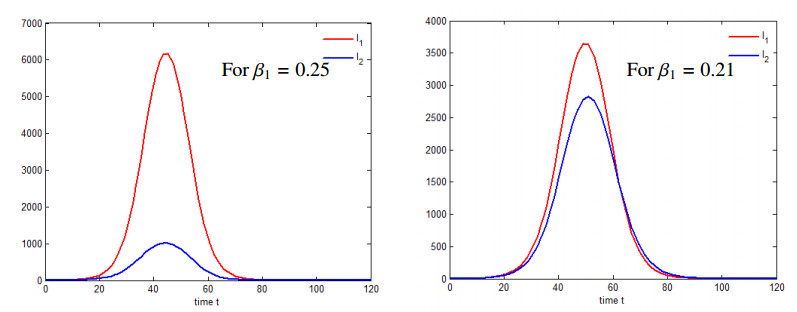
Listeria monocytogenes poses a significant threat to food safety worldwide, including in African countries. This bacterium is capable of causing severe infections, particularly in vulnerable populations. In this review, we provide an overview of the prevalence, transmission, and control measures of L. monocytogenes in the food chain across North Africa. Various factors contribute to the persistence and spread of this pathogen in food production and distribution systems, including environmental contamination, improper handling, and inadequate hygiene practices. Understanding the dynamics of L. monocytogenes in the North African food chain is crucial for implementing effective control strategies to mitigate the risk of contamination and protect public health. This review highlights the rise of virulence genes in L. monocytogenes from food production, especially milk production, over the past five years and their subsequent identification in human L. monocytogenes isolates from listeriosis cases. This underscores the persistent challenges that L. monocytogenes may pose to food safety and public health in North African countries.
Citation: Mohamed-Yousif Ibrahim Mohamed, Ihab Habib. Listeria monocytogenes in food products, and its virulence in North Africa[J]. AIMS Agriculture and Food, 2025, 10(1): 97-128. doi: 10.3934/agrfood.2025006
[1] | Masoud Saade, Samiran Ghosh, Malay Banerjee, Vitaly Volpert . An epidemic model with time delays determined by the infectivity and disease durations. Mathematical Biosciences and Engineering, 2023, 20(7): 12864-12888. doi: 10.3934/mbe.2023574 |
[2] | Sarita Bugalia, Jai Prakash Tripathi, Hao Wang . Mathematical modeling of intervention and low medical resource availability with delays: Applications to COVID-19 outbreaks in Spain and Italy. Mathematical Biosciences and Engineering, 2021, 18(5): 5865-5920. doi: 10.3934/mbe.2021295 |
[3] | Mostafa Adimy, Abdennasser Chekroun, Claudia Pio Ferreira . Global dynamics of a differential-difference system: a case of Kermack-McKendrick SIR model with age-structured protection phase. Mathematical Biosciences and Engineering, 2020, 17(2): 1329-1354. doi: 10.3934/mbe.2020067 |
[4] | Dongmei Li, Bing Chai, Weihua Liu, Panpan Wen, Ruixue Zhang . Qualitative analysis of a class of SISM epidemic model influenced by media publicity. Mathematical Biosciences and Engineering, 2020, 17(5): 5727-5751. doi: 10.3934/mbe.2020308 |
[5] | Lin Zhao, Zhi-Cheng Wang, Liang Zhang . Threshold dynamics of a time periodic and two–group epidemic model with distributed delay. Mathematical Biosciences and Engineering, 2017, 14(5&6): 1535-1563. doi: 10.3934/mbe.2017080 |
[6] | J. Amador, D. Armesto, A. Gómez-Corral . Extreme values in SIR epidemic models with two strains and cross-immunity. Mathematical Biosciences and Engineering, 2019, 16(4): 1992-2022. doi: 10.3934/mbe.2019098 |
[7] | Edoardo Beretta, Dimitri Breda . An SEIR epidemic model with constant latency time and infectious period. Mathematical Biosciences and Engineering, 2011, 8(4): 931-952. doi: 10.3934/mbe.2011.8.931 |
[8] | Sarafa A. Iyaniwura, Rabiu Musa, Jude D. Kong . A generalized distributed delay model of COVID-19: An endemic model with immunity waning. Mathematical Biosciences and Engineering, 2023, 20(3): 5379-5412. doi: 10.3934/mbe.2023249 |
[9] | Majid Jaberi-Douraki, Seyed M. Moghadas . Optimal control of vaccination dynamics during an influenza epidemic. Mathematical Biosciences and Engineering, 2014, 11(5): 1045-1063. doi: 10.3934/mbe.2014.11.1045 |
[10] | Kenji Itao, Fumiya Omata, Yoshitaka Nishikawa, Tetsuro Oda, Toshiharu Sasaki, Cherri Zhang, John Solomon Maninang, Takayuki Yamaguchi . Threshold phenomena with respect to the initiation of depopulation in a simple model of foot-and-mouth disease. Mathematical Biosciences and Engineering, 2019, 16(5): 5931-5946. doi: 10.3934/mbe.2019297 |
Listeria monocytogenes poses a significant threat to food safety worldwide, including in African countries. This bacterium is capable of causing severe infections, particularly in vulnerable populations. In this review, we provide an overview of the prevalence, transmission, and control measures of L. monocytogenes in the food chain across North Africa. Various factors contribute to the persistence and spread of this pathogen in food production and distribution systems, including environmental contamination, improper handling, and inadequate hygiene practices. Understanding the dynamics of L. monocytogenes in the North African food chain is crucial for implementing effective control strategies to mitigate the risk of contamination and protect public health. This review highlights the rise of virulence genes in L. monocytogenes from food production, especially milk production, over the past five years and their subsequent identification in human L. monocytogenes isolates from listeriosis cases. This underscores the persistent challenges that L. monocytogenes may pose to food safety and public health in North African countries.
Mathematical modeling plays a crucial role in comprehending the transmission dynamics of infectious diseases, predicting the future trajectory of outbreaks, and assessing strategies for epidemic control [1]. Its significance has been underscored by successive epidemics of viral infections, such as HIV since the 1980s [2,3], the SARS epidemic in 2002–2003 [4,5], H5N1 influenza in 2005 [6,7], H1N1 in 2009 [8,9], Ebola in 2014 [10,11], and the recent COVID-19 pandemic [12,13].
Following the classical SIR (susceptible-infected-recovered) model developed by Kermack and McKendrick after the Spanish flu epidemic [14], several notable advancements have been made in epidemiological modeling. These advancements include the development of multi-compartment models [15,16,17,18,19], models incorporating either time-varying or non-linear disease transmission rates [20,21], multi-patch models [22,23], and multi-group models that consider the effect of disease and population heterogeneity [24]. Additionally, there are epidemic models that incorporate vaccinations and other control measures [25,26,27,28,29,30]. Moreover, spatio-temporal models have been employed to describe the random movement of individuals and the spatial distribution of infections [31,32]. In [33], the authors investigated a model that integrated asymptomatic and dead-infective subpopulations, along with vaccination, antiviral treatment controls, and impulsive culling actions. Reference [34] is devoted to multiple pathogen interactions in disease transmission with a susceptible-infected-susceptible (SIS) model for each pathogen. For a comprehensive review of the literature, monographs [35,36] and review articles in [37,38] are available.
Time delays related to infection latency, disease duration and immunity waning directly influence disease transmission dynamics, response strategies, and the overall control of infectious outbreaks [39,40,41,42]. Despite advancements, limited research has been conducted on the impact of time delays in recovery and death rates within epidemiological models. In [43], the authors introduced a compartmental epidemiological model that incorporated distributed recovery and death rates. Under certain assumptions, this model can be reduced to the conventional SIR model. However, in most cases, the dynamics of the epidemic progression in this new model exhibit notable distinctions. To determine the distributed recovery and death rates, the authors evaluated COVID-19 data. By validating the model with epidemiological data from various countries, it demonstrated an improved agreement with the data compared to the SIR model. Furthermore, the authors estimated the time-dependent disease transmission rate. In [44], the authors utilized the average disease duration as the delay parameter to introduce a time delay in the recovery and death rates. The distributed model was discussed, and a delay model was derived. Epidemic characterization of the delay model was obtained. A numerical comparison was conducted among the distributed model, delay model, and conventional SIR model. Additionally, a method to estimate the disease duration using real disease incidence data was presented. The authors validated the delay model using epidemiological data from the COVID-19 epidemic and discussed the main outcomes and epidemiological implications of the proposed model. Moreover, apart from [44], the model proposed in [45] incorporated the infectivity period and disease duration instead of the incubation period, and did not include mandatory quarantine. This model considered the presence of exposed individuals and incorporated two time delays, namely the infectivity and disease duration.
In this study, we investigate epidemic models of either two viruses or viral strains. In the first model, we consider the presence of two viral strains, assuming that individuals recovered from either strain developed immunity to both strains. In the second model, we explore the scenario where individuals who have recovered from the first strain can be infected by the second strain, and similarly, individuals who have recovered from the second strain can be infected by the first strain. Here, we use the aforementioned modeling approach, based on distributed and point-wise delays in recovery and death rates.
The paper is structured as follows. In the first part, we introduce the model with distributed recovery and death rates in the presence of cross-immunity. We thoroughly examine the positiveness of solutions. Next, we reduce the distributed model to a conventional ODE model. Subsequently, we derive the delay model from the distributed model. Additionally, we determine the characteristics of epidemic progression for the delay model. Moreover, this structure is followed for the model without cross-immunity. The main outcomes of the proposed models and their epidemiological implications are discussed in the concluding section.
The recovery and death rates of infected individuals are influenced by the time elapsed since the onset of the disease. In this section, we will develop a model that takes the number of newly infected individuals and their time-dependent recovery and death rates into account [45]. We will examine various characteristics of this model and demonstrate that the conventional SIR model can be derived from the model under certain assumptions [43,44,45].
The following compartments of the total population N are considered:
● S(t) - susceptible individuals. They are not infected and can contract the disease.
● I1(t) - infected of the first viral strain. They are infectious and can transmit disease.
● I2(t) - infected of the second viral strain. They are infectious and can transmit disease.
● R1(t) - recovered from the first viral strain. They have recovered from the infection and are no longer infected. They are immune to both strains.
● R2(t) - recovered from the second viral strain. They have recovered from the infection and are no longer infected. They are immune to both strains.
● D1(t) - deceased from the first viral strain.
● D2(t) - deceased from the second viral strain.
We will use the following parameters and functions:
● The transmission rate of the first viral strain is denoted as β1, while the transmission rate of the second viral strain is denoted as β2.
● As a function of time since infection η, the recovery rate of individuals infected with the first viral strain is represented by r1(η). Similarly, as a function of time since infection η, the recovery rate of individuals infected with the second viral strain, is represented by r2(η).
● As a function of time since infection η, the mortality rate of the first viral strain is denoted as d1(η). Likewise, the mortality rate of the second viral strain is represented by d2(η).
● The number of newly infected individuals by the first viral strain and the second viral strain, denoted as J1(t) and J2(t), respectively, is determined by the relations J1(t)=(β1SI1)/N and J2(t)=(β2SI2)/N, respectively.
● The total number of individuals who have recovered from the first viral strain and the second viral strain at time t is represented by ∫t0r1(t−η)J1(η)dη and ∫t0r2(t−η)J2(η)dη, respectively.
● The total number of individuals who have died from the first viral strain and the second viral strain at time t is represented by ∫t0d1(t−η)J1(η)dη and ∫t0d2(t−η)J2(η)dη, respectively.
The two-strain model with distributed recovery and death rates has the following form:
dSdt=−J1(t)−J2(t), | (2.1) |
dI1dt=J1(t)−∫t0r1(t−η)J1(η)dη−∫t0d1(t−η)J1(η)dη, | (2.2) |
dI2dt=J2(t)−∫t0r2(t−η)J2(η)dη−∫t0d2(t−η)J2(η)dη, | (2.3) |
dR1dt=∫t0r1(t−η)J1(η)dη, | (2.4) |
dR2dt=∫t0r2(t−η)J2(η)dη, | (2.5) |
dD1dt=∫t0d1(t−η)J1(η)dη, | (2.6) |
dD2dt=∫t0d2(t−η)J2(η)dη. | (2.7) |
The system is subject to the following initial condition:
S(0)=S0>0, I1(0)=I1,0>0, I2(0)=I2,0>0, R1(0)=R2(0)=D1(0)=D2(0)=0, | (2.8) |
where I1,0 and I2,0 are sufficiently small as compared to N. We suppose that the total population is constant :
N=S(t)+I1(t)+I2(t)+R1(t)+R2(t)+D1(t)+D2(t). | (2.9) |
We establish the positiveness of the solution to this problem on a time interval t∈[0,T), where T∈(0,+∞). We assume that the recovery and death rates satisfy the following inequalities:
∫t0η(r1(t−η)+d1(t−η))dη≤1, | (2.10) |
∫t0η(r2(t−η)+d2(t−η))dη≤1, | (2.11) |
for any η≥0 and t0>η. These assumptions dictate that the number of recoveries and deaths on a given time interval cannot exceed the whole population.
Lemma 1. If conditions (2.10) and (2.11) are satisfied, then any solution of systems (2.1)–(2.7) with the initial condition (2.8) satisfies the inequality 0≤X≤N, where
X∈{S(t),I1(t),I2(t),R1(t),R2(t),D1(t),D2(t)}. |
Proof. From Eq (2.1), we observe that if there exists a time t∗>0 such that S(t∗)=0, then dSdt|t=t∗=0. This implies that S(t)≥0 for t>0. By examining Eqs (2.4)–(2.7), we can deduce that R1(t), R2(t), D1(t), and D2(t) also remain positive for all values of t≥0. At t=0, we have J1(0)=β1S0I1,0/N>0 and J2(0)=β2S0I2,0/N>0. Let t0>0 be such that J1(t) and J2(t) remain non-negative for 0≤t<t0. Next, we have the following:
I1(t0)=∫t00J1(t)dt−R1(t0)−D1(t0),I2(t0)=∫t00J2(t)dt−R2(t0)−D2(t0). |
Integrating Eqs (2.4)–(2.7) with respect to t from 0 to t0, and taking into account the initial conditions given in (2.8), we obtain the following:
R1(t0)+D1(t0)=∫t00(∫t0(r1(t−η)+d1(t−η))J1(η)dη)dt,R2(t0)+D2(t0)=∫t00(∫t0(r2(t−η)+d2(t−η))J2(η)dη)dt. |
Changing the order of integration and taking the inequalities (2.10) and (2.11) into account, we discover the following:
R1(t0)+D1(t0)=∫t00(∫t0η(r1(t−η)+d1(t−η))dt)J1(η)dη≤∫t00J1(η)dη, | (2.12) |
R2(t0)+D2(t0)=∫t00(∫t0η(r2(t−η)+d2(t−η))dt)J2(η)dη≤∫t00J2(η)dη. | (2.13) |
It follows from inequalities (2.12) and (2.13) that I1(t0) and I2(t0) are non-negative. Consequently, J1(t0) and J2(t0) are also non-negative. This implies that I1(t), I2(t), J1(t), and J2(t) remain non-negative for all t≥0. Furthermore, we have the relation (2.9). Hence, any solution to the system lies within the range of 0 and N, there by ensuring the non-negativity of the solution.
In this section, we show how models (2.1)–(2.7) can be reduced to the conventional ODE model. We consider the recovery and death rates in the following form:
r1(t)={r1,0 , 0≤t<τ10 , t>τ1, d1(t)={d1,0 , 0≤t<τ10 , t>τ1, | (2.14) |
r2(t)={r2,0 , 0≤t<τ20 , t>τ2, d2(t)={d2,0 , 0≤t<τ20 , t>τ2. | (2.15) |
The disease durations τ1 and τ2 and the parameters r1,0, r2,0, d1,0 and d2,0 are positive constants. By substituting these functions into Eqs (2.4)–(2.7), we obtain the following expressions:
dR1dt=r1,0∫tt−τ1J1(η)dη, dD1dt=d1,0∫tt−τ1J1(η)dη, | (2.16) |
dR2dt=r2,0∫tt−τ2J2(η)dη, dD2dt=d2,0∫tt−τ2J2(η)dη. | (2.17) |
By integrating Eq (2.16) from t−τ1 to t and Eq (2.17) from t−τ2 to t, we obtain the following:
R1(t)−R1(t−τ1)=r1,0∫tt−τ1(∫ss−τ1J1(η)dη)ds, | (2.18) |
D1(t)−D1(t−τ1)=d1,0∫tt−τ1(∫ss−τ1J1(η)dη)ds, | (2.19) |
R2(t)−R2(t−τ2)=r2,0∫tt−τ2(∫ss−τ2J2(η)dη)ds, | (2.20) |
D2(t)−D2(t−τ2)=d2,0∫tt−τ2(∫ss−τ2J2(η)dη)ds. | (2.21) |
Therefore,
I1(t)=∫tt−τ1J1(η)dη−(R1(t)−R1(t−τ1))−(D1(t)−D1(t−τ1)), | (2.22) |
I2(t)=∫tt−τ2J2(η)dη−(R2(t)−R2(t−τ1))−(D2(t)−D2(t−τ1)). | (2.23) |
We note that (R1(t)−R1(t−τ1)) and (D1(t)−D1(t−τ1)) correspond to the number of individuals who have recovered and died from the first viral strain during the time interval (t−τ1,t), respectively. Similarly, (R2(t)−R2(t−τ2)) and (D2(t)−D2(t−τ2)) represent the number of individuals who have recovered and died from the second viral strain during the time interval (t−τ2,t), respectively. From Eqs (2.18)–(2.21), we have the following:
I1(t)=∫tt−τ1J1(η)dη−(r1,0+d1,0)∫tt−τ1(∫ss−τ1J1(η)dη)ds, | (2.24) |
I2(t)=∫tt−τ2J2(η)dη−(r2,0+d2,0)∫tt−τ2(∫ss−τ2J2(η)dη)ds. | (2.25) |
Now, from (2.22)–(2.25),
dI1dt=β1SNI1−(r1,0+d1,0)∫tt−τ1J1(η)dη=β1SNI1−(r1,0+d1,0)[I1(t)+(r1,0+d1,0)∫tt−τ1(∫ss−τ1J1(η)dη)ds],dI2dt=β2SNI2−(r2,0+d2,0)∫tt−τ2J2(η)dη=β2SNI2−(r2,0+d2,0)[I2(t)+(r2,0+d2,0)∫tt−τ2(∫ss−τ2J2(η)dη)ds]. |
Under the assumption that (r1,0+d1,0) and (r2,0+d2,0) are sufficiently small, we can disregard the terms involving (r1,0+d1,0)2 and (r2,0+d2,0)2. Consequently, we obtain the following:
dI1dt≃β1SNI1−(r1,0+d1,0)I1,dI2dt≃β2SNI2−(r2,0+d2,0)I2. |
In this case, the system represented by Eqs (2.1)–(2.7) is simplified to the following conventional ODE model:
dSdt=−β1SNI1−β2SNI2,dI1dt=β1SNI1−(r1,0+d1,0)I1,dI2dt=β2SNI2−(r2,0+d2,0)I2,dR1dt=r1,0I1, dR2dt=r2,0I2,dD1dt=d1,0I1, dD2dt=d2,0I2. |
Therefore, assuming that the recovery and death rates have uniform distributions as given in Eqs (2.14) and (2.15), and that they are sufficiently small, we can reduce models (2.1)–(2.7) to the ODE model. However, it is important to note that, in general, these assumptions may not hold, and we can consider different distributions for recovery and death rates.
Let us recall that the duration of the first disease is denoted by τ1, and the duration of the second disease by τ2. Additionally, suppose that individuals J1(t−τ1) and J2(t−τ2) infected at time t−τ1 and t−τ2, respectively, either recover or die at time t with certain probabilities. This assumption corresponds to the following choices for the functions r1(t−η), r2(t−η), d1(t−η), and d2(t−η):
r1(t−τ1)=r1,0δ(t−η−τ1), r2(t−τ2)=r2,0δ(t−η−τ2), |
d1(t−τ1)=d1,0δ(t−η−τ1), d2(t−τ2)=d2,0δ(t−η−τ2), |
where r1,0, r2,0, d1,0, d2,0 are constants, r1,0+d1,0=1 and r2,0+d2,0=1, and δ is the Dirac delta-function. Then,
dR1dt=∫t0r1(t−η)J1(η)dη=r1,0∫t0δ(t−η−τ1)J1(η)dη=r1,0J1(t−τ1), |
dR2dt=∫t0r2(t−η)J2(η)dη=r2,0∫t0δ(t−η−τ2)J2(η)dη=r2,0J2(t−τ2), |
dD1dt=∫t0d1(t−η)J1(η)dη=d1,0∫t0δ(t−η−τ1)J1(η)dη=d1,0J1(t−τ1), |
dD2dt=∫t0d2(t−η)J2(η)dη=d2,0∫t0δ(t−η−τ2)J2(η)dη=d2,0J2(t−τ2). |
Therefore, the systems (2.1)–(2.7) are reduced to the following delay model:
dSdt=−J1(t)−J2(t), | (3.1) |
dIidt=Ji(t)−Ji(t−τi),i=1,2, | (3.2) |
dRidt=ri,0Ji(t−τi),i=1,2, | (3.3) |
dDidt=di,0Ji(t−τi),i=1,2 | (3.4) |
with J1(t)=J2(t)=0 for t<0.
Let us consider Eq (3.2) for the infected compartments:
dIidt=βiS(t)Ii(t)N−βiS(t−τi)Ii(t−τi)N, i=1,2. | (3.5) |
To determine the basic reproduction numbers R01 and R02. We set S(t)=S(t−τ1)=S(t−τ2)=S0 since the number of susceptible individuals is close to its initial condition in the beginning of the epidemic. Then, according to (3.5), we have
dIidt=βiS0N(Ii(t)−Ii(t−τi)), i=1,2. |
Substituting Ii(t)=I0ieλit, i=1,2, we have for i=1,2
λi=βiS0N(1−e−λiτi). | (3.6) |
Let
Gi(λi)=βiS0N(1−e−λiτi). |
It is evident that Eq (3.6) have solutions (i.e., λ1=λ2=0) and a non-zero solution, the sign of which is determined by G′i(0). Denote
R0i=G′i(0)=βiS0τiN. |
Let R01>1 and R02>1. This means that G′1(0)>1 and G′2(0)>1. We see that G1(λ1) and G2(λ2) are increasing functions of λ1 and λ2, respectively, with G1(λ1)→β1S0N as λ1→∞ and G2(λ2)→β2S0N as λ2→∞. This implies that Eq (3.6) has positive solutions, (i.e., there exist λ∗1>0 and λ∗2>0 such that Gi(λ∗i)=λ∗i, i=1,2).
If G′i(0)<1, then
Gi′(λi)=βiS0τiNe−λiτi<1, |
for all λ1≥0 and λ2≥0. Hence, there is no positive solution to the equation Gi(λi)=λi, i=1,2. Consequently, the basic reproduction numbers can be expressed as follows:
R0i=βiS0τiN, i=1,2. |
Next, we aim to estimate the final size of the susceptible compartment S∞=limt→∞S(t). Integrating (3.2), we obtain the following:
I1(t)=∫tt−τ1J1(η)dη,I2(t)=∫tt−τ2J2(η)dη. |
From (3.1), we obtain the following:
dS(t)dt=−β1S(t)N∫tt−τ1J1(η)dη−β2S(t)N∫tt−τ2J2(η)dη. |
Then, integrating from 0 to ∞, we obtain the following:
ln(S0S∞)=β1N∫∞0(∫tt−τ1J1(η)dη)dt+β2N∫∞0(∫tt−τ2J2(η)dη)dt. |
Changing the order of integration, we obtain the following:
ln(S0S∞)=β1N[∫0−τ1(∫η+τ10J1(η)dt)dη+∫∞0(∫η+τ1ηJ1(η)dt)dη]+β2N[∫0−τ2(∫η+τ20J2(η)dt)dη+∫∞0(∫η+τ2ηJ2(η)dt)dη].=β1N[∫0−τ1(η+τ1)J1(η)dη+∫∞0τ1J1(η)dη]+β2N[∫0−τ2(η+τ2)J2(η)dη+∫∞0τ2J2(η)dη]. |
Now, integrating (3.1) from 0 to ∞, we obtain the following:
∫∞0(J1(η)+J2(η))dη=S0−S∞. |
Since J1(t)=0 for all t∈[−τ1,0] and J2(t)=0 for all t∈[−τ2,0], then
ln(S0S∞)=β1N∫∞0τ1J1(η)dη+β2N∫∞0τ2J2(η)dη≤max(β1τ1S0N,β2τ2S0N)1S0∫∞0(J1(η)+J2(η))dη. |
Thus, we obtain an upper bound for the final size:
ln(S0S∞)≤max(R01,R02)(1−S∞S0). |
In the same way, we obtain a lower bound for the final size:
ln(S0S∞)≥min(R01,R02)(1−S∞S0) |
Hence, we obtain a two-sided estimate for S∞:
min(R01,R02)(1−S∞S0)≤ln(S0S∞)≤max(R01,R02)(1−S∞S0). | (3.7) |
Let us recall that in the case of a single strain, there is an equation for S∞ instead of the inequalities.
For the delay models (3.1)–(3.4), we compare the maximum of infected by each virus. We chose the following values for the parameters: N=104, I1,0=I2,0=1, β2=0.2, r1,0=0.7, r2,0=0.6, d1,0=0.3, d2,0=0.4, τ1=15.7 and τ2=17.7.
Figure 1 shows the number of infected individuals in time for each strain. In the first case, when R01>R02, the maximum of infected individuals is higher for the first strain. In the other case, when R01<R02, we obtain the same result. Therefore, the domination of the strains is not uniquely determined by the basic reproduction numbers but rather by the individual parameters.
Next, we choose the following values for the parameters: N=104, I1,0=I2,0=1, r1,0=0.7, r2,0=0.6, d1,0=0.3, d2,0=0.4, τ1=15.7 and τ2=17.7, and vary the values of β1 and β2 in the interval [0.1,0.5]. Figures 2–4 display the dependence of the maximal number of infected I1m and I2m, the time to the maximal number of infected for the first and the second virus and the total number of infected of the two virus of the models (3.1)–(3.4) on β1, β2.
Figure 2(a) shows when the transmission rate, β2, is fixed and the evolution of the epidemic peak is examined in relation to variations in β1. As β1 increases, the epidemic peak corresponding to the first virus also increases; for the second virus, the epidemic peak gradually decreases, and the number of individuals infected by the second virus decreases.
In other words, when comparing the impact of the first and second virus by modifying the transmission rate of the first virus, while keeping the transmission rate of the second epidemic constant, we observe that the first virus becomes more prevalent and reaches a higher epidemic peak as the transmission rate increases. On the other hand, the second virus is gradually eliminated, and its epidemic peak decreases as the transmission rate of the first virus increases.
It is interesting to note that in Figure 2(b), when β1 is fixed and β2 varies, we observe the opposite phenomenon. As β2 increases, the epidemic peak corresponding to the second virus also increases; while for the first virus, the epidemic peak gradually decreases. The last two graphs (c) and (d) present the variation of the epidemic peak for both viruses in relation to β1 and β2. These observations underscore the importance of the transmission rate in the spread of epidemics and how different values of β1 and β2 can influence the evolution of different epidemics.
The relationship between the timing of the epidemic peak and β1 and β2 can be complex. For β1>β2, if the two viruses infect the same number of individuals, then the first strain reaches the maximum faster than the other. Additionally, if the difference between β1 and β2 is large, then we can expect that the difference between t1m and t2m is also large, though this is not the case here. If β1>β2, the first strain infects more individuals to reach the maximum though it is faster, and the second strain infects less individuals to reach the maximum, though it is slower. Therefore, if β1 is very large compared to β2, this does not imply that t1m will be very large compared to t2m. As a result, the difference between the transmission rates does not strongly influence the peak time. Thus, t1m is either equal or very close to t2m (see Figure 3).
In terms of the dependence on β1 and β2 for the number of individuals affected by the first and second viruses, we can observe a similar phenomenon as seen in the epidemic peak (see Figure 4). As β1 increases, the number of individuals affected by the first virus tends to increase. This indicates that a higher transmission rate for the first virus leads to a larger population being infected by it compared to a larger population affected by the first virus. On the other hand, as β1 increases, the number of individuals affected by the second virus gradually decreases. This suggests that the second virus experiences a decline in its transmission and a lower number of individuals become infected by it.
Similarly, when β2 is varied while keeping β1 constant, higher values of β2 can lead to an increase in the number of individuals affected by the second virus; alternatively, the number of individuals affected by the first virus may decrease.
Finally, we estimate the inequality terms of (3.7). Let
Sl=min(R01,R02)(1−S∞S0),Sm=ln(S0S∞),Su=max(R01,R02)(1−S∞S0). |
In Figure 5, we can see that the graph of Sm is very close to the graph of Su. This result suggests the following conjecture:
ln(S0S∞)≃max(R01,R02)(1−S∞S0). |
In this section, we study a similar model assuming that individuals who have recovered from the first viral strain can be infected by the second viral strain, and individuals who have recovered from the second viral strain can be infected by the first viral strain.
Let J12(t)=(ϵ1β2I2R1)/N represent the number of individuals who have previously recovered from the first viral strain and are newly infected with the second viral strain. Similarly, J21(t)=(ϵ2β1I1R2)/N represents the number of individuals who have previously recovered from the second viral strain and are newly infected with the first viral strain, where ϵ1 and ϵ2 are constants that indicate the level of cross-immunity. For ϵ1=ϵ2=0, there is complete cross-immunity. For ϵ1= ϵ2=1, there is the absence of cross-immunity. In this modified system, we introduce additional variables to account for individuals who have recovered from one strain and are subsequently infected with the other strain. Let us define the following variables:
● I12(t) - infected with the first viral strain initially and later infected with the second viral strain.
● I21(t) - infected with the second viral strain initially and later infected with the first viral strain.
● R12(t) - recovered from the first viral strain initially and later infected with the second viral strain, becoming immune to both strains.
● R21(t) - recovered from the second viral strain initially and later infected with the first viral strain, becoming immune to both strains.
● D12(t) - recovered from the first viral strain initially and later die due to the second viral strain.
● D21(t) - recovered from the second viral strain initially and later die due to the first viral strain.
The individuals that are recovered and infected at time t−η with the first viral strain and the second viral strain are represented by J21(t−η) and J12(t−η), respectively. With these additional variables, the system can be expressed as follows, using the same notations as in the second section :
dSdt=−J1(t)−J2(t), | (5.1) |
dI1dt=J1(t)−∫t0r1(t−η)J1(η)dη−∫t0d1(t−η)J1(η)dη, | (5.2) |
dI2dt=J2(t)−∫t0r2(t−η)J2(η)dη−∫t0d2(t−η)J2(η)dη, | (5.3) |
dR1dt=∫t0r1(t−η)J1(η)dη−J12(t), | (5.4) |
dR2dt=∫t0r2(t−η)J2(η)dη−J21(t), | (5.5) |
dI12dt=J12(t)−∫t0r2(t−η)J12(η)dη−∫t0d2(t−η)J12(η)dη, | (5.6) |
dI21dt=J21(t)−∫t0r1(t−η)J21(η)dη−∫t0d1(t−η)J21(η)dη, | (5.7) |
dR12dt=∫t0r2(t−η)J12(η)dη, | (5.8) |
dR21dt=∫t0r1(t−η)J21(η)dη, | (5.9) |
dD1dt=∫t0d1(t−η)J1(η)dη, | (5.10) |
dD2dt=∫t0d2(t−η)J2(η)dη, | (5.11) |
dD12dt=∫t0d2(t−η)J12(η)dη, | (5.12) |
dD21dt=∫t0d1(t−η)J21(η)dη. | (5.13) |
The system is subject to the following initial condition:
S(0)=S0>0, I1(0)=I1,0>0, I2(0)=I2,0>0, R1(0)=R2(0)=I12(0)=I21(0)=R12(0)=R21(0)=D1(0)=D2(0)=D12(0)=D21(0)=0, | (5.14) |
where I1,0 and I2,0 are sufficiently small as compared to N. We assume that the total population is constant :
N=S(t)+I1(t)+I2(t)+R1(t)+R2(t)+I12(t)+I21(t)+R12(t)+R21(t)+D1(t)+D2(t)+D12(t)+D21(t). | (5.15) |
We prove the positivity of the solution to this problem on a time interval [0,T) where T∈(0,+∞). We make the assumption that the recovery and death rates satisfy the inequalities (2.10) and (2.11).
Lemma 2. If conditions (2.10) and (2.11) are satisfied, then any solution of systems (5.1)–(5.13) with the initial condition (5.14) satisfies the inequality 0≤X≤N, where
X∈{S(t),I1(t),I2(t),R1(t),R2(t),I12(t),I21(t),R12(t),R21(t),D1(t),D2(t),D12(t),D21(t)}. |
Proof. From Eq (5.1), we can observe that if there exists a t∗>0 such that S(t∗)=0, then dSdt|t=t∗=0. This implies that S(t)≥0 for t>0. Furthermore, based on Eqs (5.4), (5.5), and (5.8)–(5.13), we can conclude that R1(t), R2(t), R12(t), R21(t), D1(t), D2(t), D12(t), and D21(t) also remain positive for all values of t. At t=0, we have J1(0)=β1S0I1,0/N>0, J2(0)=β2S0I2,0/N>0 and J12(0)=J21(0)=0. Suppose t0>0 be such that J1(t), J2(t), J12(t), J21(t) remain non-negative for 0≤t<t0. Next, we have the following:
I1(t0)=∫t00J1(t)dt−R1(t0)−D1(t0)−∫t00J12(t)dt,I2(t0)=∫t00J2(t)dt−R2(t0)−D2(t0)−∫t00J21(t)dt. |
By integrating (5.4), (5.5), (5.10) and (5.11) with respect to t from 0 to t0, and using the initial conditions given in (5.14), obtain the following:
R1(t0)+D1(t0)=∫t00(∫t0(r1(t−η)+d1(t−η))J1(η)dη)dt−∫t00J12(t)dt,R2(t0)+D2(t0)=∫t00(∫t0(r2(t−η)+d2(t−η))J2(η)dη)dt−∫t00J21(t)dt. |
Changing the order of integration and using the inequalities (2.10) and (2.11), we observe the following:
R1(t0)+D1(t0)=∫t00(∫t0η(r1(t−η)+d1(t−η))dt)J1(η)dη−∫t00J12(t)dt≤∫t00J1(η)dη−∫t00J12(t)dt, | (5.16) |
R2(t0)+D2(t0)=∫t00(∫t0η(r2(t−η)+d2(t−η))dt)J2(η)dη−∫t00J21(t)dt≤∫t00J2(η)dη−∫t00J21(t)dt. | (5.17) |
It follows from the inequalities (5.16) and (5.17) that I1(t0) and I2(t0) are both non-negative. Consequently, J1(t0), J2(t0), J12(t0) and J21(t0) are also non-negative. Thus, we have shown that I1(t), I2(t), J1(t), J2(t), J12(t) and J21(t) remain non-negative for all t≥0. On the other hand, we have the following:
I12(t0)=∫t00J12(t)dt−R12(t0)−D12(t0),I21(t0)=∫t00J21(t)dt−R21(t0)−D21(t0). |
By integrating (5.8), (5.9), (5.12) and (5.13) with respect to t from 0 to t0 and using the initial conditions (5.14), we obtain the following:
R12(t0)+D12(t0)=∫t00(∫t0(r2(t−η)+d2(t−η))J12(η)dη)dt,R21(t0)+D21(t0)=∫t00(∫t0(r1(t−η)+d1(t−η))J21(η)dη)dt. |
Changing the order of integration and using inequalities (2.10) and (2.11), we observe the following:
R12(t0)+D12(t0)=∫t00(∫t0η(r2(t−η)+d2(t−η))dt)J12(η)dη≤∫t00J12(η)dη, | (5.18) |
R21(t0)+D21(t0)=∫t00(∫t0η(r1(t−η)+d1(t−η))dt)J21(η)dη≤∫t00J21(η)dη. | (5.19) |
It follows from the inequalities (5.18) and (5.19) that I12(t0) and I21(t0) are both non-negative. Consequently, I12(t) and I21(t) remain non-negative for all t≥0. Furthermore, we have (5.15). Thus, any solution of system (5.1)–(5.13) lies between 0 and N, thereby ensuring the non-negativity of the solution. ◻
In this section, we demonstrate that models (5.1)–(5.13) can be reduced to a conventional ODE model under certain assumptions. Let us assume that the recovery and death rates are given by the following functions :
r1(t)={r1,0 , 0≤t<τ10 , t>τ1, d1(t)={d1,0 , 0≤t<τ10 , t>τ1, | (5.20) |
r2(t)={r2,0 , 0≤t<τ20 , t>τ2, d2(t)={d2,0 , 0≤t<τ20 , t>τ2, | (5.21) |
The disease durations τ1,τ2>0 and the parameters r1,0, r2,0, d1,0 and d2,0 are positive constants. By substituting these functions in (5.4), (5.5) and (5.8)–(5.13), we obtain the following:
dR1dt=r1,0∫tt−τ1J1(η)dη−J12(t), dD1dt=d1,0∫tt−τ1J1(η)dη, | (5.22) |
dR2dt=r2,0∫tt−τ2J2(η)dη−J21(t), dD2dt=d2,0∫tt−τ2J2(η)dη, | (5.23) |
dR12dt=r2,0∫tt−τ2J12(η)dη, dD12dt=d2,0∫tt−τ2J12(η)dη, | (5.24) |
dR21dt=r1,0∫tt−τ1J21(η)dη, dD21dt=d1,0∫tt−τ1J21(η)dη. | (5.25) |
By integrating Eqs (5.22) and (5.25) from t−τ1 to t, and Eqs (5.23) and (5.24) from t−τ2 to t, we obtain the following:
R1(t)−R1(t−τ1)=r1,0∫tt−τ1(∫ss−τ1J1(η)dη)ds−∫tt−τ1J12(η)dη, | (5.26) |
D1(t)−D1(t−τ1)=d1,0∫tt−τ1(∫ss−τ1J1(η)dη)ds, | (5.27) |
R2(t)−R2(t−τ2)=r2,0∫tt−τ2(∫ss−τ2J2(η)dη)ds−∫tt−τ2J21(η)dη, | (5.28) |
D2(t)−D2(t−τ2)=d2,0∫tt−τ2(∫ss−τ2J2(η)dη)ds, | (5.29) |
R21(t)−R21(t−τ1)=r1,0∫tt−τ1(∫ss−τ1J21(η)dη)ds, | (5.30) |
D21(t)−D21(t−τ1)=d1,0∫tt−τ1(∫ss−τ1J21(η)dη)ds, | (5.31) |
R12(t)−R12(t−τ2)=r2,0∫tt−τ2(∫ss−τ2J12(η)dη)ds, | (5.32) |
D12(t)−D12(t−τ2)=d2,0∫tt−τ2(∫ss−τ2J12(η)dη)ds. | (5.33) |
Thus,
I1(t)=∫tt−τ1J1(η)dη−(R1(t)−R1(t−τ1))+∫tt−τ1J12(η)dη−(D1(t)−D1(t−τ1)), | (5.34) |
I2(t)=∫tt−τ2J2(η)dη−(R2(t)−R2(t−τ2))+∫tt−τ2J21(η)dη−(D2(t)−D2(t−τ2)), | (5.35) |
I21(t)=∫tt−τ1J21(η)dη−(R21(t)−R21(t−τ1))−(D21(t)−D21(t−τ1)), | (5.36) |
I12(t)=∫tt−τ2J12(η)dη−(R12(t)−R12(t−τ2))−(D12(t)−D12(t−τ2)), | (5.37) |
where (R1(t)−R1(t−τ1)) and (D1(t)−D1(t−τ1)) represent the number of individuals who have recovered and died from the first viral strain during the time interval (t−τ1,t), respectively. Similarly, (R2(t)−R2(t−τ2)) and (D2(t)−D2(t−τ2)) represent the number of individuals who have recovered and died from the second viral strain during the time interval (t−τ2,t), respectively. Moreover, the expressions (R21(t)−R21(t−τ1)), (R12(t)−R12(t−τ2)), (D21(t)−D21(t−τ1)), and (D12(t)−D12(t−τ2)) characterize the difference in the number of recovered and died individuals for two viral strains at different time intervals. Hence, from (5.26)–(5.33), we have the following:
I1(t)=∫tt−τ1J1(η)dη−(r1,0+d1,0)∫tt−τ1(∫ss−τ1J1(η)dη)ds, | (5.38) |
I2(t)=∫tt−τ2J2(η)dη−(r2,0+d2,0)∫tt−τ2(∫ss−τ2J2(η)dη)ds, | (5.39) |
I21(t)=∫tt−τ1J21(η)dη−(r1,0+d1,0)∫tt−τ1(∫ss−τ1J21(η)dη)ds, | (5.40) |
I12(t)=∫tt−τ2J12(η)dη−(r2,0+d2,0)∫tt−τ2(∫ss−τ2J12(η)dη)ds. | (5.41) |
Now, from (5.38)–(5.41),
dI1dt=β1SNI1−(r1,0+d1,0)∫tt−τ1J1(η)dη=β1SNI1−(r1,0+d1,0)[I1(t)+(r1,0+d1,0)∫tt−τ1(∫ss−τ1J1(η)dη)ds], | (5.42) |
dI2dt=β2SNI2−(r2,0+d2,0)∫tt−τ2J2(η)dη=β2SNI2−(r2,0+d2,0)[I2(t)+(r2,0+d2,0)∫tt−τ2(∫ss−τ2J2(η)dη)ds], | (5.43) |
dI21dt=ϵ2β1R2NI1−(r1,0+d1,0)∫tt−τ1J21(η)dη=ϵ2β1R2NI1−(r1,0+d1,0)[I21(t)+(r1,0+d1,0)∫tt−τ1(∫ss−τ1J21(η)dη)ds], | (5.44) |
dI12dt=ϵ1β2R1NI2−(r2,0+d2,0)∫tt−τ2J2(η)dη=ϵ1β2R1NI2−(r2,0+d2,0)[I12(t)+(r2,0+d2,0)∫tt−τ2(∫ss−τ2J12(η)dη)ds]. | (5.45) |
Assuming that (r1,0+d1,0) and (r2,0+d2,0) are small enough, we neglect the terms involving (r1,0+d1,0)2 and (r2,0+d2,0)2. Hence, we obtain the following:
dI1dt≃β1SNI1−(r1,0+d1,0)I1, | (5.46) |
dI2dt≃β2SNI2−(r2,0+d2,0)I2, | (5.47) |
dI21dt≃ϵ2β1R2NI1−(r1,0+d1,0)I21, | (5.48) |
dI12dt≃ϵ1β2R1NI2−(r2,0+d2,0)I12. | (5.49) |
In this case, systems (5.1)–(5.13) is reduced to the following conventional ODE model:
dSdt=−β1SNI1−β2SNI2,dI1dt=β1SNI1−(r1,0+d1,0)I1,dI2dt=β2SNI2−(r2,0+d2,0)I2,dR1dt=r1,0I1−ϵ1β2R1NI2,dR2dt=r2,0I2−ϵ2β1R2NI1,dI21dt=ϵ2β1R2NI1−(r1,0+d1,0)I21,dI12dt=ϵ1β2R1NI2−(r2,0+d2,0)I12,dR21dt=r1,0I21, dR12dt=r2,0I12,dD1dt=d1,0I1, dD2dt=d2,0I2, dD21dt=d1,0I21, dD12dt=d2,0I12. |
Therefore, if we assume that the recovery and death rates described in Eqs (5.20) and (5.21) follow uniform distributions and are sufficiently small, we can reduce the model given by Eqs (5.1)–(5.13) to the ODE model. However, in general, these assumptions may not hold. Another approximation of recovery and death rate distributions is considered in the next section.
Let us recall that the duration of the first and the second disease are denoted by τ1 and τ2, respectively. Furthermore, suppose that individuals J1(t−τ1) and J21(t−τ1) infected at time t−τ1 and individuals J2(t−τ2) and J12(t−τ2) infected at time t−τ2, either recover or die at time t with certain probabilities. This assumption corresponds to the following choice of the functions r1(t−η), r2(t−η), d1(t−η) and d2(t−η):
r1(t−τ1)=r1,0δ(t−η−τ1), r2(t−τ2)=r2,0δ(t−η−τ2),d1(t−τ1)=d1,0δ(t−η−τ1), d2(t−τ2)=d2,0δ(t−η−τ2), |
where r1,0, r2,0, d1,0, d2,0 are constants, r1,0+d1,0=1 and r2,0+d2,0=1, and δ is the Dirac delta-function. Then
dR1dt=∫t0r1(t−η)J1(η)dη−J12(t)=r1,0∫t0δ(t−η−τ1)J1(η)dη−J12(t)=r1,0J1(t−τ1)−J12(t),dR2dt=∫t0r2(t−η)J2(η)dη−J21(t)=r2,0∫t0δ(t−η−τ2)J2(η)dη−J21(t)=r2,0J2(t−τ2)−J21(t),dR12dt=∫t0r2(t−η)J12(η)dη=r2,0∫t0δ(t−η−τ2)J12(η)dη=r2,0J12(t−τ2),dR21dt=∫t0r1(t−η)J21(η)dη=r1,0∫t0δ(t−η−τ1)J21(η)dη=r1,0J21(t−τ1),dD1dt=∫t0d1(t−η)J1(η)dη=d1,0∫t0δ(t−η−τ1)J1(η)dη=d1,0J1(t−τ1),dD2dt=∫t0d2(t−η)J2(η)dη=d2,0∫t0δ(t−η−τ2)J2(η)dη=d2,0J2(t−τ2),dD12dt=∫t0d2(t−η)J12(η)dη=d2,0∫t0δ(t−η−τ2)J12(η)dη=d2,0J12(t−τ2),dD21dt=∫t0d1(t−η)J21(η)dη=d1,0∫t0δ(t−η−τ1)J21(η)dη=d1,0J21(t−τ1). |
Therefore, systems (5.1)–(5.13) is reduced to the following delay model:
dSdt=−J1(t)−J2(t), | (6.1) |
dIidt=Ji(t)−Ji(t−τi),i=1,2, | (6.2) |
dRidt=ri,0Ji(t−τi)−Jij(t),i,j=1,2,j≠i, | (6.3) |
dIijdt=Jij(t)−Jij(t−τj),i,j=1,2,j≠i, | (6.4) |
dRijdt=rj,0Jij(t−τj),i,j=1,2,j≠i, | (6.5) |
dDidt=di,0Ji(t−τi),i=1,2, | (6.6) |
dDijdt=dj,0Jij(t−τj),i,j=1,2,j≠i | (6.7) |
with J1(t)=J2(t)=J12(t)=J21(t)=0 for t<0.
Using the arguments similar to those in Subsections 3.2 and 3.3, it can be demonstrated that the basic reproduction numbers can be expressed as follows:
R0i=βiS0τiN, i=1,2. |
Additionally, we obtain a two-sided estimate for S∞:
min(R01,R02)(1−S∞S0)≤ln(S0S∞)≤max(R01,R02)(1−S∞S0). |
Using the Figure 5, we obtain the following:
ln(S0S∞)≃max(R01,R02)(1−S∞S0). |
For the delay models (6.1)–(6.7), we compare the maximum number of infected individuals and the timing of this peak, as well as the total number of infected individuals caused by each virus. We choose the following values for the parameters: population size N=104, initial infections I1,0=I2,0=1, delays τ1=15.7 and τ2=17.7, and transmission rates β1=0.25, β2=0.2 and the constants r1,0=0.7, r2,0=0.6, d1,0=0.3, d2,0=0.4. Additionally, we set ϵ1=0.8 and ϵ2=1.
In the first infection, where β1>β2, Figure 6 shows that the maximal number of infected individuals during the first strain is higher than the maximal number of infected individuals observed during the second strain. In the second infection, it is worth noting that the maximum value of I21 is lower than the maximum value of I12 where, in this case, the maximum depends on the parameters β1, β2, ϵ1, ϵ2, r1,0 and r2,0.
The maximum number of infected individuals behaves similarly to a single strain scenario, where the maximum is determined by the strain with the larger transmission rate (i.e, (I1+I21)m>(I2+I12)m). Furthermore, with regards to the timing of the maximum, the difference between the transmission rates does not strongly influence on the peak time. Thus, t1m is very close to t2m.
Next, let us consider another set of parameters - N=104, I1,0=I2,0=1, r1,0=0.7, r2,0=0.6, d1,0=0.3, d2,0=0.4, τ1=15.7, τ2=17.7 - and vary the values of β1 and β2 within the range of [0.1,0.5]. During the first infection with a fixed transmission rate β2 (β2<β1), increasing the transmission rate β1 causes the total number of individuals infected by the first strain (R1f+D1f) to approach the entire population N, while the total number of individuals infected by the second strain (R2f+D2f) diminishes significantly, nearly reaching zero. Conversely, with a fixed transmission rate β1 (β1<β2), by increasing the transmission rate β2, the total number of individuals infected by the second strain (R2f+D2f) approaches the entire population N, while the total number of individuals infected by the first strain (R1f+D1f) decreases significantly, nearly reaching zero. Notably, 70 of those infected by the first strain and 60 of those infected by the second strain have recovered.
In the second infection, by increasing the transmission rate β1 (β2<β1), the total number of individuals infected by the second strain (R12f+D12f) approaches 70 of the total population N, while the total number of individuals infected by the first strain (R21f+D21f) approaches a very small value close to zero. On the other hand, increasing the transmission rate β2 (β1<β2) causes the total number of individuals infected by the first strain (R21f+D21f) to approach 60 of the total population N, while the total number of individuals infected by the second strain (R12f+D12f) approaches a very small value close to zero.
Consequently, if β1 is increased, then the number of individuals affected by the first virus (R1f+D1f+R21f+D21f) also increases. This indicates that a higher transmission rate for the first virus leads to a larger proportion of the population being infected by it.
Conversely, with an increase in β1, the number of individuals affected by the second virus (R2f+D2f+R12f+D12f) gradually decreases. This suggests that the transmission of the second virus diminishes, thereby resulting in a lower number of individuals becoming infected by it.
Similarly, when β2 is changed while keeping β1 constant, increasing β2 can lead to a higher number of individuals being affected by the second virus, while the number of individuals affected by the first virus may decrease. Note that the total number of infected individuals exceeds the total population because there are individuals who have been infected by both strain.
In this paper we introduced two epidemiological models, each of which described the dynamics of the dual viral strain SIRD model with distributed recovery and death rates to track the impact of multiple strains variants during an epidemic. In the first model, some individuals may be infected by the first strain and others by the second strain. Furthermore, individuals who have recovered from either strain develop immunity to both strains. In the second model, we explored the scenario where individuals who have recovered from the first strain can be infected by the second strain, and similarly, individuals who have recovered from the second strain can be infected by the first strain.
The proposed distributed delay models, represented by integro-differential equations, offer a more accurate description of epidemic dynamics. However, these models are relatively complex and require knowledge of distributed recovery and death rates, which may not be readily available in the literature. To compensate this disadvantage of the distributed models, we derived two simplified models from each model to address different limiting cases. In the first case, assuming that the recovery and death rates are uniformly distributed, we obtained a conventional compartmental ODE model. In the second case, considering the recovery and death rate distributions as delta-function, we obtained a novel delay model that has not been previously explored in the epidemiological literature. As mentioned in [43,44,45], since distributed recovery and death rates are commonly described by a Gamma-distribution, approximating them with a delta-function can provide a more accurate representation, especially in capturing the dynamics during the initial phase of infection. The point-wise delay models, based on the duration of the disease and known recovery and death rates, provide a simple and biologically meaningful representation of epidemics.
For these delay model we determined the reproduction numbers for each strain R0j, j=1,2. We employed these delay models to perform a numerical comparison of the maximum number of infected individuals, the peak timing and the total number of infected cases by each virus. This analysis was conducted by utilizing the final size of the recovered and deceased individuals. The transmission rates of the diseases played a crucial role in determining these outcomes, as they significantly influenced the dynamics of the epidemics being studied.
We compared the two models with and without cross-immunity. The maximum number of infected individuals in the model without cross-immunity was higher than in the other model because there were individuals who had been infected with both viruses. The timing of the maximum was either equal or very close between the two models. In the model without cross-immunity, the total number of infected individuals surpassed the total population because some individuals could be infected by both strains simultaneously. As a consequence, the number of infected individuals would be higher compared to the total number of infected individuals in the other model (with cross-immunity). As result, cross-immunity between the two viruses can significantly reduce their global spread. Individuals already immunized against one of the viruses would decrease the total number of cases of diseases caused by these pathogens. If a large part of the population acquires this cross-immunity, it would limit the spread of viruses and minimize their impact on public health. In the absence of cross-immunity, the spread of both viruses would accelerate, potentially leading to serious co-infections and increasing the burden on healthcare systems. Therefore, it is crucial to understand and promote cross-immunity to better prevent and control these viral diseases.
Comparing our results with the data, we used the example of Omicron and Delta variants in COVID-19, where Omicron exhibited a higher transmission rate. In [46], the authors showed that the duration of encounters played a crucial role in modeling and significantly impacted disease progression and the competition between variants, where the faster spreading strain dominated over the slower strain. Reference [12] reports that Omicron rapidly spread across France and displayed higher fitness compared to Delta. Additionally, reference [13] estimated that the total number of individuals infected by Omicron exceeds the total number of those infected by Delta. All of these works confirm our result that the rate of transmission is crucial in which strain dominates the other. In general, the strain with a higher transmission rate (Omicron) tends to have a higher maximum number of infected individuals and a higher total number of infected individuals compared to the other strain (Delta).
The authors declare they have not used Artificial Intelligence (AI) tools in the creation of this article.
The authors declare there is no conflict of interest.
The authors express their gratitude to the referees for careful reading and valuable comments which led to an important improvement of the original version of this work. This work has been supported by PHC (Partenariat Hubert Curien) FRANCE-MAGHREB project having the code number 22MAG22–47518WK. V.V was supported by the Ministry of Science and Higher Education of the Russian Federation (megagrant agreement No. 075-15-2022-1115).
[1] |
EFSA (2022) The European Union One Health 2021 Zoonoses Report. EFSA J 20: e07666. https://doi.org/10.2903/j.efsa.2022.7666 doi: 10.2903/j.efsa.2022.7666
![]() |
[2] |
Townsend A, Strawn LK, Chapman BJ, et al. (2021) A systematic review of Listeria species and Listeria monocytogenes prevalence, persistence, and diversity throughout the fresh produce supply chain. Foods 10: 1427. https://doi.org/10.3390/foods10061427 doi: 10.3390/foods10061427
![]() |
[3] |
Tack DM, Ray L, Griffin PM, et al. (2020) Preliminary incidence and trends of infections with pathogens transmitted commonly through food—Foodborne diseases active surveillance network, 10 U.S. Sites, 2016–2019. MMWR Morb Mortal Wkly Rep 69: 509–514. https://doi.org/10.15585/mmwr.mm6917a1 doi: 10.15585/mmwr.mm6917a1
![]() |
[4] |
Santos dos. JS, Biduski B, Santos dos. LR (2021) Listeria monocytogenes: health risk and a challenge for food processing establishments. Arch Microbiol 203: 5907–5919. https://doi.org/10.1007/s00203-021-02590-2 doi: 10.1007/s00203-021-02590-2
![]() |
[5] | Habib I, Mohamed MYI (2022) Chapter 3—Foodborne infections in the Middle East. In: Savvaidis IN, Osaili TM (Eds.), Food Safety in the Middle East, Elsevier, 71–107. https://doi.org/10.1016/B978-0-12-822417-5.00005-2 |
[6] |
Habib I, Mohamed MYI, Khan M (2021) Current state of Salmonella, Campylobacter and Listeria in the food chain across the arab countries: A descriptive review. Foods 10: 2369. https://doi.org/10.3390/foods10102369 doi: 10.3390/foods10102369
![]() |
[7] |
Elafify M, Darwish WS, El-Toukhy M, et al. (2022) Prevalence of multidrug resistant Salmonella spp. in dairy products with the evaluation of the inhibitory effects of ascorbic acid, pomegranate peel extract, and D-tryptophan against Salmonella growth in cheese. Int J Food Microbiol 364: 109534. https://doi.org/10.1016/j.ijfoodmicro.2022.109534 doi: 10.1016/j.ijfoodmicro.2022.109534
![]() |
[8] |
Zahli R, Scheu AK, Abrini J, et al. (2022) Salmonella spp: Prevalence, antimicrobial resistance and molecular typing of strains isolated from poultry in Tetouan-Morocco. LWT 153: 112359. https://doi.org/10.1016/j.lwt.2021.112359 doi: 10.1016/j.lwt.2021.112359
![]() |
[9] |
El Kenawy A, McCabe M, Vicente-Serrano S, et al. (2016) Recent changes in continentality and aridity conditions over the Middle East and North Africa region, and their association with circulation patterns. Clim Res 69: 25–43. https://doi.org/10.3354/cr01389 doi: 10.3354/cr01389
![]() |
[10] |
Mohamed M-YI (2024) Campylobacteriosis in North Africa. AIMS Agric Food 9: 801–821. https://doi.org/10.3934/agrfood.2024043 doi: 10.3934/agrfood.2024043
![]() |
[11] |
Mohamed M-YI, Khalifa HO, Habib I (2025) Food pathways of Salmonella and its ability to cause gastroenteritis in North Africa. Foods 14: 253. https://doi.org/10.3390/foods14020253 doi: 10.3390/foods14020253
![]() |
[12] |
Gray J, Chandry PS, Kaur M, et al. (2021) Colonisation dynamics of Listeria monocytogenes strains isolated from food production environments. Sci Rep 11: 12195. https://doi.org/10.1038/s41598-021-91503-w doi: 10.1038/s41598-021-91503-w
![]() |
[13] |
Rafei R, Hawli M, Osman M, et al. (2020) Distribution of EMM types and macrolide resistance determinants among group A Streptococci in the Middle East and North Africa region. J Glob Antimicrob Resist 22: 334–348. https://doi.org/10.1016/j.jgar.2020.02.005 doi: 10.1016/j.jgar.2020.02.005
![]() |
[14] |
Spanu C, Jordan K (2020) Listeria monocytogenes environmental sampling program in ready‐to‐eat processing facilities: A practical approach. Compr Rev Food Sci Food Saf 19: 2843–2861. https://doi.org/10.1111/1541-4337.12619 doi: 10.1111/1541-4337.12619
![]() |
[15] | WHO (2015) WHO Estimates of the Global Burden of Foodborne Diseases: Foodborne Disease Burden Epidemiology Reference Group 2007–2015; World Health Organization: Geneva, Switzerland. |
[16] |
Dufailu OA, Yaqub MO, Owusu-Kwarteng J, et al. (2021) Prevalence and characteristics of Listeria species from selected African countries. Trop Dis Travel Med Vaccines 7: 26. https://doi.org/10.1186/s40794-021-00151-5 doi: 10.1186/s40794-021-00151-5
![]() |
[17] | Mascellino MT (2020) Prologue: Escherichia coli, Listeria, and Salmonella, New Insight into Brucella Infection and Foodborne Diseases, IntechOpen. |
[18] |
Osek J, Lachtara B, Wieczorek K (2022) Listeria monocytogenes in foods-From culture identification to whole‐genome characteristics. Food Sci Nutr 10: 2825–2854. https://doi.org/10.1002/fsn3.2910 doi: 10.1002/fsn3.2910
![]() |
[19] |
Chen M, Cheng J, Zhang J, et al. (2019) Isolation, potential virulence, and population diversity of Listeria monocytogenes from meat and meat products in China. Front Microbiol 10: 946. https://doi.org/10.3389/fmicb.2019.00946 doi: 10.3389/fmicb.2019.00946
![]() |
[20] |
Lasagabaster A, Jiménez E, Lehnherr T, et al. (2020) Bacteriophage biocontrol to fight Listeria outbreaks in seafood. Food Chem Toxicol 145: 111682. https://doi.org/10.1016/j.fct.2020.111682 doi: 10.1016/j.fct.2020.111682
![]() |
[21] |
Miceli A, Settanni L (2019) Influence of agronomic practices and pre-harvest conditions on the attachment and development of Listeria monocytogenes in vegetables. Ann Microbiol 69: 185–199. https://doi.org/10.1007/s13213-019-1435-6 doi: 10.1007/s13213-019-1435-6
![]() |
[22] |
Inoue T, Itani T, Inomata N, et al. (2017) Listeria monocytogenes septicemia and meningitis caused by Listeria enteritis complicating ulcerative colitis. Int Med 56: 2655–2659. https://doi.org/10.2169/internalmedicine.8654-16 doi: 10.2169/internalmedicine.8654-16
![]() |
[23] |
Turner LH, Kinder JM, Wilburn A, et al. (2017) Preconceptual Zika virus asymptomatic infection protects against secondary prenatal infection. PLoS Pathog 13: e1006684. https://doi.org/10.1371/journal.ppat.1006684 doi: 10.1371/journal.ppat.1006684
![]() |
[24] |
Reda WW, Abdel-Moein K, Hegazi A, et al. (2016) Listeria monocytogenes: An emerging food-borne pathogen and its public health implications. J Infect Dev Countries 10: 149–154. https://doi.org/10.3855/jidc.6616 doi: 10.3855/jidc.6616
![]() |
[25] |
King MT, Huh I, Shenai A, et al. (2018) Structural basis of VHH-mediated neutralization of the food-borne pathogen Listeria monocytogenes. J Biol Chem 293: 13626–13635. https://doi.org/10.1074/jbc.RA118.003888 doi: 10.1074/jbc.RA118.003888
![]() |
[26] |
Nouichi S, Mezali L, Hamdi TM (2022) Distribution of Salmonella virulence factors originated from sheep and cattle in Algerian slaughterhouses. J Hell Vet Med Soc 73: 5013–5020. https://doi.org/10.12681/jhvms.29148 doi: 10.12681/jhvms.29148
![]() |
[27] |
Eckstein S, Ehmann R, Gritli A, et al. (2022) Viral and Bacterial Zoonotic Agents in Dromedary Camels from Southern Tunisia: A Seroprevalence Study. Microorganisms 10: 727. https://doi.org/10.3390/microorganisms10040727 doi: 10.3390/microorganisms10040727
![]() |
[28] |
Paré G, Trudel MC, Jaana M, et al. (2015) Synthesizing information systems knowledge: A typology of literature reviews. Inf Manage 52: 183–199. https://doi.org/10.1016/j.im.2014.08.008 doi: 10.1016/j.im.2014.08.008
![]() |
[29] |
Geteneh A, Biset S, Tadesse S, et al. (2022) A vigilant observation to pregnancy associated listeriosis in Africa: Systematic review and meta-analysis. PLOS Global Public Health 2: e0001023. https://doi.org/10.1371/journal.pgph.0001023 doi: 10.1371/journal.pgph.0001023
![]() |
[30] |
Mohamed M-YI, Abu J, Aziz SA, et al. (2022) Occurrence of antibiotic resistant C. jejuni and E. coli in wild birds, chickens, humans, and the environment in Malay villages, Kedah, Malaysia. Vet Med (Praha) 67: 298–308. https://doi.org/10.17221/102/2021-VETMED doi: 10.17221/102/2021-VETMED
![]() |
[31] |
Oueslati W, Ridha Rjeibi M, Benyedem H, et al. (2023) Serotype occurrence, virulence profiles, antimicrobial resistance and molecular characterization of Salmonella isolated from hospitalized patients with gastroenteritis in great Tunisia between 2010 and 2020. Antibiotics 12: 526. https://doi.org/10.3390/antibiotics12030526 doi: 10.3390/antibiotics12030526
![]() |
[32] |
Benhalima L, Merad T, Bensouilah M, et al. (2019) Listeria monocytogenes and other Listeria species in raw milk and sausage in East Algeria. Asian J Dairy Food Res 38: 7–11. https://doi.org/10.18805/ajdfr.DR-128 doi: 10.18805/ajdfr.DR-128
![]() |
[33] |
Mohamed M-YI, Habib I (2023) Pathogenic E. coli in the food chain across the Arab Countries: A Descriptive Review. Foods 12: 3726. https://doi.org/10.3390/foods12203726 doi: 10.3390/foods12203726
![]() |
[34] |
Mohamed M-YI, Lakshmi GB, Sodagari H, et al. (2024) A one health perspective on camel meat hygiene and zoonoses: Insights from a decade of research in the Middle East. Vet Sci 11: 344. https://doi.org/10.3390/vetsci11080344 doi: 10.3390/vetsci11080344
![]() |
[35] |
Sarr M, Alou MT, Padane A, et al. (2023) A review of the literature of Listeria monocytogenes in Africa highlights breast milk as an overlooked human source. Front Microbiol 14: 1213953. https://doi.org/10.3389/fmicb.2023.1213953 doi: 10.3389/fmicb.2023.1213953
![]() |
[36] |
Eleftherios Mylonakis MD, Maria Paliou MD, Elizabeth L. Hohmann MD, et al. (2002) Listeriosis during pregnancy a case series and review of 222 cases. Lippincott Williams & Wilkins, Inc 81: 260–269. https://doi.org/10.1097/00005792-200207000-00002 doi: 10.1097/00005792-200207000-00002
![]() |
[37] |
EL-Naenaeey E-S, Abdelwahab A, Merwad A, et al. (2019) Prevalence of Listeria species in dairy cows and pregnant women with reference to virulotyping of Listeria monocytogenes in Egypt. Zagazig Vet J 47: 248–258. https://doi.org/10.21608/zvjz.2019.12896.1042 doi: 10.21608/zvjz.2019.12896.1042
![]() |
[38] |
Koopmans MM, Brouwer MC, Vázquez-Boland JA, et al. (2023) Human listeriosis. Clin Microbiol Rev 36: No.1. https://doi.org/10.1128/cmr.00060-19 doi: 10.1128/cmr.00060-19
![]() |
[39] |
Dapgh AN, ELGedawy AA, Abouelhag HA, et al. (2020) Advanced identification and characterization of Listeria species in Egyptian local soft cheese. South Asian J Res Microbiol 8: 11–18. https://doi.org/10.9734/sajrm/2020/v8i230188 doi: 10.9734/sajrm/2020/v8i230188
![]() |
[40] |
Martinez-Rios V, Gkogka E, Dalgaard P (2020) Predicting growth of Listeria monocytogenes at dynamic conditions during manufacturing, ripening and storage of cheeses—Evaluation and application of models. Food Microbiol 92: 103578. https://doi.org/10.1016/j.fm.2020.103578 doi: 10.1016/j.fm.2020.103578
![]() |
[41] |
Abdeen EE, Mousa WS, Harb OlaH, et al. (2021) Prevalence, antibiogram and genetic characterization of Listeria monocytogenes from food products in Egypt. Foods 10: 1381. https://doi.org/10.3390/foods10061381 doi: 10.3390/foods10061381
![]() |
[42] |
Mahmoud H, Karmi M, Maky M (2019) Occurrence and characterization of Listeria species isolated from processed meat in Qena, Egypt. Zagazig Vet J 47: 267–276. https://doi.org/10.21608/zvjz.2019.13161.1044 doi: 10.21608/zvjz.2019.13161.1044
![]() |
[43] | Ahmed HA, Hussein l MA, El-Ashram AMM (2013) Seafood a potential source of some zoonotic bacteria in Zagazig, Egypt, with the molecular detection of Listeria monocytogenes virulence genes. Vet Ital 49: 299–308. |
[44] |
Saleh SO, Hussien AA, Youseef AG, et al. (2024) Prevalence, antibiotic resistance, and phylogenetic analysis of Listeria monocytogenes isolated from various sources in Egypt: fish, vegetables, and humans. Iraqi J Vet Sci 38: 15–27. https://doi.org/10.33899/ijvs.2023.140242.3033 doi: 10.33899/ijvs.2023.140242.3033
![]() |
[45] |
Alegbeleye O, Sant'Ana AS (2022) Impact of temperature, soil type and compost amendment on the survival, growth and persistence of Listeria monocytogenes of non-environmental (food-source associated) origin in soil. Sci Total Environ 843: 157033. https://doi.org/10.1016/j.scitotenv.2022.157033 doi: 10.1016/j.scitotenv.2022.157033
![]() |
[46] |
Gartley S, Anderson-Coughlin B, Sharma M, et al. (2022) Listeria monocytogenes in irrigation water: An assessment of outbreaks, sources, prevalence, and persistence. Microorganisms 10: 1319. https://doi.org/10.3390/microorganisms10071319 doi: 10.3390/microorganisms10071319
![]() |
[47] |
Osek J, Lachtara B, Wieczorek K (2022) Listeria monocytogenes – how this pathogen survives in food-production environments? Front Microbiol 13: 866462. https://doi.org/10.3389/fmicb.2022.866462 doi: 10.3389/fmicb.2022.866462
![]() |
[48] |
Osman KM, Kappell AD, Fox EM, et al. (2019) Prevalence, pathogenicity, virulence, antibiotic resistance, and phylogenetic analysis of biofilm-producing Listeria monocytogenes isolated from different ecological niches in Egypt: food, humans, animals, and environment. Pathogens 9: 5. https://doi.org/10.3390/pathogens9010005 doi: 10.3390/pathogens9010005
![]() |
[49] |
Kulesh R, Shinde SV, Khan WA, et al. (2022) The occurrence of Listeria monocytogenes in goats, farm environment and invertebrates. Biol Rhythm Res 53: 831–840. https://doi.org/10.1080/09291016.2019.1660836 doi: 10.1080/09291016.2019.1660836
![]() |
[50] |
Shafik S, Abdelrahman MA (2019) Molecular characterization of Listeria monocytogenes isolated from some abattoirs in Dakahlia, Egypt. Assiut Vet Med J 65: 95–103. https://doi.org/10.21608/avmj.2019.168762 doi: 10.21608/avmj.2019.168762
![]() |
[51] | ECDC/EFSA/EMA (2015) ECDC/EFSA/EMA (European Centre for Disease Prevention and Control, European Food Safety Authority and European Medicines Agency) (2015) First joint report on the integrated analysis of the consumption of antimicrobial agents and occurrence of antimicrobial resistance in bacteria from humans and food-producing animals. EFSA J13: 4006. https://doi.org/10.2903/j.efsa.2015.4006 |
[52] |
Habib I, Mohteshamuddin K, Mohamed M-YI, et al. (2023) Domestic pets in the United Arab Emirates as reservoirs for antibiotic-resistant bacteria: A comprehensive analysis of extended-spectrum beta-lactamase producing Escherichia coli prevalence and risk factors. Animals 13: 1587. https://doi.org/10.3390/ani13101587 doi: 10.3390/ani13101587
![]() |
[53] |
Djomgoue NG, Fonbah LJ, Mbulli AI, et al. (2023) Risk factors and associated outcomes of virulence genes eae, entB, and pipD carriage in Escherichia coli, Klebsiella pneumoniae, and Salmonella spp. from HIV-1 and HIV-negative gastroenteritis patients in the Dschang Regional Hospital Annex. Cureus 15: e42329. https://doi.org/10.7759/cureus.42329 doi: 10.7759/cureus.42329
![]() |
[54] | Abdellaoui L, Bouayad L, Bensefia SA, et al. (2020) Serotyping and antibiotic sensitivity of Listeria monocytogenes isolated from cheeses produced in the region of Algiers (Algeria). Veterinaria 69: 43–49. |
[55] |
Hamdi TM, Naïm M, Martin P, et al. (2007) Identification and molecular characterization of Listeria monocytogenes isolated in raw milk in the region of Algiers (Algeria). Int J Food Microbiol 116: 190–193. https://doi.org/10.1016/j.ijfoodmicro.2006.12.038 doi: 10.1016/j.ijfoodmicro.2006.12.038
![]() |
[56] |
Bouayad L, Hamdi T-M (2012) Prevalence of Listeria spp. in ready to eat foods (RTE) from Algiers (Algeria). Food Control 23: 397–399. https://doi.org/10.1016/j.foodcont.2011.08.006 doi: 10.1016/j.foodcont.2011.08.006
![]() |
[57] |
Zakaria AI, Sabala RF (2024) Potential public health hazards related to consumption of poultry contaminated with antibiotic resistant Listeria monocytogenes in Egypt. BMC Microbiol 24: 41. https://doi.org/10.1186/s12866-024-03183-x doi: 10.1186/s12866-024-03183-x
![]() |
[58] |
Abou-Khadra S, El-Azzouny M, EL-Sheikh S (2024) Occurrence, serodiagnosis and antimicrobial resistance of Listeria monocytogenes isolated from different types of meat products and milk. Alex J Vet Sci 80: 55. https://doi.org/10.5455/ajvs.184100 doi: 10.5455/ajvs.184100
![]() |
[59] |
Abou Elez RMM, Elsohaby I, Al-Mohammadi A-R, et al. (2023) Antibacterial and anti-biofilm activities of probiotic Lactobacillus plantarum against Listeria monocytogenes isolated from milk, chicken and pregnant women. Front Microbiol 14: 1201201. https://doi.org/10.3389/fmicb.2023.1201201 doi: 10.3389/fmicb.2023.1201201
![]() |
[60] |
Radwan M, Helmy N, Mousa M (2022) Assessment of proteomic and molecular techniques in isolation of Listeria monocytogenes in minced meat. Alex J Vet Sci 74: 31. https://doi.org/10.5455/ajvs.19829 doi: 10.5455/ajvs.19829
![]() |
[61] |
Elsayed MM, Elkenany RM, Zakaria AI, et al. (2022) Epidemiological study on Listeria monocytogenes in Egyptian dairy cattle farms' insights into genetic diversity of multi-antibiotic-resistant strains by ERIC-PCR. Environ Sci Pollut 29: 54359–54377. https://doi.org/10.1007/s11356-022-19495-2 doi: 10.1007/s11356-022-19495-2
![]() |
[62] |
Gamal B, El-Malt LM, Abdel Hameed KG, et al. (2022) Evaluation the antibacterial effect of hydroalcoholic coffee extract on L. monocytogenes isolated from milk and milk products. SVU-Int J Vet Sci 5: 38–51. https://doi.org/10.21608/svu.2022.131641.1190 doi: 10.21608/svu.2022.131641.1190
![]() |
[63] | Ahmed SA, Mostafa AHM, El-Sherbini M, et al. (2022) Assessment of microbial safety and quality of market raw milk and pasteurized milk sold in Dakahlia governorate, Egypt. J Adv Vet Res 12: 456–461. |
[64] |
Ewida RM, Hasan WS, Elfaruk MS, et al. (2022) Occurrence of Listeria spp. in soft cheese and ice cream: effect of probiotic Bifidobacterium spp. On survival of Listeria monocytogenes in soft cheese. Foods 11: 3443. https://doi.org/10.3390/foods11213443 doi: 10.3390/foods11213443
![]() |
[65] |
Mohamed HMA, Katreen KG, Abd Al-Azeem MW, et al. (2022) Molecular detection of Listeria species isolated from raw milk with special reference to virulence determinants and antimicrobial resistance in Listeria monocytogenes. J Anim Health Prod 10: 492–505. https://doi.org/10.17582/journal.jahp/2022/10.4.492.505 doi: 10.17582/journal.jahp/2022/10.4.492.505
![]() |
[66] |
Dapgh AN, Salem RL (2022) Molecular detection of Listeria monocytogenes in milk and some milk products. Int J Vet Sci 514–519. https://doi.org/10.47278/journal.ijvs/2021.128 doi: 10.47278/journal.ijvs/2021.128
![]() |
[67] |
Badawy B, Gwida M, Sadat A, et al. (2022) Prevalence and antimicrobial resistance of virulent Listeria monocytogenes and Cronobacter sakazakii in dairy cattle, the environment, and dried milk with the in vitro application of natural alternative control. Antibiotics 11: 1087. https://doi.org/10.3390/antibiotics11081087 doi: 10.3390/antibiotics11081087
![]() |
[68] |
El-Baz AH, Elsayed MM (2021) Prevalence of Listeria monocytogens in farm milk and some dairy products with comparing its sensitivity to different antibiotics and H2O2 loaded on silver nanoparticles in Dakahlia governorate, Egypt. Alex J Vet Sci 68: 71–81. https://doi.org/10.5455/ajvs.30487 doi: 10.5455/ajvs.30487
![]() |
[69] |
Saleh E, El-Boudy A, Elsayed A, et al. (2021) Molecular characterization of Listeria monocytogenes isolated from raw milk and some dairy products at local markets in Damanhour city, Egypt. Damanhour J Vet Sci 6: 1–6. https://doi.org/10.21608/djvs.2021.187849 doi: 10.21608/djvs.2021.187849
![]() |
[70] |
Hassanien A, Shaker E (2021) Virulence potential of Listeria monocytogenes recovered from Ice cream and aborted women samples in Sohag city, Egypt. Adv Anim Vet Sci 9: 2309–3331. https://doi.org/10.17582/journal.aavs/2021/9.11.1829.1837 doi: 10.17582/journal.aavs/2021/9.11.1829.1837
![]() |
[71] |
Baher W, Shalaby M, Abdelghfar S (2021) Prevalence of multidrug-resistant Listeria monocytogenes in retailed goat meat and offal. Damanhour J. Vet. Sci 7: 19–22. https://doi.org/10.21608/djvs.2021.106468.1058 doi: 10.21608/djvs.2021.106468.1058
![]() |
[72] |
Harb O, Elbab G, Shawish R, et al. (2020) Genetic detection of Listeria monocytogenes recovered from fillet fish samples. Alex J Vet Sci 67: 74–79. https://doi.org/10.5455/ajvs.13697 doi: 10.5455/ajvs.13697
![]() |
[73] |
Abdel Aziz SAA, Mohamed MBED (2020) Prevalence, virulence genes, and antimicrobial resistance profile of Listeria monocytogenes isolated from retail poultry shops in Beni-Suef city, Egypt. J Adv Vet Anim Res 7: 710. https://doi.org/10.5455/javar.2020.g472 doi: 10.5455/javar.2020.g472
![]() |
[74] |
Mohamed RI, Abdelmonem MA, Amin HM (2018) Virulence and antimicrobial susceptibility profile of Listeria monocytogenes isolated from frozen vegetables available in the Egyptian market. Afr J Microbiol Res 12: 218–224. https://doi.org/10.5897/AJMR2018.8794 doi: 10.5897/AJMR2018.8794
![]() |
[75] |
Abd El-Tawab AA, Homouda SN, El-Haw SE (2018) Prevalence and molecular studies on Listeria monocytogenes isolated from chicken in El-Gharbia Governorate. Benha Vet Med J 34: 430–442. https://doi.org/10.21608/bvmj.2018.54506 doi: 10.21608/bvmj.2018.54506
![]() |
[76] |
Tahoun ABMB, Abou Elez RMM, Abdelfatah EN, et al. (2017) Listeria monocytogenes in raw milk, milking equipment and dairy workers: Molecular characterization and antimicrobial resistance patterns. J Glob Antimicrob Resist 10: 264–270. https://doi.org/10.1016/j.jgar.2017.07.008 doi: 10.1016/j.jgar.2017.07.008
![]() |
[77] |
Elshinawa SHH, Meshref AMS, Zeinhom MMA, et al. (2017) Incidence of Listeria species in some dairy products in Beni-Suef governorate. Assiut Vet Med J 63: 5–13. https://doi.org/10.21608/avmj.2016.169210 doi: 10.21608/avmj.2016.169210
![]() |
[78] |
Mohamed Y, Reda WW, Abdel-Moein K, et al. (2016) Prevalence and phylogenetic characterization of Listeria monocytogenes isolated from processed meat marketed in Egypt. J Genet Eng Biotechnol 14: 119–123. https://doi.org/10.1016/j.jgeb.2016.06.001 doi: 10.1016/j.jgeb.2016.06.001
![]() |
[79] | Ebaya MMA, AL-Ashmawy MAM, EL-Sokkary MM, et al. (2016) Comparative study on prevalence and antimicrobial susceptibility of Escherichia coli and Listeria monocytogenes isolated from raw milk in Egypt. New Egypt J Microbiol 43: 145–158. |
[80] |
Meshref AM, Zeinhom MM, Abdel-Atty NS (2015) Occurrence and distribution of Listeria species in some Egyptian foods. Alex J Vet Sci 46: 42–47. https://doi.org/10.5455/ajvs.187647 doi: 10.5455/ajvs.187647
![]() |
[81] |
Shaker EM, Hassanien AA (2015) PCR technique for detection of some virulence associated genes in Listeria monocytogenes isolated from table eggs and clinical human samples. Assiut Vet Med J 61: 219–225. https://doi.org/10.21608/avmj.2015.170040 doi: 10.21608/avmj.2015.170040
![]() |
[82] |
Awadallah MAI, Suelam ⅡA (2014) Characterization of virulent Listeria monocytogenes isolates recovered from ready-to-eat meat products and consumers in Cairo, Egypt. Vet World 7: 788–793. https://doi.org/10.14202/vetworld.2014.788-793 doi: 10.14202/vetworld.2014.788-793
![]() |
[83] | Al-Ashmawy MAM, Abdelgalil MMGKH (2014) Prevalence, detection methods and antimicrobial susceptibility of Listeria monocytogens isolated from milk and soft cheeses and its zoonotic importance. World Appl Sci J 29: 869–878. |
[84] |
Ismaiel AA-R, Ali AE-S, Enan G (2014) Incidence of Listeria in Egyptian meat and dairy samples. Food Sci Biotechnol 23: 179–185. https://doi.org/10.1007/s10068-014-0024-5 doi: 10.1007/s10068-014-0024-5
![]() |
[85] |
Abdellrazeq G, Kamar A, ElHoushy S (2014) Molecular characterization of Listeria species isolated from frozen fish. Alex J Vet Sci 40: 1. https://doi.org/10.5455/ajvs.45443 doi: 10.5455/ajvs.45443
![]() |
[86] | Edris AM, Amany M.S, Fouad MA (2014) Incidence of Listeria monocytogenes in fresh Tilapia Nilotica fish. Benha Vet Med J 26: 120–126. |
[87] |
El-Shenawy M, El-Shenawy M, Mañes J, et al. (2011) Listeria spp. in street-vended ready-to-eat foods. Interdiscip Perspect Infect Dis 2011: 1–6. https://doi.org/10.1155/2011/968031 doi: 10.1155/2011/968031
![]() |
[88] |
ElMalek A, Ali S, Hassanein R, et al. (2010) Occurrence of Listeria species in meat, chicken products and human stools in Assiut city, Egypt with PCR use for rapid identification of Listeria monocytogenes. Vet World 3: 353–359. https://doi.org/10.5455/vetworld.2010.353-359 doi: 10.5455/vetworld.2010.353-359
![]() |
[89] | Sayed M, Abdel-Azeem M, Farghaly M, et al. (2009) Using of PCR assay for identification of Listeria monocytogenes recovered from table eggs. Vet World 2: 453–455. |
[90] | Hesham TN, Hanan LE, Fathi AT, et al. (2017) Prevalence of Listeria spp. among Dairy, Meat and their Products Marketed in Tripoli, Libya. Int J Life Sci Res 5: 19–25. |
[91] |
El-Sharef N, Ghenghesh KS, Abognah YS, et al. (2006) Bacteriological quality of ice cream in Tripoli-Libya. Food Control 17: 637–641. https://doi.org/10.1016/j.foodcont.2005.04.001 doi: 10.1016/j.foodcont.2005.04.001
![]() |
[92] |
Bouymajane A, Rhazi Filali F, Oulghazi S, et al. (2021) Occurrence, antimicrobial resistance, serotyping and virulence genes of Listeria monocytogenes isolated from foods. Heliyon 7: e06169. https://doi.org/10.1016/j.heliyon.2021.e06169 doi: 10.1016/j.heliyon.2021.e06169
![]() |
[93] |
Boukili M, Rhazi Filali F, Lafkih N, et al. (2020) Prevalence, characterization and antimicrobial resistance of Listeria monocytogenes isolated from beef meat in Meknes city, Morocco. Germs 10: 74–80. https://doi.org/10.18683/germs.2020.1180 doi: 10.18683/germs.2020.1180
![]() |
[94] |
Amajoud N, Leclercq A, Soriano JM, et al. (2018) Prevalence of Listeria spp. and characterization of Listeria monocytogenes isolated from food products in Tetouan, Morocco. Food Control 84: 436–441. https://doi.org/10.1016/j.foodcont.2017.08.023 doi: 10.1016/j.foodcont.2017.08.023
![]() |
[95] |
Marnissi E, Bennani L, Cohen N, et al. (2013) Presence of Listeria monocytogenes in raw milk and traditional dairy products marketed in the north-central region of Morocco. Afr J Food Sci 7: 87–91. https://doi.org/10.5897/AJFS2013.0992 doi: 10.5897/AJFS2013.0992
![]() |
[96] |
Ennaji H, Timinouni M, Ennaji MM, et al. (2008) Characterization and antibiotic susceptibility of Listeria monocytogenes isolated from poultry and red meat in Morocco. Infect Drug Resist 1: 45–50. https://doi.org/10.2147/IDR.S3632 doi: 10.2147/IDR.S3632
![]() |
[97] |
Hmaïed F, Helel S, Le berre V, et al. (2014) Prevalence, identification by a DNA microarray-based assay of human and food isolates Listeria spp. from Tunisia. Pathologie Biologie 62: 24–29. https://doi.org/10.1016/j.patbio.2013.10.005 doi: 10.1016/j.patbio.2013.10.005
![]() |
[98] |
Mohamed M-YI, Abu J, Zakaria Z, et al. (2022) Multi-drug resistant pathogenic Escherichia coli isolated from wild birds, chicken, and the environment in Malaysia. Antibiotics 11: 1275. https://doi.org/10.3390/antibiotics11101275 doi: 10.3390/antibiotics11101275
![]() |
[99] |
Lakicevic BZ, Den Besten HMW, De Biase D (2022) Landscape of stress response and virulence genes among Listeria monocytogenes strains. Front Microbiol 12: 738470. https://doi.org/10.3389/fmicb.2021.738470 doi: 10.3389/fmicb.2021.738470
![]() |
[100] | Alía A, Martín I, Andrade MJ, et al. (2024) Chapter 20—Listeria monocytogenes cell-to-cell spread. In: Tang Y, Hindiyeh M, Liu D, et al. (Eds.), Molecular Medical Microbiology, Elsevier, 391–406. https://doi.org/10.1016/B978-0-12-818619-0.00017-4 |
[101] |
Ireton K, Mortuza R, Gyanwali GC, et al. (2021) Role of internalin proteins in the pathogenesis of Listeria monocytogenes. Mol Microbiol 116: 1407–1419. https://doi.org/10.1111/mmi.14836 doi: 10.1111/mmi.14836
![]() |
[102] |
Cheng C, Sun J, Yu H, et al. (2020) Listeriolysin O pore-forming activity is required for ERK1/2 phosphorylation during Listeria monocytogenes infection. Front Immunol 11: 1146. https://doi.org/10.3389/fimmu.2020.01146 doi: 10.3389/fimmu.2020.01146
![]() |
[103] |
Wang J, Cui M, Liu Y, et al. (2024) The mitochondrial carboxylase PCCA interacts with Listeria monocytogenes phospholipase PlcB to modulate bacterial survival. Appl Environ Microbiol 90: No.6. https://doi.org/10.1128/aem.02135-23 doi: 10.1128/aem.02135-23
![]() |
[104] |
Vazquez-Armenta FJ, Hernandez-Oñate MA, Martinez-Tellez MA, et al. (2020) Quercetin repressed the stress response factor (sigB) and virulence genes (prfA, actA, inlA, and inlC), lower the adhesion, and biofilm development of L. monocytogenes. Food Microbiol 87: 103377. https://doi.org/10.1016/j.fm.2019.103377 doi: 10.1016/j.fm.2019.103377
![]() |
[105] |
dos Santos PT, Thomasen RSS, Green MS, et al. (2020) Free fatty acids interfere with the DNA binding activity of the virulence regulator PrfA of Listeria monocytogenes. J Bacteriol 202: No.15. https://doi.org/10.1128/JB.00156-20 doi: 10.1128/JB.00156-20
![]() |
[106] |
Wiktorczyk-Kapischke N, Skowron K, Wałecka-Zacharska E (2023) Genomic and pathogenicity islands of Listeria monocytogenes—overview of selected aspects. Front Mol Biosci 10: 1161486. https://doi.org/10.3389/fmolb.2023.1161486 doi: 10.3389/fmolb.2023.1161486
![]() |
[107] |
Lecuit M (2020) Listeria monocytogenes, a model in infection biology. Cell Microbiol 22: 13186. https://doi.org/10.1111/cmi.13186 doi: 10.1111/cmi.13186
![]() |
[108] |
Hochnadel I, Hoenicke L, Petriv N, et al. (2022) Safety and efficacy of prophylactic and therapeutic vaccine based on live-attenuated Listeria monocytogenes in hepatobiliary cancers. Oncogene 41: 2039–2053. https://doi.org/10.1038/s41388-022-02222-z doi: 10.1038/s41388-022-02222-z
![]() |
[109] |
Edosa TT, Jo YH, Keshavarz M, et al. (2020) TmAtg6 plays an important role in anti-microbial defense against Listeria monocytogenes in the mealworm, Tenebrio Molitor. Int J Mol Sci 21: 1232. https://doi.org/10.3390/ijms21041232 doi: 10.3390/ijms21041232
![]() |
[110] |
Gergis AI, Hafez MR, Mohammed ZA, et al. (2024) Phenotypic and genotypic characterization of Listeria monocytogenes isolated from raw milk in Assiut city. Assiut Vet Med J 70: 232–243. https://doi.org/10.21608/avmj.2024.253310.1209 doi: 10.21608/avmj.2024.253310.1209
![]() |
[111] |
Sotohy SA, Elnaker YF, Omar AM, et al. (2024) Prevalence, antibiogram and molecular characterization of Listeria monocytogenes from ruminants and humans in New Valley and Beheira Governorates, Egypt. BMC Vet Res 20: 297. https://doi.org/10.1186/s12917-024-04138-0 doi: 10.1186/s12917-024-04138-0
![]() |
[112] |
Hasan AM (2023) Phenotypic and genotypic of Listeria monocytogenes isolated from raw milk in Assiut governorate. Int J Adv Sci Res Innovation 6: 92–113. https://doi.org/10.21608/ijasri.2023.246924.1013 doi: 10.21608/ijasri.2023.246924.1013
![]() |
[113] |
El-Demerdash AS, Raslan MT (2019) Molecular characterization of Listeria monocytogenes isolated from different animal-origin food Items from urban and rural areas. Adv Anim Vet Sci 7: 51–56. https://doi.org/10.17582/journal.aavs/2019/7.s2.51.56 doi: 10.17582/journal.aavs/2019/7.s2.51.56
![]() |
[114] |
Elsayed ME, Abd El-Hamid MI, El-Gedawy A, et al. (2022) New Insights into Listeria monocytogenes antimicrobial resistance, virulence attributes and their prospective correlation. Antibiotics 11: 1447. https://doi.org/10.3390/antibiotics11101447 doi: 10.3390/antibiotics11101447
![]() |
[115] |
Elbar S, Elkenany R, Elhadidy M, et al. (2020) Prevalence, virulence and antibiotic susceptibility of Listeria monocytogenes isolated from sheep. Mansoura Vet Med J 21: 48–52. https://doi.org/10.35943/mvmj.2020.21.2.207 doi: 10.35943/mvmj.2020.21.2.207
![]() |
[116] | Eldaly EA, El-Gaml AM, Elkenawy ME (2013) Incidence of Listeria monocytogenes in some slaughtered animals at Dakahlia province slaughterhouses—Egypt. Available from: https://www.researchgate.net/profile/Elsaid-Eldaly/publication/236681822_Incidence_of_Listeria_monocytogenes_in_some_slaughtered_animals_at_Dakahlia_province_slaughterhouses_-Egypt/links/00b7d518fd450f133b000000/Incidence-of-Listeria-monocytogenes-in-some-slaughtered-animals-at-Dakahlia-province-slaughterhouses-Egypt.pdf. |
[117] |
ECDC, EFSA (2022) The European Union Summary Report on Antimicrobial Resistance in zoonotic and indicator bacteria from humans, animals and food in 2019–2020. EFSA J 20: e07209. https://doi.org/10.2903/j.efsa.2022.7209 doi: 10.2903/j.efsa.2022.7209
![]() |
[118] |
Stavropoulos C, Tolentino B, Woods K, et al. (2021) Listeria rhomboencephalitis in an immunocompetent host: Treatment with trimethoprim-sulfamethoxazole and ampicillin: A case report and review of treatment options. Inf Dis Clin Pract 29: e204–e207. https://doi.org/10.1097/IPC.0000000000001024 doi: 10.1097/IPC.0000000000001024
![]() |
[119] |
Sutter JP, Kocheise L, Kempski J, et al. (2024) Gentamicin combination treatment is associated with lower mortality in patients with invasive listeriosis: A retrospective analysis. Infection 52: 1601–1606. https://doi.org/10.1007/s15010-024-02330-w doi: 10.1007/s15010-024-02330-w
![]() |
[120] |
Khalifa HO, Shikoray L, Mohamed M-YI, et al. (2024) Veterinary drug residues in the food chain as an emerging public health threat: Sources, analytical methods, health impacts, and preventive measures. Foods 13: 1629. https://doi.org/10.3390/foods13111629 doi: 10.3390/foods13111629
![]() |
[121] |
Andriyanov PA, Zhurilov PA, Liskova EA, et al. (2021) Antimicrobial resistance of Listeria monocytogenes strains isolated from humans, animals, and food products in Russia in 1950–1980, 2000–2005, and 2018–2021. Antibiotics 10: 1206. https://doi.org/10.3390/antibiotics10101206 doi: 10.3390/antibiotics10101206
![]() |
[122] |
Ermolaeva SA, Karpova TI, Andriyanov PA, et al. (2022) Distribution of antimicrobial resistance among clinical and food Listeria monocytogenes isolated in Moscow in 2019–2021. Clin Microbiol Antimicrob Chemother 24: 156–164. https://doi.org/10.36488/cmac.2022.2.156-164 doi: 10.36488/cmac.2022.2.156-164
![]() |
[123] |
Rodríguez-Villodres Á, Lepe JA, Blázquez J, et al. (2020) Effect of subinhibitory concentrations of ampicillin on Listeria monocytogenes. Enferm Infecc Microbiol Clin 38: 72–75. https://doi.org/10.1016/j.eimc.2019.03.010 doi: 10.1016/j.eimc.2019.03.010
![]() |
[124] |
Habib I, Elbediwi M, Mohamed M-YI, et al. (2023) Enumeration, antimicrobial resistance and genomic characterization of extended-spectrum β-lactamases producing Escherichia coli from supermarket chicken meat in the United Arab Emirates. Int J Food Microbiol 398: 110224. https://doi.org/10.1016/j.ijfoodmicro.2023.110224 doi: 10.1016/j.ijfoodmicro.2023.110224
![]() |
[125] |
Ibrahim MJ, Abdul-Aziz S, Bitrus AA, et al. (2018) Occurrence of multidrug resistant (MDR) Campylobacter species isolated from retail chicken meats in Selangor, Malaysia and their associated risk factors. Malays J Microbiol 14: 261–272. https://doi.org/10.21161/mjm.107717 doi: 10.21161/mjm.107717
![]() |
[126] |
El-Gohary FA, Abdel-Hafez LJM, Zakaria AI, et al. (2020) Enhanced antibacterial activity of silver nanoparticles combined with hydrogen peroxide against multidrug-resistant pathogens isolated from dairy farms and beef slaughterhouses in Egypt. Infect Drug Resist Volume 13: 3485–3499. https://doi.org/10.2147/IDR.S271261 doi: 10.2147/IDR.S271261
![]() |
[127] | Mohamed M-YI, Abdul-Aziz S, Jalila A, et al. (2019) Occurrence of antibiotic resistant Campylobacter in wild birds and poultry. Malays J Microbiol 15: 2. |
[128] |
Habib I, Mohamed MYI, Lakshmi GB, et al. (2022) Quantification of Campylobacter contamination on chicken carcasses sold in retail markets in the United Arab Emirates. Int J Food Contam 9: 9. https://doi.org/10.1186/s40550-022-00095-4 doi: 10.1186/s40550-022-00095-4
![]() |
[129] |
AbdelRahman MAA, Roshdy H, Samir AH, et al. (2020) Antibiotic resistance and extended-spectrum β-lactamase in Escherichia coli isolates from imported 1-day-old chicks, ducklings, and turkey poults. Vet World 13: 1037–1044. https://doi.org/10.14202/vetworld.2020.1037-1044 doi: 10.14202/vetworld.2020.1037-1044
![]() |
[130] |
Mohamed M-YI, Habib I, Khalifa HO (2024) Salmonella in the food chain within the Gulf Cooperation Council countries. AIMS Microbiol 10: 468–488. https://doi.org/10.3934/microbiol.2024023 doi: 10.3934/microbiol.2024023
![]() |
[131] |
Mohamed M-YI, Abu J, Abdul-Aziz S, et al. (2019) Occurrence of antibiotic resistant C. jejuni and E. coli in wild birds, chickens, environment and humans from Orang Asli Villages in Sungai Siput, Perak, Malaysia. Am J Anim Vet Sci 14: 158–169. https://doi.org/10.3844/ajavsp.2019.158.169 doi: 10.3844/ajavsp.2019.158.169
![]() |
[132] |
Habib I, Elbediwi M, Mohteshamuddin K, et al. (2023) Genomic profiling of extended-spectrum β-lactamase-producing Escherichia coli from pets in the United Arab Emirates: Unveiling colistin resistance mediated by mcr-1.1 and its probable transmission from chicken meat—A One Health perspective. J Infect Public Health 16: 163–171. https://doi.org/10.1016/j.jiph.2023.10.034 doi: 10.1016/j.jiph.2023.10.034
![]() |
1. | Masoud Saade, Samiran Ghosh, Malay Banerjee, Vitaly Volpert, Delay epidemic models determined by latency, infection, and immunity duration, 2024, 370, 00255564, 109155, 10.1016/j.mbs.2024.109155 | |
2. | Ali Moussaoui, Mohammed Meziane, On the date of the epidemic peak, 2024, 21, 1551-0018, 2835, 10.3934/mbe.2024126 | |
3. | Ali Moussaoui, Vitaly Volpert, The impact of immune cell interactions on virus quasi-species formation, 2024, 21, 1551-0018, 7530, 10.3934/mbe.2024331 | |
4. | Masoud Saade, Samiran Ghosh, Malay Banerjee, Vitaly Volpert, Dynamics of delay epidemic model with periodic transmission rate, 2025, 138, 0307904X, 115802, 10.1016/j.apm.2024.115802 | |
5. | V. Volpert, From SIR to delay models in epidemiology, 2025, 52, 15710645, 49, 10.1016/j.plrev.2024.11.018 | |
6. | Muhammad Tashfeen, Fazal Dayan, Muhammad Aziz ur Rehman, Thabet Abdeljawad, Aiman Mukheimer, Boundedness and Positivity Preserving Numerical Analysis of a Fuzzy-Parameterized Delayed Model for Foot and Mouth Disease Dynamics, 2024, 141, 1526-1506, 2527, 10.32604/cmes.2024.056269 |