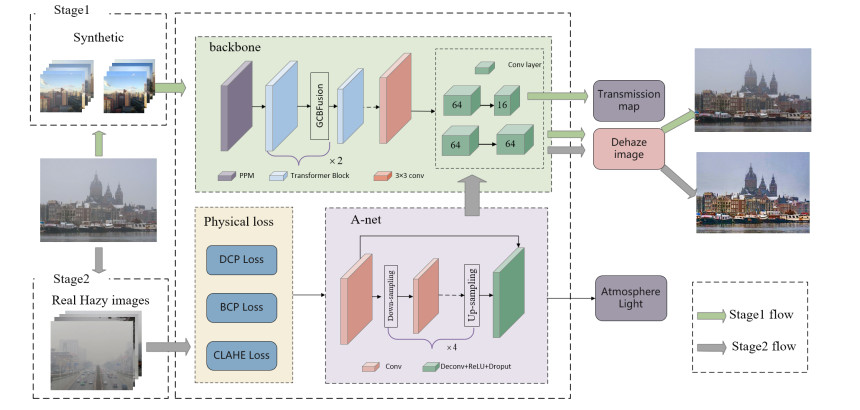
Aiming at the problems of local dehazing distortion and incomplete global dehazing of existing algorithms in real airborne cockpit environments, a two-stage dehazing method PhysiFormer combining physical a priori with a Transformer oriented flight perspective was proposed. The first stage used synthetic pairwise data to pre-train the dehazing model. First, a pyramid pooling module (PPM) was introduced in the Transformer for multiscale feature extraction to solve the problem of poor recovery of local details, then a global context fusion mechanism was used to enable the model to better perceive global information. Finally, considering that combining the physical a priori needs to rely on the estimation of the atmosphere light, an encoding-decoding structure based on the residual blocks was used to estimate the atmosphere light, which was then used for dehazing through the atmospheric scattering model for dehazing. The second stage used real images combined with physical priori to optimize the model to better fit the real airborne environment. The experimental results show that the proposed method has better naturalness image quality evaluator (NIQE) and blind/referenceless image spatial quality evaluator (BRISQUE) indexes and exhibits the best dehazing visual effect in the tests of dense haze, non-uniform haze and real haze images, which effectively improves the problems of color distortion and haze residue.
Citation: Tian Ma, Huimin Zhao, Xue Qin. A dehazing method for flight view images based on transformer and physical priori[J]. Mathematical Biosciences and Engineering, 2023, 20(12): 20727-20747. doi: 10.3934/mbe.2023917
[1] | Li Wang, Changyuan Wang, Yu Zhang, Lina Gao . An integrated neural network model for eye-tracking during human-computer interaction. Mathematical Biosciences and Engineering, 2023, 20(8): 13974-13988. doi: 10.3934/mbe.2023622 |
[2] | Ning He, Hongmei Jin, Hong'an Li, Zhanli Li . A global optimization generation method of stitching dental panorama with anti-perspective transformation. Mathematical Biosciences and Engineering, 2023, 20(9): 17356-17383. doi: 10.3934/mbe.2023772 |
[3] | Jun Chen, Yuan Xiao, Gangfeng Wang, Biao Guo . Research on the integrated management and mapping method of BOM multi-view for complex products. Mathematical Biosciences and Engineering, 2023, 20(7): 12682-12699. doi: 10.3934/mbe.2023565 |
[4] | Weijie Lu, Yonghui Xia, Yuzhen Bai . Periodic solution of a stage-structured predator-prey model incorporating prey refuge. Mathematical Biosciences and Engineering, 2020, 17(4): 3160-3174. doi: 10.3934/mbe.2020179 |
[5] | Xu Song, Jingyu Li . Asymptotic stability of spiky steady states for a singular chemotaxis model with signal-suppressed motility. Mathematical Biosciences and Engineering, 2022, 19(12): 13988-14028. doi: 10.3934/mbe.2022652 |
[6] | Dayu Xiao, Jianhua Li, Ruotong Zhao, Shouliang Qi, Yan Kang . Iterative CT reconstruction based on ADMM using shearlet sparse regularization. Mathematical Biosciences and Engineering, 2022, 19(12): 11840-11853. doi: 10.3934/mbe.2022552 |
[7] | James C. L. Chow . Computational physics and imaging in medicine. Mathematical Biosciences and Engineering, 2025, 22(1): 106-108. doi: 10.3934/mbe.2025005 |
[8] | Jianguo Xu, Cheng Wan, Weihua Yang, Bo Zheng, Zhipeng Yan, Jianxin Shen . A novel multi-modal fundus image fusion method for guiding the laser surgery of central serous chorioretinopathy. Mathematical Biosciences and Engineering, 2021, 18(4): 4797-4816. doi: 10.3934/mbe.2021244 |
[9] | Xinlin Liu, Viktor Krylov, Su Jun, Natalya Volkova, Anatoliy Sachenko, Galina Shcherbakova, Jacek Woloszyn . Segmentation and identification of spectral and statistical textures for computer medical diagnostics in dermatology. Mathematical Biosciences and Engineering, 2022, 19(7): 6923-6939. doi: 10.3934/mbe.2022326 |
[10] | Tian Ma, Boyang Meng, Jiayi Yang, Nana Gou, Weilu Shi . A half jaw panoramic stitching method of intraoral endoscopy images based on dental arch arrangement. Mathematical Biosciences and Engineering, 2024, 21(1): 494-522. doi: 10.3934/mbe.2024022 |
Aiming at the problems of local dehazing distortion and incomplete global dehazing of existing algorithms in real airborne cockpit environments, a two-stage dehazing method PhysiFormer combining physical a priori with a Transformer oriented flight perspective was proposed. The first stage used synthetic pairwise data to pre-train the dehazing model. First, a pyramid pooling module (PPM) was introduced in the Transformer for multiscale feature extraction to solve the problem of poor recovery of local details, then a global context fusion mechanism was used to enable the model to better perceive global information. Finally, considering that combining the physical a priori needs to rely on the estimation of the atmosphere light, an encoding-decoding structure based on the residual blocks was used to estimate the atmosphere light, which was then used for dehazing through the atmospheric scattering model for dehazing. The second stage used real images combined with physical priori to optimize the model to better fit the real airborne environment. The experimental results show that the proposed method has better naturalness image quality evaluator (NIQE) and blind/referenceless image spatial quality evaluator (BRISQUE) indexes and exhibits the best dehazing visual effect in the tests of dense haze, non-uniform haze and real haze images, which effectively improves the problems of color distortion and haze residue.
The aircraft cockpit is the main place for pilots to perform their tasks, and pilots must rely on the information display in the cockpit to obtain flight tasks. Vision-based intelligent cockpit systems are susceptible to the influence of bad weather, especially under hazy conditions, and the images displayed in the cockpit are degraded to varying degrees in terms of object visibility, color fidelity and edge information, which can seriously affect the pilot's judgment of the surrounding environment. Therefore, it is of great significance to study the dehazing method for the airborne cockpit environment to reduce the influence of the hazy environment on flight operation.
Some of the earlier proposed dehazing methods are mostly based on the typical atmospheric scattering model [1], which considers incident light attenuation and scattering medium effects as the main factors leading to image quality degradation; atmosphere light coefficients and the transmission map can be estimated to derive the dehazed image using this model. Traditional algorithms usually utilize various images a priori knowledge to estimate the parameters in the atmospheric scattering model [2,3,4,5] to obtain haze-free imaging. Although the algorithm is able to improve the visibility of hazy images to a certain extent, the various a priori assumptions proposed are not sufficient to reflect the characteristics of the real image, and the dehazing effect is often limited.
In recent years, researchers have proposed a large number of deep learning-based dehazing algorithms, which usually use deep learning models such as convolutional neural networks (CNN) to realize image dehazing. Although these algorithms can estimate the unknown parameters more accurately compared to the traditional methods, they still essentially rely on the atmospheric scattering model and cannot recover the image perfectly. In order to solve the problem of inaccurate estimation of atmosphere light and transmission map, some CNN methods directly restore hazy images to clear images, and common end-to-end dehazing algorithms include densely connected pyramid dehazing network (DCPDN)[6], gated context aggregation network (GCANet)[7] and feature fusion attention network (FFANet)[8]. However, they use convolution operations excessively, focus heavly on detailed information and ignore global information, so the dehazing effect is not ideal.
Recently, some researches applied the Transformer to the image dehaze task. Gao et al. [9] designed a Transformer-based channel space attention module in the dehazing network and used a multiscale parallel residual network as a backbone to extract the feature information of different scales for feature fusion. Li et al. [10] proposed a hybrid dehazing network that combined the CNN and the Vision Transformer hybrid dehazing network to capture the local and global features of haze images, respectively. The DehazeFormer [11] method improves the normalization layer, activation function and spatial information aggregation strategy on the basis of the Swin Transformer [12], which makes the network architecture based on the Transformer-based network architecture more suitable for dealing with the dehazing problem. The above methods compared to the CNN dehazing can capture long-distance dependencies, solving the problem of the lack of image details when image dehazing. This method is used for real haze image dehazing, but the effect is poor; there is a color distortion produced on the artifacts, especially for the on-board cockpit viewpoint of the fog concentration of the complex task of the environment. The dehazing is not complete and will seriously affect the pilot's ability to carry out the flight task.
In summary, this paper proposes a two-stage dehazing method PhysiFormer for airborne cockpit images that combines the Transformer with physical priori. The first stage uses synthetic pairwise data to pre-train the dehazing model, and the second stage uses real haze images combined with physical a priori to optimize the model with the trained model in a semi-supervised way in order to improve the model's robustness and generalization ability in real airborne scenarios. First, the Transformer model is improved by introducing the pooling pyramid module (PPM) for multiscale feature extraction and a global context fusion (GCBFusion) mechanism to enable the model to better perceive the global information. Then, two convolutional layers are used for decomposition to generate the transmission maps. And finally, considering that combining the physical a priori needs to rely on the atmosphere light parameters, a residual block-based encoding-decoding structure is used to estimate the atmosphere light, and the atmospheric scattering model is utilized for dehazing.
The main innovations are as follows: 1) We introduce the PPM in the Transformer model to obtain multiscale haze image features and also use the GCBFusion module to model the global information to fuse different levels of features, which is able to better recover the detail and texture information of the image. 2) Semi-supervised training based on synthesized and real haze images are combined with physical prior knowledge to make the model more conducive to real cockpit environment dehazing. 3) The PhysiFormer image dehazing method is proposed, and the test results on dense haze, non-uniform haze and haze images from aerial flight view show excellent performance, which can be used in real airborne environments.
This paper is divided into five parts. Chapter 1 is the introduction where the background of the dehazing algorithm for the airborne cockpit is introduced and the disadvantages of the existing dehazing methods are analyzed in detail so as to propose improvements. Chapter 2 is the related work and existing domestic and international dehazing methods are analyzed from different perspectives with advantages and disadvantages. Chapter 3 is the proposed methods, providing a detailed description of the proposed improved method PhysiFormer, including the overall framework as well as the specific implementation of each module and finally explaining the loss function design. Chapter 4 is the experiments where the proposed method is experimentally analyzed in dense haze, non-uniform haze and haze images from real aerial flight views to verify the effectiveness of the proposed method. Chapter 5 is the conclusions which summarizes and explains the next research plan of the proposed method.
Early image dehazing methods were generally based on manual a priori [13,14,15,16], such as dark channel prior (DCP) [2], color attenuation prior (CAP) [3], color line [4] and fog line [5]. These a priori knowledge are derived from observations and certain assumptions about a specific scene, and their generalizability needs to be improved. Therefore, the performance of these traditional model-based methods can be inherently limited by the specificity of the a priori knowledge.
With the rapid development of deep learning[17], Cai et al. [18] proposed the DehazeNet dehazing network to estimate the transmission map directly from hazy images using a three-layer CNN. Cai et al. [19] proposed an All-in-one dehazing network (AOD-Net) to estimate a transmission map and atmosphere light alone, which generates cumulative errors that are not conducive to reconstructing clear images and is a sub-optimal solution algorithm. Zhang et al. [6] proposed a densely connected pyramidal dehazing algorithm based on the Generative Adversarial Network (GAN) structure to estimate the transmission map, where the atmosphere light is estimated by a traditional method and the results are obtained using a degenerate model. Since the use of a priori information has some limitations and the reasonableness and subjectivity of a priori information selection will also affect the estimation of model parameters and the final dehazing effect to a large extent, some methods do not rely on the estimation of parameters and directly restore the hazy images to a hazy-free image. Qu et al. [20] proposed an enhanced pix2pix dehazing network using the idea of coarse-to-fine learning. Qin et al. [8] proposed an FFANet using channel and pixel attention mechanisms. Zhu et al. [21] proposed a multi-stream fusion network (MSFNet) by fusing features from three resolution scales. Long et al. [22] proposed a Bi-Shift Network based approach to remove clouds from optical remote sensing images. Liu et al. [23] proposed a new physical model based heavy haze network and a new augmentation network to supervise the mapping from hazy domain to haze free domain.
Recent works [7,24,25,26,27,28,29] tend to directly estimate the residuals between the hazy-free and hazy images. However, when these methods are extended to real hazy images, the domain gap between the synthetic and real data may lead to significant performance degradation.
Transformer [30] is a deep neural network based entirely on the attention mechanism, which has led to many breakthroughs in the field of natural language processing (NLP). Influenced by the powerful representational capabilities of the Transformer, researchers have tried to apply the Transformer to vision tasks [31,32,33]. The computational complexity of the Vision Transformer (ViT) [33] is the square of the number of pixels, and the huge computational cost limits its development in vision tasks. To solve the above problem, Swin Transformer [12] utilizes local prior knowledge to decompose features of original size by non-overlapping windows and performing region self-attentive computation only within each window, which reduces the computational complexity to a linear scale of the number of pixels. However, the window-based design limits the integration of contextual feature information within the local region and still does not allow effective modeling of global dependencies. Based on this, Zamir et al. [34] proposed Restormer, which utilizes a deep convolutional multi-head transpose attention mechanism (MDTA). However, this mechanism does not directly compute self-attention on pixels but models the global contextual relations in the channel dimension, which greatly reduces the time complexity. Qiu et al. [35] proposed a new multi-branch linear Transformer network MB-TaylorFormer, through the multi-branch and multiscale structure to extract with a multiscale sensory field. Thus, multi-level semantic information can effectively and efficiently carry out the image dehazing task. The DehazeFormer [11] improves the normalization layer, activation function, and spatial information aggregation scheme on top of the Swin Transformer, making the Transformer-based network architecture more suitable for handling the dehazing problem. Due to the superiority of DehazeFormer compared to other image dehazing tasks, it is used as the backbone network in this paper.
The proposed two-stage dehazing framework PhysiFormer of Transformer combined with physical prior is shown in Figure 1. The first stage is based on synthesizing a dehazing model pre-trained on paired data. First, multiscale features are extracted by PPM, which is a module that can capture the details and overall information in the image through different scales and then input into the backbone network to output the feature maps; feature fusion is then carried out by using the GCBFusion mechanism so that the model can better perceive the global information and finally it is decomposed by using two convolutional layers in order to generate the transmission maps. Since the physical prior is related to the three parameters atmosphere light, haze-free image and transmission maps, an independent residual block-based encoding-decoding structure is designed to estimate the atmosphere light. In the second stage, the model is optimized in a semi-supervised manner using real hazy images combined with physical prior, and the model is optimized by DCP loss, Bright Channel Prior (BCP) loss and Contrast Limited Adaptive Histogram Equalization (CLAHE) loss using real hazy images as inputs, and by optimizing the loss function in order to make the generated hazy-free image closer to the real image. The specific algorithm is described below. The training process of PhysiFormer is shown in Algorithm 1.
Algorithm 1: The training procedure for our network |
Stage1: |
Input: The hazy image set, , The clear image set, |
Output: The dehazed image: |
![]() |
Stage2: |
Input: The hazy image set, |
Output: The dehazed image: |
![]() |
The traditional single input tends to lead to the loss of many useful details and local features. In this paper, the haze features are extracted using a PPM with the structure shown in Figure 2, with a total of four layers of structure, each of which is divided into 1 × 1, 2 × 2, 3 × 3 and 6 × 6 in terms of the size of each layer. First, it goes through a down-sampling convolution operation, and then features are extracted at different scales by adaptive averaging pooling and convolution layers. Second, the feature maps at each scale are resized to the same size as the input feature maps by the up-sampling operation. Finally, the up-sampled feature map is stitched with the original down-sampled feature map and processed by a convolution layer, batch normalization layer, and activation function, and then the fused feature map is obtained. The module is able to extract features from spatial information at different scales by performing spatial pyramid pooling and convolution operations on feature maps of different sizes. Through the fusion of multiscale features, the model can better perceive the information of objects of different sizes and improve the perceptiveness and accuracy of the dehazing effect.
For feature fusion, the selective kernel fusion (SKFusion) mechanism is used in the Transformer's approach. Since the dehazing task requires the processing of the entire image, including the processes of sensing fog density, estimating transmittance, and recovering hazy-free images, SKFusion's spatial attention mechanism is based on pixel-level selection and fusion, which can limit SKFusion's performance for global contextual information across long distances. In image dehazing, global contextual information is important for the correct estimation of transmittance and the recovery of hazy-free images. Therefore, this paper uses the GlobalContextBlock (GCBlock) module instead of the original fusion module to introduce global context information. The GCBlock module structure diagram is shown in Figure 3. First, the global information relationship vector is obtained by , then the two-layer convolution can reduce the number of parameters and further extract information, and finally, the global contextual information is multiplied with the input feature map at the element level to obtain the final feature map output. The GCBFusion module consists of several GCBlock modules, each used to enhance the model's ability to perceive global contextual information. The input multiple feature maps are stacked and shape-transformed to obtain a stacked feature map, and then the final output feature map is obtained through a series of computation and fusion operations, which can better handle global information and improve the accuracy of transmittance estimation and the recovery of hazy-free images.
In the second stage of the PhysiFormer, we combine the dehazing model with the physical method to improve the accuracy and robustness of the dehazing effect. Since the physical method needs to rely on the estimation of atmosphere light, we designed an atmosphere light estimation network based on the residual block-based encoding-decoding structure in hazy images to improve its generalization to different scenes.
The mathematical description of the atmospheric scattering model can be expressed as:
(3.1) |
where is the captured hazy image, is the latent haze-free image, is the global atmospheric light and is the medium transmission map. According to the image degradation model, for a given image we assume that the atmospheric light map is homogeneous and the predicted atmospheric light is a 2D map where each pixel has the same value. Thus, it has the same feature size as the input image and the two-layer convolution in is filled with the same values. Atmospheric light as a network-independent module is shown in Figure 4 with an 8-block U-net [7] structure, where the encoder consists of 4 blocks and the decoder consists of symmetric blocks. Establish connectivity between the backbone and subnets through reconfiguration losses.
In the second stage, the model is optimized using physical losses, starting with the DCP, which is a loss designed for the dehazing task, exploits the statistical laws in the hazy image, and is able to effectively estimate the degree of haze in the image so as to recover more details of the image. Its loss function can be expressed as:
(3.2) |
where and represent the transmission estimates for the DCP and backbone networks. Although greatly improves the performance of the model on real blurred images, it has the side effect that the dehazing results are usually darker than expected. Therefore, we add a BCP.
BCP addresses the problem of image quality degradation due to insufficient light and helps to make the resulting image brighter and enhance contrast. Its loss function can be expressed as:
(3.3) |
where and represent the transmission estimates for the BCP and backbone networks. compensates for the drawbacks associated with by significantly improving the global illumination of the recovered image and restoring more detail.
In order to strike a balance between and , CLAHE is added to rebuild the loss to compensate for the difference between DCP and BCP, and to balance the effect between DCP and BCP which can maintain the clarity and detail of the image and make the image dehazing process more comprehensive.The loss function of the network output , and the result of is reconstructed as the original input by the scattering model (1), which can be expressed as:
(3.4) |
where is the original input.
Then, the loss function is redefined as:
(3.5) |
where , and are the tradeoff weights.
Physical priors usually do not handle the sky in the image correctly, which leads to artifacts and color shifts. Therefore, the original pixel values of the sky region should be preserved as much as possible by introducing the following loss function:
(3.6) |
where is a binary mask representing the sky region, and and are images recovered from m and .
In order to update both atmosphere light and transmission maps, the reconstruction loss is introduced, the network outputs , and are aggregated by a physical scattering model and the original inputs are reconstructed , integrating the various modules of the network for simultaneous optimization. The is defined as:
(3.7) |
where is the original input.
Finally, the total loss function can be expressed as:
(3.8) |
Dataset: Since large-scale accurate paired haze datasets are difficult to obtain in real airborne, a synthetic paired dataset was used for training in the first phase. We randomly choose 6000 synthetic pairs of outdoor training sets (OTS) from the RESIDE Dataset [36] and 45 pairs of images from the non-homogeneous hazy (NH-HAZE)[37] real hazy images dataset as the training data for the first stage, and choose the real hazy images from unannotated real hazy images (URHI) for the second stage. Tests were performed on the synthetic objective testing set (SOTS), NH-HAZE and realworld task-driven testing set (RTTS) datasets, and in addition, real aerial video frames with different cockpit viewpoints were selected for the tests.
Training parameters: The first stage network was trained for 100 epochs using the Adam optimizer, with and . The initial learning rate was set to with a decay rate of 0.75 per 10 epochs. The second stage network was trained with 20 epochs and the initial learning rate is set to , decaying by 0.5 every two epochs. The tradeoff weights in the loss function are set to , , .
Comparison of methods: A priori based methods (e.g., DCP [2]); methods based on deep learning for direct haze-to-clear map conversion (e.g., GCANet [7], FFA-Net [8], Multi-Scale Boosted Dehazing Network (MSBDN) [38]) and Transformer-based methods (e.g., Dehazeformer[11], U2-Former[39], MB-TaylorFormer[35]). Specifically, DCP is a traditional defogging method based on the a priori belief that most images that do not contain a sky region have pixel points that have very low values in at least one of these channels. GCANet utilizes gated context aggregation network dehazing to directly recover the final haze-free image; FFA-Net proposes a feature fusion attention using channel and pixel attention mechanisms network; MSBDN designs a multiscale enhanced dehazing network with dense feature fusion using U-Net architecture; Dehazeformer improves the normalization layer in Swin Transformer, and the activation function and spatial information aggregation strategy make the Transformer model more conducive to the dehazing task; MB-TaylorFormer can effectively and efficiently perform image dehazing through multi-branch and multiscale structure extraction with a multiscale sense field and multi-level semantic information and U2-Former is based on the U-type Transformer, which is able to utilize the variator as the core operation to perform image restoration in the deep coding and decoding space (the source code of this method is not provided, and it is only compared with the evaluation indexes provided in the paper). In addition, the rest of the methods are experimented by retaining the original paper parameter settings and tested using the official code.
Evaluation metrics: For paired datasets, peak signal to noise ratio (PSNR) and structural similarity index (SSIM) are used as evaluation metrics to evaluate the experimental results. For unpaired datasets, naturalness image quality evaluator (NIQE) and blind/referenceless image spatial quality evaluator (BRISQUE) are used as evaluation indexes for evaluation. PSNR is used to describe the similarity between the pixels of the generated image and the reference image; the higher the PSNR value, the more similar the two images are. SSIM is a metric used to evaluate the similarity between the generated image and the reference image. In addition to the metrics that consider the differences between pixel values, SSIM also considers factors such as brightness, contrast and structure. SSIM takes a value in the range of [0, 1], and the closer the result is to 1, the more similar the generated image is to the reference image. NIQE denotes the naturalness image evaluation metrics based on comparing the algorithm processing results with the model calculated based on the natural scene, and BRISQUE denotes the reference-free quality score based on the images based on the natural scene images with similar distortions, and a smaller score for both indicates a better perceived quality.
Experiments were first conducted on the SOTS outdoor synthetic dataset and the dehazing results were analyzed quantitatively and qualitatively. Table 1 presents the quantitative comparison results of the proposed method with representative or contemporary methods with better performance. The proposed method achieves optimal performance in PSNR metrics. Compared to deep learning-based image dehazing methods, traditional DCP dehazing methods are much less effective, PhysiFormer and Dehazeformer have an improvement of 0.22 compared to PSNR and SSIM, although slightly lower than Dehazeformer, has an improvement compared to all other Transformer-based methods.
PSNR/SSIM | SOTS-outdoor | NH-HAZE |
DCP | 19.19/0.834 | 11.19/0.514 |
GCANet | 29.14/0.947 | 16.34/0.626 |
FFANet | 32.75/0.969 | 18.95/0.693 |
MSBDN | 34.68/0.975 | 19.97/0.705 |
U2-former | 31.10/0.976 | - - - |
Dehazeformer | 35.15/0.987 | 19.56/0.694 |
MB-TaylorFormer | 35.21/0.981 | 20.01/0.706 |
PhysiFormer | 35.37/0.983 | 20.37/0.716 |
The dehazing results of the 3 sets of outdoor images in the SOTS dataset are shown in Figure 5. It can be seen that the images after DCP and GCANet dehazing are obviously dark and the color of the sky region is distorted, such as magnified local blocks. Although the dehazing results of FFANet and MSBDN are greatly improved compared with DCP and GCANet and the distortion is reduced and there are still some haze residues in the dehazing results. Compared with the Transformer-based dehazing methods Dehazeformer and MB-TaylorFormer, it has achieved good results in the SOTS dataset, but it is not as good as PhysiFormer for the detail processing. For example there are enlarged red blocks in the first set of the test data in Figure 5, and the sky region is whitish compared to the GT map. In qualitative comparison, the evaluation indexes of the latter three methods are closer and PhysiFormer further improves the visual effect and recovers better, especially the regions marked with box lines in each test image; the recovery of detailed texture features is more obvious.
The test was performed on five images selected from the NH-HAZE dataset, which can effectively demonstrate the performance exhibited by each algorithm in the case of haze inhomogeneity encountered in flight. As shown in the data on the righthand side of Table 1 and the proposed method, it shows good performance in both PSNR and SSIM, which are improved by 0.81 and 0.022 dB, respectively compared to the Dehazeformer method.
Figure 6 shows a comparison of the effectiveness of each method for dehazing on the NH-HAZE dataset. It can be seen that compared with the synthetic dataset, the NH-HAZE non-uniform haze dataset is more challenging. Specifically, the traditional DCP dehazing method will lead to serious color deviation phenomenon after dehazing, and the color is bluish; the GCANet overall dehazing is darker; FFANet has a great improvement compared to DCP and GCANet, but there is obvious haze residue in the hazy area; MSBDN dehazing also has haze residue; the Dehazeformer method alleviates the problem of color deviation, but compared to the real haze-free map in the ground, the color of the tree branches, etc., there is still a problem of color distortion. MB-TaylorFormer has incomplete local dehazing, overall image color distortion and darker brightness. Compared to the above algorithms, PhysiFormer has better performance in recovering image details and avoiding the problem of color bias in dehazing it has better reconstruction effect on the near and far areas such as the ground where the haze is thicker and it can recover a clearer and more natural haze-free image.
In order to verify the effectiveness of the proposed method, 30 non-pairs of real hazy dataset RTTS are selected for testing and comparison experiments with other dehazing methods, and the experimental results are shown in Figure 7. DCP and GCANet dehazing results are overall dark, and the sky color is obviously distorted. FFANet haze removal effect is not complete, and there are still haze residues in some areas. MSBDN recovered images are still a little fuzzy, especially in the long view angle of the far-away area. The same Transformer-based method shows excellent performance in the SOTS-outdoors dataset, but its dehazing effect in real haze images is poor, Dehazeformer dehazing results are overall brownish, and MB-TaylorFormer suffers from poor dehazing of the edges of the object, brightness changes and other shortcomings (same as the effect on the NH-HAZE dataset). PhysiFormer solves this problem well, and the haze-free image has brighter details and clearer edges, which is more suitable for real haze images.
In order to verify the dehazing effect of the proposed method for use in airborne cockpits in real flight situations, a dataset of aerial video frames from different cockpit viewpoints was selected for testing. The experimental results are shown in Figure 8. Image1 demonstrates the flat view image of the airplane during normal flight, and Image2 demonstrates the overhead view image. The results of DCP and GCANet dehazing are overall dark, especially the enlarged red area in the figure, and the sky color distortion is obvious, with varying degrees of artifacts. FFANet and MSBDN dehazing is not complete and there is still a large area of haze residue, which makes the recovered image still a little fuzzy. Based on the Transformer's Dehazeformer method, although a better removal of the haze, the dehazing result is still overall brownishd and did not achieve the best visual effect. MB-TaylorFormer has a poor dehazing effect on the edges of objects, poor dehazing effect on the color of the building in the area marked by the red box and the overall image recovery is not smooth enough, which affects the visual effect. Physiformer well solves the limitations of previous methods, and the dehazing image is more natural and better retains the contour and color information of the object, which helps to enhance the pilot's perception of the environment, thus ensuring flight safety.
To further evaluate the dehazing performance of the proposed algorithms in real flight environments, quantitative comparisons are made using the no-reference image quality assessment metrics BRISQUE and NIQE. The quantitative results on RTTS and aerial video frames are shown in Table 2, where the optimal performance is achieved in both metric. Compared with MB-TaylorFormer, which has the second best performance, the performance of PhysiFormer is improved by -0.196 and -1.016 on RTTS and -0.089 and -0.764 on real aerial video frames, respectively. In addition, the performance of PhysiFormer compares favorably with that of Dehazeformer, which is also a Transformer-based method, indicating that PhysiFormer generates hazy-free images with better visual quality. This indicates that the fog-free images generated by PhysiFormer have better visual quality, and its overall image quality is more advantageous compared to other methods.
NIQE/BRISQUE | RTTS | Aerial image |
DCP | 3.579/34.858 | 3.465/33.476 |
GCANet | 3.523/28.576 | 3.584/30.239 |
FFANet | 3.456/36.702 | 3.843/36.468 |
MSBDN | 3.142/26.397 | 3.114/27.397 |
Dehazeformer | 3.294/27.895 | 3.164/27.793 |
MB-TaylorFormer | 3.102/25.967 | 3.106/27.183 |
PhysiFormer | 2.906/24.951 | 3.017/26.419 |
To validate the effectiveness of the proposed feature extraction, and fusion module and physical loss, the following four sets of ablation experiments are done on RTTS datasets and aerial video frames to compare the performance of the following four model architectures: M1 model removes the physical loss and only the first stage training model was used for testing; M2 model keeps the physical loss and removes the PPM feature extraction module; M3 model keeps the physical loss and PPM module and uses the SKFusion module in the original Dehazeformer for feature fusion; M4 model is the original method in this paper.
The results of the above ablation experiments on paired datasets are shown in Figures 9 and 10, and the results of the ablation experiments on unpaired datasets are shown in Figures 11 and 12. Only the first stage trained model was used for dehazing testing in experiment M1, and the results showed haze residuals and unclear haze removal images, which indicates the limited effect in processing haze images when removing physical losses, and the haze removal effect cannot be completely removed. The results of experiments M2 and M3 showed good ablation performance, with physical losses preserved and optimized for haze removal and detail restoration. However, compared to the original method in this paper, there are still haze residues in the distant area, such as the enlarged red area in the first group of Figure 11, indicating that the module being ablated will have an impact on detail processing.
The quantitative comparison of the ablation experiments is given in Tables 3 and 4, which shows the effect of each module in the experiments on the image quality and sharpness, which is improved by the M4 model on the RTTS dataset compared to the M1 model, the M2 model and the M3 model by (-0.361, 2.289), (-0.25, -1.48) and (-0.278, 1.724), respectively, and by the NH-HAZE dataset by (1.62, 0.115), (1.03, 0.029) and (0.81, 0.022), respectively. The M4 model improves (1.62, 0.115), (1.03, 0.029) and (0.81, 0.022) over the M1, M2 and M3 models, respectively, and these quantitative results further proved the effectiveness of the proposed method and demonstrated that the individual modules play different roles in the whole dehazing process.
Model No. | PPM | GCBFusion | Physical loss | PSNR | SSIM |
M1 | 18.75 | 0.601 | |||
M2 | 19.34 | 0.687 | |||
M3 | 19.56 | 0.694 | |||
M4 | 20.37 | 0.716 |
Model No. | PPM | GCBFusion | Physical loss | NIQE | BRISQUE |
M1 | 3.267 | 27.240 | |||
M2 | 3.156 | 26.431 | |||
M3 | 3.184 | 26.675 | |||
M4 | 2.906 | 24.951 |
Time is one of the key factors affecting the on-board cockpit display, so in addition to the subjective evaluation based on vision and the objective evaluation of qualitative and quantitative, 100 images are randomly selected to compare the running time of different algorithms in hazy images of 256 × 256 and 512 × 512 sizes and analyzing them by taking the average value. As shown in Table 5, the proposed algorithm is ranked No.3 among the six dehazing algorithms. Although its processing time is slightly longer than that of Dehazeformer, which is also a Transformer-based method, the proposed algorithm is still competitive in terms of comprehensive fog removal effect. Compared with MSBDN, which has a better dehazing effect, the proposed algorithm improves 0.226 and 0.918 s on two different sizes of images, which runs faster and is more suitable for dehazing onboard cockpit scenes.
Size | DCP | GCANet | FFANet | MSBDN | Dehazformer | PhysiFormer |
256 × 256 | 1.849 s | 0.072 s | 0.423 s | 0.324 s | 0.063 s | 0.098 s |
512 × 512 | 2.763 s | 0.167 s | 1.294 s | 1.148 s | 0.158 s | 0.235 s |
In order to address the impact of hazy environment on flight operations and improve the robustness and generalization ability of the dehazing algorithm in real airborne environments, a dehazing method combining Transformer and physical prior was proposed. Based on the semi-supervised training of synthesized and real fogged images, multiscale feature extraction was introduced and the global context fusion mechanism was also used, which leads to better restoration of the details and texture information of the images and improves the model's generalization ability. Extensive experimental evaluations on public datasets and real aerial video frames show that our method achieves good results in objective evaluation metrics and, on the subjective side, the dehazed image effectively improves color distortion and solves the problem of uneven processing of different fog concentrations, which can be generalized to real hazy images for flight safety. However, in the real airborne environment[40], the pilot must quickly complete the judgment of the flight mission system. The dehazing effect needs to be refreshed in real time, and the next step will be to study how to reduce the computation while guaranteeing the performance.
The authors declare they have not used Artificial Intelligence (AI) tools in the creation of this article.
This work was supported by the National Key Research and Development Program Topics (Grant No. 2021YFB4000905), and in part by Shaanxi Natural Science Fundamental Research Program Project (No. 2022JM-508).
The authors declare there is no conflict of interest.
[1] | S. K. Nayar, S. G. Narasimhan, Vision in bad weather, in Proceedings of the Seventh IEEE International Conference on Computer Vision, 2 (1999), 820–827. https://doi.org/10.1109/ICCV.1999.790306 |
[2] | K. He, J. Sun, X. Tang, Single image haze removal using dark channel prior, in 2009 IEEE Conference on Computer Vision and Pattern Recognition, (2009), 1956–1963. https://doi.org/10.1109/CVPR.2009.5206515 |
[3] |
Q. Zhu, J. Mai, L. Shao, A fast single image haze removal algorithm using color attenuation prior, IEEE Trans. Image Process., 24 (2015), 3522–3533. https://doi.org/10.1109/TIP.2015.2446191 doi: 10.1109/TIP.2015.2446191
![]() |
[4] | R. Fattal, Dehazing using color-lines, ACM Trans. Graphics, 34 (2014), 1–14. |
[5] | D. Berman, T. Treibitz, S. Avidan, Non-local image dehazing, in 2016 IEEE Conference on Computer Vision and Pattern Recognition (CVPR), 34 (2016), 1674–1682. https://doi.org/10.1109/CVPR.2016.185 |
[6] | H. Zhang, V. M. Patel, Densely connected pyramid dehazing network, in 2018 IEEE/CVF Con-ference on Computer Vision and Pattern Recognition, (2018), 3194–3203. https://doi.org/10.1109/CVPR.2018.00337 |
[7] | D. Chen, M. He, Q. Fan, J. Liao, L. Zhang, D. Hou, et al., Gated context aggregation network for image dehazing and deraining, in 2019 IEEE Winter Conference on Applications of Computer Vision (WACV), (2019), 1375–1383. https://doi.org/10.1109/WACV.2019.00151 |
[8] | X. Qin, Z. Wang, Y. Bai, X. Xie, H. Jia, FFA-Net: Feature fusion attention network for single image dehazing, in Proceedings of the AAAI Conference on Artificial Intelligence, 34 (2020), 11908–11915. |
[9] |
G. Gao, J. Cao, C. Bao, Q. Hao, A. Ma, A novel transformer-based attention network for image dehazing, Sensors, 22 (2022), 3428. https://doi.org/10.3390/s22093428 doi: 10.3390/s22093428
![]() |
[10] |
S. Li, Q. Yuan, Y. Zhang, B. Lv, F. Wei, Image dehazing algorithm based on deep learning coupled local and global features, Appl. Sci., 12 (2022), 8552. https://doi.org/10.3390/app12178552 doi: 10.3390/app12178552
![]() |
[11] |
Y. Song, Z. He, H. Qian, X. Du, Vision transformers for single image dehazing, IEEE Trans. Image Process., 32 (2023), 1927–1941. https://doi.org/10.1109/TIP.2023.3256763 doi: 10.1109/TIP.2023.3256763
![]() |
[12] | Z. Liu, Y. Lin, Y. Gao, H. Hu, Y. Wei, Z. Zhang, et al., Swin transformer: Hierarchical vision transformer using shifted windows, in 2021 IEEE/CVF International Conference on Computer Vision (ICCV), (2021), 9992–10002. https://doi.org/10.1109/ICCV48922.2021.00986 |
[13] | W. Huang, J. Li, C. Qi, A defogging algorithm for dense fog images via low-rank and dictionary expression decomposition, J. Xi'an Jiaotong Univ., 54 (2020), 118–125. |
[14] | T. Gao, M. Liu, T. Chen, S. Wang, S. Jiang, A far and near scene fusion defogging algorithm based on the prior of dark-light channel, J. Xi'an Jiaotong Univ., 55 (2021), 78–86. |
[15] | Y. Yang, X. Chen, An image dehazing method combining adaptive brightness transformation inequality to estimate transmittance, J. Xi'an Jiaotong Univ., 55 (2021), 69–76. |
[16] | H. Huang, K. Hu, J. Song, H. Huang, A twice optimization method for solving transmittance with haze-lines, J. Xi'an Jiaotong Univ., 55 (2021), 130–138. |
[17] |
T. Ma, C. Fu, J. Yang, J. Zhang, C. Yang, RF-Net: Unsupervised low-light image enhancement based on retinex and exposure fusion, Comput. Mater. Continua, 77 (2023), 1103–1122. https://doi.org/10.32604/cmc.2023.042416 doi: 10.32604/cmc.2023.042416
![]() |
[18] |
B. Cai, X. Xu, K. Jia, C. Qing, D. Tao, Dehazenet: An end-to-end system for single image haze removal, IEEE Trans. Image Process., 25 (2016), 5187–5198. https://doi.org/10.1109/TIP.2016.2598681 doi: 10.1109/TIP.2016.2598681
![]() |
[19] | B. Li, X. Peng, Z. Wang, J. Xu, D. Feng, AOD-Net: All-in-one dehazing network, in International Conference on Computer Vision (ICCV), (2017), 4780–4788. https://doi.org/10.1109/ICCV.2017.511 |
[20] | Y. Qu, Y. Chen, J. Huang, Y. Xie, Enhanced pix2pix dehazing network, in 2019 IEEE/CVF Conference on Computer Vision and Pattern Recognition (CVPR), (2019), 8182–8160. https://doi.org/10.1109/CVPR.2019.00835 |
[21] |
X. Zhu, S. Li, Y. Gan, Y. Zhang, B. Sun, Multi-stream fusion network with generalized smooth L1 loss for single image dehazing, IEEE Trans. Image Process., 30 (2021), 7620–7635. https://doi.org/10.1109/TIP.2021.3108022 doi: 10.1109/TIP.2021.3108022
![]() |
[22] | C. Long, X. Li, Y. Jing, H. Shen, Bishift networks for thick cloud removal with multitemporal remote sensing images, Int. J. Intell. Syst., 2023 (2023). https://doi.org/10.1155/2023/9953198 |
[23] |
W. Liu, X. Hou, J. Duan, G. Qiu, End-to-end single image fog removal using enhanced cycle consistent adversarial networks, IEEE Trans. Image Process., 29 (2020), 7819–7833. https://doi.org/10.1109/TIP.2020.3007844 doi: 10.1109/TIP.2020.3007844
![]() |
[24] | J. Dong, J. Pan, Physics-based feature dehazing networks, in Computer Vision–ECCV 2020: 16th European Conference, Glasgow, (2020), 188–204. https://doi.org/10.1007/978-3-030-58577-8_12 |
[25] | Q. Deng, Z. Huang, C. C. Tsai, C. W. Lin, Hardgan: A haze-aware representation distillation GAN for single image dehazing, in European Conference on Computer Vision, (2020), 722–738. |
[26] | H. Dong, J. Pan, L. Xiang, Z. Hu, X. Zhang, F. Wang, et al., Multi-scale boosted dehazing network with dense feature fusion, in 2020 IEEE/CVF Conference on Computer Vision and Pattern Recognition 14 CMES, (2020), 2154–2164. https://doi.org/10.1109/CVPR42600.2020.00223 |
[27] | X. Liu, Y. Ma, Z. Shi, J. Chen, Griddehazenet: Attention-based multi-scale network for image dehazing, in Proceedings of the IEEE/CVF International Conference on Computer Vision, (2019), 7314–7323. |
[28] | H. Wu, Y. Qu, S. Lin, J. Shou, R. Qiao, Z. Zhang, et al., Contrastive learning for compact single image dehazing, in 2021 IEEE/CVF Conference on Computer Vision and Pattern Recognition (CVPR), (2021), 10546–10555. https://doi.org/10.1109/CVPR46437.2021.01041 |
[29] |
C. Wang, H. Z. Shen, F. Fan, M. W. Shao, C. S, Yang, J. C. Luo, et al., EAA-Net: A novel edge assisted attention network for single image dehazing, Knowledge-Based Syst., 228 (2021), 107279. https://doi.org/10.1016/j.knosys.2021.107279 doi: 10.1016/j.knosys.2021.107279
![]() |
[30] | A. Vaswani, N. Shazeer, N. Parmar, J. Uszkoreit, L. Jones, A. N. Gomez, et al., Attention is all you need, in Advances in Neural Information Processing Systems, 30 (2017). |
[31] | A. Dosovitskiy, A. Kolesnikov, D. Weissenborn, X. Zhai, T. Unterthiner, M. Dehghani, et al., An image is worth 16x16 words: Transformers for image recognition at scale, preprint, arXiv: 2010.11929. |
[32] |
T. Ma, J. An, R. Xi, J. Yang, J. Lyu, F. Li, TPE: Lightweight transformer photo enhancement based on curve adjustment, IEEE Access, 10 (2022), 74425–74435. https://doi.org/10.1109/ACCESS.2022.3191416 doi: 10.1109/ACCESS.2022.3191416
![]() |
[33] | L. Yuan, Y. Chen, T. Wang, W. Yu, Y. Shi, Z. H. Jiang, et al., Tokens-to-token vit: Training vision trans-formers from scratch on imagenet, in Proceedings of the IEEE/CVF International Conference on Computer Vision, (2021), 558–567. |
[34] | S. W. Zamir, A. Arora, S. Khan, M. Hayat, F. S. Khan, M. Yang, Restormer: Efficient transformer for high-resolution image restoration, in 2022 IEEE/CVF Conference on Computer Vision and Pattern Recognition (CVPR), (2022), 5718–5729. https://doi.org/10.1109/CVPR52688.2022.00564 |
[35] | Y. Qiu, K. Zhang, C. Wang, W. Luo, H. Li, Z. Jin, MB-TaylorFormer: Multi-branch efficient transformer expanded by Taylor formula for image dehazing, in Proceedings of the IEEE/CVF International Conference on Computer Vision, (2023), 12802–12813. |
[36] |
B. Li, W. Ren, D. Fu, D. Tao, D. Feng, W. Zeng, et al., Benchmarking single-image dehazing and beyond, IEEE Trans. Image Process., 28 (2019), 492–505. https://doi.org/10.1109/TIP.2018.2867951 doi: 10.1109/TIP.2018.2867951
![]() |
[37] | C. O. Ancuti, C. Ancuti, R. Timofte, NH-HAZE: An image dehazing benchmark with nonhomogeneous hazy and haze-free images, in Proceedings of the IEEE/CVF Conference on Computer Vision and Pattern Recognition Workshops, (2020), 444–445. |
[38] | H. Dong, J. Pan, L. Xiang, Z. Hu, X. Zhang, F. Wang, et al., Multi-scale boosted dehazing network with dense feature fusion, in 2020 IEEE/CVF Conference on Computer Vision and Pattern Recognition(CVPR), (2020), 2154–2164. https://doi.org/10.1109/CVPR42600.2020.00223 |
[39] | H. B. Ji, X. Feng, W. J. Pei, J. X. Li, G. M. Lu, U2-Former: A Nested U-shaped transformer for image restoration, preprint, arXiv: 2112.02279. |
[40] | Z. Yu, Z. Wang, J. Yu, D. Liu, H. Song, Z. Li, Cybersecurity of unmanned aerial vehicles: A survey, IEEE Aerosp. Electron. Syst. Mag., 2023 (2023). https://doi.org/10.1109/MAES.2023.3318226 |
1. | Hong'an Li, Man Liu, Jiangwen Fan, Qingfang Liu, Biomedical image segmentation algorithm based on dense atrous convolution, 2024, 21, 1551-0018, 4351, 10.3934/mbe.2024192 |
PSNR/SSIM | SOTS-outdoor | NH-HAZE |
DCP | 19.19/0.834 | 11.19/0.514 |
GCANet | 29.14/0.947 | 16.34/0.626 |
FFANet | 32.75/0.969 | 18.95/0.693 |
MSBDN | 34.68/0.975 | 19.97/0.705 |
U2-former | 31.10/0.976 | - - - |
Dehazeformer | 35.15/0.987 | 19.56/0.694 |
MB-TaylorFormer | 35.21/0.981 | 20.01/0.706 |
PhysiFormer | 35.37/0.983 | 20.37/0.716 |
NIQE/BRISQUE | RTTS | Aerial image |
DCP | 3.579/34.858 | 3.465/33.476 |
GCANet | 3.523/28.576 | 3.584/30.239 |
FFANet | 3.456/36.702 | 3.843/36.468 |
MSBDN | 3.142/26.397 | 3.114/27.397 |
Dehazeformer | 3.294/27.895 | 3.164/27.793 |
MB-TaylorFormer | 3.102/25.967 | 3.106/27.183 |
PhysiFormer | 2.906/24.951 | 3.017/26.419 |
Model No. | PPM | GCBFusion | Physical loss | PSNR | SSIM |
M1 | 18.75 | 0.601 | |||
M2 | 19.34 | 0.687 | |||
M3 | 19.56 | 0.694 | |||
M4 | 20.37 | 0.716 |
Model No. | PPM | GCBFusion | Physical loss | NIQE | BRISQUE |
M1 | 3.267 | 27.240 | |||
M2 | 3.156 | 26.431 | |||
M3 | 3.184 | 26.675 | |||
M4 | 2.906 | 24.951 |
Size | DCP | GCANet | FFANet | MSBDN | Dehazformer | PhysiFormer |
256 × 256 | 1.849 s | 0.072 s | 0.423 s | 0.324 s | 0.063 s | 0.098 s |
512 × 512 | 2.763 s | 0.167 s | 1.294 s | 1.148 s | 0.158 s | 0.235 s |
PSNR/SSIM | SOTS-outdoor | NH-HAZE |
DCP | 19.19/0.834 | 11.19/0.514 |
GCANet | 29.14/0.947 | 16.34/0.626 |
FFANet | 32.75/0.969 | 18.95/0.693 |
MSBDN | 34.68/0.975 | 19.97/0.705 |
U2-former | 31.10/0.976 | - - - |
Dehazeformer | 35.15/0.987 | 19.56/0.694 |
MB-TaylorFormer | 35.21/0.981 | 20.01/0.706 |
PhysiFormer | 35.37/0.983 | 20.37/0.716 |
NIQE/BRISQUE | RTTS | Aerial image |
DCP | 3.579/34.858 | 3.465/33.476 |
GCANet | 3.523/28.576 | 3.584/30.239 |
FFANet | 3.456/36.702 | 3.843/36.468 |
MSBDN | 3.142/26.397 | 3.114/27.397 |
Dehazeformer | 3.294/27.895 | 3.164/27.793 |
MB-TaylorFormer | 3.102/25.967 | 3.106/27.183 |
PhysiFormer | 2.906/24.951 | 3.017/26.419 |
Model No. | PPM | GCBFusion | Physical loss | PSNR | SSIM |
M1 | 18.75 | 0.601 | |||
M2 | 19.34 | 0.687 | |||
M3 | 19.56 | 0.694 | |||
M4 | 20.37 | 0.716 |
Model No. | PPM | GCBFusion | Physical loss | NIQE | BRISQUE |
M1 | 3.267 | 27.240 | |||
M2 | 3.156 | 26.431 | |||
M3 | 3.184 | 26.675 | |||
M4 | 2.906 | 24.951 |
Size | DCP | GCANet | FFANet | MSBDN | Dehazformer | PhysiFormer |
256 × 256 | 1.849 s | 0.072 s | 0.423 s | 0.324 s | 0.063 s | 0.098 s |
512 × 512 | 2.763 s | 0.167 s | 1.294 s | 1.148 s | 0.158 s | 0.235 s |