |
Hence, the system of time-independent equations is obtained as
ˆU(χ,s)=W(s;L)ˆg(χ,s), | (2.8) |
BˆU(χ,s)=ˆq(χ,s), | (2.9) |
In our method first we represent the solution U(χ,τ) of the original problem (2.1) as a contour integral
U(χ,τ)=12πi∫ΓesτˆU(χ,s)ds, | (2.10) |
where, for Res≥ω with ω appropriately large, and Γ is an initially appropriately chosen line Γ0 perpendicular to the real axis in the complex plane, with Ims→±∞. The integral (2.10) is just the inverse transform of ˆU(χ,τ), with the condition that ˆU(χ,τ) must be analytic to the right of Γ0. To make sure the contour of integration remains in the domain of analyticity of ˆU(χ,τ), we select Γ as a deformed contour in the set ΣΥϕ={s≠0:|args|<ϕ}∪{0}, which behaves as a pair of asymptotes in the left half plane, with Res→−∞ when Ims→±∞, which force esτ to decay towards both ends of Γ. In our work we have used two types of contours, the first contour is the hyperbolic contour Γ1 due to [38] with parametric representation
s(ξ)=Υ+ℶ(1−sin(η−ιξ)),ξ∈R,(Γ1) | (2.11) |
where,
ℶ>0,0<η<ϕ−π2,andΥ>0. | (2.12) |
By writing s=x+ιy, we observe that Γ1 is the left branch of the hyperbola
(x−Υ−ℶℶsinη)2−(yℶcosη)2=1, | (2.13) |
the asymptotes for (2.13) are y=±(x−Υ−ℶ)cotη, and x-intercept at s=Υ+ℶ(1−sinη). The condition (2.12) confirms that Γ1 lies in the sector ΣΥϕ=Υ+Σϕ⊂Σϕ, and grows into the left half plane. From (2.11) and (2.10), we have the following integral
U(χ,τ)=12πi∫∞−∞es(ξ)τˆU(χ,s(ξ))ˊs(ξ)dξ. | (2.14) |
Finally to approximate Eq (2.14), the trapezoidal rule with step k is used as
Uk(χ,τ)=k2πiM∑j=−MesjτˆU(χ,sj)ˊsj,forξj=jk,sj=s(ξj),s′j=s′(ξj). | (2.15) |
The second contour employed in this work is the Talbot's contour [41], though ignored by many researchers, yet it is one of the best method for numerical inverting the Laplace transform [42]. The authors in [43] have optimized the Talbot's contour for approximating the solution of parabolic PDEs. Other works on Talbot's method can be found in [44,45] and there references. In our work we have employed the improved Talbot's method [46] for numerical inversion of Laplace transform. The Talbot's contour has parametric representation of the form
s(ξ)=Mτθ(ξ),θ(ξ)=−σ+μξcot(γξ)+νιξ,−π≤ξ≤π,(Γ2) | (2.16) |
where the parameters σ,μ,ν, and γ are to be specified by the user. From (2.16) and (2.10) we have
U(χ,τ)=12πi∫π−πes(ξ)τˆU(χ,s(ξ))ˊs(ξ)dξ. | (2.17) |
We use M-panel mid-point rule with uniform spacing k=2πM, to approximate the integral (2.17) as
Uk(χ,τ)=1MiM∑j=1esjτˆU(χ,sj)ˊsj,forξj=−π+(j−12)k,sj=s(ξj),s′j=s′(ξj). | (2.18) |
To obtain the solution Uk(χ,τ), first we must solve system of 2M+1 equations given in (2.8) and (2.9) for quadrature points sj,|j|≤M. For this purpose the local meshless method is used to discretize operators L,B.
Given a set of points {χi}Ni=1inRd,whered≥1 the approximate function for ˆU(χ) using local meshless method has the form,
ˆU(χi)=∑χj∈Ωiλijϕ(‖χi−χij‖), | (2.19) |
where λi={λij}nj=1 is the expansion coefficients vector, ϕ(r) is a kernel function, r=‖χi−χj‖ is the distance between the centers χi and χj. Ω, and Ωi are global domain and local domains respectively. The sub-domain Ωi contains the center χi, and around it, its n neighboring centers. Thus we obtain n×n linear systems
(ˆU(χi1)ˆU(χi2)...ˆU(χin))=(ϕ(‖χi1−χi1‖)ϕ(‖χi1−χi2‖)...ϕ(‖χi1−χin‖)ϕ(‖χi2−χi1‖)ϕ(‖χi2−χi2‖)...ϕ(‖χi2−χin‖)............ϕ(‖χin−χi1‖)ϕ(‖χin−χi2‖)...ϕ(‖χin−χin‖))(λi1λi2...λin),i=1,2,...,N, | (2.20) |
which can be written as,
ˆUi=Φiλi,1≤i≤N, | (2.21) |
the matrix Φi contains elements in the form bikj=ϕ(‖χik−χij‖),whereχik,χij∈Ωi, the unknowns λi={λij:j=1,...,n} are obtained by solving each of the N systems in (2.21). For the differential operator L we have the form,
LˆU(χi)=∑χj∈ΩiλijLϕ(‖χi−χij‖), | (2.22) |
the above Eq (2.22) can be expressed as a dot product
LˆU(χi)=λi⋅νi, | (2.23) |
where νi is a n-row vector and λi is a n-column vector, entries of the n-column vector νi are given as
νi=Lϕ(‖χi−χij‖),χij∈Ωi, | (2.24) |
eliminating the co efficient λi from (2.21), and (2.23) we have the following expression
\begin{equation} \mathcal{L}\hat{\mathcal{U}}(\mathit{\boldsymbol{\chi}}_i) = {\mathit{\boldsymbol{\nu}}}^i(\Phi^i)^{-1}\mathit{\boldsymbol{\hat{\mathcal{U}}}}^i = {\mathit{ ϖ}}^i\mathit{\boldsymbol{\hat{\mathcal{U}}}}^i \end{equation} | (2.25) |
where,
\begin{equation} {\mathit{ ϖ}}^i = {\mathit{\boldsymbol{\nu}}}^i(\Phi^i)^{-1}, \end{equation} | (2.26) |
thus at each node \mathit{\boldsymbol{\chi}}_i the approximation of the operator \mathcal{L} via local meshless method is given as
\begin{equation} \mathcal{L}\hat{\mathcal{U}}\equiv{\bf{D}}\mathit{\boldsymbol{\hat{\mathcal{U}}}}, \end{equation} | (2.27) |
In (2.27) D is a sparse differentiation matrix obtained via local meshless method as an approximation to \mathcal{L}. The matrix D has order N \times N, it has n non-zero entries, and N-n zero entries, where N is number of centers in global domain, and n is the number of centers in local domain. The boundary operator \mathcal{B} can be discretized in similar way.
In order to solve the multi-term time fractional diffusion wave equation using our proposed method, the local meshless method and Laplace transformation is used. In our numerical scheme first the Laplace transform is applied to time dependent equation which eliminates the time variable, and this process causes no error. Then the local meshless method is utilized for approximating time independent equation. The error estimate for local meshless method is of order O(\eta^{\frac{1}{\epsilon h}}) , 0 < \eta < 1 , \epsilon is the shape parameter and h is the fill distance. In the process of approximating the integrals (2.14) and (2.17) convergence is achieved at different rates depending on the paths \Gamma_1, and \Gamma_2 . In approximating the integrals (2.14) and (2.17) the convergence order rely upon on the step k of the quadrature rule and the time domain [t_{0}, T] for \Gamma_1. The proof for the order of quadrature error for the path \Gamma_1 is given in the next theorem.
Theorem 3.1 ([38], Theorem 2.1). Let \mathcal{U}(\mathit{\boldsymbol{\chi}}, \tau) be the solution of (2.1) with \hat{f}(\mathit{\boldsymbol{\chi}}, \tau) analytic in \Sigma^{\Upsilon}_{\phi}. Let \Gamma\subset \Omega_{r}\subset \Sigma^{\Upsilon}_{\phi}, and define b > 0 by coshb = \frac{1}{\theta \tau_{1} \sin(\eta)} , where \tau_{1} = \frac{t_0}{T} , 0 < \tau_0 < T , 0 < \theta < 1.0 and let \beth = \frac{\theta \overline{r}M}{bT}. Then for Eq (2.15), with k = \frac{b}{M}\leq \frac{\overline{r}}{log 2}, we have |\mathcal{U}(\mathit{\boldsymbol{\chi}}, \tau)-\mathcal{U}_{k}(\mathit{\boldsymbol{\chi}}, \tau)|\leq CQe^{\Upsilon \tau_{1}}l(\rho_{r}M)e^{-\mu M}\left(\|\mathcal{U}_{0}\|+\|\hat{f}(\mathit{\boldsymbol{\chi}}, \tau)\|_{\Sigma^{\Upsilon}_{\phi}}\right), for \mu = \frac{\overline{r}(1-\theta)}{b} , \rho_r = \frac{\theta \overline{r} \tau_{1} \sin(\eta-r_1)}{b} , \overline{r} = 2\pi r_1 , r_1 > 0 , \tau_0\leq \tau \leq T, C = C_{\eta, r_1, \beta} , and l(x) = \mathit{\mbox{max}}(1, log(\frac{1}{x})). Hence the error estimate for the proposed scheme is
error_{est}(\Gamma_1) = |\mathcal{U}(\mathit{\boldsymbol{\chi}},\tau)-\mathcal{U}_{k}(\mathit{\boldsymbol{\chi}},\tau)| = O(l(\rho_{r}M)e^{-\mu M}). |
The authors in [46] derived the optimal values of the parameters for the Talbot's contour (\Gamma_2) defined in (2.16) as given below
\sigma = 0.6122, \; \mu = 0.5017,\; \nu = 0.2645,\; \text{and}\; \gamma = 0.6407, |
with corresponding error estimate as
error_{est}(\Gamma_2) = |\mathcal{U}(\mathit{\boldsymbol{\chi}},\tau)-\mathcal{U}_{k}(\mathit{\boldsymbol{\chi}},\tau)| = O(e^{-1.358 M}). |
To investigate the stability of the systems (2.8) and (2.9), we represent the system in discrete form as
\begin{equation} \mathit{\boldsymbol{\mathcal{M}}}\mathit{\boldsymbol{\hat{\mathcal{U}}}} = \mathfrak{b}, \end{equation} | (4.1) |
the matrix \mathit{\boldsymbol{\mathcal{M}}}_{N \times N} is sparse matrix obtained using local meshless method. For the system (4.1) the constant of stability is defined as
\begin{equation} \mathcal{C} = \sup\limits_{\mathit{\boldsymbol{\hat{\mathcal{U}}}}\neq0} \frac{\|\mathit{\boldsymbol{\hat{\mathcal{U}}}}\|}{\|\mathit{\boldsymbol{\mathcal{M}}}\mathit{\boldsymbol{\hat{\mathcal{U}}}}\|}, \end{equation} | (4.2) |
for any discrete norm \|.\| defined on R^{N} the constant \mathcal{C} is finite. From (4.2) we may write
\begin{equation} \|\mathit{\boldsymbol{\mathcal{M}}}\|^{-1} \leq \frac{\|\mathit{\boldsymbol{\hat{\mathcal{U}}}}\|}{\|\mathit{\boldsymbol{\mathcal{M}}}\mathit{\boldsymbol{\hat{\mathcal{U}}}}\| }\leq \mathcal{C}, \end{equation} | (4.3) |
Similarly for the pseudoinverse \mathit{\boldsymbol{\mathcal{M}}}^{†} of \mathit{\boldsymbol{\mathcal{M}}} , we can write
\begin{equation} \|\mathit{\boldsymbol{\mathcal{M}}}^{†}\| = \sup\limits_{\mathit{\boldsymbol{\mathcal{H}}} \neq 0} \frac{\|\mathit{\boldsymbol{\mathcal{M}}}^{†} \mathit{\boldsymbol{\mathcal{H}}}\|}{\|\mathit{\boldsymbol{\mathcal{H}}}\|}. \end{equation} | (4.4) |
Thus we have
\begin{equation} \|\mathit{\boldsymbol{\mathcal{M}}}^{†}\| \geq \sup\limits_{\mathit{\boldsymbol{\mathcal{H}}} = \mathit{\boldsymbol{\mathcal{M}}}\hat{\mathit{\boldsymbol{\mathcal{H}}}}\neq 0}\frac{\|\mathit{\boldsymbol{\mathcal{M}}}^{†}\mathit{\boldsymbol{\mathcal{M}}}\hat{\mathit{\boldsymbol{\mathcal{U}}}}\| }{\|\mathit{\boldsymbol{\mathcal{M}}}\mathit{\boldsymbol{\hat{\mathcal{U}}}}\| } = \sup\limits_{\mathit{\boldsymbol{\hat{\mathcal{U}}}} \neq 0} \frac{\|\mathit{\boldsymbol{\hat{\mathcal{U}}}}\| }{\|\mathit{\boldsymbol{\mathcal{M}}}\hat{\mathit{\boldsymbol{\mathcal{U}}}}\| } = \mathcal{C}. \end{equation} | (4.5) |
We can see that Eqs (4.3) and (4.5) confirms the bounds for the stability constant \mathcal{C} . Calculating the pseudoinverse for approximating the system (4.1) numerically be quite expansive computationally, but it confirms the stability. The MATLAB's function condest can be used to estimate \|\mathit{\boldsymbol{\mathcal{M}}}^{-1}\|_{\infty} in case of square systems, thus we have
\begin{equation} \mathcal{C} = \frac{condest(\mathit{\boldsymbol{\mathcal{M}}}')}{\|\mathit{\boldsymbol{\mathcal{M}}}\|_{\infty}} \end{equation} | (4.6) |
This work well with less number of computations for our sparse differentiation matrix \mathit{\boldsymbol{\mathcal{M}}} . Figure 1(a) shows the bounds for the constant \mathcal{C} of our system (2.8) and (2.9) for Problem 1 using the Talbot's contour \Gamma_2 . Selecting N = 80 , M = 80 , n = 15 , and \alpha = 1.8, \; \alpha_1 = 1.7, \; \alpha_2 = 1.6, \; c = 0.6 at \tau = 1 , we have 1.00\leq \mathcal{C} \leq 4.5501. It is observed that the upper and lower bounds for the stability constant are very small numbers, which guarantees that the proposed local meshless scheme is stable.
The numerical examples are given to validate our proposed Laplace transform based local meshless scheme. In our computations we have considered different 1-D and 2-D linear multi term wave-diffusion equations. In our numerical examples we have utilized the multi-quadrics(MQ) kernel function \phi (r, \varepsilon) = (1+(\varepsilon r)^2)^{\frac{1}{2}} . We have used the uncertainty principal due to [47] for optimization of the shape parameter. The accuracy of the method is measured using L_{\infty} error defined by
L_{\infty} = \|\mathcal{U}(\mathit{\boldsymbol{\chi}},\tau)-\mathcal{U}_{k}(\mathit{\boldsymbol{\chi}},\tau)\|_{\infty} = \max\limits_{1 \leq j \leq N}(|\mathcal{U}(\mathit{\boldsymbol{\chi}},\tau)-\mathcal{U}_{k}(\mathit{\boldsymbol{\chi}},\tau)|) |
is used. Here \mathcal{U}_{k} and \mathcal{U} are the numerical and exact solutions respectively.
In the first test problem we consider the following linear fractional equation
\begin{align} &D^{\alpha}_{\tau} \mathcal{U}(\chi,\tau)+D^{\alpha_1}_{\tau} \mathcal{U}(\chi,\tau)+D^{\alpha_2}_{\tau} \mathcal{U}(\chi,\tau)-D^{2}_{\chi} \mathcal{U}(\chi,\tau) = f(\chi,\tau), \end{align} | (5.1) |
where
\begin{align*} f(\chi,\tau)& = \left(\frac{6\tau^{3-\alpha}}{\Gamma(4-\alpha)}+\frac{6\tau^{3-\alpha_{1}}}{\Gamma(4-\alpha_{1})}+ \frac{6\tau^{3-\alpha_{2}}}{\Gamma(4-\alpha_{2})}\right)\frac{1}{\cosh(\chi-c)}\\&- \left(\frac{2\tau^{3}}{cosech(c-\chi)^{2}\cosh(c-\chi)^{3}}-\frac{\tau^{3}}{\cosh(c-\chi)}\right). \end{align*} |
The exact solution of the problem is
\begin{equation*} \mathcal{U}(\chi,\tau) = \frac{\tau^{3}}{\cosh(\chi-c)},\; c\in \mathbb{R}. \end{equation*} |
The boundary and initial conditions are
\begin{align} \mathcal{U}(-10,\tau)& = \frac{\tau^{3}}{\cosh(-10-c)}, \; \mathcal{U}(10,\tau) = \frac{\tau^{3}}{\cosh(10-c)}, \end{align} | (5.2) |
and
\begin{equation} \mathcal{U}_{0}(\chi) = \mathcal{U}_{1}(\chi) = 0. \end{equation} | (5.3) |
The points along the hyperbolic contour \Gamma_1 are calculated using the statement \xi = -M:k:M, and along Talbot's contour \Gamma_2 using the relation \xi_j = -\pi+(j-\frac{1}{2})k, \; \text{where}\; j = 1:M, \; \text{and}\; k = \frac{2\pi}{M}. The parameters used in our computations for the contour \Gamma_1 are \theta = 0.10, \; \eta = 0.15410, \; \tau_1 = \frac{t_0}{T}, \; r_1 = 0.13870, \; \bar{r} = 2r_1 \pi, \; \Upsilon = 2.0 The results obtained for the parameters \alpha, \; \alpha_1, \; \alpha_2, and c along the contour \Gamma_1 are displayed in Table 1, and along \Gamma_2 are displayed in Table 2. The exact and numerical spacetime solutions for the given problem is depicted in Figure 2(a) and in Figure 2(b) respectively. The absolute error and error estimate are displayed in Figure 3(a). Figure 3(b) shows error functions for various values of \alpha_j. The results confirms that our numerical scheme is accurate, stable and can solve multi-term time fractional wave-diffusion equations with less computation time.
\alpha=1.5 , | \alpha_{1}=1.4 , | \alpha_{2}=1.3 , | c=0.5 | ||||
N | n | M | L_{\infty} | c | \kappa | error_{est}(\Gamma_1) | C.TIME(sec) |
80 | 20 | 35 | 7.77 \times10^{-5} | 10.0 | 1.13 \times10^{+12} | 3.14 \times10^{-1} | 0.343148 |
55 | 7.61 \times10^{-5} | 10.0 | 1.13 \times10^{+12} | 3.64 \times10^{-2} | 1.222487 | ||
75 | 7.61 \times10^{-5} | 10.0 | 1.13 \times10^{+12} | 4.2 \times10^{-3} | 5.000861 | ||
95 | 7.61 \times10^{-5} | 10.0 | 1.13 \times10^{+12} | 4.75 \times10^{-4} | 12.067666 | ||
110 | 7.61 \times10^{-5} | 10.0 | 1.13 \times10^{+12} | 9.30 \times10^{-5} | 22.244950 | ||
40 | 15 | 80 | 5.76 \times10^{-5} | 4.5 | 1.37 \times10^{+12} | 2.4 \times10^{-3} | 2.034147 |
50 | 4.70 \times10^{-5} | 5.7 | 1.17 \times10^{+12} | 2.4 \times10^{-3} | 3.430185 | ||
60 | 4.32 \times10^{-5} | 6.9 | 1.05 \times10^{+12} | 2.4 \times10^{-3} | 4.307865 | ||
70 | 9.09 \times10^{-5} | 8.0 | 1.24 \times10^{+12} | 2.4 \times10^{-3} | 5.308744 | ||
80 | 4.82 \times10^{-5} | 9.2 | 1.14 \times10^{+12} | 2.4 \times10^{-3} | 6.327198 | ||
70 | 12 | 90 | 2.33 \times10^{-5} | 7.3 | 1.02 \times10^{+12} | 8.18 \times10^{-4} | 8.646889 |
15 | 9.09 \times10^{-5} | 8.0 | 1.24 \times10^{+12} | 8.18 \times10^{-4} | 8.534781 | ||
18 | 8.80 \times10^{-5} | 8.5 | 1.17 \times10^{+12} | 8.18 \times10^{-4} | 8.898832 | ||
21 | 4.10 \times10^{-5} | 8.8 | 1.20 \times10^{+12} | 8.18 \times10^{-4} | 8.901062 | ||
24 | 9.30 \times10^{-5} | 9.0 | 1.26 \times10^{+12} | 8.18 \times10^{-4} | 8.835825 | ||
[14] | 4.79 \times10^{-6} |
\alpha=1.8 , | \alpha_{1}=1.7 , | \alpha_{2}=1.6 , | c=0.5 | ||||
N | n | M | L_{\infty} | c | \kappa | error_{est}(\Gamma_2) | C.TIME(sec) |
70 | 12 | 10 | 3.51 \times10^{-1} | 7.3 | 1.02 \times10^{+12} | 2.02 \times10^{-6} | 0.201190 |
12 | 3.98 \times10^{-2} | 7.3 | 1.02 \times10^{+12} | 1.47 \times10^{-7} | 0.202626 | ||
14 | 4.20 \times10^{-3} | 7.3 | 1.02 \times10^{+12} | 1.06 \times10^{-8} | 0.213661 | ||
16 | 4.16 \times10^{-4} | 7.3 | 1.02 \times10^{+12} | 7.76 \times10^{-10} | 0.203777 | ||
18 | 4.99 \times10^{-5} | 7.3 | 1.02 \times10^{+12} | 5.64 \times10^{-11} | 0.204327 | ||
30 | 15 | 20 | 2.97 \times10^{-5} | 3.4 | 1.00 \times10^{+12} | 4.10 \times10^{-12} | 0.203400 |
40 | 5.99 \times10^{-5} | 4.5 | 1.37 \times10^{+12} | 4.10 \times10^{-12} | 0.212062 | ||
50 | 4.82 \times10^{-5} | 5.7 | 1.17 \times10^{+12} | 4.10 \times10^{-12} | 0.210793 | ||
70 | 9.41 \times10^{-5} | 8.0 | 1.24 \times10^{+12} | 4.10 \times10^{-12} | 0.222508 | ||
90 | 8.14 \times10^{-5} | 10.4 | 1.07 \times10^{+12} | 4.10 \times10^{-12} | 0.217812 | ||
80 | 12 | 20 | 7.56 \times10^{-5} | 8.3 | 1.15 \times10^{+12} | 4.10 \times10^{-12} | 0.211422 |
14 | 7.53 \times10^{-5} | 9.0 | 1.04 \times10^{+12} | 4.10 \times10^{-12} | 0.218499 | ||
16 | 6.68 \times10^{-5} | 9.4 | 1.17 \times10^{+12} | 4.10 \times10^{-12} | 0.224207 | ||
18 | 4.87 \times10^{-5} | 9.8 | 1.02 \times10^{+12} | 4.10 \times10^{-12} | 0.226878 | ||
20 | 4.67 \times10^{-5} | 10.0 | 1.13 \times10^{+12} | 4.10 \times10^{-12} | 0.239581 | ||
[14] | 5.69 \times10^{-5} |
As a second test problem we consider the following linear fractional equation
\begin{eqnarray} D^{\alpha}_{\tau}\mathcal{U}(\chi,\tau)+ D^{\alpha_{1}}_{\tau}\mathcal{U}(\chi,\tau)-D^{2}_{\chi} \mathcal{U}(\chi,\tau) = f(\chi,\tau), \end{eqnarray} | (5.4) |
where
\begin{eqnarray*} f(\chi,\tau) = \left(\frac{6\tau^{3-\alpha}}{\Gamma(4-\alpha)}+\frac{6\tau^{3-\alpha_{1}}}{\Gamma(4-\alpha_{1})}+\pi^{2}\tau^{3}\right)\sin(\pi \chi). \end{eqnarray*} |
The exact solution of the problem is
\begin{equation*} \mathcal{U}(\chi,\tau) = \sin(\pi \chi)\tau^{3}. \end{equation*} |
This equation is considered on [0, 1] with boundary conditions
\begin{equation} \mathcal{U}(0,\tau) = \mathcal{U}(1,\tau) = 0 \end{equation} | (5.5) |
and initial conditions
\begin{equation} \mathcal{U}_{0}(\chi) = \mathcal{U}_{1}(\chi) = 0. \end{equation} | (5.6) |
In this experiment we have utilized both the contours with the same set of optimal parameters. The numerical experiments are performed with different nodes N in the global domain n in the sub-domain. The results obtained for fractional orders \alpha , and \; \alpha_1. are displayed in Table 3 along the path \Gamma_1 , and in Table 4 along the path \Gamma_2 . The approximate and exact spacetime solutions are displayed in Figures 4(a) and Figure 4(b). The plot of absolute error and error estimate is displayed in Figure 5(a). Figure 5(b) shows the plot of error functions for various values of \alpha, and \alpha_1 . The results verifies the accuracy, stability and efficiency of the proposed local meshless scheme for multi-term time fractional wave-diffusion equations.
\alpha=1.9 , | \alpha_{1}=1.3 | ||||||
N | n | M | L_{\infty} | c | \kappa | error_{est}(\Gamma_1) | C.TIME(sec) |
60 | 22 | 40 | 8.44 \times10^{-5} | 7.6 | 1.18 \times10^{+12} | 1.83 \times10^{-1} | 0.667872 |
60 | 6.62 \times10^{-5} | 7.6 | 1.18 \times10^{+12} | 2.12 \times10^{-2} | 1.580686 | ||
80 | 8.62 \times10^{-5} | 7.6 | 1.18 \times10^{+12} | 2.4 \times10^{-3} | 7.362587 | ||
90 | 8.62 \times10^{-5} | 7.6 | 1.18 \times10^{+12} | 8.18 \times10^{-4} | 10.843996 | ||
100 | 8.62 \times10^{-5} | 7.6 | 1.18 \times10^{+12} | 2.76 \times10^{-4} | 15.784191 | ||
70 | 10 | 100 | 5.52 \times10^{-5} | 6.4 | 1.15 \times10^{+12} | 2.76 \times10^{-4} | 17.932164 |
12 | 2.74 \times10^{-5} | 7.3 | 1.02 \times10^{+12} | 2.76 \times10^{-4} | 17.859075 | ||
14 | 3.44 \times10^{-5} | 7.8 | 1.21 \times10^{+12} | 2.76 \times10^{-4} | 18.129870 | ||
18 | 5.21 \times10^{-5} | 8.5 | 1.17 \times10^{+12} | 2.76 \times10^{-4} | 18.278882 | ||
22 | 7.64 \times10^{-5} | 8.9 | 1.15 \times10^{+12} | 2.76 \times10^{-3} | 18.479720 | ||
50 | 25 | 90 | 9.85 \times10^{-5} | 6.5 | 1.01 \times10^{+12} | 8.18 \times10^{-4} | 8.730629 |
60 | 1.03 \times10^{-4} | 7.8 | 1.09 \times10^{+12} | 8.18 \times10^{-4} | 11.049126 | ||
70 | 5.40 \times10^{-5} | 9.1 | 1.14 \times10^{+12} | 8.18 \times10^{-4} | 13.349187 | ||
80 | 6.57 \times10^{-5} | 10.4 | 1.19 \times10^{+12} | 8.18 \times10^{-4} | 15.124607 | ||
90 | 6.17 \times10^{-5} | 11.8 | 1.02 \times10^{+12} | 8.18 \times10^{-4} | 17.134190 | ||
[14] | 7.0080 \times10^{-4} |
\alpha=1.7 , | \alpha_{1}=1.2 | ||||||
N | n | M | L_{\infty} | c | \kappa | error_{est}(\Gamma_2) | C.TIME(sec) |
70 | 12 | 10 | 2.56 \times10^{-1} | 7.3 | 1.02 \times10^{+12} | 2.02 \times10^{-6} | 0.202184 |
12 | 2.91 \times10^{-2} | 7.3 | 1.02 \times10^{+12} | 1.47 \times10^{-7} | 0.197720 | ||
14 | 3.10 \times10^{-3} | 7.3 | 1.02 \times10^{+12} | 1.06 \times10^{-8} | 0.200589 | ||
16 | 3.25 \times10^{-4} | 7.3 | 1.02 \times10^{+12} | 7.76 \times10^{-10} | 0.202710 | ||
18 | 5.65 \times10^{-5} | 7.3 | 1.02 \times10^{+12} | 5.64 \times10^{-11} | 0.194221 | ||
30 | 15 | 20 | 3.74 \times10^{-5} | 3.4 | 1.00 \times10^{+12} | 4.10 \times10^{-12} | 0.202098 |
40 | 6.97 \times10^{-5} | 4.5 | 1.37 \times10^{+12} | 4.10 \times10^{-12} | 0.201783 | ||
50 | 6.68 \times10^{-5} | 5.7 | 1.17 \times10^{+12} | 4.10 \times10^{-12} | 0.204199 | ||
70 | 1.00 \times10^{-4} | 8.0 | 1.24 \times10^{+12} | 4.10 \times10^{-12} | 0.205864 | ||
90 | 1.36 \times10^{-4} | 10.4 | 1.07 \times10^{+12} | 4.10 \times10^{-12} | 0.216570 | ||
80 | 12 | 20 | 9.70 \times10^{-5} | 8.3 | 1.15 \times10^{+12} | 4.10 \times10^{-12} | 0.207446 |
14 | 4.34 \times10^{-5} | 9.0 | 1.04 \times10^{+12} | 4.10 \times10^{-12} | 0.206234 | ||
16 | 8.47 \times10^{-5} | 9.4 | 1.17 \times10^{+12} | 4.10 \times10^{-12} | 0.216176 | ||
18 | 4.76 \times10^{-5} | 9.8 | 1.02 \times10^{+12} | 4.10 \times10^{-12} | 0.215397 | ||
20 | 5.66 \times10^{-5} | 10.0 | 1.13 \times10^{+12} | 4.10 \times10^{-12} | 0.244942 | ||
[14] | 1.39 \times10^{-4} |
We consider the following fractional equation
\begin{eqnarray} D^{\alpha}_{\tau}\mathcal{U}(\chi,\tau)+ D^{\alpha_{1}}_{\tau}\mathcal{U}(\chi,\tau)-D^{2}_{\chi} \mathcal{U}(\chi,\tau) = f(\chi,\tau), \end{eqnarray} | (5.7) |
where
\begin{eqnarray*} f(\chi,\tau)& = &\left(\frac{2 \tau^{2-\alpha}}{\cos(\chi)\Gamma(3-\alpha)}+\frac{2\tau^{2-\alpha_{1}}}{\cos(\chi)\Gamma(3-\alpha_{1})}\right) -\left(\frac{\tau^{2}}{\cos(\chi)}+\frac{2\tau^{2}}{cosec(\chi)^{2}\cos(\chi)^{3}} \right). \end{eqnarray*} |
The exact solution of the problem is
\begin{equation*} \mathcal{U}(\chi,\tau) = \frac{\tau^{2}}{\cos(\chi)}. \end{equation*} |
This equation is considered on [0, 1] with boundary conditions
\begin{equation} \mathcal{U}(0,\tau) = \tau^{2}, \; \mathcal{U}(1,\tau) = \frac{\tau^{2}}{\cos(1)} \end{equation} | (5.8) |
and initial conditions
\begin{equation} \mathcal{U}_{0}(\chi) = 0,\; \mathcal{U}_{1}(\chi) = 0. \end{equation} | (5.9) |
The results obtained for third test problem with fractional orders \alpha, and \alpha_1 along the hyperbolic contour \Gamma_1 are displayed in Tables 5, and along the Talbots contour are displayed in Table 6. From the Tables it can be seen the method has good results in accuracy. Figures 6(a) shows the exact spacetime solution and Figure 6(b) shows the numerical spacetime solution. Figure 7(a), and Figure 7(b) absolute error and error estimate for the contour \Gamma_1 and \Gamma_2 respectively.
\alpha=1.9 , | \alpha_{1}=1.8 , | ||||||
N | n | M | L_{\infty} | c | \kappa | error_{est}(\Gamma_1) | C.TIME(sec) |
80 | 25 | 50 | 4.20 \times10^{-5} | 10.4 | 1.19 \times10^{+12} | 6.25 \times10^{-2} | 2.249386 |
60 | 3.85 \times10^{-5} | 10.4 | 1.19 \times10^{+12} | 2.12 \times10^{-2} | 4.114330 | ||
70 | 3.86 \times10^{-5} | 10.4 | 1.19 \times10^{+12} | 7.2 \times10^{-3} | 7.301508 | ||
90 | 3.86 \times10^{-5} | 10.4 | 1.19 \times10^{+12} | 8.18 \times10^{-4} | 15.044453 | ||
100 | 3.86 \times10^{-5} | 10.4 | 1.19 \times10^{+12} | 2.76 \times10^{-4} | 21.588645 | ||
60 | 27 | 90 | 9.51 \times10^{-5} | 7.9 | 1.06 \times10^{+12} | 8.18 \times10^{-4} | 11.338375 |
70 | 8.41 \times10^{-5} | 9.2 | 1.16 \times10^{+12} | 8.18 \times10^{-4} | 13.565846 | ||
80 | 1.15 \times10^{-4} | 10.6 | 1.01 \times10^{+12} | 8.18 \times10^{-4} | 15.034979 | ||
90 | 9.66 \times10^{-5} | 11.9 | 1.10 \times10^{+12} | 8.18 \times10^{-4} | 17.556509 | ||
100 | 7.93 \times10^{-5} | 13.2 | 1.16 \times10^{+12} | 8.18 \times10^{-4} | 21.605433 | ||
85 | 20 | 95 | 8.45 \times10^{-5} | 10.6 | 1.21 \times10^{+12} | 4.75 \times10^{-4} | 18.828414 |
22 | 5.73 \times10^{-5} | 10.9 | 1.01 \times10^{+12} | 4.75 \times10^{-4} | 19.612251 | ||
24 | 1.68 \times10^{-4} | 11.0 | 1.16 \times10^{+12} | 4.75 \times10^{-4} | 19.670357 | ||
27 | 4.26 \times10^{-5} | 11.2 | 1.16 \times10^{+12} | 4.75 \times10^{-4} | 19.563284 | ||
30 | 5.36 \times10^{-5} | 11.4 | 1.09 \times10^{+12} | 4.75 \times10^{-4} | 20.041057 | ||
[14] | 8.81 \times10^{-5} |
\alpha=1.9 , | \alpha_{1}=1.8 , | ||||||
N | n | M | L_{\infty} | c | \kappa | error_{est}(\Gamma_2) | C.TIME(sec) |
70 | 12 | 10 | 3.54 \times10^{-2} | 7.3 | 1.02 \times10^{+12} | 2.02 \times10^{-6} | 0.142848 |
12 | 3.40 \times10^{-3} | 7.3 | 1.02 \times10^{+12} | 1.47 \times10^{-7} | 0.133224 | ||
14 | 2.83 \times10^{-4} | 7.3 | 1.02 \times10^{+12} | 1.06 \times10^{-8} | 0.134380 | ||
16 | 4.64 \times10^{-5} | 7.3 | 1.02 \times10^{+12} | 7.76 \times10^{-10} | 0.133445 | ||
18 | 5.19 \times10^{-5} | 7.3 | 1.02 \times10^{+12} | 5.64 \times10^{-11} | 0.136420 | ||
30 | 15 | 20 | 1.15 \times10^{-4} | 3.4 | 1.00 \times10^{+12} | 4.10 \times10^{-12} | 0.135380 |
40 | 5.10 \times10^{-5} | 4.5 | 1.37 \times10^{+12} | 4.10 \times10^{-12} | 0.142579 | ||
50 | 1.07 \times10^{-4} | 5.7 | 1.17 \times10^{+12} | 4.10 \times10^{-12} | 0.137483 | ||
70 | 1.44 \times10^{-4} | 8.0 | 1.24 \times10^{+12} | 4.10 \times10^{-12} | 0.145392 | ||
90 | 1.49 \times10^{-4} | 10.4 | 1.07 \times10^{+12} | 4.10 \times10^{-12} | 0.151340 | ||
80 | 12 | 20 | 1.29 \times10^{-4} | 8.3 | 1.15 \times10^{+12} | 4.10 \times10^{-12} | 0.144167 |
14 | 1.22 \times10^{-4} | 9.0 | 1.04 \times10^{+12} | 4.10 \times10^{-12} | 0.139500 | ||
16 | 8.31 \times10^{-5} | 9.4 | 1.17 \times10^{+12} | 4.10 \times10^{-12} | 0.148407 | ||
18 | 4.78 \times10^{-5} | 9.8 | 1.02 \times10^{+12} | 4.10 \times10^{-12} | 0.156982 | ||
20 | 9.51 \times10^{-5} | 10.0 | 1.13 \times10^{+12} | 4.10 \times10^{-12} | 0.166119 | ||
[14] | 8.81 \times10^{-5} |
We consider the two dimensional multi-term time fractional wave-diffusion equation
\begin{eqnarray} D^{\alpha}_{\tau}\mathcal{U}(\chi,\vartheta,\tau)+D^{\alpha_{1}}_{\tau}\mathcal{U}(\chi,\vartheta,\tau)-\Delta \mathcal{U}(\chi,\vartheta,\tau) = f(\chi,\vartheta,\tau), \end{eqnarray} | (5.10) |
subject to zero initial conditions and the boundary conditions are generated from the exact solution
\mathcal{U}(\chi,\vartheta,\tau) = e^{\chi+\vartheta}\tau^{2+\alpha+\alpha_{1}} |
the given 2D test problem is solved with regular nodal points in rectangular, circular and complex domains.
The rectangular domain [0, 1]^2 is descretized with N uniformly distributed points. For this problem also we have used the hyperbolic contour \Gamma_1 and Talbot's contour \Gamma_2 with the same set of optimal parameters used for Problem 1. The uniform nodes distribution with boundary stencil red and interior stencil green are shown in Figure 8. The graphs of exact and approximate solutions for the parameters \alpha = 1.3, \; \alpha_1 = 1.1, at \tau = 1 are shown in the Figure 9(a) and Figure 9(b). The results obtained for various values of N, \; n, and M along the path \Gamma_1 and \Gamma_2 are depicted in Table 7 and Table 8 respectively. From the results one can see that with large number of nodes the proposed method produced accurate results.
\alpha=1.3 , | \alpha_{1}=1.1 | ||||||
N | n | M | L_{\infty} | c | \kappa | error_{est}(\Gamma_1) | C.TIME(sec) |
900 | 14 | 70 | 4.26 \times10^{-2} | 1.1 | 1.22 \times10^{+14} | 7.20 \times10^{-3} | 755.137804 |
16 | 1.12 \times10^{-2} | 1.7 | 3.71 \times10^{+12} | 7.20 \times10^{-3} | 756.918480 | ||
18 | 5.00 \times10^{-3} | 1.9 | 1.26 \times10^{+12} | 7.20 \times10^{-3} | 751.184383 | ||
20 | 9.22 \times10^{-4} | 2.4 | 1.48 \times10^{+12} | 7.20 \times10^{-3} | 748.664343 | ||
576 | 20 | 90 | 5.15 \times10^{-4} | 1.9 | 1.51 \times10^{+12} | 8.18 \times10^{-4} | 286.863124 |
676 | 5.08 \times10^{-4} | 2.0 | 2.19 \times10^{+12} | 8.18 \times10^{-4} | 421.440624 | ||
784 | 8.58 \times10^{-4} | 2.2 | 1.77 \times10^{+12} | 8.18 \times10^{-4} | 608.764593 | ||
900 | 9.22 \times10^{-4} | 2.4 | 1.48 \times10^{+12} | 8.18 \times10^{-4} | 861.916452 | ||
729 | 20 | 20 | 1.10 \times10^{-3} | 2.1 | 1.96 \times10^{+12} | 1.55 \times10^{+0} | 22.119245 |
30 | 7.80 \times10^{-4} | 2.1 | 1.96 \times10^{+12} | 5.73 \times10^{-1} | 48.770754 | ||
50 | 7.57 \times10^{-4} | 2.1 | 1.96 \times10^{+12} | 6.25 \times10^{-2} | 138.310373 | ||
80 | 7.57 \times10^{-4} | 2.1 | 1.96 \times10^{+12} | 2.40 \times10^{-3} | 386.725304 |
\alpha=1.3 , | \alpha_{1}=1.1 | ||||||
N | n | M | L_{\infty} | c | \kappa | error_{est}(\Gamma_2) | C.TIME(sec) |
900 | 20 | 16 | 1.98 \times10^{-1} | 2.4 | 1.48 \times10^{+12} | 7.76 \times10^{-6} | 9.513650 |
18 | 2.15 \times10^{-2} | 2.4 | 1.48 \times10^{+12} | 5.64 \times10^{-11} | 10.640051 | ||
20 | 2.00 \times10^{-3} | 2.4 | 1.48 \times10^{+12} | 4.10 \times10^{-12} | 11.661281 | ||
22 | 8.40 \times10^{-4} | 2.4 | 1.48 \times10^{+12} | 2.97 \times10^{-13} | 12.824995 | ||
24 | 9.12 \times10^{-4} | 2.4 | 1.48 \times10^{+12} | 2.16 \times10^{-14} | 13.560164 | ||
900 | 14 | 24 | 4.28 \times10^{-2} | 1.1 | 1.22 \times10^{+14} | 2.16 \times10^{-14} | 13.026362 |
16 | 1.12 \times10^{-2} | 1.7 | 3.31 \times10^{+12} | 2.16 \times10^{-14} | 13.463156 | ||
18 | 5.00 \times10^{-3} | 1.9 | 1.26 \times10^{+12} | 2.16 \times10^{-14} | 13.591828 | ||
20 | 9.12 \times10^{-4} | 2.4 | 1.48 \times10^{+12} | 2.16 \times10^{-14} | 13.601463 | ||
576 | 20 | 22 | 5.11 \times10^{-4} | 1.9 | 1.51 \times10^{+12} | 2.97 \times10^{-13} | 4.451590 |
676 | 5.17 \times10^{-4} | 2.0 | 2.19 \times10^{+12} | 2.97 \times10^{-13} | 6.401350 | ||
784 | 8.14 \times10^{-4} | 2.2 | 1.77 \times10^{+12} | 2.97 \times10^{-13} | 9.076440 | ||
900 | 8.40 \times10^{-4} | 2.4 | 1.48 \times10^{+12} | 2.97 \times10^{-13} | 12.560123 | ||
961 | 8.06 \times10^{-4} | 2.4 | 2.19 \times10^{+12} | 2.97 \times10^{-13} | 14.919706 |
Here we solve the given problem in unit circle with center at (\chi, \vartheta) = (0.5, 0.5) . The domain is descretized with N uniform nodes. The computational results for different values of N, \; n, and M along \Gamma_1 and \Gamma_2 are depicted in Table 9 and Table 10 respectively. Figure 10(a) shows the uniform nodes in circular domain, whereas Figure 10(b) shows the absolute error computed along the hyperbolic path. The exact and approximate solutions are presented in Figures 11(a) and Figure 11(b). The proposed method produced results with good accuracy in circular domain.
\alpha=1.3 , | \alpha_{1}=1.1 | ||||||
N | n | M | L_{\infty} | c | \kappa | error_{est}(\Gamma_1) | C.TIME(sec) |
950 | 60 | 15 | 8.30 \times10^{-3} | 4.3 | 3.11 \times10^{+12} | 2.63 \times10^{+0} | 38.116660 |
20 | 1.20 \times10^{-3} | 4.3 | 3.11 \times10^{+12} | 1.55 \times10^{+0} | 62.890208 | ||
30 | 9.23 \times10^{-4} | 4.3 | 3.11 \times10^{+12} | 5.37 \times10^{-1} | 133.694751 | ||
40 | 9.17 \times10^{-4} | 4.3 | 3.11 \times10^{+12} | 1.83 \times10^{-2} | 369.149122 | ||
900 | 30 | 50 | 1.30 \times10^{-3} | 3.1 | 2.82 \times10^{+12} | 6.25 \times10^{-2} | 361.783503 |
40 | 2.10 \times10^{-3} | 3.5 | 5.12 \times10^{+12} | 6.25 \times10^{-2} | 366.883195 | ||
50 | 2.20 \times10^{-3} | 4.1 | 2.43 \times10^{+12} | 6.25 \times10^{-2} | 363.312789 | ||
60 | 9.17 \times10^{-4} | 4.3 | 3.11 \times10^{+12} | 6.25 \times10^{-2} | 367.839901 | ||
300 | 59 | 60 | 9.77 \times10^{-4} | 4.3 | 3.06 \times10^{+12} | 2.12 \times10^{-2} | 553.852222 |
550 | 9.77 \times10^{-4} | 4.3 | 3.06 \times10^{+12} | 2.12 \times10^{-2} | 447.562447 | ||
800 | 9.77 \times10^{-4} | 4.3 | 3.06 \times10^{+12} | 2.12 \times10^{-2} | 531.883062 | ||
1100 | 9.77 \times10^{-4} | 4.3 | 3.06 \times10^{+12} | 2.12 \times10^{-2} | 531.921143 |
\alpha=1.5 , | \alpha_{1}=1.3 | ||||||
N | n | M | L_{\infty} | c | \kappa | error_{est}(\Gamma_2) | C.TIME(sec) |
950 | 50 | 18 | 5.87 \times10^{-2} | 4.1 | 2.43 \times10^{+12} | 5.64 \times10^{-11} | 9.258343 |
20 | 7.60 \times10^{-3} | 4.1 | 2.43 \times10^{+12} | 4.10 \times10^{-12} | 9.959348 | ||
22 | 2.40 \times10^{-3} | 4.1 | 2.43 \times10^{+12} | 2.97 \times10^{-13} | 10.597861 | ||
24 | 1.90 \times10^{-3} | 4.1 | 2.43 \times10^{+12} | 2.16 \times10^{-14} | 11.241704 | ||
1050 | 10 | 26 | 9.43 \times10^{-2} | 1.2 | 7.70 \times10^{+13} | 1.57 \times10^{-15} | 9.389988 |
30 | 1.20 \times10^{-3} | 3.1 | 2.82 \times10^{+12} | 1.57 \times10^{-15} | 10.100778 | ||
50 | 1.90 \times10^{-3} | 4.1 | 2.43 \times10^{+12} | 1.57 \times10^{-15} | 11.882140 | ||
60 | 8.84 \times10^{-4} | 4.3 | 3.11 \times10^{+12} | 1.57 \times10^{-15} | 13.157724 | ||
750 | 59 | 28 | 9.36 \times10^{-4} | 4.3 | 3.06 \times10^{+12} | 1.14 \times10^{-16} | 13.662250 |
1150 | 9.36 \times10^{-4} | 4.3 | 3.06 \times10^{+12} | 1.14 \times10^{-16} | 13.759729 | ||
1250 | 9.36 \times10^{-4} | 4.3 | 3.06 \times10^{+12} | 1.14 \times10^{-16} | 13.572409 |
In the last test problem we have considered the complex shape domain. The domain is generated by r_d = \frac{1}{d}[1+2d+d^2-(d+1)\cos(d \theta)], d = 4. In this experiment also we have used the contours \Gamma_1 and \Gamma_2 with the same set of optimal parameters used in Problem 1. The results obtained for fractional orders \alpha = 1.5, \; \alpha_1 = 1.3 , and various nodes N in the global domain and n in the local domain and quadrature points along the contour \Gamma_1 and \Gamma_2 are shown in Table 11 and Table 12 respectively. The regular nodes distribution in the complex domain are shown in Figure 12(a), whereas the approximate and exact solutions are presented in Figures 12(b). Figure 13 shows the absolute error obtained using the Talbots contour. It can be seen that the proposed numerical method produced very accurate and stable results in the complex domain, this confirms the efficiency of the method for such type of equations.
\alpha=1.5 , | \alpha_{1}=1.3 | ||||||
N | n | M | L_{\infty} | c | \kappa | error_{est}(\Gamma_1) | C.TIME(sec) |
851 | 50 | 40 | 4.70 \times10^{-3} | 4.0 | 1.13 \times10^{+12} | 1.83 \times10^{-1} | 50.524737 |
50 | 6.75 \times10^{-4} | 4.0 | 1.13 \times10^{+12} | 6.25 \times10^{-2} | 79.388630 | ||
60 | 6.74 \times10^{-4} | 4.0 | 1.13 \times10^{+12} | 2.12 \times10^{-2} | 116.511151 | ||
80 | 6.74 \times10^{-4} | 4.0 | 1.13 \times10^{+12} | 2.40 \times10^{-3} | 227.719695 | ||
852 | 30 | 70 | 1.30 \times10^{-3} | 2.9 | 6.40 \times10^{+12} | 7.20 \times10^{-3} | 147.655977 |
40 | 1.20 \times10^{-3} | 3.4 | 5.83 \times10^{+12} | 7.20 \times10^{-3} | 158.960295 | ||
50 | 9.34 \times10^{-4} | 3.9 | 1.82 \times10^{+12} | 7.20 \times10^{-3} | 219.043351 | ||
60 | 9.47 \times10^{-4} | 4.2 | 1.92 \times10^{+12} | 7.20 \times10^{-3} | 268.501711 | ||
457 | 60 | 60 | 6.97 \times10^{-4} | 3.0 | 3.07 \times10^{+12} | 2.12 \times10^{-2} | 68.420275 |
542 | 4.39 \times10^{-4} | 3.3 | 1.62 \times10^{+12} | 2.12 \times10^{-2} | 89.095051 | ||
643 | 4.86 \times10^{-4} | 3.6 | 1.79 \times10^{+12} | 2.12 \times10^{-2} | 117.459116 | ||
851 | 7.29 \times10^{-4} | 4.2 | 1.90 \times10^{+12} | 2.12 \times10^{-2} | 184.241136 |
\alpha=1.5 , | \alpha_{1}=1.3 | ||||||
N | n | M | L_{\infty} | c | \kappa | error_{est}(\Gamma_2) | C.TIME(sec) |
752 | 30 | 24 | 1.10 \times10^{-3} | 2.7 | 3.89 \times10^{+12} | 2.16 \times10^{-14} | 3.188624 |
850 | 1.80 \times10^{-3} | 2.9 | 7.98 \times10^{+12} | 2.16 \times10^{-14} | 3.613122 | ||
921 | 1.50 \times10^{-3} | 3.0 | 2.48 \times10^{+13} | 2.16 \times10^{-14} | 4.028301 | ||
974 | 9.61 \times10^{-4} | 3.2 | 5.64 \times10^{+12} | 2.16 \times10^{-14} | 4.297978 | ||
1020 | 28 | 22 | 2.90 \times10^{-3} | 2.9 | 3.79 \times10^{+13} | 2.97 \times10^{-13} | 4.261794 |
40 | 1.90 \times10^{-3} | 3.7 | 2.62 \times10^{+13} | 2.97 \times10^{-13} | 5.485432 | ||
50 | 1.00 \times10^{-3} | 4.2 | 1.13 \times10^{+13} | 2.97 \times10^{-13} | 6.750489 | ||
60 | 9.32 \times10^{-4} | 4.6 | 6.25 \times10^{+12} | 2.97 \times10^{-13} | 8.366275 | ||
1095 | 70 | 18 | 6.47 \times10^{-2} | 5.1 | 2.15 \times10^{+12} | 5.64 \times10^{-11} | 10.452683 |
20 | 6.60 \times10^{-3} | 5.1 | 2.15 \times10^{+12} | 4.10 \times10^{-12} | 10.778798 | ||
22 | 9.01 \times10^{-4} | 5.1 | 2.15 \times10^{+12} | 2.97 \times10^{-13} | 11.238818 | ||
24 | 9.97 \times10^{-4} | 5.1 | 2.15 \times10^{+12} | 2.16 \times10^{-14} | 11.540931 |
In this work, a local meshless method based on Laplace transform has been utilized for the approximation of the numerical solution of 1D and 2D multi-term time fractional wave diffusion equations. We resolved the issue of time-instability which is the common short coming of time-stepping methods using the Laplace transformation, and the issues of ill-conditioning due to dense differentiation matrices and shape parameter sensitivity with localized meshless method. The stability and convergence of the method are discussed. To verify the theoretical results some test problem in 1D and a test problem in 2D are considered. For the two dimensional problem we have considered rectangular, circular, and complex domains. For numerical inversion of Laplace transform we have utilized two types of contours the hyperbolic and the improved Talbot's contour. The results obtained using these two contours were accurate and stable. However, the results show that the Talbot's contour is more efficient computationally. The benefit of this method is that it can approximate such type equations very efficiently and accurately with less computation time, and without any time instability. The obtained results proves the simplicity in implementation, efficiency, accuracy, and stability of the proposed method.
This work was supported by “the Construction team project of the introduction and cultivation of young innovative talents in Colleges and universities of Shandong Province of China, 2019 (Project Name: Big data and business intelligence social service innovation team)” and “the Social Science Planning Project of Qingdao, China,2018 (Grant No. QDSKL1801229)”.
The authors declare that no competing interests exist.
First and second authors revised the paper, solved the examples and used software to compute and sketch the results. Third author did analysis and wrote the paper. Forth proposed the problem and verified the results.
[1] | Goudos SK, Kalialakis C, Mittra R (2016) Evolutionary algorithms applied to antennas and propagation: A review of state of the art. International Journal of Antennas and Propagation. |
[2] |
Hoorfar A (2007) Evolutionary programming in electromagnetic optimization: a review. IEEE T Antenn Propag 55: 523-537. doi: 10.1109/TAP.2007.891306
![]() |
[3] |
Rahmat-Samii Y, Kovitz JM, Rajagopalan H (2012) Nature-inspired optimization techniques in communication antenna designs. Proceedings of the IEEE 100: 2132-2144. doi: 10.1109/JPROC.2012.2188489
![]() |
[4] | Johnson JM, Rahmat-Samii Y (1997) Genetic algorithms in engineering electromagnetic. IEEE Antenn Propag M 39: 7-21. |
[5] |
Weile DS, Michielssen E (1997) Genetic algorithm optimization applied to electromagnetics: A review. IEEE T Antenn Propag 45: 343-353. doi: 10.1109/8.558650
![]() |
[6] | Haupt RL, Werner DH (2007) Genetic algorithms in electromagnetic. John Wiley & Sons. |
[7] |
Robinson J, Rahmat-Samii Y (2004) Particle swarm optimization in electromagnetic. IEEE T Antenn Propag 52: 397-407. doi: 10.1109/TAP.2004.823969
![]() |
[8] |
Karimkashi S, Kishk AA (2010) Invasive weed optimization and its features in electromagnetic. IEEE T Antenn Propag 58: 1269-1278. doi: 10.1109/TAP.2010.2041163
![]() |
[9] |
Rocca P, Oliveri G, Massa A (2011) Differential evolution as applied to electromagnetic. IEEE Antenn Propag M 53: 38-49. doi: 10.1109/MAP.2011.5773566
![]() |
[10] |
Villegas FJ, Cwik T, Rahmat-Samii Y, et al. (2004) A parallel electromagnetic genetic-algorithm optimization (EGO) application for patch antenna design. IEEE T Antenn Propag 52: 2424-2435. doi: 10.1109/TAP.2004.834071
![]() |
[11] | Jayasinghe JM, Uduwawala D (2015) A Novel Multiband Miniature Planar Inverted F Antenna Design for Bluetooth and WLAN Applications. Int J Antenn Propag. |
[12] | Sun SY (2009) Design of broadband microstrip antenna utilizing genetic algorithm. In International Symposium on Electromagnetic Compatibility. |
[13] | John M, Ammann M (2006) Design of a wide-band printed antenna using a genetic algorithm on an array of overlapping sub-patches. IEEE Int Workshop on Antenna Technol.: Small Antennas and Novel Metamaterials (IWAT2006). |
[14] | Mishra RG, Mishra R, Kuchhal P, et al. (2017) Optimization and analysis of high gain wideband microstrip patch antenna using genetic algorithm. International Journal of Engineering & Technology 7: 176-179. |
[15] | Jayasinghe JW (2014) Optimization of the performance of microstrip patch antennas and arrays by using genetic algorithms. Ph.D. Thesis, University of Peradeniya. |
[16] |
Soontornpipit P, Furse CM, Chung YC (2005) Miniaturized biocompatible microstrip antenna using genetic algorithm. IEEE T Antenn Propag 53: 1939-1945. doi: 10.1109/TAP.2005.848461
![]() |
[17] | Heidari AA, Dadgarnia A (2011) Design and optimization of a circularly polarized microstrip antenna for GPS applications using ANFIS and GA. General Assembly and Scientific Symposium, XXXth URSI, 1-4. IEEE. |
[18] | Gupta R, Gurjar S, Kumar A (2013) Using genetic algorithms reduction of rectangular microstrip patches. International Journal of Advanced Research in Computer and Communication Engineering 2: 1643-1646. |
[19] |
Jayasinghe JW, Anguera J, Uduwawala DN (2012) A simple design of multi band microstrip patch antennas robust to fabrication tolerances for GSM, UMTS, LTE, and Bluetooth applications by using genetic algorithm optimization. Progress In Electromagnetics Research M 27: 255-269. doi: 10.2528/PIERM12102705
![]() |
[20] | Jayasinghe JM, Anguera J, Uduwawala DN, et al. (2015) Nonuniform Overlapping Method in Designing Microstrip Patch Antennas Using Genetic Algorithm Optimization. Int J Antenn Propag. |
[21] |
Choo H, Hutani A, Trintinalia LC, et al. (2000) Shape optimisation of broadband microstrip antennas using genetic algorithm. Electron Lett 36: 2057-2058. doi: 10.1049/el:20001452
![]() |
[22] |
Griffiths LA, Furse C, Chung YC (2006) Broadband and multiband antenna design using the genetic algorithm to create amorphous shapes using ellipses. IEEE T Antenn Propag 54: 2776-2782. doi: 10.1109/TAP.2006.882154
![]() |
[23] | Polivka M, Drahovzal M, Rohan J, et al. (2006) Multiband patch antenna with perturbation elements generated by genetic algorithm. In 2006 First European Conference on Antennas and Propagation, 1-4. |
[24] | Xiao S, Wang BZ, Yang XS, et al. (2003) Reconfigurable microstrip antenna design based on genetic algorithm. IEEE Antennas and Propagatopm Society International Symposium 1: 407-410. |
[25] | Wyant A, Venkataraman J (2008) Optimization of Reduced Size Microstrip Patch with slots using a Genetic Algorithm. Dept. of Electrical Engineering, Rochester Institute of Technology. |
[26] |
Alatan L, Aksun MI, Leblebicioglu K, et al. (1999) Use of computationally efficient method of moments in the optimization of printed antennas. IEEE T Antenn Propag 47: 725-732. doi: 10.1109/8.768813
![]() |
[27] |
Kerkhoff AJ, Rogers RL, Ling H (2004) Design and analysis of planar monopole antennas using a genetic algorithm approach. IEEE T Antenn Propag 52: 2709-2718. doi: 10.1109/TAP.2004.834429
![]() |
[28] | Koziel S, Jayasinghe JW, Saraereh O, et al. (2017) Local Optimization of a Sierpinski Carpet Fractal Antenna, IEEE 12th International Conference on Industrial and Information Systems, 1-5. |
[29] | Behera BR (2017) Sierpinski Bow-Tie antenna with genetic algorithm. Engineering Science and Technology 20: 775-782. |
[30] | Adelpour Z, Mohajeri F, Sadeghi M (2010) Dual-frequency microstrip patch antenna with modified Koch fractal geometry based on genetic algorithm. 2010 Loughborough Antennas and Propagation Conference (LAPC), 401-404. |
[31] | Spence TG, Werner DH (2004) Genetically optimized fractile microstrip patch antennas. In IEEE Antennas and Propagation Society International Symposium 4: 4424-4427. |
[32] |
Ozgun O, Mutlu S, Aksun MI, et al. (2003) Design of dual-frequency probe-fed microstrip antennas with genetic optimization algorithm. IEEE T Antenn Propag 51: 1947-1954. doi: 10.1109/TAP.2003.814732
![]() |
[33] |
Namkung J, Hines EL, Green RJ, et al. (2007) Probe‐fed microstrip antenna feed point optimization using a genetic algorithm and the method of moments. Microw Opt Techn Lett 49: 325-329. doi: 10.1002/mop.22120
![]() |
[34] | Lu Q, Edward K, Sean D, et al. (2009) Optimum design of a probe fed dual frequency patch antenna using genetic algorithm. Proceedings of the ARMMS RF and Microwave Society. |
[35] |
Raychowdhury A, Gupta B, Bhattacharjee R (2000) Bandwidth improvement of microstrip antennas through a genetic‐algorithm‐based design of a feed network. Microw Opt Techn Lett 27: 273-275. doi: 10.1002/1098-2760(20001120)27:4<273::AID-MOP17>3.0.CO;2-8
![]() |
[36] | Chattoraj N, Roy GS (2006) Application of Genetic Algorithm to the Optimization of Microstrip Antennas with and without Superstrate. Mikrotalasna revija, 32-35. |
[37] |
Aljibouri B, Lim EG, Evans H, et al. (2000) Multiobjective genetic algorithm approach for a dual-feed circular polarised patch antenna design. Electron Lett 36: 1005-1006. doi: 10.1049/el:20000766
![]() |
[38] |
Raychowdhury A, Gupta B, Bhattacharjee R (2000) Bandwidth improvement of microstrip antennas through a genetic‐algorithm‐based design of a feed network. Microw Opt Techn Lett 27: 273-275. doi: 10.1002/1098-2760(20001120)27:4<273::AID-MOP17>3.0.CO;2-8
![]() |
[39] |
Mukherjee P, Gupta B (2005) Genetic algorithm-based design optimisation of aperture-coupled rectangular microstrip antenna. Defence Sci J 55: 487. doi: 10.14429/dsj.55.2011
![]() |
[40] |
Ding M, Jin R, Geng J (2007) Optimal design of ultra wideband antennas using a mixed model of 2‐D genetic algorithm and finite‐difference time‐domain. Microw Opt Techn Lett 49: 3177-3180. doi: 10.1002/mop.22928
![]() |
[41] |
Silva CRM, Lins HWC, Martins SR, et al. (2012) A multiobjective optimization of a UWB antenna using a self organizing genetic algorithm. Microw opt Tech let 54: 1824-1828. doi: 10.1002/mop.26945
![]() |
[42] |
Kim J, Yoon T, Kim J, et al. (2005) Design of an ultra wide-band printed monopole antenna using FDTD and genetic algorithm. IEEE Microw Wirel Co 15: 395-397. doi: 10.1109/LMWC.2005.850468
![]() |
[43] | Pourahmadazar J, Shirzad H, Ghobadi C, et al. (2010) Using a MAM and genetic algorithm to optimize UWB microstrip monopole antenna with FEM and HFSS. 5th International Symposium on Telecommunications (IST), 115-119. |
[44] |
John M, Ammann MJ (2007) Wideband printed monopole design using a genetic algorithm. IEEE Antenn Wirel Pr 6: 447-449. doi: 10.1109/LAWP.2007.891962
![]() |
[45] | Sun S, Yinghua LV, Zhang J (2010) The application of genetic algorithm optimization in broadband microstrip antenna design. Antennas and Propagation Society International Symposium (APSURSI), 1-4. |
[46] | Michael K, Kucharski AA (2006) Genetic algorithm optimization for broadband patch antenna design. International Conf. on Microwaves, Radar & Wireless Communications, 748-751. |
[47] | Sun S, Yinghua LV, Zhang J (2010) The application of genetic algorithm optimization in broadband microstrip antenna design. Antennas and Propagation Society International Symposium (APSURSI), 1-4. |
[48] |
Deshmukh AA, Kumar G (2005) Compact broadband U‐slot‐loaded rectangular microstrip antennas. Microw Opt Techn Lett 46: 556-559. doi: 10.1002/mop.21049
![]() |
[49] |
Johnson JM, Rahmat-Samii Y (1999) Genetic algorithms and method of moments (GA/MOM) for the design of integrated antennas. IEEE T Antenn Propag 47: 1606-1614. doi: 10.1109/8.805906
![]() |
[50] | Noor AOA (2015) An Automated Optimization Technique of a MSPA for Broadband Communication. Eng Tech J 33. |
[51] | Lech M, Mitchell A, Waterhouse R (2000) Optimization of broadband microstrip patch antennas. Asia-Pacific Microwave Conference, 711-714. |
[52] |
Lotfi AA, Kashani FH (2004) Bandwidth optimization of the E-shaped microstrip antenna using the genetic algorithm based on fuzzy decision making. Antennas and Propagation Society International Symposium 3: 2333-2336. doi: 10.1109/APS.2004.1331839
![]() |
[53] | Bulla G, de Salles AA, Vuong TP (2010) PIFA bandwidth optimization using genetic algorithm and capacitive feeding. IEEE International Conference on Wireless Information Technology and Systems (ICWITS), 1-4. |
[54] |
Nguyen TD, Duroc Y, Vuong TP (2011) Genetic algorithm for optimization of L-shaped PIFA antennas. Int J Microw Wirel T 3: 691-699. doi: 10.1017/S1759078711000985
![]() |
[55] | Chandran PP, Viswasom S (2014) Gain and bandwidth optimization of a novel microstrip patch antenna. Fourth International Conference on Advances in Computing and Communications (ICACC), 315-318. |
[56] |
Tseng LY, Han TY (2010) An evolutionary design method using genetic local search algorithm to obtain broad/dual-band characteristics for circular polarization slot antennas. IEEE T Antenn Propag 58: 1449-1456. doi: 10.1109/TAP.2010.2044312
![]() |
[57] |
Spence TG, Werner DH, Groff RD (2004) Genetic algorithm optimization of some novel broadband and multiband microstrip antennas. Antennas and Propagation Society International Symposium 4: 4408-4411. doi: 10.1109/APS.2004.1330329
![]() |
[58] |
Jayasinghe JW, Anguera J, Uduwawala DN, et al. (2017) A multipurpose genetically engineered microstrip patch antennas: Bandwidth, gain, and polarization. Microw Opt Techn Lett 59: 941-949. doi: 10.1002/mop.30439
![]() |
[59] |
Choo H, Ling H (2003) Design of broadband and dual-band microstrip antennas on a high-dielectric substrate using a genetic algorithm. IEE Proceedings-Microwaves, Antennas and Propagation 150: 137-142. doi: 10.1049/ip-map:20030291
![]() |
[60] | Fertas K, Kimouche H, Challal M, et al. (2015) Design and optimization of a CPW-fed tri-band patch antenna using genetic algorithms. ACES Journal 30: 754-759. |
[61] | Wakrim L, Ibnyaich S, Hassani M (2016) Novel Miniaturized Multiband and Wideband PIFA Antenna for Wireless Applications. 3rd International Conference on Automation, Control, Engineering and Computer Science, 3-6. |
[62] |
Choo H, Ling H ((2002) Design of multiband microstrip antennas using a genetic algorithm. IEEE Microw Wirel Co 12: 345-347. doi: 10.1109/LMWC.2002.803144
![]() |
[63] |
Ohira M, Deguchi H, Tsuji M, et al. (2004) Multiband single-layer frequency selective surface designed by combination of genetic algorithm and geometry-refinement technique. IEEE T Antenn Propag 52: 2925-2931. doi: 10.1109/TAP.2004.835289
![]() |
[64] |
Sathi V, Taherizadeh S, Lotfi A, et al. (2010) Optimisation of multi-frequency microstrip antenna using genetic algorithm coupled with method of moments. IET Microw Antennas P 4: 477-483. doi: 10.1049/iet-map.2009.0020
![]() |
[65] | Michael K, Kucharski AA (2006) Genetic algorithm optimization for Multiband patch antenna design. First European Conference on Antennas and Propagation, 1-4. |
[66] |
Werner DH, Werner PL, Church KH (2001) Genetically engineered multiband fractal antennas. Electron Lett 37: 1150-1151. doi: 10.1049/el:20010802
![]() |
[67] |
Ozgun O, Mutlu S, Aksun ML, et al. (2003) Design of dual-frequency probe-fed microstrip antennas with genetic optimization algorithm. IEEE T Antenn Propag 51: 1947-1954. doi: 10.1109/TAP.2003.814732
![]() |
[68] | Griffiths L, Chung YC, Furse C (2005) Integrated dual band GSM microstrip monopole using GA and FDTD. Antennas and Propagation Society International Symposium 4: 48-51. |
[69] | Lu Q, Korolkiewicz E, Danaher S, et al. (2009) Optimum design of a probe fed dual frequency patch antenna using genetic algorithm. |
[70] |
Villegas FJ, Cwik T, Rahmat-Samii Y, et al. (2002) Parallel genetic-algorithm optimization of a dual-band patch antenna for wireless communications. IEEE Antennas and Propagation Society International Symposium 1: 334-337. doi: 10.1109/APS.2002.1016316
![]() |
[71] | Castellana F, Bilotti F, Vegni L (2001) Automated dual band patch antenna design by a genetic algorithm based numerical code. IEEE Antennas and Propagation Society International Symposium 4: 696-699. |
[72] | Fertas K, Kimouche H, Challal M, et al. (2015) An optimized shaped antenna for multiband applications using Genetic Algorithm. 4th International Conference on Electrical Engineering (ICEE), 1-4. |
[73] |
Saxena NK, Khan MA, Pourush PKS, et al. (2011) GA optimization of cutoff frequency of magnetically biased microstrip circular patch antenna. AEU-Int J Electron C 65: 476-479. doi: 10.1016/j.aeue.2010.04.008
![]() |
[74] |
Herscovici N, Osorio MF, Peixeiro C (2002) Miniaturization of rectangular microstrip patches using genetic algorithms. IEEE Antenn Wirel Pr 1: 94-97. doi: 10.1109/LAWP.2002.805128
![]() |
[75] |
Lamsalli M, El Hamichi A, Boussouis M, et al. (2016) Genetic algorithm optimization for microstrip patch antenna miniaturization. Prog Electrom Res LE 60: 113-120. doi: 10.2528/PIERL16041907
![]() |
[76] |
Gupta VR, Gupta N (2006) Realization of a compact microstrip antenna: An optimization approach. Int J RF and Microw C E 16: 367-373. doi: 10.1002/mmce.20157
![]() |
[77] | Chattoraj N, Roy JS (2007) Application of Genetic Algorithm to the Optimization of Gain of Magnetized Ferrite Microstrip Antenna. Eng let 14. |
[78] |
Kim D, Ju J, Choi J (2012) A mobile communication base station antenna using a genetic algorithm based Fabry-Perot resonance optimization. IEEE T Antenn Propag 60: 1053-1058. doi: 10.1109/TAP.2011.2173108
![]() |
[79] |
Jayasinghe JW, Anguera J, Uduwawala DN (2013) A high-directivity microstrip patch antenna design by using genetic algorithm optimization. Progress In Electromagnetics Research C 37: 131-144. doi: 10.2528/PIERC13010805
![]() |
[80] | Chattoraj N, Roy JS (2006) The optimization of gain of patch antennas using Genetic Algorithm. ACTA TECHNICA CSAV 51: 279. |
[81] |
Zhu X, Shao W, Li JL, et al. (2012) Design and optimization of low RCS patch antennas based on a genetic algorithm. Progress In Electromagnetics Research 122: 327-339. doi: 10.2528/PIER11100703
![]() |
[82] | Mittra R (1997) Genetic algorithm: the last word for solving all of your design problems. Antennas and Propagation Society International Symposium 3: 1672-1675. |
[83] | Lu Q, Edward K, Sean D, et al. (2010) Optimum design of a probe fed dual frequency patch antenna using genetic algorithm. ARMMS RF and Microwave Society, 20-21. |
[84] |
Kim Y, Yun W, Yoon Y (1999) Dual-frequency and dual-polarisation wideband microstrip antenna. Electron Lett 35: 1399-1400. doi: 10.1049/el:19990995
![]() |
[85] |
Jayasinghe J, Anguera J, Uduwawala D (2015) On the behavior of several fitness functions for genetically optimized microstrip antennas. Int J Sci World 3: 53-58. doi: 10.14419/ijsw.v3i1.4132
![]() |
[86] |
Lee D, Lee S (2000) Design of a coaxially fed circularly polarized rectangular microstrip antenna using a genetic algorithm. Microw Opt Techn Lett 26: 288-291. doi: 10.1002/1098-2760(20000905)26:5<288::AID-MOP4>3.0.CO;2-U
![]() |
[87] | See CH, Abd-Alhameed RA, Zhou D, et al. (2006) A new design of circularly-polarised conical-beam microstrip patch antennas using a genetic algorithm. First European Conference on Antennas and Propagation, 1-4. |
[88] |
Zentner R, Sipus Z, Bartolic J (2001) Optimization synthesis of broadband circularly polarized microstrip antennas by hybrid genetic algorithm. Microw Opt Tech Lett 31: 197-201. doi: 10.1002/mop.1397
![]() |
[89] | Grimaccia F, Mussetta M, Pirinoli P, et al. (2006) Genetical swarm optimization (GSO): A class of population-based algorithms for antenna design. ICCE'06 First International Conference on Communications and Electronics, 467-471. |
[90] |
Günel T, Erer I (2002) Application of fuzzy genetic algorithm to the problem of synthesizing circular microstrip antenna elements with thick substrates. AEU-Int J Electron C 56: 215-217. doi: 10.1078/1434-8411-54100099
![]() |
[91] |
Zhang YJ, Gong SX, Wang X, et al. (2010) A hybrid genetic-algorithm space-mapping method for the optimization of broadband aperture-coupled asymmetrical U-shaped slot antennas. J Electromagnet Waves 24: 2139-2153. doi: 10.1163/156939310793699118
![]() |
[92] |
Pissoort D, Rogier H, Olyslager F, et al. (2003) Optimization of a microstrip antenna with a genetic algorithm for use as a ground penetrating radar. J Electromagnet Waves 17: 1197-1216. doi: 10.1163/156939303322519829
![]() |
[93] | Akila M, Anusha P, Sindhu M, et al. (2017) Examination of PSO, GA-PSO and ACO algorithms for the design optimization of printed antennas. IEEE Applied Electromagnetics Conference, 1-2. |
[94] | Mishra S, Chattopadyay S, Gangopadhyaya M (2015) A comparative study of DE, PSO and BFO for optimisation of Rectangular Microstrip Patch Antenna with inset feed parameter. International Conference and Workshop on Computing and Communication (IEMCON), 1-7. |
[95] |
Boada DFM, Sakomura ES, Nascimento DC (2021) Cavity model surrogate-based optimization for electrically thick circularly polarized rectangular microstrip antennas. AEU-Int J Electron C 131: 153597. doi: 10.1016/j.aeue.2020.153597
![]() |
[96] | Koziel S, Bekasiewicz A (2016) Multi-objective design of antennas using surrogate models. World Scientific. |
[97] |
Lee W, Jung M, Kim TH, et al. (2020) Patch antenna design using the GA and the binary method for dual-band applications. J Electromagnet Waves 34: 1691-1704. doi: 10.1080/09205071.2020.1781697
![]() |
[98] |
Awan WA, Zaidi A, Hussain M, et al. (2021) The Design of a Wideband Antenna with Notching Characteristics for Small Devices Using a Genetic Algorithm. Mathematics 9: 2113. doi: 10.3390/math9172113
![]() |
1. | Kamran Kamran, Zahir Shah, Poom Kumam, Nasser Aedh Alreshidi, A Meshless Method Based on the Laplace Transform for the 2D Multi-Term Time Fractional Partial Integro-Differential Equation, 2020, 8, 2227-7390, 1972, 10.3390/math8111972 | |
2. | Abdul Ghafoor, Nazish Khan, Manzoor Hussain, Rahman Ullah, A hybrid collocation method for the computational study of multi-term time fractional partial differential equations, 2022, 128, 08981221, 130, 10.1016/j.camwa.2022.10.005 | |
3. | Siraj Ahmad, Kamal Shah, Thabet Abdeljawad, Bahaaeldin Abdalla, On the Approximation of Fractal-Fractional Differential Equations Using Numerical Inverse Laplace Transform Methods, 2023, 135, 1526-1506, 2743, 10.32604/cmes.2023.023705 | |
4. | A. Ahmadian, M. Salimi, S. Salahshour, Local RBF Method for Transformed Three Dimensional Sub-Diffusion Equations, 2022, 8, 2349-5103, 10.1007/s40819-022-01338-w | |
5. | Monireh Nosrati Sahlan, Hojjat Afshari, Jehad Alzabut, Ghada Alobaidi, Using Fractional Bernoulli Wavelets for Solving Fractional Diffusion Wave Equations with Initial and Boundary Conditions, 2021, 5, 2504-3110, 212, 10.3390/fractalfract5040212 | |
6. | Ujala Gul, Zareen A. Khan, Salma Haque, Nabil Mlaiki, A Local Radial Basis Function Method for Numerical Approximation of Multidimensional Multi-Term Time-Fractional Mixed Wave-Diffusion and Subdiffusion Equation Arising in Fluid Mechanics, 2024, 8, 2504-3110, 639, 10.3390/fractalfract8110639 | |
7. | Aisha Subhan, Kamal Shah, Suhad Subhi Aiadi, Nabil Mlaiki, Fahad M. Alotaibi, Abdellatif Ben Makhlouf, Analysis of Volterra Integrodifferential Equations with the Fractal-Fractional Differential Operator, 2023, 2023, 1099-0526, 1, 10.1155/2023/7210126 | |
8. | Tao Hu, Cheng Huang, Sergiy Reutskiy, Jun Lu, Ji Lin, A Novel Accurate Method for Multi-Term Time-Fractional Nonlinear Diffusion Equations in Arbitrary Domains, 2024, 138, 1526-1506, 1521, 10.32604/cmes.2023.030449 |
\alpha=1.5 , | \alpha_{1}=1.4 , | \alpha_{2}=1.3 , | c=0.5 | ||||
N | n | M | L_{\infty} | c | \kappa | error_{est}(\Gamma_1) | C.TIME(sec) |
80 | 20 | 35 | 7.77 \times10^{-5} | 10.0 | 1.13 \times10^{+12} | 3.14 \times10^{-1} | 0.343148 |
55 | 7.61 \times10^{-5} | 10.0 | 1.13 \times10^{+12} | 3.64 \times10^{-2} | 1.222487 | ||
75 | 7.61 \times10^{-5} | 10.0 | 1.13 \times10^{+12} | 4.2 \times10^{-3} | 5.000861 | ||
95 | 7.61 \times10^{-5} | 10.0 | 1.13 \times10^{+12} | 4.75 \times10^{-4} | 12.067666 | ||
110 | 7.61 \times10^{-5} | 10.0 | 1.13 \times10^{+12} | 9.30 \times10^{-5} | 22.244950 | ||
40 | 15 | 80 | 5.76 \times10^{-5} | 4.5 | 1.37 \times10^{+12} | 2.4 \times10^{-3} | 2.034147 |
50 | 4.70 \times10^{-5} | 5.7 | 1.17 \times10^{+12} | 2.4 \times10^{-3} | 3.430185 | ||
60 | 4.32 \times10^{-5} | 6.9 | 1.05 \times10^{+12} | 2.4 \times10^{-3} | 4.307865 | ||
70 | 9.09 \times10^{-5} | 8.0 | 1.24 \times10^{+12} | 2.4 \times10^{-3} | 5.308744 | ||
80 | 4.82 \times10^{-5} | 9.2 | 1.14 \times10^{+12} | 2.4 \times10^{-3} | 6.327198 | ||
70 | 12 | 90 | 2.33 \times10^{-5} | 7.3 | 1.02 \times10^{+12} | 8.18 \times10^{-4} | 8.646889 |
15 | 9.09 \times10^{-5} | 8.0 | 1.24 \times10^{+12} | 8.18 \times10^{-4} | 8.534781 | ||
18 | 8.80 \times10^{-5} | 8.5 | 1.17 \times10^{+12} | 8.18 \times10^{-4} | 8.898832 | ||
21 | 4.10 \times10^{-5} | 8.8 | 1.20 \times10^{+12} | 8.18 \times10^{-4} | 8.901062 | ||
24 | 9.30 \times10^{-5} | 9.0 | 1.26 \times10^{+12} | 8.18 \times10^{-4} | 8.835825 | ||
[14] | 4.79 \times10^{-6} |
\alpha=1.8 , | \alpha_{1}=1.7 , | \alpha_{2}=1.6 , | c=0.5 | ||||
N | n | M | L_{\infty} | c | \kappa | error_{est}(\Gamma_2) | C.TIME(sec) |
70 | 12 | 10 | 3.51 \times10^{-1} | 7.3 | 1.02 \times10^{+12} | 2.02 \times10^{-6} | 0.201190 |
12 | 3.98 \times10^{-2} | 7.3 | 1.02 \times10^{+12} | 1.47 \times10^{-7} | 0.202626 | ||
14 | 4.20 \times10^{-3} | 7.3 | 1.02 \times10^{+12} | 1.06 \times10^{-8} | 0.213661 | ||
16 | 4.16 \times10^{-4} | 7.3 | 1.02 \times10^{+12} | 7.76 \times10^{-10} | 0.203777 | ||
18 | 4.99 \times10^{-5} | 7.3 | 1.02 \times10^{+12} | 5.64 \times10^{-11} | 0.204327 | ||
30 | 15 | 20 | 2.97 \times10^{-5} | 3.4 | 1.00 \times10^{+12} | 4.10 \times10^{-12} | 0.203400 |
40 | 5.99 \times10^{-5} | 4.5 | 1.37 \times10^{+12} | 4.10 \times10^{-12} | 0.212062 | ||
50 | 4.82 \times10^{-5} | 5.7 | 1.17 \times10^{+12} | 4.10 \times10^{-12} | 0.210793 | ||
70 | 9.41 \times10^{-5} | 8.0 | 1.24 \times10^{+12} | 4.10 \times10^{-12} | 0.222508 | ||
90 | 8.14 \times10^{-5} | 10.4 | 1.07 \times10^{+12} | 4.10 \times10^{-12} | 0.217812 | ||
80 | 12 | 20 | 7.56 \times10^{-5} | 8.3 | 1.15 \times10^{+12} | 4.10 \times10^{-12} | 0.211422 |
14 | 7.53 \times10^{-5} | 9.0 | 1.04 \times10^{+12} | 4.10 \times10^{-12} | 0.218499 | ||
16 | 6.68 \times10^{-5} | 9.4 | 1.17 \times10^{+12} | 4.10 \times10^{-12} | 0.224207 | ||
18 | 4.87 \times10^{-5} | 9.8 | 1.02 \times10^{+12} | 4.10 \times10^{-12} | 0.226878 | ||
20 | 4.67 \times10^{-5} | 10.0 | 1.13 \times10^{+12} | 4.10 \times10^{-12} | 0.239581 | ||
[14] | 5.69 \times10^{-5} |
\alpha=1.9 , | \alpha_{1}=1.3 | ||||||
N | n | M | L_{\infty} | c | \kappa | error_{est}(\Gamma_1) | C.TIME(sec) |
60 | 22 | 40 | 8.44 \times10^{-5} | 7.6 | 1.18 \times10^{+12} | 1.83 \times10^{-1} | 0.667872 |
60 | 6.62 \times10^{-5} | 7.6 | 1.18 \times10^{+12} | 2.12 \times10^{-2} | 1.580686 | ||
80 | 8.62 \times10^{-5} | 7.6 | 1.18 \times10^{+12} | 2.4 \times10^{-3} | 7.362587 | ||
90 | 8.62 \times10^{-5} | 7.6 | 1.18 \times10^{+12} | 8.18 \times10^{-4} | 10.843996 | ||
100 | 8.62 \times10^{-5} | 7.6 | 1.18 \times10^{+12} | 2.76 \times10^{-4} | 15.784191 | ||
70 | 10 | 100 | 5.52 \times10^{-5} | 6.4 | 1.15 \times10^{+12} | 2.76 \times10^{-4} | 17.932164 |
12 | 2.74 \times10^{-5} | 7.3 | 1.02 \times10^{+12} | 2.76 \times10^{-4} | 17.859075 | ||
14 | 3.44 \times10^{-5} | 7.8 | 1.21 \times10^{+12} | 2.76 \times10^{-4} | 18.129870 | ||
18 | 5.21 \times10^{-5} | 8.5 | 1.17 \times10^{+12} | 2.76 \times10^{-4} | 18.278882 | ||
22 | 7.64 \times10^{-5} | 8.9 | 1.15 \times10^{+12} | 2.76 \times10^{-3} | 18.479720 | ||
50 | 25 | 90 | 9.85 \times10^{-5} | 6.5 | 1.01 \times10^{+12} | 8.18 \times10^{-4} | 8.730629 |
60 | 1.03 \times10^{-4} | 7.8 | 1.09 \times10^{+12} | 8.18 \times10^{-4} | 11.049126 | ||
70 | 5.40 \times10^{-5} | 9.1 | 1.14 \times10^{+12} | 8.18 \times10^{-4} | 13.349187 | ||
80 | 6.57 \times10^{-5} | 10.4 | 1.19 \times10^{+12} | 8.18 \times10^{-4} | 15.124607 | ||
90 | 6.17 \times10^{-5} | 11.8 | 1.02 \times10^{+12} | 8.18 \times10^{-4} | 17.134190 | ||
[14] | 7.0080 \times10^{-4} |
\alpha=1.7 , | \alpha_{1}=1.2 | ||||||
N | n | M | L_{\infty} | c | \kappa | error_{est}(\Gamma_2) | C.TIME(sec) |
70 | 12 | 10 | 2.56 \times10^{-1} | 7.3 | 1.02 \times10^{+12} | 2.02 \times10^{-6} | 0.202184 |
12 | 2.91 \times10^{-2} | 7.3 | 1.02 \times10^{+12} | 1.47 \times10^{-7} | 0.197720 | ||
14 | 3.10 \times10^{-3} | 7.3 | 1.02 \times10^{+12} | 1.06 \times10^{-8} | 0.200589 | ||
16 | 3.25 \times10^{-4} | 7.3 | 1.02 \times10^{+12} | 7.76 \times10^{-10} | 0.202710 | ||
18 | 5.65 \times10^{-5} | 7.3 | 1.02 \times10^{+12} | 5.64 \times10^{-11} | 0.194221 | ||
30 | 15 | 20 | 3.74 \times10^{-5} | 3.4 | 1.00 \times10^{+12} | 4.10 \times10^{-12} | 0.202098 |
40 | 6.97 \times10^{-5} | 4.5 | 1.37 \times10^{+12} | 4.10 \times10^{-12} | 0.201783 | ||
50 | 6.68 \times10^{-5} | 5.7 | 1.17 \times10^{+12} | 4.10 \times10^{-12} | 0.204199 | ||
70 | 1.00 \times10^{-4} | 8.0 | 1.24 \times10^{+12} | 4.10 \times10^{-12} | 0.205864 | ||
90 | 1.36 \times10^{-4} | 10.4 | 1.07 \times10^{+12} | 4.10 \times10^{-12} | 0.216570 | ||
80 | 12 | 20 | 9.70 \times10^{-5} | 8.3 | 1.15 \times10^{+12} | 4.10 \times10^{-12} | 0.207446 |
14 | 4.34 \times10^{-5} | 9.0 | 1.04 \times10^{+12} | 4.10 \times10^{-12} | 0.206234 | ||
16 | 8.47 \times10^{-5} | 9.4 | 1.17 \times10^{+12} | 4.10 \times10^{-12} | 0.216176 | ||
18 | 4.76 \times10^{-5} | 9.8 | 1.02 \times10^{+12} | 4.10 \times10^{-12} | 0.215397 | ||
20 | 5.66 \times10^{-5} | 10.0 | 1.13 \times10^{+12} | 4.10 \times10^{-12} | 0.244942 | ||
[14] | 1.39 \times10^{-4} |
\alpha=1.9 , | \alpha_{1}=1.8 , | ||||||
N | n | M | L_{\infty} | c | \kappa | error_{est}(\Gamma_1) | C.TIME(sec) |
80 | 25 | 50 | 4.20 \times10^{-5} | 10.4 | 1.19 \times10^{+12} | 6.25 \times10^{-2} | 2.249386 |
60 | 3.85 \times10^{-5} | 10.4 | 1.19 \times10^{+12} | 2.12 \times10^{-2} | 4.114330 | ||
70 | 3.86 \times10^{-5} | 10.4 | 1.19 \times10^{+12} | 7.2 \times10^{-3} | 7.301508 | ||
90 | 3.86 \times10^{-5} | 10.4 | 1.19 \times10^{+12} | 8.18 \times10^{-4} | 15.044453 | ||
100 | 3.86 \times10^{-5} | 10.4 | 1.19 \times10^{+12} | 2.76 \times10^{-4} | 21.588645 | ||
60 | 27 | 90 | 9.51 \times10^{-5} | 7.9 | 1.06 \times10^{+12} | 8.18 \times10^{-4} | 11.338375 |
70 | 8.41 \times10^{-5} | 9.2 | 1.16 \times10^{+12} | 8.18 \times10^{-4} | 13.565846 | ||
80 | 1.15 \times10^{-4} | 10.6 | 1.01 \times10^{+12} | 8.18 \times10^{-4} | 15.034979 | ||
90 | 9.66 \times10^{-5} | 11.9 | 1.10 \times10^{+12} | 8.18 \times10^{-4} | 17.556509 | ||
100 | 7.93 \times10^{-5} | 13.2 | 1.16 \times10^{+12} | 8.18 \times10^{-4} | 21.605433 | ||
85 | 20 | 95 | 8.45 \times10^{-5} | 10.6 | 1.21 \times10^{+12} | 4.75 \times10^{-4} | 18.828414 |
22 | 5.73 \times10^{-5} | 10.9 | 1.01 \times10^{+12} | 4.75 \times10^{-4} | 19.612251 | ||
24 | 1.68 \times10^{-4} | 11.0 | 1.16 \times10^{+12} | 4.75 \times10^{-4} | 19.670357 | ||
27 | 4.26 \times10^{-5} | 11.2 | 1.16 \times10^{+12} | 4.75 \times10^{-4} | 19.563284 | ||
30 | 5.36 \times10^{-5} | 11.4 | 1.09 \times10^{+12} | 4.75 \times10^{-4} | 20.041057 | ||
[14] | 8.81 \times10^{-5} |
\alpha=1.9 , | \alpha_{1}=1.8 , | ||||||
N | n | M | L_{\infty} | c | \kappa | error_{est}(\Gamma_2) | C.TIME(sec) |
70 | 12 | 10 | 3.54 \times10^{-2} | 7.3 | 1.02 \times10^{+12} | 2.02 \times10^{-6} | 0.142848 |
12 | 3.40 \times10^{-3} | 7.3 | 1.02 \times10^{+12} | 1.47 \times10^{-7} | 0.133224 | ||
14 | 2.83 \times10^{-4} | 7.3 | 1.02 \times10^{+12} | 1.06 \times10^{-8} | 0.134380 | ||
16 | 4.64 \times10^{-5} | 7.3 | 1.02 \times10^{+12} | 7.76 \times10^{-10} | 0.133445 | ||
18 | 5.19 \times10^{-5} | 7.3 | 1.02 \times10^{+12} | 5.64 \times10^{-11} | 0.136420 | ||
30 | 15 | 20 | 1.15 \times10^{-4} | 3.4 | 1.00 \times10^{+12} | 4.10 \times10^{-12} | 0.135380 |
40 | 5.10 \times10^{-5} | 4.5 | 1.37 \times10^{+12} | 4.10 \times10^{-12} | 0.142579 | ||
50 | 1.07 \times10^{-4} | 5.7 | 1.17 \times10^{+12} | 4.10 \times10^{-12} | 0.137483 | ||
70 | 1.44 \times10^{-4} | 8.0 | 1.24 \times10^{+12} | 4.10 \times10^{-12} | 0.145392 | ||
90 | 1.49 \times10^{-4} | 10.4 | 1.07 \times10^{+12} | 4.10 \times10^{-12} | 0.151340 | ||
80 | 12 | 20 | 1.29 \times10^{-4} | 8.3 | 1.15 \times10^{+12} | 4.10 \times10^{-12} | 0.144167 |
14 | 1.22 \times10^{-4} | 9.0 | 1.04 \times10^{+12} | 4.10 \times10^{-12} | 0.139500 | ||
16 | 8.31 \times10^{-5} | 9.4 | 1.17 \times10^{+12} | 4.10 \times10^{-12} | 0.148407 | ||
18 | 4.78 \times10^{-5} | 9.8 | 1.02 \times10^{+12} | 4.10 \times10^{-12} | 0.156982 | ||
20 | 9.51 \times10^{-5} | 10.0 | 1.13 \times10^{+12} | 4.10 \times10^{-12} | 0.166119 | ||
[14] | 8.81 \times10^{-5} |
\alpha=1.3 , | \alpha_{1}=1.1 | ||||||
N | n | M | L_{\infty} | c | \kappa | error_{est}(\Gamma_1) | C.TIME(sec) |
900 | 14 | 70 | 4.26 \times10^{-2} | 1.1 | 1.22 \times10^{+14} | 7.20 \times10^{-3} | 755.137804 |
16 | 1.12 \times10^{-2} | 1.7 | 3.71 \times10^{+12} | 7.20 \times10^{-3} | 756.918480 | ||
18 | 5.00 \times10^{-3} | 1.9 | 1.26 \times10^{+12} | 7.20 \times10^{-3} | 751.184383 | ||
20 | 9.22 \times10^{-4} | 2.4 | 1.48 \times10^{+12} | 7.20 \times10^{-3} | 748.664343 | ||
576 | 20 | 90 | 5.15 \times10^{-4} | 1.9 | 1.51 \times10^{+12} | 8.18 \times10^{-4} | 286.863124 |
676 | 5.08 \times10^{-4} | 2.0 | 2.19 \times10^{+12} | 8.18 \times10^{-4} | 421.440624 | ||
784 | 8.58 \times10^{-4} | 2.2 | 1.77 \times10^{+12} | 8.18 \times10^{-4} | 608.764593 | ||
900 | 9.22 \times10^{-4} | 2.4 | 1.48 \times10^{+12} | 8.18 \times10^{-4} | 861.916452 | ||
729 | 20 | 20 | 1.10 \times10^{-3} | 2.1 | 1.96 \times10^{+12} | 1.55 \times10^{+0} | 22.119245 |
30 | 7.80 \times10^{-4} | 2.1 | 1.96 \times10^{+12} | 5.73 \times10^{-1} | 48.770754 | ||
50 | 7.57 \times10^{-4} | 2.1 | 1.96 \times10^{+12} | 6.25 \times10^{-2} | 138.310373 | ||
80 | 7.57 \times10^{-4} | 2.1 | 1.96 \times10^{+12} | 2.40 \times10^{-3} | 386.725304 |
\alpha=1.3 , | \alpha_{1}=1.1 | ||||||
N | n | M | L_{\infty} | c | \kappa | error_{est}(\Gamma_2) | C.TIME(sec) |
900 | 20 | 16 | 1.98 \times10^{-1} | 2.4 | 1.48 \times10^{+12} | 7.76 \times10^{-6} | 9.513650 |
18 | 2.15 \times10^{-2} | 2.4 | 1.48 \times10^{+12} | 5.64 \times10^{-11} | 10.640051 | ||
20 | 2.00 \times10^{-3} | 2.4 | 1.48 \times10^{+12} | 4.10 \times10^{-12} | 11.661281 | ||
22 | 8.40 \times10^{-4} | 2.4 | 1.48 \times10^{+12} | 2.97 \times10^{-13} | 12.824995 | ||
24 | 9.12 \times10^{-4} | 2.4 | 1.48 \times10^{+12} | 2.16 \times10^{-14} | 13.560164 | ||
900 | 14 | 24 | 4.28 \times10^{-2} | 1.1 | 1.22 \times10^{+14} | 2.16 \times10^{-14} | 13.026362 |
16 | 1.12 \times10^{-2} | 1.7 | 3.31 \times10^{+12} | 2.16 \times10^{-14} | 13.463156 | ||
18 | 5.00 \times10^{-3} | 1.9 | 1.26 \times10^{+12} | 2.16 \times10^{-14} | 13.591828 | ||
20 | 9.12 \times10^{-4} | 2.4 | 1.48 \times10^{+12} | 2.16 \times10^{-14} | 13.601463 | ||
576 | 20 | 22 | 5.11 \times10^{-4} | 1.9 | 1.51 \times10^{+12} | 2.97 \times10^{-13} | 4.451590 |
676 | 5.17 \times10^{-4} | 2.0 | 2.19 \times10^{+12} | 2.97 \times10^{-13} | 6.401350 | ||
784 | 8.14 \times10^{-4} | 2.2 | 1.77 \times10^{+12} | 2.97 \times10^{-13} | 9.076440 | ||
900 | 8.40 \times10^{-4} | 2.4 | 1.48 \times10^{+12} | 2.97 \times10^{-13} | 12.560123 | ||
961 | 8.06 \times10^{-4} | 2.4 | 2.19 \times10^{+12} | 2.97 \times10^{-13} | 14.919706 |
\alpha=1.3 , | \alpha_{1}=1.1 | ||||||
N | n | M | L_{\infty} | c | \kappa | error_{est}(\Gamma_1) | C.TIME(sec) |
950 | 60 | 15 | 8.30 \times10^{-3} | 4.3 | 3.11 \times10^{+12} | 2.63 \times10^{+0} | 38.116660 |
20 | 1.20 \times10^{-3} | 4.3 | 3.11 \times10^{+12} | 1.55 \times10^{+0} | 62.890208 | ||
30 | 9.23 \times10^{-4} | 4.3 | 3.11 \times10^{+12} | 5.37 \times10^{-1} | 133.694751 | ||
40 | 9.17 \times10^{-4} | 4.3 | 3.11 \times10^{+12} | 1.83 \times10^{-2} | 369.149122 | ||
900 | 30 | 50 | 1.30 \times10^{-3} | 3.1 | 2.82 \times10^{+12} | 6.25 \times10^{-2} | 361.783503 |
40 | 2.10 \times10^{-3} | 3.5 | 5.12 \times10^{+12} | 6.25 \times10^{-2} | 366.883195 | ||
50 | 2.20 \times10^{-3} | 4.1 | 2.43 \times10^{+12} | 6.25 \times10^{-2} | 363.312789 | ||
60 | 9.17 \times10^{-4} | 4.3 | 3.11 \times10^{+12} | 6.25 \times10^{-2} | 367.839901 | ||
300 | 59 | 60 | 9.77 \times10^{-4} | 4.3 | 3.06 \times10^{+12} | 2.12 \times10^{-2} | 553.852222 |
550 | 9.77 \times10^{-4} | 4.3 | 3.06 \times10^{+12} | 2.12 \times10^{-2} | 447.562447 | ||
800 | 9.77 \times10^{-4} | 4.3 | 3.06 \times10^{+12} | 2.12 \times10^{-2} | 531.883062 | ||
1100 | 9.77 \times10^{-4} | 4.3 | 3.06 \times10^{+12} | 2.12 \times10^{-2} | 531.921143 |
\alpha=1.5 , | \alpha_{1}=1.3 | ||||||
N | n | M | L_{\infty} | c | \kappa | error_{est}(\Gamma_2) | C.TIME(sec) |
950 | 50 | 18 | 5.87 \times10^{-2} | 4.1 | 2.43 \times10^{+12} | 5.64 \times10^{-11} | 9.258343 |
20 | 7.60 \times10^{-3} | 4.1 | 2.43 \times10^{+12} | 4.10 \times10^{-12} | 9.959348 | ||
22 | 2.40 \times10^{-3} | 4.1 | 2.43 \times10^{+12} | 2.97 \times10^{-13} | 10.597861 | ||
24 | 1.90 \times10^{-3} | 4.1 | 2.43 \times10^{+12} | 2.16 \times10^{-14} | 11.241704 | ||
1050 | 10 | 26 | 9.43 \times10^{-2} | 1.2 | 7.70 \times10^{+13} | 1.57 \times10^{-15} | 9.389988 |
30 | 1.20 \times10^{-3} | 3.1 | 2.82 \times10^{+12} | 1.57 \times10^{-15} | 10.100778 | ||
50 | 1.90 \times10^{-3} | 4.1 | 2.43 \times10^{+12} | 1.57 \times10^{-15} | 11.882140 | ||
60 | 8.84 \times10^{-4} | 4.3 | 3.11 \times10^{+12} | 1.57 \times10^{-15} | 13.157724 | ||
750 | 59 | 28 | 9.36 \times10^{-4} | 4.3 | 3.06 \times10^{+12} | 1.14 \times10^{-16} | 13.662250 |
1150 | 9.36 \times10^{-4} | 4.3 | 3.06 \times10^{+12} | 1.14 \times10^{-16} | 13.759729 | ||
1250 | 9.36 \times10^{-4} | 4.3 | 3.06 \times10^{+12} | 1.14 \times10^{-16} | 13.572409 |
\alpha=1.5 , | \alpha_{1}=1.3 | ||||||
N | n | M | L_{\infty} | c | \kappa | error_{est}(\Gamma_1) | C.TIME(sec) |
851 | 50 | 40 | 4.70 \times10^{-3} | 4.0 | 1.13 \times10^{+12} | 1.83 \times10^{-1} | 50.524737 |
50 | 6.75 \times10^{-4} | 4.0 | 1.13 \times10^{+12} | 6.25 \times10^{-2} | 79.388630 | ||
60 | 6.74 \times10^{-4} | 4.0 | 1.13 \times10^{+12} | 2.12 \times10^{-2} | 116.511151 | ||
80 | 6.74 \times10^{-4} | 4.0 | 1.13 \times10^{+12} | 2.40 \times10^{-3} | 227.719695 | ||
852 | 30 | 70 | 1.30 \times10^{-3} | 2.9 | 6.40 \times10^{+12} | 7.20 \times10^{-3} | 147.655977 |
40 | 1.20 \times10^{-3} | 3.4 | 5.83 \times10^{+12} | 7.20 \times10^{-3} | 158.960295 | ||
50 | 9.34 \times10^{-4} | 3.9 | 1.82 \times10^{+12} | 7.20 \times10^{-3} | 219.043351 | ||
60 | 9.47 \times10^{-4} | 4.2 | 1.92 \times10^{+12} | 7.20 \times10^{-3} | 268.501711 | ||
457 | 60 | 60 | 6.97 \times10^{-4} | 3.0 | 3.07 \times10^{+12} | 2.12 \times10^{-2} | 68.420275 |
542 | 4.39 \times10^{-4} | 3.3 | 1.62 \times10^{+12} | 2.12 \times10^{-2} | 89.095051 | ||
643 | 4.86 \times10^{-4} | 3.6 | 1.79 \times10^{+12} | 2.12 \times10^{-2} | 117.459116 | ||
851 | 7.29 \times10^{-4} | 4.2 | 1.90 \times10^{+12} | 2.12 \times10^{-2} | 184.241136 |
\alpha=1.5 , | \alpha_{1}=1.3 | ||||||
N | n | M | L_{\infty} | c | \kappa | error_{est}(\Gamma_2) | C.TIME(sec) |
752 | 30 | 24 | 1.10 \times10^{-3} | 2.7 | 3.89 \times10^{+12} | 2.16 \times10^{-14} | 3.188624 |
850 | 1.80 \times10^{-3} | 2.9 | 7.98 \times10^{+12} | 2.16 \times10^{-14} | 3.613122 | ||
921 | 1.50 \times10^{-3} | 3.0 | 2.48 \times10^{+13} | 2.16 \times10^{-14} | 4.028301 | ||
974 | 9.61 \times10^{-4} | 3.2 | 5.64 \times10^{+12} | 2.16 \times10^{-14} | 4.297978 | ||
1020 | 28 | 22 | 2.90 \times10^{-3} | 2.9 | 3.79 \times10^{+13} | 2.97 \times10^{-13} | 4.261794 |
40 | 1.90 \times10^{-3} | 3.7 | 2.62 \times10^{+13} | 2.97 \times10^{-13} | 5.485432 | ||
50 | 1.00 \times10^{-3} | 4.2 | 1.13 \times10^{+13} | 2.97 \times10^{-13} | 6.750489 | ||
60 | 9.32 \times10^{-4} | 4.6 | 6.25 \times10^{+12} | 2.97 \times10^{-13} | 8.366275 | ||
1095 | 70 | 18 | 6.47 \times10^{-2} | 5.1 | 2.15 \times10^{+12} | 5.64 \times10^{-11} | 10.452683 |
20 | 6.60 \times10^{-3} | 5.1 | 2.15 \times10^{+12} | 4.10 \times10^{-12} | 10.778798 | ||
22 | 9.01 \times10^{-4} | 5.1 | 2.15 \times10^{+12} | 2.97 \times10^{-13} | 11.238818 | ||
24 | 9.97 \times10^{-4} | 5.1 | 2.15 \times10^{+12} | 2.16 \times10^{-14} | 11.540931 |
\alpha=1.5 , | \alpha_{1}=1.4 , | \alpha_{2}=1.3 , | c=0.5 | ||||
N | n | M | L_{\infty} | c | \kappa | error_{est}(\Gamma_1) | C.TIME(sec) |
80 | 20 | 35 | 7.77 \times10^{-5} | 10.0 | 1.13 \times10^{+12} | 3.14 \times10^{-1} | 0.343148 |
55 | 7.61 \times10^{-5} | 10.0 | 1.13 \times10^{+12} | 3.64 \times10^{-2} | 1.222487 | ||
75 | 7.61 \times10^{-5} | 10.0 | 1.13 \times10^{+12} | 4.2 \times10^{-3} | 5.000861 | ||
95 | 7.61 \times10^{-5} | 10.0 | 1.13 \times10^{+12} | 4.75 \times10^{-4} | 12.067666 | ||
110 | 7.61 \times10^{-5} | 10.0 | 1.13 \times10^{+12} | 9.30 \times10^{-5} | 22.244950 | ||
40 | 15 | 80 | 5.76 \times10^{-5} | 4.5 | 1.37 \times10^{+12} | 2.4 \times10^{-3} | 2.034147 |
50 | 4.70 \times10^{-5} | 5.7 | 1.17 \times10^{+12} | 2.4 \times10^{-3} | 3.430185 | ||
60 | 4.32 \times10^{-5} | 6.9 | 1.05 \times10^{+12} | 2.4 \times10^{-3} | 4.307865 | ||
70 | 9.09 \times10^{-5} | 8.0 | 1.24 \times10^{+12} | 2.4 \times10^{-3} | 5.308744 | ||
80 | 4.82 \times10^{-5} | 9.2 | 1.14 \times10^{+12} | 2.4 \times10^{-3} | 6.327198 | ||
70 | 12 | 90 | 2.33 \times10^{-5} | 7.3 | 1.02 \times10^{+12} | 8.18 \times10^{-4} | 8.646889 |
15 | 9.09 \times10^{-5} | 8.0 | 1.24 \times10^{+12} | 8.18 \times10^{-4} | 8.534781 | ||
18 | 8.80 \times10^{-5} | 8.5 | 1.17 \times10^{+12} | 8.18 \times10^{-4} | 8.898832 | ||
21 | 4.10 \times10^{-5} | 8.8 | 1.20 \times10^{+12} | 8.18 \times10^{-4} | 8.901062 | ||
24 | 9.30 \times10^{-5} | 9.0 | 1.26 \times10^{+12} | 8.18 \times10^{-4} | 8.835825 | ||
[14] | 4.79 \times10^{-6} |
\alpha=1.8 , | \alpha_{1}=1.7 , | \alpha_{2}=1.6 , | c=0.5 | ||||
N | n | M | L_{\infty} | c | \kappa | error_{est}(\Gamma_2) | C.TIME(sec) |
70 | 12 | 10 | 3.51 \times10^{-1} | 7.3 | 1.02 \times10^{+12} | 2.02 \times10^{-6} | 0.201190 |
12 | 3.98 \times10^{-2} | 7.3 | 1.02 \times10^{+12} | 1.47 \times10^{-7} | 0.202626 | ||
14 | 4.20 \times10^{-3} | 7.3 | 1.02 \times10^{+12} | 1.06 \times10^{-8} | 0.213661 | ||
16 | 4.16 \times10^{-4} | 7.3 | 1.02 \times10^{+12} | 7.76 \times10^{-10} | 0.203777 | ||
18 | 4.99 \times10^{-5} | 7.3 | 1.02 \times10^{+12} | 5.64 \times10^{-11} | 0.204327 | ||
30 | 15 | 20 | 2.97 \times10^{-5} | 3.4 | 1.00 \times10^{+12} | 4.10 \times10^{-12} | 0.203400 |
40 | 5.99 \times10^{-5} | 4.5 | 1.37 \times10^{+12} | 4.10 \times10^{-12} | 0.212062 | ||
50 | 4.82 \times10^{-5} | 5.7 | 1.17 \times10^{+12} | 4.10 \times10^{-12} | 0.210793 | ||
70 | 9.41 \times10^{-5} | 8.0 | 1.24 \times10^{+12} | 4.10 \times10^{-12} | 0.222508 | ||
90 | 8.14 \times10^{-5} | 10.4 | 1.07 \times10^{+12} | 4.10 \times10^{-12} | 0.217812 | ||
80 | 12 | 20 | 7.56 \times10^{-5} | 8.3 | 1.15 \times10^{+12} | 4.10 \times10^{-12} | 0.211422 |
14 | 7.53 \times10^{-5} | 9.0 | 1.04 \times10^{+12} | 4.10 \times10^{-12} | 0.218499 | ||
16 | 6.68 \times10^{-5} | 9.4 | 1.17 \times10^{+12} | 4.10 \times10^{-12} | 0.224207 | ||
18 | 4.87 \times10^{-5} | 9.8 | 1.02 \times10^{+12} | 4.10 \times10^{-12} | 0.226878 | ||
20 | 4.67 \times10^{-5} | 10.0 | 1.13 \times10^{+12} | 4.10 \times10^{-12} | 0.239581 | ||
[14] | 5.69 \times10^{-5} |
\alpha=1.9 , | \alpha_{1}=1.3 | ||||||
N | n | M | L_{\infty} | c | \kappa | error_{est}(\Gamma_1) | C.TIME(sec) |
60 | 22 | 40 | 8.44 \times10^{-5} | 7.6 | 1.18 \times10^{+12} | 1.83 \times10^{-1} | 0.667872 |
60 | 6.62 \times10^{-5} | 7.6 | 1.18 \times10^{+12} | 2.12 \times10^{-2} | 1.580686 | ||
80 | 8.62 \times10^{-5} | 7.6 | 1.18 \times10^{+12} | 2.4 \times10^{-3} | 7.362587 | ||
90 | 8.62 \times10^{-5} | 7.6 | 1.18 \times10^{+12} | 8.18 \times10^{-4} | 10.843996 | ||
100 | 8.62 \times10^{-5} | 7.6 | 1.18 \times10^{+12} | 2.76 \times10^{-4} | 15.784191 | ||
70 | 10 | 100 | 5.52 \times10^{-5} | 6.4 | 1.15 \times10^{+12} | 2.76 \times10^{-4} | 17.932164 |
12 | 2.74 \times10^{-5} | 7.3 | 1.02 \times10^{+12} | 2.76 \times10^{-4} | 17.859075 | ||
14 | 3.44 \times10^{-5} | 7.8 | 1.21 \times10^{+12} | 2.76 \times10^{-4} | 18.129870 | ||
18 | 5.21 \times10^{-5} | 8.5 | 1.17 \times10^{+12} | 2.76 \times10^{-4} | 18.278882 | ||
22 | 7.64 \times10^{-5} | 8.9 | 1.15 \times10^{+12} | 2.76 \times10^{-3} | 18.479720 | ||
50 | 25 | 90 | 9.85 \times10^{-5} | 6.5 | 1.01 \times10^{+12} | 8.18 \times10^{-4} | 8.730629 |
60 | 1.03 \times10^{-4} | 7.8 | 1.09 \times10^{+12} | 8.18 \times10^{-4} | 11.049126 | ||
70 | 5.40 \times10^{-5} | 9.1 | 1.14 \times10^{+12} | 8.18 \times10^{-4} | 13.349187 | ||
80 | 6.57 \times10^{-5} | 10.4 | 1.19 \times10^{+12} | 8.18 \times10^{-4} | 15.124607 | ||
90 | 6.17 \times10^{-5} | 11.8 | 1.02 \times10^{+12} | 8.18 \times10^{-4} | 17.134190 | ||
[14] | 7.0080 \times10^{-4} |
\alpha=1.7 , | \alpha_{1}=1.2 | ||||||
N | n | M | L_{\infty} | c | \kappa | error_{est}(\Gamma_2) | C.TIME(sec) |
70 | 12 | 10 | 2.56 \times10^{-1} | 7.3 | 1.02 \times10^{+12} | 2.02 \times10^{-6} | 0.202184 |
12 | 2.91 \times10^{-2} | 7.3 | 1.02 \times10^{+12} | 1.47 \times10^{-7} | 0.197720 | ||
14 | 3.10 \times10^{-3} | 7.3 | 1.02 \times10^{+12} | 1.06 \times10^{-8} | 0.200589 | ||
16 | 3.25 \times10^{-4} | 7.3 | 1.02 \times10^{+12} | 7.76 \times10^{-10} | 0.202710 | ||
18 | 5.65 \times10^{-5} | 7.3 | 1.02 \times10^{+12} | 5.64 \times10^{-11} | 0.194221 | ||
30 | 15 | 20 | 3.74 \times10^{-5} | 3.4 | 1.00 \times10^{+12} | 4.10 \times10^{-12} | 0.202098 |
40 | 6.97 \times10^{-5} | 4.5 | 1.37 \times10^{+12} | 4.10 \times10^{-12} | 0.201783 | ||
50 | 6.68 \times10^{-5} | 5.7 | 1.17 \times10^{+12} | 4.10 \times10^{-12} | 0.204199 | ||
70 | 1.00 \times10^{-4} | 8.0 | 1.24 \times10^{+12} | 4.10 \times10^{-12} | 0.205864 | ||
90 | 1.36 \times10^{-4} | 10.4 | 1.07 \times10^{+12} | 4.10 \times10^{-12} | 0.216570 | ||
80 | 12 | 20 | 9.70 \times10^{-5} | 8.3 | 1.15 \times10^{+12} | 4.10 \times10^{-12} | 0.207446 |
14 | 4.34 \times10^{-5} | 9.0 | 1.04 \times10^{+12} | 4.10 \times10^{-12} | 0.206234 | ||
16 | 8.47 \times10^{-5} | 9.4 | 1.17 \times10^{+12} | 4.10 \times10^{-12} | 0.216176 | ||
18 | 4.76 \times10^{-5} | 9.8 | 1.02 \times10^{+12} | 4.10 \times10^{-12} | 0.215397 | ||
20 | 5.66 \times10^{-5} | 10.0 | 1.13 \times10^{+12} | 4.10 \times10^{-12} | 0.244942 | ||
[14] | 1.39 \times10^{-4} |
\alpha=1.9 , | \alpha_{1}=1.8 , | ||||||
N | n | M | L_{\infty} | c | \kappa | error_{est}(\Gamma_1) | C.TIME(sec) |
80 | 25 | 50 | 4.20 \times10^{-5} | 10.4 | 1.19 \times10^{+12} | 6.25 \times10^{-2} | 2.249386 |
60 | 3.85 \times10^{-5} | 10.4 | 1.19 \times10^{+12} | 2.12 \times10^{-2} | 4.114330 | ||
70 | 3.86 \times10^{-5} | 10.4 | 1.19 \times10^{+12} | 7.2 \times10^{-3} | 7.301508 | ||
90 | 3.86 \times10^{-5} | 10.4 | 1.19 \times10^{+12} | 8.18 \times10^{-4} | 15.044453 | ||
100 | 3.86 \times10^{-5} | 10.4 | 1.19 \times10^{+12} | 2.76 \times10^{-4} | 21.588645 | ||
60 | 27 | 90 | 9.51 \times10^{-5} | 7.9 | 1.06 \times10^{+12} | 8.18 \times10^{-4} | 11.338375 |
70 | 8.41 \times10^{-5} | 9.2 | 1.16 \times10^{+12} | 8.18 \times10^{-4} | 13.565846 | ||
80 | 1.15 \times10^{-4} | 10.6 | 1.01 \times10^{+12} | 8.18 \times10^{-4} | 15.034979 | ||
90 | 9.66 \times10^{-5} | 11.9 | 1.10 \times10^{+12} | 8.18 \times10^{-4} | 17.556509 | ||
100 | 7.93 \times10^{-5} | 13.2 | 1.16 \times10^{+12} | 8.18 \times10^{-4} | 21.605433 | ||
85 | 20 | 95 | 8.45 \times10^{-5} | 10.6 | 1.21 \times10^{+12} | 4.75 \times10^{-4} | 18.828414 |
22 | 5.73 \times10^{-5} | 10.9 | 1.01 \times10^{+12} | 4.75 \times10^{-4} | 19.612251 | ||
24 | 1.68 \times10^{-4} | 11.0 | 1.16 \times10^{+12} | 4.75 \times10^{-4} | 19.670357 | ||
27 | 4.26 \times10^{-5} | 11.2 | 1.16 \times10^{+12} | 4.75 \times10^{-4} | 19.563284 | ||
30 | 5.36 \times10^{-5} | 11.4 | 1.09 \times10^{+12} | 4.75 \times10^{-4} | 20.041057 | ||
[14] | 8.81 \times10^{-5} |
\alpha=1.9 , | \alpha_{1}=1.8 , | ||||||
N | n | M | L_{\infty} | c | \kappa | error_{est}(\Gamma_2) | C.TIME(sec) |
70 | 12 | 10 | 3.54 \times10^{-2} | 7.3 | 1.02 \times10^{+12} | 2.02 \times10^{-6} | 0.142848 |
12 | 3.40 \times10^{-3} | 7.3 | 1.02 \times10^{+12} | 1.47 \times10^{-7} | 0.133224 | ||
14 | 2.83 \times10^{-4} | 7.3 | 1.02 \times10^{+12} | 1.06 \times10^{-8} | 0.134380 | ||
16 | 4.64 \times10^{-5} | 7.3 | 1.02 \times10^{+12} | 7.76 \times10^{-10} | 0.133445 | ||
18 | 5.19 \times10^{-5} | 7.3 | 1.02 \times10^{+12} | 5.64 \times10^{-11} | 0.136420 | ||
30 | 15 | 20 | 1.15 \times10^{-4} | 3.4 | 1.00 \times10^{+12} | 4.10 \times10^{-12} | 0.135380 |
40 | 5.10 \times10^{-5} | 4.5 | 1.37 \times10^{+12} | 4.10 \times10^{-12} | 0.142579 | ||
50 | 1.07 \times10^{-4} | 5.7 | 1.17 \times10^{+12} | 4.10 \times10^{-12} | 0.137483 | ||
70 | 1.44 \times10^{-4} | 8.0 | 1.24 \times10^{+12} | 4.10 \times10^{-12} | 0.145392 | ||
90 | 1.49 \times10^{-4} | 10.4 | 1.07 \times10^{+12} | 4.10 \times10^{-12} | 0.151340 | ||
80 | 12 | 20 | 1.29 \times10^{-4} | 8.3 | 1.15 \times10^{+12} | 4.10 \times10^{-12} | 0.144167 |
14 | 1.22 \times10^{-4} | 9.0 | 1.04 \times10^{+12} | 4.10 \times10^{-12} | 0.139500 | ||
16 | 8.31 \times10^{-5} | 9.4 | 1.17 \times10^{+12} | 4.10 \times10^{-12} | 0.148407 | ||
18 | 4.78 \times10^{-5} | 9.8 | 1.02 \times10^{+12} | 4.10 \times10^{-12} | 0.156982 | ||
20 | 9.51 \times10^{-5} | 10.0 | 1.13 \times10^{+12} | 4.10 \times10^{-12} | 0.166119 | ||
[14] | 8.81 \times10^{-5} |
\alpha=1.3 , | \alpha_{1}=1.1 | ||||||
N | n | M | L_{\infty} | c | \kappa | error_{est}(\Gamma_1) | C.TIME(sec) |
900 | 14 | 70 | 4.26 \times10^{-2} | 1.1 | 1.22 \times10^{+14} | 7.20 \times10^{-3} | 755.137804 |
16 | 1.12 \times10^{-2} | 1.7 | 3.71 \times10^{+12} | 7.20 \times10^{-3} | 756.918480 | ||
18 | 5.00 \times10^{-3} | 1.9 | 1.26 \times10^{+12} | 7.20 \times10^{-3} | 751.184383 | ||
20 | 9.22 \times10^{-4} | 2.4 | 1.48 \times10^{+12} | 7.20 \times10^{-3} | 748.664343 | ||
576 | 20 | 90 | 5.15 \times10^{-4} | 1.9 | 1.51 \times10^{+12} | 8.18 \times10^{-4} | 286.863124 |
676 | 5.08 \times10^{-4} | 2.0 | 2.19 \times10^{+12} | 8.18 \times10^{-4} | 421.440624 | ||
784 | 8.58 \times10^{-4} | 2.2 | 1.77 \times10^{+12} | 8.18 \times10^{-4} | 608.764593 | ||
900 | 9.22 \times10^{-4} | 2.4 | 1.48 \times10^{+12} | 8.18 \times10^{-4} | 861.916452 | ||
729 | 20 | 20 | 1.10 \times10^{-3} | 2.1 | 1.96 \times10^{+12} | 1.55 \times10^{+0} | 22.119245 |
30 | 7.80 \times10^{-4} | 2.1 | 1.96 \times10^{+12} | 5.73 \times10^{-1} | 48.770754 | ||
50 | 7.57 \times10^{-4} | 2.1 | 1.96 \times10^{+12} | 6.25 \times10^{-2} | 138.310373 | ||
80 | 7.57 \times10^{-4} | 2.1 | 1.96 \times10^{+12} | 2.40 \times10^{-3} | 386.725304 |
\alpha=1.3 , | \alpha_{1}=1.1 | ||||||
N | n | M | L_{\infty} | c | \kappa | error_{est}(\Gamma_2) | C.TIME(sec) |
900 | 20 | 16 | 1.98 \times10^{-1} | 2.4 | 1.48 \times10^{+12} | 7.76 \times10^{-6} | 9.513650 |
18 | 2.15 \times10^{-2} | 2.4 | 1.48 \times10^{+12} | 5.64 \times10^{-11} | 10.640051 | ||
20 | 2.00 \times10^{-3} | 2.4 | 1.48 \times10^{+12} | 4.10 \times10^{-12} | 11.661281 | ||
22 | 8.40 \times10^{-4} | 2.4 | 1.48 \times10^{+12} | 2.97 \times10^{-13} | 12.824995 | ||
24 | 9.12 \times10^{-4} | 2.4 | 1.48 \times10^{+12} | 2.16 \times10^{-14} | 13.560164 | ||
900 | 14 | 24 | 4.28 \times10^{-2} | 1.1 | 1.22 \times10^{+14} | 2.16 \times10^{-14} | 13.026362 |
16 | 1.12 \times10^{-2} | 1.7 | 3.31 \times10^{+12} | 2.16 \times10^{-14} | 13.463156 | ||
18 | 5.00 \times10^{-3} | 1.9 | 1.26 \times10^{+12} | 2.16 \times10^{-14} | 13.591828 | ||
20 | 9.12 \times10^{-4} | 2.4 | 1.48 \times10^{+12} | 2.16 \times10^{-14} | 13.601463 | ||
576 | 20 | 22 | 5.11 \times10^{-4} | 1.9 | 1.51 \times10^{+12} | 2.97 \times10^{-13} | 4.451590 |
676 | 5.17 \times10^{-4} | 2.0 | 2.19 \times10^{+12} | 2.97 \times10^{-13} | 6.401350 | ||
784 | 8.14 \times10^{-4} | 2.2 | 1.77 \times10^{+12} | 2.97 \times10^{-13} | 9.076440 | ||
900 | 8.40 \times10^{-4} | 2.4 | 1.48 \times10^{+12} | 2.97 \times10^{-13} | 12.560123 | ||
961 | 8.06 \times10^{-4} | 2.4 | 2.19 \times10^{+12} | 2.97 \times10^{-13} | 14.919706 |
\alpha=1.3 , | \alpha_{1}=1.1 | ||||||
N | n | M | L_{\infty} | c | \kappa | error_{est}(\Gamma_1) | C.TIME(sec) |
950 | 60 | 15 | 8.30 \times10^{-3} | 4.3 | 3.11 \times10^{+12} | 2.63 \times10^{+0} | 38.116660 |
20 | 1.20 \times10^{-3} | 4.3 | 3.11 \times10^{+12} | 1.55 \times10^{+0} | 62.890208 | ||
30 | 9.23 \times10^{-4} | 4.3 | 3.11 \times10^{+12} | 5.37 \times10^{-1} | 133.694751 | ||
40 | 9.17 \times10^{-4} | 4.3 | 3.11 \times10^{+12} | 1.83 \times10^{-2} | 369.149122 | ||
900 | 30 | 50 | 1.30 \times10^{-3} | 3.1 | 2.82 \times10^{+12} | 6.25 \times10^{-2} | 361.783503 |
40 | 2.10 \times10^{-3} | 3.5 | 5.12 \times10^{+12} | 6.25 \times10^{-2} | 366.883195 | ||
50 | 2.20 \times10^{-3} | 4.1 | 2.43 \times10^{+12} | 6.25 \times10^{-2} | 363.312789 | ||
60 | 9.17 \times10^{-4} | 4.3 | 3.11 \times10^{+12} | 6.25 \times10^{-2} | 367.839901 | ||
300 | 59 | 60 | 9.77 \times10^{-4} | 4.3 | 3.06 \times10^{+12} | 2.12 \times10^{-2} | 553.852222 |
550 | 9.77 \times10^{-4} | 4.3 | 3.06 \times10^{+12} | 2.12 \times10^{-2} | 447.562447 | ||
800 | 9.77 \times10^{-4} | 4.3 | 3.06 \times10^{+12} | 2.12 \times10^{-2} | 531.883062 | ||
1100 | 9.77 \times10^{-4} | 4.3 | 3.06 \times10^{+12} | 2.12 \times10^{-2} | 531.921143 |
\alpha=1.5 , | \alpha_{1}=1.3 | ||||||
N | n | M | L_{\infty} | c | \kappa | error_{est}(\Gamma_2) | C.TIME(sec) |
950 | 50 | 18 | 5.87 \times10^{-2} | 4.1 | 2.43 \times10^{+12} | 5.64 \times10^{-11} | 9.258343 |
20 | 7.60 \times10^{-3} | 4.1 | 2.43 \times10^{+12} | 4.10 \times10^{-12} | 9.959348 | ||
22 | 2.40 \times10^{-3} | 4.1 | 2.43 \times10^{+12} | 2.97 \times10^{-13} | 10.597861 | ||
24 | 1.90 \times10^{-3} | 4.1 | 2.43 \times10^{+12} | 2.16 \times10^{-14} | 11.241704 | ||
1050 | 10 | 26 | 9.43 \times10^{-2} | 1.2 | 7.70 \times10^{+13} | 1.57 \times10^{-15} | 9.389988 |
30 | 1.20 \times10^{-3} | 3.1 | 2.82 \times10^{+12} | 1.57 \times10^{-15} | 10.100778 | ||
50 | 1.90 \times10^{-3} | 4.1 | 2.43 \times10^{+12} | 1.57 \times10^{-15} | 11.882140 | ||
60 | 8.84 \times10^{-4} | 4.3 | 3.11 \times10^{+12} | 1.57 \times10^{-15} | 13.157724 | ||
750 | 59 | 28 | 9.36 \times10^{-4} | 4.3 | 3.06 \times10^{+12} | 1.14 \times10^{-16} | 13.662250 |
1150 | 9.36 \times10^{-4} | 4.3 | 3.06 \times10^{+12} | 1.14 \times10^{-16} | 13.759729 | ||
1250 | 9.36 \times10^{-4} | 4.3 | 3.06 \times10^{+12} | 1.14 \times10^{-16} | 13.572409 |
\alpha=1.5 , | \alpha_{1}=1.3 | ||||||
N | n | M | L_{\infty} | c | \kappa | error_{est}(\Gamma_1) | C.TIME(sec) |
851 | 50 | 40 | 4.70 \times10^{-3} | 4.0 | 1.13 \times10^{+12} | 1.83 \times10^{-1} | 50.524737 |
50 | 6.75 \times10^{-4} | 4.0 | 1.13 \times10^{+12} | 6.25 \times10^{-2} | 79.388630 | ||
60 | 6.74 \times10^{-4} | 4.0 | 1.13 \times10^{+12} | 2.12 \times10^{-2} | 116.511151 | ||
80 | 6.74 \times10^{-4} | 4.0 | 1.13 \times10^{+12} | 2.40 \times10^{-3} | 227.719695 | ||
852 | 30 | 70 | 1.30 \times10^{-3} | 2.9 | 6.40 \times10^{+12} | 7.20 \times10^{-3} | 147.655977 |
40 | 1.20 \times10^{-3} | 3.4 | 5.83 \times10^{+12} | 7.20 \times10^{-3} | 158.960295 | ||
50 | 9.34 \times10^{-4} | 3.9 | 1.82 \times10^{+12} | 7.20 \times10^{-3} | 219.043351 | ||
60 | 9.47 \times10^{-4} | 4.2 | 1.92 \times10^{+12} | 7.20 \times10^{-3} | 268.501711 | ||
457 | 60 | 60 | 6.97 \times10^{-4} | 3.0 | 3.07 \times10^{+12} | 2.12 \times10^{-2} | 68.420275 |
542 | 4.39 \times10^{-4} | 3.3 | 1.62 \times10^{+12} | 2.12 \times10^{-2} | 89.095051 | ||
643 | 4.86 \times10^{-4} | 3.6 | 1.79 \times10^{+12} | 2.12 \times10^{-2} | 117.459116 | ||
851 | 7.29 \times10^{-4} | 4.2 | 1.90 \times10^{+12} | 2.12 \times10^{-2} | 184.241136 |
\alpha=1.5 , | \alpha_{1}=1.3 | ||||||
N | n | M | L_{\infty} | c | \kappa | error_{est}(\Gamma_2) | C.TIME(sec) |
752 | 30 | 24 | 1.10 \times10^{-3} | 2.7 | 3.89 \times10^{+12} | 2.16 \times10^{-14} | 3.188624 |
850 | 1.80 \times10^{-3} | 2.9 | 7.98 \times10^{+12} | 2.16 \times10^{-14} | 3.613122 | ||
921 | 1.50 \times10^{-3} | 3.0 | 2.48 \times10^{+13} | 2.16 \times10^{-14} | 4.028301 | ||
974 | 9.61 \times10^{-4} | 3.2 | 5.64 \times10^{+12} | 2.16 \times10^{-14} | 4.297978 | ||
1020 | 28 | 22 | 2.90 \times10^{-3} | 2.9 | 3.79 \times10^{+13} | 2.97 \times10^{-13} | 4.261794 |
40 | 1.90 \times10^{-3} | 3.7 | 2.62 \times10^{+13} | 2.97 \times10^{-13} | 5.485432 | ||
50 | 1.00 \times10^{-3} | 4.2 | 1.13 \times10^{+13} | 2.97 \times10^{-13} | 6.750489 | ||
60 | 9.32 \times10^{-4} | 4.6 | 6.25 \times10^{+12} | 2.97 \times10^{-13} | 8.366275 | ||
1095 | 70 | 18 | 6.47 \times10^{-2} | 5.1 | 2.15 \times10^{+12} | 5.64 \times10^{-11} | 10.452683 |
20 | 6.60 \times10^{-3} | 5.1 | 2.15 \times10^{+12} | 4.10 \times10^{-12} | 10.778798 | ||
22 | 9.01 \times10^{-4} | 5.1 | 2.15 \times10^{+12} | 2.97 \times10^{-13} | 11.238818 | ||
24 | 9.97 \times10^{-4} | 5.1 | 2.15 \times10^{+12} | 2.16 \times10^{-14} | 11.540931 |