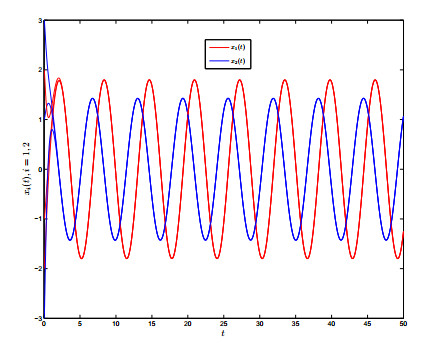
We provide a detailed analysis of the shock formation process for the non-isentropic 2d Euler equations in azimuthal symmetry. We prove that from an open set of smooth and generic initial data, solutions of the Euler equations form a first singularity or gradient blow-up. This first singularity is termed a Hölder C13 pre-shock, and our analysis provides the first detailed description of this cusp solution. The novelty of this work relative to [
Citation: Isaac Neal, Steve Shkoller, Vlad Vicol. A characteristics approach to shock formation in 2D Euler with azimuthal symmetry and entropy[J]. Communications in Analysis and Mechanics, 2025, 17(1): 188-236. doi: 10.3934/cam.2025009
[1] | Jin Gao, Lihua Dai . Anti-periodic synchronization of quaternion-valued high-order Hopfield neural networks with delays. AIMS Mathematics, 2022, 7(8): 14051-14075. doi: 10.3934/math.2022775 |
[2] | Yuehong Zhang, Zhiying Li, Wangdong Jiang, Wei Liu . The stability of anti-periodic solutions for fractional-order inertial BAM neural networks with time-delays. AIMS Mathematics, 2023, 8(3): 6176-6190. doi: 10.3934/math.2023312 |
[3] | Xiaofang Meng, Yongkun Li . Pseudo almost periodic solutions for quaternion-valued high-order Hopfield neural networks with time-varying delays and leakage delays on time scales. AIMS Mathematics, 2021, 6(9): 10070-10091. doi: 10.3934/math.2021585 |
[4] | Nina Huo, Bing Li, Yongkun Li . Global exponential stability and existence of almost periodic solutions in distribution for Clifford-valued stochastic high-order Hopfield neural networks with time-varying delays. AIMS Mathematics, 2022, 7(3): 3653-3679. doi: 10.3934/math.2022202 |
[5] | Huizhen Qu, Jianwen Zhou . $ S $-asymptotically $ \omega $-periodic dynamics in a fractional-order dual inertial neural networks with time-varying lags. AIMS Mathematics, 2022, 7(2): 2782-2809. doi: 10.3934/math.2022154 |
[6] | Dan-Ning Xu, Zhi-Ying Li . Mittag-Leffler stabilization of anti-periodic solutions for fractional-order neural networks with time-varying delays. AIMS Mathematics, 2023, 8(1): 1610-1619. doi: 10.3934/math.2023081 |
[7] | Ailing Li, Xinlu Ye . Finite-time anti-synchronization for delayed inertial neural networks via the fractional and polynomial controllers of time variable. AIMS Mathematics, 2021, 6(8): 8173-8190. doi: 10.3934/math.2021473 |
[8] | Huahai Qiu, Li Wan, Zhigang Zhou, Qunjiao Zhang, Qinghua Zhou . Global exponential periodicity of nonlinear neural networks with multiple time-varying delays. AIMS Mathematics, 2023, 8(5): 12472-12485. doi: 10.3934/math.2023626 |
[9] | Yanshou Dong, Junfang Zhao, Xu Miao, Ming Kang . Piecewise pseudo almost periodic solutions of interval general BAM neural networks with mixed time-varying delays and impulsive perturbations. AIMS Mathematics, 2023, 8(9): 21828-21855. doi: 10.3934/math.20231113 |
[10] | Xiaojin Guo, Chuangxia Huang, Jinde Cao . Nonnegative periodicity on high-order proportional delayed cellular neural networks involving $ D $ operator. AIMS Mathematics, 2021, 6(3): 2228-2243. doi: 10.3934/math.2021135 |
We provide a detailed analysis of the shock formation process for the non-isentropic 2d Euler equations in azimuthal symmetry. We prove that from an open set of smooth and generic initial data, solutions of the Euler equations form a first singularity or gradient blow-up. This first singularity is termed a Hölder C13 pre-shock, and our analysis provides the first detailed description of this cusp solution. The novelty of this work relative to [
Due to the engineering backgrounds and strong biological significance, Babcock and Westervelt [1,2] introduced an inertial term into the traditional multidirectional associative memory neural networks, and established a class of second order delay differential equations, which was called as the famous delayed inertial neural networks model. Arising from problems in different applied sciences such as mathematical physics, control theory, biology in different situations, nonlinear vibration, mechanics, electromagnetic theory and other related fields, the periodic oscillation is an important qualitative property of nonlinear differential equations [3,4,5,6,7,8,9]. Consequently, assuming that the activation functions are bounded and employing reduced-order variable substitution which convert the inertial systems into the first order differential equations, the authors in [10,11] and [12] have respectively gained the existence and stability of anti-periodic solution and periodic solution for addressed inertial neural networks models. Manifestly, the above transformation will raise the dimension in the inertial neural networks system, then some new parameters need to be introduced. This will increase huge amount of computation and be attained hard in practice [13,14]. For the above reasons, most recently, avoiding the reduced order method, the authors in [15] and [16] respectively developed some non-reduced order methods to establish the existence and stability of periodic solutions for inertial neural networks with time-varying delays.
It has been recognized that, in neural networks dynamics touching the communication, economics, biology or ecology areas, the relevant state variables are often considered as proteins and molecules, light intensity levels or electric charge, and they are naturally anti-periodic [17,18,19]. Such neural networks systems are often regarded as anti-periodic systems. Therefore, the convergence analysis and stability on the anti-periodic solutions in various neural networks systems with delays have attracted the interest of many researchers and some excellent results are reported in [20,21,22,23,24,25,26,27]. In particular, the anti-periodicity on inertial quaternion-valued high-order Hopfield neural networks with state-dependent delays has been established in [28] by employing reduced-order variable substitution. However, few researchers have utilized the non-reduced order methods to explore such topics on the following high-order inertial Hopfield neural networks involving time-varying delays:
x″i(t)=−ˉai(t)x′i(t)−ˉbi(t)xi(t)+n∑j=1ˉcij(t)Aj(xj(t))+n∑j=1ˉdij(t)Bj(xj(t−qij(t)))+n∑j=1n∑l=1θijl(t)Qj(xj(t−ηijl(t)))Ql(xl(t−ξijl(t)))+Ji(t), t≥0, | (1.1) |
associating with initial value conditions:
xi(s)=φi(s), x′i(s)=ψi(s), −τi≤s≤0, φi, ψi∈C([−τi,0],R), | (1.2) |
where n∑j=1ˉcij(t)Aj(xj(t)), n∑j=1ˉdij(t)Bj(xj(t−qij(t))) and n∑j=1n∑l=1θijl(t)Qj(xj(t−ηijl(t)))Ql(xl(t−ξijl(t))) are respectively the first-order term and the second-order term of the neural network, Aj, Bj and Qj are the nonlinear activation functions, τi=max1≤l,j≤n{supt∈Rqij(t), supt∈Rηijl(t),supt∈Rξijl(t)}, Ji,ˉcij,ˉdij,θijl,ˉai,ˉbi:R→R and qij, ηijl, ξijl:R→R+ are bounded and continuous functions, ˉai,ˉbi,qij, ηijl, ξijl are periodic functions with period T>0, the input term Ji is T-anti-periodic (Ji(t+T)=−Ji(t) for all t∈R), and i,j,l∈D:={1,2,⋯,n}.
Motivated by the above arguments, in this paper, without adopting the reduced order method, we propose a novel approach involving differential inequality techniques coupled with Lyapunov function method to demonstrate the existence and global exponential stability of anti-periodic solutions for system (1.1). Particularly, our results are new and supplement some corresponding ones of the existing literature [19,20,21,22,23,24,25,26,27,28]. In a nutshell, the contributions of this paper can be summarized as follows. 1) A class of anti-periodic high-order inertial Hopfield neural networks involving time-varying delays are proposed; 2) Under some appropriate anti-periodic assumptions, all solutions and their derivatives in the proposed neural networks model are guaranteed to converge to the anti-periodic solution and its derivative, respectively; 3) Numerical results including comparisons are presented to verify the obtained theoretical results.
The remaining parts of this paper are organized as follows. In Section 2, we make some preparations. In Section 3, the existence and the global exponential stability of the anti-periodic solution are stated and demonstrated. Section 4 shows numerical examples. Conclusions are drawn in Section 5.
To study the existence and uniqueness of anti-periodic solutions to system (1.1), we first require the following assumptions and some key lemmas:
Assumptions:
(F1) For i,j,l∈D, Aj(u),Bj(u),Qj(u) are all non-decreasing functions with Aj(0)=Bj(0)=Qj(0)=0, and there are nonnegative constants LAj, LBj, LQj and MQj such that
|Aj(u)−Aj(v)|≤LAj|u−v|, |Bj(u)−Bj(v)|≤LBj|u−v|, |Qj(u)−Qj(v)|≤LQj|u−v|, |
|Qj(u)|≤MQj, ˉcij(t+T)Aj(u)=−ˉcij(t)Aj(−u), ˉdij(t+T)Bj(u)=−ˉdij(t)Bj(−u), |
and
θijl(t+T)Qj(u)Ql(v)=−θijl(t)Qj(−u)Ql(−v), |
for all u, v∈R.
(F2) There are constants βi>0 and αi≥0,γi≥0 obeying
Ei(t)<0, 4Ei(t)Gi(t)>H2i(t), ∀t∈R, i∈D, | (2.1) |
where
Ei(t)=αiγi−ˉai(t)α2i+12α2in∑j=1(|ˉcij(t)|LAj+|ˉdij(t)|LBj) +12α2in∑j=1n∑l=1|θijl(t)|(MQjLQl+LQjMQl),Gi(t)=−ˉbi(t)αiγi+12n∑j=1(|ˉcij(t)|LAj+|ˉdij(t)|LBj)|αiγi| +12n∑j=1α2j(|ˉcji(t)|LAi+ˉd+jiLBi11−˙q+ji) +12n∑j=1(|ˉcji(t)|LAi+ˉd+jiLBi11−˙q+ji)|αjγj| +12n∑j=1n∑l=1|αiγi||θijl(t)|(MQjLQl+LQjMQl) +12n∑l=1n∑j=1(α2l+|αlγl|)θ+ljiMQjLQi11−˙ξ+lji +12n∑j=1n∑l=1(α2j+|αjγj|)θ+jilLQiMQl11−˙η+jil), |
Hi(t)=βi+γ2i−ˉai(t)αiγi−ˉbi(t)α2i, ˙q+ij=supt∈Rq′ij(t),˙η+ijl=supt∈Rη′ijl(t), ˙ξ+ijl=supt∈Rξ′ijl(t), q+ij=supt∈Rqij(t), η+ijl=supt∈Rηijl(t),ξ+ijl=supt∈Rξijl(t), ˉc+ij=supt∈R|ˉcij(t)|, ˉd+ij=supt∈R|ˉdij(t)|, i,j,l∈D. |
(F3) For i,j,l∈D, qij, ηijl and ξijl are continuously differentiable, q′ij(t)=˙qij(t)<1, η′ijl(t)=˙ηijl(t)<1 and ξ′ijl(t)=˙ξijl(t)<1 for all t∈R.
We will adopt the following notations:
θ+ijl=maxt∈[0,T]|θijl(t)|,i,j,l∈D. |
Remark 2.1. Since (1.1) can be converted into the first order functional differential equations. In view of (F1) and ([29], p176, Theorem 5.4), one can see that all solutions of (1.1) and (1.2) exist on [0, +∞).
Lemma 2.1. Under (F1), (F2) and (F3), label x(t)=(x1(t),x2(t),⋯,xn(t)) and y(t)=(y1(t),y2(t),⋯,yn(t)) as two solutions of system (1.1) satisfying
xi(s)=φxi(s), x′i(s)=ψxi(s), yi(s)=φyi(s), y′i(s)=ψyi(s), | (2.2) |
where −τi≤s≤0, i∈D, φxi,ψxi,φyi,ψyi∈C([−τi,0],R). Then, there are two positive constants λ and M=M(φx,ψx,φy,ψy) such that
|xi(t)−yi(t)|≤Me−λt, |x′i(t)−y′i(t)|≤Me−λt, for all t≥0, i∈D. |
Proof. Denote x(t)=(x1(t),x2(t),⋯,xn(t)) and y(t)=(y1(t),y2(t),⋯,yn(t)) as two solutions of (1.1) and (1.2). Let wi(t)=yi(t)−xi(t), then
w″i(t)=−ˉai(t)w′i(t)−ˉbi(t)wi(t)+n∑j=1ˉcij(t)˜Aj(wj(t))+n∑j=1ˉdij(t)˜Bj(wj(t−qij(t)))+n∑j=1n∑l=1θijl(t)[Qj(yj(t−ηijl(t)))Ql(yl(t−ξijl(t)))−Qj(yj(t−ηijl(t)))×Ql(xl(t−ξijl(t)))+Qj(yj(t−ηijl(t)))Ql(xl(t−ξijl(t)))−Qj(xj(t−ηijl(t)))Ql(xl(t−ξijl(t)))], | (2.3) |
where i,j∈D, ˜Aj(wj(t))=Aj(yj(t))−Aj(xj(t)) and
˜Bj(wj(t−qij(t)))=Bj(yj(t−qij(t)))−Bj(xj(t−qij(t))). |
According to (F2) and the periodicity in (1.1), one can select a constant λ>0 such that
Eλi(t)<0, 4Eλi(t)Gλi(t)>(Hλi(t))2, ∀t∈R, | (2.4) |
where
{Eλi(t)=λα2i+αiγi−ˉai(t)α2i+12α2in∑j=1(|ˉcij(t)|LAj+|ˉdij(t)|LBj) +12α2in∑j=1n∑l=1|θijl(t)|(MQjLQl+LQjMQl),Gλi(t)=−ˉbi(t)αiγi+λβi+λγ2i+12n∑j=1(|ˉcij(t)|LAj+|ˉdij(t)|LBj)|αiγi| +12n∑j=1α2j(|ˉcji(t)|LAi+ˉd+jiLBi11−˙q+jie2λq+ji) +12n∑j=1(|ˉcji(t)|LAi+ˉd+jiLBi11−˙q+jie2λq+ji)|αjγj| +12n∑j=1n∑l=1|αiγi||θijl(t)|(MQjLQl+LQjMQl) +12n∑l=1n∑j=1(α2l+|αlγl|)θ+ljiMQjLQie2λξ+lji11−˙ξ+lji +12n∑j=1n∑l=1(α2j+|αjγj|)θ+jilLQiMQle2λη+jil11−˙η+jil),Hλi(t)=βi+γ2i+2λαiγi−ˉai(t)αiγi−ˉbi(t)α2i, i,j∈D. |
Define the Lyapunov function by setting
K(t)=12n∑i=1βiw2i(t)e2λt+12n∑i=1(αiw′i(t)+γiwi(t))2e2λt+12n∑i=1n∑j=1(α2iˉd+ij+|αiγi|ˉd+ij)e2λq+ijLBj∫tt−qij(t)w2j(s)11−˙q+ije2λsds+12n∑i=1n∑l=1n∑j=1(α2l+|αlγl|)θ+ljiMQjLQie2λξ+lji∫tt−ξlji(t)w2i(s)11−˙ξ+ljie2λsds+12n∑i=1n∑j=1n∑l=1(α2j+|αjγj|)θ+jilLQiMQle2λη+jil∫tt−ηjil(t)w2i(s)11−˙η+jile2λsds. |
Straightforward computation yields that
K′(t)=2λ[12n∑i=1βiw2i(t)e2λt+12n∑i=1(αiw′i(t)+γiwi(t))2e2λt]+n∑i=1βiwi(t)w′i(t)e2λt+n∑i=1(αiw′i(t)+γiwi(t))(αiw″i(t)+γiw′i(t))e2λt+12n∑i=1n∑j=1(α2iˉd+ij+|αiγi|ˉd+ij)e2λq+ij11−˙q+ijLBj×[w2j(t)e2λt−w2j(t−qij(t))e2λ(t−qij(t))(1−q′ij(t))]+12n∑i=1n∑l=1n∑j=1(α2l+|αlγl|)θ+ljiMQjLQie2λξ+lji11−˙ξ+lji×[w2i(t)e2λt−w2i(t−ξlji(t))e2λ(t−ξlji(t))(1−ξ′lji(t))]+12n∑i=1n∑j=1n∑l=1(α2j+|αjγj|)θ+jilLQiMQle2λη+jil11−˙η+jil×[w2i(t)e2λt−w2i(t−ηjil(t))e2λ(t−ηjil(t))(1−η′jil(t))] |
=2λ[12n∑i=1βiw2i(t)e2λt+12n∑i=1(αiw′i(t)+γiwi(t))2e2λt]+n∑i=1(βi+γ2i)wi(t)w′i(t)e2λt+n∑i=1αi(αiw′i(t)+γiwi(t))e2λt×[−ˉai(t)w′i(t)−ˉbi(t)wi(t)+n∑j=1ˉcij(t)˜Aj(wj(t))+n∑j=1ˉdij(t)˜Bj(wj(t−qij(t)))+n∑j=1n∑l=1θijl(t)(Qj(yj(t−ηijl(t)))Ql(yl(t−ξijl(t)))−Qj(yj(t−ηijl(t)))Ql(xl(t−ξijl(t)))+Qj(yj(t−ηijl(t)))Ql(xl(t−ξijl(t)))−Qj(xj(t−ηijl(t)))Ql(xl(t−ξijl(t))))]+n∑i=1αiγi(w′i(t))2e2λt +12n∑i=1n∑j=1(α2iˉd+ij+|αiγi|ˉd+ij)e2λq+ijLBj×[w2j(t)11−˙q+ije2λt−w2j(t−qij(t))e2λ(t−qij(t))1−q′ij(t)1−˙q+ij]+12n∑i=1n∑l=1n∑j=1(α2l+|αlγl|)θ+ljiMQjLQie2λξ+lji×[w2i(t)11−˙ξ+ljie2λt−w2i(t−ξlji(t))e2λ(t−ξlji(t))1−ξ′lji(t)1−˙ξ+lji]+12n∑i=1n∑j=1n∑l=1(α2j+|αjγj|)θ+jilLQiMQle2λη+jil×[w2i(t)11−˙η+jile2λt−w2i(t−ηjil(t))e2λ(t−ηjil(t))1−η′jil(t)1−˙η+jil] |
≤e2λt{n∑i=1(βi+γ2i+2λαiγi−ˉai(t)αiγi−ˉbi(t)α2i)wi(t)w′i(t)+n∑i=1(λα2i+αiγi−ˉai(t)α2i)(w′i(t))2−n∑i=1(ˉbi(t)αiγi−λβi−λγ2i)w2i(t)+12n∑i=1n∑j=1(α2iˉd+ij+|αiγi|ˉd+ij)11−˙q+ije2λq+ijLBjw2j(t)+12n∑i=1n∑l=1n∑j=1(α2l+|αlγl|)θ+ljiMQjLQie2λξ+ljiw2i(t)11−˙ξ+lji+12n∑i=1n∑j=1n∑l=1(α2j+|αjγj|)θ+jilLQiMQle2λη+jilw2i(t)11−˙η+jil−12n∑i=1n∑j=1(α2iˉd+ij+|αiγi|ˉd+ij)LBjw2j(t−qij(t))−12n∑i=1n∑l=1n∑j=1(α2l+|αlγl|)θ+ljiMQjLQiw2i(t−ξlji(t))−12n∑i=1n∑j=1n∑l=1(α2j+|αjγj|)θ+jilLQiMQlw2i(t−ηjil(t))+n∑i=1n∑j=1(α2i|w′i(t)|+|αiγi||wi(t)|)|ˉcij(t)||˜Aj(wj(t))|+n∑i=1n∑j=1(α2i|w′i(t)|+|αiγi||wi(t)|)|ˉdij(t)||˜Bj(wj(t−qij(t)))|}+n∑i=1(α2i|w′i(t)|+|αiγi||wi(t)|)×n∑j=1n∑l=1|θijl(t)|(MQjLQl|wl(t−ξijl(t))|+LQj|wj(t−ηijl(t))|MQl)} |
=e2λt{n∑i=1(βi+γ2i+2λαiγi−ˉai(t)αiγi−ˉbi(t)α2i)wi(t)w′i(t)+n∑i=1(λα2i+αiγi−ˉai(t)α2i)(w′i(t))2+n∑i=1[−ˉbi(t)αiγi+λβi+λγ2i+12n∑j=1(α2jˉd+ji+|αjγj|ˉd+ji)11−˙q+jie2λq+jiLBi+12n∑l=1n∑j=1(α2l+|αlγl|)θ+ljiMQjLQie2λξ+lji11−˙ξ+lji+12n∑j=1n∑l=1(α2j+|αjγj|)θ+jilLQiMQle2λη+jil11−˙η+jil]w2i(t)−12n∑i=1n∑j=1(α2iˉd+ij+|αiγi|ˉd+ij)LBjw2j(t−qij(t))−12n∑i=1n∑l=1n∑j=1(α2l+|αlγl|)θ+ljiMQjLQiw2i(t−ξlji(t))−12n∑i=1n∑j=1n∑l=1(α2j+|αjγj|)θ+jilLQiMQlw2i(t−ηjil(t))+n∑i=1n∑j=1(α2i|w′i(t)|+|αiγi||wi(t)|)|ˉcij(t)||˜Aj(wj(t))|+n∑i=1n∑j=1(α2i|w′i(t)|+|αiγi||wi(t)|)|ˉdij(t)||˜Bj(wj(t−qij(t)))|}+n∑i=1(α2i|w′i(t)|+|αiγi||wi(t)|)n∑j=1n∑l=1|θijl(t)|×(MQjLQl|wl(t−ξijl(t))|+LQj|wj(t−ηijl(t))|MQl)}, ∀t∈[0,+∞). | (2.5) |
It follows from (F1) and PQ≤12(P2+Q2)(P,Q∈R) that
n∑i=1n∑j=1(α2i|w′i(t)|+|αiγi||wi(t)|)|ˉcij(t)||˜Aj(wj(t))|≤12n∑i=1n∑j=1α2i|ˉcij(t)|LAj((w′i(t))2+w2j(t))+12n∑i=1n∑j=1|αiγi||ˉcij(t)|LAj(w2i(t)+w2j(t))=12n∑i=1n∑j=1α2i|ˉcij(t)|LAj(w′i(t))2+12n∑i=1n∑j=1(|αiγi||ˉcij(t)|LAj+α2j|ˉcji(t)|LAi+|αjγj||ˉcji(t)|LAi)w2i(t), |
n∑i=1n∑j=1(α2i|w′i(t)|+|αiγi||wi(t)|)|ˉdij(t)||˜Bj(wj(t−qij(t)))|≤12n∑i=1n∑j=1α2i|ˉdij(t)|LBj((w′i(t))2+w2j(t−qij(t)))+12n∑i=1n∑j=1|αiγi||ˉdij(t)|LBj(w2i(t)+w2j(t−qij(t)))=12n∑i=1n∑j=1α2i|ˉdij(t)|LBj(w′i(t))2+12n∑i=1n∑j=1|αiγi||ˉdij(t)|LBjw2i(t)+12n∑i=1n∑j=1(α2i|ˉdij(t)|LBj+|αiγi||ˉdij(t)|LBj)w2j(t−qij(t)), |
and
n∑i=1(α2i|w′i(t)|+|αiγi||wi(t)|)×n∑j=1n∑l=1|θijl(t)|(MQjLQl|wl(t−ξijl(t))|+LQj|wj(t−ηijl(t))|MQl)≤12n∑i=1n∑j=1n∑l=1α2i|θijl(t)|MQjLQl((w′i(t))2+w2l(t−ξijl(t)))+12n∑i=1n∑j=1n∑l=1|αiγi||θijl(t)|MQjLQl((wi(t))2+w2l(t−ξijl(t)))+12n∑i=1n∑j=1n∑l=1α2i|θijl(t)|LQjMQl((w′i(t))2+w2j(t−ηijl(t)))+12n∑i=1n∑j=1n∑l=1|αiγi||θijl(t)|LQjMQl((wi(t))2+w2j(t−ηijl(t)))=12n∑i=1n∑j=1n∑l=1α2i|θijl(t)|(MQjLQl+LQjMQl)(w′i(t))2+12n∑i=1n∑j=1n∑l=1|αiγi||θijl(t)|(MQjLQl+LQjMQl)(wi(t))2+12n∑i=1n∑j=1n∑l=1(α2i+|αiγi|)|θijl(t)|MQjLQlw2l(t−ξijl(t))+12n∑i=1n∑j=1n∑l=1(α2i+|αiγi|)|θijl(t)|LQjMQlw2j(t−ηijl(t))=12n∑i=1n∑j=1n∑l=1α2i|θijl(t)|(MQjLQl+LQjMQl)(w′i(t))2+12n∑i=1n∑j=1n∑l=1|αiγi||θijl(t)|(MQjLQl+LQjMQl)(wi(t))2+12n∑l=1n∑j=1n∑i=1(α2l+|αlγl|)|θlji(t)|MQjLQiw2i(t−ξlji(t))+12n∑j=1n∑i=1n∑l=1(α2j+|αjγj|)|θjil(t)|LQiMQlw2i(t−ηjil(t)), |
which, together with (2.4) and (2.5), entails that
K′(t)≤e2λt{n∑i=1(βi+γ2i+2λαiγi−ˉai(t)αiγi−ˉbi(t)α2i)wi(t)w′i(t)+n∑i=1[λα2i+αiγi−ˉai(t)α2i+12α2in∑j=1(|ˉcij(t)|LAj+|ˉdij(t)|LBj)+12α2in∑j=1n∑l=1|θijl(t)|(MQjLQl+LQjMQl)](w′i(t))2+n∑i=1[−ˉbi(t)αiγi+λβi+λγ2i+12n∑j=1(|ˉcij(t)|LAj+|ˉdij(t)|LBj)|αiγi|+12n∑j=1α2j(|ˉcji(t)|LAi+ˉd+jiLBi11−˙q+jie2λq+ji)+12n∑j=1(|ˉcji(t)|LAi+ˉd+jiLBi11−˙q+jie2λq+ji)|αjγj|+12n∑j=1n∑l=1|αiγi||θijl(t)|(MQjLQl+LQjMQl)+12n∑l=1n∑j=1(α2l+|αlγl|)θ+ljiMQjLQie2λξ+lji11−˙ξ+lji+12n∑j=1n∑l=1(α2j+|αjγj|)θ+jilLQiMQle2λη+jil11−˙η+jil)]w2i(t)}=e2λt{n∑i=1(Eλi(t)(w′i(t))2+Gλi(t)w2i(t)+Hλi(t)wi(t)w′i(t))}=e2λt{n∑i=1Eλi(t)(w′i(t)+Hλi(t)2Eλi(t)wi(t))2+n∑i=1(Gλi(t)−(Hλi(t))24Eλi(t))w2i(t)}≤0, ∀t∈[0,+∞). |
This indicates that K(t)≤K(0) for all t∈[0,+∞), and
12n∑i=1βiw2i(t)e2λt+12n∑i=1(αiw′i(t)+γiwi(t))2e2λt≤K(0), t∈[0,+∞). |
Note that
(αiw′i(t)eλt+γiwi(t)eλt)2=(αiw′i(t)+γiwi(t))2e2λt |
and
αi|w′i(t)|eλt≤|αiw′i(t)eλt+γiwi(t)eλt|+|γiwi(t)eλt|, |
one can find a constant M>0 such that
|w′i(t)|≤Me−λt, |wi(t)|≤Me−λt, t≥0, i∈D, |
which proves Lemma 2.1.
Remark 2.2. Under the assumptions adopted in Lemma 2.1, if y(t) is an equilibrium point or a periodic solution of (1.1), one can see y(t) is globally exponentially stable. Moreover, the definition of global exponential stability can be also seen in [13,16].
Now, we set out the main result of this paper as follows.
Theorem 3.1. Under assumptions (F1)–(F3), system (1.1) possesses a global exponential stable T-anti-periodic solution.
Proof. Denote κ(t)=(κ1(t),κ2(t),⋯, κn(t)) be a solution of system (1.1) satisfying:
κi(s)=φκi(s), κ′i(s)=ψκi(s), −τi≤s≤0, φκi,ψκi∈C([−τi,0],R), i∈D. | (3.1) |
With the aid of (F1), one can see that
ˉai(t+T)=ˉai(t), ˉbi(t+T)=ˉbi(t), qij(t+T)=qij(t), |
ηijl(t+T)=ηijl(t), ξijl(t+T)=ξijl(t), Ji(t+T)=−Ji(t), |
(−1)m+1ˉcij(t+(m+1)T)Aj(κj(t+(m+1)T))=ˉcij(t)Aj((−1)m+1κj(t+(m+1)T)), |
(−1)m+1ˉdij(t+(m+1)T)Bj(κj(t+(m+1)T−qij(t)))=ˉdij(t)Bj((−1)m+1κj(t+(m+1)T−qij(t))), |
and
(−1)m+1θijl(t+(m+1)T)Qj(κj(t+(m+1)T−ηijl(t)))Ql(κl(t+(m+1)T−ξijl(t))) |
=θijl(t)Qj((−1)m+1κj(t+(m+1)T−ηijl(t)))Ql((−1)m+1κl(t+(m+1)T−ξijl(t))), |
where t∈R and i,j,l∈D.
Consequently, for any nonnegative integer m,
((−1)m+1κi(t+(m+1)T))″=−ˉai(t)((−1)m+1κi(t+(m+1)T))′−ˉbi(t)((−1)m+1κi(t+(m+1)T))+n∑j=1ˉcij(t)Aj((−1)m+1κj(t+(m+1)T))+n∑j=1ˉdij(t)Bj((−1)m+1κj(t+(m+1)T−qij(t)))+n∑j=1n∑l=1θijl(t)×Qj((−1)m+1κj(t+(m+1)T−ηijl(t)))×Ql((−1)m+1κl(t+(m+1)T−ξijl(t)))+Ji(t), for all i∈D,t+(m+1)T≥0. | (3.2) |
Clearly, (−1)m+1κ(t+(m+1)T) (t+(m+1)T≥0) satisfies (1.1), and v(t)=−κ(t+T) is a solution of system (1.1) involving initial values:
φvi(s)=−κi(s+T),ψvi(s)=−κ′i(s+T), for all s∈[−τi,0],i∈D. |
Thus, with the aid of Lemma 2.1, we can pick a constant M=M(φκ,ψκ,φv,ψv) satisfying
|κi(t)−vi(t)|≤Me−λt, |κ′i(t)−v′i(t)|≤Me−λt, for all t≥0,i∈D. |
Hence,
|(−1)pκi(t+pT)−(−1)p+1κi(t+(p+1)T)|=|κi(t+pT)−vi(t+pT)|≤Me−λ(t+pT),|((−1)pκi(t+pT))′−((−1)p+1κi(t+(p+1)T))′|=|κ′i(t+pT)−v′i(t+pT)|≤Me−λ(t+pT),} ∀i∈D, t+pT≥0. | (3.3) |
Consequently,
(−1)m+1κi(t+(m+1)T)=κi(t)+m∑p=0[(−1)p+1κi(t+(p+1)T)−(−1)pκi(t+pT)] (i∈D) |
and
((−1)m+1κi(t+(m+1)T))′=κ′i(t)+m∑p=0[((−1)p+1κi(t+(p+1)T))′−((−1)pκi(t+pT))′] (i∈D). |
Therefore, (3.3) suggests that there exists a continuous differentiable function y(t)=(y1(t),y2(t),⋯,yn(t)) such that {(−1)mκ(t+mT)}m≥1 and {((−1)mκ(t+mT))′}m≥1 are uniformly convergent to y(t) and y′(t) on any compact set of R, respectively.
Moreover,
y(t+T)=limm→+∞(−1)mκ(t+T+mT)=−lim(m+1)→+∞(−1)m+1κ(t+(m+1)T)=−y(t) |
involves that y(t) is T−anti-periodic on R. It follows from (F1)-(F3) and the continuity on (3.2) that {(κ″(t+(m+1)T)}m≥1 uniformly converges to a continuous function on any compact set of R. Furthermore, for any compact set of R, setting m⟶+∞, we obtain
y″i(t)=−ˉai(t)y′i(t)−ˉbi(t)yi(t)+n∑j=1ˉcij(t)Aj(yj(t))+n∑j=1ˉdij(t)Bj(yj(t−qij(t)))+n∑j=1n∑l=1θijl(t)Qj(yj(t−ηijl(t)))Ql(yl(t−ξijl(t)))+Ji(t),,i∈D, |
which involves that y(t) is a T−anti-periodic solution of (1.1). Again from Lemma 2.1, we gain that y(t) is globally exponentially stable. This finishes the proof of Theorem 3.1.
Remark 3.1. For inertial neural networks without high-order terms respectively, suppose
|ˉai−ˉbi|<2, Ai and Bi are bounded, i∈D, | (3.4) |
and
|ˉai−ˉbi+1|<1,i∈D, | (3.5) |
the authors gained the existence and stability on periodic solutions in [10,11] and anti-periodic solutions in [12]. Moreover, the reduced-order method was crucial in [10,11,12] when anti-periodicity and periodicity of second-order inertial neural networks were considered. However, (3.4) and (3.5) have been abandoned in Theorem 3.1 and the reduced-order method has been substituted in this paper. Therefore, our results on anti-periodicity of high-order inertial Hopfield neural networks are new and supplemental in nature.
Example 4.1. Let n=2, and consider a class of high-order inertial Hopfield neural networks in the form of
{x″1(t)=−14.92x′1(t)−27.89x1(t)+2.28(sint)A1(x1(t)) +2.19(cost)A2(x2(t)) −0.84(cos2t)B1(x1(t−0.2sin2t))+2.41(cos2t)B2(x2(t−0.3sin2t)) +4(sin2t)Q1(x1(t−0.4sin2t))Q2(x2(t−0.5sin2t))+55sint,x″2(t)=−15.11x′2(t)−31.05x2(t)−1.88(sint)A1(x1(t)) −2.33(cost)A2(x2(t)) −2.18(sin2t)B1(x1(t−0.2cos2t))+3.18(cos2t)B2(x2(t−0.3cos2t)) +3.8(sin2t)Q1(x1(t−0.4cos2t))Q2(x2(t−0.5cos2t))+48sint, | (4.1) |
where t≥0, A1(u)=A2(u)=135|u|, B1(u)=B2(u)=148u, Q1(u)=Q2(u)=155arctanu.
Using a direct calculation, one can check that (4.1) satisfies (2.4) and (F1)−(F3). Applying Theorem 3.1, it is obvious that system (4.1) has a globally exponentially stable π-anti-periodic solution. Simulations reflect that the theoretical anti-periodicity is in sympathy with the numerically observed behavior (Figures 1 and 2).
Example 4.2. Regard the following high-order inertial Hopfield neural networks involving time-varying delays and coefficients:
{x″1(t)=−(14+0.9|sint|)x′1(t)−(27+0.8|cost|)x1(t)+2.28(sint)A1(x1(t)) +2.19(cost)A2(x2(t)) −0.84(cos2t)B1(x1(t−0.2sin2t))+2.41(cos2t)B2(x2(t−0.3sin2t)) +4(sint)Q1(x1(t−0.4sin2t))Q2(x2(t−0.5sin2t))+100sint,x″2(t)=−(15+0.1|cost|)x′2(t)−(31+0.1|sint|)x2(t)−1.88(sint)A1(x1(t)) −2.33(cost)A2(x2(t)) −2.18(sin2t)B1(x1(t−0.2cos2t))+3.18(cos2t)B2(x2(t−0.3cos2t)) +3.8(sint)Q1(x1(t−0.4cos2t))Q2(x2(t−0.5cos2t))+200sint, | (4.2) |
where t≥0, A1(u)=A2(u)=135|u|, B1(u)=B2(u)=148u, Q1(u)=Q2(u)=1110(|x+1|−|x−1|). Then, by Theorem 3.1, one can find that all solutions of networks (4.2) are convergent to a π-anti-periodic solution (See Figures 3 and 4).
Remark 4.1. From the figures 1–4, one can see that the solution is similar to sinusoidal oscillation, and there exists a π-anti-periodic solution satisfying x(t+π)=−x(t). To the author's knowledge, the anti-periodicity on high-order inertial Hopfield neural networks involving time-varying delays has never been touched by using the non-reduced order method. Manifestly, the assumptions (3.4) and (3.5) adopted in [10,11] are invalid in systems (4.1) and (4.2). In addition, the most recently papers [10,11] only considered the polynomial power stability of some proportional time-delay systems, but not involved the exponential power stability of the addressed systems. And the results in [35,36,37,38,39,40,41,42,43,44,45,46,47,48,49,50,51,52,53,54,55,56,57,58,59,60,61,62,63,64,65,66,67,68,69,70,71,72,73,74, 75,76,77,78,79,80,81,82] have not touched on the anti-periodicity of inertial neural networks. This entails that the corresponding conclusions in [10,11,12,13,14,15,16,17,18,19,20,21,22,23,24,25,26,27,28,29,30,31,32,33,34,35,36,37,38,39,40,41,42,43,44,45,46,47,48, 49,50,51,52,53,54,55,56,57,58,59,60,61,62,63,64,65,66,67,68,69,70,71,72,73,74,75,76,77,78,79,80,81,82] and the references cited therein can not be applied to show the anti-periodic convergence for systems (4.1) and (4.2).
In this paper, abandoning the reduced order method, we apply inequality techniques and Lyapunov function method to establish the existence and global exponential stability of anti-periodic solutions for a class of high-order inertial Hopfield neural networks involving time-varying delays and anti-periodic environments. The obtained results are essentially new and complement some recently published results. The method proposed in this article furnishes a possible approach for studying anti-periodic on other types high-order inertial neural networks such as shunting inhibitory cellular neural networks, BAM neural networks, Cohen-Grossberg neural networks and so on.
The authors would like to express the sincere appreciation to the editor and reviewers for their helpful comments in improving the presentation and quality of the paper.
The authors confirm that they have no conflict of interest.
[1] |
T. Buckmaster, T. D. Drivas, S. Shkoller, V. Vicol, Simultaneous development of shocks and cusps for 2D Euler with azimuthal symmetry from smooth data, Ann. PDE, 8 (2022). https://doi.org/10.1007/s40818-022-00141-6 doi: 10.1007/s40818-022-00141-6
![]() |
[2] |
T. Buckmaster, S. Shkoller, V. Vicol, Formation of shocks for 2D isentropic compressible Euler, Commun. Pur. Appl. Math., 75 (2022), 2069–2120. https://doi.org/10.1002/cpa.21956 doi: 10.1002/cpa.21956
![]() |
[3] |
P. D. Lax, Development of singularities of solutions of nonlinear hyperbolic partial differential equations, J. Math. Phys., 5 (1964), 611–613. https://doi.org/10.1063/1.1704154 doi: 10.1063/1.1704154
![]() |
[4] |
T. P. Liu, Development of singularities in the nonlinear waves for quasi-linear hyperbolic partial differential equations, J. Differ. Equations, 33 (1979), 92–111. https://doi.org/10.1016/0022-0396(79)90082-2 doi: 10.1016/0022-0396(79)90082-2
![]() |
[5] |
T. C. Sideris, Formation of singularities in three-dimensional compressible fluids, Commun. Math. Phys., 101 (1985), 475–485. https://doi.org/10.1007/BF01210741 doi: 10.1007/BF01210741
![]() |
[6] |
G. Chen, R. Pan, S. Zhu, Singularity formation for the compressible Euler equations, SIAM J. Math. Anal., 49 (2017), 2591–2614. https://doi.org/10.1137/16M1062818 doi: 10.1137/16M1062818
![]() |
[7] |
G. Chen, Optimal density lower bound on nonisentropic gas dynamics, J. Differ. Equations, 268 (2020), 4017–4028. https://doi.org/10.1016/j.jde.2019.10.017 doi: 10.1016/j.jde.2019.10.017
![]() |
[8] | C. Dafermos, Hyperbolic Conservation Laws in Continuum Physics, Springer, 2005. https://doi.org/10.1007/978-3-662-49451-6 |
[9] | G. Q. Chen, D. Wang, The Cauchy problem for the Euler equations for compressible fluids, in Handbook of mathematical fluid dynamics, Vol. I (eds. S. Friedlander, and D. Serre), North-Holland, Amsterdam (2002), 421–543. https://doi.org/10.1016/s1874-5792(02)80012-x |
[10] | T. P. Liu, Shock Waves, American Mathematical Society, Providence, RI, 2021. |
[11] |
J. Rauch, BV estimates fail for most quasilinear hyperbolic systems in dimensions greater than one, Commun. Math. Phys., 106 (1986), 481–484. https://doi.org/10.1007/BF01207258 doi: 10.1007/BF01207258
![]() |
[12] | M. P. Lebaud, Description de la formation d'un choc dans le p-système, Journal de mathématiques pures et appliquées, 73 (1994), 523–566. |
[13] |
S. Chen, L. Dong, Formation and construction of shock for p-system, Sci. China Ser. A-Math., 44 (2001), 1139–1147. https://doi.org/10.1007/BF02877431 doi: 10.1007/BF02877431
![]() |
[14] |
D. X. Kong, Formation and propagation of singularities for 2×2 quasilinear hyperbolic systems, Trans. Amer. Math. Soc., 354 (2002), 3155–3179. https://doi.org/10.1090/S0002-9947-02-02982-3 doi: 10.1090/S0002-9947-02-02982-3
![]() |
[15] | T. Buckmaster, T. D. Drivas, S. Shkoller, V. Vicol, Formation and development of singularities for the compressible Euler equations, in Proceedings of the International Congress of Mathematicians 2022, EMS Press, Berlin, 5 (2023), 3636–3659. https://doi.org/10.4171/ICM2022/87 |
[16] | D. Christodoulou, The formation of shocks in 3-dimensional fluids, European Mathematical Society, 2007. https://doi.org/10.4171/031 |
[17] | D. Christodoulou, The shock development problem, European Mathematical Society, 2019. https://doi.org/10.4171/192 |
[18] |
H. Yin, Formation and construction of a shock wave for 3-d compressible Euler equations with the spherical initial data, Nagoya Math. J., 175 (2004), 125–164. https://doi.org/10.1017/S002776300000893X doi: 10.1017/S002776300000893X
![]() |
[19] |
D. Christodoulou, A. Lisibach, Shock development in spherical symmetry, Ann. PDE, 2 (2016). https://doi.org/10.1007/s40818-016-0009-1 doi: 10.1007/s40818-016-0009-1
![]() |
[20] |
J. Luk, J. Speck, Shock formation in solutions to the 2d compressible Euler equations in the presence of non-zero vorticity, Invent. Mathe., 214 (2018), 1–169. https://doi.org/10.1007/s00222-018-0799-8 doi: 10.1007/s00222-018-0799-8
![]() |
[21] |
J. Luk, J. Speck, The stability of simple plane-symmetric shock formation for 3d compressible Euler flow with vorticity and entropy, Anal PDE, 17 (2024), 831–941. https://doi.org/10.2140/apde.2024.17.831 doi: 10.2140/apde.2024.17.831
![]() |
[22] |
T. Buckmaster, S. Shkoller, V. Vicol, Formation of point shocks for 3D compressible Euler, Comm. Pur. Appl. Math., 76 (2023), 2073–2191. https://doi.org/10.1002/cpa.22068 doi: 10.1002/cpa.22068
![]() |
[23] |
T. Buckmaster, S. Shkoller, V. Vicol, Shock formation and vorticity creation for 3D Euler, Comm. Pur. Appl. Math., 76 (2023), 1965–2072. https://doi.org/10.1002/cpa.22067 doi: 10.1002/cpa.22067
![]() |
[24] |
S. Shkoller, V. Vicol, The geometry of maximal development and shock formation for the Euler equations in multiple space dimensions, Invent. Math., 237 (2024), 871–1252. https://doi.org/10.1007/s00222-024-01269-x doi: 10.1007/s00222-024-01269-x
![]() |
[25] | L. Abbrescia, J. Speck, The emergence of the singular boundary from the crease in 3d compressible Euler flow, preprint, arXiv: 2207.07107. |
[26] | L. D. Landau, E. M. Lifshitz, Fluid Mechanics, Vol. 6, Elsevier, 1987. https://doi.org/10.1016/C2013-0-03799-1 |
[27] | R. J. Walker, Algebraic Curves, Springer-Verlag, 1950. |
[28] | E. Brieskorn, H. Knörrer, Plane algebraic curves, Springer Basel, 1986. https://doi.org/10.1007/978-3-0348-5097-1 |
[29] |
S. Kumagai, An implicit function theorem: comment, J Optim Theory Appl, 31 (1980), 285–288. https://doi.org/10.1007/BF00934117 doi: 10.1007/BF00934117
![]() |
1. | Xin Long, Novel stability criteria on a patch structure Nicholson’s blowflies model with multiple pairs of time-varying delays, 2020, 5, 2473-6988, 7387, 10.3934/math.2020473 | |
2. | Luogen Yao, Qian Cao, Anti-periodicity on high-order inertial Hopfield neural networks involving mixed delays, 2020, 2020, 1029-242X, 10.1186/s13660-020-02444-3 | |
3. | Qian Cao, Xin Long, New convergence on inertial neural networks with time-varying delays and continuously distributed delays, 2020, 5, 2473-6988, 5955, 10.3934/math.2020381 | |
4. | Ramazan Yazgan, Salsabil Hajjaji, Farouk Chérif, Weighted Pseudo Almost-Automorphic Solutions of Quaternion-Valued RNNs With Mixed Delays, 2023, 55, 1370-4621, 423, 10.1007/s11063-022-10890-x | |
5. | Yanli Xu, Periodic dynamic on a class of neutral-type Rayleigh equations incorporating D operators, 2023, 0952-813X, 1, 10.1080/0952813X.2023.2165720 | |
6. | M. Sai Pravallika, S. Vasavi, S. P. Vighneshwar, Prediction of temperature anomaly in Indian Ocean based on autoregressive long short-term memory neural network, 2022, 34, 0941-0643, 7537, 10.1007/s00521-021-06878-8 | |
7. | Weiping Fan, Anti-periodic stability on a class of neutral-type Rayleigh equations involving D operators, 2022, 0952-813X, 1, 10.1080/0952813X.2022.2153275 | |
8. | Xiaojin Guo, Chuangxia Huang, Zhichun Yang, Jiping Zhang, Jinde Cao, Stability Analysis of High-order Proportional Delayed Cellular Neural Networks with D Operators, 2022, 20, 1598-6446, 660, 10.1007/s12555-020-0902-y | |
9. | Zhenjie Wang, Wenxia Cui, Wenbin Jin, Finite-time synchronization for fuzzy inertial cellular neural networks with time-varying delays via integral inequality, 2021, 41, 10641246, 3653, 10.3233/JIFS-211065 | |
10. | Jian Zhang, Ancheng Chang, Gang Yang, Periodicity on Neutral-Type Inertial Neural Networks Incorporating Multiple Delays, 2021, 13, 2073-8994, 2231, 10.3390/sym13112231 | |
11. | Chaouki Aouiti, Mahjouba Ben Rezeg, Impulsive multidirectional associative memory neural networks: New results, 2021, 14, 1793-5245, 10.1142/S1793524521500601 | |
12. | Xiaojin Guo, Chuangxia Huang, Jinde Cao, Nonnegative periodicity on high-order proportional delayed cellular neural networks involving $ D $ operator, 2020, 6, 2473-6988, 2228, 10.3934/math.2021135 | |
13. | Ankit Kumar, Subir Das, Vijay K. Yadav, Jinde Cao, Chuangxia Huang, Synchronizations of fuzzy cellular neural networks with proportional time-delay, 2021, 6, 2473-6988, 10620, 10.3934/math.2021617 | |
14. | Weiping Fan, Periodic Stability on a Class of D-Operator-Based Neutral-Type Rayleigh Equations Accompanying Mixed Delays, 2022, 1370-4621, 10.1007/s11063-022-11033-y | |
15. | Bing Hao, Tianwei Zhang, Stability analysis and synchronized control of fuzzy Mittag-Leffler discrete-time genetic regulatory networks with time delays, 2022, 43, 10641246, 587, 10.3233/JIFS-212361 | |
16. | Yuehong Zhang, Zhiying Li, Wangdong Jiang, Wei Liu, The stability of anti-periodic solutions for fractional-order inertial BAM neural networks with time-delays, 2023, 8, 2473-6988, 6176, 10.3934/math.2023312 | |
17. | Jin Gao, Lihua Dai, Anti-periodic synchronization of quaternion-valued high-order Hopfield neural networks with delays, 2022, 7, 2473-6988, 14051, 10.3934/math.2022775 | |
18. | Chengjie Chen, Fuhong Min, Yunzhen Zhang, Han Bao, ReLU-type Hopfield neural network with analog hardware implementation, 2023, 167, 09600779, 113068, 10.1016/j.chaos.2022.113068 | |
19. | Lilun Zhang, Le Li, Chuangxia Huang, Positive stability analysis of pseudo almost periodic solutions for HDCNNs accompanying $ D $ operator, 2022, 15, 1937-1632, 1651, 10.3934/dcdss.2021160 | |
20. | Xianhui Zhang, Le Li, Changchun Bao, Jian Zhang, Anti-periodicity on inertial Cohen-Grossberg neural networks involving distributed delays, 2023, 0952-813X, 1, 10.1080/0952813X.2023.2196976 | |
21. | Mei Xu, Honghui Yin, Bo Du, Periodic Solution Problems for a Class of Hebbian-Type Networks with Time-Varying Delays, 2023, 15, 2073-8994, 1985, 10.3390/sym15111985 | |
22. | Chenyang Li, Kit Ian Kou, Yanlin Zhang, Yang Liu, Advancements in exponential synchronization and encryption techniques: Quaternion-Valued Artificial Neural Networks with two-sided coefficients, 2024, 08936080, 106982, 10.1016/j.neunet.2024.106982 |