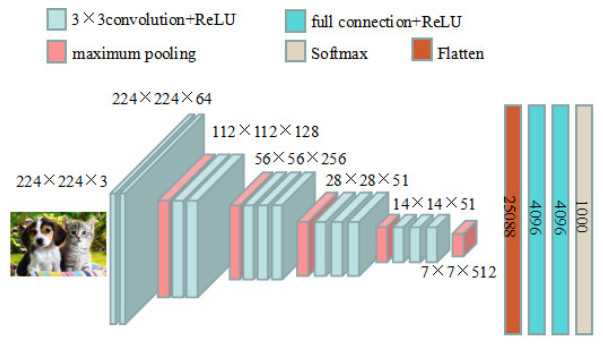
Breast cancer is the cancer with the highest incidence in women worldwide, and seriously threatens the lives and health of women. Mammography, which is commonly used for screening, is considered to be the most effective means of diagnosing breast cancer. Currently, computer-assisted breast mass systems based on mammography can help doctors improve film reading efficiency, but improving the accuracy of assisted diagnostic systems and reducing the false positive rate are still challenging tasks. In the image classification field, convolutional neural networks have obvious advantages over other classification algorithms. Aiming at the very small percentage of breast lesion area in breast X-ray images, in this paper, the classical VGG16 network model is improved by simplifying the network structure, optimizing the convolution form and introducing an attention mechanism. The improved model achieves 99.8 and 98.05% accuracy on the Mammographic Image Analysis Society (MIAS) and The Digital Database for Screening Mammography (DDSM), respectively, which is obviously superior to some methods of recent studies.
Citation: Yi Dong, Jinjiang Liu, Yihua Lan. A classification method for breast images based on an improved VGG16 network model[J]. Electronic Research Archive, 2023, 31(4): 2358-2373. doi: 10.3934/era.2023120
[1] | Zengyu Cai, Liusen Xu, Jianwei Zhang, Yuan Feng, Liang Zhu, Fangmei Liu . ViT-DualAtt: An efficient pornographic image classification method based on Vision Transformer with dual attention. Electronic Research Archive, 2024, 32(12): 6698-6716. doi: 10.3934/era.2024313 |
[2] | Jiange Liu, Yu Chen, Xin Dai, Li Cao, Qingwu Li . MFCEN: A lightweight multi-scale feature cooperative enhancement network for single-image super-resolution. Electronic Research Archive, 2024, 32(10): 5783-5803. doi: 10.3934/era.2024267 |
[3] | Ahmed Abul Hasanaath, Abdul Sami Mohammed, Ghazanfar Latif, Sherif E. Abdelhamid, Jaafar Alghazo, Ahmed Abul Hussain . Acute lymphoblastic leukemia detection using ensemble features from multiple deep CNN models. Electronic Research Archive, 2024, 32(4): 2407-2423. doi: 10.3934/era.2024110 |
[4] | Huimin Qu, Haiyan Xie, Qianying Wang . Multi-convolutional neural network brain image denoising study based on feature distillation learning and dense residual attention. Electronic Research Archive, 2025, 33(3): 1231-1266. doi: 10.3934/era.2025055 |
[5] | Haijun Wang, Wenli Zheng, Yaowei Wang, Tengfei Yang, Kaibing Zhang, Youlin Shang . Single hyperspectral image super-resolution using a progressive upsampling deep prior network. Electronic Research Archive, 2024, 32(7): 4517-4542. doi: 10.3934/era.2024205 |
[6] | Chetan Swarup, Kamred Udham Singh, Ankit Kumar, Saroj Kumar Pandey, Neeraj varshney, Teekam Singh . Brain tumor detection using CNN, AlexNet & GoogLeNet ensembling learning approaches. Electronic Research Archive, 2023, 31(5): 2900-2924. doi: 10.3934/era.2023146 |
[7] | Jinjiang Liu, Yuqin Li, Wentao Li, Zhenshuang Li, Yihua Lan . Multiscale lung nodule segmentation based on 3D coordinate attention and edge enhancement. Electronic Research Archive, 2024, 32(5): 3016-3037. doi: 10.3934/era.2024138 |
[8] | Mashael S. Maashi, Yasser Ali Reyad Ali, Abdelwahed Motwakel, Amira Sayed A. Aziz, Manar Ahmed Hamza, Amgad Atta Abdelmageed . Anas platyrhynchos optimizer with deep transfer learning-based gastric cancer classification on endoscopic images. Electronic Research Archive, 2023, 31(6): 3200-3217. doi: 10.3934/era.2023162 |
[9] | Chengyong Yang, Jie Wang, Shiwei Wei, Xiukang Yu . A feature fusion-based attention graph convolutional network for 3D classification and segmentation. Electronic Research Archive, 2023, 31(12): 7365-7384. doi: 10.3934/era.2023373 |
[10] | Kuntha Pin, Jung Woo Han, Yunyoung Nam . Retinal diseases classification based on hybrid ensemble deep learning and optical coherence tomography images. Electronic Research Archive, 2023, 31(8): 4843-4861. doi: 10.3934/era.2023248 |
Breast cancer is the cancer with the highest incidence in women worldwide, and seriously threatens the lives and health of women. Mammography, which is commonly used for screening, is considered to be the most effective means of diagnosing breast cancer. Currently, computer-assisted breast mass systems based on mammography can help doctors improve film reading efficiency, but improving the accuracy of assisted diagnostic systems and reducing the false positive rate are still challenging tasks. In the image classification field, convolutional neural networks have obvious advantages over other classification algorithms. Aiming at the very small percentage of breast lesion area in breast X-ray images, in this paper, the classical VGG16 network model is improved by simplifying the network structure, optimizing the convolution form and introducing an attention mechanism. The improved model achieves 99.8 and 98.05% accuracy on the Mammographic Image Analysis Society (MIAS) and The Digital Database for Screening Mammography (DDSM), respectively, which is obviously superior to some methods of recent studies.
Breast cancer is a high-incidence malignant tumor, and is one of the most common malignant tumors that seriously affects women's physical and mental health, and endangers their lives. Evidence shows that early detection, early diagnosis and early treatment are key to preventing worsening in breast cancer patients. Current breast cancer screening techniques include body checks, ultrasound, X-rays and magnetic resonance imaging. X-ray photography can detect clustered microcalcifications and masses in the breast. X-rays are the most effective and important diagnostic tools for identifying and screening cancerous tissue. However, reading X-ray photography requires doctors to maintain extremely high concentration and attention, and the number of breast images to be read in clinical practice is too large, which can easily cause visual fatigue, leading to subjectivity in breast image diagnosis and not guaranteeing early breast cancer diagnosis accuracy. With mammography digitization, establishing medical image-based diagnostic aids with the help of computer image processing, mathematical statistics and artificial intelligence technology has become a hotspot in breast cancer research.
In the traditional computer-aided diagnosis of mammogram classification, it is usually necessary to preprocess the data set first, then design and extract the features, and, finally, select the classifier for classification. By summarizing the studies of the past 20 years, J. Tang found that CAD systems have a mixed role in practice. They believed that the features of the mass might be masked, or similar to, the features of normal breast parenchyma, so more consideration should be given to the features of breast images [1]. Liu used LDA+k-NN classification and SVM with a Gaussian kernel to classify the DDSM data set, and obtained an accuracy of 96.15% [2]. Mohanty proposed an FS algorithm called forest optimization to classify normal and abnormal mammogram lesions using different classifiers, such as SVM, k-nearest neighbor (k-NN), naive Bayesian and C4.5. The highest accuracy achieved by the C4.5 classifier using the DDSM data set was 99.08%; using the MIAS data set, the accuracy of the naive Bayesian classifier reached 97.86% [3]. Dina A et al. used Adaboosted and bagged the k-NN, J48 decision tree (DT), random forest (RF) DT and random tree (RT) DT classifiers to integrate and develop multiple classifier systems (MCS), which are significantly superior to a single classifier [4]. Although the method based on traditional machine learning has made some achievements in the recognition of breast images, it needs to manually extract the features of the images, and the effectiveness of the extracted features will directly affect the recognition results. In addition, mammograms are single-channel grayscale images with low contrast. Manual feature extraction requires profound medical background and rich clinical experience, which increases the difficulty in feature extraction and recognition of masses.
In recent years, deep learning has made great progress in computer vision, pattern recognition and other application fields and has become one of the popular topics of current research. Unlike traditional machine learning, deep learning can automatically extract image features and integrate feature extraction and classification. With the development of computer hardware and the improvement in computing power, deep learning has shown performance close to human beings, among which the most remarkable achievement is the use of convolutional neural networks (CNNs).
In 2012, AlexNet won first place in image classification with a 15.32% error rate on the imageNet2012 image classification task, which also marked the explosion and rise in deep learning scholarly research [5]. Subsequently, an increasing number of scholars have also applied models, such as VGGNet, ResNet, MobileNetV2 and DenseNet in deep learning to medical image processing [6,7,8,9]. At present, there are three methods for deep learning exploration and research. First, the structure of the convolutional neural network is improved. Second, the network input is improved. Third, the training methods are improved.
An increasing number of convolutional neural network models are emerging, among which, in 2021, Tsinghua University, Kuangshi Technology and Hong Kong University of Science and Technology jointly proposed the RepVGG network, which is improved based on the VGG network by adding identity and residual branches to the VGG network block. It is equivalent to applying the essence of the ResNet network to the VGG network and applying this block during training. When reasoning, it is transformed into a single-path VGG model. The highlight of this model is the use of different network architectures for network training and reasoning, which focuses the training more on accuracy, and the reasoning more on speed [10]. The ParNet network also proves that shallow networks can perform very well, as long as they have parallel substructures [11]. In 2022, ConvNet was benchmarked against the Swin transformer, which was very popular in the computer vision field in 2021 [12]. Through a series of transformation experiments, a standard ResNet model gradually changed into a ConvNet series model. It achieved over 87.8% accuracy on ImageNet-1K.
In the breast image classification field, Ansi Pan et al. simplified the VGG16 model and proposed the SVGG16 model [13]. In Man-man Hu's paper, the improved AlexNet model simplified the AlexNet model and then added a SENet attention module. The improved ResNet18 model proposed was obtained by modifying the residual module after optimizing the ResNet18 model, and both models achieved good accuracy in the MIAS data set of mammography images [14]. The DenseNet121 transfer learning model proposed by Yuhang Yang et al. is based on the DenseNet121 model with the addition of an attention module, and has the best effect on the BreaKHis data set of breast case images [15]. The improved MobileNetV2 model proposed by Mingzhu Meng et al. improved the fully connected layer in the MobileNetV2 model, and achieved 98% accuracy in mammogram images collected by local hospitals [16].
Although deep learning has made some achievements in mammography classification, there are still many problems:
● Mammography data sets are very limited. An effective method that can use limited data sets to design the neural network model so that the data can play a maximum role is still lacking.
● In mammogram images, the proportion of breast lesions is very small, and the general neural network model accuracy is very low in identifying such a small target. Using traditional classification algorithms, feature extraction is difficult due to the feature similarity between mammogram images and breast density factors. However, most of the existing convolutional neural networks are dedicated to improving the existing models, using large convolutional cores and many layers of the network. Although the prediction accuracy is improved to some extent, such improvement may easily lead to a longer training time of the model or too many parameters, which greatly increases the time and computing power of the operation, greatly reduces the efficiency of the prediction, and may also cause overfitting.
Based on the above problems, the main contributions of this study are as follows:
1) In view of the insufficient number of training samples of mammography, this paper explores the use of an extreme method of data enhancement, trying to expand and enhance the small, input sample mammography images by means of image denoising and image rotation, to improve the training effect of the model and effectively alleviate the overfitting phenomenon caused by insufficient data.
2) This paper will focus on discussing how to adopt a more simplified model to minimize the redundancy of parameters, and, at the same time, more effectively and accurately classify whether there are breast lesions and whether the breast lesions are benign or malignant, comprehensively and accurately screen out malignant lesions, and improve the efficiency of prediction without reducing the accuracy of prediction to be better applied in practical applications.
VGGNet is a deep convolution network structure proposed by the Visual Geometry group of Oxford University. They won second place in the 2014 ILSVRC classification task with a 7.32% error rate and first place in the localization task with a 25.32% error rate. VGG can be seen as a deepened version of AlexNet. VGGNet uses a convolution kernel size of 3 × 3 and a maximum pooling size of 2 × 2 for the entire network. The commonly used VGG16 refers to the total number of convolution kernels and fully connected layers being 16, excluding the maximum pooled layers. The structure of the original VGG16 model is shown in Figure 1, which includes five convolution layer modules, two full connection layers and one output layer. Each convolution layer module contains two or three convolution layers and one maximum pooling layer.
Ioffe and Szegedy proposed batch normalization for the first time [17]. Batch normalization was proposed to address increasingly deep neural networks. The authors normalized the input values between layers. The probability distribution of the output results of the previous layer was transformed into the standard normal distribution. After the standard normal transformation, the data before the input activation function fall into the sensitive area of the activation function. In this way, gradient disappearance can be avoided in the backpropagation process. The "batch" is because the normalization operation is performed on the small batch training data. Batch normalization is a method that greatly improves the training effect, and it allows for less careful parameter initialization. It carries out batch normalization processing for the hidden layer output data at all levels in the training process. Through batch normalization analysis and processing, the change in the data distribution of the hidden layer in the training can be reduced, thus reducing the impact on the neural network parameters, improving the convergence speed, and enhancing the stability. Batch normalization needs to be solved after the convolution layer and before the activation function.
Depthwise separable convolution, which consists of two parts, depthwise convolution and pointwise convolution, is used in lightweight networks such as MobileNet. Its advantages over traditional convolution are the relatively low numbers of parameters and operations.
If a 64 × 64 RGB image is input, the final output is 4 feature maps of equal size.
The general convolution is shown in Figure 2:
The convolution layer has four filters, each filter has three convolution kernels, and the size of the convolution kernels is 3 × 3. Then, the number of convolution parameters shown in the figure above is 3 × 3 × 3 × 4 = 108 parameters.
For the same RGB image of size 64 × 64, unlike before, the depthwise convolution is a 2D convolution (shown in Figure 3), with the same number of filters and input channels; therefore, the number of output feature maps is equal to the number of input channels.
The convolution layer has 3 filters, and each filter has a kernel, so the number of parameters is 3 × 3 × 3 = 27.
Depthwise convolution only extracts the features on each channel but does not establish the depth connection between pixels, so we need to use pointwise convolution to establish this connection. Pointwise convolution (shown in Figure 4) establishes the connection of each pixel point in depth.
The pointwise convolution layer has 4 filters, where each filter has three convolution kernels, and the size of the convolution kernels is 1 × 1. Therefore, the number of parameters of the pointwise convolution layer is 1 × 1 × 3 × 4 = 12, and the number of parameters required by the depthwise separable convolution is 27 + 12 = 39.
ECANet (shown in Figure 5) is a form of implementation of the channel attention mechanism that uses a 1 × 1 convolution layer after the global average pooling layer, removing the fully connected layer [14]. The module avoids dimensional reduction, and effectively captures cross-channel interactions.
ECANet can achieve good results with only a few involved parameters. ECANet accomplishes cross-channel information interaction by one-dimensional convolution, and the size of the convolution kernel is adapted by a function so that layers with a large number of channels can have more cross-channel interaction. The adaptive function is shown in Eq (1):
K=|logC2γ+bγ|,γ=2,b=1 | (1) |
Steps:
• The input feature graph is transformed from a matrix of [h, w, c] to a vector of [1, 1, c] by global average pooling.
• The adaptive one-dimensional convolution kernel size kernel_size is calculated.
• The kernel_size is used in the one-dimensional convolution to obtain the weights for each channel of the feature map.
• The normalized weight and the original input feature map are multiplied channel by channel to generate the weighted feature map.
In the CNN model, a large number of convolution layers are superimposed to optimize the extracted features. Increasing the number of convolution layers can improve the recognition ability of the model and help improve the optimization effect. However, adding more convolution layers requires more training data and higher computational power, which significantly increases the training complexity. The depth of the network should be proportional to the size of the data set. Multiple convolution-checking input images are used for the convolution operation. Although low-level and high-level image features can be extracted, many features may be redundant, resulting in underfitting or overfitting problems in training.
Based on the above problems, Ansi Pan et al. simplified the VGG16 model and proposed the SVGG16 model (the structure of the model is shown in Figure 6) and obtained an accuracy of 90.34% in the region of interest (ROI) of 125 × 125 DDSM. In the convolution layer of the SVGG16 model, the number of convolution kernels is set as 32, 64,128,256 and 512 (the number of convolution kernels in the VGG16 convolution layer module is 64,128,256,512 and 512). The SVGG16 model retains only one fully connected layer, the number of neurons is set to 1024, and dropout with a deactivation rate of 0.5 is added. In the input layer of the SVGG16 model, the input is a 125 × 125 single-channel ROI image, and the number of neurons in the output layer is 2, which represents the probability of the input image containing and not containing lumps. If the probability of containing a lump is greater than the probability of not containing a lump, the image is considered to contain a lump; otherwise, the image is considered to contain no lump. In the convolution layer module, the size of the convolution kernel is 3 × 3 pixels, and the step size and padding are both 1. ReLU is used as the activation function of the convolution layer and the fully connected layer. Compared with the original VGG16 model, the SVGG16 model uses fewer convolution layers and fewer convolution kernels.
The SVGG16 model largely solves the overfitting problem of the VGG16 model for mammogram images. However, this model is only suitable for low-resolution ROI images, and can only deal with the binary lump recognition problem.
In this paper, we further optimize the SVGG16 model by making the following improvements:
• During neural network training, the change in the weight and bias of the previous layer will impact the input distribution of the latter layer, gradually mapping to the nonlinear function and approaching the limit saturation area of the value interval, which makes it increasingly difficult to train the neural network. Therefore, to improve the convergence speed of the model and reduce the internal covariate bias, the back of each convolution in the model is unified for batch normalization (BN), and the ReLU activation function is added to the back as the connection between the convolution layer and the maximum pooling layer.
• With the gradual deepening of the convolution network, an increasing number of parameters will be generated, which will lead to a reduction in the running speed and computational power of the model and affect the prediction performance of the model. Therefore, in this paper, the ordinary convolution kernel in the first 512 is replaced by a depthwise separable convolution, which does not change the prediction effect of the model but can also improve the problem of computational power decline caused by the large number of channels and parameters in the late stage of ordinary convolution.
• The channel attention mechanism has been shown to have great potential in improving the performance of deep convolution neural networks. However, most of the existing approaches focus on developing more complex attention modules to achieve better performance, which inevitably increases the model complexity. Hu improved the predictive power of the model by adding SENet to the AlexNet network; however, the SENet module contained dimension reduction operations, which led to the loss of some information. Therefore, to overcome the contradiction between performance and complexity, in this paper, ECANet is added between the convolution layer and the fully connected layer. The module adopts a dimensional reduction local cross-channel interaction strategy, which can be effectively realized by one-dimensional convolution. Additionally, the module only adds a small number of parameters, but it can obtain significant performance gains. It can focus the network on the place where it needs to focus more, effectively avoid dimension reduction and capture cross-channel interaction.
The structure of the improved model is shown in Figure 7.
There are two main data sets used in this paper: Mammographic Image Analysis Society (MIAS) and The Digital Database for Screening Mammography (DDSM).
The MIAS provides a data annotation file that lists detailed data information, including the number, background organizational characteristics, anomaly category, severity of the anomaly, center coordinates of the anomaly location and center radius of the anomaly location. There are a total of 322 images in the MIAS, among which 207 are normal images without lesions and 115 are abnormal images. The resolution of the decompressed MIAS data set is approximately 4000 × 2000. Among the 115 abnormal images, 64 were benign lesions and 51 were malignant lesions.
The DDSM contains 2620 instances, each of which has a view of the patient's left and right breasts in two different directions. The instances also recorded information about the lesions identified by the physician, such as the type of lesion (the location of microcalcifications and benign or malignant lumps), lesion characteristics, and lesion contour information drawn by the physician. The resolution size of the DDSM data set after decompression is approximately 2400 × 4000. A total of 2390 CC images (including 934 malignant cases, 872 benign cases and 584 normal cases) were selected from the DDSM data set for study.
To further study the characteristics of breast masses, under the premise of not affecting the image processing effect, in this paper, the decompressed DDSM data image was sampled, reducing the computational complexity of image processing. Sampling is carried out in the following ways: the extracted image data are subsampled at a size of 8×8 pixels, and the original pixel size of 50 × 50 is increased to 400 × 400. The gray level of the original image is reduced from 12 to 8, that is, from 4096 to 256 gray level. The sampled images are the source data for the ROI data images used in this article. The ROI image resolution was 125 × 125, and a total of 3179 images were selected (including 2338 normal cases and 841 lump cases).
In this paper, a computer-aided design system based on BI-RADS was adopted to classify all original mammogram data into three categories (normal, benign and malignant lesions) for the MIAS data set. For the DDSM data set, due to limited computing power, the resolution of the whole original mammogram was compressed to one-quarter of the original mammogram, and then the data were classified into three categories (normal, benign and malignant lesions). For ROI pictures in DDSM, two classification methods (normal and lump) were used.
In the mammography process, there will be some noise due to photoelectronic noise and other factors, which will affect image recognition accuracy. Therefore, it is necessary to preprocess the data set before sending it into the neural network. Mammogram images have more noise because of the special characteristics of the breast tissue. In this paper, the median filter is adopted to denoise the image. Median filtering is a kind of nonlinear filtering image denoising method that can suppress noise. First, a 5 × 5 template is selected, the template is sequentially moved on the pixels of the original image, the gray level of pixels covered by the template are sorted according to their size, and the output pixel at this position is the median gray value covered in the region. The feature of median filtering is that it can eliminate the isolated noise and retain the information of the image and edge sharpness, so that each part of the image is clearer.
Similar to other deep learning methods, data enhancement cannot only effectively alleviate the overfitting phenomenon caused by insufficient data, but also effectively improve the training effect of the model. Based on the insufficient number of mammography training samples and the position of the breast in the picture being relatively fixed, the data enhancement strategy adopted in this paper is to first find the center point of the original image, then rotate it at certain intervals in the counterclockwise direction to obtain a number of enhanced data, and then uniformly scale it to 224 × 224, which not only expands the data, but also allows us to obtain a multidirectional image of the breast position. For the MIAS data, because of the relatively small quantity of data, an omni-directional rotational data enhancement method was adopted. We chose to rotate every degree, and 360 rotations were performed to obtain the enhanced data at 360 times the original data. The CC plots used in the DDSM database were chosen to be rotated every 7.5 degrees, and 48 rotations were performed to obtain enhanced data that were 48 times larger than the original data. The ROI map used in the DDSM database was chosen to be rotated every 5 degrees, and 72 rotations were performed to obtain enhanced data that were 72 times larger than the original data.
In evaluating the classification model performance, we used accuracy, precision and recall to assess the ability of the model to classify and recognize images.
If the number of samples is N, the samples are categorized into positive and negative samples, then TP refers to the number of positive samples correctly predicted into positive categories, FP refers to the number of negative samples incorrectly predicted into positive categories (FP in statistical hypothesis testing are type Ⅰ error), TN refers to the number of negative samples correctly predicted into negative categories, and FN refers to the number of negative samples correctly predicted into negative categories (FN in statistical hypothesis testing are type Ⅱ error).
The accuracy rate examines the degree to which the classification of the classifier is correct. It requires that the result label predicted by the given data in the data set should correspond to the training label one by one. The expression in words is shown in Eq (2):
Accuracy=TP+TNN | (2) |
Precision measures the ratio of the number of true positive values predicted by the model function to the number of all true values. The text expression is shown in Eq (3):
Precision=TPTP+FP | (3) |
Recall measures the ability of a model function's class to predict correctly. The text expression is shown in Eq (4):
Recall=TPTP+FN | (4) |
The experiments in this paper used the improved VGG16 network model to classify the mammogram images of normal breasts, benign lesions and malignant lesions. In the experiment, MIAS was used to improve the model and train the parameters, and then CC image data of DDSM and ROI were used to further verify the model effect.
An RTX 3090 with 25.4 GB of video memory and 60.9 GB of memory was used in this experiment. The network model was built using the TensorFlow2.6.0 and Keras2.6.0 frameworks. In the experiment, the initial learning rate was set to 0.001, 20% of the data set was used for testing, and 80% was used to train the network model. The Adam optimizer was used to uniformly train 10 epochs.
In this paper, the abovementioned improved AlexNet model, improved ResNet18 model, SVGG16 model, RepVGG model, ResNet18 model, improved MobileNetV2 model, DenseNet121 transfer learning model, GoogLeNet and the improved VGG16 were trained simultaneously on the MIAS data set model to obtain the respective loss rate, accuracy and recall [1,2,3,4,5,6]. The specific results are shown in Figure 8, and Tables 1 and 2.
Model | Parameter | Times (s) | Loss | Accuracy | Precision | Recall |
Improved AlexNet | 220,179 | 2560 | 0.0457 | 0.9847 | 0.9851 | 0.9844 |
SVGG16 | 8,644,355 | 1154 | 0.0266 | 0.9926 | 0.9927 | 0.9926 |
RepVGG | 7,854,499 | 2369 | 0.0122 | 0.9962 | 0.9963 | 0.9962 |
ResNet18 | 11,186,179 | 1834 | 0.0165 | 0.9944 | 0.9944 | 0.9944 |
Improved ResNet18 | 1,065,091 | 2994 | 0.1111 | 0.9646 | 0.9678 | 0.9606 |
Improved MobileNetV2 | 4,107,551 | 4745 | 0.0408 | 0.9885 | 0.9893 | 0.9878 |
DenseNet121 | 7,034,307 | 5306 | 0.0138 | 0.9954 | 0.9955 | 0.9953 |
GoogLeNet | 6,751,435 | 2349 | 0.0097 | 0.9972 | 0.9972 | 0.9972 |
Improved VGG16 | 8,666,824 | 1478 | 0.0061 | 0.9980 | 0.9980 | 0.9980 |
Model | Loss | Accuracy | Precision | Recall |
Improved AlexNet | 0.0307 | 0.9887 | 0.9889 | 0.9886 |
SVGG16 | 0.0210 | 0.9944 | 0.9944 | 0.9943 |
RepVGG | 3.8456 | 0.6353 | 0.6355 | 0.6347 |
ResNet18 | 1.7089 | 0.7536 | 0.7542 | 0.7533 |
Improved ResNet18 | 7.3758 | 0.6587 | 0.6589 | 0.6586 |
Improved MobileNetV2 | 0.2280 | 0.9340 | 0.9368 | 0.9315 |
DenseNet121 | 0.0226 | 0.9907 | 0.9909 | 0.9906 |
GoogLeNet | 0.0288 | 0.9918 | 0.9923 | 0.9914 |
Improved VGG16 | 0.0001 | 1.0000 | 1.0000 | 1.0000 |
Overall, in the training stage, GoogLeNet has the fastest convergence rate in loss, accuracy, precision and recall, while the improved VGG16 converges only second to GoogLeNet (Figure 8). The improved VGG16 obtained significantly better results than the other models, and in the testing phase, the accuracy, precision and recall already reached 100% (Table 1), and the total training time was longer than that of SVGG16.
Compared with other models, it is easy to find, from the training stage, (Table 1): compared with SVGG16, the improved VGG16 model has slightly increased total model parameters and total training time, but from the results obtained, compared with other models with fewer parameters, this improvement is worthwhile. Among the other models, RepVGG and GoogLeNet also achieved relatively high results, and the total training time and total model parameters were only slightly higher than those of the improved VGG16 model. Although the DenseNet121 transfer learning model and improved MobileNetV2 can achieve relatively good results, the total training time is long, and the computational efficiency is greatly reduced. ResNet18 was able to achieve some results, although, with the largest parameters of the model, it is seen that the residual structure in the model adds a certain amount of computation and makes the network structure relatively more complex. Although the improved AlexNet and the improved ResNet18 have fewer model parameters, the total training time is also not optimal, and the results obtained are relatively poorer.
In the test phase (Table 2), RepVGG, ResNet18 and improved ResNet18 performed poorly, indicating that for the MIAS data set, the convolutional network of the model was deeper and the model structure was more complex, which still led to overfitting.
Through the comparison of several models, it is clear that SVGG16, GoogLeNet and the improved VGG16 perform well in all aspects. Compared with the three models, SVGG16 has the worst training results, but its prediction ability is slightly better than that of GoogLeNet. GoogLeNet takes the longest time, but it can converge the fastest during model training. The improved VGG16 model has the most parameters, but the training and testing results of the model are the best, and the model parameters do not make the total training time excessively long, which is still a good method.
To verify the generalization ability of the improved VGG16 model, this paper continued further verification on the DDSM and its ROI data set, and the results are shown in Tables 3–6.
Model | Loss | Accuracy | Precision | Recall |
Improved VGG16 | 0.0176 | 0.9939 | 0.9885 | 0.9884 |
SVGG16 | 0.2117 | 0.9099 | 0.8601 | 0.7862 |
Model | Loss | Accuracy | Precision | Recall |
Improved VGG16 | 0.3150 | 0.9188 | 0.7975 | 0.9337 |
SVGG16 | 0.2209 | 0.9070 | 0.8483 | 0.7950 |
Model | Loss | Accuracy | Precision | Recall |
Improved VGG16 | 0.0558 | 0.9805 | 0.9809 | 0.9802 |
SVGG16 | 0.1451 | 0.9461 | 0.9477 | 0.9446 |
Model | Loss | Accuracy | Precision | Recall |
Improved VGG16 | 0.2604 | 0.9186 | 0.9194 | 0.9179 |
SVGG16 | 0.2912 | 0.8944 | 0.8972 | 0.8923 |
The above results clearly show that the improved VGG16 model can maintain an obvious lead in training, and achieve relatively high prediction results in testing, regardless of the 125 × 125 ROI data set or the DDSM data set of 224 × 224. The effectiveness of the improved VGG16 model is further proven.
In summary, the improved VGG16 model is indeed relatively simple, and can achieve a certain balance between prediction accuracy and prediction efficiency, which has certain positive significance in the classification of mammogram images.
In this paper, an improved VGG16 model is proposed by introducing an attention mechanism based on VGG16, and streamlining and optimizing the network structure model. Subsequently, a series of classification prediction experiments were carried out, and compared with the experimental results of SVGG16, the DenseNet121 transfer learning model, improved MobileNetV2, ResNet18, RepVGG and other models, and the overall results are better than those of the existing methods.
When using the MIAS data set, although ResNet18 was only an 18-layer network, due to the addition of a residual network, the network parameters reached 11,186,179, far exceeding other models, and the complexity of the model structure was indeed great. Although GoogLeNet converges at the fastest speed during model training, it takes 2349 seconds, 871 seconds longer than the 1478 seconds of the improved VGG16, but the result is not better than the improved VGG16. It can be seen that the Inception module in the GoogLeNet network will, indeed, bring great performance improvement, but the Inception module is a "network within a network" structure, which increases the width and depth of the network to some extent and extends the operation time. It can be seen from these two models that the complexity of the model structure will also affect the predictive performance of the model. The data set used in this paper is mammogram images, which, like most medical images, are only single channel pictures. Such a complex network structure model is indeed a big deal.
The DenseNet121 transfer learning model has the deepest network structure among all the models, and the training time is 5306 seconds, making it the longest training time in this paper. It can be seen that too many convolutional layers will significantly increase the complexity of training. The depth of the network should be properly adjusted according to the size of the data set. Excessive convolution kernels can better extract image features, but many redundant features will be generated, which will also cause underfitting or overfitting problems in training and affect the accuracy of model prediction.
The improved VGG16, through the introduction of ECANet, greatly improves the predictive ability of the model and stands out among multiple models. The results of training are the best among all models, and the accuracy, precision and recall in testing have reached 100%, which, once again, confirms that the attention mechanism can indeed help the convolutional network improve the predictive ability of the model. Depthwise separable convolution used by the improved VGG16 can indeed balance the problem of increasing computing power caused by the introduction of the BN algorithm and ECANet without too much change in parameters and computing power of the whole model.
In addition, although the improved VGG16 adopts the BN algorithm, compared with SVGG16, the convergence speed is indeed improved, but the convergence effect is slightly worse than that of GoogLeNet. Therefore, the improved VGG16 still has room for further improvement.
By simplifying and optimizing the classical VGG16 network structure model and introducing the attention mechanism, a new classification model is proposed in this paper, which achieves a better prediction effect. Although some progress has been made in classification accuracy, there are still some areas that need to be improved. The convergence rate of the improved VGG16 during training is indeed lower than that of GoogLeNet. How to improve the convergence rate needs to be further studied. Only the convolution network algorithm is used to design the model without considering the possibility of some algorithms in the computer vision field to achieve mammography image classification. Classification was only limited to judging whether there were lesions in the mammogram images, benign or malignant lesions, and BI-RADS image classification was not used. In a follow-up study, more algorithms will be adopted to continue to optimize the model to have better application value in clinical practice.
This research was funded by the Young Backbone Teachers in Higher Education Institutions in Henan Province (2019GGJS184).
We declare that there are no conflicts of interest.
[1] |
J. Tang, R. M. Rangayyan, J. Xu, Y. Yang, I. E. Naqa, Computer-aided breast cancer detection and diagnosis using mammography: recent advance, IEEE Trans. Inf. Technol. Biomed., 13 (2008), 236–251. https://doi.org/10.1109/TITB.2008.2009441 doi: 10.1109/TITB.2008.2009441
![]() |
[2] |
X. Liu, J. Tang, Mass classification in mammograms using selected geometry and texture features and a new SVM-based feature selection method, IEEE Syst. J., 8 (2014), 910–920. https://doi.org/10.1109/JSYST.2013.2286539. doi: 10.1109/JSYST.2013.2286539
![]() |
[3] | F. Mohanty, S. Rup, B. Dash, B. Majhi, M. N. S. Swamy, Mammogram classification using contourlet features with forest optimization-based feature selection approach, 78 (2019), 12805–12834. https://doi.org/10.1007/s11042-018-5804-0 |
[4] |
D. A. Ragab, M. Sharkas, O. Attallah, Breast cancer diagnosis using an efficient CAD system based on multiple classifiers, Diagnostics, 9 (2019), 165–191. https://doi.org/10.3390/diagnostics9040165 doi: 10.3390/diagnostics9040165
![]() |
[5] |
A. Krizhevsky, I. Sutskever, G. E. Hinton, ImageNet classification with deep convolutional neural networks, Commun. ACM, 60 (2017), 84–90. https://doi.org/10.1145/3065386 doi: 10.1145/3065386
![]() |
[6] | K. Simonyan, A. Zisserman, Very deep convolutional networks for large-scale image recognition, preprint, arXiv: 1409.1556. |
[7] | K. He, X. Zhang, S. Ren, J. Sun, Deep residual learning for image recognition, in Proceedings of the IEEE Conference on Computer Vision and Pattern Recognition (CVPR), (2016), 770–778. Available from: https://openaccess.thecvf.com/content_cvpr_2016/html/He_Deep_Residual_Learning_CVPR_2016_paper.html. |
[8] | M. Sandler, A. Howard, M. Zhu, A. Zhmoginov, L. Chen, MobileNetV2: inverted residuals and linear bottlenecks, in 2018 IEEE/CVF Conference on Computer Vision and Pattern Recognition, (2018), 4510–4520. https://doi.org/10.1109/CVPR.2018.00474 |
[9] | G. Huang, Z. Liu, L. Maaten, K. Weinberger, Densely connected convolutional networks, in 2017 IEEE Conference on Computer Vision and Pattern Recognition (CVPR), (2017), 2261–2269. https://doi.org/10.1109/CVPR.2017.243 |
[10] | X. Ding, X. Zhang, N. Ma, J. Han, G. Ding, J. Sun, RepVGG: making VGG-style ConvNets great again, in 2021 IEEE/CVF Conference on Computer Vision and Pattern Recognition (CVPR), (2021), 13728–13737. https://doi.org/10.1109/CVPR46437.2021.01352 |
[11] | A. Goyal, A. Bochkovskiy, J. Deng, V. Koltun, Non-deep networks, preprint, arXiv: 2110.07641. |
[12] | Z. Liu, H. Mao, C. Wu, C. Feichtenhofer, T. Darrell, S. Xie, A ConvNet for the 2020s, in 2022 IEEE/CVF Conference on Computer Vision and Pattern Recognition (CVPR), (2022), 11966–11976. https://doi.org/10.1109/CVPR52688.2022.01167 |
[13] |
A. Pan, S. Xu, S. Cheng, Y. She, Breast mass image recognition based on SVGG16, J. South-Cent. Minzu Univ. (Nat. Sci. Ed.), 40 (2021), 410–416. https://doi.org/10.12130/znmdzk.20210412 doi: 10.12130/znmdzk.20210412
![]() |
[14] | M. Hu, Breast Disease Image Classification Based on Improved Convolutional Neural Network and Multi-scale Feature Fusion, MD. thesis, Donghua University, 2023. https://doi.org/10.27012/d.cnki.gdhuu.2022.001136 |
[15] | Y. Yang, M. Liu, X. Wang, Z. Xiao, Y. Jiang, Breast cancer image recognition based on DenseNet and transfer learning, J. Jilin Univ. (Inf. Sci. Ed.), 40 (2022), 213–218. Available from: http://xuebao.jlu.edu.cn/xxb/CN/Y2022/V40/I2/213. |
[16] |
M. Meng, L. Li, G. He, M. Zhang, D. Shen, C. Pan, et al., A preliminary study of MobileNetV2 to downgrade classification in mammographic BI-RADS 4 lesions, J. Clin. Radiol., 41 (2022), 1868–1873. https://doi.org/10.13437/j.cnki.jcr.2022.10.031 doi: 10.13437/j.cnki.jcr.2022.10.031
![]() |
[17] | S. Ioffe, C. Szegedy, Batch normalization: accelerating deep network training by reducing internal covariate shift, in Proceedings of the 32nd International Conference on Machine Learning, (2015), 448–456. Available from: http://proceedings.mlr.press/v37/ioffe15.html. |
[18] | Q. Wang, B. Wu, P. Zhu, P. Li, W. Zuo, Q. Hu, ECA-Net: efficient channel attention for deep convolutional neural networks, in 2020 IEEE/CVF Conference on Computer Vision and Pattern Recognition (CVPR), (2020), 11531–11539. https://doi.org/10.1109/CVPR42600.2020.01155 |
1. | Hui Xu, Xinyang Zhao, Qiyun Yin, Junting Dou, Ruopeng Liu, Wengang Wang, Isolating switch state detection system based on depth information guidance, 2024, 32, 2688-1594, 836, 10.3934/era.2024040 | |
2. | Gunjan Shandilya, Vatsala Anand, 2024, Taming Breast Cancer Diagnosis Through Transfer Learning: A Binary Classification Framework for Enhanced Disease Detection, 979-8-3503-8747-6, 255, 10.1109/ICTACS62700.2024.10840454 |
Model | Parameter | Times (s) | Loss | Accuracy | Precision | Recall |
Improved AlexNet | 220,179 | 2560 | 0.0457 | 0.9847 | 0.9851 | 0.9844 |
SVGG16 | 8,644,355 | 1154 | 0.0266 | 0.9926 | 0.9927 | 0.9926 |
RepVGG | 7,854,499 | 2369 | 0.0122 | 0.9962 | 0.9963 | 0.9962 |
ResNet18 | 11,186,179 | 1834 | 0.0165 | 0.9944 | 0.9944 | 0.9944 |
Improved ResNet18 | 1,065,091 | 2994 | 0.1111 | 0.9646 | 0.9678 | 0.9606 |
Improved MobileNetV2 | 4,107,551 | 4745 | 0.0408 | 0.9885 | 0.9893 | 0.9878 |
DenseNet121 | 7,034,307 | 5306 | 0.0138 | 0.9954 | 0.9955 | 0.9953 |
GoogLeNet | 6,751,435 | 2349 | 0.0097 | 0.9972 | 0.9972 | 0.9972 |
Improved VGG16 | 8,666,824 | 1478 | 0.0061 | 0.9980 | 0.9980 | 0.9980 |
Model | Loss | Accuracy | Precision | Recall |
Improved AlexNet | 0.0307 | 0.9887 | 0.9889 | 0.9886 |
SVGG16 | 0.0210 | 0.9944 | 0.9944 | 0.9943 |
RepVGG | 3.8456 | 0.6353 | 0.6355 | 0.6347 |
ResNet18 | 1.7089 | 0.7536 | 0.7542 | 0.7533 |
Improved ResNet18 | 7.3758 | 0.6587 | 0.6589 | 0.6586 |
Improved MobileNetV2 | 0.2280 | 0.9340 | 0.9368 | 0.9315 |
DenseNet121 | 0.0226 | 0.9907 | 0.9909 | 0.9906 |
GoogLeNet | 0.0288 | 0.9918 | 0.9923 | 0.9914 |
Improved VGG16 | 0.0001 | 1.0000 | 1.0000 | 1.0000 |
Model | Loss | Accuracy | Precision | Recall |
Improved VGG16 | 0.0176 | 0.9939 | 0.9885 | 0.9884 |
SVGG16 | 0.2117 | 0.9099 | 0.8601 | 0.7862 |
Model | Loss | Accuracy | Precision | Recall |
Improved VGG16 | 0.3150 | 0.9188 | 0.7975 | 0.9337 |
SVGG16 | 0.2209 | 0.9070 | 0.8483 | 0.7950 |
Model | Loss | Accuracy | Precision | Recall |
Improved VGG16 | 0.0558 | 0.9805 | 0.9809 | 0.9802 |
SVGG16 | 0.1451 | 0.9461 | 0.9477 | 0.9446 |
Model | Loss | Accuracy | Precision | Recall |
Improved VGG16 | 0.2604 | 0.9186 | 0.9194 | 0.9179 |
SVGG16 | 0.2912 | 0.8944 | 0.8972 | 0.8923 |
Model | Parameter | Times (s) | Loss | Accuracy | Precision | Recall |
Improved AlexNet | 220,179 | 2560 | 0.0457 | 0.9847 | 0.9851 | 0.9844 |
SVGG16 | 8,644,355 | 1154 | 0.0266 | 0.9926 | 0.9927 | 0.9926 |
RepVGG | 7,854,499 | 2369 | 0.0122 | 0.9962 | 0.9963 | 0.9962 |
ResNet18 | 11,186,179 | 1834 | 0.0165 | 0.9944 | 0.9944 | 0.9944 |
Improved ResNet18 | 1,065,091 | 2994 | 0.1111 | 0.9646 | 0.9678 | 0.9606 |
Improved MobileNetV2 | 4,107,551 | 4745 | 0.0408 | 0.9885 | 0.9893 | 0.9878 |
DenseNet121 | 7,034,307 | 5306 | 0.0138 | 0.9954 | 0.9955 | 0.9953 |
GoogLeNet | 6,751,435 | 2349 | 0.0097 | 0.9972 | 0.9972 | 0.9972 |
Improved VGG16 | 8,666,824 | 1478 | 0.0061 | 0.9980 | 0.9980 | 0.9980 |
Model | Loss | Accuracy | Precision | Recall |
Improved AlexNet | 0.0307 | 0.9887 | 0.9889 | 0.9886 |
SVGG16 | 0.0210 | 0.9944 | 0.9944 | 0.9943 |
RepVGG | 3.8456 | 0.6353 | 0.6355 | 0.6347 |
ResNet18 | 1.7089 | 0.7536 | 0.7542 | 0.7533 |
Improved ResNet18 | 7.3758 | 0.6587 | 0.6589 | 0.6586 |
Improved MobileNetV2 | 0.2280 | 0.9340 | 0.9368 | 0.9315 |
DenseNet121 | 0.0226 | 0.9907 | 0.9909 | 0.9906 |
GoogLeNet | 0.0288 | 0.9918 | 0.9923 | 0.9914 |
Improved VGG16 | 0.0001 | 1.0000 | 1.0000 | 1.0000 |
Model | Loss | Accuracy | Precision | Recall |
Improved VGG16 | 0.0176 | 0.9939 | 0.9885 | 0.9884 |
SVGG16 | 0.2117 | 0.9099 | 0.8601 | 0.7862 |
Model | Loss | Accuracy | Precision | Recall |
Improved VGG16 | 0.3150 | 0.9188 | 0.7975 | 0.9337 |
SVGG16 | 0.2209 | 0.9070 | 0.8483 | 0.7950 |
Model | Loss | Accuracy | Precision | Recall |
Improved VGG16 | 0.0558 | 0.9805 | 0.9809 | 0.9802 |
SVGG16 | 0.1451 | 0.9461 | 0.9477 | 0.9446 |
Model | Loss | Accuracy | Precision | Recall |
Improved VGG16 | 0.2604 | 0.9186 | 0.9194 | 0.9179 |
SVGG16 | 0.2912 | 0.8944 | 0.8972 | 0.8923 |