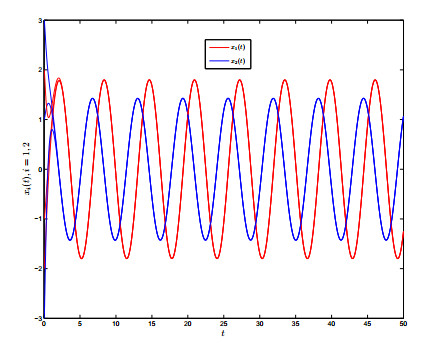
Psychiatric disorders may extremely impair the quality of life with patients and are important reasons of social disability. Several data have shown that psychiatric disorders are associated with an altered composition of gut microbiota. Dietary intake could determine the microbiota, which contribute to produce various metabolites of fermentation such as short chain fatty acids. Some of the metabolites could result in epigenetic alterations leading to the disease susceptibility. Epigenetic dysfunction is in fact implicated in various psychiatric and neurologic disorders. For example, it has been shown that neuroepigenetic dysregulation occurs in psychiatric disorders including schizophrenia. Several studies have demonstrated that the intestinal microbiome may influence the function of central nervous system. Furthermore, it has been proved that the alterations in the gut microbiota-composition might affect in the bidirectional communication between gut and brain. Similarly, evidences demonstrating the association between psychiatric disorders and the gut microbiota have come from preclinical studies. It is clear that an intricate symbiotic relationship might exist between host and microbe, although the practical significance of the gut microbiota has not yet to be determined. In this review, we have summarized the function of gut microbiota in main psychiatric disorders with respect to the mental health. In addition, we would like to discuss the potential mechanisms of the disorders for the practical diagnosis and future treatment by using bioengineering of microbiota and their metabolites.
Citation: Kurumi Taniguchi, Yuka Ikeda, Nozomi Nagase, Ai Tsuji, Yasuko Kitagishi, Satoru Matsuda. Implications of Gut-Brain axis in the pathogenesis of Psychiatric disorders[J]. AIMS Bioengineering, 2021, 8(4): 243-256. doi: 10.3934/bioeng.2021021
[1] | Jin Gao, Lihua Dai . Anti-periodic synchronization of quaternion-valued high-order Hopfield neural networks with delays. AIMS Mathematics, 2022, 7(8): 14051-14075. doi: 10.3934/math.2022775 |
[2] | Yuehong Zhang, Zhiying Li, Wangdong Jiang, Wei Liu . The stability of anti-periodic solutions for fractional-order inertial BAM neural networks with time-delays. AIMS Mathematics, 2023, 8(3): 6176-6190. doi: 10.3934/math.2023312 |
[3] | Xiaofang Meng, Yongkun Li . Pseudo almost periodic solutions for quaternion-valued high-order Hopfield neural networks with time-varying delays and leakage delays on time scales. AIMS Mathematics, 2021, 6(9): 10070-10091. doi: 10.3934/math.2021585 |
[4] | Nina Huo, Bing Li, Yongkun Li . Global exponential stability and existence of almost periodic solutions in distribution for Clifford-valued stochastic high-order Hopfield neural networks with time-varying delays. AIMS Mathematics, 2022, 7(3): 3653-3679. doi: 10.3934/math.2022202 |
[5] | Huizhen Qu, Jianwen Zhou . $ S $-asymptotically $ \omega $-periodic dynamics in a fractional-order dual inertial neural networks with time-varying lags. AIMS Mathematics, 2022, 7(2): 2782-2809. doi: 10.3934/math.2022154 |
[6] | Dan-Ning Xu, Zhi-Ying Li . Mittag-Leffler stabilization of anti-periodic solutions for fractional-order neural networks with time-varying delays. AIMS Mathematics, 2023, 8(1): 1610-1619. doi: 10.3934/math.2023081 |
[7] | Ailing Li, Xinlu Ye . Finite-time anti-synchronization for delayed inertial neural networks via the fractional and polynomial controllers of time variable. AIMS Mathematics, 2021, 6(8): 8173-8190. doi: 10.3934/math.2021473 |
[8] | Huahai Qiu, Li Wan, Zhigang Zhou, Qunjiao Zhang, Qinghua Zhou . Global exponential periodicity of nonlinear neural networks with multiple time-varying delays. AIMS Mathematics, 2023, 8(5): 12472-12485. doi: 10.3934/math.2023626 |
[9] | Yanshou Dong, Junfang Zhao, Xu Miao, Ming Kang . Piecewise pseudo almost periodic solutions of interval general BAM neural networks with mixed time-varying delays and impulsive perturbations. AIMS Mathematics, 2023, 8(9): 21828-21855. doi: 10.3934/math.20231113 |
[10] | Xiaojin Guo, Chuangxia Huang, Jinde Cao . Nonnegative periodicity on high-order proportional delayed cellular neural networks involving $ D $ operator. AIMS Mathematics, 2021, 6(3): 2228-2243. doi: 10.3934/math.2021135 |
Psychiatric disorders may extremely impair the quality of life with patients and are important reasons of social disability. Several data have shown that psychiatric disorders are associated with an altered composition of gut microbiota. Dietary intake could determine the microbiota, which contribute to produce various metabolites of fermentation such as short chain fatty acids. Some of the metabolites could result in epigenetic alterations leading to the disease susceptibility. Epigenetic dysfunction is in fact implicated in various psychiatric and neurologic disorders. For example, it has been shown that neuroepigenetic dysregulation occurs in psychiatric disorders including schizophrenia. Several studies have demonstrated that the intestinal microbiome may influence the function of central nervous system. Furthermore, it has been proved that the alterations in the gut microbiota-composition might affect in the bidirectional communication between gut and brain. Similarly, evidences demonstrating the association between psychiatric disorders and the gut microbiota have come from preclinical studies. It is clear that an intricate symbiotic relationship might exist between host and microbe, although the practical significance of the gut microbiota has not yet to be determined. In this review, we have summarized the function of gut microbiota in main psychiatric disorders with respect to the mental health. In addition, we would like to discuss the potential mechanisms of the disorders for the practical diagnosis and future treatment by using bioengineering of microbiota and their metabolites.
Alzheimer's disease;
attention deficit hyperactivity disorder;
autism spectrum disorder;
blood brain barrier;
central nervous system;
deoxyribonucleic acid;
gamma amino butyric acid;
gastrointestinal;
G protein-coupled receptor;
histone deacetylases;
lipopolysaccharide;
reactive oxygen species;
superoxide dismutase
Due to the engineering backgrounds and strong biological significance, Babcock and Westervelt [1,2] introduced an inertial term into the traditional multidirectional associative memory neural networks, and established a class of second order delay differential equations, which was called as the famous delayed inertial neural networks model. Arising from problems in different applied sciences such as mathematical physics, control theory, biology in different situations, nonlinear vibration, mechanics, electromagnetic theory and other related fields, the periodic oscillation is an important qualitative property of nonlinear differential equations [3,4,5,6,7,8,9]. Consequently, assuming that the activation functions are bounded and employing reduced-order variable substitution which convert the inertial systems into the first order differential equations, the authors in [10,11] and [12] have respectively gained the existence and stability of anti-periodic solution and periodic solution for addressed inertial neural networks models. Manifestly, the above transformation will raise the dimension in the inertial neural networks system, then some new parameters need to be introduced. This will increase huge amount of computation and be attained hard in practice [13,14]. For the above reasons, most recently, avoiding the reduced order method, the authors in [15] and [16] respectively developed some non-reduced order methods to establish the existence and stability of periodic solutions for inertial neural networks with time-varying delays.
It has been recognized that, in neural networks dynamics touching the communication, economics, biology or ecology areas, the relevant state variables are often considered as proteins and molecules, light intensity levels or electric charge, and they are naturally anti-periodic [17,18,19]. Such neural networks systems are often regarded as anti-periodic systems. Therefore, the convergence analysis and stability on the anti-periodic solutions in various neural networks systems with delays have attracted the interest of many researchers and some excellent results are reported in [20,21,22,23,24,25,26,27]. In particular, the anti-periodicity on inertial quaternion-valued high-order Hopfield neural networks with state-dependent delays has been established in [28] by employing reduced-order variable substitution. However, few researchers have utilized the non-reduced order methods to explore such topics on the following high-order inertial Hopfield neural networks involving time-varying delays:
x″i(t)=−ˉai(t)x′i(t)−ˉbi(t)xi(t)+n∑j=1ˉcij(t)Aj(xj(t))+n∑j=1ˉdij(t)Bj(xj(t−qij(t)))+n∑j=1n∑l=1θijl(t)Qj(xj(t−ηijl(t)))Ql(xl(t−ξijl(t)))+Ji(t), t≥0, | (1.1) |
associating with initial value conditions:
xi(s)=φi(s), x′i(s)=ψi(s), −τi≤s≤0, φi, ψi∈C([−τi,0],R), | (1.2) |
where n∑j=1ˉcij(t)Aj(xj(t)), n∑j=1ˉdij(t)Bj(xj(t−qij(t))) and n∑j=1n∑l=1θijl(t)Qj(xj(t−ηijl(t)))Ql(xl(t−ξijl(t))) are respectively the first-order term and the second-order term of the neural network, Aj, Bj and Qj are the nonlinear activation functions, τi=max1≤l,j≤n{supt∈Rqij(t), supt∈Rηijl(t),supt∈Rξijl(t)}, Ji,ˉcij,ˉdij,θijl,ˉai,ˉbi:R→R and qij, ηijl, ξijl:R→R+ are bounded and continuous functions, ˉai,ˉbi,qij, ηijl, ξijl are periodic functions with period T>0, the input term Ji is T-anti-periodic (Ji(t+T)=−Ji(t) for all t∈R), and i,j,l∈D:={1,2,⋯,n}.
Motivated by the above arguments, in this paper, without adopting the reduced order method, we propose a novel approach involving differential inequality techniques coupled with Lyapunov function method to demonstrate the existence and global exponential stability of anti-periodic solutions for system (1.1). Particularly, our results are new and supplement some corresponding ones of the existing literature [19,20,21,22,23,24,25,26,27,28]. In a nutshell, the contributions of this paper can be summarized as follows. 1) A class of anti-periodic high-order inertial Hopfield neural networks involving time-varying delays are proposed; 2) Under some appropriate anti-periodic assumptions, all solutions and their derivatives in the proposed neural networks model are guaranteed to converge to the anti-periodic solution and its derivative, respectively; 3) Numerical results including comparisons are presented to verify the obtained theoretical results.
The remaining parts of this paper are organized as follows. In Section 2, we make some preparations. In Section 3, the existence and the global exponential stability of the anti-periodic solution are stated and demonstrated. Section 4 shows numerical examples. Conclusions are drawn in Section 5.
To study the existence and uniqueness of anti-periodic solutions to system (1.1), we first require the following assumptions and some key lemmas:
Assumptions:
(F1) For i,j,l∈D, Aj(u),Bj(u),Qj(u) are all non-decreasing functions with Aj(0)=Bj(0)=Qj(0)=0, and there are nonnegative constants LAj, LBj, LQj and MQj such that
|Aj(u)−Aj(v)|≤LAj|u−v|, |Bj(u)−Bj(v)|≤LBj|u−v|, |Qj(u)−Qj(v)|≤LQj|u−v|, |
|Qj(u)|≤MQj, ˉcij(t+T)Aj(u)=−ˉcij(t)Aj(−u), ˉdij(t+T)Bj(u)=−ˉdij(t)Bj(−u), |
and
θijl(t+T)Qj(u)Ql(v)=−θijl(t)Qj(−u)Ql(−v), |
for all u, v∈R.
(F2) There are constants βi>0 and αi≥0,γi≥0 obeying
Ei(t)<0, 4Ei(t)Gi(t)>H2i(t), ∀t∈R, i∈D, | (2.1) |
where
Ei(t)=αiγi−ˉai(t)α2i+12α2in∑j=1(|ˉcij(t)|LAj+|ˉdij(t)|LBj) +12α2in∑j=1n∑l=1|θijl(t)|(MQjLQl+LQjMQl),Gi(t)=−ˉbi(t)αiγi+12n∑j=1(|ˉcij(t)|LAj+|ˉdij(t)|LBj)|αiγi| +12n∑j=1α2j(|ˉcji(t)|LAi+ˉd+jiLBi11−˙q+ji) +12n∑j=1(|ˉcji(t)|LAi+ˉd+jiLBi11−˙q+ji)|αjγj| +12n∑j=1n∑l=1|αiγi||θijl(t)|(MQjLQl+LQjMQl) +12n∑l=1n∑j=1(α2l+|αlγl|)θ+ljiMQjLQi11−˙ξ+lji +12n∑j=1n∑l=1(α2j+|αjγj|)θ+jilLQiMQl11−˙η+jil), |
Hi(t)=βi+γ2i−ˉai(t)αiγi−ˉbi(t)α2i, ˙q+ij=supt∈Rq′ij(t),˙η+ijl=supt∈Rη′ijl(t), ˙ξ+ijl=supt∈Rξ′ijl(t), q+ij=supt∈Rqij(t), η+ijl=supt∈Rηijl(t),ξ+ijl=supt∈Rξijl(t), ˉc+ij=supt∈R|ˉcij(t)|, ˉd+ij=supt∈R|ˉdij(t)|, i,j,l∈D. |
(F3) For i,j,l∈D, qij, ηijl and ξijl are continuously differentiable, q′ij(t)=˙qij(t)<1, η′ijl(t)=˙ηijl(t)<1 and ξ′ijl(t)=˙ξijl(t)<1 for all t∈R.
We will adopt the following notations:
θ+ijl=maxt∈[0,T]|θijl(t)|,i,j,l∈D. |
Remark 2.1. Since (1.1) can be converted into the first order functional differential equations. In view of (F1) and ([29], p176, Theorem 5.4), one can see that all solutions of (1.1) and (1.2) exist on [0, +∞).
Lemma 2.1. Under (F1), (F2) and (F3), label x(t)=(x1(t),x2(t),⋯,xn(t)) and y(t)=(y1(t),y2(t),⋯,yn(t)) as two solutions of system (1.1) satisfying
xi(s)=φxi(s), x′i(s)=ψxi(s), yi(s)=φyi(s), y′i(s)=ψyi(s), | (2.2) |
where −τi≤s≤0, i∈D, φxi,ψxi,φyi,ψyi∈C([−τi,0],R). Then, there are two positive constants λ and M=M(φx,ψx,φy,ψy) such that
|xi(t)−yi(t)|≤Me−λt, |x′i(t)−y′i(t)|≤Me−λt, for all t≥0, i∈D. |
Proof. Denote x(t)=(x1(t),x2(t),⋯,xn(t)) and y(t)=(y1(t),y2(t),⋯,yn(t)) as two solutions of (1.1) and (1.2). Let wi(t)=yi(t)−xi(t), then
w″i(t)=−ˉai(t)w′i(t)−ˉbi(t)wi(t)+n∑j=1ˉcij(t)˜Aj(wj(t))+n∑j=1ˉdij(t)˜Bj(wj(t−qij(t)))+n∑j=1n∑l=1θijl(t)[Qj(yj(t−ηijl(t)))Ql(yl(t−ξijl(t)))−Qj(yj(t−ηijl(t)))×Ql(xl(t−ξijl(t)))+Qj(yj(t−ηijl(t)))Ql(xl(t−ξijl(t)))−Qj(xj(t−ηijl(t)))Ql(xl(t−ξijl(t)))], | (2.3) |
where i,j∈D, ˜Aj(wj(t))=Aj(yj(t))−Aj(xj(t)) and
˜Bj(wj(t−qij(t)))=Bj(yj(t−qij(t)))−Bj(xj(t−qij(t))). |
According to (F2) and the periodicity in (1.1), one can select a constant λ>0 such that
Eλi(t)<0, 4Eλi(t)Gλi(t)>(Hλi(t))2, ∀t∈R, | (2.4) |
where
{Eλi(t)=λα2i+αiγi−ˉai(t)α2i+12α2in∑j=1(|ˉcij(t)|LAj+|ˉdij(t)|LBj) +12α2in∑j=1n∑l=1|θijl(t)|(MQjLQl+LQjMQl),Gλi(t)=−ˉbi(t)αiγi+λβi+λγ2i+12n∑j=1(|ˉcij(t)|LAj+|ˉdij(t)|LBj)|αiγi| +12n∑j=1α2j(|ˉcji(t)|LAi+ˉd+jiLBi11−˙q+jie2λq+ji) +12n∑j=1(|ˉcji(t)|LAi+ˉd+jiLBi11−˙q+jie2λq+ji)|αjγj| +12n∑j=1n∑l=1|αiγi||θijl(t)|(MQjLQl+LQjMQl) +12n∑l=1n∑j=1(α2l+|αlγl|)θ+ljiMQjLQie2λξ+lji11−˙ξ+lji +12n∑j=1n∑l=1(α2j+|αjγj|)θ+jilLQiMQle2λη+jil11−˙η+jil),Hλi(t)=βi+γ2i+2λαiγi−ˉai(t)αiγi−ˉbi(t)α2i, i,j∈D. |
Define the Lyapunov function by setting
K(t)=12n∑i=1βiw2i(t)e2λt+12n∑i=1(αiw′i(t)+γiwi(t))2e2λt+12n∑i=1n∑j=1(α2iˉd+ij+|αiγi|ˉd+ij)e2λq+ijLBj∫tt−qij(t)w2j(s)11−˙q+ije2λsds+12n∑i=1n∑l=1n∑j=1(α2l+|αlγl|)θ+ljiMQjLQie2λξ+lji∫tt−ξlji(t)w2i(s)11−˙ξ+ljie2λsds+12n∑i=1n∑j=1n∑l=1(α2j+|αjγj|)θ+jilLQiMQle2λη+jil∫tt−ηjil(t)w2i(s)11−˙η+jile2λsds. |
Straightforward computation yields that
K′(t)=2λ[12n∑i=1βiw2i(t)e2λt+12n∑i=1(αiw′i(t)+γiwi(t))2e2λt]+n∑i=1βiwi(t)w′i(t)e2λt+n∑i=1(αiw′i(t)+γiwi(t))(αiw″i(t)+γiw′i(t))e2λt+12n∑i=1n∑j=1(α2iˉd+ij+|αiγi|ˉd+ij)e2λq+ij11−˙q+ijLBj×[w2j(t)e2λt−w2j(t−qij(t))e2λ(t−qij(t))(1−q′ij(t))]+12n∑i=1n∑l=1n∑j=1(α2l+|αlγl|)θ+ljiMQjLQie2λξ+lji11−˙ξ+lji×[w2i(t)e2λt−w2i(t−ξlji(t))e2λ(t−ξlji(t))(1−ξ′lji(t))]+12n∑i=1n∑j=1n∑l=1(α2j+|αjγj|)θ+jilLQiMQle2λη+jil11−˙η+jil×[w2i(t)e2λt−w2i(t−ηjil(t))e2λ(t−ηjil(t))(1−η′jil(t))] |
=2λ[12n∑i=1βiw2i(t)e2λt+12n∑i=1(αiw′i(t)+γiwi(t))2e2λt]+n∑i=1(βi+γ2i)wi(t)w′i(t)e2λt+n∑i=1αi(αiw′i(t)+γiwi(t))e2λt×[−ˉai(t)w′i(t)−ˉbi(t)wi(t)+n∑j=1ˉcij(t)˜Aj(wj(t))+n∑j=1ˉdij(t)˜Bj(wj(t−qij(t)))+n∑j=1n∑l=1θijl(t)(Qj(yj(t−ηijl(t)))Ql(yl(t−ξijl(t)))−Qj(yj(t−ηijl(t)))Ql(xl(t−ξijl(t)))+Qj(yj(t−ηijl(t)))Ql(xl(t−ξijl(t)))−Qj(xj(t−ηijl(t)))Ql(xl(t−ξijl(t))))]+n∑i=1αiγi(w′i(t))2e2λt +12n∑i=1n∑j=1(α2iˉd+ij+|αiγi|ˉd+ij)e2λq+ijLBj×[w2j(t)11−˙q+ije2λt−w2j(t−qij(t))e2λ(t−qij(t))1−q′ij(t)1−˙q+ij]+12n∑i=1n∑l=1n∑j=1(α2l+|αlγl|)θ+ljiMQjLQie2λξ+lji×[w2i(t)11−˙ξ+ljie2λt−w2i(t−ξlji(t))e2λ(t−ξlji(t))1−ξ′lji(t)1−˙ξ+lji]+12n∑i=1n∑j=1n∑l=1(α2j+|αjγj|)θ+jilLQiMQle2λη+jil×[w2i(t)11−˙η+jile2λt−w2i(t−ηjil(t))e2λ(t−ηjil(t))1−η′jil(t)1−˙η+jil] |
≤e2λt{n∑i=1(βi+γ2i+2λαiγi−ˉai(t)αiγi−ˉbi(t)α2i)wi(t)w′i(t)+n∑i=1(λα2i+αiγi−ˉai(t)α2i)(w′i(t))2−n∑i=1(ˉbi(t)αiγi−λβi−λγ2i)w2i(t)+12n∑i=1n∑j=1(α2iˉd+ij+|αiγi|ˉd+ij)11−˙q+ije2λq+ijLBjw2j(t)+12n∑i=1n∑l=1n∑j=1(α2l+|αlγl|)θ+ljiMQjLQie2λξ+ljiw2i(t)11−˙ξ+lji+12n∑i=1n∑j=1n∑l=1(α2j+|αjγj|)θ+jilLQiMQle2λη+jilw2i(t)11−˙η+jil−12n∑i=1n∑j=1(α2iˉd+ij+|αiγi|ˉd+ij)LBjw2j(t−qij(t))−12n∑i=1n∑l=1n∑j=1(α2l+|αlγl|)θ+ljiMQjLQiw2i(t−ξlji(t))−12n∑i=1n∑j=1n∑l=1(α2j+|αjγj|)θ+jilLQiMQlw2i(t−ηjil(t))+n∑i=1n∑j=1(α2i|w′i(t)|+|αiγi||wi(t)|)|ˉcij(t)||˜Aj(wj(t))|+n∑i=1n∑j=1(α2i|w′i(t)|+|αiγi||wi(t)|)|ˉdij(t)||˜Bj(wj(t−qij(t)))|}+n∑i=1(α2i|w′i(t)|+|αiγi||wi(t)|)×n∑j=1n∑l=1|θijl(t)|(MQjLQl|wl(t−ξijl(t))|+LQj|wj(t−ηijl(t))|MQl)} |
=e2λt{n∑i=1(βi+γ2i+2λαiγi−ˉai(t)αiγi−ˉbi(t)α2i)wi(t)w′i(t)+n∑i=1(λα2i+αiγi−ˉai(t)α2i)(w′i(t))2+n∑i=1[−ˉbi(t)αiγi+λβi+λγ2i+12n∑j=1(α2jˉd+ji+|αjγj|ˉd+ji)11−˙q+jie2λq+jiLBi+12n∑l=1n∑j=1(α2l+|αlγl|)θ+ljiMQjLQie2λξ+lji11−˙ξ+lji+12n∑j=1n∑l=1(α2j+|αjγj|)θ+jilLQiMQle2λη+jil11−˙η+jil]w2i(t)−12n∑i=1n∑j=1(α2iˉd+ij+|αiγi|ˉd+ij)LBjw2j(t−qij(t))−12n∑i=1n∑l=1n∑j=1(α2l+|αlγl|)θ+ljiMQjLQiw2i(t−ξlji(t))−12n∑i=1n∑j=1n∑l=1(α2j+|αjγj|)θ+jilLQiMQlw2i(t−ηjil(t))+n∑i=1n∑j=1(α2i|w′i(t)|+|αiγi||wi(t)|)|ˉcij(t)||˜Aj(wj(t))|+n∑i=1n∑j=1(α2i|w′i(t)|+|αiγi||wi(t)|)|ˉdij(t)||˜Bj(wj(t−qij(t)))|}+n∑i=1(α2i|w′i(t)|+|αiγi||wi(t)|)n∑j=1n∑l=1|θijl(t)|×(MQjLQl|wl(t−ξijl(t))|+LQj|wj(t−ηijl(t))|MQl)}, ∀t∈[0,+∞). | (2.5) |
It follows from (F1) and PQ≤12(P2+Q2)(P,Q∈R) that
n∑i=1n∑j=1(α2i|w′i(t)|+|αiγi||wi(t)|)|ˉcij(t)||˜Aj(wj(t))|≤12n∑i=1n∑j=1α2i|ˉcij(t)|LAj((w′i(t))2+w2j(t))+12n∑i=1n∑j=1|αiγi||ˉcij(t)|LAj(w2i(t)+w2j(t))=12n∑i=1n∑j=1α2i|ˉcij(t)|LAj(w′i(t))2+12n∑i=1n∑j=1(|αiγi||ˉcij(t)|LAj+α2j|ˉcji(t)|LAi+|αjγj||ˉcji(t)|LAi)w2i(t), |
n∑i=1n∑j=1(α2i|w′i(t)|+|αiγi||wi(t)|)|ˉdij(t)||˜Bj(wj(t−qij(t)))|≤12n∑i=1n∑j=1α2i|ˉdij(t)|LBj((w′i(t))2+w2j(t−qij(t)))+12n∑i=1n∑j=1|αiγi||ˉdij(t)|LBj(w2i(t)+w2j(t−qij(t)))=12n∑i=1n∑j=1α2i|ˉdij(t)|LBj(w′i(t))2+12n∑i=1n∑j=1|αiγi||ˉdij(t)|LBjw2i(t)+12n∑i=1n∑j=1(α2i|ˉdij(t)|LBj+|αiγi||ˉdij(t)|LBj)w2j(t−qij(t)), |
and
n∑i=1(α2i|w′i(t)|+|αiγi||wi(t)|)×n∑j=1n∑l=1|θijl(t)|(MQjLQl|wl(t−ξijl(t))|+LQj|wj(t−ηijl(t))|MQl)≤12n∑i=1n∑j=1n∑l=1α2i|θijl(t)|MQjLQl((w′i(t))2+w2l(t−ξijl(t)))+12n∑i=1n∑j=1n∑l=1|αiγi||θijl(t)|MQjLQl((wi(t))2+w2l(t−ξijl(t)))+12n∑i=1n∑j=1n∑l=1α2i|θijl(t)|LQjMQl((w′i(t))2+w2j(t−ηijl(t)))+12n∑i=1n∑j=1n∑l=1|αiγi||θijl(t)|LQjMQl((wi(t))2+w2j(t−ηijl(t)))=12n∑i=1n∑j=1n∑l=1α2i|θijl(t)|(MQjLQl+LQjMQl)(w′i(t))2+12n∑i=1n∑j=1n∑l=1|αiγi||θijl(t)|(MQjLQl+LQjMQl)(wi(t))2+12n∑i=1n∑j=1n∑l=1(α2i+|αiγi|)|θijl(t)|MQjLQlw2l(t−ξijl(t))+12n∑i=1n∑j=1n∑l=1(α2i+|αiγi|)|θijl(t)|LQjMQlw2j(t−ηijl(t))=12n∑i=1n∑j=1n∑l=1α2i|θijl(t)|(MQjLQl+LQjMQl)(w′i(t))2+12n∑i=1n∑j=1n∑l=1|αiγi||θijl(t)|(MQjLQl+LQjMQl)(wi(t))2+12n∑l=1n∑j=1n∑i=1(α2l+|αlγl|)|θlji(t)|MQjLQiw2i(t−ξlji(t))+12n∑j=1n∑i=1n∑l=1(α2j+|αjγj|)|θjil(t)|LQiMQlw2i(t−ηjil(t)), |
which, together with (2.4) and (2.5), entails that
K′(t)≤e2λt{n∑i=1(βi+γ2i+2λαiγi−ˉai(t)αiγi−ˉbi(t)α2i)wi(t)w′i(t)+n∑i=1[λα2i+αiγi−ˉai(t)α2i+12α2in∑j=1(|ˉcij(t)|LAj+|ˉdij(t)|LBj)+12α2in∑j=1n∑l=1|θijl(t)|(MQjLQl+LQjMQl)](w′i(t))2+n∑i=1[−ˉbi(t)αiγi+λβi+λγ2i+12n∑j=1(|ˉcij(t)|LAj+|ˉdij(t)|LBj)|αiγi|+12n∑j=1α2j(|ˉcji(t)|LAi+ˉd+jiLBi11−˙q+jie2λq+ji)+12n∑j=1(|ˉcji(t)|LAi+ˉd+jiLBi11−˙q+jie2λq+ji)|αjγj|+12n∑j=1n∑l=1|αiγi||θijl(t)|(MQjLQl+LQjMQl)+12n∑l=1n∑j=1(α2l+|αlγl|)θ+ljiMQjLQie2λξ+lji11−˙ξ+lji+12n∑j=1n∑l=1(α2j+|αjγj|)θ+jilLQiMQle2λη+jil11−˙η+jil)]w2i(t)}=e2λt{n∑i=1(Eλi(t)(w′i(t))2+Gλi(t)w2i(t)+Hλi(t)wi(t)w′i(t))}=e2λt{n∑i=1Eλi(t)(w′i(t)+Hλi(t)2Eλi(t)wi(t))2+n∑i=1(Gλi(t)−(Hλi(t))24Eλi(t))w2i(t)}≤0, ∀t∈[0,+∞). |
This indicates that K(t)≤K(0) for all t∈[0,+∞), and
12n∑i=1βiw2i(t)e2λt+12n∑i=1(αiw′i(t)+γiwi(t))2e2λt≤K(0), t∈[0,+∞). |
Note that
(αiw′i(t)eλt+γiwi(t)eλt)2=(αiw′i(t)+γiwi(t))2e2λt |
and
αi|w′i(t)|eλt≤|αiw′i(t)eλt+γiwi(t)eλt|+|γiwi(t)eλt|, |
one can find a constant M>0 such that
|w′i(t)|≤Me−λt, |wi(t)|≤Me−λt, t≥0, i∈D, |
which proves Lemma 2.1.
Remark 2.2. Under the assumptions adopted in Lemma 2.1, if y(t) is an equilibrium point or a periodic solution of (1.1), one can see y(t) is globally exponentially stable. Moreover, the definition of global exponential stability can be also seen in [13,16].
Now, we set out the main result of this paper as follows.
Theorem 3.1. Under assumptions (F1)–(F3), system (1.1) possesses a global exponential stable T-anti-periodic solution.
Proof. Denote κ(t)=(κ1(t),κ2(t),⋯, κn(t)) be a solution of system (1.1) satisfying:
κi(s)=φκi(s), κ′i(s)=ψκi(s), −τi≤s≤0, φκi,ψκi∈C([−τi,0],R), i∈D. | (3.1) |
With the aid of (F1), one can see that
ˉai(t+T)=ˉai(t), ˉbi(t+T)=ˉbi(t), qij(t+T)=qij(t), |
ηijl(t+T)=ηijl(t), ξijl(t+T)=ξijl(t), Ji(t+T)=−Ji(t), |
(−1)m+1ˉcij(t+(m+1)T)Aj(κj(t+(m+1)T))=ˉcij(t)Aj((−1)m+1κj(t+(m+1)T)), |
(−1)m+1ˉdij(t+(m+1)T)Bj(κj(t+(m+1)T−qij(t)))=ˉdij(t)Bj((−1)m+1κj(t+(m+1)T−qij(t))), |
and
(−1)m+1θijl(t+(m+1)T)Qj(κj(t+(m+1)T−ηijl(t)))Ql(κl(t+(m+1)T−ξijl(t))) |
=θijl(t)Qj((−1)m+1κj(t+(m+1)T−ηijl(t)))Ql((−1)m+1κl(t+(m+1)T−ξijl(t))), |
where t∈R and i,j,l∈D.
Consequently, for any nonnegative integer m,
((−1)m+1κi(t+(m+1)T))″=−ˉai(t)((−1)m+1κi(t+(m+1)T))′−ˉbi(t)((−1)m+1κi(t+(m+1)T))+n∑j=1ˉcij(t)Aj((−1)m+1κj(t+(m+1)T))+n∑j=1ˉdij(t)Bj((−1)m+1κj(t+(m+1)T−qij(t)))+n∑j=1n∑l=1θijl(t)×Qj((−1)m+1κj(t+(m+1)T−ηijl(t)))×Ql((−1)m+1κl(t+(m+1)T−ξijl(t)))+Ji(t), for all i∈D,t+(m+1)T≥0. | (3.2) |
Clearly, (−1)m+1κ(t+(m+1)T) (t+(m+1)T≥0) satisfies (1.1), and v(t)=−κ(t+T) is a solution of system (1.1) involving initial values:
φvi(s)=−κi(s+T),ψvi(s)=−κ′i(s+T), for all s∈[−τi,0],i∈D. |
Thus, with the aid of Lemma 2.1, we can pick a constant M=M(φκ,ψκ,φv,ψv) satisfying
|κi(t)−vi(t)|≤Me−λt, |κ′i(t)−v′i(t)|≤Me−λt, for all t≥0,i∈D. |
Hence,
|(−1)pκi(t+pT)−(−1)p+1κi(t+(p+1)T)|=|κi(t+pT)−vi(t+pT)|≤Me−λ(t+pT),|((−1)pκi(t+pT))′−((−1)p+1κi(t+(p+1)T))′|=|κ′i(t+pT)−v′i(t+pT)|≤Me−λ(t+pT),} ∀i∈D, t+pT≥0. | (3.3) |
Consequently,
(−1)m+1κi(t+(m+1)T)=κi(t)+m∑p=0[(−1)p+1κi(t+(p+1)T)−(−1)pκi(t+pT)] (i∈D) |
and
((−1)m+1κi(t+(m+1)T))′=κ′i(t)+m∑p=0[((−1)p+1κi(t+(p+1)T))′−((−1)pκi(t+pT))′] (i∈D). |
Therefore, (3.3) suggests that there exists a continuous differentiable function y(t)=(y1(t),y2(t),⋯,yn(t)) such that {(−1)mκ(t+mT)}m≥1 and {((−1)mκ(t+mT))′}m≥1 are uniformly convergent to y(t) and y′(t) on any compact set of R, respectively.
Moreover,
y(t+T)=limm→+∞(−1)mκ(t+T+mT)=−lim(m+1)→+∞(−1)m+1κ(t+(m+1)T)=−y(t) |
involves that y(t) is T−anti-periodic on R. It follows from (F1)-(F3) and the continuity on (3.2) that {(κ″(t+(m+1)T)}m≥1 uniformly converges to a continuous function on any compact set of R. Furthermore, for any compact set of R, setting m⟶+∞, we obtain
y″i(t)=−ˉai(t)y′i(t)−ˉbi(t)yi(t)+n∑j=1ˉcij(t)Aj(yj(t))+n∑j=1ˉdij(t)Bj(yj(t−qij(t)))+n∑j=1n∑l=1θijl(t)Qj(yj(t−ηijl(t)))Ql(yl(t−ξijl(t)))+Ji(t),,i∈D, |
which involves that y(t) is a T−anti-periodic solution of (1.1). Again from Lemma 2.1, we gain that y(t) is globally exponentially stable. This finishes the proof of Theorem 3.1.
Remark 3.1. For inertial neural networks without high-order terms respectively, suppose
|ˉai−ˉbi|<2, Ai and Bi are bounded, i∈D, | (3.4) |
and
|ˉai−ˉbi+1|<1,i∈D, | (3.5) |
the authors gained the existence and stability on periodic solutions in [10,11] and anti-periodic solutions in [12]. Moreover, the reduced-order method was crucial in [10,11,12] when anti-periodicity and periodicity of second-order inertial neural networks were considered. However, (3.4) and (3.5) have been abandoned in Theorem 3.1 and the reduced-order method has been substituted in this paper. Therefore, our results on anti-periodicity of high-order inertial Hopfield neural networks are new and supplemental in nature.
Example 4.1. Let n=2, and consider a class of high-order inertial Hopfield neural networks in the form of
{x″1(t)=−14.92x′1(t)−27.89x1(t)+2.28(sint)A1(x1(t)) +2.19(cost)A2(x2(t)) −0.84(cos2t)B1(x1(t−0.2sin2t))+2.41(cos2t)B2(x2(t−0.3sin2t)) +4(sin2t)Q1(x1(t−0.4sin2t))Q2(x2(t−0.5sin2t))+55sint,x″2(t)=−15.11x′2(t)−31.05x2(t)−1.88(sint)A1(x1(t)) −2.33(cost)A2(x2(t)) −2.18(sin2t)B1(x1(t−0.2cos2t))+3.18(cos2t)B2(x2(t−0.3cos2t)) +3.8(sin2t)Q1(x1(t−0.4cos2t))Q2(x2(t−0.5cos2t))+48sint, | (4.1) |
where t≥0, A1(u)=A2(u)=135|u|, B1(u)=B2(u)=148u, Q1(u)=Q2(u)=155arctanu.
Using a direct calculation, one can check that (4.1) satisfies (2.4) and (F1)−(F3). Applying Theorem 3.1, it is obvious that system (4.1) has a globally exponentially stable π-anti-periodic solution. Simulations reflect that the theoretical anti-periodicity is in sympathy with the numerically observed behavior (Figures 1 and 2).
Example 4.2. Regard the following high-order inertial Hopfield neural networks involving time-varying delays and coefficients:
{x″1(t)=−(14+0.9|sint|)x′1(t)−(27+0.8|cost|)x1(t)+2.28(sint)A1(x1(t)) +2.19(cost)A2(x2(t)) −0.84(cos2t)B1(x1(t−0.2sin2t))+2.41(cos2t)B2(x2(t−0.3sin2t)) +4(sint)Q1(x1(t−0.4sin2t))Q2(x2(t−0.5sin2t))+100sint,x″2(t)=−(15+0.1|cost|)x′2(t)−(31+0.1|sint|)x2(t)−1.88(sint)A1(x1(t)) −2.33(cost)A2(x2(t)) −2.18(sin2t)B1(x1(t−0.2cos2t))+3.18(cos2t)B2(x2(t−0.3cos2t)) +3.8(sint)Q1(x1(t−0.4cos2t))Q2(x2(t−0.5cos2t))+200sint, | (4.2) |
where t≥0, A1(u)=A2(u)=135|u|, B1(u)=B2(u)=148u, Q1(u)=Q2(u)=1110(|x+1|−|x−1|). Then, by Theorem 3.1, one can find that all solutions of networks (4.2) are convergent to a π-anti-periodic solution (See Figures 3 and 4).
Remark 4.1. From the figures 1–4, one can see that the solution is similar to sinusoidal oscillation, and there exists a π-anti-periodic solution satisfying x(t+π)=−x(t). To the author's knowledge, the anti-periodicity on high-order inertial Hopfield neural networks involving time-varying delays has never been touched by using the non-reduced order method. Manifestly, the assumptions (3.4) and (3.5) adopted in [10,11] are invalid in systems (4.1) and (4.2). In addition, the most recently papers [10,11] only considered the polynomial power stability of some proportional time-delay systems, but not involved the exponential power stability of the addressed systems. And the results in [35,36,37,38,39,40,41,42,43,44,45,46,47,48,49,50,51,52,53,54,55,56,57,58,59,60,61,62,63,64,65,66,67,68,69,70,71,72,73,74, 75,76,77,78,79,80,81,82] have not touched on the anti-periodicity of inertial neural networks. This entails that the corresponding conclusions in [10,11,12,13,14,15,16,17,18,19,20,21,22,23,24,25,26,27,28,29,30,31,32,33,34,35,36,37,38,39,40,41,42,43,44,45,46,47,48, 49,50,51,52,53,54,55,56,57,58,59,60,61,62,63,64,65,66,67,68,69,70,71,72,73,74,75,76,77,78,79,80,81,82] and the references cited therein can not be applied to show the anti-periodic convergence for systems (4.1) and (4.2).
In this paper, abandoning the reduced order method, we apply inequality techniques and Lyapunov function method to establish the existence and global exponential stability of anti-periodic solutions for a class of high-order inertial Hopfield neural networks involving time-varying delays and anti-periodic environments. The obtained results are essentially new and complement some recently published results. The method proposed in this article furnishes a possible approach for studying anti-periodic on other types high-order inertial neural networks such as shunting inhibitory cellular neural networks, BAM neural networks, Cohen-Grossberg neural networks and so on.
The authors would like to express the sincere appreciation to the editor and reviewers for their helpful comments in improving the presentation and quality of the paper.
The authors confirm that they have no conflict of interest.
[1] |
Mathee K, Cickovski T, Deoraj A, et al. (2020) The gut microbiome and neuropsychiatric disorders: implications for attention deficit hyperactivity disorder (ADHD). J Med Microbiol 69: 14-24. doi: 10.1099/jmm.0.001112
![]() |
[2] |
Vigo D, Thornicroft G, Atun R (2016) Estimating the true global burden of mental illness. Lancet Psychiatry 3: 171-178. doi: 10.1016/S2215-0366(15)00505-2
![]() |
[3] |
Os J, Kenis G, Rutten BP (2010) The environment and schizophrenia. Nature 468: 203-212. doi: 10.1038/nature09563
![]() |
[4] |
Köhler-Forsberg O, Petersen L, Gasse C, et al. (2019) A nationwide study in denmark of the association between treated infections and the subsequent risk of treated mental disorders in children and adolescents. JAMA Psychiatry 76: 271-279. doi: 10.1001/jamapsychiatry.2018.3428
![]() |
[5] |
Goldsmith DR, Rapaport MH, Miller BJ (2016) A meta-analysis of blood cytokine network alterations in psychiatric patients: comparisons between schizophrenia, bipolar disorder and depression. Mol Psychiatry 21: 1696-1709. doi: 10.1038/mp.2016.3
![]() |
[6] |
Krefft M, Frydecka D, Zalsman G, et al. (2021) A pro-inflammatory phenotype is associated with behavioural traits in children with Prader-Willi syndrome. Eur Child Adolesc Psychiatry 30: 899-908. doi: 10.1007/s00787-020-01568-7
![]() |
[7] |
Shahbazi R, Yasavoli-Sharahi H, Alsadi N, et al. (2020) Matar, C. Probiotics in treatment of viral respiratory infections and neuroinflammatory disorders. Molecules 25: 4891. doi: 10.3390/molecules25214891
![]() |
[8] |
Barona M, Brown M, Clark C, et al. (2019) White matter alterations in anorexia nervosa: Evidence from a voxel-based meta-analysis. Neurosci Biobehav Rev 100: 285-295. doi: 10.1016/j.neubiorev.2019.03.002
![]() |
[9] |
Bastiaanssen TFS, Cryan JF (2021) The microbiota-gut-brain axis in mental health and medication response: parsing directionality and causality. Int J Neuropsychopharmacol 24: 216-220. doi: 10.1093/ijnp/pyaa088
![]() |
[10] |
Martins LB, Braga Tibães JR, Sanches M, et al. (2021) Nutrition-based interventions for mood disorders. Expert Rev Neurother 21: 303-315. doi: 10.1080/14737175.2021.1881482
![]() |
[11] |
Cerdó T, Diéguez E, Campoy C (2020) Impact of gut microbiota on neurogenesis and neurological diseases during infancy. Curr Opin Pharmacol 50: 33-37. doi: 10.1016/j.coph.2019.11.006
![]() |
[12] |
Gubert C, Kong G, Renoir T, et al. (2020) Exercise, diet and stress as modulators of gut microbiota: Implications for neurodegenerative diseases. Neurobiol Dis 134: 104621. doi: 10.1016/j.nbd.2019.104621
![]() |
[13] |
Berding K, Vlckova K, Marx W, et al. Diet and the microbiota-gut-brain axis: sowing the seeds of good mental health (2021) . doi: 10.1093/advances/nmaa181
![]() |
[14] |
Rea K, Dinan TG, Cryan JF (2020) Gut microbiota: a perspective for psychiatrists. Neuropsychobiology 79: 50-62. doi: 10.1159/000504495
![]() |
[15] |
Zinchenko E, Navolokin N, Shirokov A, et al. (2019) Pilot study of transcranial photobiomodulation of lymphatic clearance of beta-amyloid from the mouse brain: breakthrough strategies for non-pharmacologic therapy of Alzheimer's disease. Biomed Opt Express 10: 4003-4017. doi: 10.1364/BOE.10.004003
![]() |
[16] |
Mishra SP, Shukla SK, Pandey BL (2014) A preliminary evaluation of comparative effectiveness of riluzole in therapeutic regimen for irritable bowel syndrome. Asian Pac J Trop Biomed 4: S335-S340. doi: 10.12980/APJTB.4.2014C205
![]() |
[17] |
Barberio B, Zamani M, Black CJ, et al. (2021) Prevalence of symptoms of anxiety and depression in patients with inflammatory bowel disease: a systematic review and meta-analysis. Lancet Gastroenterol Hepatol 6: 359-370. doi: 10.1016/S2468-1253(21)00014-5
![]() |
[18] |
Dinan TG, Cryan JF (2017) Gut instincts: microbiota as a key regulator of brain development, ageing and neurodegeneration. J Physiol 595: 489-503. doi: 10.1113/JP273106
![]() |
[19] |
Wang J, Gu X, Yang J, et al. (2019) Gut microbiota dysbiosis and increased plasma LPS and TMAO levels in patients with preeclampsia. Front Cell Infect Microbiol 9: 409. doi: 10.3389/fcimb.2019.00409
![]() |
[20] |
Stasi C, Caserta A, Nisita C, et al. (2019) The complex interplay between gastrointestinal and psychiatric symptoms in irritable bowel syndrome: A longitudinal assessment. J Gastroenterol Hepatol 34: 713-719. doi: 10.1111/jgh.14375
![]() |
[21] |
Kelly JR, Clarke G, Cryan JF, et al. (2016) Brain-gut-microbiota axis: challenges for translation in psychiatry. Ann Epidemiol 26: 366-372. doi: 10.1016/j.annepidem.2016.02.008
![]() |
[22] |
Winter G, Hart RA, Charlesworth RPG, et al. (2018) Gut microbiome and depression: what we know and what we need to know. Rev Neurosci 29: 629-643. doi: 10.1515/revneuro-2017-0072
![]() |
[23] |
Zheng P, Zeng B, Zhou C, et al. (2016) Gut microbiome remodeling induces depressive-like behaviors through a pathway mediated by the host's metabolism. Mol Psychiatry 21: 786-796. doi: 10.1038/mp.2016.44
![]() |
[24] |
Yang C, Qu Y, Fujita Y, et al. (2017) Possible role of the gut microbiota-brain axis in the antidepressant effects of (R)-ketamine1 in a social defeat stress model. Transl Psychiatry 7: 1294. doi: 10.1038/s41398-017-0031-4
![]() |
[25] |
Lyte M, Daniels KM, Schmitz-Esser S (2019) Fluoxetine-induced alteration of murine gut microbial community structure: evidence for a microbial endocrinology-based mechanism of action responsible for fluoxetine-induced side effects. Peer J 7: e6199. doi: 10.7717/peerj.6199
![]() |
[26] |
Saini A, Ardine M, Berruti A (2008) Major depressive disorder. N Engl J Med 358: 1868-1869. doi: 10.1056/NEJMc080186
![]() |
[27] |
Arneth BM (2018) Gut-brain axis biochemical signalling from the gastrointestinal tract to the central nervous system: gut dysbiosis and altered brain function. Postgrad Med J 94: 446-452. doi: 10.1136/postgradmedj-2017-135424
![]() |
[28] |
Borre YE, O'Keeffe GW, Clarke G, et al. (2014) Microbiota and neurodevelopmental windows: implications for brain disorders. Trends Mol Med 20: 509-518. doi: 10.1016/j.molmed.2014.05.002
![]() |
[29] |
Barbara G, Stanghellini V, Brandi G, et al. (2005) Interactions between commensal bacteria and gut sensorimotor function in health and disease. Am J Gastroenterol 100: 2560-2568. doi: 10.1111/j.1572-0241.2005.00230.x
![]() |
[30] |
Strandwitz P (2018) Neurotransmitter modulation by the gut microbiota. Brain Res 1693: 128-133. doi: 10.1016/j.brainres.2018.03.015
![]() |
[31] |
Sivamaruthi BS, Suganthy N, Kesika P, et al. (2020) The role of microbiome, dietary supplements, and probiotics in autism spectrum disorder. Int J Environ Res Public Health 17: 2647. doi: 10.3390/ijerph17082647
![]() |
[32] |
Song Q, Wang Y, Huang L, et al. (2021) Review of the relationships among polysaccharides, gut microbiota, and human health. Food Res Int 140: 109858. doi: 10.1016/j.foodres.2020.109858
![]() |
[33] |
Salami M (2021) Interplay of good bacteria and central nervous system: cognitive aspects and mechanistic considerations. Front Neurosci 15: 613120. doi: 10.3389/fnins.2021.613120
![]() |
[34] |
Niederseer D, Wernly B, Aigner E, et al. (2021) NAFLD and cardiovascular diseases: epidemiological, mechanistic and therapeutic considerations. J Clin Med 10: 467. doi: 10.3390/jcm10030467
![]() |
[35] |
Burgueño JF, Fritsch J, Santander AM, et al. (2019) Intestinal epithelial cells respond to chronic inflammation and dysbiosis by synthesizing H2O2. Front Physiol 10: 1484. doi: 10.3389/fphys.2019.01484
![]() |
[36] |
Belizário JE, Faintuch J, Garay-Malpartida M (2018) Gut microbiome dysbiosis and immunometabolism: New frontiers for treatment of metabolic diseases. Mediators Inflamm 2018: 2037838. doi: 10.1155/2018/2037838
![]() |
[37] | Andreyev AY, Kushnareva YE, Starkova NN, et al. (2020) Metabolic ROS signaling: to immunity and beyond. Biochemistry 85: 1650-1667. |
[38] | Saint-Georges-Chaumet Y, Attaf D, Pelletier E, et al. (2015) Targeting microbiota-mitochondria inter-talk: Microbiota control mitochondria metabolism. Cell Mol Biol 61: 121-124. |
[39] |
Li M, Gu MM, Lang Y, et al. (2019) The vanillin derivative VND3207 protects intestine against radiation injury by modulating p53/NOXA signaling pathway and restoring the balance of gut microbiota. Free Radi Biol Med 145: 223-236. doi: 10.1016/j.freeradbiomed.2019.09.035
![]() |
[40] |
Wang W, Im J, Kim S, et al. (2020) ROS-induced SIRT2 upregulation contributes to cisplatin sensitivity in ovarian cancer. Antioxidants 9: 1137. doi: 10.3390/antiox9111137
![]() |
[41] |
Muralitharan RR, Marques FZ (2021) Diet-related gut microbial metabolites and sensing in hypertension. J Hum Hypertens 35: 162-169. doi: 10.1038/s41371-020-0388-3
![]() |
[42] |
Zhou Y, Jiang Q, Zhao S, et al. (2019) Impact of buckwheat fermented milk combined with high-fat diet on rats' gut microbiota and short-chain fatty acids. J Food Sci 84: 3833-3842. doi: 10.1111/1750-3841.14958
![]() |
[43] |
Skonieczna-Żydecka K, Grochans E, Maciejewska D, et al. (2018) Short chain fatty acids profile is changed in Polish depressive women. Nutrients 10: 1939. doi: 10.3390/nu10121939
![]() |
[44] |
Hu L, Zhu S, Peng X, et al. (2020) High salt elicits brain inflammation and cognitive dysfunction, accompanied by alternations in the gut microbiota and decreased SCFA production. J Alzheimers Dis 77: 629-640. doi: 10.3233/JAD-200035
![]() |
[45] |
Luceri C, Femia AP, Fazi M, et al. (2016) Effect of butyrate enemas on gene expression profiles and endoscopic/histopathological scores of diverted colorectal mucosa: A randomized trial. Dig Liver Dis 48: 27-33. doi: 10.1016/j.dld.2015.09.005
![]() |
[46] | Häselbarth L, Ouwens DM, Teichweyde N, et al. (2016) The small chain fatty acid butyrate antagonizes the TCR-stimulation-induced metabolic shift in murine epidermal gamma delta T cells. EXCLI J 19: 334-350. |
[47] |
Haase S, Haghikia A, Wilck N, et al. (2018) Impacts of microbiome metabolites on immune regulation and autoimmunity. Immunology 154: 230-238. doi: 10.1111/imm.12933
![]() |
[48] |
Macfarlane GT, Macfarlane S (2012) Bacteria, colonic fermentation, and gastrointestinal health. J AOAC Int 95: 50-60. doi: 10.5740/jaoacint.SGE_Macfarlane
![]() |
[49] |
Ray S, Das S, Panda PK, et al. (2018) Identification of a new alanine racemase in Salmonella Enteritidis and its contribution to pathogenesis. Gut Pathog 10: 30. doi: 10.1186/s13099-018-0257-6
![]() |
[50] |
Gilmore MS, Skaugen M, Nes I (1996) Enterococcus faecalis cytolysin and lactocin S of Lactobacillus sake. Antonie Van Leeuwenhoek 69: 129-138. doi: 10.1007/BF00399418
![]() |
[51] |
Pidgeon SE, Fura JM, Leon W, et al. (2015) Metabolic profiling of bacteria by unnatural C-terminated d-Amino Acids. Angew Chem 127: 6256-6260. doi: 10.1002/ange.201409927
![]() |
[52] |
Sasabe J, Miyoshi Y, Rakoff-Nahoum S, et al. (2016) Interplay between microbial d-amino acids and host d-amino acid oxidase modifies murine mucosal defence and gut microbiota. Nat Microbiol 1: 16125. doi: 10.1038/nmicrobiol.2016.125
![]() |
[53] |
Nagano T, Yamao S, Terachi A, et al. (2019) d-amino acid oxidase promotes cellular senescence via the production of reactive oxygen species. Life Sci Alliance 2: e201800045. doi: 10.26508/lsa.201800045
![]() |
[54] |
Zhong C, Zhu N, Zhu Y, et al. (2020) Antimicrobial peptides conjugated with fatty acids on the side chain of D-amino acid promises antimicrobial potency against multidrug-resistant bacteria. Eur J Pharm Sci 141: 105123. doi: 10.1016/j.ejps.2019.105123
![]() |
[55] |
Arai T, Ashraful Hoque M, Nishino N, et al. (2013) Cyclic tetrapeptides with -SS- bridging between amino acid side chains for potent histone deacetylases' inhibition. Amino Acids 45: 835-843. doi: 10.1007/s00726-013-1527-8
![]() |
[56] |
Hergueta T, Weiller E (2013) Evaluating depressive symptoms in hypomanic and manic episodes using a structured diagnostic tool: validation of a new Mini International Neuropsychiatric Interview (M.I.N.I.) module for the DSM-5 ‘With Mixed Features’ specifier. Int J Bipolar Disord 1: 21. doi: 10.1186/2194-7511-1-21
![]() |
[57] |
Ryu JC, Zimmer ER, Rosa-Neto P, et al. (2019) Consequences of metabolic disruption in Alzheimer's disease pathology. Neurotherapeutics 16: 600-610. doi: 10.1007/s13311-019-00755-y
![]() |
[58] |
Laversenne V, Nazeeruddin S, Källstig EC, et al. (2020) Anti-Aβ antibodies bound to neuritic plaques enhance microglia activity and mitigate tau pathology. Acta Neuropathol Commun 8: 198. doi: 10.1186/s40478-020-01069-3
![]() |
[59] |
Guo LX, Tong Y, Wang J, et al. (2020) Determination and comparison of short-chain fatty acids in serum and colon content samples: Alzheimer's disease rat as a case study. Molecules 25: 5739. doi: 10.3390/molecules25235739
![]() |
[60] |
Liu H, Zhang JJ, Li X, et al. (2015) Post-occlusion administration of sodium butyrate attenuates cognitive impairment in a rat model of chronic cerebral hypoperfusion. Pharmacol Biochem Behav 135: 53-59. doi: 10.1016/j.pbb.2015.05.012
![]() |
[61] |
Infante R, Scaglione C, Incensi A, et al. (2020) Longitudinal skin biopsy study of phosphorylated alpha-synuclein in a patient with Parkinson disease and orthostatic hypotension. J Neuropathol Exp Neurol 79: 813-816. doi: 10.1093/jnen/nlaa048
![]() |
[62] |
Mannal N, Kleiner K, Fauler M, et al. (2021) Multi-electrode array analysis identifies complex dopamine responses and glucose sensing properties of substantia nigra neurons in mouse brain slices. Front Synaptic Neurosci 13: 635050. doi: 10.3389/fnsyn.2021.635050
![]() |
[63] |
Unger MM, Spiegel J, Dillmann KU, et al. (2016) Short chain fatty acids and gut microbiota differ between patients with Parkinson's disease and age-matched controls. Parkinsonism Relat Disord 32: 66-72. doi: 10.1016/j.parkreldis.2016.08.019
![]() |
[64] |
Ostendorf F, Metzdorf J, Gold R, et al. (2020) Propionic acid and fasudil as treatment against rotenone toxicity in an in vitro model of Parkinson's disease. Molecules 25: 2502. doi: 10.3390/molecules25112502
![]() |
[65] |
Ostendorf F, Metzdorf J, Gold R, et al. (2020) Differential effects of Δ9-tetrahydrocannabinol dosing on correlates of schizophrenia in the sub-chronic PCP rat model. PLoS One 15: e0230238. doi: 10.1371/journal.pone.0230238
![]() |
[66] |
Gubert C, Kong G, Uzungil V, et al. (2020) Microbiome profiling reveals gut dysbiosis in the metabotropic glutamate receptor 5 knockout mouse model of schizophrenia. Front Cell Dev Biol 8: 582320. doi: 10.3389/fcell.2020.582320
![]() |
[67] |
Hu M, Zheng P, Xie Y, et al. (2018) Propionate protects haloperidol-induced neurite lesions mediated by neuropeptide Y. Front Neurosci 12: 743. doi: 10.3389/fnins.2018.00743
![]() |
[68] |
Girardi Paskulin LM, Kottwitz Bierhals CCB, Oliveira Dos Santos N, et al. (2017) Depressive symptoms of the elderly people and caregiver's burden in home care. Invest Educ Enferm 35: 210-220. doi: 10.17533/udea.iee.v35n2a10
![]() |
[69] |
Wu M, Tian T, Mao Q, et al. (2020) Associations between disordered gut microbiota and changes of neurotransmitters and short-chain fatty acids in depressed mice. Transl Psychiatry 10: 350. doi: 10.1038/s41398-020-01038-3
![]() |
[70] |
Mendonça IP, Duarte-Silva E, Chaves-Filho AJM, et al. (2020) Neurobiological findings underlying depressive behavior in Parkinson's disease: A review. Int Immunopharmacol 83: 106434. doi: 10.1016/j.intimp.2020.106434
![]() |
[71] |
Maigoro AY, Lee S (2021) Gut microbiome-based analysis of lipid a biosynthesis in individuals with autism spectrum disorder: an in ailico valuation. Nutrients 13: 688. doi: 10.3390/nu13020688
![]() |
[72] |
Rose DR, Yang H, Serena G, et al. (2018) Differential immune responses and microbiota profiles in children with autism spectrum disorders and co-morbid gastrointestinal symptoms. Brain Behav Immun 70: 354-368. doi: 10.1016/j.bbi.2018.03.025
![]() |
[73] |
Huo W, Qi P, Cui L, et al. (2020) Polysaccharide from wild morels alters the spatial structure of gut microbiota and the production of short-chain fatty acids in mice. Biosci Microbiota Food Health 39: 219-226. doi: 10.12938/bmfh.2020-018
![]() |
[74] |
Dickerson FB, Stallings C, Origoni A, et al. Effect of probiotic supplementation on schizophrenia symptoms and association with gastrointestinal functioning: a randomized, placebo-controlled trial (2014) . doi: 10.4088/PCC.13m01579
![]() |
[75] |
Varela RB, Valvassori SS, Lopes-Borges J, et al. (2015) Sodium butyrate and mood stabilizers block ouabain-induced hyperlocomotion and increase BDNF, NGF and GDNF levels in brain of Wistar rats. J Psychiatr Res 61: 114-121. doi: 10.1016/j.jpsychires.2014.11.003
![]() |
[76] |
Yamawaki Y, Yoshioka N, Nozaki K, et al. (2018) Sodium butyrate abolishes lipopolysaccharide-induced depression-like behaviors and hippocampal microglial activation in mice. Brain Res 1680: 13-38. doi: 10.1016/j.brainres.2017.12.004
![]() |
[77] |
Lee J, Venna VR, Durgan DJ, et al. (2020) Young versus aged microbiota transplants to germ-free mice: increased short-chain fatty acids and improved cognitive performance. Gut Microbes 12: 1814107. doi: 10.1080/19490976.2020.1814107
![]() |
[78] |
D'Mello SR (2019) Regulation of central nervous system development by class I histone deacetylases. Dev Neurosci 41: 149-165. doi: 10.1159/000505535
![]() |
[79] |
Sinn DI, Kim SJ, Chu K, et al. (2007) Valproic acid-mediated neuroprotection in intracerebral hemorrhage via histone deacetylase inhibition and transcriptional activation. Neurobiol Dis 26: 464-472. doi: 10.1016/j.nbd.2007.02.006
![]() |
[80] |
Nestler EJ, Peña CJ, Kundakovic M, et al. (2016) Epigenetic basis of mental illness. Neuroscientist 22: 447-463. doi: 10.1177/1073858415608147
![]() |
[81] |
Kwon B, Houpt TA (2010) Phospho-acetylation of histone H3 in the amygdala after acute lithium chloride. Brain Res 1333: 36-47. doi: 10.1016/j.brainres.2010.03.068
![]() |
[82] |
Xiang B, Liu K, Yu M, et al. (2018) Systematic genetic analyses of genome-wide association study data reveal an association between the key nucleosome remodeling and deacetylase complex and bipolar disorder development. Bipolar Disord 20: 370-380. doi: 10.1111/bdi.12580
![]() |
[83] |
Arent CO, Valvassori SS, Fries GR, et al. (2011) Neuroanatomical profile of antimaniac effects of histone deacetylases inhibitors. Mol Neurobiol 43: 207-214. doi: 10.1007/s12035-011-8178-0
![]() |
[84] |
Guidotti A, Auta J, Chen Y, et al. (2011) Epigenetic GABAergic targets in schizophrenia and bipolar disorder. Neuropharmacology 60: 1007-1016. doi: 10.1016/j.neuropharm.2010.10.021
![]() |
[85] |
Kramer JM (2013) Epigenetic regulation of memory: implications in human cognitive disorders. Biomol Concepts 4: 1-12. doi: 10.1515/bmc-2012-0026
![]() |
[86] |
Martínez-Pacheco H, Picazo O, López-Torres A, et al. (2020) Biochemical and Behavioral Characterization of IN14, a New Inhibitor of HDACs with Antidepressant-Like Properties. Biomolecules 10: 299. doi: 10.3390/biom10020299
![]() |
[87] |
Cuadrado-Tejedor M, Pérez-González M, García-Muñoz C, et al. (2019) Taking advantage of the selectivity of histone deacetylases and phosphodiesterase inhibitors to design better therapeutic strategies to treat Alzheimer's disease. Front Aging Neurosci 11: 149. doi: 10.3389/fnagi.2019.00149
![]() |
[88] |
Mazzocchi M, Collins LM, Sullivan AM, et al. (2020) The class II histone deacetylases as therapeutic targets for Parkinson's disease. Neuronal Signal 4: NS20200001. doi: 10.1042/NS20200001
![]() |
[89] |
Marsh JL, Lukacsovich T, Thompson LM (2009) Animal models of polyglutamine diseases and therapeutic approaches. J Biol Chem 284: 7431-7435. doi: 10.1074/jbc.R800065200
![]() |
[90] |
Frost G, Sleeth ML, Sahuri-Arisoylu M, et al. (2014) The short-chain fatty acid acetate reduces appetite via a central homeostatic mechanism. Nat Commun 5: 3611. doi: 10.1038/ncomms4611
![]() |
[91] |
Braniste V, Al-Asmakh M, Kowal C, et al. (2014) The gut microbiota influences blood-brain barrier permeability in mice. Sci Transl Med 6: 263ra158. doi: 10.1126/scitranslmed.3009759
![]() |
[92] |
Zhou Z, Xu N, Matei N, et al. (2021) Sodium butyrate attenuated neuronal apoptosis via GPR41/Gbetagamma/PI3K/Akt pathway after MCAO in rats. J Cereb Blood Flow Metab 41: 267-281. doi: 10.1177/0271678X20910533
![]() |
[93] |
Simonini MV, Camargo LM, Dong E, et al. (2006) The benzamide MS-275 is a potent, long-lasting brain region-selective inhibitor of histone deacetylases. Proc Natl Acad Sci USA 103: 1587-1592. doi: 10.1073/pnas.0510341103
![]() |
[94] |
Tseng CJ, Gilbert TM, Catanese MC, et al. (2020) In vivo human brain expression of histone deacetylases in bipolar disorder. Transl Psychiatry 10: 224. doi: 10.1038/s41398-020-00911-5
![]() |
[95] |
Kennedy H, Montreuil TC (2021) The late positive potential as a reliable neural marker of cognitive reappraisal in children and youth: a brief review of the research literature. Front Psychol 11: 608522. doi: 10.3389/fpsyg.2020.608522
![]() |
[96] |
Gilbert TM, Zürcher NR, Wu CJ, et al. (2019) PET neuroimaging reveals histone deacetylase dysregulation in schizophrenia. J Clin Invest 129: 364-372. doi: 10.1172/JCI123743
![]() |
[97] |
Wang C, Schroeder FA, Hooker JM (2014) Visualizing epigenetics: current advances and advantages in HDAC PET imaging techniques. Neuroscience 264: 186-197. doi: 10.1016/j.neuroscience.2013.09.018
![]() |
[98] |
Seo YJ, Muench L, Reid A, et al. (2013) Radionuclide labeling and evaluation of candidate radioligands for PET imaging of histone deacetylase in the brain. Bioorg Med Chem Lett 23: 6700-6705. doi: 10.1016/j.bmcl.2013.10.038
![]() |
[99] |
Gilbert TM, Zürcher NR, Catanese MC, et al. (2019) Neuroepigenetic signatures of age and sex in the living human brain. Nat Commun 10: 2945. doi: 10.1038/s41467-019-11031-0
![]() |
[100] |
Omori K, Miyakawa H, Watanabe A, et al. (2021) The combined effects of magnesium oxide and inulin on intestinal microbiota and cecal short-chain fatty acids. Nutrients 13: 152. doi: 10.3390/nu13010152
![]() |
[101] |
Minami NS, Sousa RS, Oliveira FLC, et al. (2020) Subacute ruminal acidosis in zebu cattle: clinical and behavioral aspects. Animals 11: 21. doi: 10.3390/ani11010021
![]() |
[102] |
Takewaki D, Suda W, Sato W, et al. (2020) Alterations of the gut ecological and functional microenvironment in different stages of multiple sclerosis. Proc Natl Acad Sci USA 117: 22402-22412. doi: 10.1073/pnas.2011703117
![]() |
[103] |
Borgo F, Riva A, Benetti A, et al. (2017) Microbiota in anorexia nervosa: The triangle between bacterial species, metabolites and psychological tests. PLoS One 12: e0179739. doi: 10.1371/journal.pone.0179739
![]() |
[104] |
Geirnaert A, Calatayud M, Grootaert C, et al. (2017) Butyrate-producing bacteria supplemented in vitro to Crohn's disease patient microbiota increased butyrate production and enhanced intestinal epithelial barrier integrity. Sci Rep 7: 11450. doi: 10.1038/s41598-017-11734-8
![]() |
[105] | Kilinçarslan S, Evrensel A (2020) The effect of fecal microbiota transplantation on psychiatric symptoms among patients with inflammatory bowel disease: an experimental study. Actas Esp Psiquiatr 48: 1-7. |
[106] | Tjellström B, Högberg L, Stenhammar L, et al. (2013) Faecal short-chain fatty acid pattern in childhood coeliac disease is normalised after more than one year's gluten-free diet. Microb Ecol Health Dis 24: 20905. |
[107] | Lerner A, Freire de Carvalho J, Kotrova A, et al. (2021) Gluten-free diet can ameliorate the symptoms of non-celiac autoimmune diseases . |
[108] |
Mohan M, Okeoma CM, Sestak K (2020) Dietary gluten and neurodegeneration: a case for preclinical studies. Int J Mol Sci 21: 5407. doi: 10.3390/ijms21155407
![]() |
[109] |
Bruce-Keller AJ, Salbaum JM, Luo M, et al. (2015) Obese-type gut microbiota induce neurobehavioral changes in the absence of obesity. Biol Psychiatry 77: 607-615. doi: 10.1016/j.biopsych.2014.07.012
![]() |
[110] |
Simeonova D, Ivanovska M, Murdjeva M, et al. (2018) Recognizing the leaky gut as a trans-diagnostic target for neuroimmune disorders using clinical chemistry and molecular immunology assays. Curr Top Med Chem 18: 1641-1655. doi: 10.2174/1568026618666181115100610
![]() |
1. | Xin Long, Novel stability criteria on a patch structure Nicholson’s blowflies model with multiple pairs of time-varying delays, 2020, 5, 2473-6988, 7387, 10.3934/math.2020473 | |
2. | Luogen Yao, Qian Cao, Anti-periodicity on high-order inertial Hopfield neural networks involving mixed delays, 2020, 2020, 1029-242X, 10.1186/s13660-020-02444-3 | |
3. | Qian Cao, Xin Long, New convergence on inertial neural networks with time-varying delays and continuously distributed delays, 2020, 5, 2473-6988, 5955, 10.3934/math.2020381 | |
4. | Ramazan Yazgan, Salsabil Hajjaji, Farouk Chérif, Weighted Pseudo Almost-Automorphic Solutions of Quaternion-Valued RNNs With Mixed Delays, 2023, 55, 1370-4621, 423, 10.1007/s11063-022-10890-x | |
5. | Yanli Xu, Periodic dynamic on a class of neutral-type Rayleigh equations incorporating D operators, 2023, 0952-813X, 1, 10.1080/0952813X.2023.2165720 | |
6. | M. Sai Pravallika, S. Vasavi, S. P. Vighneshwar, Prediction of temperature anomaly in Indian Ocean based on autoregressive long short-term memory neural network, 2022, 34, 0941-0643, 7537, 10.1007/s00521-021-06878-8 | |
7. | Weiping Fan, Anti-periodic stability on a class of neutral-type Rayleigh equations involving D operators, 2022, 0952-813X, 1, 10.1080/0952813X.2022.2153275 | |
8. | Xiaojin Guo, Chuangxia Huang, Zhichun Yang, Jiping Zhang, Jinde Cao, Stability Analysis of High-order Proportional Delayed Cellular Neural Networks with D Operators, 2022, 20, 1598-6446, 660, 10.1007/s12555-020-0902-y | |
9. | Zhenjie Wang, Wenxia Cui, Wenbin Jin, Finite-time synchronization for fuzzy inertial cellular neural networks with time-varying delays via integral inequality, 2021, 41, 10641246, 3653, 10.3233/JIFS-211065 | |
10. | Jian Zhang, Ancheng Chang, Gang Yang, Periodicity on Neutral-Type Inertial Neural Networks Incorporating Multiple Delays, 2021, 13, 2073-8994, 2231, 10.3390/sym13112231 | |
11. | Chaouki Aouiti, Mahjouba Ben Rezeg, Impulsive multidirectional associative memory neural networks: New results, 2021, 14, 1793-5245, 10.1142/S1793524521500601 | |
12. | Xiaojin Guo, Chuangxia Huang, Jinde Cao, Nonnegative periodicity on high-order proportional delayed cellular neural networks involving $ D $ operator, 2020, 6, 2473-6988, 2228, 10.3934/math.2021135 | |
13. | Ankit Kumar, Subir Das, Vijay K. Yadav, Jinde Cao, Chuangxia Huang, Synchronizations of fuzzy cellular neural networks with proportional time-delay, 2021, 6, 2473-6988, 10620, 10.3934/math.2021617 | |
14. | Weiping Fan, Periodic Stability on a Class of D-Operator-Based Neutral-Type Rayleigh Equations Accompanying Mixed Delays, 2022, 1370-4621, 10.1007/s11063-022-11033-y | |
15. | Bing Hao, Tianwei Zhang, Stability analysis and synchronized control of fuzzy Mittag-Leffler discrete-time genetic regulatory networks with time delays, 2022, 43, 10641246, 587, 10.3233/JIFS-212361 | |
16. | Yuehong Zhang, Zhiying Li, Wangdong Jiang, Wei Liu, The stability of anti-periodic solutions for fractional-order inertial BAM neural networks with time-delays, 2023, 8, 2473-6988, 6176, 10.3934/math.2023312 | |
17. | Jin Gao, Lihua Dai, Anti-periodic synchronization of quaternion-valued high-order Hopfield neural networks with delays, 2022, 7, 2473-6988, 14051, 10.3934/math.2022775 | |
18. | Chengjie Chen, Fuhong Min, Yunzhen Zhang, Han Bao, ReLU-type Hopfield neural network with analog hardware implementation, 2023, 167, 09600779, 113068, 10.1016/j.chaos.2022.113068 | |
19. | Lilun Zhang, Le Li, Chuangxia Huang, Positive stability analysis of pseudo almost periodic solutions for HDCNNs accompanying $ D $ operator, 2022, 15, 1937-1632, 1651, 10.3934/dcdss.2021160 | |
20. | Xianhui Zhang, Le Li, Changchun Bao, Jian Zhang, Anti-periodicity on inertial Cohen-Grossberg neural networks involving distributed delays, 2023, 0952-813X, 1, 10.1080/0952813X.2023.2196976 | |
21. | Mei Xu, Honghui Yin, Bo Du, Periodic Solution Problems for a Class of Hebbian-Type Networks with Time-Varying Delays, 2023, 15, 2073-8994, 1985, 10.3390/sym15111985 | |
22. | Chenyang Li, Kit Ian Kou, Yanlin Zhang, Yang Liu, Advancements in exponential synchronization and encryption techniques: Quaternion-Valued Artificial Neural Networks with two-sided coefficients, 2024, 08936080, 106982, 10.1016/j.neunet.2024.106982 |