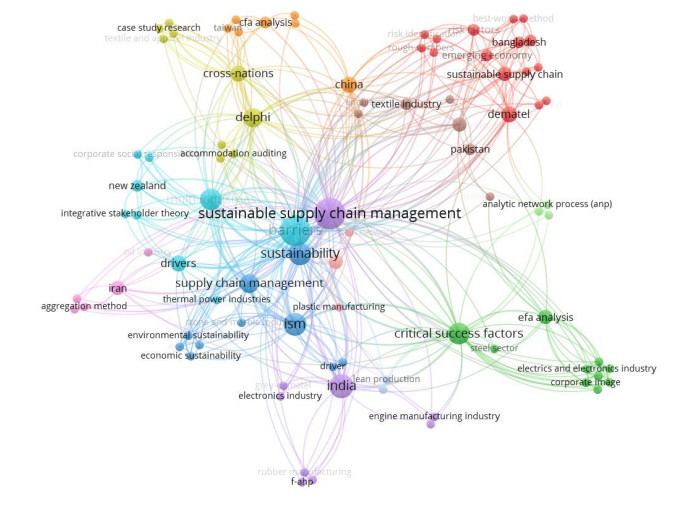
In the engineering sector, machining plays a significant role. The ability of the cutting zone to dissipate heat has grown in importance for the machining industry’s sustainability. Government legislation is pushing the industry to use fewer conventional lubricants as concerns about the effects on the environment and human health grow. This shifts attention to Minimum Quantity Lubrication (MQL) and biodegradable oils. The purpose of this study is to show how well two vegetable oils, in their raw forms, perform as cutting fluids during the MQL turning process of AISI 304 stainless steel. Each vegetable oil’s physiochemical and lubricating qualities were examined separately. After that, the two oils that comprised the hybrid vegetable oil were blended at a ratio of 0.5:0.5. During machining with an external threading tool, the hybrid vegetable oil was compared to its equivalent mineral-based oil in terms of cutting temperature and surface roughness. The Taguchi L9 orthogonal array was used in the study. According to the data, the cutting temperature was lowest when cutting with mineral oil, and highest when cutting with a hybrid mixture. In general, the mineral oil produced a reduced surface roughness compared to the vegetable oil mixture by about 68.6%. The combo of palm kernel and yellow orleander oil marginally outperformed mineral by about 2.3% when it came to cutting temperature. The significance of this study is to develop a more sustainable and environmentally friendly lubricants for industrial applications.
Citation: Rasaq Kazeem, Tien-Chien Jen, Godwin Akande, Stephen Akinlabi, Esther Akinlabi. Performance evaluation of hybrid biodegradable oils as a lubricant during cylindrical turning of AISI 304 austenitic stainless steel[J]. AIMS Materials Science, 2024, 11(2): 256-294. doi: 10.3934/matersci.2024015
[1] | G. Nandini, M. Venkatachalam, Raúl M. Falcón . On the r-dynamic coloring of subdivision-edge coronas of a path. AIMS Mathematics, 2020, 5(5): 4546-4562. doi: 10.3934/math.2020292 |
[2] | Meiqin Jin, Ping Chen, Shuangliang Tian . Interval edge colorings of the generalized lexicographic product of some graphs. AIMS Mathematics, 2024, 9(11): 30597-30611. doi: 10.3934/math.20241477 |
[3] | T. Deepa, M. Venkatachalam, Raúl M. Falcón . On the r-dynamic coloring of the direct product of a path with either a path or a cycle. AIMS Mathematics, 2020, 5(6): 6496-6520. doi: 10.3934/math.2020419 |
[4] | Shuangliang Tian, Ping Chen . Edge-coloring of generalized lexicographic product of graphs. AIMS Mathematics, 2024, 9(6): 15988-15995. doi: 10.3934/math.2024774 |
[5] | Syed Ahtsham Ul Haq Bokhary, Zill-e-Shams, Abdul Ghaffar, Kottakkaran Sooppy Nisar . On the metric basis in wheels with consecutive missing spokes. AIMS Mathematics, 2020, 5(6): 6221-6232. doi: 10.3934/math.2020400 |
[6] | Gaixiang Cai, Fengru Xiao, Guidong Yu . The identification numbers of lollipop graphs. AIMS Mathematics, 2025, 10(4): 7813-7827. doi: 10.3934/math.2025358 |
[7] | Kiki A. Sugeng, Fery Firmansah, Wildan, Bevina D. Handari, Nora Hariadi, Muhammad Imran . Several properties of antiadjacency matrices of directed graphs. AIMS Mathematics, 2024, 9(10): 27834-27847. doi: 10.3934/math.20241351 |
[8] | Yanyi Li, Lily Chen . Injective edge coloring of generalized Petersen graphs. AIMS Mathematics, 2021, 6(8): 7929-7943. doi: 10.3934/math.2021460 |
[9] | Shahbaz Ali, Muhammad Khalid Mahmmod, Raúl M. Falcón . A paradigmatic approach to investigate restricted hyper totient graphs. AIMS Mathematics, 2021, 6(4): 3761-3771. doi: 10.3934/math.2021223 |
[10] | Hongyu Chen, Li Zhang . A smaller upper bound for the list injective chromatic number of planar graphs. AIMS Mathematics, 2025, 10(1): 289-310. doi: 10.3934/math.2025014 |
In the engineering sector, machining plays a significant role. The ability of the cutting zone to dissipate heat has grown in importance for the machining industry’s sustainability. Government legislation is pushing the industry to use fewer conventional lubricants as concerns about the effects on the environment and human health grow. This shifts attention to Minimum Quantity Lubrication (MQL) and biodegradable oils. The purpose of this study is to show how well two vegetable oils, in their raw forms, perform as cutting fluids during the MQL turning process of AISI 304 stainless steel. Each vegetable oil’s physiochemical and lubricating qualities were examined separately. After that, the two oils that comprised the hybrid vegetable oil were blended at a ratio of 0.5:0.5. During machining with an external threading tool, the hybrid vegetable oil was compared to its equivalent mineral-based oil in terms of cutting temperature and surface roughness. The Taguchi L9 orthogonal array was used in the study. According to the data, the cutting temperature was lowest when cutting with mineral oil, and highest when cutting with a hybrid mixture. In general, the mineral oil produced a reduced surface roughness compared to the vegetable oil mixture by about 68.6%. The combo of palm kernel and yellow orleander oil marginally outperformed mineral by about 2.3% when it came to cutting temperature. The significance of this study is to develop a more sustainable and environmentally friendly lubricants for industrial applications.
The number of studies and discussions in terms of sustainable supply chain management (SSCM) has been increasing rapidly in recent years. As a result, emerged concepts related to sustainability, such as sustainable production and sustainable development, have gradually become more popular globally under various industries [1,2]. Incredibly, although it is easier to focus on the manufacturers and assess their efforts towards sustainability by eco-friendly products, conducted sustainability standards [1,3]. It is nevertheless clear that the SSCM can only be obtained by the contributions of all stakeholders in the supply chain consist of suppliers or customers [4]. In recent years, managers' cognition has been changed in this regard. In general, all issues related to the natural environment, community, or financial benefits are carefully considered under global competitive conditions [5,6]. Consequently, several scholars believed that the top management should integrate sustainability philosophy into business strategies to solve the pressures from stakeholders [3,7]. The evolution of the sustainability philosophy has influenced the organizations' development strategy. Some recent studies documented that under different research contexts would identify various parameters as well as barriers [1,8]. Such as, Zimon et al. (2020) have highlighted various drivers and barriers for the SSCM through the conditions of seventeen Sustainable Development Goals (SDGs) of the United Nations [2]. Combination employing ISM and AHP approach to pointed out twenty most essential factors of the SSCM by the Indian engine sector [9]. In the Iranian mining industries, forty-one SSCM barriers have been identified [10]. Besides, the different research methods of the scholars will also present inconsistent findings related to the parameters' importance, such as Delphi technique [7,11]; ISM approach [3,12]; or conducting simultaneously two techniques to address research objectives [8,9]. Thus, it is clear that these diversities have suggested many promising future research gaps for other scholars to be concerned with the SSCM practice topic in identifying the prevailing parameters.
In Taiwan, numerous sustainability initiatives have been discussed and widely adopted in the various supply chains [13]. However, most studies only focus on Taiwan's vital industries, such as semiconductor manufacturing or electronics assembly [13,14]. Lack of research about the SSCM of agriculture sectors; meanwhile, the agricultural industries have great significance to the households of indigenous people, especially in rural areas [15]. Besides, several sustainable development policies have been published to improve the livelihood of that community group [16]. Recently, Taiwan's fresh fruit export industry is seriously affected by import bans from the Chinese market, and the total expected export value will be reduced by twenty percent in 2021 [17]. Thereby, this problem has a significant impact on Taiwan's fruit supply chain, particularly affecting farmers' livelihoods. Besides, excessive dependence on one market has seriously affected the sustainability of this supply chain. Thus, forcing Taiwan's fruit supply chain to change to ensure sustainability. According to the existing literature on the sustainable supply chain management topic, the authors aim to identify which essential parameters are given the attention and effect on the SSCM of the industry. Therefore, the Taiwanese fresh-fruit sector has been chosen for practical research to examine which parameters must be considered. This article aims to solve research objectives as follows: RO1. Identification of critical parameters for SSCM under the Taiwanese fresh-fruit sector; RO2. Evaluation to identify the most significant SSCM parameters in this context.
Concentrating on the discussion in parameters contribute to the Taiwanese sustainable fresh-fruit supply chain context as a unique research and promising. The Technique for Order of Preference by Similarity to Ideal Solution (TOPSIS) is one of the multi-criteria decision-making methods and is an outstanding technique to widely conducted [4]. Its ability has an excellent quality to solve complex problems and evaluate the importance of parameters [4,18]. Thereby, to solve two research objectives that this manuscript would conduct the TOPSIS tool to present the most significant SSCM parameters by the empirical research in Taiwan. Hence, this study would reveal some new methodological insights that bridge academia and practice for SSCM regard. Importantly, the research findings will contribute several theoretical implications for the SSCM literature. Besides, it would suggest some suggestions for managers several practical implications in this regard.
The existing literature recently revealed that the SSCM implementation topic had gained significant discussion in academics and practices thanks to the positive impact of sustainability in order to enhance business performance [2,19]. Although there are still controversial discussions about the definition of SSCM; however, most scholars agree that the SSCM implementation is in line with the economic, social, and environmental considerations to making business strategies [2,8]. Several recent studies indicated that under different research contexts would identify various parameters and barriers [1]. The examples can be Narimissa et al. (2020) suggested ten drivers and barrier to implement SSCM in the Iranian oil sector [7]. Consistent with that, Zimon et al. (2020) proposed the model for conducting SDGs in the SSCM [2]. After that, Pandey et al. (2021) employed the hybrid method to pointed out twenty most essential factors of the SSCM by the Indian engine sector [9]. Hence, these studies suggested promising future research gaps for other scholars to be concerned with the SSCM practice topic in identifying the prevailing parameters.
Furthermore, to determine the research gaps and identify the critical variables for SSCM; hence, the authors collect all papers related to SSCM practices and relevant impact elements based on the WoS and SCOPUS database. Several keywords for searching include "sustainable"; "supply chain management"; "parameter"; "barriers"; "elements"; "critical factors". As a result, a total of thirty-one journal papers have been assembled. They are discussed in various contexts, such as nations, industries, and approaches. A systematic analysis of all previous studies on the SSCM implementation topic has been conducted (presented Figure 1). The research gaps are identified in the following points relying on the existing SSCM implementation studies.
Firstly, the SSCM literature has witnessed the lack of empirical research in Taiwan, especially agriculture sector [13,14]; meanwhile, developing nations are the most preferred research context, for instance, India (n = 10) and China (n = 4). This is the first empirical research in Taiwanese food industry for the SSCM practices regard.
Secondly, sustainability studies regarding supply chain management have focused mainly on manufacturing, while the agricultural sector has experienced no specific research. Moreover, Taiwan's economy was well-known by the semiconductor manufacturing industry [14]; thus, conducting the study for the fresh-fruit supply chain has a significant impact on Taiwan's sustainable development, notably would broaden the SSCM practices literature in various sectors.
Thirdly, in terms of research method conducted, the majority of documents prioritize employed qualitative methods, such as ISM (n = 9) and Delphi (n = 6). However, the existing literature does not record any studies that have conducted the TOPSIS approach to consider this issue. Besides, some researchers proposed the TOPSIS as an outstanding technique, its ability is deemed good-quality to solve complex problems and enough to evaluate the importance of elements [4,18]. Hence, conducting the TOPSIS technique in this study would offer some new methodological insights that bridge academia and practice for SSCM regard.
According to the existing sustainability literature about supply chain management, twelve critical parameters reported to contribute to SSCM practices are demonstrated in Table 1.
Coding | Parameters | Brief Description | Sources |
P1 | Planning & Implementation | The optimal design of the fresh fruit supply chain is inevitable, and it requires proper planning and implementation. | [4,7,20,21,22] |
P2 | Knowledge & Training of fruit preservation | The preservation of post-harvest products needs to apply the latest knowledge and disseminate it to the stakeholders of the chain, particularly in farms and micro corporates. | [4,23,24,25] |
P3 | Farming area/ farmer | As the first stage of the food supply chain, the essential role of farms to provide fresh products to the wholesalers, factories, and end customers. | [5,15,26,27,28] |
P4 | Processing & Packaging | Fresh products need to maintain optimum quality for the longest time. Therefore, standardization and synchronization of processing and packaging must be attention for all the stages. | [4,20,29,30,31] |
P5 | Warehouse/Storage | Warehouse acts as a transit station in the middle of the food supply chain, the quality of products will be significantly affected by storage operation in warehouses. | [18,24,30,32] |
P6 | Distribution & Logistics service | The smooth flow of goods in the food supply chain will depend heavily on distribution channels and logistics services. | [5,18,33,34] |
P7 | Product quality & Safety | Fresh goods have stringent requirements for standard product quality and safety. It is the participation of the whole chain and must be guaranteed from the stage of planting and harvesting to the end stage. | [4,35,36] |
P8 | Contracting | Offering a variety of contracts for choose to ensure the benefits of each counterpart in the food supply chain. Thereby, it helps to reduce risks and conflicts among members. | [12,18,37,38] |
P9 | Technology adoption | Adopting the latest technology to the supply chain is part of the stakeholders' efforts to obtain sustainability, such as minimizing the impact on the natural environment, reducing costs. | [39,40,41] |
P10 | Customer | As the end-stage in the supply chain and consume products, the perceptions and reactions of this group is the driver and will significantly impact the sustainability initiatives of other stakeholders. | [20,34,42,43] |
P11 | Organizational social responsibilities | Conducting social programs for the local communities has been launched in the sustainable development goals. Forcing the various organization to perform. | [1,21,44,45] |
P12 | Collaboration | Collaboration among supply chain members would reinforce sustainability and obtain better performance by reducing financial constraints and production scale. | [5,6,31,46,47]. |
Pointing out optimal solutions according to various parameter selections, these results can be a resource to refer to before decision-making in many different practice situations. The authors assemble data through surveying with ten professionals in the SSCM expertise. They would play the role of decision-makers relying on their experience and knowledge. Collecting data has been done based on twelve clarified SSCM parameters. Employing the TOPSIS approach analyzes the expert's response to solving the second research objective.
The authors can rank all SSCM parameters to determine the most significant variables for the current Taiwanese fresh-fruit supply chain. In this study, ten experts in the Taiwanese fresh-fruit supply chain were asked to rate all criteria. The language terms will be defined from 1 to 10; in detail, "1" means "Extremely unimportant", and "10" means "Extremely important". Consequently, the TOPSIS algorithm could be conducted by the following process:
Step one: Relying on m alternative and n criteria having a rating of ith decision-makers and jth criteria. A matrix format as [xij]m∗n can be expressed for a MCDM issues as below.
{x11x12......x1nx21x22......x2n..xm1xm2......xmn}i=1,2,...,m;j=1,2,...,n |
Step two: The normalize decision matrix has been calculated in Eq 1, where i = 1, 2, ..., n; and j = 1, 2, ..., m. And then, obtaining the normalized decision matrix by calculate the value rij.
rij=xij√n∑i=1x2ij | (1) |
{r11r12......r1nr21r22......r2n..rm1rm2......rmn} |
Step three: The authors then construct the weighted normalized matrix by calculate vij.
vij=wj∗rij | (2) |
{v11v12......v1nv21v22......v2n..vm1vm2......vmn} |
In details, wj = weights of different attributes; (i = 1, 2, …, n; j = 1, 2, …, m)
Step four: Identifying the ideal solution consist of positive ideal solution (A+) and negative ideal solution (A-), the distance of these ideal solutions has been revealed by maximum and minimize value as below.
A+=(v+1,v+2,...,v+n)withvj+={maxxi.vij,mini.vij} | (3) |
A−=(v−1,v−2,...,v−n)withvj−={mini.vij,maxxi.vij} | (4) |
Step five: Determining each alternative's distance by calculating the Euclidean distance from the positive and negative ideal solutions. Those solutions are calculated by two values as s+i and s−i, and can be shown in Eqs 5 and 6, respectively.
s+i=√∑j(v+ij−v+j)2 | (5) |
s−i=√∑j(v−ij−v−j)2 | (6) |
with i = 1, 2, ..., m and j = 1, 2, ..., n.
Step six: The authors calculate the closeness coefficient values (CCi) of each alternative by the Eq 7:
CCi=s−is−i+s+iwithi=1,2,...,m | (7) |
Step seven: Determining the optimal solutions according to the CCi values range from 0 to 1 scale, the great solution according to the alternative value closest to 1 cutoff. Further, the authors also can determine exactly the rank of these alternatives thanks to these CCi values. These values will provide the key findings for the study to discuss.
Integration sustainability initiatives into business strategies are widely conducted in almost all Taiwanese sectors, most of them concentrated on essential industries like semiconductor manufacturing or electronics assembly compared to agriculture industries [13,14,48]. However, the agriculture sector has a significant influence on the indigenous people's livelihood are struggling with different risks to obtain sustainable development goals [16]. For instance, in recent years observed that Taiwan's fresh fruit supply chain has been easily vulnerable to customer pressuring [17]. Thereby, this problem has a significant impact on social performance, particularly affecting farmers' livelihoods. Thus, forcing Taiwan's fruit supply chain to change to attain sustainability through identifying SSCM prevailing parameters.
The authors assemble data for this study by interviewing ten experts regarding the Taiwanese fresh fruit supply chain. These respondents have a long experience along with their expertise in SSCM practices. Besides, they offer responses about twelve SSCM parameters. The respondents' personal information was also described in Table 2.
Category | Frequency | Percentage | |
Gender | Male | 7 | 70.0% |
Female | 3 | 30.0% | |
Age group | 31-40 | 3 | 30.0% |
41-50 | 5 | 50.0% | |
51-60 | 1 | 10.0% | |
over 60 | 1 | 10.0% | |
Degree | Bachelor degree | 4 | 40.0% |
Master degree | 4 | 40.0% | |
Ph.D. degree | 2 | 20.0% | |
Organizational | Process Manufacturing | 6 | 60.0% |
Service | 4 | 40.0% | |
Working Position | Top Managers | 3 | 30.0% |
Sales Department | 3 | 30.0% | |
Financial Management | 1 | 10.0% | |
Human Resource Management | 2 | 20.0% | |
Production Management | 1 | 10.0% |
The second research objective requires determining the importance level of SSCM parameters. The Taiwanese fresh-fruit supply chain has been chosen for this empirical research to address this objective. The study assembles data for analysis relying on the excellent knowledge and experiences of ten experts (Es) in this regard. Following the sequence of seven steps in TOPSIS analysis, the findings would report the significance of all twelve SSCM parameters. Further, they are considered good suggestions for the top managers in the Taiwanese fresh-fruit supply chain toward sustainability.
According to the years of experience of each respondent, that decides the weightage for each decision-maker (presented in Table 3). The normalized matrix of the respondents' answers has been conducted (follow Eq 1) to determine (xij) values.
Parameters | E1 | E2 | E3 | E4 | E5 | E6 | E7 | E8 | E9 | E10 |
Weight | 0.13 | 0.10 | 0.18 | 0.12 | 0.11 | 0.11 | 0.06 | 0.05 | 0.05 | 0.09 |
P1 | 0.0414 | 0.0319 | 0.0574 | 0.0489 | 0.0315 | 0.0160 | 0.0142 | 0.0177 | 0.0052 | 0.0276 |
P2 | 0.0322 | 0.0283 | 0.0574 | 0.0381 | 0.0315 | 0.0279 | 0.0142 | 0.0132 | 0.0173 | 0.0221 |
P3 | 0.0460 | 0.0212 | 0.0574 | 0.0272 | 0.0276 | 0.0359 | 0.0142 | 0.0132 | 0.0138 | 0.0166 |
P4 | 0.0184 | 0.0319 | 0.0319 | 0.0272 | 0.0394 | 0.0279 | 0.0170 | 0.0155 | 0.0173 | 0.0276 |
P5 | 0.0322 | 0.0177 | 0.0447 | 0.0326 | 0.0355 | 0.0319 | 0.0227 | 0.0132 | 0.0173 | 0.0276 |
P6 | 0.0414 | 0.0319 | 0.0574 | 0.0272 | 0.0315 | 0.0359 | 0.0198 | 0.0110 | 0.0173 | 0.0276 |
P7 | 0.0414 | 0.0354 | 0.0319 | 0.0326 | 0.0315 | 0.0359 | 0.0142 | 0.0110 | 0.0173 | 0.0276 |
P8 | 0.0414 | 0.0354 | 0.0574 | 0.0272 | 0.0355 | 0.0319 | 0.0113 | 0.0132 | 0.0138 | 0.0276 |
P9 | 0.0368 | 0.0248 | 0.0447 | 0.0326 | 0.0276 | 0.0359 | 0.0198 | 0.0177 | 0.0173 | 0.0276 |
P10 | 0.0414 | 0.0319 | 0.0574 | 0.0381 | 0.0276 | 0.0319 | 0.0198 | 0.0132 | 0.0017 | 0.0221 |
P11 | 0.0276 | 0.0212 | 0.0574 | 0.0326 | 0.0276 | 0.0279 | 0.0170 | 0.0199 | 0.0104 | 0.0276 |
P12 | 0.0414 | 0.0283 | 0.0574 | 0.0435 | 0.0315 | 0.0359 | 0.0198 | 0.0110 | 0.0138 | 0.0276 |
Then, conducting two Eqs 2 and 3 to explore (rij) and (vij) values, respectively. Table 3 demonstrate the weighted normalized decision matrix for this research.
Thereby, the next step can be shown in Table 4, in detail, two essential values are pointed out includes positive (A+) and negative (A−) ideal solutions.
Ideal Solutions | E1 | E2 | E3 | E4 | E5 | E6 | E7 | E8 | E9 | E10 |
Positive (A+) | 0.0460 | 0.0354 | 0.0574 | 0.0489 | 0.0394 | 0.0359 | 0.0227 | 0.0199 | 0.0173 | 0.0276 |
Negative (A−) | 0.0184 | 0.0177 | 0.0319 | 0.0272 | 0.0276 | 0.0160 | 0.0113 | 0.0110 | 0.0017 | 0.0166 |
The Euclidean distance would be considered in step five by conducting two Eqs as 5 and 6 for each SSCM parameter. As a result, Table 5 and Table 6 are illustrate the positive and negative ideal solution values, respectively.
Parameters | E1 | E2 | E3 | E4 | E5 | E6 | E7 | E8 | E9 | E10 |
P1 | 0.0046 | 0.0035 | 0 | 0 | 0.0079 | 0.0200 | 0.0085 | 0.0022 | 0.0121 | 0 |
P2 | 0.0138 | 0.0071 | 0 | 0.0109 | 0.0079 | 0.0080 | 0.0085 | 0.0066 | 0 | 0.0055 |
P3 | 0 | 0.0142 | 0 | 0.0218 | 0.0118 | 0 | 0.0085 | 0.0066 | 0.0035 | 0.0110 |
P4 | 0.0276 | 0.0035 | 0.0255 | 0.0218 | 0 | 0.0080 | 0.0057 | 0.0044 | 0 | 0 |
P5 | 0.0138 | 0.0177 | 0.0128 | 0.0163 | 0.0039 | 0.0040 | 0 | 0.0066 | 0 | 0 |
P6 | 0.0046 | 0.0035 | 0 | 0.0218 | 0.0079 | 0 | 0.0028 | 0.0088 | 0 | 0 |
P7 | 0.0046 | 0 | 0.0255 | 0.0163 | 0.0079 | 0 | 0.0085 | 0.0088 | 0 | 0 |
P8 | 0.0046 | 0 | 0 | 0.0218 | 0.0039 | 0.0040 | 0.0113 | 0.0066 | 0.0035 | 0 |
P9 | 0.0092 | 0.0106 | 0.0128 | 0.0163 | 0.0118 | 0 | 0.0028 | 0.0022 | 0 | 0 |
P10 | 0.0046 | 0.0035 | 0 | 0.0109 | 0.0118 | 0.0040 | 0.0028 | 0.0066 | 0.0155 | 0.0055 |
P11 | 0.0184 | 0.0142 | 0 | 0.0163 | 0.0118 | 0.0080 | 0.0057 | 0 | 0.0069 | 0 |
P12 | 0.0046 | 0.0071 | 0 | 0.0054 | 0.0079 | 0 | 0.0028 | 0.0088 | 0.0035 | 0 |
Parameters | E1 | E2 | E3 | E4 | E5 | E6 | E7 | E8 | E9 | E10 |
P1 | 0.0230 | 0.0142 | 0.0255 | 0.0218 | 0.0039 | 0 | 0.0028 | 0.0066 | 0.0035 | 0.0110 |
P2 | 0.0138 | 0.0106 | 0.0255 | 0.0109 | 0.0039 | 0.0120 | 0.0028 | 0.0022 | 0.0155 | 0.0055 |
P3 | 0.0276 | 0.0035 | 0.0255 | 0 | 0 | 0.0200 | 0.0028 | 0.0022 | 0.0121 | 0 |
P4 | 0 | 0.0142 | 0 | 0 | 0.0118 | 0.0120 | 0.0057 | 0.0044 | 0.0155 | 0.0110 |
P5 | 0.0138 | 0 | 0.0128 | 0.0054 | 0.0079 | 0.0160 | 0.0113 | 0.0022 | 0.0155 | 0.0110 |
P6 | 0.0230 | 0.0142 | 0.0255 | 0 | 0.0039 | 0.0200 | 0.0085 | 0 | 0.0155 | 0.0110 |
P7 | 0.0230 | 0.0177 | 0 | 0.0054 | 0.0039 | 0.0200 | 0.0028 | 0 | 0.0155 | 0.0110 |
P8 | 0.0230 | 0.0177 | 0.0255 | 0 | 0.0079 | 0.0160 | 0 | 0.0022 | 0.0121 | 0.0110 |
P9 | 0.0184 | 0.0071 | 0.0128 | 0.0054 | 0 | 0.0200 | 0.0085 | 0.0066 | 0.0155 | 0.0110 |
P10 | 0.0230 | 0.0142 | 0.0255 | 0.0109 | 0 | 0.0160 | 0.0085 | 0.0022 | 0 | 0.0055 |
P11 | 0.0092 | 0.0035 | 0.0255 | 0.0054 | 0 | 0.0120 | 0.0057 | 0.0088 | 0.0086 | 0.0110 |
P12 | 0.0230 | 0.0106 | 0.0255 | 0.0163 | 0.0039 | 0.0200 | 0.0085 | 0 | 0.0121 | 0.0110 |
Finally, the authors calculate the closeness ratio (CCi) values for all essential SSCM parameters to determine the importance level by Eq 7. Table 7 reports the ranking of twelve SSCM parameters for the Taiwanese fresh fruit sector based on their CCi values. Consequently, the final rank of the SSCM parameters in terms of the Taiwanese fresh-fruit industry is P12 > P6 > P10 > P8 > P1 > P2 > P9 > P3 > P7 > P5 > P11 > P4. Additionally, Figure 2 is expressed the results of these findings analysis related to the comparison among the ranked key parameters of SSCM implementation on the basis of CCi values.
Parameters | s+i | s−i | CCi | Ranking |
Collaboration (P12) | 0.0162 | 0.0481 | 0.7485 | 1 |
Distribution & Logistic service (P6) | 0.0256 | 0.0472 | 0.6485 | 2 |
Customer (P10) | 0.0251 | 0.0431 | 0.6318 | 3 |
Contracting (P8) | 0.0266 | 0.0456 | 0.6313 | 4 |
Planning & Implementation (P1) | 0.0268 | 0.0453 | 0.6286 | 5 |
Knowledge & Training of fruit preservation (P2) | 0.0251 | 0.0389 | 0.6079 | 6 |
Technology adoption (P9) | 0.0279 | 0.0382 | 0.5777 | 7 |
Farming area/ farmer (P3) | 0.0326 | 0.0445 | 0.5772 | 8 |
Product quality & Safety (P7) | 0.0339 | 0.0407 | 0.5453 | 9 |
Warehouse/Storage (P5) | 0.0317 | 0.0346 | 0.5214 | 10 |
Organizational social responsibilities (P11) | 0.0330 | 0.0350 | 0.5151 | 11 |
Processing & Packaging (P4) | 0.0449 | 0.0300 | 0.4006 | 12 |
The experts' responses have documented that "Collaboration" has obtained the highest priority (CCi = 0.7485) among the SSCM parameters in the Taiwanese fresh-fruit sector. Meanwhile, the second importance level belongs to "Distribution & Logistics service" (CCi = 0.6485), and followed by "Customer" issues (CCi = 0.6318). On the contrary, the TOPSIS analysis reported that three parameters consist of "Warehouse/Storage" (CCi = 0.5214), "Organizational social responsibilities" (CCi = 0.5151), and "Processing & Packaging" (CCi = 0.4006) are located at the bottom of the ranking table. In the middle of the rankings are other variables; in detail, "Contracting" (CCi = 0.6313) and "Planning & Implementation" (CCi = 0.6286) have ranked fourth and fifth place, respectively. Meanwhile, other parameters consist of "Knowledge & Training of fruit preservation" (CCi = 0.6079), "Technology adoption" (CCi = 0.5777), "Farming area/ farmer" (CCi = 0.5772) and "Product quality and safety" (CCi = 0.5453) are the less critical level consecutively. These criteria have been recognized as critical parameters for the sustainability of the Taiwanese fresh-fruit supply chain, their importance level as the reference sources for this industry to consider and carry out. Particularly in the current competitive period, when even the most minor efforts are attention and could improve performance.
In Taiwan's context, the agricultural industry has great significance to the livelihood of indigenous people, especially in remote areas [15]. Meanwhile, several sustainable development policies have been launched to enhance living conditions and improve the livelihood of the indigenous people [16]. Further, connecting sustainable agricultural development to other fields and therefore make a meaningful contribution towards sustainability [15]. Regarding the food supply chain, particularly in the fresh-fruit supply chain, which should face various risks from the production process to the end customers [1,34]. Thus, the Taiwanese fresh-fruit sector can refer to these twelve identified SSCM parameters as a reference resource in making decisions through these research findings. To contribute some managerial implications, based on ten experts' opinions, the data has been collected to analyze and discuss twelve identified parameters. The results analysis report that these variables have a different ranking from highest-priority to lowest. These findings indicate the importance level of various parameters for the SSCM of the Taiwanese fresh-fruit sector.
In terms of the food supply chain, there is a presence and participation of many different stakeholders [5]. Thus, several solutions regarding collaboration have been discussed to promote and improve intimate cooperation among members in the food supply chain, besides targeting further obtaining supply chain sustainability [47]. Importantly, collaborating among suppliers will achieve better performance due to they could remove financial constraints and production scale, particularly suitable in the context of small and medium enterprises of agriculture industries [46]. Hence, in line with previous studies regarding the "Collaboration" variable [6,46,47], ten experts in this study believed that collaboration among members of the Taiwanese fresh-fruit supply chain is the most significance to obtain sustainability. In this research, "Distribution & Logistics service" variable obtained second place in the preoccupation of experts about SSCM practices. As the risk sector, the agriculture industry must determine the optimal time from the stage in collecting fresh products in various farms, distribution to the warehouses, then sending to the end customers [5]. Besides, avoiding damage to fresh fruit during transportation is very essential [33,34]. Dhaoui et al. (2020) explored the close relationship between the consumers' and distribution channels of these fresh products in Greece, such as fruit and vegetables. "Customer" parameter is rated higher than "Contracting" variable, two criteria are statistically significant at third and fourth positions, respectively. An interesting fact in the supply chain is that manufacturers that act as suppliers can also be customers of other suppliers [18]. The critical role of the customers variable in the food supply chain has been proven in other prior studies [34,42]. Thus, customers' expectations have become the targets of various suppliers [20,43]. To avoid risks from customers parameter, expanding the market scope to find more different customers has become a solution for suppliers in this area [31].
Notably, considering "Contracting" as the supply chain parameters, Shen et al. [37] have divided into nine contracting clusters based on information. Besides, contracting with information updating among counterparts has been suggested to attain a sustainable supply chain. Surprisingly, these findings are consistent with the exploring of Chaudhuri et al. [12] and Xiao et al. [38], they have identified and particularly interested in contracting to reduce the risk for the food supply chain in India and China, respectively. Further, the study findings have contributed to the existing literature some promising ideas for the top managers when considering various proposed SSCM parameters. Although the remaining variables have lower CCi values; however, their significance for the food supply chain has been determined and verified once again by the fresh-fruit supply chain in Taiwan. Consequently, unfortunately, the analysis results witnessed the lowest values of some parameters, for instance, "Warehouse/Storage" [24,30,32], "Organizational social responsibilities" [1,44,45], and "Packaging & Processing" [4,20,30]. But the role of these criteria should not be underestimated or ignored. Ideally, to attain SSCM needs to conduct simultaneously different blueprints by the best efforts of all members.
Sustainability target is an inevitable trend worldwide [23]. It forces all various industries and nations to adopt different solutions to obtain [1]. In fact, fresh agriculture products have great significance on the livelihoods of the Taiwanese indigenous communities [15,16]. Therefore, determining critical parameters for the fruits chain in Taiwan is the main contribution insight; as a result, twelve essential variables have been identified. Moreover, as a different approach insight, the authors employed the TOPSIS technique for this study to explore the importance level of all parameters. It is considered an excellent tool to address research objectives through comparison among closeness coefficients. Consequently, based on the experts' responses, this study successfully determined the role of these essential variables in the Taiwanese fresh-fruit chain. Analysis outcomes revealed that "Collaboration" at the peak of the list, "Distribution & Logistics service" obtained second place, and followed by "Customer" get the third position with their CCi values as 0.7485, 0.6485, 0.6318, respectively. On the contrary, at the bottom of the prioritized list is three essential parameters consisting of "Warehouse/Storage", "Organizational social responsibilities", and "Processing & Packaging" with their CCi values as 0.5214, 0.5151, 0.4006, respectively. Unsurprisingly, collaboration with the right partner toward obtain sustainability together is very emerging attention; for instance, Song et al. [49] reported that it is the most considerable risk factor in the telecommunications sector. Meanwhile, Pandey et al. [9] considered the collaboration parameter less essential in an engine manufacturing industry. The contradictory assessments compared to the findings of this study have presented in some previous studies, such as, Prasad et al. [50] believed that customer's influence ranks bottom among the twenty SSCM critical factors of the Indian steel industry. Hence, differences in the importance ranking of SSCM components in practices have been observed depending on various industries and countries' contexts, consistent with the suggestions of Panigrahi et al. [1] and Zimon et al. [2].
As part of agricultural sustainability, the whole fruit supply chain members must carry out simultaneously these twelve identified critical parameters. Hence, these research results are a positive signal for senior managers to refer to making business decisions. This research is the first empirical research concentrating on the fresh-fruit supply chain in Taiwan to determine essential SSCM parameters, and provide the new theoretical insights to existing supply chain literature; unfortunately, this study cannot avoid certain limitations. Nevertheless, it can become promising suggestions to consider for others scholars to develop further. Firstly, this manuscript adopted the TOPSIS technique to analyze only ten professionals' responses. Therefore, the survey on a broader scale can be conducted to examine the impact of these variables on the SSCM performance in practices. Secondly, to broaden the concern of SSCM literature, particularly in the food supply chain, other scholars can suggest more parameters contribute to SSCM implementation. Thirdly, the Taiwanese fresh-fruit supply chain is considered as the case to examine the appropriateness of these SSCM parameters. Hence, the authors believe that these proposed SSCM criteria need to explore in another context, such as the national context, different supply chains of the agriculture sectors.
The authors acknowledge the chief editor and the reviewers for their valuable comments to improve the manuscript.
All authors declare no conflicts of interest in this paper.
[1] |
Maruda RW, Krolczyk GM, Nieslony P, et al. (2016) The influence of the cooling conditions on the cutting tool wear and the chip formation mechanism. J Manuf Process 24: 107–115. https://doi.org/10.1016/j.jmapro.2016.08.006 doi: 10.1016/j.jmapro.2016.08.006
![]() |
[2] |
Zhu D, Zhang X, Ding H (2013) Tool wear characteristics in machining of nickel-based superalloys. Int J Mach Tool Manu 64: 60–77. https://doi.org/10.1016/j.ijmachtools.2012.08.001 doi: 10.1016/j.ijmachtools.2012.08.001
![]() |
[3] | Kishawy HA, Hosseini A (2019) Machining Difficult-to-Cut Materials, Switzerland: Springer Cham. https://doi.org/10.1007/978-3-319-95966-5 |
[4] |
Abukhshim NA, Mativenga PT, Sheikh MA (2006). Heat generation and temperature prediction in metal cutting: A review and implications for high-speed machining. Int J Mach Tool Manu 46: 782–800. https://doi.org/10.1016/j.ijmachtools.2005.07.024 doi: 10.1016/j.ijmachtools.2005.07.024
![]() |
[5] |
Wang B, Liu Z, Su G, et al. (2015) Investigations of critical cutting speed and ductile-to-brittle transition mechanism for workpiece material in ultra-high speed machining. Int J Mech Sci 104: 44–59. https://doi.org/10.1016/j.ijmecsci.2015.10.004 doi: 10.1016/j.ijmecsci.2015.10.004
![]() |
[6] |
Prasad YVRK, Seshacharyulu TJMR (1998) Modelling of hot deformation for microstructural control. Int Mater Rev 43: 243–258. https://doi.org/10.1179/imr.1998.43.6.243 doi: 10.1179/imr.1998.43.6.243
![]() |
[7] |
Fonda RW, Bingert JF (2004) Microstructural evolution in the heat-affected zone of a friction stir weld. Metall Mater Trans A 35: 1487–1499. https://doi.org/10.1007/s11661-004-0257-7 doi: 10.1007/s11661-004-0257-7
![]() |
[8] |
Kini MV, Chincholkar AM (2010) Effect of machining parameters on surface roughness and material removal rate in finish turning of ±30 glass fibre reinforced polymer pipes. Mater Design 31: 3590–3598. https://doi.org/10.1016/j.matdes.2010.01.013 doi: 10.1016/j.matdes.2010.01.013
![]() |
[9] |
Moganapriya C, Rajasekar R, Ponappa K, et al. (2018) Influence of coating material and cutting parameters on surface roughness and material removal rate in turning process using Taguchi method. Mater Today Proc 5: 8532–8538. https://doi.org/10.1016/j.matpr.2017.11.550 doi: 10.1016/j.matpr.2017.11.550
![]() |
[10] |
Daniyan IA, Tlhabadira I, Daramola OO, et al. (2019) Design and optimization of machining parameters for effective AISI P20 removal rate during milling operation. Procedia CIRP 84: 861–867. https://doi.org/10.1016/j.procir.2019.04.301 doi: 10.1016/j.procir.2019.04.301
![]() |
[11] |
Okokpujie IP, Ohunakin OS, Bolu CA (2021) Multi-objective optimization of machining factors on surface roughness, material removal rate and cutting force on end-milling using MWCNTs nano-lubricant. Prog Addit Manuf 6: 155–178. https://doi.org/10.1007/s40964-020-00161-3 doi: 10.1007/s40964-020-00161-3
![]() |
[12] |
Cakir AK (2021) Analysis of surface roughness, sound level and machine current in the turning of hardened AISI S1 steel. Trans Indian Inst Met 74: 691–703. https://doi.org/10.1007/s12666-021-02196-8 doi: 10.1007/s12666-021-02196-8
![]() |
[13] |
Tekıner Z, Yeşılyurt S (2004) Investigation of the cutting parameters depending on process sound during turning of AISI 304 austenitic stainless steel. Mater Design 25: 507–513. https://doi.org/10.1016/j.matdes.2003.12.011 doi: 10.1016/j.matdes.2003.12.011
![]() |
[14] |
Şahinoğlu A, Güllü A (2020) Investigation of the relationship between current, sound intensity, vibration and surface roughness in machining of CuZn39Pb3 material on lathe machine. J Polytech 23: 615–624. http://doi.org/10.2339/politeknik.426106 doi: 10.2339/politeknik.426106
![]() |
[15] |
Fedai Y (2023) Exploring the impact of the turning of AISI 4340 steel on tool wear, surface roughness, sound intensity, and power consumption under dry, MQL, and nano-MQL conditions. Lubricants 11: 442. https://doi.org/10.3390/lubricants11100442 doi: 10.3390/lubricants11100442
![]() |
[16] |
Rafighi M, Özdemir M, Şahinoğlu A, et al. (2022) Experimental assessment and topsis optimization of cutting force, surface roughness, and sound intensity in hard turning of AISI 52100 steel. Surf Rev Lett 29: 2250150. https://doi.org/10.1142/S0218625X22501505 doi: 10.1142/S0218625X22501505
![]() |
[17] |
Mia M, Dey PR, Hossain MS, et al. (2018) Taguchi S/N based optimization of machining parameters for surface roughness, tool wear and material removal rate in hard turning under MQL cutting condition. Measurement 122: 380–391. https://doi.org/10.1016/j.measurement.2018.02.016 doi: 10.1016/j.measurement.2018.02.016
![]() |
[18] |
Kazeem RA, Fadare DA, Ikumapayi OM, et al. (2022) Advances in the application of vegetable-oil-based cutting fluids to sustainable machining operations—a review. Lubricants 10: 69. https://doi.org/10.3390/lubricants10040069 doi: 10.3390/lubricants10040069
![]() |
[19] |
Yin Q, Li C, Dong L, et al. (2021) Effects of physicochemical properties of different base oils on friction coefficient and surface roughness in MQL milling AISI 1045. Int J Pr Eng Man-GT 8: 1629–1647. https://doi.org/10.1007/s40684-021-00318-7 doi: 10.1007/s40684-021-00318-7
![]() |
[20] |
Sharma J, Sidhu BS (2014) Investigation of effects of dry and near dry machining on AISI D2 steel using vegetable oil. J Clean Prod 66: 619–623. https://doi.org/10.1016/j.jclepro.2013.11.042 doi: 10.1016/j.jclepro.2013.11.042
![]() |
[21] |
Kuram E, Ozcelik B, Demirbas E, et al. (2011) Evaluation of new vegetable-based cutting fluids on thrust force and surface roughness in drilling of AISI 304 using Taguchi method. Mater Manuf Process 26: 1136–1146. https://doi.org/10.1080/10426914.2010.536933 doi: 10.1080/10426914.2010.536933
![]() |
[22] |
Xavior MA, Adithan M (2009) Determining the influence of cutting fluids on tool wear and surface roughness during turning of AISI 304 austenitic stainless steel. J Mater Process Technol 209: 900–909. https://doi.org/10.1016/j.jmatprotec.2008.02.068 doi: 10.1016/j.jmatprotec.2008.02.068
![]() |
[23] |
Bai X, Zhou F, Li C, et al. (2020) Physicochemical properties of degradable vegetable-based oils on minimum quantity lubrication milling. Int J Adv Manuf Technol 106: 4143–4155. https://doi.org/10.1007/s00170-019-04695-x doi: 10.1007/s00170-019-04695-x
![]() |
[24] |
Saleem MQ, Mehmood A (2022) Eco-friendly precision turning of superalloy Inconel 718 using MQL based vegetable oils: Tool wear and surface integrity evaluation. J Manuf Process 73: 112–127. https://doi.org/10.1016/j.jmapro.2021.10.059 doi: 10.1016/j.jmapro.2021.10.059
![]() |
[25] |
Shankar S, Manikandan M, Raja G, et al. (2020) Experimental investigations of vibration and acoustics signals in milling process using kapok oil as cutting fluid. Mech Ind 21: 521. https://doi.org/10.1051/meca/2020066 doi: 10.1051/meca/2020066
![]() |
[26] |
Sen B, Gupta MK, Mia M, et al. (2021). Performance assessment of minimum quantity castor-palm oil mixtures in hard-milling operation. Materials 14: 198. https://doi.org/10.3390/ma14010198 doi: 10.3390/ma14010198
![]() |
[27] |
Kazeem RA, Fadare DA, Abutu J, et al. (2020) Performance evaluation of jatropha oil-based cutting fluid in turning AISI 1525 steel alloy. CIRP J Manuf Sci Tec 31: 418–430. https://doi.org/10.1016/j.cirpj.2020.07.004 doi: 10.1016/j.cirpj.2020.07.004
![]() |
[28] |
Baderna D, Lomazzi E, Passoni A, et al. (2015) Chemical characterization and ecotoxicity of three soil foaming agents used in mechanized tunneling. J Hazard Mater 296: 210–220. https://doi.org/10.1016/j.jhazmat.2015.04.040 doi: 10.1016/j.jhazmat.2015.04.040
![]() |
[29] |
Alaba ES, Kazeem RA, Adebayo AS, et al. (2023) Evaluation of palm kernel oil as cutting lubricant in turning AISI 1039 steel using Taguchi-grey relational analysis optimization technique. Adv Ind Manuf Eng 6: 100115. https://doi.org/10.1016/j.aime.2023.100115 doi: 10.1016/j.aime.2023.100115
![]() |
[30] |
Ikumapayi OM, Kazeem RA, Ogedengbe TS, et al. (2023) Performance evaluation of African star seed (chrysophyllum albidum) oil as a cutting lubricant in milling of ASTM A36 steel. Adv Mater Process Te 1–15. https://doi.org/10.1080/2374068X.2023.2192391 doi: 10.1080/2374068X.2023.2192391
![]() |
[31] |
Kazeem RA, Enobun IO, Akande IG, et al. (2023) Evaluation of palm kernel oil as lubricants in cylindrical turning of AISI 304 austenitic stainless steel using Taguchi-grey relational methodology. Mater Res Express 10: 126505. https://doi.org/10.1088/2053-1591/ad11fe doi: 10.1088/2053-1591/ad11fe
![]() |
[32] |
Abegunde PO, Kazeem RA, Akande IG, et al. (2023) Performance assessment of some selected vegetable oils as lubricants in turning of AISI 1045 steel using a Taguchi-based grey relational analysis approach. Tribol-Mater Surf In 17: 187–202. https://doi.org/10.1080/17515831.2023.2235227 doi: 10.1080/17515831.2023.2235227
![]() |
[33] |
Said Z, Gupta M, Hegab H, et al. (2019) A comprehensive review on minimum quantity lubrication (MQL) in machining processes using nano-cutting fluids. Int J Adv Manuf Tech 105: 2057–2086. https://doi.org/10.1007/s00170-019-04382-x doi: 10.1007/s00170-019-04382-x
![]() |
[34] |
Tazehkandi AH, Shabgard M, Pilehvarian F (2015) On the feasibility of a reduction in cutting fluid consumption via spray of biodegradable vegetable oil with compressed air in machining Inconel 706. J Clean Prod 104: 422–435. https://doi.org/10.1016/j.jclepro.2015.05.039 doi: 10.1016/j.jclepro.2015.05.039
![]() |
[35] |
Sarıkaya M, Gupta MK, Tomaz I, et al. (2021) A state-of-the-art review on tool wear and surface integrity characteristics in machining of superalloys. CIRP J Manuf Sci Tec 35: 624–658. https://doi.org/10.1016/j.cirpj.2021.08.005 doi: 10.1016/j.cirpj.2021.08.005
![]() |
[36] |
Usca UA, Uzun M, Kuntoğlu M, et al. (2021) Investigations on tool wear, surface roughness, cutting temperature, and chip formation in machining of Cu-B-CrC composites. Int J Adv Manuf Tech 116: 3011–3025. https://doi.org/10.1007/s00170-021-07670-7 doi: 10.1007/s00170-021-07670-7
![]() |
[37] |
Liu D, Liu Z, Zhao J, et al. (2022) Tool wear monitoring through online measured cutting force and cutting temperature during face milling Inconel 718. Int J Adv Manuf Tech 122: 729–740. https://doi.org/10.1007/s00170-022-09950-2 doi: 10.1007/s00170-022-09950-2
![]() |
[38] |
Rajaguru J, Arunachalam N (2020) A comprehensive investigation on the effect of flood and MQL coolant on the machinability and stress corrosion cracking of super duplex stainless steel. J Mater Process Technol 276: 116417. https://doi.org/10.1016/j.jmatprotec.2019.116417 doi: 10.1016/j.jmatprotec.2019.116417
![]() |
[39] |
Yasir M, Danish M, Mia M, et al. (2021). Investigation into the surface quality and stress corrosion cracking resistance of AISI 316L stainless steel via precision end-milling operation. Int J Adv Manuf Tech 112: 1065–1076. https://doi.org/10.1007/s00170-020-06413-4 doi: 10.1007/s00170-020-06413-4
![]() |
[40] |
Hegab H, Umer U, Soliman M, et al. (2018) Effects of nano-cutting fluids on tool performance and chip morphology during machining Inconel 718. Int J Adv Manuf Tech 96: 3449–3458. https://doi.org/10.1007/s00170-018-1825-0 doi: 10.1007/s00170-018-1825-0
![]() |
[41] |
Liew PJ, Shaaroni A, Sidik NAC, et al. (2017) An overview of current status of cutting fluids and cooling techniques of turning hard steel. Int J Heat Mass Tran 114: 380–394. https://doi.org/10.1016/j.ijheatmasstransfer.2017.06.077 doi: 10.1016/j.ijheatmasstransfer.2017.06.077
![]() |
[42] |
Aramesh M, Montazeri S, Veldhuis SC (2018) A novel treatment for cutting tools for reducing the chipping and improving tool life during machining of Inconel 718. Wear 414: 79–88. https://doi.org/10.1016/j.wear.2018.08.002 doi: 10.1016/j.wear.2018.08.002
![]() |
[43] |
Debnath S, Reddy MM, Yi QS (2016) Influence of cutting fluid conditions and cutting parameters on surface roughness and tool wear in turning process using Taguchi method. Measurement 78: 111–119. https://doi.org/10.1016/j.measurement.2015.09.011 doi: 10.1016/j.measurement.2015.09.011
![]() |
[44] | Nasirudeen AR, Lasisi D, Balogun LA (2019) Physico-chemical properties of yellow oleander (Thevetia peruviana) and their effects on the qualities of biodiesel. Arid Zone J Eng Tech Env 15: 859–866. Available from: https://www.azojete.com.ng/index.php/azojete/article/view/140. |
[45] |
Bachchhav B, Bagchi H (2021) Effect of surface roughness on friction and lubrication regimes. Mater Today Proc 38: 169–173. https://doi.org/10.1016/j.matpr.2020.06.252 doi: 10.1016/j.matpr.2020.06.252
![]() |
[46] |
Oliaei SNB, Karpat Y (2016) Investigating the influence of built-up edge on forces and surface roughness in micro scale orthogonal machining of titanium alloy Ti6Al4V. J Mater Process Technol 235: 28–40. https://doi.org/10.1016/j.jmatprotec.2016.04.010 doi: 10.1016/j.jmatprotec.2016.04.010
![]() |
[47] |
Meddour I, Yallese MA, Khattabi R (2015) Investigation and modeling of cutting forces and surface roughness when hard turning of AISI 52100 steel with mixed ceramic tool: Cutting conditions optimization. Int J Adv Manuf Tech 77: 1387–1399. https://doi.org/10.1007/s00170-014-6559-z doi: 10.1007/s00170-014-6559-z
![]() |
[48] |
Mikolajczyk T (2014) Modeling of minimal thickness cutting layer influence on surface roughness in turning. Appl Mech Mater 656: 262–269. https://doi.org/10.4028/www.scientific.net/AMM.656.262 doi: 10.4028/www.scientific.net/AMM.656.262
![]() |
[49] |
Akhtar W, Sun J, Chen W (2016) Effect of machining parameters on surface integrity in high speed milling of super alloy GH4169/Inconel 718. Mater Manuf Process 31: 620–627. https://doi.org/10.1080/10426914.2014.994769 doi: 10.1080/10426914.2014.994769
![]() |
[50] |
Uddin MS, Rosman H, Hall C (2017) Enhancing the corrosion resistance of biodegradable Mg-based alloy by machining-induced surface integrity: Influence of machining parameters on surface roughness and hardness. Int J Adv Manuf Tech 90: 2095–2108. https://doi.org/10.1007/s00170-016-9536-x doi: 10.1007/s00170-016-9536-x
![]() |
[51] |
Su G, Xiao X, Du J (2020). On cutting temperatures in high and ultrahigh-speed machining. Int J Adv Manuf Tech 107: 73–83. https://doi.org/10.1007/s00170-020-05054-x doi: 10.1007/s00170-020-05054-x
![]() |
[52] |
Zhang X, Peng Z, Li Z, et al. (2020) Influences of machining parameters on tool performance when high-speed ultrasonic vibration cutting titanium alloys. J Manuf Process 60: 188–199. https://doi.org/10.1016/j.jmapro.2020.10.053 doi: 10.1016/j.jmapro.2020.10.053
![]() |
[53] |
Kartal F, Yerlikaya Z, Okkaya H (2017) Effects of machining parameters on surface roughness and macro surface characteristics when the machining of Al-6082 T6 alloy using AWJT. Measurement 95: 216–222. https://doi.org/10.1016/j.measurement.2016.10.007 doi: 10.1016/j.measurement.2016.10.007
![]() |
[54] |
Diniardi E, Yudistirani SA, Basri H, et al. (2021) Analysis of the effect of cutting variables against surface hardness. J Appl Sci Adv Tech 3: 81–88. https://doi.org/10.24853/jasat.3.3.81-88 doi: 10.24853/jasat.3.3.81-88
![]() |
[55] |
Pang X, Zhang Y, Wang C, et al. (2020) Effect of cutting parameters on cutting force and surface quality in cutting of articular cartilage. Procedia CIRP 89: 116–121. https://doi.org/10.1016/j.procir.2020.05.127 doi: 10.1016/j.procir.2020.05.127
![]() |
[56] |
Majak D, Olugu EU, Lawal SA (2020) Analysis of the effect of sustainable lubricants in the turning of AISI 304 stainless steel. Procedia Manuf 43: 495–502. https://doi.org/10.1016/j.promfg.2020.02.183 doi: 10.1016/j.promfg.2020.02.183
![]() |
[57] |
Xavior MA, Adithan M (2009) Determining the influence of cutting fluids on tool wear and surface roughness during turning of AISI 304 austenitic stainless steel. J Mater Process Technol 209: 900–909. https://doi.org/10.1016/j.jmatprotec.2008.02.068 doi: 10.1016/j.jmatprotec.2008.02.068
![]() |
[58] |
Sarıkaya M, Yılmaz V, Gullu A (2016) Analysis of cutting parameters and cooling/lubrication methods for sustainable machining in turning of Haynes 25 superalloy. J Clean Prod 133: 172–181. https://doi.org/10.1016/j.jclepro.2016.05.122 doi: 10.1016/j.jclepro.2016.05.122
![]() |
[59] |
Manjunath K, Tewary S, Khatri N, et al. (2021) Monitoring and predicting the surface generation and surface roughness in ultraprecision machining: A critical review. Machines 9: 369. https://doi.org/10.3390/machines9120369 doi: 10.3390/machines9120369
![]() |
[60] |
Chakraborty P, Asfour S, Cho S, et al. (2008) Modeling tool wear progression by using mixed effects modeling technique when end-milling AISI 4340 steel. J Mater Process Technol 205: 190–202. https://doi.org/10.1016/j.jmatprotec.2007.11.197 doi: 10.1016/j.jmatprotec.2007.11.197
![]() |
[61] |
Flynn JM, Shokrani A, Newman ST, et al. (2016) Hybrid additive and subtractive machine tools—Research and industrial developments. Int J Mach Tool Manu 101: 79–101. https://doi.org/10.1016/j.ijmachtools.2015.11.007 doi: 10.1016/j.ijmachtools.2015.11.007
![]() |
[62] |
Dabees S, Mirzaei S, Kaspar P, et al. (2022) Characterization and evaluation of engineered coating techniques for different cutting tools. Materials 15: 5633. https://doi.org/10.3390/ma15165633 doi: 10.3390/ma15165633
![]() |
[63] |
Yeganefar A, Niknam SA, Asadi R (2019) The use of support vector machine, neural network, and regression analysis to predict and optimize surface roughness and cutting forces in milling. Int J Adv Manuf Technol 105: 951–965. https://doi.org/10.1007/s00170-019-04227-7 doi: 10.1007/s00170-019-04227-7
![]() |
[64] |
Praveen N, Mallik US, Shivasiddaramaiah AG, et al. (2023) Effect of CNC end milling parameters on Cu-Al-Mn ternary shape memory alloys using Taguchi method. J Inst Eng India Ser D https://doi.org/10.1007/s40033-023-00579-3 doi: 10.1007/s40033-023-00579-3
![]() |
[65] |
Guo Y, Yang X, Kang J, et al. (2022) Ductile machining of single-crystal germanium for freeform surfaces diamond turning based on a long-stroke fast tool servo. J Manuf Process 82: 615–627. https://doi.org/10.1016/j.jmapro.2022.08.013 doi: 10.1016/j.jmapro.2022.08.013
![]() |
[66] |
Patange AD, Jegadeeshwaran R (2021) Review on tool condition classification in milling: A machine learning approach. Mater Today Proc 46: 1106–1115. https://doi.org/10.1016/j.matpr.2021.01.523 doi: 10.1016/j.matpr.2021.01.523
![]() |
[67] |
Saglam H, Yaldiz S, Unsacar F (2007) The effect of tool geometry and cutting speed on main cutting force and tool tip temperature. Mater Design 28: 101–111. https://doi.org/10.1016/j.matdes.2005.05.015 doi: 10.1016/j.matdes.2005.05.015
![]() |
[68] |
Sheikh-Ahmad JY, Almaskari F, Hafeez F (2019) Thermal aspects in machining CFRPs: Effect of cutter type and cutting parameters. Int J Adv Manuf Tech 100: 2569–2582. https://doi.org/10.1007/s00170-018-2881-1 doi: 10.1007/s00170-018-2881-1
![]() |
[69] |
Käsemodel RB, De Souza AF, Voigt R, et al. (2020) CAD/CAM interfaced algorithm reduces cutting force, roughness, and machining time in free-form milling. Int J Adv Manuf Tech 107: 1883–1900. https://doi.org/10.1007/s00170-020-05143-x doi: 10.1007/s00170-020-05143-x
![]() |
[70] |
Safiei W, Rahman MM, Yusoff AR, et al. (2021) Effects of SiO2-Al2O3-ZrO2 tri-hybrid nanofluids on surface roughness and cutting temperature in end milling process of aluminum alloy 6061-T6 using uncoated and coated cutting inserts with minimal quantity lubricant method. Arab J Sci Eng 46: 7699–7718. https://doi.org/10.1007/s13369-021-05533-7 doi: 10.1007/s13369-021-05533-7
![]() |
1. | Abbott Po Shun Chen, Yung-Fu Huang, Manh-Hoang Do, Exploring the Challenges to Adopt Green Initiatives to Supply Chain Management for Manufacturing Industries, 2022, 14, 2071-1050, 13516, 10.3390/su142013516 | |
2. | Nahiyah Jaidi, Jane Liu, Zahrotush Sholikhah, Mega Murti Andhini, Ambidexterity Behavior of Creative SMEs for Disruptive Flows of Innovation: A Comparative Study of Indonesia and Taiwan, 2022, 8, 2199-8531, 141, 10.3390/joitmc8030141 | |
3. | Vu-Dung-Van Phan, Yung-Fu Huang, Thi-Them Hoang, Manh-Hoang Do, Evaluating Barriers to Supply Chain Resilience in Vietnamese SMEs: The Fuzzy VIKOR Approach, 2023, 11, 2079-8954, 121, 10.3390/systems11030121 | |
4. | Yung-Fu Huang, Abbott Po-Shun Chen, Manh-Hoang Do, Jen-Chieh Chung, Assessing the Barriers of Green Innovation Implementation: Evidence from the Vietnamese Manufacturing Sector, 2022, 14, 2071-1050, 4662, 10.3390/su14084662 | |
5. | Manh-Hoang Do, Yung-Fu Huang, Barriers to university social responsibility implementation in the Vietnamese higher education, 2023, 0951-354X, 10.1108/IJEM-11-2021-0432 | |
6. | Manh-Hoang Do, Yung-Fu Huang, Vu-Dung-Van Phan, Analyzing the barriers to green supply chain management implementation: a case study of the Vietnamese agriculture sector, 2023, 1741-0398, 10.1108/JEIM-10-2021-0459 | |
7. | Muhamad Rusliyadi, Yu Hua Chen, 2025, chapter 8, 9798369353509, 133, 10.4018/979-8-3693-5350-9.ch008 | |
8. | Imadeddine Oubrahim, 2025, Chapter 18, 978-3-031-81377-1, 204, 10.1007/978-3-031-81378-8_18 | |
9. | Damian Dubisz, Arkadiusz Kawa, Correlation Between Key Parameters of Wood Biomass Reverse Supply Chains and Their Impact on Total Biomass Acquisition Levels, 2023, 66, 2956-9141, 1, 10.12841/wood.1644-3985.449.06 |
Coding | Parameters | Brief Description | Sources |
P1 | Planning & Implementation | The optimal design of the fresh fruit supply chain is inevitable, and it requires proper planning and implementation. | [4,7,20,21,22] |
P2 | Knowledge & Training of fruit preservation | The preservation of post-harvest products needs to apply the latest knowledge and disseminate it to the stakeholders of the chain, particularly in farms and micro corporates. | [4,23,24,25] |
P3 | Farming area/ farmer | As the first stage of the food supply chain, the essential role of farms to provide fresh products to the wholesalers, factories, and end customers. | [5,15,26,27,28] |
P4 | Processing & Packaging | Fresh products need to maintain optimum quality for the longest time. Therefore, standardization and synchronization of processing and packaging must be attention for all the stages. | [4,20,29,30,31] |
P5 | Warehouse/Storage | Warehouse acts as a transit station in the middle of the food supply chain, the quality of products will be significantly affected by storage operation in warehouses. | [18,24,30,32] |
P6 | Distribution & Logistics service | The smooth flow of goods in the food supply chain will depend heavily on distribution channels and logistics services. | [5,18,33,34] |
P7 | Product quality & Safety | Fresh goods have stringent requirements for standard product quality and safety. It is the participation of the whole chain and must be guaranteed from the stage of planting and harvesting to the end stage. | [4,35,36] |
P8 | Contracting | Offering a variety of contracts for choose to ensure the benefits of each counterpart in the food supply chain. Thereby, it helps to reduce risks and conflicts among members. | [12,18,37,38] |
P9 | Technology adoption | Adopting the latest technology to the supply chain is part of the stakeholders' efforts to obtain sustainability, such as minimizing the impact on the natural environment, reducing costs. | [39,40,41] |
P10 | Customer | As the end-stage in the supply chain and consume products, the perceptions and reactions of this group is the driver and will significantly impact the sustainability initiatives of other stakeholders. | [20,34,42,43] |
P11 | Organizational social responsibilities | Conducting social programs for the local communities has been launched in the sustainable development goals. Forcing the various organization to perform. | [1,21,44,45] |
P12 | Collaboration | Collaboration among supply chain members would reinforce sustainability and obtain better performance by reducing financial constraints and production scale. | [5,6,31,46,47]. |
Category | Frequency | Percentage | |
Gender | Male | 7 | 70.0% |
Female | 3 | 30.0% | |
Age group | 31-40 | 3 | 30.0% |
41-50 | 5 | 50.0% | |
51-60 | 1 | 10.0% | |
over 60 | 1 | 10.0% | |
Degree | Bachelor degree | 4 | 40.0% |
Master degree | 4 | 40.0% | |
Ph.D. degree | 2 | 20.0% | |
Organizational | Process Manufacturing | 6 | 60.0% |
Service | 4 | 40.0% | |
Working Position | Top Managers | 3 | 30.0% |
Sales Department | 3 | 30.0% | |
Financial Management | 1 | 10.0% | |
Human Resource Management | 2 | 20.0% | |
Production Management | 1 | 10.0% |
Parameters | E1 | E2 | E3 | E4 | E5 | E6 | E7 | E8 | E9 | E10 |
Weight | 0.13 | 0.10 | 0.18 | 0.12 | 0.11 | 0.11 | 0.06 | 0.05 | 0.05 | 0.09 |
P1 | 0.0414 | 0.0319 | 0.0574 | 0.0489 | 0.0315 | 0.0160 | 0.0142 | 0.0177 | 0.0052 | 0.0276 |
P2 | 0.0322 | 0.0283 | 0.0574 | 0.0381 | 0.0315 | 0.0279 | 0.0142 | 0.0132 | 0.0173 | 0.0221 |
P3 | 0.0460 | 0.0212 | 0.0574 | 0.0272 | 0.0276 | 0.0359 | 0.0142 | 0.0132 | 0.0138 | 0.0166 |
P4 | 0.0184 | 0.0319 | 0.0319 | 0.0272 | 0.0394 | 0.0279 | 0.0170 | 0.0155 | 0.0173 | 0.0276 |
P5 | 0.0322 | 0.0177 | 0.0447 | 0.0326 | 0.0355 | 0.0319 | 0.0227 | 0.0132 | 0.0173 | 0.0276 |
P6 | 0.0414 | 0.0319 | 0.0574 | 0.0272 | 0.0315 | 0.0359 | 0.0198 | 0.0110 | 0.0173 | 0.0276 |
P7 | 0.0414 | 0.0354 | 0.0319 | 0.0326 | 0.0315 | 0.0359 | 0.0142 | 0.0110 | 0.0173 | 0.0276 |
P8 | 0.0414 | 0.0354 | 0.0574 | 0.0272 | 0.0355 | 0.0319 | 0.0113 | 0.0132 | 0.0138 | 0.0276 |
P9 | 0.0368 | 0.0248 | 0.0447 | 0.0326 | 0.0276 | 0.0359 | 0.0198 | 0.0177 | 0.0173 | 0.0276 |
P10 | 0.0414 | 0.0319 | 0.0574 | 0.0381 | 0.0276 | 0.0319 | 0.0198 | 0.0132 | 0.0017 | 0.0221 |
P11 | 0.0276 | 0.0212 | 0.0574 | 0.0326 | 0.0276 | 0.0279 | 0.0170 | 0.0199 | 0.0104 | 0.0276 |
P12 | 0.0414 | 0.0283 | 0.0574 | 0.0435 | 0.0315 | 0.0359 | 0.0198 | 0.0110 | 0.0138 | 0.0276 |
Ideal Solutions | E1 | E2 | E3 | E4 | E5 | E6 | E7 | E8 | E9 | E10 |
Positive (A+) | 0.0460 | 0.0354 | 0.0574 | 0.0489 | 0.0394 | 0.0359 | 0.0227 | 0.0199 | 0.0173 | 0.0276 |
Negative (A−) | 0.0184 | 0.0177 | 0.0319 | 0.0272 | 0.0276 | 0.0160 | 0.0113 | 0.0110 | 0.0017 | 0.0166 |
Parameters | E1 | E2 | E3 | E4 | E5 | E6 | E7 | E8 | E9 | E10 |
P1 | 0.0046 | 0.0035 | 0 | 0 | 0.0079 | 0.0200 | 0.0085 | 0.0022 | 0.0121 | 0 |
P2 | 0.0138 | 0.0071 | 0 | 0.0109 | 0.0079 | 0.0080 | 0.0085 | 0.0066 | 0 | 0.0055 |
P3 | 0 | 0.0142 | 0 | 0.0218 | 0.0118 | 0 | 0.0085 | 0.0066 | 0.0035 | 0.0110 |
P4 | 0.0276 | 0.0035 | 0.0255 | 0.0218 | 0 | 0.0080 | 0.0057 | 0.0044 | 0 | 0 |
P5 | 0.0138 | 0.0177 | 0.0128 | 0.0163 | 0.0039 | 0.0040 | 0 | 0.0066 | 0 | 0 |
P6 | 0.0046 | 0.0035 | 0 | 0.0218 | 0.0079 | 0 | 0.0028 | 0.0088 | 0 | 0 |
P7 | 0.0046 | 0 | 0.0255 | 0.0163 | 0.0079 | 0 | 0.0085 | 0.0088 | 0 | 0 |
P8 | 0.0046 | 0 | 0 | 0.0218 | 0.0039 | 0.0040 | 0.0113 | 0.0066 | 0.0035 | 0 |
P9 | 0.0092 | 0.0106 | 0.0128 | 0.0163 | 0.0118 | 0 | 0.0028 | 0.0022 | 0 | 0 |
P10 | 0.0046 | 0.0035 | 0 | 0.0109 | 0.0118 | 0.0040 | 0.0028 | 0.0066 | 0.0155 | 0.0055 |
P11 | 0.0184 | 0.0142 | 0 | 0.0163 | 0.0118 | 0.0080 | 0.0057 | 0 | 0.0069 | 0 |
P12 | 0.0046 | 0.0071 | 0 | 0.0054 | 0.0079 | 0 | 0.0028 | 0.0088 | 0.0035 | 0 |
Parameters | E1 | E2 | E3 | E4 | E5 | E6 | E7 | E8 | E9 | E10 |
P1 | 0.0230 | 0.0142 | 0.0255 | 0.0218 | 0.0039 | 0 | 0.0028 | 0.0066 | 0.0035 | 0.0110 |
P2 | 0.0138 | 0.0106 | 0.0255 | 0.0109 | 0.0039 | 0.0120 | 0.0028 | 0.0022 | 0.0155 | 0.0055 |
P3 | 0.0276 | 0.0035 | 0.0255 | 0 | 0 | 0.0200 | 0.0028 | 0.0022 | 0.0121 | 0 |
P4 | 0 | 0.0142 | 0 | 0 | 0.0118 | 0.0120 | 0.0057 | 0.0044 | 0.0155 | 0.0110 |
P5 | 0.0138 | 0 | 0.0128 | 0.0054 | 0.0079 | 0.0160 | 0.0113 | 0.0022 | 0.0155 | 0.0110 |
P6 | 0.0230 | 0.0142 | 0.0255 | 0 | 0.0039 | 0.0200 | 0.0085 | 0 | 0.0155 | 0.0110 |
P7 | 0.0230 | 0.0177 | 0 | 0.0054 | 0.0039 | 0.0200 | 0.0028 | 0 | 0.0155 | 0.0110 |
P8 | 0.0230 | 0.0177 | 0.0255 | 0 | 0.0079 | 0.0160 | 0 | 0.0022 | 0.0121 | 0.0110 |
P9 | 0.0184 | 0.0071 | 0.0128 | 0.0054 | 0 | 0.0200 | 0.0085 | 0.0066 | 0.0155 | 0.0110 |
P10 | 0.0230 | 0.0142 | 0.0255 | 0.0109 | 0 | 0.0160 | 0.0085 | 0.0022 | 0 | 0.0055 |
P11 | 0.0092 | 0.0035 | 0.0255 | 0.0054 | 0 | 0.0120 | 0.0057 | 0.0088 | 0.0086 | 0.0110 |
P12 | 0.0230 | 0.0106 | 0.0255 | 0.0163 | 0.0039 | 0.0200 | 0.0085 | 0 | 0.0121 | 0.0110 |
Parameters | s+i | s−i | CCi | Ranking |
Collaboration (P12) | 0.0162 | 0.0481 | 0.7485 | 1 |
Distribution & Logistic service (P6) | 0.0256 | 0.0472 | 0.6485 | 2 |
Customer (P10) | 0.0251 | 0.0431 | 0.6318 | 3 |
Contracting (P8) | 0.0266 | 0.0456 | 0.6313 | 4 |
Planning & Implementation (P1) | 0.0268 | 0.0453 | 0.6286 | 5 |
Knowledge & Training of fruit preservation (P2) | 0.0251 | 0.0389 | 0.6079 | 6 |
Technology adoption (P9) | 0.0279 | 0.0382 | 0.5777 | 7 |
Farming area/ farmer (P3) | 0.0326 | 0.0445 | 0.5772 | 8 |
Product quality & Safety (P7) | 0.0339 | 0.0407 | 0.5453 | 9 |
Warehouse/Storage (P5) | 0.0317 | 0.0346 | 0.5214 | 10 |
Organizational social responsibilities (P11) | 0.0330 | 0.0350 | 0.5151 | 11 |
Processing & Packaging (P4) | 0.0449 | 0.0300 | 0.4006 | 12 |
Coding | Parameters | Brief Description | Sources |
P1 | Planning & Implementation | The optimal design of the fresh fruit supply chain is inevitable, and it requires proper planning and implementation. | [4,7,20,21,22] |
P2 | Knowledge & Training of fruit preservation | The preservation of post-harvest products needs to apply the latest knowledge and disseminate it to the stakeholders of the chain, particularly in farms and micro corporates. | [4,23,24,25] |
P3 | Farming area/ farmer | As the first stage of the food supply chain, the essential role of farms to provide fresh products to the wholesalers, factories, and end customers. | [5,15,26,27,28] |
P4 | Processing & Packaging | Fresh products need to maintain optimum quality for the longest time. Therefore, standardization and synchronization of processing and packaging must be attention for all the stages. | [4,20,29,30,31] |
P5 | Warehouse/Storage | Warehouse acts as a transit station in the middle of the food supply chain, the quality of products will be significantly affected by storage operation in warehouses. | [18,24,30,32] |
P6 | Distribution & Logistics service | The smooth flow of goods in the food supply chain will depend heavily on distribution channels and logistics services. | [5,18,33,34] |
P7 | Product quality & Safety | Fresh goods have stringent requirements for standard product quality and safety. It is the participation of the whole chain and must be guaranteed from the stage of planting and harvesting to the end stage. | [4,35,36] |
P8 | Contracting | Offering a variety of contracts for choose to ensure the benefits of each counterpart in the food supply chain. Thereby, it helps to reduce risks and conflicts among members. | [12,18,37,38] |
P9 | Technology adoption | Adopting the latest technology to the supply chain is part of the stakeholders' efforts to obtain sustainability, such as minimizing the impact on the natural environment, reducing costs. | [39,40,41] |
P10 | Customer | As the end-stage in the supply chain and consume products, the perceptions and reactions of this group is the driver and will significantly impact the sustainability initiatives of other stakeholders. | [20,34,42,43] |
P11 | Organizational social responsibilities | Conducting social programs for the local communities has been launched in the sustainable development goals. Forcing the various organization to perform. | [1,21,44,45] |
P12 | Collaboration | Collaboration among supply chain members would reinforce sustainability and obtain better performance by reducing financial constraints and production scale. | [5,6,31,46,47]. |
Category | Frequency | Percentage | |
Gender | Male | 7 | 70.0% |
Female | 3 | 30.0% | |
Age group | 31-40 | 3 | 30.0% |
41-50 | 5 | 50.0% | |
51-60 | 1 | 10.0% | |
over 60 | 1 | 10.0% | |
Degree | Bachelor degree | 4 | 40.0% |
Master degree | 4 | 40.0% | |
Ph.D. degree | 2 | 20.0% | |
Organizational | Process Manufacturing | 6 | 60.0% |
Service | 4 | 40.0% | |
Working Position | Top Managers | 3 | 30.0% |
Sales Department | 3 | 30.0% | |
Financial Management | 1 | 10.0% | |
Human Resource Management | 2 | 20.0% | |
Production Management | 1 | 10.0% |
Parameters | E1 | E2 | E3 | E4 | E5 | E6 | E7 | E8 | E9 | E10 |
Weight | 0.13 | 0.10 | 0.18 | 0.12 | 0.11 | 0.11 | 0.06 | 0.05 | 0.05 | 0.09 |
P1 | 0.0414 | 0.0319 | 0.0574 | 0.0489 | 0.0315 | 0.0160 | 0.0142 | 0.0177 | 0.0052 | 0.0276 |
P2 | 0.0322 | 0.0283 | 0.0574 | 0.0381 | 0.0315 | 0.0279 | 0.0142 | 0.0132 | 0.0173 | 0.0221 |
P3 | 0.0460 | 0.0212 | 0.0574 | 0.0272 | 0.0276 | 0.0359 | 0.0142 | 0.0132 | 0.0138 | 0.0166 |
P4 | 0.0184 | 0.0319 | 0.0319 | 0.0272 | 0.0394 | 0.0279 | 0.0170 | 0.0155 | 0.0173 | 0.0276 |
P5 | 0.0322 | 0.0177 | 0.0447 | 0.0326 | 0.0355 | 0.0319 | 0.0227 | 0.0132 | 0.0173 | 0.0276 |
P6 | 0.0414 | 0.0319 | 0.0574 | 0.0272 | 0.0315 | 0.0359 | 0.0198 | 0.0110 | 0.0173 | 0.0276 |
P7 | 0.0414 | 0.0354 | 0.0319 | 0.0326 | 0.0315 | 0.0359 | 0.0142 | 0.0110 | 0.0173 | 0.0276 |
P8 | 0.0414 | 0.0354 | 0.0574 | 0.0272 | 0.0355 | 0.0319 | 0.0113 | 0.0132 | 0.0138 | 0.0276 |
P9 | 0.0368 | 0.0248 | 0.0447 | 0.0326 | 0.0276 | 0.0359 | 0.0198 | 0.0177 | 0.0173 | 0.0276 |
P10 | 0.0414 | 0.0319 | 0.0574 | 0.0381 | 0.0276 | 0.0319 | 0.0198 | 0.0132 | 0.0017 | 0.0221 |
P11 | 0.0276 | 0.0212 | 0.0574 | 0.0326 | 0.0276 | 0.0279 | 0.0170 | 0.0199 | 0.0104 | 0.0276 |
P12 | 0.0414 | 0.0283 | 0.0574 | 0.0435 | 0.0315 | 0.0359 | 0.0198 | 0.0110 | 0.0138 | 0.0276 |
Ideal Solutions | E1 | E2 | E3 | E4 | E5 | E6 | E7 | E8 | E9 | E10 |
Positive (A+) | 0.0460 | 0.0354 | 0.0574 | 0.0489 | 0.0394 | 0.0359 | 0.0227 | 0.0199 | 0.0173 | 0.0276 |
Negative (A−) | 0.0184 | 0.0177 | 0.0319 | 0.0272 | 0.0276 | 0.0160 | 0.0113 | 0.0110 | 0.0017 | 0.0166 |
Parameters | E1 | E2 | E3 | E4 | E5 | E6 | E7 | E8 | E9 | E10 |
P1 | 0.0046 | 0.0035 | 0 | 0 | 0.0079 | 0.0200 | 0.0085 | 0.0022 | 0.0121 | 0 |
P2 | 0.0138 | 0.0071 | 0 | 0.0109 | 0.0079 | 0.0080 | 0.0085 | 0.0066 | 0 | 0.0055 |
P3 | 0 | 0.0142 | 0 | 0.0218 | 0.0118 | 0 | 0.0085 | 0.0066 | 0.0035 | 0.0110 |
P4 | 0.0276 | 0.0035 | 0.0255 | 0.0218 | 0 | 0.0080 | 0.0057 | 0.0044 | 0 | 0 |
P5 | 0.0138 | 0.0177 | 0.0128 | 0.0163 | 0.0039 | 0.0040 | 0 | 0.0066 | 0 | 0 |
P6 | 0.0046 | 0.0035 | 0 | 0.0218 | 0.0079 | 0 | 0.0028 | 0.0088 | 0 | 0 |
P7 | 0.0046 | 0 | 0.0255 | 0.0163 | 0.0079 | 0 | 0.0085 | 0.0088 | 0 | 0 |
P8 | 0.0046 | 0 | 0 | 0.0218 | 0.0039 | 0.0040 | 0.0113 | 0.0066 | 0.0035 | 0 |
P9 | 0.0092 | 0.0106 | 0.0128 | 0.0163 | 0.0118 | 0 | 0.0028 | 0.0022 | 0 | 0 |
P10 | 0.0046 | 0.0035 | 0 | 0.0109 | 0.0118 | 0.0040 | 0.0028 | 0.0066 | 0.0155 | 0.0055 |
P11 | 0.0184 | 0.0142 | 0 | 0.0163 | 0.0118 | 0.0080 | 0.0057 | 0 | 0.0069 | 0 |
P12 | 0.0046 | 0.0071 | 0 | 0.0054 | 0.0079 | 0 | 0.0028 | 0.0088 | 0.0035 | 0 |
Parameters | E1 | E2 | E3 | E4 | E5 | E6 | E7 | E8 | E9 | E10 |
P1 | 0.0230 | 0.0142 | 0.0255 | 0.0218 | 0.0039 | 0 | 0.0028 | 0.0066 | 0.0035 | 0.0110 |
P2 | 0.0138 | 0.0106 | 0.0255 | 0.0109 | 0.0039 | 0.0120 | 0.0028 | 0.0022 | 0.0155 | 0.0055 |
P3 | 0.0276 | 0.0035 | 0.0255 | 0 | 0 | 0.0200 | 0.0028 | 0.0022 | 0.0121 | 0 |
P4 | 0 | 0.0142 | 0 | 0 | 0.0118 | 0.0120 | 0.0057 | 0.0044 | 0.0155 | 0.0110 |
P5 | 0.0138 | 0 | 0.0128 | 0.0054 | 0.0079 | 0.0160 | 0.0113 | 0.0022 | 0.0155 | 0.0110 |
P6 | 0.0230 | 0.0142 | 0.0255 | 0 | 0.0039 | 0.0200 | 0.0085 | 0 | 0.0155 | 0.0110 |
P7 | 0.0230 | 0.0177 | 0 | 0.0054 | 0.0039 | 0.0200 | 0.0028 | 0 | 0.0155 | 0.0110 |
P8 | 0.0230 | 0.0177 | 0.0255 | 0 | 0.0079 | 0.0160 | 0 | 0.0022 | 0.0121 | 0.0110 |
P9 | 0.0184 | 0.0071 | 0.0128 | 0.0054 | 0 | 0.0200 | 0.0085 | 0.0066 | 0.0155 | 0.0110 |
P10 | 0.0230 | 0.0142 | 0.0255 | 0.0109 | 0 | 0.0160 | 0.0085 | 0.0022 | 0 | 0.0055 |
P11 | 0.0092 | 0.0035 | 0.0255 | 0.0054 | 0 | 0.0120 | 0.0057 | 0.0088 | 0.0086 | 0.0110 |
P12 | 0.0230 | 0.0106 | 0.0255 | 0.0163 | 0.0039 | 0.0200 | 0.0085 | 0 | 0.0121 | 0.0110 |
Parameters | s+i | s−i | CCi | Ranking |
Collaboration (P12) | 0.0162 | 0.0481 | 0.7485 | 1 |
Distribution & Logistic service (P6) | 0.0256 | 0.0472 | 0.6485 | 2 |
Customer (P10) | 0.0251 | 0.0431 | 0.6318 | 3 |
Contracting (P8) | 0.0266 | 0.0456 | 0.6313 | 4 |
Planning & Implementation (P1) | 0.0268 | 0.0453 | 0.6286 | 5 |
Knowledge & Training of fruit preservation (P2) | 0.0251 | 0.0389 | 0.6079 | 6 |
Technology adoption (P9) | 0.0279 | 0.0382 | 0.5777 | 7 |
Farming area/ farmer (P3) | 0.0326 | 0.0445 | 0.5772 | 8 |
Product quality & Safety (P7) | 0.0339 | 0.0407 | 0.5453 | 9 |
Warehouse/Storage (P5) | 0.0317 | 0.0346 | 0.5214 | 10 |
Organizational social responsibilities (P11) | 0.0330 | 0.0350 | 0.5151 | 11 |
Processing & Packaging (P4) | 0.0449 | 0.0300 | 0.4006 | 12 |