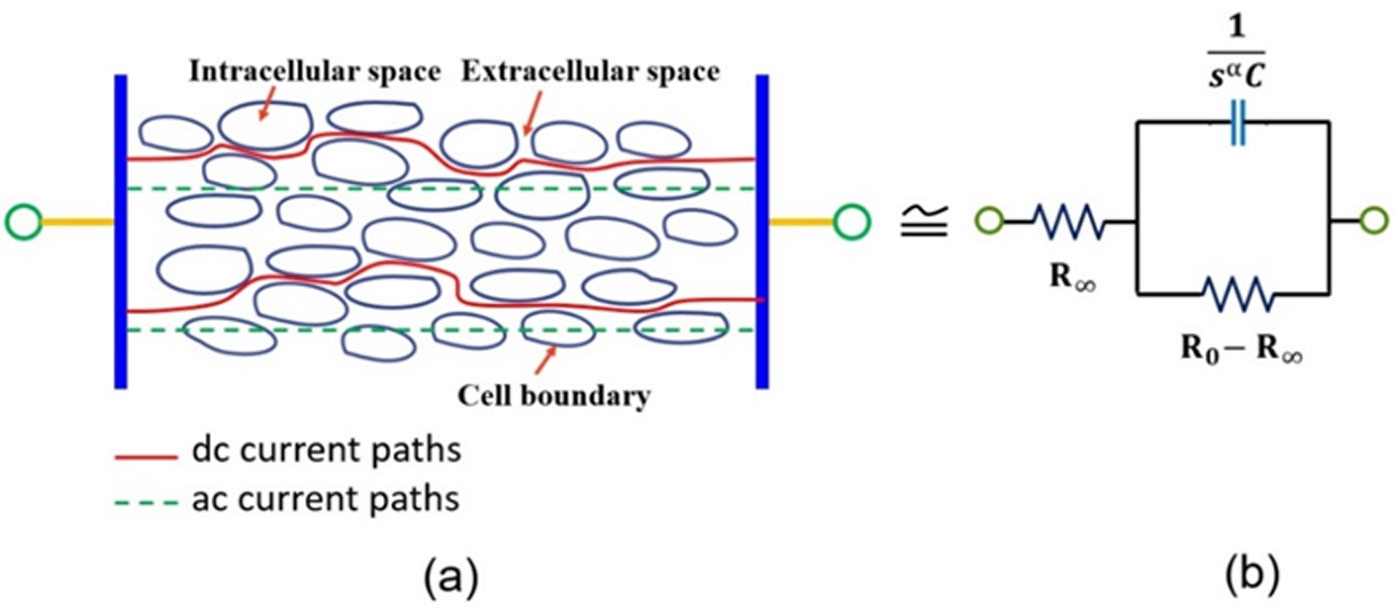
In real life, there are a lot of uncertainties in engineering structure design, and the potential uncertainties will have an important impact on the structural performance responses. Therefore, it is of great significance to consider the uncertainty in the initial stage of structural design to improve product performance. The consensus can be reached that the mechanical structure obtained by the reliability and robustness design optimization method considering uncertainty not only has low failure risk but also has highly stable performance. As a large mechanical system, the uncertainty design optimization of key vehicle structural performances is particularly important. This survey mainly discusses the current situation of the uncertain design optimization framework of automobile structures, and successively summarizes the uncertain design optimization of key automobile structures, uncertainty analysis methods, and multi-objective iterative optimization models. The uncertainty analysis method in the design optimization framework needs to consider the existing limited knowledge and limited test data. The importance of the interval model as a non-probabilistic model in the uncertainty analysis and optimization process is discussed. However, it should be noted that the interval model ignores the actual uncertainty distribution rule, which makes the design scheme still have some limitations. With the further improvement of design requirements, the efficiency, accuracy, and calculation cost of the entire design optimization framework of automobile structures need to be further improved iteratively. This survey will provide useful theoretical guidance for engineers and researchers in the automotive engineering field at the early stage of product development.
Citation: Xiang Xu, Chuanqiang Huang, Chongchong Li, Gang Zhao, Xiaojie Li, Chao Ma. Uncertain design optimization of automobile structures: A survey[J]. Electronic Research Archive, 2023, 31(3): 1212-1239. doi: 10.3934/era.2023062
[1] | Svetlana Kashina, Andrea Monserrat del Rayo Cervantes-Guerrero, Francisco Miguel Vargas-Luna, Gonzalo Paez, Jose Marco Balleza-Ordaz . Tissue-specific bioimpedance changes induced by graphene oxide ex vivo: a step toward contrast media development. AIMS Biophysics, 2025, 12(1): 54-68. doi: 10.3934/biophy.2025005 |
[2] | Abhishek Mallick, Atanu Mondal, Somnath Bhattacharjee, Arijit Roy . Application of nature inspired optimization algorithms in bioimpedance spectroscopy: simulation and experiment. AIMS Biophysics, 2023, 10(2): 132-172. doi: 10.3934/biophy.2023010 |
[3] | Arijit Roy, Somnath Bhattacharjee, Soumyajit Podder, Advaita Ghosh . Measurement of bioimpedance and application of Cole model to study the effect of moisturizing cream on human skin. AIMS Biophysics, 2020, 7(4): 362-379. doi: 10.3934/biophy.2020025 |
[4] | D Kelly, L Mackenzie, David A. Saint . Mechanoelectric feedback does not contribute to the Frank-Starling relation in the rat and guinea pig heart. AIMS Biophysics, 2014, 1(1): 16-30. doi: 10.3934/biophy.2014.1.16 |
[5] | Moataz M. Fahmy, Sohier M. El-Kholey, Seham Elabd, Mamdouh M. Shawki . Effect of changing the alternating electric current frequency on the viability of human liver cancer cell line (HEPG2). AIMS Biophysics, 2025, 12(1): 1-13. doi: 10.3934/biophy.2025001 |
[6] | Alexander G. Volkov, Yuri B. Shtessel . Propagation of electrotonic potentials in plants: Experimental study and mathematical modeling. AIMS Biophysics, 2016, 3(3): 358-379. doi: 10.3934/biophy.2016.3.358 |
[7] | Alaa AL-Rahman Gamal, El-Sayed Mahmoud El-Sayed, Tarek El-Hamoly, Heba Kahil . Development and bioevaluation of controlled release 5-aminoisoquinoline nanocomposite: a synergistic anticancer activity against human colon cancer. AIMS Biophysics, 2022, 9(1): 21-41. doi: 10.3934/biophy.2022003 |
[8] | Zachary Mekus, Jessica Cooley, Aaron George, Victoria Sabo, Morgan Strzegowski, Michelle Starz-Gaiano, Bradford E. Peercy . Effects of cell packing on chemoattractant distribution within a tissue. AIMS Biophysics, 2018, 5(1): 1-21. doi: 10.3934/biophy.2018.1.1 |
[9] |
Maria Teresa Caccamo, Giuseppe Mavilia, Letterio Mavilia, Pietro Calandra, Domenico Lombardo, Salvatore Magazù .
Thermal investigation of montmorillonite/BSA by fourier transform infrared spectroscopy measurements |
[10] | Enrica Serretiello, Martina Iannaccone, Federica Titta, Nicola G. Gatta, Vittorio Gentile . Possible pathophysiological roles of transglutaminase-catalyzed reactions in the pathogenesis of human neurodegenerative diseases. AIMS Biophysics, 2015, 2(4): 441-457. doi: 10.3934/biophy.2015.4.441 |
In real life, there are a lot of uncertainties in engineering structure design, and the potential uncertainties will have an important impact on the structural performance responses. Therefore, it is of great significance to consider the uncertainty in the initial stage of structural design to improve product performance. The consensus can be reached that the mechanical structure obtained by the reliability and robustness design optimization method considering uncertainty not only has low failure risk but also has highly stable performance. As a large mechanical system, the uncertainty design optimization of key vehicle structural performances is particularly important. This survey mainly discusses the current situation of the uncertain design optimization framework of automobile structures, and successively summarizes the uncertain design optimization of key automobile structures, uncertainty analysis methods, and multi-objective iterative optimization models. The uncertainty analysis method in the design optimization framework needs to consider the existing limited knowledge and limited test data. The importance of the interval model as a non-probabilistic model in the uncertainty analysis and optimization process is discussed. However, it should be noted that the interval model ignores the actual uncertainty distribution rule, which makes the design scheme still have some limitations. With the further improvement of design requirements, the efficiency, accuracy, and calculation cost of the entire design optimization framework of automobile structures need to be further improved iteratively. This survey will provide useful theoretical guidance for engineers and researchers in the automotive engineering field at the early stage of product development.
The method of monitoring physiological condition by means of bioimpedance remains a novel technique for more than 50 years or so. The non-destructive method of bioimpedance measurement for fruits and vegetables is extensively used for various purposes such as to access health conditions [1],[2], effect of storage conditions [3], physiological changes including assessing the quality of fruits and vegetables [4]–[8]. On the other hand, investigations are also carried out to understand the science behind the characteristics of bioimpedance of plant tissues [9]–[17]
The necessity of invariability of bioimpedance parameters is extremely important in practical application of Cole model for discriminating fruits (or vegetables) from a reference fruit (or vegetable). Say for example someone wants to compare the Cole parameters for two different species of a given fruit. In such cases, the parameters can be compared if the parameters are not dependent on position (of a given fruit or sample). If the position (of measurement) dependent variability is high, one cannot distinguish two different samples under investigation. Thus, the measurement method should be robust enough to establish invariability of bioimpedance parameters which can be treated as reference parameters for comparison purpose.
Cole model is widely applied for living biological tissue [15],[18]–[21]. In general, lump tissue is represented by Cole equivalent circuit and single dispersion model is accepted for its simplicity [7],[14] (Figure 1). Among the various method of bioimpedance measurements, direct measurement (i.e. using LCR meter) with two-electrode configuration is the most generic one due to its high degree of reliability [5],[20]. The electrical current paths inside a tissue is schematically shown in Figure 1 (a). It is intuitive from this figure, that if impedance is measured for the same tissue at other position, keeping the distance between the electrode invariant, one should obtain the same results (within the limits of experimental error for living tissue). On the other hand, if the distance between the electrodes is not kept same for measuring impedance at two different positions, the variability in measured parameters are clearly observed [14],[15],[22]. Thus, in order to obtain the comparable results among the samples, it is quite obvious to keep the distance between the electrode invariant. The reasons of choosing apple and potato are as follows. First of all, apple is a fruit whereas potato is a vegetable (tuber) and both of them are extensively used as food materials and therefore have importance in agriculture sector. Further, apple grows in open atmosphere whereas potato grows under soil. Not only these, the tissue of apple will have a natural tendency towards ripen (or to be eaten) so that seed can be dispersed. On the other hand, potato tissue will have tendency to fight against external environment to sustain so as to produce the next generation potato. The porosity (fraction of empty space) of apple is highly different than that of potato.
In this work, a specific electrode-pair is designed and is used to measure bioimpedance of apple and potato tissues. It is needless to mention that, though apple is a fruit, potato is a vegetable and providing us two different kind of living tissue to be investigated under this study. Applying single dispersion Cole model, impedance parameters are extracted by curve fitting. It is established here that the tissue of apple and potato can be specified in terms of bioimpedance parameters which in turn can be used to discriminate similar tissue of different species with 98% confidence. Further, with 99% confidence, it is evidently shown here that the position of measurement of bioimpedance of a given fruit or vegetable is not a factor of variability. Thus, our process of measurement can directly be applied in assessing quality (or condition) of fruits or vegetables. In addition to this, our method can be applied to monitor the ripening of fruits similar to citrus fruit like orange [8].
Single dispersion Cole bioimpedance model is a widely accepted model is extensively used for various kinds of biological tissue
where,
Separation of Z into real and imaginary parts is not straight forward due to the non-integer value of α (0 < α ≤1), which turns the
Thus, the modulus of Z becomes
Experimentally, Eq. (4) |Z| is obtained as a function of frequency f (= ω/2π). The experimental data is then fitted (non-linear curve fitting) and values of R0, R∞, C, α are obtained. From these parameters, the relaxation time τ is calculated using Eq. (2).
In general, the physiological parameters are non-repetitive and standard deviation is large. For example, if at identical condition, the cell size is measured for a given tissue, the variation of more than 20% is not uncommon while the grain size of a metallic conductor is measured, the result is found to be within the tolerance level (about 5% or so). The variation in cell size would therefore lead to a large variation in the tissue related parameters. Thus, it is extremely important to specify the tissue by means of Cole model parameters as well as relaxation time. On the other hand, it is essential to keep the distance (or gap) between the electrode-pair invariant. Intuitively, this is due to the fact that the Cole parameters (except α) are highly dependent on the gap between the electrodes. Hence, to discriminate tissues from one another, it is essential to obtain the invariability of impedance irrespective of measuring position (of tissue) of fruits or vegetable.
An electrode-pair is designed and fabricated for the purpose of bioimpedance measurement of fruit or vegetable. A schematic of the fabricated electrode-pair is shown in Figure 2. The depth of penetration and diameter of the electrodes are 1.5 cm and 0.28 mm respectively and these are maintained throughout this work unless otherwise stated. The electrodes are separated by plastic material (of negligibly low leakage current). The plastic holder of the electrode-pair is made by cutting a disposable syringe. The gap between the electrode is kept at 3 mm. For electrode material, stain-less steel is chosen for its inertness of reacting with any other materials and retain sufficient strength at low wire diameter.
Sample | Species/Description | Designation of Measurement |
Apple-1 | Apple-1 and Apple-2 are of same species (Green Granny Smith) and both are purchased from same local shop. Apple-1 and Apple-2 can be considered to be biologically identical. | A11, A12, A13, A14, A15 |
Apple-2 | A21, A22, A23, A24, A25 | |
Apple-3 | Apple-3 (Red Washington) is of different species that of Apple-1 and Apple-2. Apple-3 is biologically different from Apple-1 and Apple-2. | A31, A32, A33, A34, A35 |
Potato-1 | Potato-1 and Potato-2 are of same species (Kufri Chandramukhi) and both are purchased from same local shop. Potato-1 and Potato-2 can be considered to be biologically identical. | P11, P12, P13, P14, P15 |
Potato-2 | P21, P22, P23, P24, P25 | |
Potato-3 | Potato-3 (Kufri Jyoti) is of different species that of Potato-1 and Potato-2. Potato-3 is biologically different from Potato-1 and Potato-2. | P31, P32, P33, P34, P35 |
Remarks: In this table, Amn (or Pmn) represents impedance measurement of Apple-m (or Potato-m) at position n.
The sample matrix is shown Table 1. In order to remove any parasitic effect that may arise from electrodes and probes, the LCR meter (Model: 8101G, Make: Gw Instek) is calibrated before taking any measurement data. Each time electrodes are cleaned by medicated sprit and dried properly before inserting into fruit or vegetable. By inserting the electrode-pair at desired position of the sample, impedance is measured in the frequency range of 1 Hz to 1 MHz with amplitude of 1 V (peak to peak). All measurements are conducted at identical condition. In order to avoid any physical damage in the tissue due to high electric field, small signal is chosen for measurement. The impedance is found to decrease with the increase in frequency and becomes sufficiently flat around the frequency of 1 MHz and thus restricts the choice of upper limit on the frequency of interest. For each sample, five measurements are done at five different positions and each measurement contains four hundred data points. Typical measurement data are plotted as a function of frequency and is shown in Figure 3.
Experimentally |Z| is obtained as a function of frequency for different samples and for different position of a given sample as mentioned in Table 1. The non-linear curve fitting is performed by commercial MS Excel 2016 package. As a common practice, in the curve fitting process, the value of R0 and R∞ is considered to be the measured values R0 and R∞. Hence in this work, the measured impedances at 1 Hz and 1 MHz are considered to be R0 and R∞ respectively. The value of α and C are estimated by fitting the experimental curve with the Eq. (4). The characteristic time constant τ is then estimated using Eq. (2) for each set of data.
From the experimental plots of |Z| as a function of frequency (see Figure 3) it is not possible to distinguish the species of a given fruit or vegetable and this is because the plots of different species almost overlap on each other when plotted simultaneously in a single graph. On the other hand, the important parameters related to equivalent circuit of these plots may differ significantly.
The fitted Cole plots are shown in Figure 3(b) and Figure 3(d) for apple and potato respectively. Since, the sample Apple-1 and Apple-2 are from same species, the Cole plots of these do not differ and follow one another for the entire span of frequency range. While the Cole plot for the sample Apple-3 slightly differ from the other two, especially at the low frequency range. This is probably due to the fact that the species of sample Apple-3 is different from that of sample Apple-1 or Apple-2. Exactly similar observation is found for potato samples (Figure 3. (d)). From the fitted Cole plots, it is noticed that the distinguishable capability of bioimpedance method is better at the low frequency range for apple as well for potato. This fact suggests that the bioimpedance study of apple and potato can be limited to 3 kHz. By reducing the frequency range while keeping the number data point same, we can obtain better experimental results.
In this study, all ANOVA are performed using commercial MS Excel 2016 package. The Cole parameters for apple samples are shown in Table 2. ANOVA on τ is performed considering position and sample as two factors. The first factor is considered to understand whether position of measurement is a factor or not. On the other hand, the second factor is considered to understand the discriminating capability of the bioimpedance measurement.
To test these hypotheses, two apples of same species (Green Granny Smith in this case) are taken from a local shop and thus the biological differences between the apples are minimized. Then for each apple, impedance is measured at five different positions. The measured and calculated data is shown in Table 2. Result of ANOVA for tissue of apple is shown in Table 3. Interestingly it is found that none of the factors are significant (since, Fcritical > F-value) at the confidence level as high as 99% when ANOVA is performed among the sample Apple-1 and Apple-2. This means that one the Cole parameters i.e. the relaxation time are indistinguishable as far position is concerned. The two samples (Apple-1/Apple-2) are also found to be indistinguishable. Or in other words, the tissues of Apple-1 and Apple-2 are statistically same. Thus, with respect to our electrode system, the relaxation time is invariable and it is does depend on the position of measurement and remains same for biologically same samples.
On the other hand, though the position is found be indistinguishable in Apple-1 and Apple-3 (or Apple-2 and Apple-3), they are found to be disguisable (Fcritical < F-value) when ANOVA is performed for these two pair of samples. Thus, again as before, the position of measurement is not found to be a factor. However, in the present two cases, the sample is found to be a factor and is discriminated by respective relaxation time. This means the tissues of Apple-1 and Apple-3 are different. This fact proves that the relaxation time of apple tissue can be used to discriminate different species of apple.
Sample | R0 (kΩ) |
R∞ (kΩ) |
α | C (nF secα−1) |
τ (µsec) |
Regression coefficient |
12.93 | 0.64 | 0.719 | 76.65 | 63 | 0.9989 | |
14.21 | 0.74 | 0.703 | 88.30 | 70 | 0.9987 | |
Apple-1 | 13.58 | 0.77 | 0.691 | 99.99 | 65 | 0.9983 |
13.75 | 0.65 | 0.705 | 91.55 | 72 | 0.9989 | |
12.12 | 0.63 | 0.692 | 103.83 | 60 | 0.9983 | |
12.83 | 0.61 | 0.694 | 98.25 | 63 | 0.9980 | |
13.67 | 0.70 | 0.708 | 86.55 | 69 | 0.9985 | |
Apple-2 | 13.90 | 0.72 | 0.696 | 103.47 | 76 | 0.9985 |
12.47 | 0.67 | 0.693 | 112.05 | 70 | 0.9983 | |
10.97 | 0.70 | 0.694 | 113.46 | 59 | 0.9984 | |
11.85 | 0.83 | 0.745 | 62.35 | 57 | 0.9993 | |
10.53 | 0.77 | 0.747 | 64.20 | 52 | 0.9991 | |
Apple-3 | 11.35 | 0.84 | 0.754 | 55.15 | 51 | 0.9994 |
13.75 | 0.84 | 0.767 | 47.47 | 65 | 0.9997 | |
11.74 | 0.84 | 0.751 | 56.65 | 53 | 0.9993 |
Remarks: Apple-1&Apple-2 are of same species and are taken from same lot. While Apple-3 is different from the other two.
Sample | Factor | P-value | F-value | Fcritical | Distinguishability | Level of Confidence |
Apple-1 & Apple-2 | Position | 0.119 | 3.627 | 15.977 | No | 99% |
Sample | 0.594 | 0.334 | 21.197 | No | ||
Apple-1 & Apple-3 | Position | 0.146 | 3.153 | 10.899 | No | 98% |
Sample | 0.017 | 15.384 | 14.039 | Yes |
Sample | R0 (kΩ) |
R∞ (kΩ) |
α | C (nF secα−1) |
τ (µsec) | Regression coefficient |
5.17 | 0.14 | 0.6400 | 341 | 48 | 0.9888 | |
5.48 | 0.16 | 0.7000 | 204 | 41 | 0.9956 | |
Potato-1 | 5.58 | 0.13 | 0.6638 | 246 | 47 | 0.9920 |
5.65 | 0.12 | 0.6505 | 325 | 60 | 0.9922 | |
5.09 | 0.11 | 0.6797 | 243 | 51 | 0.9951 | |
5.14 | 0.12 | 0.6275 | 445 | 60 | 0.9897 | |
5.43 | 0.13 | 0.6532 | 286 | 48 | 0.9918 | |
Potato-2 | 6.66 | 0.16 | 0.6077 | 450 | 68 | 0.9890 |
4.70 | 0.12 | 0.6415 | 363 | 47 | 0.9891 | |
5.67 | 0.12 | 0.5979 | 580 | 68 | 0.9865 | |
5.52 | 0.12 | 0.6360 | 420 | 70 | 0.9934 | |
5.57 | 0.15 | 0.6099 | 610 | 68 | 0.9906 | |
Potato-3 | 6.70 | 0.14 | 0.6202 | 432 | 78 | 0.9912 |
5.86 | 0.11 | 0.6362 | 393 | 69 | 0.9916 | |
5.46 | 0.13 | 0.6436 | 349 | 57 | 0.9924 |
Remarks: Potato -1 & Potato -2 are of same species and are taken from same lot, while Potato -3 is different from the other two.
Sample | Factor | P-value | F-value | Fcritical | Distinguishability | Level of Confidence |
Potato-1 & Potato-2 | Position | 0.6046 | 0.7537 | 15.977 | No | 99% |
Sample | 0.2124 | 2.1975 | 21.198 | No | ||
Potato-1& Potato-3 | Position | 0.6201 | 0.7218 | 10.899 | No | 98% |
Sample | 0.0182 | 14.856 | 14.040 | Yes |
The various Cole parameters for potato samples are tabulated in Table 4. Similar to apple samples, ANOVA is performed on τ for potato samples and the result of the ANOVA is shown in Table 5. Based on the ANOVA, we have obtained similar conclusion as in apples. That is position of measurement is not a factor and different tissue of potato samples can be distinguished based on the relaxation time.
In order to gain confidence on our discrimination method described above, the entire experiment is repeated by taking another two specimens of each type using the same electrode-pair. When data is analyzed similar outcome is resulted. Thus, our method is found to be repetitive and robust.
It is intuitive from the Figure 1(a), that the values of the Cole parameters are highly dependent on (a) cell size, (b) porosity, (c) constituents etc. In order to assess the cell size, extensive analysis is performed on the cellular images obtained using a confocal microscope (Olympus CX41). The average cell size of apple and potato (of typical species used in bioimpedance measurement) are estimated to be (10–12) × 103 µm2 and (18–20) × 103 µm2 respectively. The measured cell areas agree well with the similar measurement presented in published references [23],[24]. Thus, the cell size of potato is about 1.8 times larger than that of apple.
On the other hand, the porosity of the apple and potato are in the range of 0.15–0.22 and 0.03–0.08 respectively [25]. Hence, apple tissue contains much more extracellular space than that of the potato. The estimated cell size and porosity in the tissues under investigation can be used to explain the capacitance obtained experimentally. The capacitance of potato tissue is found larger than that of apple tissue (see Table II and Table IV). In the cellular structure, two nearby cells and extracellular space between cells behave like the plates and dielectric material respectively of a parallel plate capacitor. As the capacitance of a parallel plate capacitor is proportional to the plate area and is inversely proportional to the separation between the plates (the extracellular space), the capacitance (C) is found larger in potato tissue as its cell size is larger and extracellular space (porosity) is smaller.
The dc resistance on the other hand depends on the length the dc current path. Longer the dc current path, larger is the dc resistance R0. Typical dc current paths are drawn manually (based on the principle that dc current flows through the extracellular space) and are shown in Figure 4. It is clear from this figure that the detouring of dc current path of apple tissue is greater than that of potato tissue and as a result apple shows larger R0 which is indeed found experimentally (see Table 2 and Table 4). Thus, the orientation of cells in the tissue highly affects the dc resistance. More the misorientation among the cells, longer is the dc current path and yields larger dc resistance. Another factor that may affect the dc resistance is the resistivity of the constituents of the extracellular space in tissues and such investigation is beyond the scope of this work. Therefore, the comparison of Cole parameters of the tissues of Apple and Potato from the biophysical point of view is tentative.
An experimental method is established here to distinguish different species of fruits or vegetable by means of measuring Cole parameters. Cole parameters are extracted for apple and potato tissue by specially designed electrode-pair using direct measurement technique. Among the various Cole parameters, the relaxation time τ is found to be most promising tissue characterizing parameter, since it contains all other Cole parameters of a given tissue. With statistical relevance, it is shown that tissues of different species of apple as well as potato can be distinguished by our experimental method. Our method can have wide application to distinguish unhealthy (or treated) fruits or vegetables from healthy one. The method is not costly and therefore this method has potential application in accessing plant tissue.
[1] |
Z. Zhang, X. Jia, T. Yang, Y. Gu, W. Wang, L. Chen, Multi-objective optimization of lubricant volume in an ELSD considering thermal effects, Int. J. Therm. Sci., 164 (2021), 106884. https://doi.org/10.1016/j.ijthermalsci.2021.106884 doi: 10.1016/j.ijthermalsci.2021.106884
![]() |
[2] |
C. Yu, J. Liu, J. Zhang, K. Xue, S. Zhang, J. Liao, et al., Design and optimization and experimental verification of a segmented double-helix blade roller for straw returning cultivators, J. Chin. Inst. Eng., 44 (2021), 379–387. https://doi.org/10.1080/02533839.2021.1903342 doi: 10.1080/02533839.2021.1903342
![]() |
[3] |
Y. Cao, J. Yao, J. Li, X. Chen, J. Wu, Optimization of microbial oils production from kitchen garbage by response surface methodology, J. Renew. Sustain. Ener., 5 (2013), 053105. https://doi.org/10.1063/1.4821218 doi: 10.1063/1.4821218
![]() |
[4] |
K. Cao, Z. Li, Y. Gu, L. Zhang, L. Chen, The control design of transverse interconnected electronic control air suspension based on seeker optimization algorithm, P. I. Mech. Eng. D-J. Aut., 235 (2021), 2200–2211. https://doi.org/10.1177/0954407020984667 doi: 10.1177/0954407020984667
![]() |
[5] |
G. Wang, H. Yuan, Stability and critical spinning speed of a flexible liquid-filled rotor in thermal environment with nonlinear variable-temperature, Appl. Math. Model., 95 (2021), 143–158. https://doi.org/10.1016/j.apm.2021.01.056 doi: 10.1016/j.apm.2021.01.056
![]() |
[6] |
G. Wang, H. Yuan, Dynamics and stability analysis of an axially functionally graded hollow rotor partially filled with liquid, Compos. Struct., 266 (2021), 113821. https://doi.org/10.1016/j.compstruct.2021.113821 doi: 10.1016/j.compstruct.2021.113821
![]() |
[7] |
G. Wang, W. Yang, H. Yuan, Dynamics and stability analysis of a flexible liquid-filled rotor in a constant thermal environment, J. Vib. Control, 28 (2021), 2913–2924. https://doi.org/10.1177/10775463211022489 doi: 10.1177/10775463211022489
![]() |
[8] |
D. Zhang, N. Zhang, N. Ye, J. Fang, X. Han, Hybrid learning algorithm of radial basis function networks for reliability analysis, IEEE Trans. Reliab., 70 (2021), 887–900. https://doi.org/10.1109/TR.2020.3001232 doi: 10.1109/TR.2020.3001232
![]() |
[9] |
X. Du, H. Xu, F. Zhu, A data mining method for structure design with uncertainty in design variables, Comput. Struct., 244 (2021), 106457. https://doi.org/10.1016/j.compstruc.2020.106457 doi: 10.1016/j.compstruc.2020.106457
![]() |
[10] |
E. Acar, G. Bayrak, Y. Jung, I. Lee, P. Ramu, S. S. Ravichandran, Modeling, analysis and optimization under uncertainties: A review, Struct. Multidiscip. Optimiz., 64 (2021), 2909–2945. https://doi.org/10.1007/s00158-021-03026-7 doi: 10.1007/s00158-021-03026-7
![]() |
[11] |
M. Zimmermann, S. Königs, C. Niemeyer, J. Fender, C. Zeherbauer, R. Vitale, et al., On the design of large systems subject to uncertainty, J. Eng. Design, 28 (2017), 233–254. https://doi.org/10.1080/09544828.2017.1303664 doi: 10.1080/09544828.2017.1303664
![]() |
[12] | Y. Noh, K. K. Choi, I. Lee, Reduction of ordering effect in reliability-based design optimizatioin using dimension reduction method, AIAA J. 47 (2009), 994–1004. https://doi.org/10.2514/1.40224 |
[13] |
U. Lee, N. Kang, I. Lee, Selection of optimal target reliability in RBDO through reliability-based design for market systems (RBDMS) and application to electric vehicle design, Struct. Multidiscip. Optimiz., 60 (2019), 949–963. https://doi.org/10.1007/s00158-019-02245-3 doi: 10.1007/s00158-019-02245-3
![]() |
[14] |
K. Wang, P. Wang, X. Chen, L. T. Zhao, Multiobjective optimization design of toll plaza, Math. Probl. Eng., 2020 (2020), 2324894. https://doi.org/10.1155/2020/2324894 doi: 10.1155/2020/2324894
![]() |
[15] | C. Yu, D. Zhu, Y. Gao, K. Xue, S. Zhang, J. Liao, et al., Optimization and experiment of counter-rotating straw returning cultivator based on discrete element method, J. Adv. Mech. Des. Syst., 14 (2020). https://doi.org/10.1299/jamdsm.2020jamdsm0097 |
[16] |
S. Liu, J. Sun, H. Zhou, F. Wei, M. Lu, M. Lei, Experimental and numerical study on fatigue performance for TIG welding and EB welding of RAFM steel plate, Fusion Eng. Des., 146 (2019), 2663–2666. https://doi.org/10.1016/j.fusengdes.2019.04.076 doi: 10.1016/j.fusengdes.2019.04.076
![]() |
[17] |
J. Mendoza, E. Bismut, D. Straub, J. Köhler, Optimal life-cycle mitigation of fatigue failure risk for structural systems, Reliab. Eng. Syst. Safe., 222 (2022), 108390. https://doi.org/10.1016/j.ress.2022.108390 doi: 10.1016/j.ress.2022.108390
![]() |
[18] |
C. A. Castiglioni, R. Pucinotti, Failure criteria and cumulative damage models for steel components under cyclic loading, J. Constr. Steel Res., 65 (2009), 751–765. https://doi.org/10.1016/j.jcsr.2008.12.007 doi: 10.1016/j.jcsr.2008.12.007
![]() |
[19] | D. M. Harland, R. D. Lorenz, Space systems failures: Disasters and rescues of satellites, rockets and space probes, in Springer Praxis Books, Springer Praxis, 2005. https://doi.org/10.1007/978-0-387-27961-9 |
[20] |
A. D. Kiureghian, O. Ditlevsen, Aleatory or epistemic? Does it matter?, Struct. Safe., 31 (2009), 105–112. https://doi.org/10.1016/j.strusafe.2008.06.020 doi: 10.1016/j.strusafe.2008.06.020
![]() |
[21] |
M. A. Hariri-Ardebili, F. Pourkamali-Anaraki, Structural uncertainty quantification with partial information, Expert Syst. Appl., 198 (2022), 116736. https://doi.org/10.1016/j.eswa.2022.116736 doi: 10.1016/j.eswa.2022.116736
![]() |
[22] | K. Bowcutt. A perspective on the future of aerospace vehicle design, in 12th AIAA International Space Planes and Hypersonic Systems and Technologies: American Institute of Aeronautics and Astronautics, 2003. |
[23] |
J. Fang, Y. Gao, G. Sun, Y. Zhang, Q. Li, Crashworthiness design of foam-filled bitubal structures with uncertainty, Int. J. Non-Lin. Mech., 67 (2014), 120–132. https://doi.org/10.1016/j.ijnonlinmec.2014.08.005 doi: 10.1016/j.ijnonlinmec.2014.08.005
![]() |
[24] |
S. A. Latifi Rostami, A. Kolahdooz, J. Zhang, Robust topology optimization under material and loading uncertainties using an evolutionary structural extended finite element method, Eng. Anal. Bound. Elem., 133 (2021), 61–70. https://doi.org/10.1016/j.enganabound.2021.08.023 doi: 10.1016/j.enganabound.2021.08.023
![]() |
[25] |
X. Wang, Z. Meng, B. Yang, C. Cheng, K. Long, J. Li, Reliability-based design optimization of material orientation and structural topology of fiber-reinforced composite structures under load uncertainty, Compos. Struct., 2022 (2022), 115537. https://doi.org/10.1016/j.compstruct.2022.115537 doi: 10.1016/j.compstruct.2022.115537
![]() |
[26] |
M. E. Riley, R. V. Grandhi, Quantification of model-form and predictive uncertainty for multi-physics simulation, Comput. Struct., 89 (2011), 1206–1213. https://doi.org/10.1016/j.compstruc.2010.10.004 doi: 10.1016/j.compstruc.2010.10.004
![]() |
[27] |
B. Do, M. Ohsaki, M. Yamakawa, Bayesian optimization for robust design of steel frames with joint and individual probabilistic constraints, Eng. Struct., 245 (2021), 112859. https://doi.org/10.1016/j.engstruct.2021.112859 doi: 10.1016/j.engstruct.2021.112859
![]() |
[28] |
Z. Meng, Y. Pang, Y. Pu, X. Wang, New hybrid reliability-based topology optimization method combining fuzzy and probabilistic models for handling epistemic and aleatory uncertainties, Comput. Method. Appl. Mech., 363 (2020), 112886. https://doi.org/10.1016/j.cma.2020.112886 doi: 10.1016/j.cma.2020.112886
![]() |
[29] |
C. Yang, H. Ouyang, A novel load-dependent sensor placement method for model updating based on time-dependent reliability optimization considering multi-source uncertainties, Mech. Syst. Signal Proces., 165 (2022), 108386. https://doi.org/10.1016/j.ymssp.2021.108386 doi: 10.1016/j.ymssp.2021.108386
![]() |
[30] |
G. A. da Silva, E. L. Cardoso, A. T. Beck, Comparison of robust, reliability-based and non-probabilistic topology optimization under uncertain loads and stress constraints, Probabilist. Eng. Mech., 59 (2020), 103039. https://doi.org/10.1016/j.probengmech.2020.103039 doi: 10.1016/j.probengmech.2020.103039
![]() |
[31] |
J. M. King, R. V. Grandhi, T. W. Benanzer, Quantification of epistemic uncertainty in re-usable launch vehicle aero-elastic design, Eng. Optimiz., 44 (2012), 489–504. https://doi.org/10.1080/0305215X.2011.588224 doi: 10.1080/0305215X.2011.588224
![]() |
[32] |
Y. C. Tsao, V. V. Thanh, A multi-objective fuzzy robust optimization approach for designing sustainable and reliable power systems under uncertainty, Appl. Soft Comput., 92 (2020), 106317. https://doi.org/10.1016/j.asoc.2020.106317 doi: 10.1016/j.asoc.2020.106317
![]() |
[33] |
L. Wang, B. Ni, X. Wang, Z. Li, Reliability-based topology optimization for heterogeneous composite structures under interval and convex mixed uncertainties, Appl. Math. Model., 99 (2021), 628–652. https://doi.org/10.1016/j.apm.2021.06.014 doi: 10.1016/j.apm.2021.06.014
![]() |
[34] |
L. A. Zadeh, Fuzzy sets as a basis for a theory of possibility, Fuzzy Set. Syst., 1 (1978), 3–28. https://doi.org/10.1016/0165-0114(78)90029-5 doi: 10.1016/0165-0114(78)90029-5
![]() |
[35] |
H. Lü, K. Yang, X. Huang, H. Yin, W. B. Shangguan, D. Yu, An efficient approach for the design optimization of dual uncertain structures involving fuzzy random variables, Comput. Method. Appl. Mech., 371 (2020), 113331. https://doi.org/10.1016/j.cma.2020.113331 doi: 10.1016/j.cma.2020.113331
![]() |
[36] | R. E. Moore, Methods and Application of Interval Analysis, SIAM: Philadelphia, USA, 1979. https://doi.org/10.1137/1.9781611970906 |
[37] |
S. P. Gurav, J. F. L. Goosen, F. vanKeulen, Bounded-But-Unknown uncertainty optimization using design sensitivities and parallel computing: Application to MEMS, Comput. Struct., 83 (2005), 1134–1149. https://doi.org/10.1016/j.compstruc.2004.11.021 doi: 10.1016/j.compstruc.2004.11.021
![]() |
[38] |
R. Yuan, D. Ma, H. Zhang, Flow characteristics and grain size distribution of granular gangue mineral by compaction treatment, Adv. Mater. Sci. Eng., 2017 (2017), 2509286. https://doi.org/10.1155/2017/2509286 doi: 10.1155/2017/2509286
![]() |
[39] | G. Sun, T. Pang, J. Fang, G. Li, Q. Li, Parameterization of criss-cross configurations for multiobjective crashworthiness optimization, Int. J. Mech. Sci., 124–125 (2017), 145–157. https://doi.org/10.1016/j.ijmecsci.2017.02.027 |
[40] |
J. Fang, G. Sun, N. Qiu, T. Pang, S. Li, Q. Li, On hierarchical honeycombs under out-of-plane crushing, Int. J. Solids Struct., 135 (2018), 1–13. https://doi.org/10.1016/j.ijsolstr.2017.08.013. doi: 10.1016/j.ijsolstr.2017.08.013
![]() |
[41] |
J. Fu, Q. Liu, K. Liufu, Y. Deng, J. Fang, Q. Li, Design of bionic-bamboo thin-walled structures for energy absorption, Thin-Wall. Struct., 135 (2019), 400–413. https://doi.org/10.1016/j.tws.2018.10.003 doi: 10.1016/j.tws.2018.10.003
![]() |
[42] |
J. Fang, Y. Gao, G. Sun, G. Zheng, Q. Li, Dynamic crashing behavior of new extrudable multi-cell tubes with a functionally graded thickness, Int. J. Mech. Sci., 103 (2015), 63–73. https://doi.org/10.1016/j.ijmecsci.2015.08.029 doi: 10.1016/j.ijmecsci.2015.08.029
![]() |
[43] |
J. Fang, Y. Gao, G. Sun, N. Qiu, Q. Li, On design of multi-cell tubes under axial and oblique impact loads, Thin-Wall. Struct., 95 (2015), 115–126. https://doi.org/10.1016/j.tws.2015.07.002 doi: 10.1016/j.tws.2015.07.002
![]() |
[44] |
J. Fang, G. Sun, N. Qiu, N. H. Kim, Q. Li, On design optimization for structural crashworthiness and its state of the art, Struct. Multidiscip. Optimiz., 55 (2017), 1091–1119. https://doi.org/10.1007/s00158-016-1579-y doi: 10.1007/s00158-016-1579-y
![]() |
[45] |
N. Qiu, Y. Gao, J. Fang, Z. Feng, G. Sun, Q. Li, Crashworthiness analysis and design of multi-cell hexagonal columns under multiple loading cases, Finite Elem. Anal. Des., 104 (2015), 89–101. https://doi.org/10.1016/j.finel.2015.06.004 doi: 10.1016/j.finel.2015.06.004
![]() |
[46] | S. Kodiyalam, High performance computing for multidisciplinary design optimization and robustness of vehicle structures, in Computational Fluid and Solid Mechanics 2003, Elsevier Science Ltd, (2003), 2305–2307. https://doi.org/10.1016/B978-008044046-0.50566-2 |
[47] |
K. Hamza, K. Saitou, Design Optimization of Vehicle Structures for Crashworthiness Using Equivalent Mechanism Approximations, J. Mech. Des., 127 (2004), 485–492. https://doi.org/10.1115/1.1862680 doi: 10.1115/1.1862680
![]() |
[48] |
X. Gu, G. Sun, G. Li, X. Huang, Y. Li, Q. Li, Multiobjective optimization design for vehicle occupant restraint system under frontal impact, Struct. Multidiscip. Optimiz., 47 (2013), 465–477. https://doi.org/10.1007/s00158-012-0811-7 doi: 10.1007/s00158-012-0811-7
![]() |
[49] |
L. Chen, W. Li, Y. Yang, W. Miao, Evaluation and optimization of vehicle pedal comfort based on biomechanics, Proc. Inst. Mech. Eng. Part D J. Automob. Eng., 234 (2019), 1402–1412. https://doi.org/10.1177/0954407019878355 doi: 10.1177/0954407019878355
![]() |
[50] |
X. L. Zhang, T. Wu, Y. Shao, J. Song, Structure optimization of wheel force transducer based on natural frequency and comprehensive sensitivity, Chin. J. Mech. Eng., 30 (2017), 973–981. https://doi.org/10.1007/s10033-017-0149-6 doi: 10.1007/s10033-017-0149-6
![]() |
[51] |
X. Xu, X. Chen, Z. Liu, Y. Zhang, Y. Xu, J. Fang, et al., A feasible identification method of uncertainty responses for vehicle structures, Struct. Multidiscip. Optimiz., 64 (2021), 3861–3876. https://doi.org/10.1007/s00158-021-03065-0 doi: 10.1007/s00158-021-03065-0
![]() |
[52] |
X. Liu, X. Liu, Z. Zhou, L. Hu, An efficient multi-objective optimization method based on the adaptive approximation model of the radial basis function, Struct. Multidiscip. Optimiz., 63 (2021), 1385–1403. https://doi.org/10.1007/s00158-020-02766-2 doi: 10.1007/s00158-020-02766-2
![]() |
[53] |
J. Fang, Y. Gao, G. Sun, C. Xu, Q. Li, Multiobjective robust design optimization of fatigue life for a truck cab, Reliab. Eng. Syst. Safe., 135 (2015), 1–8. https://doi.org/10.1016/j.ress.2014.10.007 doi: 10.1016/j.ress.2014.10.007
![]() |
[54] |
X. Gu, G. Sun, G. Li, L. Mao, Q. Li, A Comparative study on multiobjective reliable and robust optimization for crashworthiness design of vehicle structure, Struct. Multidiscip. Optimiz., 48 (2013), 669–684. https://doi.org/10.1007/s00158-013-0921-x doi: 10.1007/s00158-013-0921-x
![]() |
[55] |
J. Zhou, F. Lan, J. Chen, F. Lai, Uncertainty optimization design of a vehicle body structure considering random deviations, Automot. Innov., 1 (2018), 342–351. https://doi.org/10.1007/s42154-018-0041-9 doi: 10.1007/s42154-018-0041-9
![]() |
[56] |
J. Zhu, X. Wang, H. Zhang, Y. Li, R. Wang, Z. Qiu, Six sigma robust design optimization for thermal protection system of hypersonic vehicles based on successive response surface method, Chinese J. Aeronaut., 32 (2019), 2095–2108. https://doi.org/10.1016/j.cja.2019.04.009 doi: 10.1016/j.cja.2019.04.009
![]() |
[57] |
X. Wang, L. Shi, A new metamodel method using Gaussian process based bias function for vehicle crashworthiness design, Int. J. Crashworthines., 19 (2014), 311–321. https://doi.org/10.1080/13588265.2014.898932 doi: 10.1080/13588265.2014.898932
![]() |
[58] |
N. Qiu, J. Zhang, F. Yuan, Z. Jin, Y. Zhang, J. Fang, Mechanical performance of triply periodic minimal surface structures with a novel hybrid gradient fabricated by selective laser melting, Eng. Struct., 263 (2022), 114377. https://doi.org/10.1016/j.engstruct.2022.114377 doi: 10.1016/j.engstruct.2022.114377
![]() |
[59] |
L. Chen, P. Ma, J. Tian, X. Liang, Prediction and optimization of lubrication performance for a transfer case based on computational fluid dynamics, Eng. Appl. Comp. Fluid, 13 (2019), 1013–1023. https://doi.org/10.1080/19942060.2019.1663765 doi: 10.1080/19942060.2019.1663765
![]() |
[60] |
X. Xu, Y. Zhang, X. Wang, J. Fang, J. Chen, J. Li, Searching superior crashworthiness performance by constructing variable thickness honeycombs with biomimetic cells, Int. J. Mech. Sci., 235 (2022), 107718. https://doi.org/10.1016/j.ijmecsci.2022.107718 doi: 10.1016/j.ijmecsci.2022.107718
![]() |
[61] |
X. Xu, Y. Zhang, J. Wang, F. Jiang, C. H. Wang, Crashworthiness design of novel hierarchical hexagonal columns, Compos. Struct., 194 (2018), 36–48. https://doi.org/10.1016/j.compstruct.2018.03.099 doi: 10.1016/j.compstruct.2018.03.099
![]() |
[62] |
X. Song, L. Lai, S. Xiao, Y. Tang, M. Song, J. Zhang, et al., Bionic design and multi-objective optimization of thin-walled structures inspired by conchs, Electron. Res. Arch., 31 (2023), 575–598. https://doi.org/10.3934/era.2023028 doi: 10.3934/era.2023028
![]() |
[63] |
J. Fang, Y. Gao, G. Sun, Q. Li, Multiobjective reliability-based optimization for design of a vehicledoor, Finite Elem. Anal. Des., 67 (2013), 13–21. https://doi.org/10.1016/j.finel.2012.11.007 doi: 10.1016/j.finel.2012.11.007
![]() |
[64] |
N. Qiu, Z. Jin, J. Liu, L. Fu, Z. Chen, N. H. Kim, Hybrid multi-objective robust design optimization of a truck cab considering fatigue life, Thin-Wall. Struct., 162 (2021), 107545. https://doi.org/10.1016/j.tws.2021.107545 doi: 10.1016/j.tws.2021.107545
![]() |
[65] |
G. Sun, H. Zhang, J. Fang, G. Li, Q. Li, A new multi-objective discrete robust optimization algorithm for engineering design, Appl. Math. Model., 53 (2018), 602–621. https://doi.org/10.1016/j.apm.2017.08.016 doi: 10.1016/j.apm.2017.08.016
![]() |
[66] | F. Lei, X. Lv, J. Fang, T. Pang, Q. Li, G. Sun, Injury biomechanics-based nondeterministic optimization of front-end structures for safety in pedestrian-vehicle impact, Thin-Wall. Struct., 167 (2021). https://doi.org/10.1016/j.tws.2021.108087 |
[67] |
H. Lu, D. Yu, Brake squeal reduction of vehicle disc brake system with interval parameters by uncertain optimization, J. Sound Vib., 333 (2014), 7313–7325. https://doi.org/10.1016/j.jsv.2014.08.027 doi: 10.1016/j.jsv.2014.08.027
![]() |
[68] |
J. Wu, Z. Luo, Y. Zhang, N. Zhang, An interval uncertain optimization method for vehicle suspensions using Chebyshev metamodels, Appl. Math. Model., 38 (2014), 3706–3723. https://doi.org/10.1016/j.apm.2014.02.012 doi: 10.1016/j.apm.2014.02.012
![]() |
[69] |
A. Jamali, M. Salehpour, N. Nariman-zadeh, Robust Pareto active suspension design for vehicle vibration model with probabilistic uncertain parameters, Multibody Syst. Dyn., 30 (2013), 265–285. https://doi.org/10.1007/s11044-012-9337-4 doi: 10.1007/s11044-012-9337-4
![]() |
[70] |
X. Gu, W. Wang, L. Xia, P. Jiang, A system optimisation design approach to vehicle structure under frontal impact based on SVR of optimised hybrid kernel function, Int. J. Crashworthines., 26 (2021), 1–15. https://doi.org/10.1080/13588265.2019.1634335 doi: 10.1080/13588265.2019.1634335
![]() |
[71] |
Z. Liu, C. Jiang, Y. Li, Y. Bai, Fatigue life optimization for spot-welded Structures of vehicle body considering uncertainty of welding spots, China Mech. Eng., 26 (2015), 2544–2549. https://doi.org/10.3969/j.issn.1004-132X.2015.18.024 doi: 10.3969/j.issn.1004-132X.2015.18.024
![]() |
[72] |
L. Farkas, D. Moens, S. Donders, D. Vandepitte, Optimisation study of a vehicle bumper subsystem with fuzzy parameters, Mech. Syst. Signal Pr., 32 (2012), 59–68. https://doi.org/10.1016/j.ymssp.2011.11.014 doi: 10.1016/j.ymssp.2011.11.014
![]() |
[73] |
E. Acar, K. Solanki, System reliability based vehicle design for crashworthiness and effects of various uncertainty reduction measures, Struct. Multidiscip. Optimiz., 39 (2009), 311–325. https://doi.org/10.1007/s00158-008-0327-3 doi: 10.1007/s00158-008-0327-3
![]() |
[74] |
Q. Zhao, H. Zhang, Z. Zhu, R. Jiang, L. Yuan, Reliability-based topology optimization for vehicle suspension control arm, Aut. Eng., 40(2018), 313–319. https://doi.org/10.19562/j.chinasae.qcgc.2018.03.011 doi: 10.19562/j.chinasae.qcgc.2018.03.011
![]() |
[75] |
M. Grujicic, G. Arakere, W. C. Bell, H. Marvi, H. V. Yalavarthy, B. Pandurangan, et al., Reliability-based design optimization for durability of ground vehicle suspension system components, J. Mater. Eng. Perform., 19 (2010), 301–313. https://doi.org/10.1007/s11665-009-9482-y doi: 10.1007/s11665-009-9482-y
![]() |
[76] |
M. Rais-Rohani, K. N. Solanki, E. Acar, C. D. Eamon, Shape and sizing optimisation of automotive structures with deterministic and probabilistic design constraints, Int. J. Vehicle Des., 54 (2010), 309–338. https://doi.org/10.1504/IJVD.2010.036839 doi: 10.1504/IJVD.2010.036839
![]() |
[77] | X. Xu, J. Chen, Z. Lin, Y. Qiao, X. Chen, Y. Zhang, et al., Optimization design for the planetary gear train of an electric vehicle under uncertainties, Actuators, 11 (2022). https://doi.org/10.3390/act11020049 |
[78] |
G. Sun, H. Zhang, R. Wang, X. Lv, Q. Li, Multiobjective reliability-based optimization for crashworthy structures coupled with metal forming process, Struct. Multidiscip. Optimiz., 56 (2017), 1571–1587. https://doi.org/10.1007/s00158-017-1825-y doi: 10.1007/s00158-017-1825-y
![]() |
[79] |
Y. Xu, Y. Gao, C. Wu, J. Fang, Q. Li, Robust topology optimization for multiple fiber-reinforced plastic (FRP) composites under loading uncertainties, Struct. Multidiscip. Optimiz., 59 (2019), 695–711. https://doi.org/10.1007/s00158-018-2175-0 doi: 10.1007/s00158-018-2175-0
![]() |
[80] | C. van Mierlo, L. Burmberger, M. Daub, F. Duddeck, M. G. R. Faes, D. Moens, Interval methods for lack-of-knowledge uncertainty in crash analysis, Mech. Syst. Signal Proces., 168 (2022). https://doi.org/10.1016/j.ymssp.2021.108574 |
[81] |
C. Lin, F. Gao, Y. Bai, Multiobjective reliability-based design optimisation for front structure of an electric vehicle using hybrid metamodel accuracy improvement strategy-based probabilistic sufficiency factor method, Int. J. Crashworthines., 23 (2018), 290–301. https://doi.org/10.1080/13588265.2017.1317466 doi: 10.1080/13588265.2017.1317466
![]() |
[82] |
F. Y. Li, G. Y. Li, Interval-based uncertain multi-objective optimization design of vehicle crashworthiness, CMC-Comput. Mater. Con., 15 (2010), 199–219. https://doi.org/10.2478/s11533-009-0061-0 doi: 10.2478/s11533-009-0061-0
![]() |
[83] | J. Li, Y. Fang, Z. Zhan, Y. Jiang, An enhanced surrogate model based vehicle robust design method under materials and manufacturing uncertainties, in ASME International Mechanical Engineering Congress and Exposition, 2016. https://doi.org/10.1115/IMECE2016-67714 |
[84] | R. J. Yang, L. Gu, C. H. Tho, K. K. Choi, B. Youn, Reliability-based multidisciplinary design optimization of vehicle structures. in 43rd AIAA/ASME/ASCE/AHS/ASC Structures, Structural Dynamics, and Materials Conference, 2002. https://doi.org/10.2514/6.2002-1758 |
[85] |
L. Cao, J. Liu, L. Xie, C. Jiang, R. Bi, Non-probabilistic polygonal convex set model for structural uncertainty quantification, Appl. Math. Model., 89 (2021), 504–518. https://doi.org/10.1016/j.apm.2020.07.025 doi: 10.1016/j.apm.2020.07.025
![]() |
[86] | X. Meng, J. Liu, L. Cao, Z. Yu, D. Yang, A general frame for uncertainty propagation under multimodally distributed random variables, Comput. Method. Appl. Mech. Eng., 367 (2020). https://doi.org/10.1016/j.cma.2020.113109 |
[87] | G. Chen, D. Yang, A unified analysis framework of static and dynamic structural reliabilities based on direct probability integral method, Mech. Syst. Signal Proces., 158 (2021). https://doi.org/10.1016/j.ymssp.2021.107783 |
[88] | X. Y. Long, D. L. Mao, C. Jiang, F. Y. Wei, G. J. Li, Unified uncertainty analysis under probabilistic, evidence, fuzzy and interval uncertainties, Comput. Method. Appl. Mech. Eng., 355 (2019), 1–26. https://doi.org/10.1016/j.cma.2019.05.041 |
[89] |
X. Xu, X. Chen, Z. Liu, J. Yang, Y. Xu, Y. Zhang, et al., Multi-objective reliability-based design optimization for the reducer housing of electric vehicles, Eng. Optimiz., 54 (2022), 1324–1340. https://doi.org/10.1080/0305215X.2021.1923704 doi: 10.1080/0305215X.2021.1923704
![]() |
[90] | N. Qiu, C. Park, Y. Gao, J. Fang, G. Sun, N. H. Kim, Sensitivity-based parameter calibration and model validation under model error, J. Mech. Des., 140 (2017). https://doi.org/10.1115/1.4038298 |
[91] |
Z. L. Huang, C. Jiang, Y. S. Zhou, Z. Luo, Z. Zhang, An incremental shifting vector approach for reliability-based design optimization, Struct. Multidiscip. Optimiz., 53 (2016), 523–543. https://doi.org/10.1007/s00158-015-1352-7 doi: 10.1007/s00158-015-1352-7
![]() |
[92] |
W. Gao, D. Wu, K. Gao, X. Chen, F. Tin-Loi, Structural reliability analysis with imprecise random and interval fields, Appl. Math. Model., 55 (2018), 49–67. https://doi.org/10.1016/j.apm.2017.10.029 doi: 10.1016/j.apm.2017.10.029
![]() |
[93] |
N. Qiu, Y. Gao, J. Fang, G. Sun, Q. Li, N. H. Kim, Crashworthiness optimization with uncertainty from surrogate model and numerical error, Thin-Wall. Struct., 129 (2018), 457–472. https://doi.org/10.1016/j.tws.2018.05.002 doi: 10.1016/j.tws.2018.05.002
![]() |
[94] |
G. S. Song, M. C. Kim, Theoretical approach for uncertainty quantification in probabilistic safety assessment using sum of lognormal random variables, Nucl. Eng. Technol., 54 (2022), 2084–2093. https://doi.org/10.1016/j.net.2021.12.033 doi: 10.1016/j.net.2021.12.033
![]() |
[95] |
N. A. W. Walton, R. Crowder, N. Satvat, N. R. Brown, V. Sobes, Demonstration of a random sampling approach to uncertainty propagation for generic pebble-bed fluoride-salt-cooled high temperature reactor (gFHR), Nucl. Eng. Des., 395 (2022), 111843. https://doi.org/10.1016/j.nucengdes.2022.111843 doi: 10.1016/j.nucengdes.2022.111843
![]() |
[96] |
X. P. Du, W. Chen, Sequential optimization and reliability assessment method for efficient probabilistic design, J. Mech. Des., 126 (2004), 225–233. https://doi.org/10.1115/1.1649968 doi: 10.1115/1.1649968
![]() |
[97] |
Y. Lei, N. Yang, D. Xia, Probabilistic structural damage detection approaches based on structural dynamic response moments, Smart Struct. Syst., 20 (2017), 207–217. https://doi.org/10.12989/sss.2017.20.2.207 doi: 10.12989/sss.2017.20.2.207
![]() |
[98] |
P. Hoang-Anh, T. Viet-Hung, V. Tien-Chuong, Fuzzy finite element analysis for free vibration response of functionally graded semi-rigid frame structures, Appl. Math. Model., 88 (2020), 852–869. https://doi.org/10.1016/j.apm.2020.07.014 doi: 10.1016/j.apm.2020.07.014
![]() |
[99] |
Z. Chen, Z. Lu, C. Ling, K. Feng, Reliability analysis model of time-dependent multi-mode system under fuzzy uncertainty: Applied to undercarriage structures, Aerosp. Sci. Technol., 120 (2022), 107278. https://doi.org/10.1016/j.ast.2021.107278 doi: 10.1016/j.ast.2021.107278
![]() |
[100] |
P. Wang, H. Zhu, H. Tian, G. Cai, Analytic target cascading with fuzzy uncertainties based on global sensitivity analysis for overall design of launch vehicle powered by hybrid rocket motor, Aerosp. Sci. Technol., 114 (2021), 106680. https://doi.org/10.1016/j.ast.2021.106680 doi: 10.1016/j.ast.2021.106680
![]() |
[101] |
S. Yin, D. Yu, H. Yin, B. Xia, A new evidence-theory-based method for response analysis of acoustic system with epistemic uncertainty by using Jacobi expansion, Comput. Method. Appl. Mech. Eng., 322 (2017), 419–440. https://doi.org/10.1016/j.cma.2017.04.020 doi: 10.1016/j.cma.2017.04.020
![]() |
[102] |
H. Tang, D. Li, J. Li, S. Xue, Epistemic uncertainty quantification in metal fatigue crack growth analysis using evidence theory, Int. J. Fatigue, 99 (2017), 163–174. https://doi.org/10.1016/j.ijfatigue.2017.03.004 doi: 10.1016/j.ijfatigue.2017.03.004
![]() |
[103] |
Z. Zhang, X. X. Ruan, M. F. Duan, C. Jiang, An efficient epistemic uncertainty analysis method using evidence theory, Comput. Method. Appl. Mech. Eng., 339 (2018), 443–466. https://doi.org/10.1016/j.cma.2018.04.033 doi: 10.1016/j.cma.2018.04.033
![]() |
[104] |
X. Tang, K. Yuan, N. Gu, P. Li, R. Peng, An interval quantification-based optimization approach for wind turbine airfoil under uncertainties, Energy, 244 (2022), 122623. https://doi.org/10.1016/j.energy.2021.122623 doi: 10.1016/j.energy.2021.122623
![]() |
[105] |
Z. Niu, H. Zhu, X. Huang, A. Che, S. Fu, S. Meng, et al., Uncertainty quantification method for elastic wave tomography of concrete structure using interval analysis, Measurement, 205 (2022), 112160. https://doi.org/10.1016/j.measurement.2022.112160 doi: 10.1016/j.measurement.2022.112160
![]() |
[106] |
B. Y. Ni, C. Jiang, P. G. Wu, Z. H. Wang, W. Y. Tian, A sequential simulation strategy for response bounds analysis of structures with interval uncertainties, Comput. Struct., 266 (2022), 106785. https://doi.org/10.1016/j.compstruc.2022.106785 doi: 10.1016/j.compstruc.2022.106785
![]() |
[107] | T. Tang, H. Luo, Y. Song, H. Fang, J. Zhang, Chebyshev inclusion function based interval kinetostatic modeling and parameter sensitivity analysis for Exechon-like parallel kinematic machines with parameter uncertainties, Mech. Mach. Theory, 157 (2021). https://doi.org/10.1016/j.mechmachtheory.2020.104209 |
[108] |
C. Viegas, D. Daney, M. Tavakoli, A. T. de Almeida, Performance analysis and design of parallel kinematic machines using interval analysis, Mech. Mach. Theory, 115 (2017), 218–236. https://doi.org/10.1016/j.mechmachtheory.2017.05.003 doi: 10.1016/j.mechmachtheory.2017.05.003
![]() |
[109] | M. Ma, L. Wang, Reliability-based topology optimization framework of two-dimensional phononic crystal band-gap structures based on interval series expansion and mapping conversion method, Int. J. Mech. Sci., 196 (2021). https://doi.org/10.1016/j.ijmecsci.2020.106265 |
[110] | Z. Qiu, X. Li, A new model for the eigenvalue buckling analysis with unknown-but-bounded parameters, Aerosp. Sci. Technol., 113 (2021). https://doi.org/10.1016/j.ast.2021.106634 |
[111] |
B. Xia, H. Lu, D. Yu, C. Jiang, Reliability-based design optimization of structural systems under hybrid probabilistic and interval model, Comput. Struct., 160 (2015), 126–134. https://doi.org/10.1016/j.compstruc.2015.08.009 doi: 10.1016/j.compstruc.2015.08.009
![]() |
[112] | L. Wang, Z. Chen, G. Yang, Q. Sun, J. Ge, An interval uncertain optimization method using back-propagation neural network differentiation, Comput. Method. Appl. Mech. Eng., 366 (2020). https://doi.org/10.1016/j.cma.2020.113065 |
[113] |
S. H. Chen, L. Ma, G. W. Meng, R. Guo, An efficient method for evaluating the natural frequencies of structures with uncertain-but-bounded parameters, Comput. Struct., 87 (2009), 582–590. https://doi.org/10.1016/j.compstruc.2009.02.009 doi: 10.1016/j.compstruc.2009.02.009
![]() |
[114] |
N. Impollonia, G. Muscolino, Interval analysis of structures with uncertain-but-bounded axial stiffness, Comput. Method. Appl. Mech. Eng., 200 (2011), 1945–1962. https://doi.org/10.1016/j.cma.2010.07.019 doi: 10.1016/j.cma.2010.07.019
![]() |
[115] |
G. Zhao, G. Wen, J. Liu, A novel analysis method for vibration systems under time-varying uncertainties based on interval process model, Probabilistic Eng. Mech., 70 (2022), 103363. https://doi.org/10.1016/j.probengmech.2022.103363 doi: 10.1016/j.probengmech.2022.103363
![]() |
[116] |
L. Wang, J. Liu, C. Yang, D. Wu, A novel interval dynamic reliability computation approach for the risk evaluation of vibration active control systems based on PID controllers, Appl. Math. Model., 92 (2021), 422–446. https://doi.org/10.1016/j.apm.2020.11.007 doi: 10.1016/j.apm.2020.11.007
![]() |
[117] |
W. Gao, D. Wu, C. Song, F. Tin-Loi, X. Li, Hybrid probabilistic interval analysis of bar structures with uncertainty using a mixed perturbation Monte-Carlo method, Finite Elem. Anal. Des., 47 (2011), 643–652. https://doi.org/10.1016/j.finel.2011.01.007 doi: 10.1016/j.finel.2011.01.007
![]() |
[118] |
Z. P. Qiu, X. J. Wang, Solution theorems for the standard eigenvalue problem of structures with uncertain-but-bounded parameters, J. Sound Vib., 282 (2005), 381–399. https://doi.org/10.1016/j.jsv.2004.02.024 doi: 10.1016/j.jsv.2004.02.024
![]() |
[119] |
F. Li, Z. Luo, J. Rong, N. Zhang, Interval multi-objective optimisation of structures using adaptive Kriging approximations, Comput. Struct., 119 (2013), 68–84. https://doi.org/10.1016/j.compstruc.2012.12.028 doi: 10.1016/j.compstruc.2012.12.028
![]() |
[120] |
J. A. Fernandez-Prieto, J. Canada-Bago, M. A. Gadeo-Martos, J. R. Velasco, Optimisation of control parameters for genetic algorithms to test computer networks under realistic traffic loads, Appl. Soft Comput., 12 (2012), 1875–1883. https://doi.org/10.1016/j.asoc.2012.04.018 doi: 10.1016/j.asoc.2012.04.018
![]() |
[121] |
Y. G. Xu, G. R. Li, Z. P. Wu, A novel hybrid genetic algorithm using local optimizer based on heuristic pattern move, Appl. Artif. Intell., 15 (2001), 601–631. https://doi.org/10.1080/088395101750363966 doi: 10.1080/088395101750363966
![]() |
[122] |
C. Jiang, X. Han, G. P. Liu, A sequential nonlinear interval number programming method for uncertain structures, Comput. Meth. Appl. Mech. Eng., 197 (2008), 4250–4265. https://doi.org/10.1016/j.cma.2008.04.027 doi: 10.1016/j.cma.2008.04.027
![]() |
[123] |
J. Cheng, M. Y. Tang, Z. Y. Liu, J. R. Tan, Direct reliability-based design optimization of uncertain structures with interval parameters, J. Zhejiang Univ-Sci. A, 17 (2016), 841–854. https://doi.org/10.1631/jzus.A1600143 doi: 10.1631/jzus.A1600143
![]() |
[124] |
Y. P. Li, G. H. Huang, P. Guo, Z. F. Yang, S. L. Nie, A dual-interval vertex analysis method and its application to environmental decision making under uncertainty, Eur. J. Oper. Res., 200 (2010), 536–550. https://doi.org/10.1016/j.ejor.2009.01.013 doi: 10.1016/j.ejor.2009.01.013
![]() |
[125] |
C. Wang, H. G. Matthies, Non-probabilistic interval process model and method for uncertainty analysis of transient heat transfer problem, Int. J. Therm. Sci., 144 (2019), 147–157. https://doi.org/10.1016/j.ijthermalsci.2019.06.002 doi: 10.1016/j.ijthermalsci.2019.06.002
![]() |
[126] |
S. Nayak, S. Chakraverty, Non-probabilistic approach to investigate uncertain conjugate heat transfer in an imprecisely defined plate, Int. J. Heat Mass Tran., 67 (2013), 445–454. https://doi.org/10.1016/j.ijheatmasstransfer.2013.08.036 doi: 10.1016/j.ijheatmasstransfer.2013.08.036
![]() |
[127] |
Z. Qiu, X. Wang, J. Chen, Exact bounds for the static response set of structures with uncertain-but-bounded parameters, Int. J. Solids Struct., 43 (2006), 6574–6593. https://doi.org/10.1016/j.ijsolstr.2006.01.012 doi: 10.1016/j.ijsolstr.2006.01.012
![]() |
[128] |
Z. Qiu, Y. Xia, H. Yang, The static displacement and the stress analysis of structures with bounded uncertainties using the vertex solution theorem, Comput. Method. Appl. Mech. Eng., 196 (2007), 4965–4984. https://doi.org/10.1016/j.cma.2007.06.022 doi: 10.1016/j.cma.2007.06.022
![]() |
[129] |
Z. P. Qiu, S. H. Chen, I. Elishakoff, Bounds of eigenvalues for structures with an interval description of uncertain-but-non-random parameters, Chaos Solition. Fract., 7 (1996), 425–434. https://doi.org/10.1016/0960-0779(95)00065-8 doi: 10.1016/0960-0779(95)00065-8
![]() |
[130] |
Z. P. Qiu, X. J. Wang, Parameter perturbation method for dynamic responses of structures with uncertain-but-bounded parameters based on interval analysis, Int. J. Solids Struct., 42 (2005), 4958–4970. https://doi.org/10.1016/j.ijsolstr.2005.02.023 doi: 10.1016/j.ijsolstr.2005.02.023
![]() |
[131] |
Z. Qiu, L. Ma, X. Wang, Non-probabilistic interval analysis method for dynamic response analysis of nonlinear systems with uncertainty, J. Sound Vib., 319 (2009), 531–540. https://doi.org/10.1016/j.jsv.2008.06.006 doi: 10.1016/j.jsv.2008.06.006
![]() |
[132] |
D. Meng, T. Xie, P. Wu, C. He, Z. Hu, Z. Lv, An uncertainty-based design optimization strategy with random and interval variables for multidisciplinary engineering systems, Structures, 32 (2021), 997–1004. https://doi.org/10.1016/j.istruc.2021.03.020 doi: 10.1016/j.istruc.2021.03.020
![]() |
[133] |
X. Liu, X. Yu, J. Tong, X. Wang, X. Wang, Mixed uncertainty analysis for dynamic reliability of mechanical structures considering residual strength, Reliab. Eng. Syst. Safe., 209 (2021), 107472. https://doi.org/10.1016/j.ress.2021.107472 doi: 10.1016/j.ress.2021.107472
![]() |
[134] |
J. Wang, Z. Lu, Probabilistic safety model and its efficient solution for structure with random and interval mixed uncertainties, Mech. Mach. Theory, 147 (2020), 103782. https://doi.org/10.1016/j.mechmachtheory.2020.103782 doi: 10.1016/j.mechmachtheory.2020.103782
![]() |
[135] |
D. M. Do, K. Gao, W. Yang, C. Q. Li, Hybrid uncertainty analysis of functionally graded plates via multiple-imprecise-random-field modelling of uncertain material properties, Comput. Method. Appl. Mech. Eng., 368 (2020), 113116. https://doi.org/10.1016/j.cma.2020.113116 doi: 10.1016/j.cma.2020.113116
![]() |
[136] |
J. Wang, Z. Lu, M. Zhong, T. Wang, C. Sun, H. Li, Coupled thermal–structural analysis and multi-objective optimization of a cutting-type energy-absorbing structure for subway vehicles, Thin-Wall. Struct., 141 (2019), 360-373. https://doi.org/10.1016/j.tws.2019.04.026 doi: 10.1016/j.tws.2019.04.026
![]() |
[137] | M. Luo, Y. Chen, D. Gao, L. Wang, Inversion study of vehicle frontal collision and front bumper collision, Electron. Res. Arch. 31 (2023), 776–792. https://doi.org/10.3934/era.2023039 |
[138] |
W. Li, J. Wang, Z. Du, H. Ma, L. Zhang, L. Duan, Lightweight design method and application of MEWP bracket based on multi-level optimization, Electron. Res. Arch., 30 (2022), 4416–4435. https://doi.org/10.3934/era.2022224 doi: 10.3934/era.2022224
![]() |
[139] |
X. Xu, G. Xu, J. Chen, Z. Liu, X. Chen, Y. Zhang, et al., Multi-objective design optimization using hybrid search algorithms with interval uncertainty for thin-walled structures, Thin-Wall. Struct., 175 (2022), 109218. https://doi.org/10.1016/j.tws.2022.109218 doi: 10.1016/j.tws.2022.109218
![]() |
[140] |
Y. Wu, W. Li, J. Fang, Q. Lan, Multi-objective robust design optimization of fatigue life for a welded box girder, Eng. Optimiz., 50 (2018), 1252–1269. https://doi.org/10.1080/0305215X.2017.1395023 doi: 10.1080/0305215X.2017.1395023
![]() |
[141] |
Y. Wu, L. Sun, P. Yang, J. Fang, W. Li, Energy absorption of additively manufactured functionally bi-graded thickness honeycombs subjected to axial loads, Thin-Wall. Struct., 164 (2021), 107810. https://doi.org/10.1016/j.tws.2021.107810 doi: 10.1016/j.tws.2021.107810
![]() |
[142] |
Y. Su, H. Tang, S. Xue, D. Li, Multi-objective differential evolution for truss design optimization with epistemic uncertainty, Adv. Struct. Eng., 19 (2016), 1403–1419. https://doi.org/10.1177/1369433216643250 doi: 10.1177/1369433216643250
![]() |
[143] |
X. Liu, Q. Fu, N. Ye, L. Yin, The multi-objective reliability-based design optimization for structure based on probability and ellipsoidal convex hybrid model, Struct. Safe., 77 (2019), 48–56. https://doi.org/10.1016/j.strusafe.2018.11.004 doi: 10.1016/j.strusafe.2018.11.004
![]() |
[144] | T. Vo-Duy, D. Duong-Gia, V. Ho-Huu, T. Nguyen-Thoi, An effective couple method for reliability-based multi-objective optimization of truss structures with static and dynamic constraints, Int. J. Comput. Method., 17 (2020). https://doi.org/10.1142/S0219876219500166 |
[145] |
F. S. Lobato, M. A. da Silva, A. A. Cavalini, V. Steffen, Reliability-based robust multi-objective optimization applied to engineering system design, Eng. Optimiz., 52 (2020), 1–21. https://doi.org/10.1080/0305215X.2019.1577413 doi: 10.1080/0305215X.2019.1577413
![]() |
[146] |
P. Wang, S. Zheng, G. Wu, Multidisciplinary design optimization of vehicle body structure based on collaborative optimization and multi-objective genetic algorithm, Chinese J. Mech. Eng., 47 (2011), 102–108. https://doi.org/10.3901/JME.2011.02.102 doi: 10.3901/JME.2011.02.102
![]() |
[147] |
H. Yin, H. Fang, Y. Xiao, G. Wen, Q. Qing, Multi-objective robust optimization of foam-filled tapered multi-cell thin-walled structures, Struct. Multidiscip. Optimiz., 52 (2015), 1051–1067. https://doi.org/10.1007/s00158-015-1299-8 doi: 10.1007/s00158-015-1299-8
![]() |
[148] |
A. Khakhali, N. Nariman-zadeh, A. Darvizeh, A. Masoumi, B. Notghi, Reliability-based robust multi-objective crashworthiness optimisation of S-shaped box beams with parametric uncertainties, Int. J. Crashworthines., 15 (2010), 443–456. https://doi.org/10.1080/13588261003696458 doi: 10.1080/13588261003696458
![]() |
[149] |
A. U. Ebenuwa, K. F. Tee, Y. Zhang, Fuzzy-based multi-objective design optimization of buried pipelines, Int. J. Uncertain. Fuzz., 29 (2021), 209–229. https://doi.org/10.1142/S0218488521500104 doi: 10.1142/S0218488521500104
![]() |
[150] | E. Untiedt. A Parametrized Model for Optimization with Mixed Fuzzy and Possibilistic Uncertainty, Springer, Berlin, 2010. https://doi.org/10.1007/978-3-642-13935-2_9 |
[151] |
X. Liu, Z. Zhang, L. Yin, A multi-objective optimization method for uncertain structures based on nonlinear interval number programming method, Mech. Based Des. Struct. Mach., 45 (2017), 25–42. https://doi.org/10.1080/15397734.2016.1141365 doi: 10.1080/15397734.2016.1141365
![]() |
[152] | T. Xie, Q. Zhou, J. Hu, L. Shu, P. Jiang, A sequential multi-objective robust optimization approach under interval uncertainty based on support vector machines, in 2017 IEEE International Conference on Industrial Engineering and Engineering Management (IEEM), 2017, 2088–2092. https://doi.org/10.1109/IEEM.2017.8290260 |
[153] |
H. Chagraoui, M. Soula, M. Guedri, Robust multi-objective collaborative optimization of complex structures. Adv. Acous. Vib., 5 (2017), 247–258. https://doi.org/10.1007/978-3-319-41459-1_24 doi: 10.1007/978-3-319-41459-1_24
![]() |
[154] | F. Li, G. Li, G. Sun, Z. Luo, Z. Zhang, Multi-disciplinary optimization for multi-objective uncertainty design of thin walled beams, CMC-Comput. Mater. Con., 19 (2010), 37–56. http://hdl.handle.net/10453/22026 |
[155] | X. Zhang, W. Sun, J. Huo, X. Ding, Interval multi-objective optimization design based on physical programming, Przeglad Elektrotechiczhy, 88 (2012), 379–381. |
[156] | J. Cheng, G. Duan, Z. Liu, X. Li, Y. Feng, X. Chen, Interval multiobjective optimization of structures based on radial basis function, interval analysis, and NSGA-Ⅱ, J. Zhejiang Univ-Sci. A, 15 (2014), 774–788. https://doi.org/10.1631/jzus.A1300311 |
[157] |
X. Feng, J. Wu, Y. Zhang, M. Jiang, Suspension kinematic/compliance uncertain optimization using a chebyshev polynomial approach, SAE Int. J. Mater. Manu., 8 (2015), 257–262. https://doi.org/10.4271/2015-01-0432 doi: 10.4271/2015-01-0432
![]() |
[158] | X. Li, C. Jiang, X. Han, An uncertainty multi-objective optimization based on interval analysis and its application, China Mech. Eng., 22 (2011), 1100–1106. http://www.cmemo.org.cn/EN/Y2011/V22/I9/1100 |
[159] |
C. Jiang, H. C. Xie, Z. G. Zhang, X. Han, A new interval optimization method considering tolerance design, Eng. Optimiz., 47 (2015), 1637–1650. https://doi.org/10.1080/0305215X.2014.982632 doi: 10.1080/0305215X.2014.982632
![]() |
1. | Arijit Roy, Somnath Bhattacharjee, Soumyajit Podder, Advaita Ghosh, Measurement of bioimpedance and application of Cole model to study the effect of moisturizing cream on human skin, 2020, 7, 2377-9098, 362, 10.3934/biophy.2020025 | |
2. | Amalia C. Nur’aidha, Dhananjaya Y.H Kumarajati, Experimental Study of Electrode Design and Configuration for Bioimpedance Measurement, 2021, 1823, 1742-6588, 012009, 10.1088/1742-6596/1823/1/012009 | |
3. | Rhea Patel, Madhuri Vinchurkar, Rajul Patkar, Gopal Pranjale, Maryam Shojaei Baghini, 2021, Impedance Based Biosensor for Agricultural Pathogen Detection, 978-1-6654-4156-8, 385, 10.1109/NANO51122.2021.9514277 | |
4. | Valerii Kryvonosov, Nataliia Prudnikova, Lilia Martyniuk, Justification of the electrical scheme of biological tissue replacementunder the action of DC voltage, 2022, 13, 26631342, 10.31548/machenergy.13(4).2022.60-71 | |
5. | Jirat Chimnoy, Khunanon Karawanich, Pipat Prommee, 2024, Low-Cost Technique for Extracting the Cole-Cole Model Parameters, 979-8-3503-8155-9, 1, 10.1109/ECTI-CON60892.2024.10594987 | |
6. | Patricia Vazquez-Guerrero, Rohisha Tuladhar, Costas Psychalinos, Ahmed Elwakil, Maurice J. Chacron, Fidel Santamaria, Fractional order memcapacitive neuromorphic elements reproduce and predict neuronal function, 2024, 14, 2045-2322, 10.1038/s41598-024-55784-1 | |
7. | Md. Tushar Abdullah, Chitra Roy, Md. Ibrahim Al Imran, 2024, Exploring Age-Related Changes in Bioimpedance across Diverse Biological Samples, 979-8-3503-5549-9, 1, 10.1109/ICISET62123.2024.10939557 |
Sample | Species/Description | Designation of Measurement |
Apple-1 | Apple-1 and Apple-2 are of same species (Green Granny Smith) and both are purchased from same local shop. Apple-1 and Apple-2 can be considered to be biologically identical. | A11, A12, A13, A14, A15 |
Apple-2 | A21, A22, A23, A24, A25 | |
Apple-3 | Apple-3 (Red Washington) is of different species that of Apple-1 and Apple-2. Apple-3 is biologically different from Apple-1 and Apple-2. | A31, A32, A33, A34, A35 |
Potato-1 | Potato-1 and Potato-2 are of same species (Kufri Chandramukhi) and both are purchased from same local shop. Potato-1 and Potato-2 can be considered to be biologically identical. | P11, P12, P13, P14, P15 |
Potato-2 | P21, P22, P23, P24, P25 | |
Potato-3 | Potato-3 (Kufri Jyoti) is of different species that of Potato-1 and Potato-2. Potato-3 is biologically different from Potato-1 and Potato-2. | P31, P32, P33, P34, P35 |
Remarks: In this table, Amn (or Pmn) represents impedance measurement of Apple-m (or Potato-m) at position n.
Sample | R0 (kΩ) |
R∞ (kΩ) |
α | C (nF secα−1) |
τ (µsec) |
Regression coefficient |
12.93 | 0.64 | 0.719 | 76.65 | 63 | 0.9989 | |
14.21 | 0.74 | 0.703 | 88.30 | 70 | 0.9987 | |
Apple-1 | 13.58 | 0.77 | 0.691 | 99.99 | 65 | 0.9983 |
13.75 | 0.65 | 0.705 | 91.55 | 72 | 0.9989 | |
12.12 | 0.63 | 0.692 | 103.83 | 60 | 0.9983 | |
12.83 | 0.61 | 0.694 | 98.25 | 63 | 0.9980 | |
13.67 | 0.70 | 0.708 | 86.55 | 69 | 0.9985 | |
Apple-2 | 13.90 | 0.72 | 0.696 | 103.47 | 76 | 0.9985 |
12.47 | 0.67 | 0.693 | 112.05 | 70 | 0.9983 | |
10.97 | 0.70 | 0.694 | 113.46 | 59 | 0.9984 | |
11.85 | 0.83 | 0.745 | 62.35 | 57 | 0.9993 | |
10.53 | 0.77 | 0.747 | 64.20 | 52 | 0.9991 | |
Apple-3 | 11.35 | 0.84 | 0.754 | 55.15 | 51 | 0.9994 |
13.75 | 0.84 | 0.767 | 47.47 | 65 | 0.9997 | |
11.74 | 0.84 | 0.751 | 56.65 | 53 | 0.9993 |
Remarks: Apple-1&Apple-2 are of same species and are taken from same lot. While Apple-3 is different from the other two.
Sample | Factor | P-value | F-value | Fcritical | Distinguishability | Level of Confidence |
Apple-1 & Apple-2 | Position | 0.119 | 3.627 | 15.977 | No | 99% |
Sample | 0.594 | 0.334 | 21.197 | No | ||
Apple-1 & Apple-3 | Position | 0.146 | 3.153 | 10.899 | No | 98% |
Sample | 0.017 | 15.384 | 14.039 | Yes |
Sample | R0 (kΩ) |
R∞ (kΩ) |
α | C (nF secα−1) |
τ (µsec) | Regression coefficient |
5.17 | 0.14 | 0.6400 | 341 | 48 | 0.9888 | |
5.48 | 0.16 | 0.7000 | 204 | 41 | 0.9956 | |
Potato-1 | 5.58 | 0.13 | 0.6638 | 246 | 47 | 0.9920 |
5.65 | 0.12 | 0.6505 | 325 | 60 | 0.9922 | |
5.09 | 0.11 | 0.6797 | 243 | 51 | 0.9951 | |
5.14 | 0.12 | 0.6275 | 445 | 60 | 0.9897 | |
5.43 | 0.13 | 0.6532 | 286 | 48 | 0.9918 | |
Potato-2 | 6.66 | 0.16 | 0.6077 | 450 | 68 | 0.9890 |
4.70 | 0.12 | 0.6415 | 363 | 47 | 0.9891 | |
5.67 | 0.12 | 0.5979 | 580 | 68 | 0.9865 | |
5.52 | 0.12 | 0.6360 | 420 | 70 | 0.9934 | |
5.57 | 0.15 | 0.6099 | 610 | 68 | 0.9906 | |
Potato-3 | 6.70 | 0.14 | 0.6202 | 432 | 78 | 0.9912 |
5.86 | 0.11 | 0.6362 | 393 | 69 | 0.9916 | |
5.46 | 0.13 | 0.6436 | 349 | 57 | 0.9924 |
Remarks: Potato -1 & Potato -2 are of same species and are taken from same lot, while Potato -3 is different from the other two.
Sample | Factor | P-value | F-value | Fcritical | Distinguishability | Level of Confidence |
Potato-1 & Potato-2 | Position | 0.6046 | 0.7537 | 15.977 | No | 99% |
Sample | 0.2124 | 2.1975 | 21.198 | No | ||
Potato-1& Potato-3 | Position | 0.6201 | 0.7218 | 10.899 | No | 98% |
Sample | 0.0182 | 14.856 | 14.040 | Yes |
Sample | Species/Description | Designation of Measurement |
Apple-1 | Apple-1 and Apple-2 are of same species (Green Granny Smith) and both are purchased from same local shop. Apple-1 and Apple-2 can be considered to be biologically identical. | A11, A12, A13, A14, A15 |
Apple-2 | A21, A22, A23, A24, A25 | |
Apple-3 | Apple-3 (Red Washington) is of different species that of Apple-1 and Apple-2. Apple-3 is biologically different from Apple-1 and Apple-2. | A31, A32, A33, A34, A35 |
Potato-1 | Potato-1 and Potato-2 are of same species (Kufri Chandramukhi) and both are purchased from same local shop. Potato-1 and Potato-2 can be considered to be biologically identical. | P11, P12, P13, P14, P15 |
Potato-2 | P21, P22, P23, P24, P25 | |
Potato-3 | Potato-3 (Kufri Jyoti) is of different species that of Potato-1 and Potato-2. Potato-3 is biologically different from Potato-1 and Potato-2. | P31, P32, P33, P34, P35 |
Sample | R0 (kΩ) |
R∞ (kΩ) |
α | C (nF secα−1) |
τ (µsec) |
Regression coefficient |
12.93 | 0.64 | 0.719 | 76.65 | 63 | 0.9989 | |
14.21 | 0.74 | 0.703 | 88.30 | 70 | 0.9987 | |
Apple-1 | 13.58 | 0.77 | 0.691 | 99.99 | 65 | 0.9983 |
13.75 | 0.65 | 0.705 | 91.55 | 72 | 0.9989 | |
12.12 | 0.63 | 0.692 | 103.83 | 60 | 0.9983 | |
12.83 | 0.61 | 0.694 | 98.25 | 63 | 0.9980 | |
13.67 | 0.70 | 0.708 | 86.55 | 69 | 0.9985 | |
Apple-2 | 13.90 | 0.72 | 0.696 | 103.47 | 76 | 0.9985 |
12.47 | 0.67 | 0.693 | 112.05 | 70 | 0.9983 | |
10.97 | 0.70 | 0.694 | 113.46 | 59 | 0.9984 | |
11.85 | 0.83 | 0.745 | 62.35 | 57 | 0.9993 | |
10.53 | 0.77 | 0.747 | 64.20 | 52 | 0.9991 | |
Apple-3 | 11.35 | 0.84 | 0.754 | 55.15 | 51 | 0.9994 |
13.75 | 0.84 | 0.767 | 47.47 | 65 | 0.9997 | |
11.74 | 0.84 | 0.751 | 56.65 | 53 | 0.9993 |
Sample | Factor | P-value | F-value | Fcritical | Distinguishability | Level of Confidence |
Apple-1 & Apple-2 | Position | 0.119 | 3.627 | 15.977 | No | 99% |
Sample | 0.594 | 0.334 | 21.197 | No | ||
Apple-1 & Apple-3 | Position | 0.146 | 3.153 | 10.899 | No | 98% |
Sample | 0.017 | 15.384 | 14.039 | Yes |
Sample | R0 (kΩ) |
R∞ (kΩ) |
α | C (nF secα−1) |
τ (µsec) | Regression coefficient |
5.17 | 0.14 | 0.6400 | 341 | 48 | 0.9888 | |
5.48 | 0.16 | 0.7000 | 204 | 41 | 0.9956 | |
Potato-1 | 5.58 | 0.13 | 0.6638 | 246 | 47 | 0.9920 |
5.65 | 0.12 | 0.6505 | 325 | 60 | 0.9922 | |
5.09 | 0.11 | 0.6797 | 243 | 51 | 0.9951 | |
5.14 | 0.12 | 0.6275 | 445 | 60 | 0.9897 | |
5.43 | 0.13 | 0.6532 | 286 | 48 | 0.9918 | |
Potato-2 | 6.66 | 0.16 | 0.6077 | 450 | 68 | 0.9890 |
4.70 | 0.12 | 0.6415 | 363 | 47 | 0.9891 | |
5.67 | 0.12 | 0.5979 | 580 | 68 | 0.9865 | |
5.52 | 0.12 | 0.6360 | 420 | 70 | 0.9934 | |
5.57 | 0.15 | 0.6099 | 610 | 68 | 0.9906 | |
Potato-3 | 6.70 | 0.14 | 0.6202 | 432 | 78 | 0.9912 |
5.86 | 0.11 | 0.6362 | 393 | 69 | 0.9916 | |
5.46 | 0.13 | 0.6436 | 349 | 57 | 0.9924 |
Sample | Factor | P-value | F-value | Fcritical | Distinguishability | Level of Confidence |
Potato-1 & Potato-2 | Position | 0.6046 | 0.7537 | 15.977 | No | 99% |
Sample | 0.2124 | 2.1975 | 21.198 | No | ||
Potato-1& Potato-3 | Position | 0.6201 | 0.7218 | 10.899 | No | 98% |
Sample | 0.0182 | 14.856 | 14.040 | Yes |