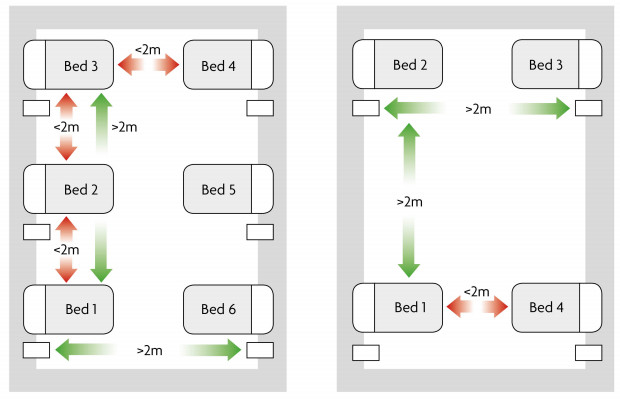
Fermented alcoholic beverages and their by-products, including beer and breweries' bio-wastes like spent yeasts, grain, and hops, contain a plethora of natural bioactive compounds that have recently gained attention for their valorization as functional ingredients in several novel foods and nutraceuticals, as well as in drugs and cosmetics applications. Within this article, the natural bio-functional compounds of fermented beer product and breweries' by-products with anti-inflammatory, antithrombotic, and anti-oxidant bioactivities are thoroughly reviewed. The important roles of yeasts involved for such bioactives to be present in the fermented product and in the brewery bio-wastes are also outlined. The health promoting benefits of beer moderate consumption resulting from these bioactives, as part of a balanced diet, against inflammation-related chronic disorders is also discussed, along with the detrimental effects of beer consumption abuse and the potential benefits of alternative non-alcoholic beers. The mechanisms of action and synergism of the natural bioactives present in the fermented beer product and in breweries' by-products, with anti-inflammatory, anti-thrombotic, and antioxidant properties are also presented. Current research and future perspectives on valorizing bioactives of fermented beer and brewery by-products, such as spent yeasts, grain and hops in health-promoting functional foods, supplements, nutraceuticals cosmetics, cosmeceuticals, and pharmaceuticals are also thoroughly evaluated, while the limitations of their use are also discussed.
Citation: Alexandros Tsoupras, Eirini A. Panagopoulou, George Z. Kyzas. Anti-inflammatory, antithrombotic and anti-oxidant bioactives of beer and brewery by-products, as ingredients of bio-functional foods, nutraceuticals, cosmetics, cosmeceuticals and pharmaceuticals with health promoting properties[J]. AIMS Agriculture and Food, 2024, 9(2): 568-606. doi: 10.3934/agrfood.2024032
[1] | David Moreno-Martos, Sean Foley, Benjamin Parcell, Dumitru Trucu, Raluca Eftimie . A computational investigation of COVID-19 transmission inside hospital wards and associated costs. Mathematical Biosciences and Engineering, 2022, 19(7): 6504-6522. doi: 10.3934/mbe.2022306 |
[2] | Qian Shen . Research of mortality risk prediction based on hospital admission data for COVID-19 patients. Mathematical Biosciences and Engineering, 2023, 20(3): 5333-5351. doi: 10.3934/mbe.2023247 |
[3] | Haonan Zhong, Wendi Wang . Mathematical analysis for COVID-19 resurgence in the contaminated environment. Mathematical Biosciences and Engineering, 2020, 17(6): 6909-6927. doi: 10.3934/mbe.2020357 |
[4] | Zhihao Zhang, Ting Zeng, Yijia Wang, Yinxia Su, Xianghua Tian, Guoxiang Ma, Zemin Luan, Fengjun Li . Prediction Model of hospitalization time of COVID-19 patients based on Gradient Boosted Regression Trees. Mathematical Biosciences and Engineering, 2023, 20(6): 10444-10458. doi: 10.3934/mbe.2023459 |
[5] | Sarita Bugalia, Vijay Pal Bajiya, Jai Prakash Tripathi, Ming-Tao Li, Gui-Quan Sun . Mathematical modeling of COVID-19 transmission: the roles of intervention strategies and lockdown. Mathematical Biosciences and Engineering, 2020, 17(5): 5961-5986. doi: 10.3934/mbe.2020318 |
[6] | Sarafa A. Iyaniwura, Musa Rabiu, Jummy F. David, Jude D. Kong . Assessing the impact of adherence to Non-pharmaceutical interventions and indirect transmission on the dynamics of COVID-19: a mathematical modelling study. Mathematical Biosciences and Engineering, 2021, 18(6): 8905-8932. doi: 10.3934/mbe.2021439 |
[7] | Xinmiao Rong, Liu Yang, Huidi Chu, Meng Fan . Effect of delay in diagnosis on transmission of COVID-19. Mathematical Biosciences and Engineering, 2020, 17(3): 2725-2740. doi: 10.3934/mbe.2020149 |
[8] | Jie Bai, Xiunan Wang, Jin Wang . An epidemic-economic model for COVID-19. Mathematical Biosciences and Engineering, 2022, 19(9): 9658-9696. doi: 10.3934/mbe.2022449 |
[9] | Yukun Tan, Durward Cator III, Martial Ndeffo-Mbah, Ulisses Braga-Neto . A stochastic metapopulation state-space approach to modeling and estimating COVID-19 spread. Mathematical Biosciences and Engineering, 2021, 18(6): 7685-7710. doi: 10.3934/mbe.2021381 |
[10] | Ziqiang Cheng, Jin Wang . Modeling epidemic flow with fluid dynamics. Mathematical Biosciences and Engineering, 2022, 19(8): 8334-8360. doi: 10.3934/mbe.2022388 |
Fermented alcoholic beverages and their by-products, including beer and breweries' bio-wastes like spent yeasts, grain, and hops, contain a plethora of natural bioactive compounds that have recently gained attention for their valorization as functional ingredients in several novel foods and nutraceuticals, as well as in drugs and cosmetics applications. Within this article, the natural bio-functional compounds of fermented beer product and breweries' by-products with anti-inflammatory, antithrombotic, and anti-oxidant bioactivities are thoroughly reviewed. The important roles of yeasts involved for such bioactives to be present in the fermented product and in the brewery bio-wastes are also outlined. The health promoting benefits of beer moderate consumption resulting from these bioactives, as part of a balanced diet, against inflammation-related chronic disorders is also discussed, along with the detrimental effects of beer consumption abuse and the potential benefits of alternative non-alcoholic beers. The mechanisms of action and synergism of the natural bioactives present in the fermented beer product and in breweries' by-products, with anti-inflammatory, anti-thrombotic, and antioxidant properties are also presented. Current research and future perspectives on valorizing bioactives of fermented beer and brewery by-products, such as spent yeasts, grain and hops in health-promoting functional foods, supplements, nutraceuticals cosmetics, cosmeceuticals, and pharmaceuticals are also thoroughly evaluated, while the limitations of their use are also discussed.
There can be high mortality and morbidity for patients due to the spread of infections in hospitals (i.e., nosocomial or HAI) from infected patients to healthy patients, either directly through sharing the same rooms and environment, or indirectly through healthcare workers (HCWs) attending infected and susceptible patients. The novel coronavirus disease (COVID-19) that has been reported since January 2020, was shown to cause a relatively high prevalence of nosocomial infections: between 12.5−15% in the UK [1,2] and up to 44% in China [3]. In comparison, other coronaviruses such as SARS (Severe Acute Respiratory Syndrome, 2003) and MERS (Middle East Respiratory Syndrome, 2012), had estimated nosocomial infection prevalence of 36% and 56%, respectively [1].
In UK hospitals, patients symptoms and results of real-time polymerase chain reaction (RT-PCR) inform targeted infection prevention and control (IPC) measures [1,2]. Patients who do not meet the clinical criteria for the COVID-19 disease may not be tested, and are usually admitted into shared bays, i.e., rooms of up to 6 beds that usually share the same bathroom facilities [2]. There can be problems with this approach, as highlighted in a recent study [2] in which it was shown that more than half of the patients who acquired COVID-19 in a hospital setting were identified as having been in the same hospital bay with other infected patients.
There is also the issue of asymptomatic cases, which pose a particular risk for healthcare transmission. Various studies in the literature have estimated the proportion of asymptomatic COVID-19 infections: from 17.9% asymptomatic passengers on board of the Princess Cruises ship [4], to 30.8% asymptomatic Japanese nationals evacuated from Wuhan [5], 50–75% asymptomatic residents of an Italian village (Vo'Euganeo) [6], and even 81% asymptomatic passengers of an un-named cruise ship [7]. Since asymptomatic infections cannot be recognised if they are not confirmed by laboratory tests [5], it is highly likely that asymptomatic patients are found in hospitals, and they can be involved in the spread of COVID-19 inside these hospitals. In fact, a study in Beijing [8] showed that 5% of hospitalised patients were asymptomatic. This complicates the issue of nosocomial transmission of COVID-19, since hospitals do not test/re-test routinely all patients hospitalised for various interventions (not COVID-19 related).
In regard to nosocomial transmissions, a 2015 report by the National Institute for Health Research [9] concluded that while hospital patients preferred a single-room accommodation, staff preferred a mix of single-rooms and shared-rooms accommodation. Moreover, a study examining hospital design [9] mentioned that it was impossible to perform an analysis of the impact of single-room hospital design on the transmission of HAIs, and given that some of the studies they cited showed a decrease in infections while other studies showed an increase in infections, the authors concluded that there was no clear evidence of the impact of single rooms on HAIs. Since some of the published COVID-19 studies [2] emphasised that ≈55% of HAIs were connected with infected patients in shared bays, it is important to understand COVID-19 transmission in these hospital bays.
The common limitation of clinical studies that focus on the spread of COVID-19 inside hospitals (see, for example, [1,2,10]), is that they identify and count only PCR-symptomatic patients and not hospitalised patients that were exposed to the disease but were discharged before developing symptoms. Also, these studies cannot identify contacts between patients and staff, which might have had undiagnosed COVID-19 [2]. To address this lack of information, mathematical modelling and computational simulations are the perfect tool to test various scenarios regarding the mechanisms that can reduce the COVID-19 transmission in hospitals. Currently there are very few mathematical studies that focus on the nosocomial transmission of SARS-CoV-2 (despite the huge number of studies focused on the general viral spread through communities: e.g., from studies that focus only on symptomatic transmission [11,12,13,14,15], to studies that focus on both symptomatic and asymptomatic transmission [16,17,18,19], or transmission during the latent stages of the exposed period and during the symptomatic infectious period [14,20], transmission by various age classes [21,22], the impact of control measures such as school or workplace closures, or self-imposed and government-imposed social distancing measures [11,18,22,23,24,25]). We are aware of only one modelling study in [26], where the authors used a classical SEIR continuous model to investigate the transmission dynamics of COVID-19 in English hospitals, following interactions between patients and HCWs.
Here we present a new study that considers a mathematical modelling approach to investigate the spread of COVID-19 among patients hospitalised in 4-bed versus 6-bed bays, which represents a standard bay structure in many hospitals in the UK; see also Figure 1. To this end we consider a network model that accounts for stochastic fluctuations between interconnected individuals inside the same hospital bay, where every single bed can be occupied by a susceptible, exposed, infectious or a recovered individual. With the help of this model we investigate computationally a variety of scenarios: from variations in the recovery and incubation periods; to the position of infected individuals in specific beds inside 6-bed bays; the effect of removing infected individuals from these bays; the effect of accommodating patients in single rooms; the effect of having asymptomatic individuals; and the role of periodic testing of hospitalised patients.
Since we are interested in investigating the transmission of infectious diseases (including COVID-19) across 4-bed and 6-bed hospital bays, which are the standard bays in many UK hospitals, in the following we consider an individual-based (network) model that tracks susceptible (S; i.e., patients vulnerable to develop an infection), exposed (E; i.e., patients incubating the virus), infected (I; i.e., patients that can transmit the disease) and recovered (R) individuals in each of the 4 or 6 beds inside these hospital bays. Following the approach in [27], each individual In,t is described by a set of characteristics, In,t=[Cn,1,t,Cn,2,t,...,Cn,m,t], where n=1,...N describes the number of individuals in the hospital bay, m is the number of characteristics, and t is the time. These characteristics are as follows:
● Cn,1,t is the epidemiological class. It can be 0 (susceptible), 1 (exposed), 2 (infected) or 3 (recovered).
● Cn,2,t is the bed at which the individual is placed.
● Cn,3,t is the time since the individual acquired the virus (start of the incubation period).
● Cn,4,t is the duration of the incubation period.
● Cn,5,t is the time since the individual has become infectious (virus transmission is now possible).
● Cn,6,t is the time that takes the individual to recover once becomes infectious.
The following rules are used to update each of these states:
● Epidemiological class update:
- A susceptible individual might become exposed with probability β if he/she interacts with an infected individual. Throughout this study we assume that the infection probability β depends on the distance between the susceptible individual and the infectious one. This modelling assumption is consistent with the clinical assumption of predominant transmission of SARS-CoV-2 via respiratory droplets [28]. Some recent studies have suggested that airborne transmission may be possible in certain circumstances, however to date the authors report that there is no perfect experimental data proving or disproving airborne transmission of SARS-CoV-2 [29]. Moreover, the evidence seems inconsistent with airborne transmission especially in well-ventilated spaces [29]. For this reason, and in accordance with current public health recommendations [30], in this study we assume that a distance of at least 2 m distance should be maintained to minimise the spread of SARS-CoV-2. Thus, here we consider the infection probability β to be a fixed number that depends on the distance between beds: probability is 1 if the distance is 0, probability is almost 0 if the distance is greater than 2; see the exponential distribution in Figure 2. The distance dij between two beds i and j (see also Figure 1) is given by matrices Dk=(dij), with i,j=1..k and k∈{4,6}:
D4=(02.565.52.505.5665.502.55.562.50),D6=(01.536.35.75.51.501.55.75.55.731.505.55.76.36.35.75.501.535.75.55.71.501.55.55.76.331.50). | (2.1) |
Therefore, the probability of becoming infected following the "contact" between individuals in beds i and j (where "contact" is understood in a very loose sense) is β(dij); see also Figure 2.
We assume that there are a finite number of "contacts" within a day, and at each time step we simulate a "contact" between an infected individual and a susceptible one. Throughout this study we consider a time step Δt=0.1 days, which corresponds to an assumption of 10 contacts per day. (Note that changing the time step, i.e., the number of "contacts", impacts the speed at which the disease spreads but not the type of spread.)
- An exposed individual becomes infected once his/her incubation period is finished (i.e., Cn,3,t>Cn,4,t).
- An infected individual recovers if the time since he/she has been infected surpasses the expected duration of the infection (i.e., Cn,5,t>Cn,6,t).
● Incubation time update: If the individual is exposed, the incubation time update is increased by Δt at each iteration, until the individual becomes infectious.
● Incubation stage: We describe the duration of the incubation stage using an Erlang distribution with mean 1/σ and shape 2. Since the results obtained with this Erlang distribution are relatively similar to the results obtained with an exponential distribution (not shown here; for details see [31]), throughout this study we use only Erlang distributions.
● Infection time update: If the individual is infected, the infection time update is increased by Δt at each iteration, until the individual recovers.
● Recovery stage: Similar to the incubation stage, to describe the duration of the recovery stage we use an Erlang distribution with mean 1/γ and shape 2.
Before we show the numerical results, we needs to discuss briefly the parameter values for which these results are obtained. Even if this model can be generally applied to the spread of various infectious diseases through hospital bays, here we focus on the spread of COVID-19. Therefore, the parameter values (i.e., mean recovery period, mean incubation period) are chosen for this particular disease, COVID-19, and are summarised in Table 1.
Parameters (& units) | Description | Range | Most common values | References |
σ (day−1) | Incubation rate | 0.071−0.5 | 0.143, 0.192 | [11,12,13,14,15,16,18,19,20,22,23,24,25,32,33,34,35,36,37,38] |
1/σ (day) | Mean incubation period | 2−14 | 7, 5.2 | [11,12,13,14,15,16,18,19,20,22,23,24,25,32,33,34,35,36,37,38] |
γ (day−1) | Recovery rate | 0.055−0.621 | 0.143−0.25 | [11,12,13,14,15,16,18,19,21,22,23,24,32,33,35,36,37,39,40] |
1/γ (day) | Mean recovery / infection period | 1.61−18 | 4−7 | [11,12,13,14,15,16,18,19,21,22,23,24,32,33,35,36,37,39,40] |
For the numerical simulations presented here, we fix 1γ=7 days, 1σ=5.2 days, and we run the simulations up to Tmax=60 days. We run this network model 300 times, and we compute the mean and standard deviation of these 300 runs for individuals in each epidemiological state (i.e., susceptibles, exposed, infectives, recovered), for each of the 4-beds or 6-beds bays. We already mentioned before that the probability of infection β is given by a decaying exponential which depends on the distance between beds (see Figure 2). Finally, as initial conditions for these simulations, we assume that all patients are susceptible, with the exception of the individual placed in bed 2 (see Figure 1), who is exposed. This describes the situation where an individual, not being thought to have the disease (although they were exposed), is introduced into a hospital bay full of patients that can become infected. For simplicity, and to be able to compare the outcome of various simulations, we arbitrarily chose to place the exposed individual in bed 2. However, in Section 3.2 we discuss also what happens when we place the initial exposed individual in a different bed.
We start this numerical investigation of our network model by assuming that hospitalised patients are accommodated in shared bays with either 4 beds or 6 beds. In Figure 3 we show the baseline dynamics of this model when an infected patient is hospitalised in bed 2 of a: (a), (b) 4-bed bay; (c), (d) 6-bed bay. We observe that in a 4-bed bay the average number of exposed and infected individuals decreases towards zero faster than in a 6-bed bay (see panels (a), (c)). Moreover, we note that for a 4-bed bay, individuals placed in beds 3 and 4 have an almost zero probability of becoming infected, when the original exposed patient was placed in bed 2 (see Figure 3(b)). For a 6-bed bay, individuals placed in beds 4, 5, and 6 have an almost zero probability of becoming infected, when the original exposed patient was placed in bed 2 (see Figure 3(d)). This means that the distance between the infected bed 2 and the beds on the opposite walls is large enough to avoid the infection.
In a 4-bed bay it does not matter where we place the first exposed individual, as the disease spreads equally in all beds due to the symmetry of bed distribution. However, in a 6-bed bay, it is important where we place the exposed individual: the disease is more likely to reach all the individuals in a room if the exposed individual is placed in beds 2 or 5. Next we compare how the disease evolves depending on where we place the first exposed individual in a 6-bed bay.
In Figure 4 we assume that the first exposed individual is placed in bed 1, which is different from the case in Figure 3(b), (d) where we assumed that the first exposed individual was placed in bed 2.
We see that by placing the first exposed individual at bed 1, the disease spreads a bit slower compared to the case when this individual is placed in bed 2. More precisely, when we place him/her in bed 1, the average number of susceptible individuals decreases and reaches a steady value around t=35 days, whereas when we place him/her in bed 2 the steady value is reached around t=28 days.
The parameters that characterise the transmission of COVID-19, e.g., mean incubation period and mean recovery period, are not fixed, with various studies showing different estimations; see Table 1. Here we focus on a 6-bed bay, and investigate the impact of incubation and recovery rates, showing only results for the minimum and the maximum values of these parameters. When varying the incubation rate we fix the mean recovery period to 1/γ=7 days, and when varying the recovery rate we fix the mean incubation period to 1/σ=5.2 days. (Here we do not vary β, since this parameter is given by the distance between beds; see caption of Figure 2.)
Figure 5 shows model dynamics when we modify the recovery period. The most significant difference between the cases 1/γ=1.61 days and 1/γ=18 days is in the amplitude and shape of the infected curve. For the case where the recovery period is 18 days (Figure 5(c)) there is a time interval during which the number of infected individuals reaches a value of 2 (within one standard deviation). Since the recovery periods are larger (the mean recovery period is 18 days), there is more time in which susceptible individuals can become infected. This also affects the values of the average number of susceptible and recovered individuals. In terms of the probability of being at each epidemiological state per bed, there is a higher probability of becoming infected when the mean recovery period is 18 days (panels (d)), due to larger exposure to infected individuals.
The effects of varying the incubation period are shown in Figure 6. The most significant differences between the cases 1/σ=2 days and 1/σ=14 days can be found in the average numbers of exposed and infected individuals. When the mean incubation period is longer (Figure 6(c)), the mean value for the exposed and infected individuals reaches zero later in time. In terms of the probability of being at each epidemiological state per bed, the probability of being infected is higher for earlier times (t=10 days), when the mean incubation period is shorter (panels (c)).
Here we investigate the effect of removing from the bay every patient that is classified as infected (we remove this patient when the incubation period ends). We do this in order to reproduce the situation in which patients are transferred to separate COVID-19-only bays right at the moment they present clinical symptoms (assuming all of them are symptomatic individuals). Once we remove the infected patient, we place a new susceptible individual in the free bed. We emphasise that we modelled this out of mathematical interest (to keep the same number of patients in a bay and continue the simulations), as the standard clinical practice does not put a new person back in a bay with potential incubating patients.
Since various studies reported pre-symptomatic transmission of SARS-CoV-2 during the incubation period [41,42,43], and following the approach in some published modelling studies [14,20] which accounted for the spread of infection during the latent period, in the following we assume that exposed individuals can transmit the disease, but at a much lower rate compared to the infected ones (we consider here an overlap between exposed and pre-symptomatic cases). Since we could not find in the literature any values for the probability of transmission, in this sub-section we set the probability of infection from the exposed individuals to be 20% of the probability of infection from the infected individuals. In Figure 7 we can see the differences between 4-bed bays and 6-bed bays.
On one hand, in a 4-bed bay the disease disappears after a month. This is when we have 4 susceptible and 0 exposed in all the runs (Figure 7(a)) or the probability of being in the exposed state is zero for times greater than 30 days (Figure 7(b)). On the other hand, in a 6-bed bay the disease disappears after 50 days, which is when the probability of being in the exposed state is zero for t>50 days (Figure 7(d)). Both bays have in common the fact that the individuals across the aisle from the infected bed 2 (i.e., beds 3 and 4 in a 4-bed bay and beds 4, 5 and 6 in a 6-bed bay) do not become infected.
Until now, we have assumed that patients in a hospital bay can become infected by interacting with exposed/infected individuals from the same bay. However, sometimes they have to share bathrooms with individuals from other bays, which increases the probability of becoming infected (it has been shown that the virus can survive for several hours on different surfaces [45]). Moreover, patients interact with hospital staff (doctors, nurses, etc.), who might transmit the disease with a very low probability [44], even if they follow the preventive measures (washing their hands, changing their clothes, wearing masks, etc.).
To implement this aspect in our simulations, we note that the few studies that estimated the secondary attack rates for COVID-19 (defined as the probability that infection occurs among susceptibles, following contacts with an infectious person or infectious environment [46]) have shown wide ranges: from 0.45% in [47], to 35% in [48], and even 68.57% in [49]. The variability in the estimations for these attack rates might have been caused by the small sample sizes considered. To address this issue, a meta-analysis study focused on Taiwan patients [50] estimated a secondary attack rate for the nosocomial spread of SARS-CoV-2 between 0.73–2.93%. The authors of this study also emphasised the low attack rates that characterised the beginning of the epidemics (the data in [50] focused on cases between January and April 2020), and mentioned that higher attack rates were expected if the epidemics would continue. They also emphasised that in Taiwan the nosocomial attack rates were very low, due to staff training and increased awareness as a result of previous experiences with SARS.
Based on these estimates, for our study we assume that each individual has a probability of 5% of becoming infected each day due to interactions with hospital staff and/or sharing the bathroom.
In Figure 8 we see, for the first time in our simulations, that all patients have become infected in both 4-bed bays and 6-bed bays. Before considering interactions with staff members and sharing bathrooms, individuals at beds 3 and 4 (4-bed bay) and individuals at beds 4, 5 and 6 (6-bed bay) rarely became infected because the distance between beds was large enough to avoid the spread of the disease. However, if there are other ways of catching the disease, these patients will surely become infected. In fact, the probability of being recovered by t=60 days is 1 for all the beds in both bays.
In order to investigate the impact of disease spread following interactions with hospital staff, we now place only one individual in a single-bed room (which has an en-suite bathroom). Thus, the only way the patient can become infected is through interactions with the hospital staff. Since in this case we cannot talk about distance between beds, for the probability of infection β we assume that at the end of each day there is a fixed probability (5%) of becoming infected if the individual is susceptible.
As stated in [51,52], the probability of infection is reduced by 85% when wearing masks. Here, we assume that the probability of infection is 5% per day when staff are wearing masks. Then we assume that the probability of becoming infected when staff are not wearing masks is 90%. In Figures 9(a) and (b) we show the probability of an individual (in a 1-bed room) of being at each epidemiological state when staff are wearing masks (sub-panel (a)) or not wearing masks (sub-panel (b)). It is clear that the use of masks increases the probability of the patient remaining in the susceptible state: from 0% after 10 days when the staff are not wearing masks, to 13% after 10 days when the staff are wearing masks.
Since it has been shown that a large number of the people infected with COVID-19 do not present with symptoms [6], but they can spread the virus efficiently [53] next we investigate the effects these asymptomatic individuals have on model dynamics. To this end, we split the infected individuals into "infected asymptomatic" and "infected symptomatic". Therefore, the epidemiological class Cn,1,t for each individuals can now take the following values: 0 (susceptible), 1 (exposed), 2 (infected asymptomatic), 3 (infected symptomatic) and 4 (recovered). Moreover, after the incubation period has passed, we randomly decide if the new individual is asymptomatic or symptomatic, with a maximum probability of 80% of being asymptomatic as suggested by various studies [7,54,6]. We assume that both exposed and infected asymptomatic individuals can transmit the disease, with the exposed individuals having a lower transmission probability (i.e., 20% lower, as considered also in Sub-section 3.4). We remove the infected symptomatic individuals the moment they become infected, and we remove the infected asymptomatic individuals after they test positive.
Here, we compare the effects of running RT-PCR tests every day (Figure 10) or every 3 days (Figure 11) on hospitalised patients that have not been diagnosed with COVID-19. We see that it is better to run tests more often, as the probability of being susceptible (per bed) is higher when running tests every day (see panels (b), (d)). The results are similar for the 4-bay case and 6-bay case.
When running tests every 3 days (Figure 11), if we look at the infected asymptomatic individuals (in panels (a), (c)), sometimes we see two asymptomatic individuals in a bay. This is much higher compared to the case when tests are run every day, when the level of asymptomatics is zero (Figure 10(a), (c)). Moreover, for the case where tests are performed every 3 days, we note a periodic behaviour in the average number of individuals per time – caused by the removal of asymptomatics after they test positive.
In this study we used modelling and computational approaches to investigate the question of the spread of an infectious disease through single rooms and shared hospital bays. This is an important aspect to be addressed due to the associated hospital costs of having single rooms or shared bays, and due to the very large numbers of patients that need to be hospitalised (with or without disease) in the context of a pandemic, as the one currently caused by SARS-CoV-2.
To investigate the probability that an infectious disease would spread among patients hospitalised in single rooms and in shared hospital bays with 4 beds or 6 beds, we used a simple network model that considered the probability that each bed had a susceptible, exposed, infected (symptomatic or asymptomatic) or recovered individual. We built the model in steps, starting with the four epidemiological classes considered by the majority of early models for COVID-19 (i.e., susceptibles, exposed, infected and recovered; [14,17]). Then, we introduced asymptomatic infected individuals, to investigate their role in disease transmission across hospital bays (and how the results are different from the case where we assumed that all infected individuals are symptomatic – which was the standard assumption at the beginning of this pandemics). With the help of this model we tested a variety of scenarios: the effect of placing the initial exposed individual in different beds inside the 6-bed bay (see Figure 4); the effect of varying the recovery and incubation periods (see Figures 5 and 6); the effect of removing infected individuals from the bays (see Figure 7); the effect of interaction with members of staff and sharing bathrooms, thus increasing the daily probability of infection (see Figure 8); the role of asymptomatic individuals and the effect of frequent testing (every day or every 3 days) that can be used to identify asymptomatic individuals (see Figures 10 and 11); and finally the effect of having only 1 patient per room, in which case the probability of infection depends only on interactions with staff members (see Figure 9).
We showed that patients hospitalised in single rooms have a higher probability to remain susceptible (i.e., not become infected) compared to patients in 4-bed bays; see Figures 9(a) and 8. We also showed that periodic testing combined with removing infected individuals from the bays reduces the probability of exposure and infection; see Figure 4.
We conclude our theoretical study by emphasising that healthcare associated infectious diseases seem to spread slower in single (1-bed) rooms versus shared bays. Moreover, the COVID-19 infection spreads slower in 4-bed bays compared to 6-bed bays. This predictive result is important since a 2015 report by National Institute for Health Research on the impact of single-room accommodation on patient and staff experiences [9] concluded that there was no clear evidence of a reduction in HAIs through single rooms: while many studies showed a reduction in infection rates, other studies showed no differences or higher infection rates. In our theoretical study we have shown that, from an epidemiological point of view, there are differences in the disease transmission when there is a single patient per room, or multiple patients per room. Moreover, when there are multiple room occupancies, it is important to keep the number of patients as low as possible (e.g., 4 instead of 6), to slow down the spread of infectious diseases.
Regarding periodic testing, current guidance [58] advocates for routine testing of asymptomatic HCWs every 7 days, depending on available testing capacity. Moreover, it is suggested that elective patients are to be tested for COVID-19 no more than 72 hours before admission, and cancer patients receiving long-course treatments should be tested every week [58]. The guidance from Health Protection Scotland [56] is that all patients over 70 years of age should tested for COVID-19 on admission to hospital. Moreover, the advice is that if the test results are negative, then the patients should be tested every 4 days until they are discharged [56]. Our simulation results investigated the effects of testing every 1–3 days. However, we emphasise that patient choice would need to be taken into account when developing policies for more frequent testing, as sampling has been reported to be uncomfortable. In addition, resources for testing would need to be increased in order for this to be feasible.
Finally, since frequent RT-PCR testing may not be economically wise, laboratories certified under the Clinical Laboratory Improvement Amendments [57] may use a specimen pooling strategy, which allows laboratories to test more samples with fewer testing material and thus lower costs. This pooling strategy means combining respiratory samples from different people and conducting one laboratory test on the combined pool of samples [57].
To conclude, in this study we have shown that transmission could be reduced by reducing the number of patients per room and by carrying out more regular testing. It is likely that a number of strategies will be required to reduce the risk of COVID-19 transmission and ensure the safe delivery of healthcare.
It is a pleasure to acknowledge Wai-Lum Sung (graphic designer at the University of Aberdeen) for his assistance in the preparation of Figure 1, and Kathryn Brechin the Associate Nurse Director of Ninewells Hospital who created the first draft of Figure 1.
The authors declare no conflict of interest.
[1] |
Fahey D (2009) Old-time breweries: Academic and breweriana historians. Ohio Hist 116: 101–121. https://doi.org/10.1353/ohh.0.0062 doi: 10.1353/ohh.0.0062
![]() |
[2] |
de Gaetano G, Costanzo S, Di Castelnuovo A, et al. (2016) Effects of moderate beer consumption on health and disease: A consensus document. Nutr, Metab Cardiovasc Dis 26: 443–467. https://doi.org/10.1016/j.numecd.2016.03.007 doi: 10.1016/j.numecd.2016.03.007
![]() |
[3] |
Humia BV, Santos KS, Barbosa AM, et al. (2019) Beer molecules and its sensory and biological properties: A review. Molecules 24: 1568. https://doi.org/10.3390/molecules24081568 doi: 10.3390/molecules24081568
![]() |
[4] | Tsoupras A, Gkika DA, Voorhout A, et al. (2024) Beneficial effects of beer, brewery by-products, and their bioactives: Potential applications in novel health-promoting products in natural products in beverages. Reference Series in Phytochemistry. |
[5] | World Health Organization (2013) Health topics: Cardiovascular diseases. Available from: http://www who int/cardiovascular_diseases/en/. |
[6] |
Tsoupras AB, Iatrou C, Frangia C, et al. (2009) The implication of platelet activating factor in cancer growth and metastasis: Potent beneficial role of PAF-inhibitors and antioxidants. Infect Disord: Drug Targets 9: 390–399. https://doi.org/10.2174/187152609788922555 doi: 10.2174/187152609788922555
![]() |
[7] |
Tsoupras A, Lordan R, Zabetakis I (2018) Inflammation, not cholesterol, is a cause of chronic disease. Nutrients 10: 604. https://doi.org/10.3390/nu10050604 doi: 10.3390/nu10050604
![]() |
[8] | Tsoupras A, Moran D, Lordan R (2023) Chapter 12—Functional properties of the fermented alcoholic beverages: Apple cider and beer. 319–339. In: Zabetakis I, Lordan R, Tsoupras A (Eds.), Functional Foods and Their Implications for Health Promotion, Academic Press, 319–339. https://doi.org/10.1016/B978-0-12-823811-0.00013-4 |
[9] |
Ambra R, Pastore G, Lucchetti S (2021) The role of bioactive phenolic compounds on the impact of beer on health. Molecules 26: 486. https://doi.org/10.3390/molecules26020486 doi: 10.3390/molecules26020486
![]() |
[10] |
Tsoupras A, Ni VLJ, O'Mahony É, et al. (2023) Wine-making: "with one stone two birds"? A holistic review of the bio-functional compounds, applications and health benefits of wine and wineries' by-products. Fermentation 9: 838. https://doi.org/10.20944/preprints202306.1034.v1 doi: 10.20944/preprints202306.1034.v1
![]() |
[11] |
Tsoupras A, Lordan R, O'Keefe E, et al. (2020) Structural elucidation of Irish ale bioactive polar lipids with antithrombotic properties. Biomolecules 10: 1075. https://doi.org/10.3390/biom10071075 doi: 10.3390/biom10071075
![]() |
[12] |
Salanță LC, Coldea TE, Ignat MV, et al. (2020) Functionality of special beer processes and potential health benefits. Processes 8: 1613. https://doi.org/10.3390/pr8121613 doi: 10.3390/pr8121613
![]() |
[13] |
Tomita J, Mochizuki S, Fujimoto S, et al. (2017) Acute improvement of endothelial functions after oral ingestion of isohumulones, bitter components of beer. Biochem Biophys Res Commun 484: 740–745. https://doi.org/10.1016/j.bbrc.2017.01.133 doi: 10.1016/j.bbrc.2017.01.133
![]() |
[14] |
Arranz S, Chiva-Blanch G, Valderas-Martínez P, et al. (2012) Wine, beer, alcohol and polyphenols on cardiovascular disease and cancer. Nutrients 4: 759–781. https://doi.org/10.3390/nu4070759 doi: 10.3390/nu4070759
![]() |
[15] |
Chen X, Li Z, Hong H, et al. (2021) Xanthohumol suppresses inflammation in chondrocytes and ameliorates osteoarthritis in mice. Biomed Pharmacother 137: 111238. https://doi.org/10.1016/j.biopha.2021.111238 doi: 10.1016/j.biopha.2021.111238
![]() |
[16] |
Fukizawa S, Yamashita M, Wakabayashi K, et al. (2020) Anti-obesity effect of a hop-derived prenylflavonoid isoxanthohumol in a high-fat diet-induced obese mouse model. Biosci Microbiota, Food Health 39: 175–182. https://doi.org/10.12938/bmfh.2019-040 doi: 10.12938/bmfh.2019-040
![]() |
[17] |
Yao J, Zhang B, Ge C, et al. (2015) Xanthohumol, a polyphenol chalcone present in hops, activating Nrf2 enzymes to confer protection against oxidative damage in PC12 cells. J Agric Food Chem 63: 1521–1531. https://doi.org/10.1021/jf505075n doi: 10.1021/jf505075n
![]() |
[18] |
Yen TL, Hsu CK, Lu WJ, et al. (2012) Neuroprotective effects of xanthohumol, a prenylated flavonoid from hops (Humulus lupulus), in ischemic stroke of rats. J Agric Food Chem 60: 1937–1944. https://doi.org/10.1021/jf204909p doi: 10.1021/jf204909p
![]() |
[19] |
Lordan R, O'Keeffe E, Tsoupras A, et al. (2019) Total, neutral, and polar lipids of brewing ingredients, by-products and beer: Evaluation of antithrombotic activities. Foods 8: 171. https://doi.org/10.3390/foods8050171 doi: 10.3390/foods8050171
![]() |
[20] |
Schutte R, Papageorgiou M, Najlah M, et al. (2020) Drink types unmask the health risks associated with alcohol intake—prospective evidence from the general population. Clin Nutr 39: 3168–3174. https://doi.org/10.1016/j.clnu.2020.02.009 doi: 10.1016/j.clnu.2020.02.009
![]() |
[21] |
Schutte R, Smith L, Wannamethee G (2022) Alcohol—The myth of cardiovascular protection. Clin Nutr 41: 348–355. https://doi.org/10.1016/j.clnu.2021.12.009 doi: 10.1016/j.clnu.2021.12.009
![]() |
[22] |
Fărcaş AC, Socaci SA, Mudura E, et al. (2017) Exploitation of brewing industry wastes to produce functional ingredients. Brew Technol 137–156. https://doi.org/10.5772/intechopen.69231 doi: 10.5772/intechopen.69231
![]() |
[23] |
Rachwał K, Waśko A, Gustaw K, et al. (2020) Utilization of brewery wastes in food industry. PeerJ 8: e9427. https://doi.org/10.7717/peerj.9427 doi: 10.7717/peerj.9427
![]() |
[24] |
Boronat A, Soldevila-Domenech N, Rodríguez-Morató J, et al. (2020) Beer phenolic composition of simple phenols, prenylated flavonoids and alkylresorcinols. Molecules 25: 2582. https://doi.org/10.3390/molecules25112582 doi: 10.3390/molecules25112582
![]() |
[25] |
Bamforth C (2017) Progress in brewing science and beer production. Annu Rev Chem Biomol Eng 8: 161–176. https://doi.org/10.1146/annurev-chembioeng-060816-101450 doi: 10.1146/annurev-chembioeng-060816-101450
![]() |
[26] |
Ano Y, Dohata A, Taniguchi Y, et al. (2017) Iso-α-acids, bitter components of beer, prevent inflammation and cognitive decline induced in a mouse model of Alzheimer's disease. J Biol Chem 292: 3720–3728. https://doi.org/10.1074/jbc.M116.763813 doi: 10.1074/jbc.M116.763813
![]() |
[27] |
Karabín M, Hudcová T, Jelínek L, et al. (2016) Biologically active compounds from hops and prospects for their use. Compr Rev Food Sci Food Saf 15: 542–567. https://doi.org/10.1111/1541-4337.12201 doi: 10.1111/1541-4337.12201
![]() |
[28] |
Steenackers B, De Cooman L, De Vos D (2015) Chemical transformations of characteristic hop secondary metabolites in relation to beer properties and the brewing process: A review. Food Chem 172: 742–756. https://doi.org/10.1016/j.foodchem.2014.09.139 doi: 10.1016/j.foodchem.2014.09.139
![]() |
[29] |
Roldán-López D, Muñiz-Calvo S, Daroqui N, et al. (2022) The potential role of yeasts in the mitigation of health issues related to beer consumption. Crit Rev Food Sci Nutr 64: 3059–3074. https://doi.org/10.1080/10408398.2022.2129584 doi: 10.1080/10408398.2022.2129584
![]() |
[30] |
Merten D, Erman L, Marabelli GP, et al. (2022) Potential health effects of brewers' spent grain as a functional food ingredient assessed by markers of oxidative stress and inflammation following gastro-intestinal digestion and in a cell model of the small intestine. Food Funct 13: 5327–5342. https://doi.org/10.1039/D1FO03090F doi: 10.1039/D1FO03090F
![]() |
[31] |
Wang J, Li M, Zheng F, et al. (2018) Cell wall polysaccharides: Before and after autolysis of brewer's yeast. World J Microbiol Biotechnol 34: 137. https://doi.org/10.1007/s11274-018-2508-6 doi: 10.1007/s11274-018-2508-6
![]() |
[32] |
Lynch KM, Steffen EJ, Arendt EK (2016) Brewers' spent grain: A review with an emphasis on food and health. J Inst Brew 122: 553–568. https://doi.org/10.1002/jib.363 doi: 10.1002/jib.363
![]() |
[33] |
Mussatto SI, Dragone G, Roberto IC (2007) Ferulic and p-coumaric acids extraction by alkaline hydrolysis of brewer's spent grain. Ind Crops Prod 25: 231–237. https://doi.org/10.1016/j.indcrop.2006.11.001 doi: 10.1016/j.indcrop.2006.11.001
![]() |
[34] |
Aliyu S, Bala M (2011) Brewer's spent grain: A review of its potentials and applications. Afr J Biotechnol 10: 324–331. https://doi.org/10.5897/AJBx10.006 doi: 10.5897/AJBx10.006
![]() |
[35] |
Tarragon E, Moreno JJ (2020) Polyphenols and taste 2 receptors. Physiological, pathophysiological and pharmacological implications. Biochem Pharmacol 178: 114086. https://doi.org/10.1016/j.bcp.2020.114086 doi: 10.1016/j.bcp.2020.114086
![]() |
[36] |
Claudia Salanță L, Corina Fărcaş A, Borșa A, et al. (2023) Current strategies for the management of valuable compounds from hops waste for a circular economy. Food Chem: X 19: 100876. https://doi.org/10.1016/j.fochx.2023.100876 doi: 10.1016/j.fochx.2023.100876
![]() |
[37] |
Carvalheira M, Amorim CL, Oliveira AC, et al. (2022) Valorization of brewery waste through polyhydroxyalkanoates production supported by a metabolic specialized microbiome. Life 12: 1347. https://doi.org/10.3390/life12091347 doi: 10.3390/life12091347
![]() |
[38] | Rakowska R, Sadowska A, Dybkowska E, et al. (2017) Spent yeast as natural source of functional food additives. Roczniki Państwowego Zakładu Higieny 68: 115–121. https://pubmed.ncbi.nlm.nih.gov/28646828/ |
[39] |
Magalhães PJ, Carvalho DO, Cruz JM, et al. (2009) Fundamentals and health benefits of xanthohumol, a natural product derived from hops and beer. Nat Prod Commun 4: 591–560. https://doi.org/10.1177/1934578X0900400501 doi: 10.1177/1934578X0900400501
![]() |
[40] |
Fukuda T, Obara K, Saito J, et al. (2019) Effects of hop bitter acids, bitter components in beer, on cognition in healthy adults: A randomized controlled trial. J Agric Food Chem 68: 206–212. https://doi.org/10.1021/acs.jafc.9b06660 doi: 10.1021/acs.jafc.9b06660
![]() |
[41] |
Morimoto-Kobayashi Y, Ohara K, Ashigai H, et al. (2015) Matured hop extract reduces body fat in healthy overweight humans: A randomized, double-blind, placebo-controlled parallel group study. Nutr J 15: 25. https://doi.org/10.1186/s12937-016-0144-2 doi: 10.1186/s12937-016-0144-2
![]() |
[42] |
Nardini M (2023) An overview of bioactive phenolic molecules and antioxidant properties of beer: Emerging trends. Molecules 28: 3221. https://doi.org/10.3390/molecules28073221 doi: 10.3390/molecules28073221
![]() |
[43] |
Nicacio K de J, Ferreira MS, Katchborian‐Neto A, et al. (2022) Anti‐inflammatory markers of hops cultivars (Humulus lupulus L.) evaluated by untargeted metabolomics strategy. Chem Biodiversity 19: e202100966. https://doi.org/10.1002/cbdv.202100966 doi: 10.1002/cbdv.202100966
![]() |
[44] |
Shafreen RMB, Lakshmi SA, Pandian SK, et al. (2020) Unraveling the antioxidant, binding and health-protecting properties of phenolic compounds of beers with main human serum proteins: In vitro and in silico approaches. Molecules 25: 4962. https://doi.org/10.3390/molecules25214962 doi: 10.3390/molecules25214962
![]() |
[45] |
Vazquez-Cervantes GI, Ortega DR, Blanco Ayala T, et al. (2021) Redox and anti-inflammatory properties from hop components in beer-related to neuroprotection. Nutrients 13: 2000. https://doi.org/10.3390/nu13062000 doi: 10.3390/nu13062000
![]() |
[46] |
Neumann HF, Frank J, Venturelli S, et al. (2022) Bioavailability and cardiometabolic effects of xanthohumol: Evidence from animal and human studies. Mol Nutr Food Res 66: 2100831. https://doi.org/10.1002/mnfr.202100831 doi: 10.1002/mnfr.202100831
![]() |
[47] |
Vesaghhamedani S, Ebrahimzadeh F, Najafi E, et al. (2022) Xanthohumol: An underestimated, while potent and promising chemotherapeutic agent in cancer treatment. Prog Biophys Mol Biol 172: 3–14. https://doi.org/10.1016/j.pbiomolbio.2022.04.002 doi: 10.1016/j.pbiomolbio.2022.04.002
![]() |
[48] |
Hsieh M, Hsieh M, Lo Y, et al. (2022) Xanthohumol targets the JNK1/2 signaling pathway in apoptosis of human nasopharyngeal carcinoma cells. Environ Toxicol 37: 1509–1520. https://doi.org/10.1002/tox.23502 doi: 10.1002/tox.23502
![]() |
[49] |
Samuels JS, Shashidharamurthy R, Rayalam S (2018) Novel anti-obesity effects of beer hops compound xanthohumol: Role of AMPK signaling pathway. Nutr Metabol 15: 42. https://doi.org/10.1186/s12986-018-0277-8 doi: 10.1186/s12986-018-0277-8
![]() |
[50] |
Trius-Soler M, Tresserra-Rimbau A, Moreno JJ, et al. (2022) Effect of moderate beer consumption (with and without ethanol) on osteoporosis in early postmenopausal women: Results of a pilot parallel clinical trial. Front Nutr 9: 1014140. https://doi.org/10.3389/fnut.2022.1014140 doi: 10.3389/fnut.2022.1014140
![]() |
[51] |
Oliveira Neto JR, De Oliveira TS, Ghedini PC, et al. (2017) Antioxidant and vasodilatory activity of commercial beers. J Funct Foods 34: 130–138. https://doi.org/10.1016/j.jff.2017.04.019 doi: 10.1016/j.jff.2017.04.019
![]() |
[52] |
Ambrož M, Lněničková K, Matoušková P, et al. (2019) Antiproliferative effects of hop-derived prenylflavonoids and their influence on the efficacy of oxaliplatine, 5-fluorouracil and irinotecan in human colorectalC cells. Nutrients 11: 879. https://doi.org/10.3390/nu11040879 doi: 10.3390/nu11040879
![]() |
[53] |
Yamashita M, Fukizawa S, Nonaka Y (2020) Hop-derived prenylflavonoid isoxanthohumol suppresses insulin resistance by changing the intestinal microbiota and suppressing chronic inflammation in high fat diet-fed mice. Eur Rev Med Pharmacol Sci 24: 1537–1547. https://doi.org/10.26355/eurrev_202002_20212 doi: 10.26355/eurrev_202002_20212
![]() |
[54] |
Mahli A, Seitz T, Freese K, et al. (2019) Therapeutic application of micellar solubilized xanthohumol in a western-type diet-induced mouse model of obesity, diabetes and non-alcoholic fatty liver disease. Cells 8: 359. https://doi.org/10.3390/cells8040359 doi: 10.3390/cells8040359
![]() |
[55] |
Dorn C, Massinger S, Wuzik A, et al. (2013) Xanthohumol suppresses inflammatory response to warm ischemia–reperfusion induced liver injury. Exp Mol Pathol 94: 10–16. https://doi.org/10.1016/j.yexmp.2012.05.003 doi: 10.1016/j.yexmp.2012.05.003
![]() |
[56] |
Pinto C, Duque AL, Rodríguez-Galdón B, et al. (2012) Xanthohumol prevents carbon tetrachloride-induced acute liver injury in rats. Food Chem Toxicol 50: 3405–3412. https://doi.org/10.1016/j.fct.2012.07.035 doi: 10.1016/j.fct.2012.07.035
![]() |
[57] |
Saito K, Matsuo Y, Imafuji H, et al. (2018) Xanthohumol inhibits angiogenesis by suppressing nuclear factor‐κB activation in pancreatic cancer. Cancer Sci 109: 132–140. https://doi.org/10.1111/cas.13441 doi: 10.1111/cas.13441
![]() |
[58] |
Soldevila-Domenech N, Boronat A, Mateus J, et al. (2019) Generation of the antioxidant hydroxytyrosol from tyrosol present in beer and red wine in a randomized clinical trial. Nutrients 11: 2241. https://doi.org/10.3390/nu11092241 doi: 10.3390/nu11092241
![]() |
[59] |
Silva S, Oliveira AI, Cruz A, et al. (2022) Physicochemical properties and antioxidant activity of Portuguese craft beers and raw materials. Molecules 27: 8007. https://doi.org/10.3390/molecules27228007 doi: 10.3390/molecules27228007
![]() |
[60] |
McCarthy AL, O'Callaghan YC, Piggott CO, et al. (2013) Brewers' spent grain; bioactivity of phenolic component, its role in animal nutrition and potential for incorporation in functional foods: A review. Proc Nutr Soc 72: 117–125. https://doi.org/10.1017/S0029665112002820 doi: 10.1017/S0029665112002820
![]() |
[61] |
Olas B, Bryś M (2020) Beer components and their beneficial effect on the hemostasis and cardiovascular diseases—Truth or falsehood. Food Chem Toxicol 146: 111782. https://doi.org/10.1016/j.fct.2020.111782 doi: 10.1016/j.fct.2020.111782
![]() |
[62] |
Vlachogianni IC, Fragopoulou E, Stamatakis GM, et al. (2015) Platelet Activating Factor (PAF) biosynthesis is inhibited by phenolic compounds in U-937 cells under inflammatory conditions. Prostaglandins Other Lipid Mediators 121: 176–183. https://doi.org/10.1016/j.prostaglandins.2015.09.001 doi: 10.1016/j.prostaglandins.2015.09.001
![]() |
[63] |
Tsoupras AB, Fragopoulou E, Nomikos T, et al. (2007) Characterization of the de novo biosynthetic enzyme of platelet activating factor, DDT-insensitive cholinephosphotransferase, of human mesangial cells. Mediators Inflammation 2007: 027683. https://doi.org/10.1155/2007/27683 doi: 10.1155/2007/27683
![]() |
[64] |
Moreira MM, Morais S, Carvalho DO, et al. (2013) Brewer's spent grain from different types of malt: Evaluation of the antioxidant activity and identification of the major phenolic compounds. Food Res Int 54: 382–388. https://doi.org/10.1016/j.foodres.2013.07.023 doi: 10.1016/j.foodres.2013.07.023
![]() |
[65] |
Ikram S, Huang L, Zhang H, et al. (2017) Composition and nutrient value proposition of brewers spent grain. J Food Sci 82: 2232–2242. https://doi.org/10.1111/1750-3841.13794 doi: 10.1111/1750-3841.13794
![]() |
[66] |
Birsan RI, Wilde P, Waldron KW, et al. (2021) Anticholinesterase activities of different solvent extracts of brewer's spent grain. Foods 10: 930. https://doi.org/10.3390/foods10050930 doi: 10.3390/foods10050930
![]() |
[67] |
Hsu Y-Y, Kao T-H (2019) Evaluation of prenylflavonoids and hop bitter acids in surplus yeast. J Food Sci Technol 56: 1939–1953. https://doi.org/10.1007/s13197-019-03660-6 doi: 10.1007/s13197-019-03660-6
![]() |
[68] |
Vieira EF, Carvalho J, Pinto E, et al. (2016) Nutritive value, antioxidant activity and phenolic compounds profile of brewer's spent yeast extract. J Food Compos Anal 52: 44–51. https://doi.org/10.1016/j.jfca.2016.07.006 doi: 10.1016/j.jfca.2016.07.006
![]() |
[69] | Kao TH (2018) Health potential for beer brewing byproducts. In: Shiomi N (Ed.), Current Topics Superfoods. https://doi.org/10.5772/intechopen.76126 |
[70] |
Bonatto D (2022) The multiple roles of lipid metabolism in yeast physiology during beer fermentation. Genet Mol Biol 45: e20210325. https://doi.org/10.1590/1678-4685-GMB-2021-0325 doi: 10.1590/1678-4685-GMB-2021-0325
![]() |
[71] |
Gordon R, Power A, Chapman J, et al. (2018) A review on the source of lipids and their interactions during beer fermentation that affect beer quality. Fermentation 4: 89. https://doi.org/10.3390/fermentation4040089 doi: 10.3390/fermentation4040089
![]() |
[72] |
Lordan R, O'Keeffe E, Dowling D, et al. (2019) The in vitro antithrombotic properties of ale, lager, and stout beers. Food Biosci 28: 83–88. https://doi.org/10.1016/j.fbio.2019.01.012 doi: 10.1016/j.fbio.2019.01.012
![]() |
[73] |
Antonopoulou S, Demopoulos CA (2023) Protective effect of olive oil microconstituents in atherosclerosis: Emphasis on PAF implicated atherosclerosis theory. Biomolecules 13: 700. https://doi.org/10.3390/biom13040700 doi: 10.3390/biom13040700
![]() |
[74] |
Simopoulos AP (2008) The importance of the omega-6/omega-3 fatty acid ratio in cardiovascular disease and other chronic diseases. Exp Biol Med 233: 674–688. https://doi.org/10.3181/0711-MR-311 doi: 10.3181/0711-MR-311
![]() |
[75] |
Tsoupras A, Moran D, Pleskach H, et al. (2021) Beneficial anti-platelet and anti-inflammatory properties of Irish apple juice and cider bioactives. Foods 10: 412. https://doi.org/10.3390/foods10020412 doi: 10.3390/foods10020412
![]() |
[76] |
Tsoupras A, Moran D, Byrne T, et al. (2021) Anti-inflammatory and anti-platelet properties of lipid bioactives from apple cider by-products. Molecules 26: 2869. https://doi.org/10.3390/molecules26102869 doi: 10.3390/molecules26102869
![]() |
[77] | Fragopoulou E, Antonopoulou S, Tsoupras A, et al. (2004) Antiatherogenic properties of red/white wine, musts, grape-skins, and yeast. Chem Phys Lipids 130: 66. |
[78] |
Moran D, Fleming M, Daly E, et al. (2021) Anti-platelet properties of apple must/skin yeasts and of their fermented apple cider products. Beverages 7: 54. https://doi.org/10.3390/beverages7030054 doi: 10.3390/beverages7030054
![]() |
[79] |
Papadimitriou N, Bouras E, Van den Brandt PA, et al. (2022) A prospective diet-wide association study for risk of colorectal cancer in EPIC. Clin Gastroenterol Hepatol 20: 864–873. https://doi.org/10.1016/j.cgh.2021.04.028 doi: 10.1016/j.cgh.2021.04.028
![]() |
[80] |
Nogueira LC, do Rio RF, Lollo PC, et al. (2017) Moderate alcoholic beer consumption: The effects on the lipid profile and insulin sensitivity of adult men. J Food Sci 82: 1720–1725. https://doi.org/10.1111/1750-3841.13746 doi: 10.1111/1750-3841.13746
![]() |
[81] |
Hans S, Harishkumar R, Shiels K, et al. (2024) Bioactive lipids derived from red wine, beers, and their dealcoholized variants inhibit platelet-activating factor (PAF) induced platelet activation in vitro. Food Biosci 104053. https://doi.org/10.1016/j.fbio.2024.104053 doi: 10.1016/j.fbio.2024.104053
![]() |
[82] |
Panagiotakos DB, Kouli G-M, Magriplis E, et al. (2019) Beer, wine consumption, and 10-year CVD incidence: The ATTICA study. Eur J Clin Nutr 73: 1015–1023. https://doi.org/10.1038/s41430-018-0296-6 doi: 10.1038/s41430-018-0296-6
![]() |
[83] |
Dorans KS, Mostofsky E, Levitan EB, et al. (2015) Alcohol and incident heart failure among middle-aged and elderly men: Cohort of Swedish men. Circ: Heart Failure 8: 422–427. https://doi.org/10.1161/CIRCHEARTFAILURE.114.001787 doi: 10.1161/CIRCHEARTFAILURE.114.001787
![]() |
[84] |
Vilahur G, Casani L, Guerra JM, et al. (2012) Intake of fermented beverages protect against acute myocardial injury: Target organ cardiac effects and vasculoprotective effects. Basic Res Cardiol 107: 291. https://doi.org/10.1007/s00395-012-0291-3 doi: 10.1007/s00395-012-0291-3
![]() |
[85] |
Gémes K, Janszky I, Ahnve S, et al. (2016) Light-to-moderate drinking and incident heart failure—The Norwegian HUNT study. Int J Cardiol 203: 553–560. https://doi.org/10.1016/j.ijcard.2015.10.179 doi: 10.1016/j.ijcard.2015.10.179
![]() |
[86] |
Chiva-Blanch G, Condines X, Magraner E, et al. (2014) The non-alcoholic fraction of beer increases stromal cell derived factor 1 and the number of circulating endothelial progenitor cells in high cardiovascular risk subjects: A randomized clinical trial. Atherosclerosis 233: 518–524. https://doi.org/10.1016/j.atherosclerosis.2013.12.048 doi: 10.1016/j.atherosclerosis.2013.12.048
![]() |
[87] |
Chiva-Blanch G, Magraner E, Condines X, et al. (2015) Effects of alcohol and polyphenols from beer on atherosclerotic biomarkers in high cardiovascular risk men: A randomized feeding trial. Nutr, Metab Cardiovasc Dis 25: 36–45. https://doi.org/10.1016/j.numecd.2014.07.008 doi: 10.1016/j.numecd.2014.07.008
![]() |
[88] |
Joosten MM, Witkamp RF, Hendriks HF (2011) Alterations in total and high—molecular-weight adiponectin after 3 weeks of moderate alcohol consumption in premenopausal women. Metabolism 60: 1058–1063. https://doi.org/10.1016/j.metabol.2011.01.001 doi: 10.1016/j.metabol.2011.01.001
![]() |
[89] |
Nova E, San Mauro-Martín I, Díaz-Prieto LE, et al. (2019) Wine and beer within a moderate alcohol intake is associated with higher levels of HDL-c and adiponectin. Nutr Res 63: 42–50. https://doi.org/10.1016/j.nutres.2018.12.007 doi: 10.1016/j.nutres.2018.12.007
![]() |
[90] |
Trius‐Soler M, Martínez‐Carrasco P, Tresserra‐Rimbau A, et al. (2023) Effect of moderate beer consumption (with and without ethanol) on cardiovascular health in postmenopausal women. J Sci Food Agric 103: 7506–7516. https://doi.org/10.1002/jsfa.12826 doi: 10.1002/jsfa.12826
![]() |
[91] |
Padro T, Muñoz-García N, Vilahur G, et al. (2018) Moderate beer intake and cardiovascular health in overweight individuals. Nutrients 10: 1237. https://doi.org/10.3390/nu10091237 doi: 10.3390/nu10091237
![]() |
[92] |
Krnic M, Modun D, Budimir D, et al. (2011) Comparison of acute effects of red wine, beer and vodka against hyperoxia-induced oxidative stress and increase in arterial stiffness in healthy humans. Atherosclerosis 218: 530–535. https://doi.org/10.1016/j.atherosclerosis.2011.07.004 doi: 10.1016/j.atherosclerosis.2011.07.004
![]() |
[93] |
Nishiwaki M, Kora N, Matsumoto N (2017) Ingesting a small amount of beer reduces arterial stiffness in healthy humans. Physiol Rep 5: e13381. https://doi.org/10.14814/phy2.13381 doi: 10.14814/phy2.13381
![]() |
[94] |
Karatzi K, Rontoyanni VG, Protogerou AD, et al. (2013) Acute effects of beer on endothelial function and hemodynamics: a single-blind, crossover study in healthy volunteers. Nutrition 29: 1122–1126. https://doi.org/10.1016/j.nut.2013.02.016 doi: 10.1016/j.nut.2013.02.016
![]() |
[95] |
Schrieks IC, Joosten MM, Klöpping-Ketelaars W, et al. (2016) Moderate alcohol consumption after a mental stressor attenuates the endocrine stress response. Alcohol 57: 29–34. https://doi.org/10.1016/j.alcohol.2016.10.006 doi: 10.1016/j.alcohol.2016.10.006
![]() |
[96] |
Laguzzi F, Risérus U, Marklund M, et al. (2018) Circulating fatty acids in relation to alcohol consumption: Cross-sectional results from a cohort of 60-year-old men and women. Clin Nutr 37: 2001–2010. https://doi.org/10.1016/j.clnu.2017.09.007 doi: 10.1016/j.clnu.2017.09.007
![]() |
[97] |
Russell FD, Bürgin-Maunder CS (2012) Distinguishing health benefits of eicosapentaenoic and docosahexaenoic acids. Mar Drugs 10: 2535–2559. https://doi.org/10.3390/md10112535 doi: 10.3390/md10112535
![]() |
[98] |
Kok EH, Karppinen TT, Luoto T, et al. (2016) Beer drinking associates with lower burden of amyloid beta aggregation in the brain: H elsinki sudden death series. Alcohol: Clin Exp Res 40: 1473–1478. https://doi.org/10.1111/acer.13102 doi: 10.1111/acer.13102
![]() |
[99] |
Liu R, Guo X, Park Y, et al. (2013) Alcohol consumption, types of alcohol, and Parkinson's disease. PLoS One 8: e66452. https://doi.org/10.1371/journal.pone.0066452 doi: 10.1371/journal.pone.0066452
![]() |
[100] |
Alonso-Andrés P, Martín M, Albasanz JL (2019) Modulation of adenosine receptors and antioxidative effect of beer extracts in in vitro models. Nutrients 11: 1258. https://doi.org/10.3390/nu11061258 doi: 10.3390/nu11061258
![]() |
[101] |
Hernández-Quiroz F, Nirmalkar K, Villalobos-Flores LE, et al. (2020) Influence of moderate beer consumption on human gut microbiota and its impact on fasting glucose and β-cell function. Alcohol 85: 77–94. https://doi.org/10.1016/j.alcohol.2019.05.006 doi: 10.1016/j.alcohol.2019.05.006
![]() |
[102] |
Martínez-Montoro JI, Quesada-Molina M, Gutiérrez-Repiso C, et al. (2022) Effect of moderate consumption of different phenolic-content beers on the human gut microbiota composition: A randomized crossover trial. Antioxidants 11: 696. https://doi.org/10.3390/antiox11040696 doi: 10.3390/antiox11040696
![]() |
[103] |
Zugravu C-A, Medar C, Manolescu LSC, et al. (2023) Beer and microbiota: Pathways for a Positive and Healthy Interaction. Nutrients 15: 844. https://doi.org/10.3390/nu15040844 doi: 10.3390/nu15040844
![]() |
[104] |
Kesse-Guyot E, Andreeva VA, Jeandel C, et al. (2012) Alcohol consumption in midlife and cognitive performance assessed 13 years later in the SU. VI. MAX 2 cohort. PLoS One 7: e52311. https://doi.org/10.1371/journal.pone.0052311 doi: 10.1371/journal.pone.0052311
![]() |
[105] |
Heymann D, Stern Y, Cosentino S, et al. (2016) The association between alcohol use and the progression of Alzheimer's disease. Curr Alzheimer Res 13: 1356–1362. https://doi.org/10.2174/1567205013666160603005035 doi: 10.2174/1567205013666160603005035
![]() |
[106] |
Ano Y, Yoshikawa M, Takaichi Y, et al. (2019) Iso-α-acids, bitter components in beer, suppress inflammatory responses and attenuate neural hyperactivation in the hippocampus. Front Pharmacol 10: 81. https://doi.org/10.3389/fphar.2019.00081 doi: 10.3389/fphar.2019.00081
![]() |
[107] |
Niemelä O, Aalto M, Bloigu A, et al. (2022) Alcohol drinking patterns and laboratory indices of health: Does type of alcohol preferred make a difference? Nutrients 14: 4529. https://doi.org/10.3390/nu14214529 doi: 10.3390/nu14214529
![]() |
[108] |
Demoury C, Karakiewicz P, Parent M-E (2016) Association between lifetime alcohol consumption and prostate cancer risk: A case-control study in Montreal, Canada. Cancer Epidemiol 45: 11–17. https://doi.org/10.1016/j.canep.2016.09.004 doi: 10.1016/j.canep.2016.09.004
![]() |
[109] |
Hay JL, Kiviniemi MT, Orom H, et al. (2023) Moving beyond the "Health Halo" of alcohol: What will it take to achieve population awareness of the cancer risks of alcohol? Cancer Epidemiol, Biomarkers Prev 32: 9–11. https://doi.org/10.1158/1055-9965.EPI-22-1102 doi: 10.1158/1055-9965.EPI-22-1102
![]() |
[110] |
Bazsefidpar N, Yazdi APG, Karimi A, et al. (2024) Brewers spent grain protein hydrolysate as a functional ingredient for muffins: Antioxidant, antidiabetic, and sensory evaluation. Food Chem 435: 137565. https://doi.org/10.1016/j.foodchem.2023.137565 doi: 10.1016/j.foodchem.2023.137565
![]() |
[111] |
Ktenioudaki A, Chaurin V, Reis SF, et al. (2012) Brewer's spent grain as a functional ingredient for breadsticks. Int J Food Sci Technol 47: 1765–1771. https://doi.org/10.1111/j.1365-2621.2012.03032.x doi: 10.1111/j.1365-2621.2012.03032.x
![]() |
[112] |
Baiano A, la Gatta B, Rutigliano M, et al. (2023) Functional bread produced in a circular economy perspective: The use of brewers' spent grain. Foods 12: 834. https://doi.org/10.3390/foods12040834 doi: 10.3390/foods12040834
![]() |
[113] |
Cappa C, Alamprese C (2017) Brewer's spent grain valorization in fiber-enriched fresh egg pasta production: Modelling and optimization study. LWT-Food Sci Technol 82: 464–470. https://doi.org/10.1016/j.lwt.2017.04.068 doi: 10.1016/j.lwt.2017.04.068
![]() |
[114] |
Lomuscio E, Bianchi F, Cervini M, et al. (2022) Durum wheat fresh pasta fortification with trub, a beer industry by-product. Foods 11: 2496. https://doi.org/10.3390/foods11162496 doi: 10.3390/foods11162496
![]() |
[115] |
Avramia I, Amariei S (2021) Spent brewer's yeast as a source of insoluble β-glucans. Int J Mol Sci 22: 825. https://doi.org/10.3390/ijms22020825 doi: 10.3390/ijms22020825
![]() |
[116] |
Helkar PB, Sahoo AK, Patil N (2016) Review: Food industry by-products used as a functional food ingredients. Int J Waste Resour 6: 1–6. https://doi.org/10.4172/2252-5211.1000248 doi: 10.4172/2252-5211.1000248
![]() |
[117] |
Amorim M, Pereira JO, Gomes D, et al. (2016) Nutritional ingredients from spent brewer's yeast obtained by hydrolysis and selective membrane filtration integrated in a pilot process. J Food Eng 185: 42–47. https://doi.org/10.1016/j.jfoodeng.2016.03.032 doi: 10.1016/j.jfoodeng.2016.03.032
![]() |
[118] |
Verouti SN, Tsoupras AB, Alevizopoulou F, et al. (2013) Paricalcitol effects on activities and metabolism of platelet activating factor and on inflammatory cytokines in hemodialysis patients. Int J Artif Organs 36: 87–96. https://doi.org/10.5301/ijao.5000187 doi: 10.5301/ijao.5000187
![]() |
[119] |
Almendinger M, Rohn S, Pleissner D (2020) Malt and beer-related by-products as potential antioxidant skin-lightening agents for cosmetics. Sustainable Chem Pharm 17: 100282. https://doi.org/10.1016/j.scp.2020.100282 doi: 10.1016/j.scp.2020.100282
![]() |
[120] |
Bucci PL, Santos MV, Montanari J, et al. (2020) Nanoferulic: From a by‐product of the beer industry toward the regeneration of the skin. J Cosmet Dermatol 19: 2958–2964. https://doi.org/10.1111/jocd.13407 doi: 10.1111/jocd.13407
![]() |
[121] |
Di Domenico M, Feola A, Ambrosio P, et al. (2020) Antioxidant effect of beer polyphenols and their bioavailability in dental-derived stem cells (D-dSCs) and human intestinal epithelial lines (Caco-2) cells. Stem Cells Int 2020: 8835813. https://doi.org/10.1155/2020/8835813 doi: 10.1155/2020/8835813
![]() |
[122] |
Bonifácio-Lopes T, Teixeira JA, Pintado M (2020) Current extraction techniques towards bioactive compounds from brewer's spent grain–A review. Crit Rev Food Sci Nutr 60: 2730–2741. https://doi.org/10.1080/10408398.2019.1655632 doi: 10.1080/10408398.2019.1655632
![]() |
[123] |
Ameer K, Shahbaz HM, Kwon J (2017) Green extraction methods for polyphenols from plant matrices and their byproducts: A review. Compr Rev Food Sci Food Saf 16: 295–315. https://doi.org/10.1111/1541-4337.12253 doi: 10.1111/1541-4337.12253
![]() |
[124] |
Guido LF, Moreira MM (2017) Techniques for extraction of brewer's spent grain polyphenols: A review. Food Bioprocess Technol 10: 1192–1209. https://doi.org/10.1007/s11947-017-1913-4 doi: 10.1007/s11947-017-1913-4
![]() |
[125] |
Verni M, Pontonio E, Krona A, et al. (2020) Bioprocessing of brewers' spent grain enhances its antioxidant activity: Characterization of phenolic compounds and bioactive peptides. Front Microbiol 11: 1831. https://doi.org/10.3389/fmicb.2020.01831 doi: 10.3389/fmicb.2020.01831
![]() |
[126] |
Shon YJ, Kim WC, Lee S-H, et al. (2023) Antimelanogenic potential of brewer's spent grain extract through modulation of the MAPK/MITF axis. Sustainable Mater Technol 38: e00721. https://doi.org/10.1016/j.susmat.2023.e00721 doi: 10.1016/j.susmat.2023.e00721
![]() |
[127] |
Ribeiro-Oliveira R, Martins ZE, Faria MÂ, et al. (2022) Protein hydrolysates from brewing by-products as natural alternatives to ACE-inhibitory drugs for hypertension management. Life 12: 1554. https://doi.org/10.3390/life12101554 doi: 10.3390/life12101554
![]() |
[128] |
Cian RE, Hernández‐Chirlaque C, Gámez‐Belmonte R, et al. (2020) Molecular action mechanism of anti‐inflammatory hydrolysates obtained from brewers' spent grain. J Sci Food Agric 100: 2880–2888. https://doi.org/10.1002/jsfa.10313 doi: 10.1002/jsfa.10313
![]() |
[129] |
Oliveira AS, Ferreira C, Pereira JO, et al. (2022) Spent brewer's yeast (Saccharomyces cerevisiae) as a potential source of bioactive peptides: An overview. Int J Biol Macromol 208: 1116–1126. https://doi.org/10.1016/j.ijbiomac.2022.03.094 doi: 10.1016/j.ijbiomac.2022.03.094
![]() |
[130] |
Costa EM, Oliveira AS, Silva S, et al. (2023) Spent yeast waste streams as a sustainable source of bioactive peptides for skin applications. Int J Mol Sci 24: 2253. https://doi.org/10.3390/ijms24032253 doi: 10.3390/ijms24032253
![]() |
[131] |
García-Curiel L, Pérez-Flores JG, Contreras-López E, et al. (2023) Evaluating the application of an arabinoxylan-rich fraction from brewers' spent grain as a release modifier of drugs. Nat Prod Res 38: 1759–1765. https://doi.org/10.1080/14786419.2023.2214841 doi: 10.1080/14786419.2023.2214841
![]() |
[132] |
Sousa P, Tavares-Valente D, Pereira CF, et al. (2024) Circular economyeast: Saccharomyces cerevisiae as a sustainable source of glucans and its safety for skincare application. Int J Biol Macromol 265: 130933. https://doi.org/10.1016/j.ijbiomac.2024.130933 doi: 10.1016/j.ijbiomac.2024.130933
![]() |
[133] |
Zheng Y, Wang Z, Huang Y, et al. (2024) Extraction and preparation of cellulose nanocrystal from Brewer's spent grain and application in pickering emulsions. Bioact Carbohydr Diet Fibre 31: 100418. https://doi.org/10.1016/j.bcdf.2024.100418 doi: 10.1016/j.bcdf.2024.100418
![]() |
[134] |
Leeyaphan C, Varothai S, Trakanwittayarak S, et al. (2022) A randomized controlled trial to compare the effectiveness and safety of adsorbent lotion containing tapioca starch, spent grain wax, Butyrospermum parkii extract, argania spinosa kernel oil, aloe barbadensis, rosehip oil, and allantoin with a low‐potency topical corticosteroid in the treatment of intertrigo. J Cosmet Dermatol 21: 679–688. https://doi.org/10.1111/jocd.14125 doi: 10.1111/jocd.14125
![]() |
[135] |
Pereira OR, Santos G, Sousa MJ (2022) Hop by-products: Pharmacological activities and potential application as cosmetics. Cosmetics 9: 139. https://doi.org/10.3390/cosmetics9060139 doi: 10.3390/cosmetics9060139
![]() |
[136] |
Weber N, Biehler K, Schwabe K, et al. (2019) Hop extract acts as an antioxidant with antimicrobial effects against Propionibacterium acnes and Staphylococcus aureus. Molecules 24: 223. https://doi.org/10.3390/molecules24020223 doi: 10.3390/molecules24020223
![]() |
[137] |
Venturelli S, Burkard M, Biendl M, et al. (2016) Prenylated chalcones and flavonoids for the prevention and treatment of cancer. Nutrition 32: 1171–1178. https://doi.org/10.1016/j.nut.2016.03.020 doi: 10.1016/j.nut.2016.03.020
![]() |
[138] |
Zanoli P, Zavatti M (2008) Pharmacognostic and pharmacological profile of Humulus lupulus L. J Ethnopharmacol 116: 383–396. https://doi.org/10.1016/j.jep.2008.01.011 doi: 10.1016/j.jep.2008.01.011
![]() |
[139] |
Das P, Dutta T, Manna S, et al. (2022) Facile green synthesis of non-genotoxic, non-hemolytic organometallic silver nanoparticles using extract of crushed, wasted, and spent Humulus lupulus (hops): Characterization, anti-bacterial, and anti-cancer studies. Environ Res 204: 111962. https://doi.org/10.1016/j.envres.2021.111962 doi: 10.1016/j.envres.2021.111962
![]() |
[140] |
Onder FC, Kalin S, Sahin N, et al. (2023) Major bioactive prenylated flavonoids from Humulus lupulus L., their applications in human diseases and Structure-Activity Relationships (SAR)—A review. Pharm Sci 30: 1–20. http://doi.org/10.34172/ps.2023.18 doi: 10.34172/ps.2023.18
![]() |
[141] |
Michalkova R, Mirossay L, Kello M, et al. (2023) Anticancer potential of natural chalcones: In vitro and in vivo evidence. Int J Mol Sci 24: 10354. https://doi.org/10.3390/ijms241210354 doi: 10.3390/ijms241210354
![]() |
[142] |
Liu Z, Jiang S, Hao B, et al. (2024) A proteomic landscape of pharmacologic perturbations for functional relevance. J Pharm Anal 14: 128–139. https://doi.org/10.1016/j.jpha.2023.08.021 doi: 10.1016/j.jpha.2023.08.021
![]() |
[143] |
Lu X, Liu M, Dong H, et al. (2022) Dietary prenylated flavonoid xanthohumol alleviates oxidative damage and accelerates diabetic wound healing via Nrf2 activation. Food Chem Toxicol 160: 112813. https://doi.org/10.1016/j.fct.2022.112813 doi: 10.1016/j.fct.2022.112813
![]() |
[144] |
Liu M, Hansen PE, Wang G, et al. (2015) Pharmacological profile of xanthohumol, a prenylated flavonoid from hops (Humulus lupulus). Molecules 20: 754–779. https://doi.org/10.3390/molecules20010754 doi: 10.3390/molecules20010754
![]() |
[145] |
Svolacchia F, Brongo S, Catalano A, et al. (2023) Natural products for the prevention, treatment and progression of breast cancer. Cancers 15: 2981. https://doi.org/10.3390/cancers15112981 doi: 10.3390/cancers15112981
![]() |
[146] |
Buckett L, Sus N, Spindler V, et al. (2023) The pharmacokinetics of individual conjugated xanthohumol metabolites show efficient glucuronidation and higher bioavailability of micellar than native xanthohumol in a randomized, double‐blind, crossover trial in healthy humans. Molecular Nutr Food Res 67: 2200684. https://doi.org/10.1002/mnfr.202200684 doi: 10.1002/mnfr.202200684
![]() |
[147] |
Nurzynska A, Klimek K, Michalak A, et al. (2023) Do curdlan hydrogels improved with bioactive compounds from hop exhibit beneficial properties for skin wound healing? Int J Mol Sci 24: 10295. https://doi.org/10.3390/ijms241210295 doi: 10.3390/ijms241210295
![]() |
1. | Konstantinos Giannakou, Maria Kyprianidou, Margarita Christofi, Anastasios Kalatzis, Georgia Fakonti, Mandatory COVID-19 Vaccination for Healthcare Professionals and Its Association With General Vaccination Knowledge: A Nationwide Cross-Sectional Survey in Cyprus, 2022, 10, 2296-2565, 10.3389/fpubh.2022.897526 | |
2. | Lulla Opatowski, Laura Temime, Contributions of modelling for the control of COVID-19 nosocomial transmission, 2022, 41, 23525568, 101054, 10.1016/j.accpm.2022.101054 | |
3. | Wenyu Chen, Ming Yao, Liang Dong, Pingyang Shao, Ye Zhang, Binjie Fu, The application framework of big data technology during the COVID-19 pandemic in China, 2022, 150, 0950-2688, 10.1017/S0950268822000577 | |
4. | Jordana Vaz Hendler, Patricia Miranda do Lago, Gabriel Cardozo Müller, João Carlos Santana, Jefferson Pedro Piva, Liane Esteves Daudt, Risk factors for severe COVID-19 infection in Brazilian children, 2021, 25, 14138670, 101650, 10.1016/j.bjid.2021.101650 | |
5. | Tom Dudding, Sadiyah Sheikh, Florence Gregson, Jennifer Haworth, Simon Haworth, Barry G. Main, Andrew J. Shrimpton, Fergus W. Hamilton, Anthony J. Ireland, Nick A. Maskell, Jonathan P. Reid, Bryan R. Bzdek, Mark Gormley, Markus Metsälä, A clinical observational analysis of aerosol emissions from dental procedures, 2022, 17, 1932-6203, e0265076, 10.1371/journal.pone.0265076 | |
6. | David Moreno-Martos, Sean Foley, Benjamin Parcell, Dumitru Trucu, Raluca Eftimie, A computational investigation of COVID-19 transmission inside hospital wards and associated costs, 2022, 19, 1551-0018, 6504, 10.3934/mbe.2022306 | |
7. | Meghann Gallouche, Caroline Landelle, Sylvie Larrat, Aurélie Truffot, Jean-Luc Bosson, Alban Caporossi, Towards real-time monitoring of COVID-19 nosocomial clusters using SARS-CoV-2 genomes in a university hospital of the French Alps, 2023, 26669919, 104650, 10.1016/j.idnow.2023.104650 | |
8. | F. McAndrew, R.G. Abeysuriya, R. Sacks-Davis, M. Sammann, D.M. Lister, D. West, S.S. Majumdar, N. Scott, Admission screening testing of patients and staff N95 respirators are cost-effective in reducing COVID-19 hospital-acquired infections, 2024, 152, 01956701, 81, 10.1016/j.jhin.2024.06.015 | |
9. | Sreejita Ghosh, Dibyajit Lahiri, Moupriya Nag, Rina Rani Ray, Debasmita Bhattacharya, 2024, Chapter 7, 978-981-99-9461-8, 145, 10.1007/978-981-99-9462-5_7 | |
10. | D.R.M. Smith, S. Chervet, T. Pinettes, G. Shirreff, S. Jijón, A. Oodally, K. Jean, L. Opatowski, S. Kernéis, L. Temime, How have mathematical models contributed to understanding the transmission and control of SARS-CoV-2 in healthcare settings? A systematic search and review, 2023, 141, 01956701, 132, 10.1016/j.jhin.2023.07.028 | |
11. | George Shirreff, Anne C.M. Thiébaut, Bich-Tram Huynh, Guillaume Chelius, Antoine Fraboulet, Didier Guillemot, Lulla Opatowski, Laura Temime, Hospital population density and risk of respiratory infection: is close contact density dependent?, 2024, 17554365, 100807, 10.1016/j.epidem.2024.100807 |
Parameters (& units) | Description | Range | Most common values | References |
σ (day−1) | Incubation rate | 0.071−0.5 | 0.143, 0.192 | [11,12,13,14,15,16,18,19,20,22,23,24,25,32,33,34,35,36,37,38] |
1/σ (day) | Mean incubation period | 2−14 | 7, 5.2 | [11,12,13,14,15,16,18,19,20,22,23,24,25,32,33,34,35,36,37,38] |
γ (day−1) | Recovery rate | 0.055−0.621 | 0.143−0.25 | [11,12,13,14,15,16,18,19,21,22,23,24,32,33,35,36,37,39,40] |
1/γ (day) | Mean recovery / infection period | 1.61−18 | 4−7 | [11,12,13,14,15,16,18,19,21,22,23,24,32,33,35,36,37,39,40] |
Parameters (& units) | Description | Range | Most common values | References |
σ (day−1) | Incubation rate | 0.071−0.5 | 0.143, 0.192 | [11,12,13,14,15,16,18,19,20,22,23,24,25,32,33,34,35,36,37,38] |
1/σ (day) | Mean incubation period | 2−14 | 7, 5.2 | [11,12,13,14,15,16,18,19,20,22,23,24,25,32,33,34,35,36,37,38] |
γ (day−1) | Recovery rate | 0.055−0.621 | 0.143−0.25 | [11,12,13,14,15,16,18,19,21,22,23,24,32,33,35,36,37,39,40] |
1/γ (day) | Mean recovery / infection period | 1.61−18 | 4−7 | [11,12,13,14,15,16,18,19,21,22,23,24,32,33,35,36,37,39,40] |