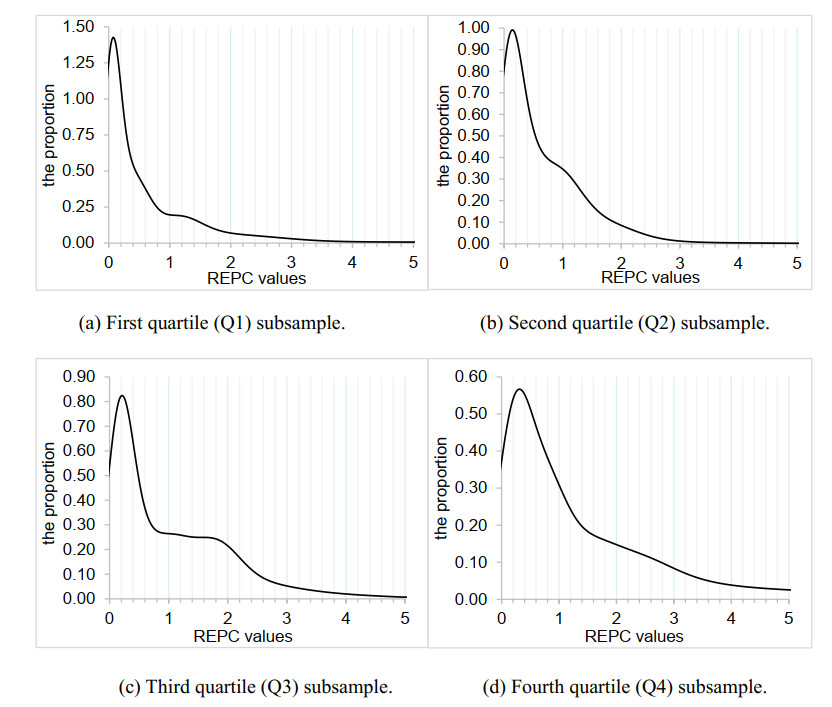
Source: Authors' calculations
We examined distribution dynamics and the long-run evolution of cross-country relative per capita carbon emissions (REPC) and intensity (RCI) vis-à-vis countries' two factors: industrial and services sectors' output. Unlike other researchers, we employed two visual tools of the distribution dynamics approach and used a panel of 217 countries. We ranked the countries based on the two factors and grouped them into four quartiles (Q1 to Q4) for each factor, resulting in eight subsamples. The results suggested long-run absolute convergence in REPC (RCI) only among highly industrialized (Q3 and Q4) countries. However, two to four convergence clubs emerged within the remaining subsamples. Besides a few (many) of the least (the most) industrialized countries converging towards the global average RCI, clubs occur at levels significantly below or above the worldwide average. The convergence was more (less) significant and towards higher (lower) REPC and RCI values for economies with low (high) industrialization. We constructed a policy priority list consisting of the least services-oriented (Q1) countries with REPC (RCI) values of 3 (7.4) and 20 (30) percent probability of further divergence from the global average in the coming years. From the perspective of climate policies aiming at reducing and converging carbon emissions, these countries require the urgent development and implementation of coordinated, bespoke policies and ongoing monitoring.
Citation: Yigang Wei, Tsun Se Cheong, Michal Wojewodzki, Xunpeng Shi. Global long-run convergence of carbon emissions and intensity vis-à-vis countries' industrial profiles[J]. Green Finance, 2024, 6(4): 612-629. doi: 10.3934/GF.2024023
[1] | Colin Nolden . Collecting silences: creating value by assetizing carbon emission mitigations and energy demand reductions. Green Finance, 2022, 4(2): 137-158. doi: 10.3934/GF.2022007 |
[2] | Tadhg O'Mahony . State of the art in carbon taxes: a review of the global conclusions. Green Finance, 2020, 2(4): 409-423. doi: 10.3934/GF.2020022 |
[3] | Jaspreet K. Sra, Annie L. Booth, Raymond A. K. Cox . Voluntary carbon information disclosures, corporate-level environmental sustainability efforts, and market value. Green Finance, 2022, 4(2): 179-206. doi: 10.3934/GF.2022009 |
[4] | Larissa M. Batrancea, Anca Nichita, Horia Tulai, Mircea-Iosif Rus, Ema Speranta Masca . Fueling economies through credit and industrial activities. A way of financing sustainable economic development in Brazil. Green Finance, 2025, 7(1): 24-39. doi: 10.3934/GF.20250002 |
[5] | Massimiliano Calvia . Fossil energy use and carbon emissions: An easy-to-implement technical policy experiment. Green Finance, 2024, 6(3): 407-429. doi: 10.3934/GF.2024016 |
[6] | Jieqiong Yang, Panzhu Luo . Review on international comparison of carbon financial market. Green Finance, 2020, 2(1): 55-74. doi: 10.3934/GF.2020004 |
[7] | Shuyang Chen . Designing the nationwide emission trading scheme in China. Green Finance, 2023, 5(3): 431-451. doi: 10.3934/GF.2023017 |
[8] | Brojeswar Pal, Anindya Mandal, Shib Sankar Sana . A closed-loop green supply chain with retailers' competition and product recycling in the green environment under the cap-and-trade policy. Green Finance, 2024, 6(1): 117-161. doi: 10.3934/GF.2024006 |
[9] | Karuna Rana, Shiv Raj Singh, Neha Saxena, Shib Sankar Sana . Growing items inventory model for carbon emission under the permissible delay in payment with partially backlogging. Green Finance, 2021, 3(2): 153-174. doi: 10.3934/GF.2021009 |
[10] | Sebastian Goers, Friedrich Schneider . Economic, ecological and social benefits through redistributing revenues from increased mineral oil taxation in Austria: A triple dividend. Green Finance, 2019, 1(4): 442-456. doi: 10.3934/GF.2019.4.442 |
We examined distribution dynamics and the long-run evolution of cross-country relative per capita carbon emissions (REPC) and intensity (RCI) vis-à-vis countries' two factors: industrial and services sectors' output. Unlike other researchers, we employed two visual tools of the distribution dynamics approach and used a panel of 217 countries. We ranked the countries based on the two factors and grouped them into four quartiles (Q1 to Q4) for each factor, resulting in eight subsamples. The results suggested long-run absolute convergence in REPC (RCI) only among highly industrialized (Q3 and Q4) countries. However, two to four convergence clubs emerged within the remaining subsamples. Besides a few (many) of the least (the most) industrialized countries converging towards the global average RCI, clubs occur at levels significantly below or above the worldwide average. The convergence was more (less) significant and towards higher (lower) REPC and RCI values for economies with low (high) industrialization. We constructed a policy priority list consisting of the least services-oriented (Q1) countries with REPC (RCI) values of 3 (7.4) and 20 (30) percent probability of further divergence from the global average in the coming years. From the perspective of climate policies aiming at reducing and converging carbon emissions, these countries require the urgent development and implementation of coordinated, bespoke policies and ongoing monitoring.
Anthropogenic emissions of greenhouse gases (hereafter GHG) have significantly impacted global climate, with carbon dioxide being the main culprit. Since the Framework Convention on Climate Change in 1992, the international community has been negotiating commitments to reduce the emissions building up in the atmosphere (Liu et al., 2023). One of the critical issues has been the burden-sharing of the global mitigation effort between countries, with the debate on global climate policies heavily focused on economic costs, fairness, and feasibility of the proposed mitigation plans (Shahrour et al., 2023; Wojewodzki et al., 2023).
Clear distribution rules have not governed the allocation of emissions obligations among countries, and it is unlikely to have a universally accepted aggregated allocation principle due to the complicity of international climate governance (European Parliament, 2023). Instead, it is the function of several factors such as energy mix, economic development, climate, land use patterns, and their interactions (Aldy, 2006). Furthermore, in international negotiations on climate change, different allocation principles explicitly related to national characteristics are increasingly proposed as justifications for why other countries should reduce or increase their energy consumption (Ciscar et al., 2013). One of the most popular ways is to share the carbon mitigation burden based on per capita emissions allocation schemes endorsed by some countries and non-governmental organizations on equity grounds (Mattoo and Subramanian, 2012). Moreover, if countries' emissions show evidence of a convergent trend, per capita targets may represent a more acceptable basis for political compromise than absolute levels, especially from the perspective of high emitters (Wojewodzki et al., 2023). Another popular allocation scheme method (especially among developing countries such as China and India) is based on carbon intensity, i.e., emissions per unit of the country's GDP (Bhattacharya et al., 2020). Some studies show that for the same country or region, per capita emissions and carbon intensity could be on divergent paths (e.g., Parker and Bhatti, 2020).
Different industrial structures can effectively reflect a country's degree of economic development, which is closely related to its technological means and ability to pay for a reduction in emissions. Generally speaking, the higher the services industry share in GDP, the faster the economic development. Moreover, compared to heavy industries, the services sector is considered more environmentally friendly because it generally involves less material production and fossil fuel combustion (e.g., Hou et al., 2021). Therefore, one could expect countries that rely more on the industrial sector and countries with the worst performance in the service sector to generate more carbon emissions.
Sizeable literature has investigated the relationship between industrial structure and energy consumption or CO2 emissions, as well as the convergence characteristics. However, most researchers focus on the individual country, e.g., China (Wu et al., 2021), while much smaller research output has taken a cross-country or global perspective (Bhattacharya et al., 2020). Furthermore, the results are inconclusive even with the same examination country and method. For example, Zhou et al. (2013) used the GMM dynamic model. They found a reducing effect of the ratio of services industry value added to provincial GDP (a proxy of upgrading the industrial structure) on carbon intensity in China. Contrary to Zhou et al. (2013), Zhang et al. (2020) documented that upgrading the industrial structure has no impact on emission intensity. In contrast, Wu et al. (2021) showed a significantly positive relationship between emissions and energy-dependent industrial upgrades in China. Rahman and Kashem (2017) used the ARDL approach and reported long- and short-run cointegration between the growth of industrial production and per capita carbon emissions in Bangladesh. They also found a positive relationship and causality between industrial development and emissions. Notwithstanding, using the same method, Abokyi et al. (2019) unveiled a significant U-shaped association between growth in the industry value added and emissions in Ghana's long- and short-run study.
Concerning convergence studies, Wang and Zhang (2014) examined beta (β), sigma (σ), and stochastic convergence of per capita CO2 emissions across six Chinese sectors (industry, transportation, storage, postal, wholesale, retail, trade, catering services, and telecommunications services). They found evidence of σ and stochastic convergence in all sectors. Yu et al. (2018) documented conditional β-convergence and the emergence of three convergence clubs in carbon intensity across China's 24 industrial sectors. Moutinho et al. (2014) explored emissions patterns in Portugal's 21 high-emitting industrial sectors and reported the presence of σ-convergence. In the US-focused study of per capita emissions, Apergis and Payne (2017) found evidence of "multiple convergence clubs in the aggregate, by sector (residential, commercial, industrial, transport, and electric power)" (p.365).
Few studies take an international perspective to examine the association between industrialization and CO2 emissions. Acheampong and Boateng (2019) estimated the effect of industrial value-added on emissions intensity in a panel of five countries: Australia, Brazil, China, India, and the US. They also documented that the impact of industrial structure varies among examined countries in magnitude and sign. When ranked from the largest to the smallest, Acheampong and Boateng (2019) found a negative (positive) effect in India, Brazil, Australia, and the US (China). Li and Lin (2015) applied the STIRPAT model to a sample of 73 countries and showed that industrialization significantly boosts emissions only in the lower-middle- and high-income countries. Wang and Wang (2021) examined the relationship between industrial structure, urbanization, and carbon emissions in a global study of 137 countries. Like Li and Lin (2015), the authors confirmed a positive association between industrial structure and emissions only among high-income countries. However, Wang and Wang (2021) found negative (inverted U-shaped) correlations across a group of upper-middle- (low-income) economies.
Under the concept of equal distribution, many researchers have studied the convergence of per capita CO2 emissions worldwide (e.g., Nguyen Van, 2005; Westerlund and Basher, 2008; Camarero et al., 2013). In comparison, the convergent patterns of carbon intensity received relatively little attention (Payne, 2020). Additionally, the convergence of cross-country carbon emissions vis-à-vis countries' industrial structures has received scant attention. In a study of per capita emissions across 21 EU member states and Turkey, Jobert et al. (2010) documented a significantly positive effect of industry structure on the speed of convergence in 101 countries using the Bayesian shrinkage estimation technique. Bhattacharya et al. (2020) reported multiple convergence clubs for territory- and consumption-based CO2 emissions intensity in a panel of 70 countries. Furthermore, they find that growth in industry value added decreases the probability of a country belonging to a low-carbon intensity club.
1 The countries with a significant role of industrialization are as follows: Bulgaria, Czechia, Germany, Hungary, Ireland, Poland, Portugal, Romania, Sweden and Turkey. It is worth mentioning that most of these countries encountered a comparatively slow pace of convergence in carbon emissions (Jobert et al., 2010).
The above-outlined mixed results and limited international studies on the CO2 emissions-industrialization nexus highlight a gap in the existing literature. Given the above backdrop, we aim to provide a new perspective on the countries' industrial profiles vis-à-vis transitional dynamics and the long-run convergence patterns in relative per capita carbon emissions (hereafter REPC) and intensity (hereafter RCI). To do that, we rank 217 countries in our global sample based on their (1) annual industrial value added to the GDP ratio and (2) annual services value added to the GDP ratio and grouped them into four quartiles (Q1 to Q4) for each factor, resulting in eight subsamples. Consequently, each quartile (subsample) represents a group of countries with similar industrial or service contributions to GDP. The first (Q1) and fourth (Q4) quartile subsamples based on industrial and services output denote countries with the lowest and highest 25 percent of values for a given factor, respectively. The third (Q3) and fourth (Q4) quartile subsamples represent countries with values between the 25th and 50th and between the 50th and 75th percentiles. For instance, Hong Kong, with its extremely low (high) importance of industrial (services) sectors during 2000-2016, belongs to the first, Q1 (fourth, Q4) quartile subsample. We focus on testing whether cross-country REPC and RCI levels tend to converge or diverge, i.e., whether the emissions gap is becoming larger or smaller.
The novel contributions of this study are as follows. We are the first to use two visual tools of the distribution dynamics approach (hereafter DDA) to unveil global cross-country transitional dynamics and long-run convergence of carbon emissions (and intensity) vis-à-vis countries' industrial profiles2. This, in turn, can help the policymakers monitor and implement appropriate and bespoke environmental policies targeting individual constituents from the list. Additionally, by providing a long-run global perspective on convergence patterns of two primary emissions measures across countries with different industrial structures, we offer assistance in developing effective carbon emission mitigation policies. For instance, countries with considerable emission reduction potential could achieve the same emissions with lower abatement costs. Thus, identifying such countries could provide a valuable reference for international climate cooperation and contribute to reducing the world's total emission costs. Furthermore, we use the mobility probability plot tool to generate a policy priority list of entities with precise (above the global average) REPC and RCI levels and significant (specific) probabilities of further increases in the coming years.
2 It is worth mentioning that the DDA provides information on the whole shape of the studied distribution, whereas traditional time series econometrics can only offer discrete forecasted values (Maasoumi et al., 2007).
The paper proceeds as follows. In the next section, we review studies on convergence in the literature focusing on industrial structure and CO2 emissions. In Section 3, we introduce the data and methodology. In Section 4, we discuss the results, and in Section 5, we present conclusions and generic policy implications.
The data employed is downloaded from the World Bank's World Development Indicators (WDI) database. We use two emission variables: (1) Relative carbon emissions per capita (REPC) and (2) relative carbon intensity (RCI). The REPC (RCI) variable is measured in metric tons of CO2 emissions per capita (kg of CO2 emissions per 2010 USD of GDP) in each country divided by the world average emissions per capita (per GDP) in this year. Using relative values (instead of absolute) is advantageous because it enables direct observation of the disparity among the countries (e.g., Williams et al., 2022). If a country has an annual REPC or RCI value of one, its per capita emissions (carbon intensity) equal the yearly global average. An annual REPC or RCI value higher and smaller than one represents a carbon emissions level greater and lower than this year's global average, respectively. Because the data for 2017 was unavailable for many countries at the time of collection, the sample period is from 2000 to 2016.
Quah (1993) developed the nonparametric DDA, which can be divided into the traditional Markov transition matrix analysis and the stochastic kernel approach. One problem with the former approach is that the demarcation of the state associated with the selection of grid values is an arbitrary process. Therefore, the latter approach is advantageous, as the demarcation process can be done objectively (Wu et al., 2016). As a result, this study employed the stochastic kernel method. The bivariate kernel estimator is illustrated below in equation (1).
(1) |
K is the normal density function, x is a variable representing the relative CO2 emissions of a country at time t, y is a variable representing the relative CO2 emissions of that country at time t+1, Xi,t is an observed value of relative CO2 emissions at time t. Moreover, Xi,t+1 is the observed value of relative CO2 emissions at time t+1. Furthermore, n is the number of observations, with h1 and h2 representing the bandwidths. Following Silverman (1986), the adaptive kernel with flexible bandwidth is used to address the suspected sparseness of the dataset. Finally, under the assumption of the evolution being first order and time-invariant, i.e., the distribution at time t +τ depends on t but not on prior distributions, the association between the distributions at times t and t +τ is expressed in equation (2).
(2) |
is the kernel density function of relative emissions' distribution at time t, is the τ-period-ahead density function of z conditional on x, while term represents the transition probability kernel mapping the distribution between time t and t +τ. Subsequently, the ergodic density function is depicted in the following equation (3).
(3) |
is the ergodic density function with infinite τ. The ergodic distribution can be viewed as a forecast of the steady-state long-run equilibrium of REPC and RCI variables. It can be used to predict the future trend of emissions developments. The mobility probability plot (hereafter MPP) graphical tool of the DDA has been developed by Cheong and Wu (2018) to interpret the mobility probability of entities within the distribution. It can be calculated by computing , i.e., the net upward mobility probability as presented in equation (4) below.
(4) |
The MPP plots the net upward mobility probability against the relative emissions. A positive (negative) value implies that the country has a net probability of moving upwards (down) within the distribution in years to come. The MPP is conveniently expressed in percentages and ranges from -100 to 100. Consequently, it delivers precise information about the distribution dynamics of the studied variable in a user-friendly format by showing the exact net upward mobility probability for countries with different (specific) relative values. Since its inception, the MPP tool has been employed to reveal transitional dynamics of housing prices in China (Liu et al., 2022a, 2022b), cross-country information transparency (Williams et al., 2022), or the US firms' credit ratings (Lee et al., 2021).
This section discusses the graphical results of two DDA display tools: Ergodic distribution and MPP. As mentioned in Section 1, we rank 217 countries based on their (1) annual industrial value added to the GDP ratio and (2) annual services value added to the GDP ratio and group them into four quartiles (Q1 to Q4) for each factor, resulting in eight subsamples. For instance, Hong Kong, with its extremely low (high) importance of industrial (services) sectors during 2000-2016, belongs to the first, Q1 (fourth, Q4) quartile subsample3. However, Sierra Leone was characterized by meager industrial and service output throughout the sample period and, as such, belongs to the Q1 group based on both factors.
3 We wish to stress that some countries moved up or down the quartiles based on one or both factors. For example, based on the annual industrial value added to GDP ratio, China fell into the third quartile, the Q3 subsample, during the entire analysis period. However, regarding annual services output, China belongs to the Q1 subsample between 2000 and 2014 but moves up to Q2 from 2015.
Figure 1 shows the ergodic distributions4 of the REPC across countries divided into four groups based on the value added to their industrial sectors. Distributions in panels (a) to (c) are bimodal, whereas in panel (d) unimodal. Such results signify a long-run absolute convergence for the most industrialized countries but the emergence of two convergence clubs across the other three samples. This indicates that heavily industrialized nations are aligning their emissions to relatively low levels due to similar industrial processes and coordinated policies to adopt clean technologies. Furthermore, the significant peaks in panels (a), (b), (c), and (d) are at REPC values significantly below the global average value of one, i.e., around a value of 0.1, 0.2, 0.25, and 0.35. This suggests that most countries will congregate around meager REPC values in the long run in each sample. However, minor peaks occur above the global average, ranging from a REPC value of 1.15 (second quartile) to 2.7 (fourth quartile).
4 As explained in the previous section, ergodic distribution offers a glimpse into the long-run steady-state equilibrium, assuming the distribution dynamics are unchanged.
Figure 1 also indicates that the greater the degree of industrialization, the more spread the distribution. The convergence process is the most (the least) significant across the economies, with the lowest (the highest) dependence on the industrial sectors' output. Such results are at odds with those of Jobert et al. (2010), who found that industrialization has a nonsignificant (significantly positive) effect on the speed of convergence in 12 (10) European countries. This discrepancy could be due to the different estimation methods and samples (geographical and economic contexts), as our study includes a global sample of 217 countries from 2000 to 2016. In contrast, Jobert et al. focused on Europe from 1971 to 2006. Additionally, because distributions are heavily skewed to the right, the results suggest that cross-country absolute convergence to the global average is unattainable without significant changes in the distribution dynamics of the REPC. Instead, we find disturbing evidence of a future divergence in per capita carbon emissions, especially between economies with relatively high and low importance of industrial sectors.
Figure 2, presenting MPPs, suggests that only countries with extremely low emission values (far below the global mean) have a high net probability (between 10 and 50 percent) of moving upward in the distribution in future years. Such results are good news from the perspective of climate policies aiming at carbon reduction/convergence. Furthermore, starting from the graph's origin, MPPs' first intersections with the horizontal axis are at the same values as major peaks in corresponding distributions (see Figure 1). It appears that the shape of the ergodic distribution is determined by its respective MPP (annual transitions).
Figure 2 also shows that only the first quartile countries with above the global average REPC values have a small probability (up to 8 percent) of moving upward within the distribution in the following years. Additionally, the further (and above) the global mean is the current REPC, the more likely it is to move downward in years to come, reaching a net mobility probability of -100 or the so-called 'development trap' at REPC values ranging from 5.5 (third quartile countries) to 11.55 (fourth quartile countries). From the perspective of cross-country emissions' future convergence, the development trap is an encouraging piece of information. This is because whenever a country's REPC reaches a threshold value corresponding to a net mobility probability of -100, it will inevitably decline in the next period.
5 For readability, the REPC (RIC) scale on the horizontal axis in Figures 2 and 4 (6 and 8) ends at nine.
Figure 3 provides insights into the prospects of long-run convergence in REPC across countries grouped by services sectors value added. In all panels, we can observe two clubs. This, in turn, suggests that, for instance, in the Q1 group, most (some) of the countries in each of the four subsamples of countries ranked by their services sector output will converge to an extremely low (low) REPC value of 0.05 (0.85) in the long run. Similarly, the primary (minor) peaks in panels (b), (c), and (d) take a value of 0.12, 0.3, and 0.6 (1.6, 1.9, and 1.2), respectively. Thus, except for one peak in panel (c), the remaining minor peaks occur at values significantly above the global average REPC. Additionally, we can observe that the most prominent peak (1.9) appears in the first quartile distribution, whereas distributions in panels (c) and (d) have the shortest peaks (0.6). This finding implies the most (least) significant long-run convergence process in REPC across the countries characterized by the least (highly) services-oriented economic profiles.
When analyzing the MPP visual tool, special attention should be devoted to sections of MPPs located above the horizontal axis and with REPC values significantly above the global average. We aim to identify countries with worryingly high current per capita carbon emissions and unveil precise probabilities of their emissions further diverging above the global average REPC in the following years. Such information is crucial from the perspective of international environmental policies aiming at future carbon convergence and reduction. Consequently, the MPP offers the so-called "policy priority list" consisting of countries that should be monitored and, if necessary, subjected to coordinated international efforts (pragmatic policies) to prevent or reverse the forecast divergence.
In Figure 4, we can observe that the first quartile countries with REPC values from 2.2 to 3.6 have non-negative net upward mobility probabilities, which means that their relative emissions are expected to remain high or move further up in the distribution. Especially the countries with REPC of around three merit the highest place in the policy priority list due to the most significant probability (around 20 percent) of future divergence above the global average. Countries with the most upgraded industrial profiles (black plot) and REPC values ranging from 3.5 to 3.8 are characterized by the "stickiness" of their high relative emissions signified by net upward mobility probabilities equal to (or slightly above) zero. Thus, these entities also merit a place on the priority list.
In summary, Figures 3 and 4 highlight that most countries with underdeveloped service sectors tend to be low-income and have lower energy consumption and industrial activity (e.g., Sierra Leone and other African countries), resulting in lower per capita emissions. Their documented most significant convergence to extremely low REPC values reflects this as a long-term phenomenon and potential poverty trap. Notwithstanding, there are noteworthy outliers with worryingly high REPC values and positive net upward mobility probabilities on the other spectrum of the same subsample. One such country is China characterized by a high level of industrial production and a relatively low services sector. Between 2000 and 2016, China's per capita carbon emissions have followed an upward trajectory (Xiong et al., 2019). The unbalanced economic output stems from China's rapid industrialization and economic growth, prioritizing energy/carbon-intensive, heavy industries such as steel, cement, and chemical production. Our results suggest that despite efforts to promote energy conservation and bold pledges regarding cutting emissions, REPC levels are poised to remain significantly above the global average or increase further. This, in turn, highlights the need for a more balanced economic approach that includes developing the services sector and implementing effective carbon reduction strategies in the industrial sector.
Next, we discuss the distribution dynamics of the second variable: relative carbon intensity (RCI). Figure 5 indicates trimodal (bimodal) distribution representing RCI across countries with below (above) the global average of industrial sectors value added, i.e., first and second (third and fourth) quartile samples. The emergence of convergence clubs implies that absolute convergence in global RCI will not occur in the long run, assuming no changes in the transitional dynamics. Moreover, we can spot that the distribution in panel (a) is characterized by the most significant convergence process, with most countries congregating around relative emissions below the global mean (twin peaks centered around the RCI values of 0.3 and 0.6). Because the same distribution is heavily right-skewed, we can expect that in the future, few outliers with very high RCI levels will coexist with many below-average emitters.
The distribution in panel (d) delivers mixed messages. On the one hand, the prominent peak occurs near the global average RCI. This is good news and suggests that many of the most industrialized countries converge towards the international mean in the long run. On the other hand, the distribution is the most spread out, i.e., the convergence process is the least significant. By examining the four panels, it is evident that while industrialization generally leads to higher carbon intensities, there is a potential for such countries to converge towards the global average. This, in turn, makes it easier to design and implement uniform policies and regulations, such as carbon pricing mechanisms, at least across many of the most industrialized economies. Overall, such results corroborate those of Bhattacharya et al. (2020), who found that growth in industry value added decreases the probability of a country belonging to a low-carbon intensity club.
Figure 6 shows that the first and second-quartile (less industrialized) countries have greater aggregate net mobility probability in years to come compared to highly industrialized emitters. As for the policy priority list, we can conclude that the second quartile countries with the range of extreme RCI values from 7 to 9.4 are the most problematic from the future emissions convergence perspective. In particular, entities with RCI values around 7.4 have the most significant probability (up to 30 percent) of moving further up in the distribution and away from the global average. Therefore, these countries should rank the highest on the policy priority list. Two such countries will likely be Central Asian countries: Uzbekistan and Mongolia. Both belong to the Q2 subsample and have persistently very high RCIs throughout the investigation period. Both nations rely heavily on fossil fuels in their energy production and have growing industrial sectors (mining and heavy industry) often unequipped with green technologies, leading to high RCIs. Accordingly, the results suggest that these (and similar) countries must increase their efforts to reduce their carbon emissions to combat climate change by focusing on energy sector reforms, industrial policies, economic incentives, and international cooperation.
The distributions in Figure 7 are starkly different from those in Figure 3. For instance, the first (fourth) quartile distribution is characterized by the lowest (the highest) prominent peak. In contrast with Figure 3, distributions shown in panels (a) and (b) of Figure 7 are significantly more spread out than those in panels (c) and (d). Figures 3 and 7 indicate that the future convergence in REPC and RCI is the most significant across the least and the most services-oriented countries, respectively. Multiple peaks in panels (a), (b), and (d) imply the emergence of clubs in the long-run steady-state equilibrium. However, the distribution in panel (c) is unimodal, translating to an optimistic forecast of absolute long-run convergence towards an RCI value of around 0.75 among countries from the third quartile (by industrial structure upgrading) group.
Figure 8 shows that the black MPP (fourth quartile countries) follows a different pattern for most of its length compared with the other plots. Specifically, the black MPP is the most volatile except for the range of RCI values from 0.9 to 2.2 below the remaining plots. This proves that the most services-oriented countries have significantly larger aggregate net mobility probability and a greater tendency to move down in the distribution in years to come. On another positive note, the development trap for this group of countries occurs at comparatively the lowest RCI value of 3.2. On the contrary, the red MPP (the least services-oriented entities) encounters the development trap at an extreme RCI value of 13, i.e., thirteen times the global average.
Furthermore, we can observe that the Q1 countries with RCI values of around 6.5 have net upward mobility probability oscillating just below zero, i.e., a high degree of stickiness in their disturbingly high RCIs in coming years. Therefore, Figures 4 and 8 suggest that some of the nations with the most underdeveloped service sectors are the most problematic due to alarmingly high carbon emissions (intensities) in conjunction with the highest (negligible) likelihood of further divergence (convergence) towards the global mean. Such results underscore the urgent need for comprehensive, coordinated, and targeted policies focused on economic diversification and technological advancements to help these countries reduce their relative carbon emissions (per capita and dollar of GDP alike).
Using a panel of 217 countries and two graphical tools of the DDA, this study examines the role of industrialization and industrial structure upgrading in global convergence-divergence patterns and the evolution of the two popular measures of carbon emissions. The results suggest long-run absolute convergence in REPC (RCI) only among the most industrialized countries (Q3 countries by industrial structure upgrading). Two to four convergence clubs emerge in the long run in the remaining six subsamples. Furthermore, the convergence process is more (less) significant and towards higher (lower) relative emissions values for entities with lower (higher) dependence on industrial sectors. Moreover, apart from a small number (many) of the least (the most) industrialized countries converging towards the global average RCI level, the observed clubs occur at relative emissions levels significantly below or above the world average. Finally, using the MPP tool, we pinpoint countries from the least services-oriented sample (Q1) and REPC values around 3 to have high (up to 20 percent) probabilities of further diverging from the global average in the coming years. By the same token, we identify the second quartile (Q2) entities (by industrial sector value added) with RCI values around 7.4 to have the most significant probabilities (up to 30 percent) of forecast divergence. From the perspective of global climate policies aiming at reducing and converging emissions, these countries merit the highest spots in the policy priority list.
Our findings have significant implications for the environmental and energy policies of the identified outliers from the Q2 (Q1) countries with RCI (REPC) of 7.4 (3), e.g., Uzbekistan and Mongolia (China). These countries must increase their efforts to reduce carbon emissions to combat climate change by focusing on energy sector reforms, industrial policies, economic incentives, and international cooperation. This can include subsidies, tax incentives, and other supporting measures to promote R & D and adopt low-carbon technologies. Moreover, policymakers need to implement stricter environmental regulations that require industries to limit their carbon emissions and adopt cleaner technologies. Likewise, policymakers should implement national programs to improve energy efficiency, particularly in industrial processes and power generation. Furthermore, relevant governing bodies should establish and enforce standards for industrial emissions, including regular monitoring and reporting requirements, and provide training programs for industries on energy efficiency and emissions reduction practices. Moreover, there is an urgent need to increase investment (public and private) in developing renewable energy sources, such as vastly underutilized wind and solar energy potential in China, Mongolia, and Uzbekistan. Finally, instead of building new coal-fired power plants, the existing ones should be gradually phased out.
Beijing Municipal Social Science Foundation (20GLC054)
Tsun Se Cheong: Writing – review & editing, Writing – original draft, Visualization, Validation, Software, Resources, Methodology, Investigation, Formal analysis, Data curation. Yigang Wei: Writing – review & editing, Writing – original draft, Visualization, Validation, Investigation, Conceptualization. Michal Wojewodzki: Writing – review & editing, Writing – original draft, Visualization, Project administration, Validation, Formal analysis. Xunpeng Shi: Writing – review & editing, Writing – original draft, Resources, Project administration, Conceptualization, Funding acquisition.
The authors declare they have not used Artificial Intelligence (AI) tools in the creation of this article.
The authors declare no conflict of interest.
[1] |
Abokyi E, Appiah-Konadu P, Abokyi F, et al. (2019) Industrial growth and emissions of CO2 in Ghana: the role of financial development and fossil fuel consumption. Energy Rep 5: 1339–1353. https://doi.org/10.1016/j.egyr.2019.09.002 doi: 10.1016/j.egyr.2019.09.002
![]() |
[2] |
Acheampong AO, Boateng EB (2019) Modelling carbon emission intensity: application of artificial neural network. J Clean Prod 225: 833–856. https://doi.org/10.1016/j.jclepro.2019.03.352 doi: 10.1016/j.jclepro.2019.03.352
![]() |
[3] |
Apergis N, Payne JE (2017) Per capita carbon dioxide emissions across US states by sector and fossil fuel source: evidence from club convergence tests. Energy Econ 63: 365–372. https://doi.org/10.1016/j.eneco.2016.11.027 doi: 10.1016/j.eneco.2016.11.027
![]() |
[4] |
Bhattacharya M, Inekwe J, Sadorsky P (2020) Consumption-based and territory-based carbon emissions intensity: determinants and forecasting using club convergence across countries. Energy Econ 86: 104632. https://doi.org/10.1016/j.eneco.2019.104632 doi: 10.1016/j.eneco.2019.104632
![]() |
[5] |
Camarero M, Picazo-Tadeo AJ, Tamarit C (2013) Are the determinants of CO2 emissions converging among OECD countries? Econ Lett 118: 159–162. https://doi.org/10.1016/j.econlet.2012.10.009 doi: 10.1016/j.econlet.2012.10.009
![]() |
[6] |
Cheong TS, Wu Y (2018) Convergence and transitional dynamics of China's industrial output: a county-level study using a new framework of distribution dynamics analysis. China Econ Rev 48: 125–138. https://doi.org/10.1016/j.chieco.2015.11.012 doi: 10.1016/j.chieco.2015.11.012
![]() |
[7] |
Ciscar JC, Saveyn B, Soria A, et al. (2013) A comparability analysis of global burden sharing GHG reduction scenarios. Energy Policy 55: 73–81. https://doi.org/10.1016/j.enpol.2012.10.044 doi: 10.1016/j.enpol.2012.10.044
![]() |
[8] | European Parliament (2023) Interactive timeline: a guide to climate change negotiations. Available from: https://www.europarl.europa.eu/infographic/climate-negotiations-timeline/index_en.html |
[9] |
Hou H, Wang J, Yuan M, et al. (2021) Estimating the mitigation potential of the Chinese service sector using embodied carbon emissions accounting. Environ Impact Assess Rev 86: 106510. https://doi.org/10.1016/j.eiar.2020.106510 doi: 10.1016/j.eiar.2020.106510
![]() |
[10] |
Jobert T, Karanfil F, Tykhonenko A (2010) Convergence of per capita carbon dioxide emissions in the EU: legend or reality? Energy Econ 32: 1364–1373. https://doi.org/10.1016/j.eneco.2010.03.005 doi: 10.1016/j.eneco.2010.03.005
![]() |
[11] |
Lee WC, Shen J, Cheong TS, et al. (2021) Detecting conflicts of interest in credit rating changes: a distribution dynamics approach. Financ Innov 7: 45. https://doi.org/10.1186/s40854-021-00263-z doi: 10.1186/s40854-021-00263-z
![]() |
[12] |
Li K, Lin B (2015) Impacts of urbanization and industrialization on energy consumption/CO2 emissions: does the level of development matter? Renew Sustain Energy Rev 52: 1107–1122. https://doi.org/10.1016/j.rser.2015.07.185 doi: 10.1016/j.rser.2015.07.185
![]() |
[13] | Liu X, Cheong TS, Yu J, et al. (2022a) Transitional dynamics and spatial convergence of house-price-to-income ratio in urban China. Econ Bull 42: 979–989. |
[14] | Liu X, Yu J, Cheong TS, et al. (2022b) The future evolution of housing price-to-income ratio in 171 Chinese cities. Ann Econ Financ 23: 159–196. |
[15] |
Liu X, Wojewodzki M, Cai Y, et al. (2023) The dynamic relationships between carbon prices and policy uncertainties. Technol Forecast Soc Change 188: 122325. https://doi.org/10.1016/j.techfore.2023.122325 doi: 10.1016/j.techfore.2023.122325
![]() |
[16] |
Maasoumi E, Racine J, Stengos T (2007) Growth and convergence: a profile of distribution dynamics and mobility. J Econometrics 136: 483–508. https://doi.org/10.1016/j.jeconom.2005.11.012 doi: 10.1016/j.jeconom.2005.11.012
![]() |
[17] |
Mattoo A, Subramanian A (2012) Equity in climate change: an analytical review. World Dev 40: 1083–1097. https://doi.org/10.1016/j.worlddev.2011.11.007 doi: 10.1016/j.worlddev.2011.11.007
![]() |
[18] |
Moutinho V, Robaina-Alves M, Mota J (2014) Carbon dioxide emissions intensity of Portuguese industry and energy sectors: a convergence analysis and econometric approach. Renew Sust Energ Rev 40: 438–449. https://doi.org/10.1016/j.rser.2014.07.169 doi: 10.1016/j.rser.2014.07.169
![]() |
[19] |
Nguyen Van P (2005) Distribution dynamics of CO2 emissions. Environ Resour Econ 32: 495–508. https://doi.org/10.1007/s10640-005-7687-6 doi: 10.1007/s10640-005-7687-6
![]() |
[20] |
Parker S, Bhatti MI (2020) Dynamics and drivers of per capita CO2 emissions in Asia. Energy Econ 89: 104798. https://doi.org/10.1016/j.eneco.2020.104798 doi: 10.1016/j.eneco.2020.104798
![]() |
[21] |
Payne JE (2020) The convergence of carbon dioxide emissions: a survey of the empirical literature. J Econ Stud 47: 1757–1785. https://doi.org/10.1108/JES-12-2019-0548 doi: 10.1108/JES-12-2019-0548
![]() |
[22] | Quah D (1993) Galton's fallacy and tests of the convergence hypothesis. Scand J Econ 95: 427–443. |
[23] |
Rahman MM, Kashem MA (2017) Carbon emissions, energy consumption and industrial growth in Bangladesh: empirical evidence from ARDL cointegration and Granger causality analysis. Energy Policy 110: 600–608. https://doi.org/10.1016/j.enpol.2017.09.006 doi: 10.1016/j.enpol.2017.09.006
![]() |
[24] |
Shahrour MH, Arouri M, Lemand R (2023) On the foundations of firm climate risk exposure. Rev Account Financ 22: 620–635. https://doi.org/10.1108/RAF-05-2023-0163 doi: 10.1108/RAF-05-2023-0163
![]() |
[25] | Silverman BW (1986) Density Estimation for Statistics and Data Analysis. Chapman and Hall, New York, NY. |
[26] |
Wang Q, Wang L (2021) The nonlinear effects of population aging, industrial structure, and urbanization on carbon emissions: a panel threshold regression analysis of 137 countries. J Clean Prod 287: 125381. https://doi.org/10.1016/j.jclepro.2020.125381 doi: 10.1016/j.jclepro.2020.125381
![]() |
[27] |
Wang J, Zhang K (2014) Convergence of carbon dioxide emissions in different sectors in China. Energy 65: 605–611. https://doi.org/10.1016/j.energy.2013.11.015 doi: 10.1016/j.energy.2013.11.015
![]() |
[28] |
Westerlund J, Basher SA (2008) Testing for convergence in carbon dioxide emissions using a century of panel data. Environ Resour Econ 40: 109–120. https://doi.org/10.1007/s10640-007-9143-2 doi: 10.1007/s10640-007-9143-2
![]() |
[29] |
Williams AD, Cheong TS, Wojewodzki M (2022) Transitional dynamics and the evolution of information transparency: a global analysis. Estud Econ 49: 31–62. https://doi.org/10.4067/S0718-52862022000100031 doi: 10.4067/S0718-52862022000100031
![]() |
[30] |
Wojewodzki M, Wei Y, Cheong TS, et al. (2023) Urbanisation, agriculture and convergence of carbon emissions nexus: global distribution dynamics analysis. J Clean Prod 385: 135697. https://doi.org/10.1016/j.jclepro.2022.135697 doi: 10.1016/j.jclepro.2022.135697
![]() |
[31] |
Wu L, Sun L, Qi P, et al. (2021) Energy endowment, industrial structure upgrading, and CO2 emissions in China: revisiting resource curse in the context of carbon emissions. Resour Policy 74: 102329. https://doi.org/10.1016/j.resourpol.2021.102329 doi: 10.1016/j.resourpol.2021.102329
![]() |
[32] |
Wu J, Wu Y, Guo X, et al. (2016) Convergence of carbon dioxide emissions in Chinese cities: a continuous dynamic distribution approach. Energy Policy 91: 207–219. https://doi.org/10.1016/j.enpol.2015.12.028 doi: 10.1016/j.enpol.2015.12.028
![]() |
[33] |
Xiong W, Liu Z, Wang S, et al. (2020) Visualizing the evolution of per capita carbon emissions of Chinese cities, 2001–2016. Environ Plann A: Econ Space 52: 702–706. https://doi.org/10.1177/0308518X19881665 doi: 10.1177/0308518X19881665
![]() |
[34] |
Yu S, Hu X, Fan JL, et al. (2018) Convergence of carbon emissions intensity across Chinese industrial sectors. J Clean Prod 194: 179–192. https://doi.org/10.1016/j.jclepro.2018.05.121 doi: 10.1016/j.jclepro.2018.05.121
![]() |
[35] |
Zhang F, Deng X, Phillips F, et al. (2020) Impacts of industrial structure and technical progress on carbon emission intensity: evidence from 281 cities in China. Technol Forecast Soc Change 154: 119949. https://doi.org/10.1016/j.techfore.2020.119949 doi: 10.1016/j.techfore.2020.119949
![]() |
[36] |
Zhou X, Zhang J, Li J (2013) Industrial structural transformation and carbon dioxide emissions in China. Energy Policy 57: 43–51. https://doi.org/10.1016/j.enpol.2012.07.017 doi: 10.1016/j.enpol.2012.07.017
![]() |