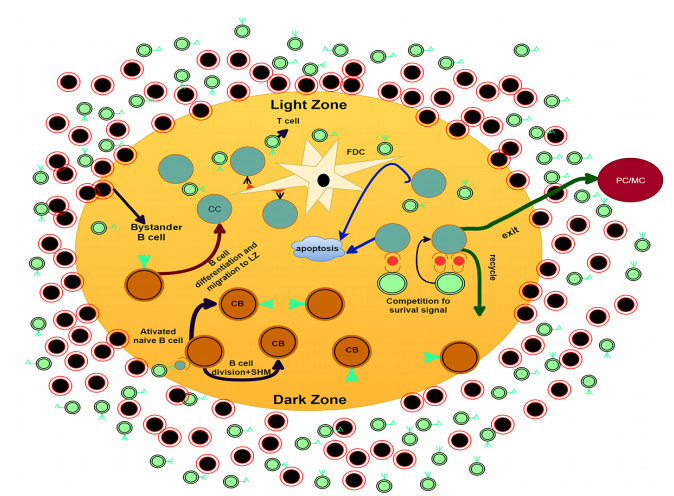
Although the densities of crystal quartz and vitreous silica differ only by about 17% (2.65 and 2.20 g/cm3, respectively), the helium permeability of silica glass is six orders more elevated than that of crystal quartz. This vast difference has puzzled researchers for decades considering that silica glass and quartz crystal have the same chemical composition. This work discusses the mechanism of high helium permeation through silica glass. It briefly reviews the experimental data and its contradictions with the continuous random network theory. A recently proposed nanoflake model for silica glass structure is utilized to explain the origin of glass permeation to helium. According to the nanoflake model, the formation of nanoflakes not only brings a one-dimensional medium-range ordering structure into silica glass but simultaneously creates regions where van der Waals bonds replace the oxygen-silicon covalent bonds. It is the weakness of van der Waals bonds that causes the helium mobility in these areas to increase.
Citation: Shangcong Cheng. On the mechanism of helium permeation through silica glass[J]. AIMS Materials Science, 2024, 11(3): 438-448. doi: 10.3934/matersci.2024022
[1] | Samantha Erwin, Stanca M. Ciupe . Germinal center dynamics during acute and chronic infection. Mathematical Biosciences and Engineering, 2017, 14(3): 655-671. doi: 10.3934/mbe.2017037 |
[2] | Kelum Gajamannage, Erik M. Bollt . Detecting phase transitions in collective behavior using manifold's curvature. Mathematical Biosciences and Engineering, 2017, 14(2): 437-453. doi: 10.3934/mbe.2017027 |
[3] | Ankai Liu, Felicia Maria G. Magpantay, Kenzu Abdella . A framework for long-lasting, slowly varying transient dynamics. Mathematical Biosciences and Engineering, 2023, 20(7): 12130-12153. doi: 10.3934/mbe.2023540 |
[4] | Tatyana S. Turova . Structural phase transitions in neural networks. Mathematical Biosciences and Engineering, 2014, 11(1): 139-148. doi: 10.3934/mbe.2014.11.139 |
[5] | Mika Yoshida, Kinji Fuchikami, Tatsuya Uezu . Realization of immune response features by dynamical system models. Mathematical Biosciences and Engineering, 2007, 4(3): 531-552. doi: 10.3934/mbe.2007.4.531 |
[6] | Arminda Moreno-Díaz, Gabriel de Blasio, Moreno-Díaz Jr. . Distributed, layered and reliable computing nets to represent neuronal receptive fields. Mathematical Biosciences and Engineering, 2014, 11(2): 343-361. doi: 10.3934/mbe.2014.11.343 |
[7] | Dominique Duncan, Thomas Strohmer . Classification of Alzheimer's disease using unsupervised diffusion component analysis. Mathematical Biosciences and Engineering, 2016, 13(6): 1119-1130. doi: 10.3934/mbe.2016033 |
[8] | Francesca Sapuppo, Elena Umana, Mattia Frasca, Manuela La Rosa, David Shannahoff-Khalsa, Luigi Fortuna, Maide Bucolo . Complex spatio-temporal features in meg data. Mathematical Biosciences and Engineering, 2006, 3(4): 697-716. doi: 10.3934/mbe.2006.3.697 |
[9] | Dong Liang, Jianhong Wu, Fan Zhang . Modelling Population Growth with Delayed Nonlocal Reaction in 2-Dimensions. Mathematical Biosciences and Engineering, 2005, 2(1): 111-132. doi: 10.3934/mbe.2005.2.111 |
[10] | Michele L. Joyner, Chelsea R. Ross, Colton Watts, Thomas C. Jones . A stochastic simulation model for Anelosimus studiosus during prey capture: A case study for determination of optimal spacing. Mathematical Biosciences and Engineering, 2014, 11(6): 1411-1429. doi: 10.3934/mbe.2014.11.1411 |
Although the densities of crystal quartz and vitreous silica differ only by about 17% (2.65 and 2.20 g/cm3, respectively), the helium permeability of silica glass is six orders more elevated than that of crystal quartz. This vast difference has puzzled researchers for decades considering that silica glass and quartz crystal have the same chemical composition. This work discusses the mechanism of high helium permeation through silica glass. It briefly reviews the experimental data and its contradictions with the continuous random network theory. A recently proposed nanoflake model for silica glass structure is utilized to explain the origin of glass permeation to helium. According to the nanoflake model, the formation of nanoflakes not only brings a one-dimensional medium-range ordering structure into silica glass but simultaneously creates regions where van der Waals bonds replace the oxygen-silicon covalent bonds. It is the weakness of van der Waals bonds that causes the helium mobility in these areas to increase.
It has been known for a long time that hosts face a virtually limitless number of antigens, so the innate immunity alone is not enough for a full-scale disease prevention. Therefore, adaptive immunity is required for the defense against various antigens [1]. GC [2] is a main structure to produce specific immunity, which comes into being in secondary lymphoid organs, e.g., spleen, lymph node, pharyngeal tonsil. According to a series of experiments, after pathogens invade the human body, the affinity of antibodies increases dramatically over a short period of time. This phenomenon is known as affinity maturation [3], which takes place mainly in the GC through a series of physiological events including clonal expansion, high rate mutation of immunoglobulin, positive selection and cyclic re-entry [4,5,6,7,8].
To describe the reactions occurring inside the GC and the roles played by different cells, MacLennan [4] introduced a classic affinity maturation mechanism in 1994 while experimental biologists start to unfold the detailed dynamics in GC to explain the rapid maturation of antibody affinity. At the same time, theorists set out to establish a series of mathematical models [9,10,11,12,13], which explained the experimental data and carried out a quantitative and systematic analysis of immunological processes. The rise and development of the GC research provide an excellent testing ground for mathematical modeling [14]. For example, in 1993, the deterministic model built by Kepler and Perelson [10] concluded that during the immune response, it is necessary for centroblasts (CBs) to travel back to the DZ from the LZ in order to accelerate affinity maturation.
Up to now, many details of the immune response in GC remain unclear[15], such as why GC has the structure of light and dark zones? How B cells interact with Follicular Helper T Cells (Tfh) and what factors determine whether B cells differentiate into plasma or memory cells [16], and how GCs choose to produce broadly neutralizing antibodies against HIV? In order to solve these problems, theoretical biologists use mathematical models to delve deeply into the structure and dynamics of the GC development, based on differential equations or large-scale simulations. For example, Meyer-Hermann et al. incorporated two-photon imaging data into his simulation of cell movement and migration to check the role of chemokines in GC formation [17,18,19]. He also built a stochastic model describing morphological properties of the GC reaction, revealing necessary conditions for the development of DZ in the first phase of monoclonal expansion [20]. Later, two mechanisms[21] have been disclosed to explain the rapid affinity maturation: the first is the introduction of a refractory time to limit the interaction between B cells and Follicular Dendritic Cells (FDCs), and the second is the competition between B cells for the help from Tfh [22]. Both mechanisms help achieve good affinity maturation in silico, which is consistent with experiment [23].
Recently, there has been a lot of theoretical work [24,25,26,27,28]. Meyer-Hermann et al. proposed how the B cells go out of the GC and broadly neutralize antibodies with extensive simulations [25,26]. Wang et al.[29] proposed a silico model of affinity maturation driven by antigen variation, which revealed that the induction probability of cross-reactive antibodies is very low. Since the conflicting choices imposed by different antigen variants may hinder the maturation of affinity, the sequential immunization of antigen variants is more conducive to the induction of cross-reactive antibodies than the cocktail mechanism. Marco Molari and Remi Monasson [27] used the agent-based model to construct the kinetic process which found that the maturation rate and antibody group expansion is controlled by antigen availability, and combined with experiments his model confirms the existence of a non-monotonic relationship between the average affinity and the antigen dose.
In biological experiments, it has been observed that the volume distribution of GCs is not normal but right-skewed (mean > median), because the frequency of small GC appearance is very high [30,31]. But there are few experiments and simulations to analyze the underlying principle behind observations like this, which are related to GC morphology, such as the appearance of the light and the dark zone or the GC volume distribution just mentioned. These problems are obviously important, because the immune system has evolved over a long period of time to its current form, certainly in order to utilize as efficiently as possible different resources to acquire robust resistance to pathogens. Therefore, an in-depth quantitative study on the GC morphology not only helps us understand the accelerated affinity maturation, but also suggests ways of controlling the GC formation.
In this article, we first build a stochastic model in which the impact of the GC morphology on affinity maturation is quantitatively studied. If the existence of DZ and LZ is eliminated, the most important change in GCs is that centrocytes (CCs) and CBs will mix and push each other in a confined space, resulting in an unnecessary competition between the B cells and thus leading to a decline of cell affinity. Henceforth the CXCL12-expressing reticular cells (CRCs) in DZ and FDCs in LZ serve to separate CBs involved in somatic hyper-mutation (SHM) from CCs in selection competition, which maintains the delicate yet compulsory competition between specific types of B cells and as a result bestows efficient affinity maturation. Next, we check the influence of the GC size on affinity maturation. Simulation results show that the GC has a critical volume for affinity maturation, beyond which the affinity of plasma cells is greatly improved. In addition, this phase transition is clearly seen in a deterministic model (without considering the spatial dimensions) derived from the stochastic one. More detailed studies show that the phase transition point is determined by the distance in the shape space between the BCR and the target antigen epitope in the lymph node. The final problem to consider is whether a 2D model can be used to represent the real GC reactions, so we extend the model to 3D for comparison and and get the same conclusion as in 2D, indicating that the 2D model could be useful in the current model.
In this paper, we construct a stochastic model as an extension of the one proposed in an earlier article [32], and a new deterministic model displayed in Appendix A based on the stochastic one. The simulation is carried out with the Gillespie algorithm [33].
More details are considered in the current model together with some newly revealed regulatory biochemical reactions (Figure 1). Below, several points of the current version of the stochastic model are explained and stressed. We simulate the responses of all individual cells as discrete events occurring at different rates that depend on states of cells and environment cues.
We use a rectangular grid to represent the entire GC, with a lattice spacing of 10 µm, providing a platform for different cells to migrate randomly. The same type of cells are assumed to exclude each other and unable to occupy the same grid point, while different types can. However, CBs and CCs are still assumed to repel each other. The state of a cell is described by a vector containing many entries, such as spatial location, protein concentration, and various reaction rates displayed in Table 1. During the GC growth, each possible state change is regarded as a reaction. When a reaction takes place, the corresponding vector entries of the cell will be updated. The complete regulatory network for affinity maturation in a GC remains elusive with few studies and guesses being available[16,34]. Therefore, a somewhat artificial "functional" factor, the signal integrator protein (SIP), was introduced into the model, which conglomerates different factors for simplicity and suppose to regulate various response rates in the whole GC growth[32]. The model is explained in detail in the following.
No. | Name of vector entries | Notes |
1 | Cell type | CBs/CCs/Tfh |
2 | Shape space coordinates | - |
3 | Real space coordinates | - |
4 | Moving speed | - |
5 | Proliferation rate | - |
6 | Differentiation rate | - |
7 | Apoptosis rate | - |
8 | Status marker-01 | CC-FDC |
9 | Status marker-01 | CC-T |
10 | Molecular concentration-01 | ICOSL |
11 | Molecular concentration-02 | PD-L1 |
12 | Molecular concentration-03 | ICOS |
13 | Molecular concentration-04 | SIP |
14 | Molecular concentration-06 | uptaken antigens from FDCs |
15 | Molecular concentration-07 | help signals |
16 | Molecular concentration-08 | pMHC |
17 | Synthetic rate or degradation rate of | SIP/help signal/ICOSL/PD-L1 |
In the model, we use a four-dimensional lattice as the shape space to describe different gene types of the BCR repertoire and the target epitope of the antigen (Figure 2) [26]. The BCR repertoire is enriched by rapid division and proliferation of B cells with high frequency somatic mutations. The epitope of the antigen is represented with a unique point—say, the origin ϕ∗=(0,0,0,0). A mutation is represented by a jump to a nearest neighbour in the shape space, which changes the affinity of an antibody:
α=kx×eky−kz×δ(ϕ,ϕ∗) |
where α is the affinity of the BCR, with a maximum value of 1, and ϕ is the coordinate of the BCR repertoire in the shape space, and the constant kx=0.0037,ky=5.6,kz=0.7.
According to experiments, we know the average speed of motion of B and T cells in the GC, which is described as random walks in the current model. A cell moves in all directions to the nearest lattice point with the same probability. In a two-dimensional plane, there are four directions to go. However, B cells cannot move to a lattice point already occupied by another B cell. When FDCs are distributed in a polarized way in the follicle, the CCs and Tfh cells will sense the concentration of CXCL13 secreted by FDCs and the probability of moving changes, the magnitude of which is determined by the concentration of CXCL13, being assumed to be inversely proportional to the distance from the FDCs: Ccxcl13=((x−xFDC)2+(y−yFDC)2)−12, where x,y is the location of cells, and xFDC,yFDC is the average position of FDCs. In this model, we assume that the probability of a cell moving in the direction of FDC will be 1/Ccxcl13 times higher than in other directions. We deploy 100 activated T cells in the paracortex, which ensures that the Tfh cells entering the GC accounts for 5% of the total number of cells. These activated T cells actively respond to the CXCL13 secreted by FDCs [37] and gather around the primary follicle but are prevented from entering by bystander B cells [38,39,40]. Nevertheless, the ICOSL from the B cells, engaged in the ICOS signaling, optimizes phosphoinositide-3 kinase (PI3K)-dependent pseudopod dynamics, which promotes T cells' persistent motility and enables them crossing the T-B border and entering the primary follicle. When T cells are activated in the paracortex, the generation rate of ICOS is assumed to be 50hr−1. When T cells are in contact with bystander B cells, due to the activation of ICOS, the speed SI of T cells changes according to:
SI=Tv∗(P1∗nA11+P1∗nA1) | (2.1) |
where P1=4×10−4,A1=8 and n is the concentration of ICOS. Due to the physical blockage of bystander B cells, the rate at which T cells enter a follicle is reduced. In our model, the basic moving speed of T cells (Tv) is taken to be 5 µm/min.
In order to promote CCs with high affinity, we assume the following competition mechanism. If one cell is in contact with the an FDC site, and its neighboring cell is not, these two B cells have a chance to exchange their positions with a probability proportional to
CA=cA+x1∗(x2+x3∗ΔA) |
where ΔA is the ratio of their BCR affinities. The constant cA=2.0 gives the cell's basic competitiveness, and x1=40.0,x2=−4/3,x3=4/3.
Similar competition is assumed to exist also in the binding of B cells with Tfh cells. In the stochastic model, B cells have the opportunity to contact with Tfh cells by presenting the pMHC molecule to the TCR on Tfh cells. It is natural to assume that this process is controlled by the pMHC density on the surface of the B cells. Thus a B cell in contact with a Tfh cell may be replaced by a neighbouring one with more pMHC. The exchange probability is
CT=y1∗(y2∗Δy3T)1+(y2∗Δy3T) | (2.2) |
where ΔT is the concentration difference of pMHC and y1=5.0,y2=6.0,y3=0.001.
In the experiment by Quah et al.[42], however, it was observed that B cells can exchange their BCRs through membrane contact, which may alleviate the competition more or less. But we do not take this into account in the current model.
In the early stage of the immune response, B cells proliferate rapidly in the DZ, along with SHM to change its BCR repertoire. The proliferation rate is approximately 9hr−1 and is controlled by SIP, expressed as:
R1=19∗(1+(k1∗nm1)1+(1+(k1∗nm1)) | (2.3) |
where n is concentration of SIP, m1=6,k1=4.3519×10−4. At the initial stage, since the seeder B cells are assumed to contain a high concentration of SIP (cl=1000), they rapidly divide. SIP and other proteins are disseminated randomly to daughter cells after B cell proliferation. Over time, the cell division rate will decrease with the decrease of intracellular SIP concentration, so B cells need help signals from Tfh cells to synthesize more SIP.
In the simulation, the rate of B cell divisions depends not only on R1, but also on the number of vacancies in a neighborhood of the cell. Because each grid point can only be occupied by a cell of the same type, the number of B cells may seriously affect the rate of its division. For example, when there are eight B cells around a B cell, this B cell will not be able to divide if we assume that each one is fixed to the occupied lattice site. But this assumption is apparently not consistent with experimental observation. In fact, B cells can push other cells away for division. In order to cope with this problem, we assume that in a neighbourhood of a radius of 100 µm, if there are vacancies, the B cell may divide normally and the newly generated daughter-cell will be randomly put into to a vacancy in this neighbourhood.
There is still no clear conclusion on how CBs differentiate into CCs. It has been stated based on experimental observation that the transition is independent of location [43]. It might be triggered by LZ-derived signals such as the receipt of the T cell help. In our model, the differentiation rate is controlled by the concentration of SIP. When the concentration of SIP in CBs was low, CBs began to differentiate into CCs which, neither proliferating nor mutating, express BCR on the surface of the cell membrane. They are prone to an activated state of apoptosis and have a life span of about 6 hours. In the model, the concentration of SIP in CBs determines the differentiation rate
R2=17∗11+k2∗nm2, | (2.4) |
where n is the concentration of SIP in the B cells, m2=5 is Hill's coefficient, k2=0.0102. Proliferation of B cells leads to a decrease of the SIP concentration, which not only promotes the differentiation of CBs into CCs, but also increases the sensitivity of B cells to apoptosis. Therefore, the CCs with higher affinity are able to enter LZ to capture antigens and present them to Tfh cells to reduce the rate of apoptosis. After CCs receive help signals from Tfh cells, they start to synthesize and accumulate the SIP. These selected CCs may differentiate into CBs and return to the DZ, where the mutation and selection is restarted. The differentiation rate R3 of CCs to CBs is
R3=17∗k3∗nm31+k3∗nm3, | (2.5) |
where n is the concentration of the SIP, m3=4 is Hill's coefficient, k3=0.0011.
According to experiments in vivo, CCs survive in the LZ for about 6–16 hours. SHM generates B cells with different affinities that interact with antigens on FDCs. Only those B cells with high affinity effectively seize antigens from FDCs. Those with low affinities are very likely to be eliminated due to their high propensity for apoptosis, which is essential in the regulation of the GC growth during the immune response. If the rate of apoptosis is too high, B cells will all die in a short time so that a high immunity level is not achievable. Conversely, if the rate of apoptosis is too low, B cells will occupy most lattice points in the GC. As a result, the SHM cannot proceed smoothly, and finally slows down the affinity maturation. During the simulation, the state vector of apoptotic B cells will be deleted and removed from the computer memory. In the model, we assume that the apoptotic rate increases with the decrease of SIP, which reads
R4=16∗11+k4∗nm4, | (2.6) |
where n is the concentration of the SIP, m4=5 is the Hill's coefficient, k4=0.05.
FDCs, the most powerful antigen-presenting cells (APCs) in GC, efficiently absorb, process, and present antigens. The presented antigen binds to BCR to form an immunological synapse and plays an important role in activating B cells. B cells with high affinity will endocytose antigens, and then within the B cell, antigens will be decomposed into polypeptide fragments, part of which then bind to the MHC class II molecule to form a complex and are transferred to the B cell surface. These complexes can be recognized by Tfh cells. In the model, CCs enter the LZ, and may assume three states[23]: "un-selected", "contact with antigen" and "selected by antigen".
CCs that are not in contact with antigens on FDCs will not be recognized by Tfh cells. Therefore, the concentration of SIP in B cells do not increase. The apoptosis is determined by the concentration of SIP in B cells, so CCs with low SIP are eliminated by apoptosis at a rate of R4.
The "contact with antigen" CCs successfully contact with antigens on FDCs, but are still waiting to form the antibody-antigen complex. We assume that the binding rate is related to the affinity of the BCR, and the CCs remain bound to the antigens for about 5 minutes. The antigen binding rate R5 is
R5=ka∗e(kb−kc∗δ(ϕ,ϕ∗)) | (2.7) |
where ka=2.696,kb=0.8,kc=0.25. δ(ϕ,ϕ∗) represent the distance between the BCR and the antigen in the shape space δ(ϕ,ϕ∗)=∑4i=1|ϕi−ϕ∗i|.
CCs selected by antigens have successfully ingested antigens from FDCs. In this model, the reaction rate of B cells to take up antigens is related to the affinity, which is
R6=kx∗e(ky−kz∗δ(ϕ,ϕ∗)) | (2.8) |
where kx=0.0056,ky=5.6,kz=0.7. With the highest affinity, a CC will take up one Ag about every 4 minutes. The rate of uptaking antigens from FDCs is larger when the affinity is higher. CCs perform Ag processing and load peptide fragments to the MHC class II molecules to form pMHC complexes on cell surface.
Professor Qi used TIRF microscopy to observe Tfh cells entangled with B cells. During T-B entanglement, two co-stimulatory molecules of ICOS-ICOSL and CD40L-CD40 constitute a positive feedback for improving affinity maturation of BCR[44]. Recently, Shi and Hou [45] identified the role of another pair PD-1-PD-L1, and PD-1 controls the location and function of Tfh cells. PD-1 can damp the TCR signalling and thereby reduce the ligand sensitivity of Tfh cells, which should promote the overall stringency of selection in GCs. In real biological experiments, PD-L1 deficient B cells can get more help from Tfh cells. Based on these data, it was shown that the interaction of PD-1 and PD-L1 could also increase the affinity of BCR. These findings should be reflected in the stochastic model. Similarly, during the simulation, CCs after uptaking antigens could dwell in three different states: "not selected by Tfh cell", "contact with Tfh cells" and "selected by Tfh cells".
CCs that are "not selected by Tfh cells" have not obtained help signal from Tfh cells since entering the LZ. Therefore, these CCs will be difficult to survive unless they contact with a Tfh cell during their wandering in the LZ.
Once CCs have access to a Tfh cell site, they have the chance of switching to the state of contact with the Tfh cell. However, in this situation, no help signals are transmitted from Tfh cells to CCs. The binding rate is regulated by the concentration of the antigen taken by CCs and of the PD-L1 on the surface of the cell. If it has too much PD-L1, the probability of binding will be close to zero. The T-B binding rate R7 is given as:
R7=(ki+kj∗eA−kmkn)∗(w1+wu∗pn) | (2.9) |
where A is the concentration of antigens, ki=10,kj=2,km=10,kn=30. p is the concentration of PD-L1, wu=0.4,n=5,w=10. The binding sites for the antigen and the PD-L1 are independent of each other, so the total rate R7 is a product of these two independent factors.
CCs selected by Tfh cells are mainly those with a high concentration of peptides on the cell surface. When a CC is selected, the MHC complex and ICOS-ICOSL work together to obtain help signals from the Tfh cells. In the model constructed in this paper, the rate R8 of getting the help signal is
R8=F(A)∗F(S)F(A)=KA∗AmA1+KA∗AmAF(S)=c1+KS∗SmS1+KS∗SmS | (2.10) |
where A and S are the concentrations of antigens and ICOSL. The two factors F(A) and F(S) indicate the action from the antigen and ICOSL, respectively, which are assumed to work independently here. mA=8 and mS=2 are Hill's coefficients. The Constants: KA=6×10−4,Ks=4,c1=18.5. The T-B interaction allows CCs procuring help signals from Tfh cells and then synthesizing SIP with a rate of 1hr−1. Therefore, CCs with higher affinity have lower apoptotic rate, which live longer in the GC.
B cell's membrane protein ICOSL serves to increase the T-B interaction, since the ICOSL-ICOS and CD40L–CD40 pairs make a positive feedback loop prompting generation of more help signals[32]. In the model, the synthesis rate R9 of membrane protein ICOSL depends on the SIP in B cells
R9=c2∗k9∗nm91+k9∗nm9 | (2.11) |
where c2 is the average synthesis rate, about 12hr−1. n is the concentration of SIP and m9=4,k9=4×10−4. The average degradation rate of ICOSL is set to 0.083hr−1. PD-1 expressed in Tfh cells and PD-L1 expressed in B cells hinders their contact. In vivo, the interaction between PD-1 and PD-L1 helps maintain the strictness of affinity-based selection. In the current model, the synthesis rate R10 of PD-L1 depends on the concentration of the antigens
R10=P1+p2∗nm10 | (2.12) |
where n is the concentration of antigens. The highest synthesis rate of PD-L1 is P=0.03, and m10=4, p2=2×10−4.
CCs with high affinity in the LZ is more likely to undergo multiple selection and mutation cycles, which may differentiate into plasma or memory cells, and leave the GC within 7 hours. In the model, the rate R11 at which CC differentiates into plasma or memory cells is determined by the concentration of SIP
R11=17∗k11∗nm111+k11∗nm11 | (2.13) |
where n is the concentration of the SIP, m11=4 is the Hill's coefficient and k11=0.0011. CCs may differentiate into plasma or memory cells when the concentration of SIP is high enough. At the same time, in the literature [12,25], the probability of "CC going back to CB" is four times that of "CC differentiation into plasma or memory cell". In our model, the antibody secreted by plasma cells is assumed not affecting the immune response in GC. Once the plasma or memory cells are produced, they leave the GC straightforwardly and the corresponding state vectors are deleted.
The parameters in the above formula were described in detail in the literature [32], and the initial conditions of the model are listed in Table 2.
Parameter | 2D | 3D | Illustration | References |
Lattice spacing | 10 µm | 10 µm | Radius of B cell is about 5 µm | - |
Radius of GC | 200 µm | 150 µm | - | - |
Number of seeder cells | 3 | 3 | About three cells enter the follicle. | [29][46][47] |
Shape space dimension | 4 | 4 | Range of affinity (0–1) | [9][48] |
Number of T cells | 100 | 2500 | - | [49] |
T cell moving speed | 10.8 µm/min | 10.8 µm/min | - | [50][51] |
Number of FDCs | 20 | 225 | - | [32] |
Length of FDC arms | 20 µm | 20 µm | - | [32] |
Basic rate of proliferation | 1/9 h | 1/6 h | - | [52] |
CB moving speed | 1.5 µm/min | 1.5 µm/min | - | [50][51] |
Basic rate of CB differentiation | 1/7 h | 1/4.7 h | - | [20] |
CC moving speed | 5 µm/min | 5 µm/min | - | [50][51] |
Tfh cells moving speed | 10.8 µm/min | 10.8 µm/min | - | [50][51] |
Probability of recycling for selected CC | 0.8 | - | - | [53] |
Basic rate of CC differentiation | 1/6 h | 1/4 h | - | [52] |
Basic rate of FDC-CC dissociation | 1/0.1 h | 1/0.1 h | - | |
Basic rate of FDC-T dissociation | 1/0.5 h | 1/0.5 h | - | [54] |
Synthesis Rate of SIP | 1 h | 1 h | After CBs obtain help signals from Tfh cells | [32] |
We analyze the spatiotemporal stochastic model based on the Gillespie algorithm to study the immune response in the GC within 21 days. In silico, we can easily change the morphology of GC and analyze its impact on affinity maturation [55,56]. The GC space is a 120×120 lattice with the grid spacing being 10 µm, which was divided into two parts: the paracortex area and the primary follicle area (Figure 3A).
At the beginning of the simulation, T and B cells are activated by chemokines and move to the T-B border. Later, the B cells are further activated by T cells and move to the primary follicle to initiate the GC growth. At this time, FDCs start to be deployed in a polarized manner. Naïve B cells in the primary follicle make up the boundary of the GC as bystanders. With the proliferation and differentiation of B cells (Figure 3B), the LZ and DZ gradually appear in the GC, where the chemokines CXCL12 and CXCL13 play important roles. The positioning of CBs in the DZ depends on the expression of the CXCL12 receptors, CXCR4[37]. When CBs differentiate into CCs, the CXCR5, the receptor of CXCL13, promotes CCs motility towards the LZ. (Figure 3A–C: Simulation diagram in silico, T cells (blue), naïve B cells (red), FDC (green), CBs (black), CCs (pink)).
Signal integrator protein (SIP), as an important molecule in our model, is involved in the whole immune response process. So we plot the total SIP concentration and the average over time in different cells in Figure 4. B cells include CCs and CBs. The total SIP concentration in (a) initially decreases rapidly due to apoptosis and then increases as CCs receive help signals from Tfh cells, starting to produce SIP. The produced SIP (b) produced is slightly higher than the loss due to apoptosis and the differentiation into plasma or memory cells. The total concentration (c) and the average (d) in CBs also decrease rapidly at the early stage due to apoptosis and the differentiation into CCs, but remain stable afterwards because CCs start to return to the DZ and become CBs. As CBs turn CCs and leave the DZ, the total concentration (e) in CCs rapidly increases initially, and and even the average concentration in CCs (f) keeps increasing since the average affinity increases over time as expected.
In our model, we take a new factor into consideration, the effect of the interaction between PD-1 and PD-L1. We investigate the function of the PD-1 and PD-L1 interaction between Tfh cells and B cells. As can be seen from Figure 5, compared to the previous model, the proportion of high-affinity B cells increases in the presence of the PD-1-PD-L1 molecules, together with the average affinity of the output B cells. The PD-1 acts to dampen the TCR signaling and thereby reduce the ligand sensitivity of the Tfh cells, which promotes the stringency of affinity-based selection. Correspondingly, due to the strict selection, a large number of B cells undergo apoptosis and the number of output B cells is reduced. These data highlight the importance of the PD-1 for the innate immune response.
GCs are organized into two functionally distinct compartments: the DZ, where B cells divide and undergo somatic hypermutation, and the LZ, where they are selected for affinity-enhancing mutations after interacting with Tfh cells. Both GC zones experience very high rate of apoptosis [57]. In the primary follicle, the FDC is in the central region. At the T-B border, there are many CRCs interlaced with FDCs. When GC starts to take shape, FDCs stay in a polarized distribution, located in the LZ. In the DZ, there are mostly CRCs. This kind of distribution in the GC is observed [43]. Therefore, it is natural to ask, if polarization is eliminated, that is, if the light and dark zone disappear and CBs and CCs mix with each other, what will happen to the affinity maturation?
In 2013, Bannard et al. [43] used CXCR4-deficient GC B cells, confined in the LZ, to confirm that the differentiation from CBs to CCs is independent of their position. He also provided strong evidence that the spatial separation of LZ and DZ is critical to maintain an effective GC response. In biological experiments, CXCR4-deficient GC B cells have fewer accumulations of genetic mutations, mainly because there is less AID (activation-induced cytidine deaminase) in LZ than in DZ cells. The physiological function of AID is to introduce point mutation in the variable region during SHM. In our model, we may assume a uniform concentrations of AID in GC cells to ensure an equal mutation rate everywhere, so that the impact of AID can be eliminated.
The degree of mixing of CBs with CCs is described with a mixing index Ip that counts the number of CCs in a radius of 20 µm around the CBs under investigation. By changing the distance between the FDCs and the GC center, the mixing index between CBs and CCs is varying. When FDCs are in the center of the GC, the mixing index is the greatest, but when FDCs are maximally polarized, CCs and CBs are separated to the maximum extent, naturally forming the LZ and DZ in GC.
As can be seen from the GC volume change in Figure 6A, on the tenth day, the number of B cells in a GC without partition (Figure 6A black line) is much smaller than that with spatial polarization (Figure 6A red line). It is because that B cells inside this type of GCs do not compete properly. Because of the mixing, CBs may occupy the sites of FDCs, and due to the crowdedness in the lattice, CCs cannot walk freely, limiting their opportunities to acquire antigens from FDCs, causing excessively senseless competition between CBs and CCs. As the mixing degree Ip is getting higher, the average affinity of the output plasma cells keeps decreasing (Figure 6B), which indicates the importance of the polarization of FDCs in the LZ. Together, they separate SHM and mitotic process from selection, resulting in a reasonable and effective competition between CCs.
In the following, we discuss the impact of GC volume on affinity maturation. In the simulation, based on experimental observations, GCs assume typical cellular components which remain stable at certain growth stage [31]: the density of FDCs and the number of Tfh cells in the GC keep constant. Six different radii, 50,100,150,200,300,400 µm, are used in the simulation which produce different affinities for the output cells. From Figure 7A, we see that a radius of 200 µm is most suitable for affinity maturation. A phase transition is observed at a radii between 100 and 150 µm where the standard deviation of the affinity also reaches a maximum, indicating a maximal fluctuation. With further increase of the radius, the affinity is rising mildly, while the standard deviation declines, and the simulation results become more and more stable. When the radius exceeds an optimal value, the affinity starts to fall gradually. As shown in Figure 7C, when the volume increases, the SHM drives a large fraction of mutations, pushing the genotypes of B cells away from the target epitope of the antigen, increasing the proportion of low-affinity B cells and making it more difficult to select high-affinity CCs.
To explore the nature of the phase transition, we simulate the development and affinity maturation of the GC with deterministic dynamics as shown in Figure 8A. With low concentration of SIP, the volume of the GC decays to almost zero with time and only reaches a stable volume at high concentrations. The higher the concentration of SIP, the larger the size of the GC (as shown in Figure 8A). A jump of the affinity is observed when the concentration of SIP in initial B cells is 228, where we clearly see a phase transition (Figure 8B) which sees about 110 B cells in the GC around the third day, being the largest during the whole growth. Similar results are obtained for a critical radius of 100 µm in the stochastic model (Figure 8C). As can be seen from Figure 9, the boundary (black line) of the basin of attraction divides the phase space into two different regions. If the concentration of SIP (which dictates the volume of the GC) and affinity in initial B cells are located in the green region, the affinity maturation is successfully achieved. On the contrary, in the red region, the affinity is basically unchanged. It is clear that if the initial affinity is low, a large volume is required and alternatively a small volume needs high initial affinity to complete the maturation process.
The existence of the phase transition could also be explained qualitatively in a straightforward manner. If the number of CBs generated by divisions is too small, there is not enough mutation accumulation to produce cells with high affinity. As a result, these B cells cannot get enough help from Tfh cells and most of them will execute apoptosis, so the GC eventually collapses. In order to achieve affinity maturation, the GC needs a sufficient number of B cells undergoing SHM. The phase transition point pins down the lower bound of this number in average.
Below, we will investigate what controls the critical number of B cells at the phase transition. In the stochastic model, the initial B cells are assumed to have a BCR repertoire(1, 1, 1, 1), the distance between the BCR repertoire and the target epitope of the antigen is δ(ϕ,ϕ∗)=4. This distance plays an important role in determining the critical GC volume as will be seen next. If we set the initial BCR repertoire to (1, 1, 0, 0) and (2, 2, 2, 2) respectively in the stochastic model, the distance to the target epitope will be δ(ϕ,ϕ∗)=2 and 8. As can be seen from Figure 10A, B, the affinities in these two cases do not reach the same level. When δ(ϕ,ϕ∗)=2, even if the volume of the GC is very small, the average affinity of output cells is greater than 0.5. Moreover, the phase transition is not very obvious, indicating that there is not much constraint on the volume in this case. However, when δ(ϕ,ϕ∗)=8, the maximum affinity is 0.2, achieved at a radius as large as 400 µm. With δ(ϕ,ϕ∗)=2 increasing to δ(ϕ,ϕ∗)=4, the GC radius to produce the highest average affinity increases from 150 µm to 200 µm. Therefore, we may draw the conclusion that the critical GC volume depends on the distance δ(ϕ,ϕ∗), which may be checked experimentally.
Recently, J. Tas [58] and R. Murugan et al. [59] showed that volumes of early GCs are highly diversified, including hundreds of different B cell clones, which is possibly accounted for by in vivo seeding GC precursor cells with high or low affinity. In the simulation above, the precursor cells are not considered. However, due to the high frequency mutation rate, the B cells themselves at the early stage could be highly diversified. Some experiments[30,31] have confirmed that most of the GCs in the lymph nodes are very small. According to our finding, if the initial B cells have relatively high affinity, the corresponding GCs produce high affinity plasma and memory cells with meager resources. On the contrary, those with lower affinity require a larger volume of GC for maturation, because a large number of mutations are needed to get high affinity. In order to save resources and produce high affinity plasma and memory cells, it is highly advantageous for the initial B cells to enjoy a high level of affinity[60]. This also explains Schwickert's [61] data that competition from high affinity B cells prevents low affinity ones from activation, proliferation and eventually reaching the pre-GC stage.
When the affinity of initial activated B cells is low, it is important to find a mechanism to ensure the production of high affinity plasma cells. In biological experiments, it has been observed that GCs of different sizes grow in lymph nodes, between which B cells migrate, and rare high-affinity precursors participate in the formation of GC. Therefore, it is reasonable to speculate that some B cells with high affinity may be able to move to neighboring GCs to help them grow and promote the production of high affinity plasma cells. To verify this hypothesis, we initially supply additional B cells with high affinity and check the affinity of the output cells in the stochastic model. In one trial, five B cells with an affinity of 0.24 are put to the follicle in addition to the original three B cells with an affinity of 0.06. From Figure 11A, we see that the affinity of the output plasma cells is 0.35, much higher than 0.2, the affinity achieved without this additional input. However, if we change the affinity of these five B cells from 0.24 to 0.49 (Figure 11B), the affinity of plasma cells is improved further to 0.54 and the standard deviation becomes much smaller, which indicates that the affinity improvement most likely results from the newly added cells. From the results of Figure 11, it is reasonable to say that B cells with high affinity or precursor cells entering other GCs help produce high affinity output cells, thus contributing to the collective maturation in the whole lymphoid.
All previous simulations are carried out on a two-dimensional lattice. However, realistic biological experiments are performed and observed in three-dimensional space. Therefore, whether the difference in dimensions would significantly change the results, remains to be answered. First, we check the change of reaction rates going from 2D to 3D. In Meyer-Hermann's article [62], the values of the parameters in the 3D model are directly computed from the 2D. The proliferation rate, differentiation rate, etc. must be multiplied by 3/2 to ensure the results from two simulations are comparable, while the concentrations of FDCs and Tfh cells in GC should be kept constant. As a result, much more FDCs and T cells ( = 2700) are needed in three dimensions. However, the scaling of the number of B cells is not so obvious and it is hard to make a direct comparison. So a normalization of the B cell number is needed. As can be seen from Figure 12B, however, after the normalization the number of B cells in the GC in the 3D simulation is still nearly 20% less than that in 2D with comparable conditions. The main reason is that, in our simulation the number of B cells in the 3D is nearly 15 times of that in 2D. As a result even if the shape space and the mutation rate is the same, the mutation range in the shape space of B cells expands. Many B cells stride randomly to a region with very low affinity, so the affinity distribution of B cells changes. As shown in Figure 12D, the proportion with affinity below 0.06 in 3D is greater than that in 2D. As a result, many B cells die quickly because their affinity is too low to get help from Tfh cells. However, if we continue to increase the number of T cells outside the GC from 2700 to 6000 in 3D, arriving at a higher concentration than in 2D, B cells start to get more help, reaching a quantity comparable to that in the 2D case. This also explains why large volume of the GC in Figure 7A and Figure 7C leads to a decrease in the affinity.
In the 3D simulation, we also check how the radius of the GC alter the affinity maturation. As can be seen from Figure 7B, a phase transition occurs at a radius of 50 µm, where the fluctuation rises significantly compared to the 2D case. With a radius of 35 µm, the peak number of B cells in the GC is also about 110, being consistent with the results from the 2D simulation (Figure 8D) and the deterministic model. Therefore, if the number of B cells is too small, the resulting few mutations are unable to support a sufficient exploration of the shape space so that affinity maturation could not be achieved before the decay of the GC.
Based on the latest experimental observations, we established a stochastic spatiotemporal model to explore the affinity maturation for adaptive immunity. FDCs present antigens in the form of immune complexes to the naive B cells and allure CCs to compete for antigens. The morphology of the LZ and DZ plays a very important role in this process. When FDCs are distributed in an unpolarized way, the GC will decay and disappear quickly, so that the affinity level of the output plasma or memory cells will be very low. By slowly increasing the polarization of FDCs in the simulation, the structure of the light and the dark zone gradually appears, and the function of affinity maturation gradually recovers, which does show the importance of polarization in the follicle. However, in the process of simulation, because of cell crowdedness, we assume that cellular movement may sometimes be blocked. How much this assumption is consistent with experimental observations remains to be explored.
Next, we check how the volume of a GC affects the affinity maturation and whether there is an optimal GC volume. By analyzing the average affinity of differentiated plasma and memory cells in both stochastic and deterministic simulations, we find that with the increase of the volume, the average affinity of output cells undergoes a phase transition, and only beyond the critical volume affinity maturation is achieved. It turns out that the distance δ(ϕ,ϕ∗) in the shape space between the activated B-cell and the target epitope of antigen determines the phase transition point. Assuming that the seeder B cells that generate GCs do not contain precursor cells with high affinity, the size of the GC is a good readout of a successful antibody response, and only those GCs that exceed a critical volume will achieve affinity maturation. Conversely, different GC volumes observed in the lymph node may be used as an indicator of this distance if the communication of B cells between GCs could be excluded. Overall, if the number of cell divisions during high frequency mutation is too small to produce enough strains with different affinities, a devastating impact on the subsequent affinity maturation will result.
In this paper, we only consider the case with single well-defined antigens and emphasize the roles of the GC volume, FDC polarity and specific protein factors. Thus, B cells in GC only need to cope with the single target antigen rather than multiple or complex antigens. When multivalent antigens are considered in future work, the benefits of cocktail immunization must be considered in silico. The detailed interaction between antigen and antibody molecules has to be included in the extension of the model, just as done in a recent investigation [63].
The affinity of naive B cells is assumed very low, being set at 0.06. By the end of the maturation process, the mean affinity of all generated plasma and memory cells rises to a value as high as 0.45. However, biological experiments observed that the affinity of plasma cells is about 10 times that of the naive B cells, which suggests other mechanisms at work in the GC, yet to be explored in the future.
This work was supported by the National Natural Science Foundation of China under Grants No. 11775035, and also by the Fundamental Research Funds for the Central Universities with contract number 2019XD-A10.
The authors declare there is no conflict of interest.
Based on the stochastic model described in materials and methods, a series of equations can be written down to describe the averaged dynamics in the GC. The deterministic model does not consider the space dimension, and uses the number of B cells to indicate the volume of the GC. In the deterministic model, we set the affinity of BCR to 10 different levels (0–9) from low to high, which determines the binding rate of B cells to antigens. Correspondingly, the shape space is simplified to a one-dimensional lattice with 10 sites (0–9), over which cells with specific BCR move randomly with equal probability in either direction except at the level 0 and 9 where moving in only one direction is possible. B cells rapidly clone at the early stage, producing a large number of offsprings with different affinities. In the deterministic model, we coarse-grain the states of B cells, assuming that B cells with the same affinity level have the same properties, such as the rate of division, the rate of differentiation, and the ability to bind to the antigen, etc. The affinities in the initial B cells are assumed to be zero. The explanation of symbols and their values in the model are displayed in Table A1.
symbol | meaning | value | References |
n0 | The total number of FDC sites | 80 | [32] |
i | The level of affinity | 0–9 | [12] |
CCiFDC | The number of CCs with affinity level i that are binding with FDCs | - | |
nFDC | FDC sites that have not been occupied by CCs | - | |
T0 | The total number of activated T cells | 20 | [32] |
T | The number of T cells that are not contact with CCs | - | |
Bi | The number of CBs with affinity level i | - | |
mr | The probability of no mutation | 0.5 | [20][32] |
Pi | The total concentration of SIP with affinity level i | - | |
TRi | The number of CCs with affinity level i that have been rescued by T cells | - | |
FRiT | The number of CCs with affinity level i that are binding with Tfh cells | - | |
Rc | The probability of CCs' recycling into the DZ | 0.8 | [21] |
ΣBi | The sum of the numbers of CCs and CBs with affinity level i | - | |
Pi/ΣBi | The average SIP concentration in a B cell at level i | - | |
R1,R2,R3,R4,R5,R6 | Defined in Materials and Methods | - | |
CCi | The number of CCs with affinity level i | - | |
ENCF | The possibility of CCs encountering with FDC sites | 0.0001 | assumed |
kr | The dissociation rate of CCs with FDCs | 0.01 | assumed |
ka | The degradation rate of ingested antigens in CCs | 1 | [32] |
Agi | The ingested antigens by CCs with level of affinity i | - | |
FRi | The number of CCs with affinity level i that had contacted with FDC sites | - | |
ENCT | The possibility of CCs encountering with Tfh cells. | 0.0001 | assumed |
SPi | The concentration of help signals in B cells with affinity level i. | - | |
ks | The degradation rate of help signals in FRi | 1 | [32] |
PBi | The number of output cells of different affinity levels, that is, plasma or memory cells | - | |
kp | The degradation rate of total concentration of SIP | 1 | [32] |
Next, we write down all the equations and explain each of them below.
nFDC=n0−9∑i=0[CCiFDC] | (A.1) |
which describes the change of the FDC sites. n0(=80) is the total number of FDC sites and nFDC is the number of sites that are not been occupied by CCs. CCiFDC is the number of CCs with affinity level i and binding with FDC.
T=T0−9∑i=0[CCiTfh] | (A.2) |
where T0(=20) is the total number of Tfh cells and T is the number of Tfh cells that are not in contact with CCs. CCiTfh is the number of CCs with an affinity level i and binding with Tfh cells.
dBidt=mr∗R1(Pi/ΣBi)∗Bi+mr2∗R1(Pi−1/ΣBi−1)∗Bi−1+mr2∗R1(Pi+1/ΣBi+1)∗Bi+1−R2(Pi/ΣBi)∗Bi+R3(Pi/ΣBi))∗Rc∗TRi | (A.3) |
which describes the process of B cell proliferation and differentiation. Bi is the number of CBs with affinity level i. The probability of each mutation is mr. Pi is the sum of the concentration of SIP in cells with affinity level i. TRi is the number of CCs rescued by Tfh cells with an affinity level of i. These CCs either differentiate into plasma cells or recycle into the DZ to continue division. The Rc(=0.8) is the probability of CCs' recycling into the DZ. ΣBi is the sum of the numbers of CCs and CBs with an affinity level i, so the average SIP concentration in a B cell at level i is Pi/ΣBi, which then give the rates R1,R2,R3 defined in materials and methods.
dCCidt=R2(Pi/ΣBi)∗Bi−R4(Pi/ΣBi)∗CCi−ENCF∗R5(i)∗CCi∗nFDC | (A.4) |
which describes the death of CCs and its interaction with FDCs. CCi is the number of CCs with affinity level i. ENCF (=0.0001) is the possibility of CCs encountering with FDC sites. The rates R4,R5 are functions of the SIP concentration and defined in Materials and Methods.
dCCiFDCdt=ENCF∗R5(i)∗CCi∗nFDC−kr∗CCiFDC | (A.5) |
dAgidt=R6(i)∗CCiFDC−ka∗Agi | (A.6) |
which describe the binding of CCs with FDCs and the change of the antigen concentration in CCi. kr(=0.01) is the dissociation rate of CCs with FDCs. ka(=1) is the degradation rate of ingested antigens. Agi is the concentration of the ingested antigens by CCs with affinity level i. R6 is a rate defined in materials and methods.
dFRidt=kr∗CCiFDC−R4(Pi/ΣBi)∗FRi−ENCT∗R7(Agi)∗FRi∗T | (A.7) |
where FRi is the number of CCs with affinity level i that had contacted with FDC sites and ENCT (=0.0001) is the probability of CCs encountering with Tfh cells.
In the deterministic model, the positive feedback of ICOS-ICOSL and the impact of PD-L1-PD-1 molecular pairs are not considered. Although these molecular pairs contribute to affinity maturation, the decisive factor is still the concentrations of antigens engulfed by CCs. Therefore, this model is not identical with the stochastic model and the impact of molecular pairs is not considered. As in the stochastic model, the higher the antigen concentration, the faster the binding rate of CCs to Tfh cells. The reaction rates R7 and R8 :
R7=Ki+Kj∗eA−KmKnR8=(KA∗AmA1+KA∗AmA)∗c1 |
where A is the concentration of antigens, Ki=10,Kj=2,Km=10,Kn=30. mA=8 is Hill's coefficient, KA=6×10−4,c1=18.5.
dFRiTdt=ENCT∗R7(Agi)∗FRi∗T−kT∗FRiT | (A.8) |
dSPidt=R8(Agi)∗TRi−ks∗SPi | (A.9) |
which describe the binding of CCs with Tfh cells and the help signals from Tfh cells. kT(=0.05) is the dissociation rate of CCs from the Tfh cells. FRiT is the number of CCs with affinity level i that are binding with Tfh cells. SPi is the concentration of help signals in CCs. ks(=1) is the degradation rate of help signals in TRi.
dTRidt=kT∗FRiT−R4(Pi/ΣBi)∗TRi−R3(Pi/ΣBi)∗TRi | (A.10) |
dPBidt=R3(Pi/ΣBi)∗(1−Rc)∗TRi | (A.11) |
which describe the process of CCs differentiating into CBs and into memory or plasma cells. PBi represents output cells with different affinity levels, namely plasma cells and memory cells.
dPidt=R11(SPi)−R4(Pi/ΣBi)∗CCi∗Pi−kp∗Pi+mr2∗R1(Pi+1/ΣBi+1)∗Bi+1∗Pi+1+mr2∗R1(Pi−1/ΣBi−1)∗Bi−1∗Pi−1−mr∗R1(Pi/ΣBi)∗Bi∗Pi | (A.12) |
which describes the concentration changes of SIP. kp is the degradation rate of total concentrations of SIP. In this model, the synthesis rate of SIP R11 is affected by the concentration of help signals in CCs:
R11=c3+KB∗SPmB1+KB∗SPmB | (A.13) |
where SP is the concentration of help signals and Hill's coefficient mB=4. The constant KB=4×10−4,c3=1.
[1] |
Bruckner R (1971) Properties and structure of vitreous silica. Ⅱ. J Non-Cryst Solids 5: 177–216. https://doi.org/10.1016/0022-3093(71)90032-9 doi: 10.1016/0022-3093(71)90032-9
![]() |
[2] | Varshneya AK, Mauro JC (2019) Fundamentals of Inorganic Glasses, 3rd Eds, Amsterdam: Elsevier. https://doi.org/10.1016/C2017-0-04281-7 |
[3] | Doremus RH (1994) Glass Science, 2nd Eds, New Jersey: John Wiley & Sons, Inc. |
[4] | Shelby JE (2005) Introduction to Glass Science and Technology, 2nd Eds, Cambridge: The Royal Society of Chemistry. |
[5] |
Elsey HM (1926) The diffusion of helium and hydrogen through quartz glass at room temperature. J Am Chem Soc 48: 1600–1601. https://doi.org/10.1021/ja01417a501 doi: 10.1021/ja01417a501
![]() |
[6] |
Norton FJ (1953) Helium diffusion through glass. J Am Chem Soc 36: 90–96. https://doi.org/10.1111/j.1151-2916.1953.tb12843.x doi: 10.1111/j.1151-2916.1953.tb12843.x
![]() |
[7] |
Norton FJ (1957) Permeation of gases through solids. J Appl Phys 28: 34–39. https://doi.org/10.1063/1.1722570 doi: 10.1063/1.1722570
![]() |
[8] |
McAfee KB (1958) Stress‐enhanced diffusion in glass. Ⅰ. Glass under tension and compression. J Chem Phys 28: 218–226. https://doi.org/10.1063/1.1744096 doi: 10.1063/1.1744096
![]() |
[9] |
Leiby CC, Chen CL (1960) Diffusion coefficients, solubilities, and permeabilities for He, Ne, H2, and N2 in Vycor glass. J Appl Phys 31: 268–274. https://doi.org/10.1063/1.1735556 doi: 10.1063/1.1735556
![]() |
[10] |
Shelby JE (1973) Effect of phase separation on helium migration in sodium silicate glasses. J Am Ceram Soc 56: 263–266. https://doi.org/10.1111/j.1151-2916.1973.tb12484.x doi: 10.1111/j.1151-2916.1973.tb12484.x
![]() |
[11] |
Shelby JE (1974) Helium diffusion and solubility in K2O-SiO2 glasses. J Am Ceram Soc 57: 260–263. https://doi.org/10.1111/j.1151-2916.1974.tb10883.x doi: 10.1111/j.1151-2916.1974.tb10883.x
![]() |
[12] |
Hacarlioglu P, Lee D, Gibbs GV, et al. (2008) Activation energies for permeation of He and H2 through silica membranes: An ab initio calculation study. J Membrane Sci 313: 277–283. http://doi.org:10.1016/j.memsci.2008.01.018 doi: 10.1016/j.memsci.2008.01.018
![]() |
[13] |
Shackelford JF (2014) Gas solubility and diffusion in oxide glasses—implications for nuclear wasteforms. Procedia Mater Sci 7: 278–285. http://doi.org:10.1016/j.mspro.2014.10.036 doi: 10.1016/j.mspro.2014.10.036
![]() |
[14] |
Yoshioka T, Nakata A, Tung KL, et al. (2019) Molecular dynamics simulation study of solid vibration permeation in microporous amorphous silica network voids. Membranes 9: 132. http://doi.org:10.3390/membranes9100132 doi: 10.3390/membranes9100132
![]() |
[15] |
Cheng S (2017) A nanoflake model for the medium range structure in vitreous silica. Phys Chem Glasses-B 58: 33–40. https://doi.org/10.13036/17533562.57.2.104 doi: 10.13036/17533562.57.2.104
![]() |
[16] |
Cheng S (2022) Two-stage model of silicate glass transition. GJSFR 22: 1–9. http://doi.org/10.34257/GJSFRAVOL22IS7PG1 doi: 10.34257/GJSFRAVOL22IS7PG1
![]() |
[17] |
Zachariasen WH (1932) The atomic arrangement in glass. J Am Chem Soc 54: 3841–3851. https://doi.org/10.1021/ja01349a006 doi: 10.1021/ja01349a006
![]() |
[18] |
Warren BE (1934) X-ray determination of the glass structure. J Am Ceram Soc 17: 249–254. https://doi.org/10.1111/j.1151-2916.1934.tb19316.x doi: 10.1111/j.1151-2916.1934.tb19316.x
![]() |
[19] |
Warren BE, Biscoe J (1938) Fourier analysis of X-ray patterns of soda-silica glass. J Am Ceram Soc 21: 259–265. https://doi.org/10.1111/j.1151-2916.1938.tb15774.x doi: 10.1111/j.1151-2916.1938.tb15774.x
![]() |
[20] | Cheng S (2022) Research Advancements in the Field of Materials Science, London: Index of Sciences Ltd. |
[21] |
Cheng S (2023) The origin of anomalous density behavior of silica glass. Materials 16: 6218. https://doi.org/10.3390/ma16186218. doi: 10.3390/ma16186218
![]() |
[22] |
Rayleigh L (1936) Studies on the passage of helium at ordinary temperature through glasses, crystals, and organic materials. P Roy Soc A-Math Phy 156: 350–357. https://doi.org/10.1098/rspa.1936.0152 doi: 10.1098/rspa.1936.0152
![]() |
[23] |
Rayleigh L (1937) An attempt to detect the passage of helium through a crystal lattice at high temperatures. P Roy Soc A-Math Phy 163: 376–380. https://doi.org/10.1098/RSPA.1937.0233 doi: 10.1098/RSPA.1937.0233
![]() |
[24] |
Greaves GN (1985) EXAFS and the structure of glass. J Non-Cryst Solids 71: 203–217. https://doi.org/10.1016/0022-3093(85)90289-3 doi: 10.1016/0022-3093(85)90289-3
![]() |
[25] |
Greaves GN, Fontaine A, Lagarde P, et al. (1981) Local structure of silicate glasses. Nature 293: 611–616. https://doi.org/10.1038/293611a0 doi: 10.1038/293611a0
![]() |
[26] |
Ojovan MI (2021) The modified random network (MRN) model within the configuron percolation theory (CPT) of glass transition. Ceramics 4: 121–134. https://doi.org/10.3390/ceramics4020011 doi: 10.3390/ceramics4020011
![]() |
[27] |
Israelachivili JN (1981) The force between surfaces. Philos Mag A 43: 753–770. http://doi.org/10.1080/01418618108240406 doi: 10.1080/01418618108240406
![]() |
[28] | Shelekhin AB, Dixon AG, Ma YH (1995) Theory of gas diffusion and permeation in inorganic molecular-sieve membranes. Aiche J 41: 58–67. https://aiche.onlinelibrary.wiley.com/doi/10.1002/aic.690410107 |
[29] | Royal Society of Chemistry (2024) Available from: https://www.rsc.org/periodic-table/element/2/helium. |
[30] |
Griffith A (1921) The phenomena of rupture and flow in solids. Philos T R Soc A 221: 163–198. https://doi.org/10.1098/rsta.1921.0006 doi: 10.1098/rsta.1921.0006
![]() |
[31] |
Otto WH (1955) Relationship of tensile strength of glass fibers to diameter. J Am Ceram Soc 38: 122–124. https://doi.org/10.1111/j.1151-2916.1955.tb14588.x doi: 10.1111/j.1151-2916.1955.tb14588.x
![]() |
No. | Name of vector entries | Notes |
1 | Cell type | CBs/CCs/Tfh |
2 | Shape space coordinates | - |
3 | Real space coordinates | - |
4 | Moving speed | - |
5 | Proliferation rate | - |
6 | Differentiation rate | - |
7 | Apoptosis rate | - |
8 | Status marker-01 | CC-FDC |
9 | Status marker-01 | CC-T |
10 | Molecular concentration-01 | ICOSL |
11 | Molecular concentration-02 | PD-L1 |
12 | Molecular concentration-03 | ICOS |
13 | Molecular concentration-04 | SIP |
14 | Molecular concentration-06 | uptaken antigens from FDCs |
15 | Molecular concentration-07 | help signals |
16 | Molecular concentration-08 | pMHC |
17 | Synthetic rate or degradation rate of | SIP/help signal/ICOSL/PD-L1 |
Parameter | 2D | 3D | Illustration | References |
Lattice spacing | 10 µm | 10 µm | Radius of B cell is about 5 µm | - |
Radius of GC | 200 µm | 150 µm | - | - |
Number of seeder cells | 3 | 3 | About three cells enter the follicle. | [29][46][47] |
Shape space dimension | 4 | 4 | Range of affinity (0–1) | [9][48] |
Number of T cells | 100 | 2500 | - | [49] |
T cell moving speed | 10.8 µm/min | 10.8 µm/min | - | [50][51] |
Number of FDCs | 20 | 225 | - | [32] |
Length of FDC arms | 20 µm | 20 µm | - | [32] |
Basic rate of proliferation | 1/9 h | 1/6 h | - | [52] |
CB moving speed | 1.5 µm/min | 1.5 µm/min | - | [50][51] |
Basic rate of CB differentiation | 1/7 h | 1/4.7 h | - | [20] |
CC moving speed | 5 µm/min | 5 µm/min | - | [50][51] |
Tfh cells moving speed | 10.8 µm/min | 10.8 µm/min | - | [50][51] |
Probability of recycling for selected CC | 0.8 | - | - | [53] |
Basic rate of CC differentiation | 1/6 h | 1/4 h | - | [52] |
Basic rate of FDC-CC dissociation | 1/0.1 h | 1/0.1 h | - | |
Basic rate of FDC-T dissociation | 1/0.5 h | 1/0.5 h | - | [54] |
Synthesis Rate of SIP | 1 h | 1 h | After CBs obtain help signals from Tfh cells | [32] |
symbol | meaning | value | References |
n0 | The total number of FDC sites | 80 | [32] |
i | The level of affinity | 0–9 | [12] |
CCiFDC | The number of CCs with affinity level i that are binding with FDCs | - | |
nFDC | FDC sites that have not been occupied by CCs | - | |
T0 | The total number of activated T cells | 20 | [32] |
T | The number of T cells that are not contact with CCs | - | |
Bi | The number of CBs with affinity level i | - | |
mr | The probability of no mutation | 0.5 | [20][32] |
Pi | The total concentration of SIP with affinity level i | - | |
TRi | The number of CCs with affinity level i that have been rescued by T cells | - | |
FRiT | The number of CCs with affinity level i that are binding with Tfh cells | - | |
Rc | The probability of CCs' recycling into the DZ | 0.8 | [21] |
ΣBi | The sum of the numbers of CCs and CBs with affinity level i | - | |
Pi/ΣBi | The average SIP concentration in a B cell at level i | - | |
R1,R2,R3,R4,R5,R6 | Defined in Materials and Methods | - | |
CCi | The number of CCs with affinity level i | - | |
ENCF | The possibility of CCs encountering with FDC sites | 0.0001 | assumed |
kr | The dissociation rate of CCs with FDCs | 0.01 | assumed |
ka | The degradation rate of ingested antigens in CCs | 1 | [32] |
Agi | The ingested antigens by CCs with level of affinity i | - | |
FRi | The number of CCs with affinity level i that had contacted with FDC sites | - | |
ENCT | The possibility of CCs encountering with Tfh cells. | 0.0001 | assumed |
SPi | The concentration of help signals in B cells with affinity level i. | - | |
ks | The degradation rate of help signals in FRi | 1 | [32] |
PBi | The number of output cells of different affinity levels, that is, plasma or memory cells | - | |
kp | The degradation rate of total concentration of SIP | 1 | [32] |
No. | Name of vector entries | Notes |
1 | Cell type | CBs/CCs/Tfh |
2 | Shape space coordinates | - |
3 | Real space coordinates | - |
4 | Moving speed | - |
5 | Proliferation rate | - |
6 | Differentiation rate | - |
7 | Apoptosis rate | - |
8 | Status marker-01 | CC-FDC |
9 | Status marker-01 | CC-T |
10 | Molecular concentration-01 | ICOSL |
11 | Molecular concentration-02 | PD-L1 |
12 | Molecular concentration-03 | ICOS |
13 | Molecular concentration-04 | SIP |
14 | Molecular concentration-06 | uptaken antigens from FDCs |
15 | Molecular concentration-07 | help signals |
16 | Molecular concentration-08 | pMHC |
17 | Synthetic rate or degradation rate of | SIP/help signal/ICOSL/PD-L1 |
Parameter | 2D | 3D | Illustration | References |
Lattice spacing | 10 µm | 10 µm | Radius of B cell is about 5 µm | - |
Radius of GC | 200 µm | 150 µm | - | - |
Number of seeder cells | 3 | 3 | About three cells enter the follicle. | [29][46][47] |
Shape space dimension | 4 | 4 | Range of affinity (0–1) | [9][48] |
Number of T cells | 100 | 2500 | - | [49] |
T cell moving speed | 10.8 µm/min | 10.8 µm/min | - | [50][51] |
Number of FDCs | 20 | 225 | - | [32] |
Length of FDC arms | 20 µm | 20 µm | - | [32] |
Basic rate of proliferation | 1/9 h | 1/6 h | - | [52] |
CB moving speed | 1.5 µm/min | 1.5 µm/min | - | [50][51] |
Basic rate of CB differentiation | 1/7 h | 1/4.7 h | - | [20] |
CC moving speed | 5 µm/min | 5 µm/min | - | [50][51] |
Tfh cells moving speed | 10.8 µm/min | 10.8 µm/min | - | [50][51] |
Probability of recycling for selected CC | 0.8 | - | - | [53] |
Basic rate of CC differentiation | 1/6 h | 1/4 h | - | [52] |
Basic rate of FDC-CC dissociation | 1/0.1 h | 1/0.1 h | - | |
Basic rate of FDC-T dissociation | 1/0.5 h | 1/0.5 h | - | [54] |
Synthesis Rate of SIP | 1 h | 1 h | After CBs obtain help signals from Tfh cells | [32] |
symbol | meaning | value | References |
n0 | The total number of FDC sites | 80 | [32] |
i | The level of affinity | 0–9 | [12] |
CCiFDC | The number of CCs with affinity level i that are binding with FDCs | - | |
nFDC | FDC sites that have not been occupied by CCs | - | |
T0 | The total number of activated T cells | 20 | [32] |
T | The number of T cells that are not contact with CCs | - | |
Bi | The number of CBs with affinity level i | - | |
mr | The probability of no mutation | 0.5 | [20][32] |
Pi | The total concentration of SIP with affinity level i | - | |
TRi | The number of CCs with affinity level i that have been rescued by T cells | - | |
FRiT | The number of CCs with affinity level i that are binding with Tfh cells | - | |
Rc | The probability of CCs' recycling into the DZ | 0.8 | [21] |
ΣBi | The sum of the numbers of CCs and CBs with affinity level i | - | |
Pi/ΣBi | The average SIP concentration in a B cell at level i | - | |
R1,R2,R3,R4,R5,R6 | Defined in Materials and Methods | - | |
CCi | The number of CCs with affinity level i | - | |
ENCF | The possibility of CCs encountering with FDC sites | 0.0001 | assumed |
kr | The dissociation rate of CCs with FDCs | 0.01 | assumed |
ka | The degradation rate of ingested antigens in CCs | 1 | [32] |
Agi | The ingested antigens by CCs with level of affinity i | - | |
FRi | The number of CCs with affinity level i that had contacted with FDC sites | - | |
ENCT | The possibility of CCs encountering with Tfh cells. | 0.0001 | assumed |
SPi | The concentration of help signals in B cells with affinity level i. | - | |
ks | The degradation rate of help signals in FRi | 1 | [32] |
PBi | The number of output cells of different affinity levels, that is, plasma or memory cells | - | |
kp | The degradation rate of total concentration of SIP | 1 | [32] |