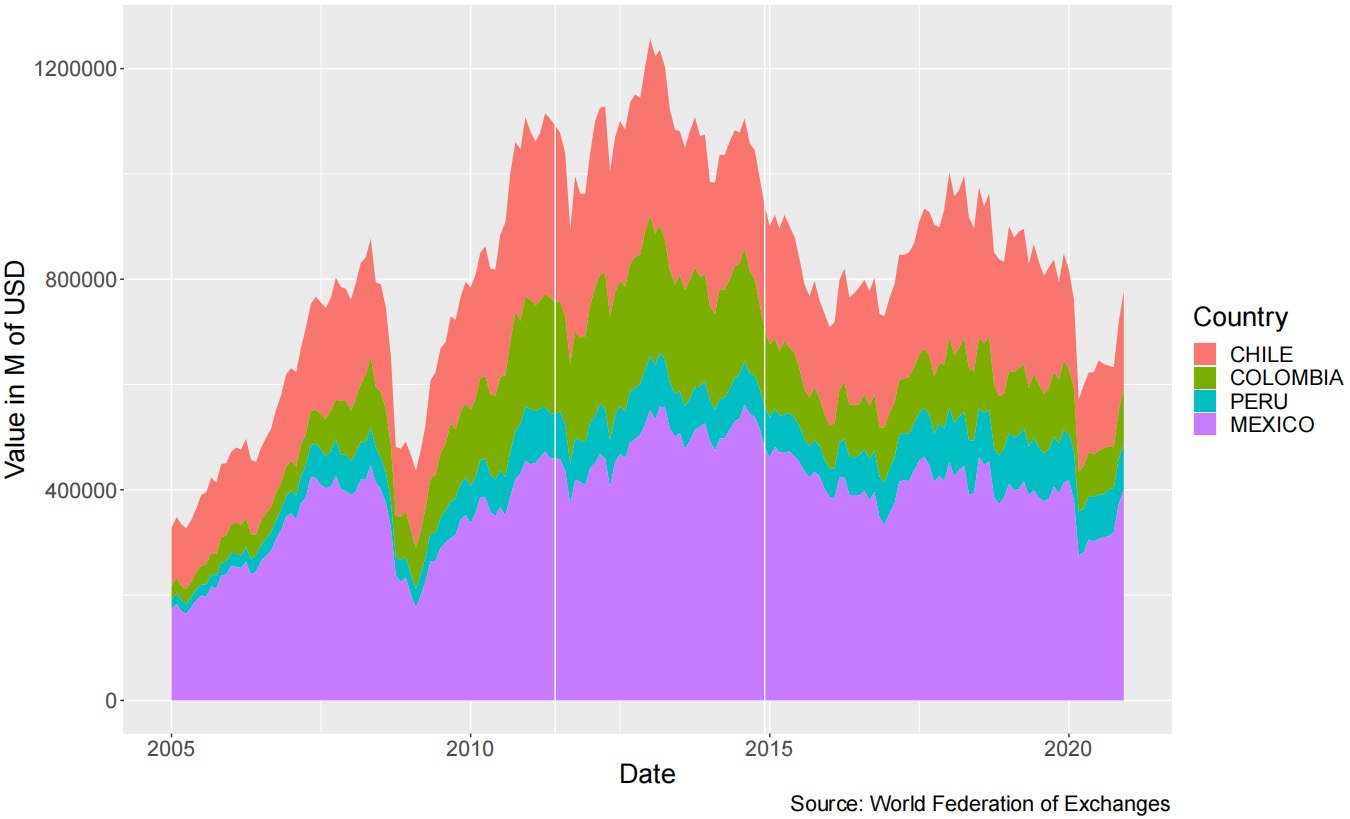
The FeldsteinHorioka1980 study on investment flows through the correlation of domestic saving and investment concluded that liberalization of capital markets does not necessarily lead to a movement of capital looking for a better allocation of resources, as classical theory would suggest. Ever since, literature has been prolific regarding this "puzzle", with arguments for and against this conclusion. This paper aims to analyze the issue from a different perspective. In recent years, the stock markets of Chile, Colombia, Mexico and Peru joined the Latin American Integrated Market through an agreement that allows investors in any of the participating markets to invest in the others as if they were investing locally. Compositional methods are used to assess the hypothesis of a potential flow of capital between markets generated by the creation of the joint market. First, cross-sectional methods for compositional data were used to test the hypothesis. As a result, it was not possible to find a change in the composition of the investment in the four markets produced by the creation of the joint market. Secondly, vector autoregressive models were estimated and tested for structural breaks in the parameters. However, these models were not found to be informative. In conclusion, it was not possible to reject the Felstein-Horioka hypothesis, supporting the idea that liberalization is not enough to generate capital flows between markets.
Citation: Juan David Vega Baquero, Miguel Santolino. Capital flows in integrated capital markets: MILA case[J]. Quantitative Finance and Economics, 2022, 6(4): 622-639. doi: 10.3934/QFE.2022027
[1] | Md Qamruzzaman, Jianguo Wei . Do financial inclusion, stock market development attract foreign capital flows in developing economy: a panel data investigation. Quantitative Finance and Economics, 2019, 3(1): 88-108. doi: 10.3934/QFE.2019.1.88 |
[2] | Cunyi Yang, Li Chen, Bin Mo . The spillover effect of international monetary policy on China's financial market. Quantitative Finance and Economics, 2023, 7(4): 508-537. doi: 10.3934/QFE.2023026 |
[3] | Mustafa Tevfik Kartal, Özer Depren, Serpil Kılıç Depren . The determinants of main stock exchange index changes in emerging countries: evidence from Turkey in COVID-19 pandemic age. Quantitative Finance and Economics, 2020, 4(4): 526-541. doi: 10.3934/QFE.2020025 |
[4] | Dumisani Pamba, Sophia Mukorera, Peter Moores-Pitt . The asymmetric effects of cross-border equity flow volatility on equity market returns in SANEK countries. Quantitative Finance and Economics, 2025, 9(1): 40-75. doi: 10.3934/QFE.2025002 |
[5] | Muath Asmar, Susanne Trimbath . Regulatory reform and trade settlement failures in USA equity markets: Does regulatory reform matter?. Quantitative Finance and Economics, 2022, 6(4): 537-552. doi: 10.3934/QFE.2022023 |
[6] | Fisnik Morina, Simon Grima . The impact of pension fund assets on economic growth in transition countries, emerging economies, and developed countries. Quantitative Finance and Economics, 2022, 6(3): 459-504. doi: 10.3934/QFE.2022020 |
[7] | Sofía Orazi, Lisana B. Martinez, Hernán P. Vigier . Determinants and evolution of financial inclusion in Latin America: A demand side analysis. Quantitative Finance and Economics, 2023, 7(2): 187-206. doi: 10.3934/QFE.2023010 |
[8] | Md Akther Uddin, Mohammad Enamul Hoque, Md Hakim Ali . International economic policy uncertainty and stock market returns of Bangladesh: evidence from linear and nonlinear model. Quantitative Finance and Economics, 2020, 4(2): 236-251. doi: 10.3934/QFE.2020011 |
[9] | Samuel Asante Gyamerah . Modelling the volatility of Bitcoin returns using GARCH models. Quantitative Finance and Economics, 2019, 3(4): 739-753. doi: 10.3934/QFE.2019.4.739 |
[10] | Anouar Ben Mabrouk . Wavelet-based systematic risk estimation: application on GCC stock markets: the Saudi Arabia case. Quantitative Finance and Economics, 2020, 4(4): 542-595. doi: 10.3934/QFE.2020026 |
The FeldsteinHorioka1980 study on investment flows through the correlation of domestic saving and investment concluded that liberalization of capital markets does not necessarily lead to a movement of capital looking for a better allocation of resources, as classical theory would suggest. Ever since, literature has been prolific regarding this "puzzle", with arguments for and against this conclusion. This paper aims to analyze the issue from a different perspective. In recent years, the stock markets of Chile, Colombia, Mexico and Peru joined the Latin American Integrated Market through an agreement that allows investors in any of the participating markets to invest in the others as if they were investing locally. Compositional methods are used to assess the hypothesis of a potential flow of capital between markets generated by the creation of the joint market. First, cross-sectional methods for compositional data were used to test the hypothesis. As a result, it was not possible to find a change in the composition of the investment in the four markets produced by the creation of the joint market. Secondly, vector autoregressive models were estimated and tested for structural breaks in the parameters. However, these models were not found to be informative. In conclusion, it was not possible to reject the Felstein-Horioka hypothesis, supporting the idea that liberalization is not enough to generate capital flows between markets.
The findings of Feldstein and Horioka (1980) marked the beginning of a discussion in economics: Does the liberalization of capital markets generate net transfers of financial capital between countries? Classical theory would say yes, but the authors concluded that the data do not agree. In particular, they used data on 21 Organisation for Economic Co-operation and Development (OECD) countries for the period 1960–1974 to assess the relationship between domestic saving and domestic investment. The idea behind is that with perfect mobility of capital, there should be no correlation between these two variables since the investment decisions would respond to the opportunities in the global market. Indeed, they found that "international differences in domestic savings rates among major industrial countries have corresponded to almost equal differences in domestic investment rates" (Feldstein and Horioka, 1980, p. 328). As explained later by Ford and Horioka (2017), the liberalization of capital markets is not enough to generate net transfers of financial capital between countries, so the integration of goods markets is also necessary to compensate the transfers of capital.
Following these findings, the literature on the matter has been prolific, trying to argue both, i.e., in favor and against the conclusions drawn. For instance, Narayan (2005) used a cointegration approach to verify whether saving and investment were correlated in China between the 1950s and the 1990s, concluding that, due to the fixed exchange rate regime operating during the largest part of the period, the Feldstein-Horioka (F-H) puzzle holds and the correlation between savings and investment is high. On the other hand, Fouquau et al. (2008) made use of panel threshold regression models to assess the impact of other variables on the relationship between savings and investment, finding that openness, country size and current account size have an influence.
Conversely, Coakley J, Kulasi F, Smith S (1996) tried to explain the correlation between savings and investment through the use of a theoretical model in which the correlation is due to the cointegration of the variables, as explained in the stationarity of the current account balance (since the current account is equal to savings minus investment). Furthermore, Drakos et al. (2017) analyzed the relationship between savings and investment from long-term and short-term perspectives, seeking for an explanation of the high correlation between the variables; they found that this is consistent with the existence of solvency constraints in the long run.
More specifically, in Latin America, Bellod-Redondo (1996) analyzed the capital flows in Mexico before and after the integration into the North Atlantic Free Trade Agreement (NAFTA), concluding that saving rates limit the investment. Another enquiry on the topic for Latin American countries was conducted by Sinha and Sinha (1998), who used a cointegration test to determine whether this can be the actual explanation for the high correlation between savings and investment and found that, for some of the countries in the sample, there was a long-term relationship between the two variables, while, for others, there was not; this coincided with high macroeconomic instability. More recently, Ibarra-Yunez (2008) tried to verify the F–H puzzle in the region, finding that, despite the opening of the economies during the recent years, there is no evidence of capital movements.
Additionally, there is extensive research on spillovers and connections in financial markets. Some of the most recent approaches include those by Chen and Dong (2020), Dong et al. (2020) and Jia et al. (2021).
At this point in the discussion, the aim of this paper is to use a more specific setting to assess the movement of capital in fully integrated markets. More precisely, this approach does not enquire into the overall investment of a country, as it focuses on the portfolio investment and will analyze flows in the Latin American Integrated Market (MILA), which is an agreement between four markets (Chile, Colombia, Mexico and Peru), that allows perfect mobility between them. The latter considers that this type of investment can be less subject to restrictions and less dependent on other types of international transfers, such as those in goods and services markets.
For this purpose, the participation of the four markets that form the MILA has been assessed over time, trying to find a change in the structure of the market generated by the entrance in force of the agreement. Since the assessment focuses on the participation, compositional methods are a natural approach to the problem. These methods have been widely developed in the recent decades, but applications in economics remain rare1. Therefore, this is also an opportunity to employ novel methods and contribute to the debate around the F-H puzzle.
1See, for example, Belles-Sampera et al. (2016), who studied capital allocation problems using the compositional framework, or Boonen et al. (2019), who forecasted risk allocations through compositional data.
In this case, there are two options: first, data can be treated as cross-sectional, applying compositional models and measures of distance to assess the hypothesis; second, treat data as time series, using these models to search for a change in the dynamics of the market structure. Therefore, both perspectives are used to verify the F-H puzzle, and the results are evaluated to determine which provides better insight into the problem.
The paper is structured as follows. Section 2 provides insight into the MILA market and its participating countries. Section 3 explains the methodology used to assess the F-H hypothesis, and Section 4 presents the dataset. Finally, Section 5 shows the main results of the evaluation of the F-H puzzle and Section 6 concludes the study.
The MILA is an agreement signed by the stock exchanges from Chile, Colombia and Peru, which started operating as an interconnected market in 2011. By the end of 2014, Mexico joined the three founding members to form the current MILA. The four countries from the Pacific Alliance (a Latin American trading bloc) signed the agreement which allows investors from any of the participating markets to invest in stocks from any of the exchanges. By the end of 2020, the market operated with more than 700 listed companies and had a capitalization above USD 770 billion. Operationally, the instruments are kept in the four separated exchanges, but they are interconnected for investors to be able to trade in any of the markets. A short anecdotal review of the history of each one of the markets is presented below in order to understand the different backgrounds that led to the foundation of the current MILA.
The Bolsa de Comercio de Santiago was founded in 18932, working during several years in parallel with the Bolsa de Corredores de Valparaíso. During part of the 20th century, the latter was the main stock market in Chile because of the important economic activity taking place in Valparaíso, the country's main port. Nevertheless, as the years passed, and due to several regulatory changes, the Bolsa de Comercio de Santiago became the most important stock market in Chile. Since 1908, investors in both exchanges had the possibility to trade stocks in both markets through Inter Exchange Operations. In 2005, the two exchanges, together with the Bolsa Electrónica (founded in 1989), signed agreements to allow investors to trade stocks in all markets. In 2018, the Bolsa de Corredores de Valparaíso ceased operations.
2https://www.bolsadesantiago.com.
As part of the participation in international entities and the alliances with other stock markets around the world, the Bolsa de Comercio de Santiago joined, as a founding member, the Ibero-American Federation of Stock Exchanges and Securities Markets in 1973 and, in 1991, the World Federation of Exchanges. In 2000, the Mercado de Valores Extranjeros (Foreign Stock Exchange) was created as a platform for local investors to trade foreign stocks. Finally, in 2011, the Bolsa de Comercio de Santiago joined the MILA, creating the biggest stock market in the region.
The Bolsa de Valores de Colombia3 was born in 2001 after the fusion of the Bolsa de Bogota (created in 1928), the Bolsa de Medellin (created in 1961) and the Bolsa de Occidente (created in 1983). Since the creation of the Bolsa de Bogota, the trading of stocks in the country has been closely linked to the performance of the business activity. Indeed, following the economic instability after the Great Depression, the first years of the trading activity were slow and the strongly restrictive legal frame during the following decades did not allow for important growth of the trading activity. It was not until the creation of the Bolsa de Medellin that the stock markets started gaining some dynamism, paired with the important growth in the economic activity during the 1960s. This led to the creation of the Bolsa de Occidente a few years later.
The technological improvements achieved in the 1990s helped the three markets experience an increase in their activity. Starting in the 2000s, the aim of the three markets to attract more international investment led to their fusion and the creation of the current Bolsa de Valores de Colombia.
After several years of informal trading, in 1894, the Bolsa Nacional was born in Mexico City4. A few months later, another stock exchange, the Bolsa de Mexico, started operating in the same city. Later, both exchanges found that it was more beneficial to work together and decided to merge under the name Bolsa de Mexico. In 1933, with the new legislation about the stock exchanges, the Bolsa de Valores de México was created as the sole exchange in the country. In the 1950s, the Bolsa de Monterrey and the Bolsa de Occidente (in Guadalajara) were created to stimulate the economic activity in those regions. In 1975, the new legislation on capital markets in Mexico led to the merge of all stock exchanges into the new Bolsa Mexicana de Valores.
By the end of the 1980s, and through the 1990s, the Bolsa Mexicana de Valores gained considerable dynamism due to the electronic negotiation, the incursion of international stockbrokers and an important increase in the number of listed companies. Additionally, in 2003, it started the investment of local agents in international stocks, continuing the internationalization of the Mexican stock market. In 2014, the Bolsa Mexicana de Valores joined the Latin American Integrated Market (MILA), after several years of technical adjustments.
The Bolsa de Comercio de Lima started operating in 18615. For several years, there were no shares from private companies traded in the stock exchange, but it was creating valuations for them. After the high inflation period in the 1870s, the stock market regained some dynamism. The name was changed to Bolsa Comercial de Lima in 1898, with an increased offer for investors and changes in the benefits for traders and other agents in the market. The beginning of the 20th century brought important growth in the trading activity of the Peruvian stock market, which was stopped by the uncertainty generated by the Great Depression and World War II. This led to large reforms and the creation of the new Bolsa de Comercio de Lima in 1951.
At the beginning of the 1960s, the hundredth anniversary of the Peruvian stock market was a transition period in which the main objective was to strengthen the market and increase capitalization by incorporating new firms. This growth period led to further reforms and the creation of the current Bolsa de Valores de Lima (BVL) in 1971. During the following years, the BVL experienced important dynamism, marked by the incorporation of new technologies, it then became one of the most profitable stock markets in the world by the beginning of the 1990s. In 1995, the BVL started operating electronically, and from 2002, the market information was available for all users online, increasing the transparency and efficiency of the market. In 2011, the BVL joined the MILA with Chile and Colombia, with the aim of increasing the investment possibilities for all agents. In recent years, more innovations have come to the market, such as the creation, in 2013, of the Mercado Alternativo de Valores (Alternative stocks market), which is for the negotiation of shares from smaller companies, the incorporation of new indices and a remarkable internationalization strategy.
The four stock markets that form the MILA have important participation in the company shares, although private and public bonds, derivatives and currencies are also traded. Regarding the main sectors trading in the exchanges, it is worth noting that all of them have important participation in the financial services, energy and mining and food and beverage companies within the most traded stocks.
Additionally, the Bolsa de Comercio de Santiago has important companies in sectors such as retail, real state and transportation services, while the Bolsa de Valores de Colombia has important public services firms participating in the market. On the other hand, the Bolsa Mexicana de Valores combines public services and transportation enterprises in the main traded shares, and the Bolsa de Valores de Lima has retail and industrial companies among the most relevant in the exchange market.
Although each stock market continues to have its own indices, financial services firms like the S & P Dow Jones Indices6 carry indices of the joint market, such as the S & P MILA Pacific Alliance Select, which includes the largest, most liquid companies of the MILA; or the S & P MILA Andean 40, which includes the 40 largest and most liquid stocks in Chile, Colombia and Peru.
6https://www.spglobal.com/spdji.
This section is dedicated to introducing the methodology used through the paper. The compositional data framework is explained in the first subsection. Later, the cross-sectional methods with compositional data are shown in the second subsection. After, considering that the data corresponds to a multivariate time series, this framework is explained in the third subsection and the series are modeled by means of vector autoregressive (VAR) models comparing the traditional and the compositional data approaches. Throughout the analysis, the focus is on assessing whether there was a change in the composition of the MILA market in June 2011 (when the markets of Chile, Colombia and Peru created the joint market) or in December 2014 (when Mexico joined the three founding members). The dataset (explained in more detail in Section 4) includes monthly observations from January 2005 to December 2020, meaning that there are observations before and after these breaking points.
A composition is a multivariate series in which the variables carry only relative information (Pawlowsky-Glahn et al., 2011). It can be expressed as a vector Y=[y1,...,yn], where each yj is a non-negative value and ∑nj=1yj=κ. The value of κ is usually normalized to the unit (κ=1). Formally, compositional data are defined in the sample space described by
Sn={Y∈Rn|yj≥0,j=1,...,n,n∑j=1yj=1} | (1) |
The characteristics of Sn impose several restrictions at the moment of modeling the series. Therefore, Aitchison J (1986) defined what is referred to as the Aitchison geometry. This includes transformations to express compositional series (defined in the sample space Sn) in Rn and then being able to apply conventional statistical techniques to the transformed series. For instance, the Aitchison geometry defines the centered log ratio (clr) transformation as
clr(Y)=[lny1g(Y),...,lnyng(Y)]; with g(Y)=(n∏j=1yj)1/n | (2) |
This transformation allows only for compositions with strictly positive values (yj>0), which imposes a limitation on the composition. Further discussion on the topic can be found in Aitchison J (1986). On the other hand, even if the transformed elements can (in theory) take any value in R, they still have the limitation of adding up to zero because of the construction of the transformation. Therefore, the isometric log ratio (ilr) transformation is defined in the Aitchison geometry. In this case, an orthonormal basis e={e1,...,en−1} is used to create the matrix V with rows equal to clr(ej) such that V⋅V′=In−1 and V′⋅V=In−(1/n)1′n1n, with In being the identity matrix of size n and 1n, an n-row vector of ones. Then, the irl transformation is defined as
ilr(Y)=clr(Y)⋅V′ | (3) |
As a result, the size of ilr(Y) will be n−1. For the specification of e, the binary partitions approach followed by Egozcue et al. (2003) can be used. The method assigns +1 to part of the elements in the compositions and –1 to the others. In the next step, one of these subgroups is taken and again divided into two groups, while the other is assigned zeros. This process is done subsequently, creating two subgroups at each step until all subgroups contain only one element. The result is an (n−1)×n matrix with +1, -1 and 0 as elements, and it can be used as the orthonormal basis for the matrix V.
Throughout this paper, the composition Y corresponds to the composition of the MILA market at each time t in the sample, which is explained in more detail in Section 4. Therefore, the size n of the composition is 4, while the size of the transformed series ilr(Y) is 3.
The first approach is to analyze the compositional series as cross-sectional data. Therefore, it is possible to estimate a mixture model adjusted for compositional data as explained by Comas-Cufí et al. (2016). In this case, it is assumed that the dataset follows a linear combination of a finite set of K distributions and has a probability density function (pdf) of the form π1f1(⋅;θ1)+⋯+πKfK(⋅;θK), where θ1,⋯,θK are the parameters of the pdfs f1,⋯,fK, respectively, and π1,⋯,πK are positive numbers with ∑Kk=1πk=1 and the weights of each individual pdf (McLachlan and Peel, 2004). Considering that compositional data are defined in the simplex Sn, the Dirichlet distribution is commonly used for modeling. However, Comas-Cufí et al. (2016) explained that an orthonormal transformation can be used to transform the data so that they can be modeled using distributions defined in the real space. In this case, the ilr transformation defined previously is applied, so a Gaussian distribution can be used and a Gaussian mixture model is estimated. Therefore, in this estimation, each one of the fk distributions corresponds to a Gaussian distribution with the parameters θk=[μk,σk] for k=1,…,K.
The idea behind this approach is that, assuming the data comes from a mixture of K=3 Gaussian distributions (one for each period analyzed), the model should assign the observations to these distributions following the three periods under analysis. If the model assigns the observations to the three distributions in a different fashion, then there is no evidence of a change in the composition of the MILA market due to the entrance in force of the agreement and the F-H puzzle could not be rejected.
On the other hand, it is possible to use different measures of distance between compositions to check whether the entrance in force of the agreement produced a change in the composition of the joint market, meaning that there was a potential movement of capital that would contradict the F-H puzzle. For all three measures of distance, it is calculated for each period Yt with respect to the previous one Yt−1 to see if there was an immediate shift in the composition of the MILA market that would indicate any potential movement of capital at the moment of implementation of the agreement. This was done by comparing the results for the breaking points of the MILA market (detailed in Section 4) with those of the rest of the observations to obtain an idea of a "normal" value of each distance measure. If the variation at the period of interest appears as "atypical", it could indicate a potential flow of capital induced by the entrance in force of the agreement.
The first of these measures is the Aitchison distance for two compositions Yt and Yt−1∈Sn, which has been defined by Aitchison J (1986) as
ADΔ(Yt,Yt−1)=√1nn∑i=1n∑j=1(lnyi,tyj,t−1−lnˆyi,tˆyj,t−1)2 | (4) |
Also, following Thomas and Lovell (2014), it is possible to use measures like the compositional Kullback-Leibler divergence, which measures the difference of two compositions and is defined by Martín-Fernádez et al. (ND) as
KLΔ(Yt,Yt−1)=n∑i=1lnyi,tyi,t−1 | (5) |
Thomas and Lovell (2014) also proposed the cosine similarity, which ranges between –1 (completely opposite vectors) and 1 (completely proportional vectors), with 0 meaning completely orthogonal vectors. The cosine similarity is defined as
CSΔ(Yt,Yt−1)=∑ni=1yi,tyi,t−1√∑ni=1y2i,t√∑ni=1y2i,t−1 | (6) |
Following Fuller (1996), a time series is a real function x(t,ω) for t∈T (time) and ω∈Ω (all possible realizations of the variable). Therefore, x is defined on T×Ω. For a fixed t, x is a random variable on a probability space. On the other hand, for a fixed ω, x is a function of time called a realization or sample function, and it is what can be actually observed in practice. Therefore, each one of the observations in the time series is a random variable itself and has its own distribution. When looking at the distribution of the observations over time (the sample function), it was found to be a joint distribution function of all individual random variables.
With this in mind, a time series is told to be strictly stationary if the joint distribution is the same, independent of the time t. Nevertheless, as mentioned before, in reality, it is only possible to observe one realization of the time series, which makes it impossible to obtain the joint distribution function. Therefore, it is common to consider the weak stationarity of time series by examining only the first two moments of the distribution. Indeed, a time series is said to be weakly stationary if the expected value of x is constant for all t and the covariance matrix is only a function of the distance between the realizations and does not depend on t itself.
In practice, the stationarity of a time series is tested using unit roots tests. The augmented Dickey-Fuller test (Said and Dickey, 1984) assesses the null hypothesis of unit roots in the characteristic equation of the time series, against the alternative of a stationary series. The idea behind this is that all roots of the characteristic equation of a stationary series should lie inside the unit circle.
For stationary time series, it is possible to find a stochastic difference equation of the form
p∑i=0αixt−i=ξt or the equivalent xt=p∑i=1βixt−i+ξ′t | (7) |
where αi is a scalar coefficient with α0≠0 and αp≠0, p is the order of the autoregressive time series, ξ follows a normal distribution with mean zero and variance σξ, N(0,σξ), βi is a coefficient in terms of α and ξ′ follows N(0,σξ′). The latter equation is known as the generic form of an autoregressive process.
The VAR model can be seen as an extension of the autoregressive model defined previously for which the variable x is replaced for a vector X=[x1,...,xn], usually referred to as a multivariate time series. In this case, the vector X comprises the market capitalization for each of the members of the MILA market, meaning that the size is n=4, and it can be observed through the period under analysis, as defined in Section 4. With this in mind, the general form of the VAR model is
Xt=p∑i=1ρiXt−i+ϵt | (8) |
where ρi is a matrix of parameters in Rn×n and ϵt is the error term with multivariate normal distribution with zero means and a covariance matrix Σϵ, Nn(0,Σϵ). However, in real life, many observed series are not stationary. Nevertheless, it is common that the changes in the variable follow a stationary process. In these cases, it is possible to estimate a VAR in differences to model the series. It is defined as
ΔXt=p∑i=1ρiΔXt−i+ϵt | (9) |
where Δ is the difference operator defined as ΔXt=Xt−Xt−1.
The time-series approach can be extended to compositional data. In this case, after applying the ilr transformation, it is possible to use conventional statistical models and estimate a VAR model. Therefore, the generic VAR model for a composition Y (after applying the ilr transformation) is defined as
ilr(Yt)=p∑i=1ϱiilr(Yt−i)+εt | (10) |
Similar to the previous case, if the transformed series is not stationary, then it is possible to differentiate the series by applying the Δ operator, and, if the series in differences is stationary, then the model to estimate would be
Δilr(Yt)=p∑i=1ϱiΔilr(Yt−i)+εt | (11) |
After deciding which models fit better to the data, then it is time to examine whether the F-H puzzle holds for this specific setting. In order to do this, the model will be first estimated for the whole sample (in this case, from January 2005 to December 2020, as explained in more detail in Section 4). Later, the model will be estimated for three different subsamples: before June 2011 (when the MILA market started operating with Chile, Colombia and Peru), from July 2011 to November 2014 and from December 2014 onward (when Mexico joined the MILA). Finally, the coefficients obtained in the four models will be compared in order to see if the entrance in force of the agreement produced a change in the participation of each market in total, meaning that there was a recomposition of the investment induced by the agreement. Thus, if the coefficients of the general model are different from those for the models in the subsamples, this would mean that the dynamics of the series changed and there was a recomposition of the market.
The variable to be used is the monthly market capitalization of each stock market. The data are publicly available from the World Federation of Exchanges and are expressed in US dollars as common currency. The dataset is composed by utilizing the market capitalization of the four markets from January 2005 to December 2020. Figure 1 shows the dataset in levels, while Figure 2 shows the composition formed by the four markets. In both figures, there are two white lines dividing the graph: the first one corresponds to June 2011, when Chile, Colombia and Peru joined the MILA, and the second one to December 2014, when Mexico joined the three founding members and formed the current MILA. Therefore, the analysis will be concentrated on finding differences in the market capitalization of the four markets before and after the entrance in force of the agreement.
The use of this dataset has an important implication: by using a common currency (to be able to compare the data from the four markets), the fluctuations in the exchange rates in each country are disregarded. Therefore, an assumption is required to proceed: the four countries are affected in the same sense by shocks in the currency exchange markets. This means that, if one country suffers devaluation, the others will also go through the same process. To assess this assumption, the exchange rate fluctuations of the four currencies were verified for the period of interest. When checking for their pairwise correlations, all of them were above 0.59, meaning that there is a strong correlation between the exchange rates of all countries against the US dollar, validating the assumption made. However, there is still room for a caveat regarding the potential effects of currency exchange fluctuations in the results.
Figure 3 shows the results of the estimation of the Gaussian mixed model with three distributions. There seem to be two breaking points in the series, but they do not correspond to the entrance in force of the MILA market, which means that it is not possible to find a recomposition of the capital market caused by the agreement. Therefore, it is not possible to reject the F-H puzzle.
Regarding the results of the distance measures, Figure 4 shows that the distance between the composition of the MILA market each period, with respect to the period before, has been close to zero along the whole period under analysis, without any important variation around the moments of implementation of the MILA market.
Likewise, the cosine similarity estimation results are presented in Figure 5. It is possible to see that the values were close to one along the whole period, particularly in the moments of the integration of the markets, which means that there is no evidence of recomposition of the MILA market with the entrance in force of the agreement.
Finally, Figure 6 shows similar results for the compositional Kullback-Leibler divergence. Thus, across the period under analysis, the divergence between the composition of the MILA market for a month and the month before remained close to zero, particularly in the periods of interest when the joint market started operating.
As can be seen, the cross-sectional methods used agree with the F-H puzzle, as none of them have revealed any change in the composition of the market capitalization in the MILA with the entrance in force of the agreement.
To define the (compositional) time-series models to estimate, it is necessary to perform some diagnostic tests in the series. First, the stationarity of the series was tested since it is the first assumption when using time-series techniques. The augmented Dickey-Fuller test assesses the null hypothesis of a unit root in the characteristic equation of the series, which implies that the series is non-stationary. As shown in Table 1, none of the four series of market capitalization showed stationary in levels. After differentiating the series one time, the results of the test show that the series are stationary (Table 1), meaning that the series are integrated of order one and the model to be estimated is the one in Equation 9. In the case of the compositions, the situation is similar. Table 2 reports the results for the ilr transformed series. Recall that the size of ilr(Y) is 3 and there is no direct correspondence with the four countries that form the original composition because of the construction of the transformation. Therefore, the variables are renamed V.1, V.2 and V.3. The test results show that the levels are not stationary, unlike the first differences; so, the model in Equation 11 is the one to estimate.
Levels | First differences | |||
Statistic | P-value | Statistic | P-value | |
Chile | -1.90 | 0.62 | -4.69 | 0.01 |
Colombia | -1.50 | 0.79 | -5.43 | 0.01 |
Peru | -2.69 | 0.29 | -5.16 | 0.01 |
Mexico | -2.63 | 0.31 | -5.31 | 0.01 |
Levels | First differences | |||
Statistic | P-value | Statistic | P-value | |
V.1 | -1.81 | 0.66 | -5.72 | 0.01 |
V.2 | -1.70 | 0.70 | -5.68 | 0.01 |
V.3 | -2.24 | 0.48 | -5.60 | 0.01 |
At this point, it is necessary to determine how many lags are needed in each model. In this case, the information criteria to be used will be the Akaike's information criterion (AIC) and the Bayesian information criterion (BIC). Both use a likelihood method to define the fitness of a model to a sample. The AIC statistic is defined as AIC=−2ℓ+2K, where ℓ is the log-likelihood of the estimation and K is the number of parameters in the model; the BIC is defined as BIC=−2ln(ℓ)+Kln(n), where n is the sample size. In both cases, a lower value of the statistic implies a better fit of the model, although the BIC gives a higher relevance to the parsimony of the model by penalizing more a higher number of parameters.
As shown in Table 3, both the AIC and the BIC conclude that, for the model with the differences of the market capitalization, the more lags added the best. The number of lags was tested to up to 36, and the AIC continued to decrease at each time. Considering that the model should be parsimonious, the chosen number of lags for the estimated model was 12, consistent with the periodicity of the series. Therefore, the model to be estimated is expressed in Equation 12:
ΔXt=12∑i=1ρiΔXt−i+ϵt | (12) |
No. of lags | Market capitalization differences | Ilr transformed series differences | ||
AIC | BIC | AIC | BIC | |
1 | 16085.49 | 16150.43 | -1843.73 | -1804.76 |
2 | 16010.39 | 16127.09 | -1823.78 | -1755.70 |
3 | 15932.34 | 16100.63 | -1803.59 | -1706.50 |
6 | 15728.65 | 16050.69 | -1749.24 | -1565.68 |
12 | 15317.52 | 15942.24 | -1620.94 | -1267.14 |
24 | 14379.77 | 15589.55 | -1481.95 | -799.11 |
36 | 12544.07 | 14309.25 | -1510.65 | -515.45 |
For the case of the compositional model, the results vary substantially. Thus, the information criteria show that the models with only one lag had the best adjustment, meaning that the model to be estimated is the one in Equation 13:
Δilr(Yt)=ϱΔilr(Yt−1)+εt | (13) |
The results for the model in Equation 12 show that almost all coefficients are non-significant except, for a few coefficients in random lags which do not have further interpretation. For instance, in the equation for Chile, only the second lag of Mexico, the third of Peru, the eleventh of Chile and the twelfth of Colombia are significant; in the equation for Colombia, only the eleventh lag of Peru is significant. In the equation for Peru, the significant coefficients are its first and eighth lags; lags 2, 3, 8 and 11 of Chile; Mexico's eighth lag and Colombia's eleventh lag. Finally, in the equation for Mexico, its second lag, Peru's second and ninth lags and Chile's eleventh lag are significant. These results mean that it was not possible to find a dynamic process generating the data of the market capitalization of the MILA market.
This was confirmed by the Granger causality test (Granger, 1969), which shows that none of the variables contain information that can be used to explain the others. Table 4 reports the statistic and p-value results for the test with the null hypothesis that each dependent variable is explained by the others. Furthermore, when estimating the three separated models for the periods under study, the results were found to be very similar to those of the first model, i.e., most of the coefficients were not significantly different from zero. This implies that the model also has little predictive power in the subsamples, and that there is no difference between the three periods studied. The latter can be seen as a confirmation of the F-H puzzle, as there is no change in the coefficients of the model in the periods under analysis.
Dependent variable | Statistic | P-value |
Chile | 1.33 | 0.10 |
Colombia | 1.05 | 0.40 |
Peru | 1.15 | 0.26 |
Mexico | 0.83 | 0.75 |
For the compositional model (Equation 13) the results are similar. Figure 7 reports the coefficient results for the compositional VAR model. In the left panel, V.1 corresponds to the proportion of Chile, Colombia and Peru with respect to Mexico, as explained by the first lags of itself and the other variables. V.2, in the middle, corresponds to the proportion of Chile and Colombia with respect to Peru, as explained by the same variables as before; the right panel shows the estimation results for V.3, which is the proportion of Chile with respect to Colombia.
As can be seen in Figure 7, almost all coefficients were non-significant, except for the equation for V.2, in which its own lag is significant. Also, the separated models for the three periods exhibited a similar result. Furthermore, the Granger test concludes that there is no causality between the variables and the previous observations of the others (Table 5). Furthermore, when comparing the coefficients of the general model for the whole sample and the models for the three subperiods, the results were statistically the same for most of them, which means that it is not possible to find a recomposition of the integrated capital market after the entrance in force of the agreement. This would mean that the F-H puzzle still holds in this specific setting.
Dependent variable | Statistic | P-value |
V.1 | 1.67 | 0.01 |
V.2 | 1.06 | 0.39 |
V.3 | 1.06 | 0.38 |
Different methods were applied to assess the F-H hypothesis, according to which the liberalization of capital markets does not necessarily lead to a movement of capital between countries. The particular case of the MILA was used by considering the specificity of the setting, in which only stock markets were liberalized to allow investors to trade shares in other markets as they would do locally. The hypothesis testing strategy was based on the market capitalization of the MILA market and the relative importance of each of the participants within the total, looking for any change in the composition of the market that could indicate a flow of capital between the stock exchanges. As a result, it is not possible to find a change in the participation of the four markets in the MILA caused by the entrance in force of the agreement. Therefore, there was no indication of capital flows within the markets after the implementation of the agreement, meaning that the F-H puzzle holds also in this specific setting.
From the methodological point of view, it was possible to use compositional methods to assess the hypothesis by analyzing the series as cross-sectional data, achieving consistent results. On the other hand, compositional and traditional time series models did not provide useful information to assess the hypothesis, as the estimated models did not deliver significant results. This sheds light on the potential of compositional methods for analyzing problems for which other approaches fail to come up with meaningful results.
This paper contributes to the debate on the effects of capital markets' liberalization, and its findings can provide policymakers with arguments in favor or against the applications of certain policies, particularly in the field of international investment. Further research on the topic could aim to implement similar methodologies in different settings, such as the European Union, which not only allows for the mobility of capital, but also incorporates other factors, such as labor.
The Spanish Ministry of Science and Innovation supported this study under the grant PID2019-105986GB-C21, as did the Secretaria d'Universitats i Recerca of the Catalan Government, under the grant 2020-PANDE-00074.
All authors declare no conflict of interest in this paper.
[1] | Aitchison J (1986) The statistical analysis of compositional data. Chapman & Hall, London. |
[2] |
Belles-Sampera J, Guillen M, Santolino M (2016) Compositional methods applied to capital allocation problems. J Risk 19: 1–15. https://doi.org/10.21314/JOR.2016.345 doi: 10.21314/JOR.2016.345
![]() |
[3] | Bellod-Redondo JF (1996) Ahorro e inversión en el largo plazo: El caso de la América Latina. El Trimest Econ 43: 1113–1137. |
[4] |
Boonen TJ, Guillen M, Santolino M (2019) Forecasting compositional risk allocations. Insur Math Econ 84: 79–86. https://doi.org/10.1016/j.insmatheco.2018.10.002 doi: 10.1016/j.insmatheco.2018.10.002
![]() |
[5] |
Chen S, Dong H (2020) Dynamic network connectedness of Bitcoin markets: Evidence from realized volatility. Front Phys 8: 582817. https://doi.org/10.3389/fphy.2020.582817 doi: 10.3389/fphy.2020.582817
![]() |
[6] |
Coakley J, Kulasi F, Smith S (1996) Current account solvency and the Feldstein-Horioka puzzle. Econ J 106: 620–627. https://doi.org/10.2307/2235567 doi: 10.2307/2235567
![]() |
[7] | Comas-Cufí M, Martín-Fernández JA, Mateu-Figueras (2016) Log-ratio methods in mixture models for compositional data sets. SORT-STAT OPER RES T 1: 349–374. |
[8] |
Dong H, Chen L, Zhang X, et al. (2020) The asymmetric effect of volatility spillover in global virtual financial asset markets: The case of Bitcoin. Emerg Mark Finance Trade 56: 1293–1311. https://doi.org/10.1080/1540496X.2019.1671819 doi: 10.1080/1540496X.2019.1671819
![]() |
[9] |
Drakos AA, Kouretas GP, Stavroyiannis S, et al. (2017) Is the Feldstein-Horioka puzzle still with us? national saving-investment dynamics and international capital mobility: A panel data analysis across EU member countries. J INT FINANC MARK I 47: 76–88. https://doi.org/10.1016/j.intfin.2016.11.006 doi: 10.1016/j.intfin.2016.11.006
![]() |
[10] |
Egozcue J, Pawlowsky-Glahn V, Mateu-Figueras G, et al. (2003) Isometric logratio transformations for compositional data analysis. Math Geol 35: 279–300. https://doi.org/10.1023/A:1023818214614 doi: 10.1023/A:1023818214614
![]() |
[11] |
Feldstein M, Horioka C (1980) Domestic saving and international capital flows. Econ J 90: 314–329. https://doi.org/10.2307/2231790 doi: 10.2307/2231790
![]() |
[12] |
Ford N, Horioka C (2017) The 'real' explanation of the Feldstein-Horioka puzzle. Appl Econ Lett 24: 95–97. https://doi.org/10.1080/13504851.2016.1164814 doi: 10.1080/13504851.2016.1164814
![]() |
[13] |
Fouquau J, Hurlin C, Rabaud I (2008) The Feldstein-Horioka puzzle: A panel smooth transition regression approach. Econ Model 25: 284–299. https://doi.org/10.1016/j.econmod.2007.06.008 doi: 10.1016/j.econmod.2007.06.008
![]() |
[14] | Fuller WA (1996) Introduction to Statistical Time Series. John Wiley and Sons, New York. |
[15] |
Granger CWJ (1969) Investigating causal relations by econometric models and cross-spectral methods. Econometrica 37: 424–438. https://doi.org/10.2307/1912791 doi: 10.2307/1912791
![]() |
[16] |
Ibarra-Yunez A (2008) Capital mobility and mexico's challenge toward financial integration. Lat Am Econ Rev 9: 256–27. https://doi.org/10.1080/10978520902873250 doi: 10.1080/10978520902873250
![]() |
[17] |
Jia S, Dong H, Yang H (2021) Asymmetric risk spillover of the international crude oil market in the perspective of crude oil dual attributes. Front Environ Sci 9: 720278. https://doi.org/10.3389/fenvs.2021.720278 doi: 10.3389/fenvs.2021.720278
![]() |
[18] | Martín-Fernádez JA, Bren M, Barcelo-Vidal C, et al. (1999) A measure of difference for compositional data based on measures of divergence. |
[19] | McLachlan G, Peel D (2004) Finite Mixture Models. Wiley Series in Probability and Statistics, John Wiley and Sons, New York. |
[20] |
Narayan P (2005) The saving and investment nexus for China: Evidence from cointegration tests. Appl Econ 37: 1979–1990. https://doi.org/10.1080/00036840500278103 doi: 10.1080/00036840500278103
![]() |
[21] | Pawlowsky-Glahn V, Egozcue JJ, Tolosana-Delgado R (2007) Lecture notes on compositional data analysis. |
[22] |
Said SE, Dickey DA (1984) Testing for unit roots in autoregressive-moving average models of unknown order. Biometrika 71: 599–607. https://doi.org/10.1093/biomet/71.3.599 doi: 10.1093/biomet/71.3.599
![]() |
[23] |
Sinha D, Sinha T (1998) An exploration of the long-run relationship between saving and investment in the developing economies: A tale of latin american countries. J Post Keynesian Econ 20: 435–443. https://doi.org/10.1080/01603477.1998.11490162 doi: 10.1080/01603477.1998.11490162
![]() |
[24] | Thomas P, Lovell D (2014) Compositional data analysis (CoDA) approaches to distance in information retrieval. In: Geva, S., et al., The 37th International ACM SIGIR Conference on Research and Development in Information Retrieval, SIGIR '14, Gold Coast, QLD, Australia, 991–994. https://doi.org/10.1145/2600428.2609492 |
1. | Jian Li, Mingming Ni, Zhiyi Li, Impact of rural capital outflow on sustainable economic growth: evidence from Shaanxi Province of China, 2023, 36, 1331-677X, 10.1080/1331677X.2023.2192777 |
Levels | First differences | |||
Statistic | P-value | Statistic | P-value | |
Chile | -1.90 | 0.62 | -4.69 | 0.01 |
Colombia | -1.50 | 0.79 | -5.43 | 0.01 |
Peru | -2.69 | 0.29 | -5.16 | 0.01 |
Mexico | -2.63 | 0.31 | -5.31 | 0.01 |
Levels | First differences | |||
Statistic | P-value | Statistic | P-value | |
V.1 | -1.81 | 0.66 | -5.72 | 0.01 |
V.2 | -1.70 | 0.70 | -5.68 | 0.01 |
V.3 | -2.24 | 0.48 | -5.60 | 0.01 |
No. of lags | Market capitalization differences | Ilr transformed series differences | ||
AIC | BIC | AIC | BIC | |
1 | 16085.49 | 16150.43 | -1843.73 | -1804.76 |
2 | 16010.39 | 16127.09 | -1823.78 | -1755.70 |
3 | 15932.34 | 16100.63 | -1803.59 | -1706.50 |
6 | 15728.65 | 16050.69 | -1749.24 | -1565.68 |
12 | 15317.52 | 15942.24 | -1620.94 | -1267.14 |
24 | 14379.77 | 15589.55 | -1481.95 | -799.11 |
36 | 12544.07 | 14309.25 | -1510.65 | -515.45 |
Dependent variable | Statistic | P-value |
Chile | 1.33 | 0.10 |
Colombia | 1.05 | 0.40 |
Peru | 1.15 | 0.26 |
Mexico | 0.83 | 0.75 |
Dependent variable | Statistic | P-value |
V.1 | 1.67 | 0.01 |
V.2 | 1.06 | 0.39 |
V.3 | 1.06 | 0.38 |
Levels | First differences | |||
Statistic | P-value | Statistic | P-value | |
Chile | -1.90 | 0.62 | -4.69 | 0.01 |
Colombia | -1.50 | 0.79 | -5.43 | 0.01 |
Peru | -2.69 | 0.29 | -5.16 | 0.01 |
Mexico | -2.63 | 0.31 | -5.31 | 0.01 |
Levels | First differences | |||
Statistic | P-value | Statistic | P-value | |
V.1 | -1.81 | 0.66 | -5.72 | 0.01 |
V.2 | -1.70 | 0.70 | -5.68 | 0.01 |
V.3 | -2.24 | 0.48 | -5.60 | 0.01 |
No. of lags | Market capitalization differences | Ilr transformed series differences | ||
AIC | BIC | AIC | BIC | |
1 | 16085.49 | 16150.43 | -1843.73 | -1804.76 |
2 | 16010.39 | 16127.09 | -1823.78 | -1755.70 |
3 | 15932.34 | 16100.63 | -1803.59 | -1706.50 |
6 | 15728.65 | 16050.69 | -1749.24 | -1565.68 |
12 | 15317.52 | 15942.24 | -1620.94 | -1267.14 |
24 | 14379.77 | 15589.55 | -1481.95 | -799.11 |
36 | 12544.07 | 14309.25 | -1510.65 | -515.45 |
Dependent variable | Statistic | P-value |
Chile | 1.33 | 0.10 |
Colombia | 1.05 | 0.40 |
Peru | 1.15 | 0.26 |
Mexico | 0.83 | 0.75 |
Dependent variable | Statistic | P-value |
V.1 | 1.67 | 0.01 |
V.2 | 1.06 | 0.39 |
V.3 | 1.06 | 0.38 |