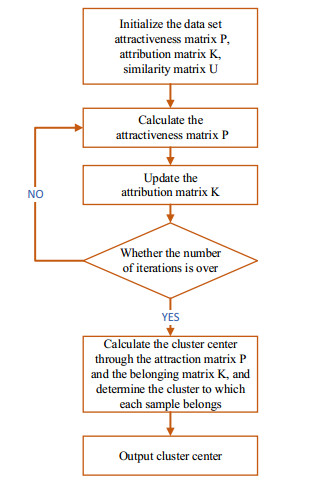
Technological innovation in the biomedical industry is the basis for improving the core competitiveness of the biomedical industry and promoting the high-quality development of the industry. However, the technological innovation capacity of China's biomedical industry is not balanced, and there are great differences between regions. Therefore, accurately grasping the status quo of technological innovation in China's biomedical industry and assessing regional differences are of great significance and effect for the country to formulate targeted policies and systems. In view of this, this paper designs a two-stage biomedical industry technological innovation capability evaluation index system from the perspective of the innovation value chain. According to the panel data of China's biomedical industry from 2012 to 2018, a grey relational clustering model based on panel data is constructed and used to evaluate the technological innovation capability of China's biomedicine industry from two dimensions: the level of technological research and development and the ability to transform technological achievements.
Citation: Pin Lv. Evaluation of biomedical industry technological innovation ability based on a grey panel clustering model[J]. Mathematical Biosciences and Engineering, 2023, 20(1): 1538-1557. doi: 10.3934/mbe.2023070
[1] | Tinghui Li, Jieying Wen, Danwei Zeng, Ke Liu . Has enterprise digital transformation improved the efficiency of enterprise technological innovation? A case study on Chinese listed companies. Mathematical Biosciences and Engineering, 2022, 19(12): 12632-12654. doi: 10.3934/mbe.2022590 |
[2] | Zhi-ying Han, Yong Liu, Xue-ge Guo, Jun-qian Xu . Regional differences of high-quality development level for manufacturing industry in China. Mathematical Biosciences and Engineering, 2022, 19(5): 4368-4395. doi: 10.3934/mbe.2022202 |
[3] | Fei Wang, Benhai Guo, Zixing Wang, Yuxin Wu . The impact of digital economy on the export competitiveness of China's manufacturing industry. Mathematical Biosciences and Engineering, 2023, 20(4): 7253-7272. doi: 10.3934/mbe.2023314 |
[4] | Xiran Yang, Yong Qi . Study on the evolution driving mechanism of IPM to regional economic development. Mathematical Biosciences and Engineering, 2022, 19(7): 6699-6711. doi: 10.3934/mbe.2022315 |
[5] | Yi Liu, Xuan Zhao, Feng Mao . The synergy degree measurement and transformation path of China's traditional manufacturing industry enabled by digital economy. Mathematical Biosciences and Engineering, 2022, 19(6): 5738-5753. doi: 10.3934/mbe.2022268 |
[6] | Yue Liu, Chunying Ma, Zhehao Huang . Can the digital economy improve green total factor productivity? An empirical study based on Chinese urban data. Mathematical Biosciences and Engineering, 2023, 20(4): 6866-6893. doi: 10.3934/mbe.2023296 |
[7] | Delong Cui, Hong Huang, Zhiping Peng, Qirui Li, Jieguang He, Jinbo Qiu, Xinlong Luo, Jiangtao Ou, Chengyuan Fan . Next-generation 5G fusion-based intelligent health-monitoring platform for ethylene cracking furnace tube. Mathematical Biosciences and Engineering, 2022, 19(9): 9168-9199. doi: 10.3934/mbe.2022426 |
[8] | María-Isabel Sánchez-Segura, German-Lenin Dugarte-Peña, Antonio de Amescua, Fuensanta Medina-Domínguez, Eugenio López-Almansa, Eva Barrio Reyes . Smart occupational health and safety for a digital era and its place in smart and sustainable cities. Mathematical Biosciences and Engineering, 2021, 18(6): 8831-8856. doi: 10.3934/mbe.2021436 |
[9] | Yue Liu, Liming Chen, Han Luo, Yuzhao Liu, Yixian Wen . The impact of intellectual property rights protection on green innovation: A quasi-natural experiment based on the pilot policy of the Chinese intellectual property court. Mathematical Biosciences and Engineering, 2024, 21(2): 2587-2607. doi: 10.3934/mbe.2024114 |
[10] | Yung-Pin Cheng, Ching-Wei Li, Yi-Cheng Chen . Apply computer vision in GUI automation for industrial applications. Mathematical Biosciences and Engineering, 2019, 16(6): 7526-7545. doi: 10.3934/mbe.2019378 |
Technological innovation in the biomedical industry is the basis for improving the core competitiveness of the biomedical industry and promoting the high-quality development of the industry. However, the technological innovation capacity of China's biomedical industry is not balanced, and there are great differences between regions. Therefore, accurately grasping the status quo of technological innovation in China's biomedical industry and assessing regional differences are of great significance and effect for the country to formulate targeted policies and systems. In view of this, this paper designs a two-stage biomedical industry technological innovation capability evaluation index system from the perspective of the innovation value chain. According to the panel data of China's biomedical industry from 2012 to 2018, a grey relational clustering model based on panel data is constructed and used to evaluate the technological innovation capability of China's biomedicine industry from two dimensions: the level of technological research and development and the ability to transform technological achievements.
The current research on the technological innovation of the biomedical industry mainly focuses on the connotation and definition of the biomedical industry, the development status and capability level evaluation of the biomedical industry, the evaluation of technological innovation capabilities and the identification of influencing factors, the construction of measurement methods for evaluating the technological innovation and development capability of the biomedical industry, the development mechanism and path design. In terms of the connotation and definition of the biomedical industry, Wen, Huang, and others pointed out that the biomedical industry refers to an industry that combines modern biotechnology and medicine [1]. Hinterhuber believed that the biomedical industry could not be limited to the biotechnology industry alone. In addition to having the foundation of modern biotechnology, it must also integrate these technologies with the pharmaceutical industry to realize the manufacture and production of new drugs. Not only biotechnology; but also industrial technology in the production link, marketing methods in the sales link, etc. were involved here [2]. In terms of the analysis of the development status of the biomedical industry, and the development ability or level evaluation, it mainly analyzed the development status of the biomedical industry [3,4], identified the factors of the development level of the biomedical industry, and built an industrial development evaluation index system and evaluation methods. At present, the evaluation of the development capability or level of the biomedical industry mainly starts from the analysis of influencing factors that affect the level of industrial development, the design of the evaluation index system, and the construction of evaluation methods. The factors affecting the development level of the biomedical industry mainly include market structure, enterprise scale, technological innovation, collaborative resources, new economic growth efficiency, and so on [5,6]. Based on these influencing factors, this paper is intended to design the industrial development capability or level evaluation index system, and construct a multi-level grey evaluation model [7], multiple regression model [8], stochastic frontier analysis method [9], data envelopment model [10], Malmquist Index model [11] and so on. The research on the evaluation of the technological innovation ability of the biomedical industry and the identification of its influencing factors mainly constructs the evaluation index system of technological innovation of the biomedical industry and establishes evaluation methods [12]. In terms of the evaluation index system, Xu and Sun designed a three-stage data envelopment analysis (DEA) model evaluation index system with 8 secondary indexes and 10 tertiary indexes from the three perspectives of input variables, output variables, and environmental variables [13]. Zhang and Chen made a comprehensive evaluation of 16 industries in Jiaxing City, Zhejiang Province, from the perspective of development potential. They constructed an industrial development potential evaluation index system with 5 secondary indexes and 16 primary indexes from two aspects: the ability to maintain growth and the ability to sustain development [14]. In order to establish an evaluation index system in line with the characteristics of China's biomedicine, a strategic emerging industry, Zhang and others constructed a biomedicine evaluation index system covering 11 secondary indexes considered from the four aspects of the conversion rate of scientific and technological achievements, the growth rate of R&D expenditure, the growth rate of the total value of new products, and the growth rate of per capita R&D expenditure [15]. Wen and others divided the industrial innovation capability into four dimensions (enterprise-level technological innovation capability, industrial-level network innovation capability, regional-level cluster innovation capability, and national-level policy innovation capability) and constructed 8 secondary indexes and 26 tertiary indexes about the evaluation index system of the innovation ability of the biomedical industry [1]. In terms of the construction of evaluation methods for the technological innovation capability of the biomedical industry, factor analysis methods [16], the DEA model [17], and the Malmquist index model [11] are mainly used to evaluate the technological innovation capability and efficiency of the biomedical industry. It points out the problems and advantages in the development of the biomedical industry; and gives countermeasures. Among them, He and others analyzed how to improve the efficiency of common technical services in the biomedical industry from the two aspects of common technology supply and application sharing. They used the chain network DEA method to evaluate the efficiency of common technical services in 31 provinces, cities, and regions in China [18]. Stuart, Ozedemir and Ding analyzed the vertical alliance network of the biomedical industry by taking the university-biotechnology R&D company-pharmaceutical alliance chain as an example. They believe that R&D companies occupy an intermediate position in the tripartite vertical alliance network of the biomedical industry and play a key role in resource allocation [19]. Achilladelis and Antonakis analyzed the originality and commercial significance of 1736 innovative drugs from 1800 to 1990. They pointed out that the technological innovation of the pharmaceutical industry not only relies on scientific and technological progress and market demand, but also is jointly promoted by social needs, government legislation, new raw materials, and competition among enterprises [20]. However, the selection and application of these methods are too singular and subjective, and they contain large human factors. It is difficult to effectively mine the information contained in the panel data of the regional biomedical industry development, making the evaluation results not objective enough.
According to the above discussion and analysis, there are still two problems in the assessment of the technological innovation capability of the biomedical industry. 1) The indexes for assessing the level of development need to be improved. At present, there are relatively few studies on the dynamic development trend of technological innovation and the evaluation of innovation ability. Most of the existing related studies discuss and analyze it as a whole, while this approach ignores the differences in innovation activities between different regions. It includes innovation input, innovation environment support, and innovation output. At the same time, the stage of development will be more or less ignored. 2) The development level measurement method needs to be improved. Regarding the evaluation and research of the biomedical industry, most of the methods used are relatively simplistic and involve more subjective factors, which makes it impossible to objectively evaluate the data and information mined.
To describe the characteristics, structure, and element composition of the object more objectively, the method of constructing an index system is usually adopted to evaluate the object under certain principles. Therefore, based on the five principles of objectiveness, comparability, scientificity, usability, and systemic principles, this article establishes an evaluation index system based on the influencing factors and essential characteristics of the technological innovation capability of the biomedical industry. The evaluation index system of technological innovation in the biomedical industry needs to be constructed from different angles and levels. The selected evaluation indexes cover the characteristics of the technological innovation capability of the biomedical industry as much as possible, to objectively and comprehensively describe the development status of the technological innovation capability of the biomedical industry in China.
With the deepening of academic research on innovation theory, the innovation process is no longer simply regarded as a single-stage input-output process. Many scholars have begun to refine and decompose the technological innovation process of high-tech industries, and they decompose the technological innovation process into two sub-stages: technology research and development and technology transformation [21,22,23,24]. Yin et al. [25] took the number of technical personnel and R&D investment as the input indexes of the first stage of innovation activities of pharmaceutical companies, and the number of patent applications as the output indexes of this stage. Based on the two-stage correlation, they chose the output index of the first stage as the input index of the second stage, and the output of the second stage is measured by the main business income. Zheng et al. [26] selected the number of R&D and technical personnel and R&D capital stock as the input indexes of the first stage, and they selected the number of invention patent applications, the number of utility model patent applications, and intangible assets as the intermediate output indexes. They then used the main business income as the final output indicator. Based on this, they constructed a two-stage innovation efficiency evaluation index system to evaluate the innovation efficiency of listed pharmaceutical companies in China Zhou et al. [27] used the panel data of the pharmaceutical manufacturing industry in 23 provinces (autonomous regions and municipalities) in China from 2009 to 2018 as the research sample, and they used the Malmquist index model to compare the dynamic innovation efficiency values of the pharmaceutical manufacturing industry in the 23 provinces and cities in the stage of technological R&D and achievement transformation. Yu et al. [28] found that the number of R&D institutions is most closely related to the competitiveness of the pharmaceutical manufacturing industry, followed by the contribution rate of new products, the intensity of R&D expenditures, the proportion of R&D personnel in employees, and the proportion of new product development expenditures in industry sales revenue, etc. Liu and Ma [29] added technology acquisition capabilities such as the investment intensity of technology introduction and digestion and absorption, the investment intensity of technological transformation, and the investment intensity of domestic technology purchases into the resource input indexes. They also added the second-level indicator of government investment intensity in scientific research funding to the first-level indicator of the innovation environment.
Through literature review, most of the research on the index system of technological innovation capability related to the biomedical industry is based on a certain set of subjective logic, classifying the research results of scholars, and revising and increasing or decreasing the indexes according to the latest research results. Although there is no general consensus on the index system in the academic circle, generally speaking, the core evaluation system of technological innovation capability in the pharmaceutical industry tends to be an input-output system based on the entire process of technological innovation. Among them, the resource input capability of technological innovation is the primary aspect to measure the technological innovation capability of the biomedical industry. The output capability of technological innovation reflects the actual results produced by the combination of various elements, and it is the second important aspect of judging the technological innovation capability of an industry. In addition, due to the increasingly fierce competition in the field of new drugs at home and abroad in recent years, the R&D institutions independently established by enterprises have played an increasingly significant role in improving the efficiency and effectiveness of innovation and increasing the probability of successful technological innovation. At the same time, the stock of human resources, economic resources and advanced equipment in a region can reflect the potential technological innovation capability of the region, and can promote the sustainable innovation and development of the region and enterprises. These can be used as environmental support and are the third aspect used to measure the technological innovation capability. Based on this, this paper constructs the evaluation index system of technological innovation capability from the two perspectives of technology research and development and technological achievement transformation. Based on the calculation results of these two stages, we analyze the differences in the technological innovation capabilities of the biomedical industry between provinces. Also, we analyze the reasons for unbalanced, uncoordinated, and large development differences in combination with factors such as geographic location and economic development status.
1) In the technology research and development stage, this paper selected evaluation indexes based on the three perspectives of technological innovation input, technological innovation output, and innovation environment support. Combining existing relevant research literature, common indexes for measuring innovation and R&D in the biomedical industry included the number of companies with R&D activities, R&D internal expenditures, and new product development expenditures. This article also uses the full-time equivalent of R&D personnel to better measure the degree of attention paid to the R&D activities of biomedical companies. In terms of investment in technological innovation, the secondary indexes selected in this article are: the number of enterprises with R&D activities, the number of full-time equivalents of R&D personnel, internal R&D expenditures, and new product development expenditures, which are recorded as x11,x12,x13,x14. Correspondingly, the weights are denoted as w11,w12,w13,w14. In terms of technological innovation output, this article focuses more on patents and new product development, so the number of patent applications, the number of invention patent applications, the number of valid invention patents, and the number of new product development projects are selected as secondary indexes, which are recorded as x15,x16,x17,x18. The weights are recorded as w15,w16,w17,w18. In terms of innovation environment support, five indexes, including newly added fixed assets, number of enterprises with R&D institutions, number of R&D institutions, expenditures of R&D institutions, and equipment value of R&D institutions, are selected, which are recorded as x19,x110,x111,x112,x113, and the weights are recorded as w19,w110,w111,w112,w113, respectively. These 13 index systems are used to measure the provincial biomedical industry development technology R&D level and characterize the provincial biomedical industry's innovation capability in the R&D stage.
2) In the stage of technological achievement transformation, evaluation indexes are selected from three aspects: innovation achievement input, intermediate technology input, and industrialization benefit. In terms of investment in innovation achievements, 6 indexes are selected, namely, the number of patent applications, the number of invention patent applications, the number of pharmaceutical industry companies, the number of new product development projects, the amount of fixed asset investment, and the number of valid invention patents by companies, which are recorded as x21,x22,x23,x24,x25,x26, and the weights are respectively recorded as w21,w22,w23,w24,w25,w26. In terms of intermediate technology input, the two selected indexes are domestic technology expenditure and technological transformation expenditure, which are recorded as x27,x28, respectively, and the corresponding weights are recorded as w27,w28. In terms of industrialization benefits, the three selected indexes are new product sales revenue, export delivery value, and profit, which are recorded as x29,x210x211, respectively, and the corresponding weights are recorded as w29,w210,w211.
Grey relational clustering is a method for classifying research objects according to their degree of relevance. Under the two-dimensional data, a large number of research results on the grey relational model (GRA) [30,31] are the basis of grey relational clustering, and different GRA models can be selected according to the needs of practical problems. Liu et al. [32] pointed out that there are many practical problems and scientific problems in reality, and it is urgent to use high-dimensional data such as panel data to study. Therefore, the association model and cluster analysis of panel data are very valuable research directions. Among the clustering methods under panel data, the advantage of grey relational clustering is that it does not have high requirements on the number of samples and the length of time. The grey relational clustering under panel data needs to solve two problems: One is the grey relational analysis of panel data, and the other is to cluster according to the degree of relevance. Clustering models based on panel data indexes have a wide range of applications, such as regional division of air quality [33], regional ecological environment assessment [34], human resource allocation [35], etc.
At present, most of the assessment of technological innovation capability in the biomedical industry uses factor analysis, structural equation models, DEA models and other methods. These methods are highly subjective in application and single in form. They cannot fully mine the information contained in panel data, resulting in large errors in calculation results and low credibility. The grey clustering method has great advantages in dealing with the problem of "poor information" clustering [36]. Comprehensive evaluation using cluster analysis can classify object categories and attributes, combined with the spatio-temporal attributes of panel data. Based on the grey relational analysis and hierarchical clustering method, this paper uses the multi-index grey relational analysis clustering method to process panel data. The proposed grey clustering evaluation method extends the traditional grey clustering method from the original two-dimensional cross-section static situation problem to the three-dimensional panel data problem, and it expands the research scope of the grey clustering method. Moreover, this method can reflect the implicit relationship between the evaluation objects from the change of the development trend, thereby increasing the reliability of the evaluation subject, accurately grasp the differences and characteristics of different attributes of the research objects, and help improve the timeliness of policies and programs.
Panel data refers to intercepting multiple cross-sections and measuring the value of the same cross-section research object at different time nodes. It has spatial and temporal characteristics. Multi-index panel data is three-dimensional structure data composed of multiple two-level, two-dimensional data tables on a plane, including three dimensions of information: time dimension, object dimension, and index dimension. Multi-index panel data can be represented by a three-dimensional matrix, and the three-dimensional matrix can be converted into a two-bit matrix sequence.
Record the index value of the j-th index of the i-th object in the multi-index panel data X at the T time node as xij(t), so the original data structure can be represented by a matrix.
1) The indicator observation matrix X(0)(i) of the i-th sample at different time nodes:
X(0)(i)=(xi1(1)xi1(2)…xi1(T)xi2(1)xi2(2)…xi2(T)…xim(1)…xim(2)………xim(T)),i=1,2,3,···,N | (1) |
2) The sample observation matrix X(1)(j) of the j-th indicator at different time nodes:
X(1)(j)=(x1j(1)x1j(2)…x1j(T)x2j(1)x2j(2)…x2j(T)…xNj(1)…xNj(2)………xNj(T)),j=1,2,3,···,m | (2) |
3) The sample indicator observation matrix X(2)(t) at time t:
X(2)(t)=(x11(t)x12(t)…x1m(t)x21(t)x22(t)…x2m(t)…xN1(t)…xN2(t)………xNm(t)),t=1,2,3,···,T | (3) |
N—the total number of samples; m—the number of indexes; T—the maximum length of the time series.
Use behavior matrix X(0),X(1),X(2) to represent multi-indicator panel data:
1) Behavioral horizontal matrix sequence of system factors X(0)
X(0)={X(0)(i)|i=1,2,3,···,N} | (4) |
2) Behavioral Index Matrix Sequence of System Factors X(1)
X(1)={X(1)(j)|j=1,2,3,···,m} | (5) |
3) Behavioral Time Matrix Sequence of System Factors X(2)
X(2)={X(2)(t)|t=1,2,3,···,T} | (6) |
Using panel data can not only observe the absolute level of development of a certain indicator of the research object in a period of time, but also extract the development trend of the indicator through panel data. Based on this, this paper uses "horizontal" distance, "incremental" distance and "variation" distance to measure whether there is a correlation between the changing trend of panel data, and then analyze the temporal and spatial characteristics of the data through the model.
Record d1ij as the "horizontal" distance between research object i and research object j, which can reflect the absolute development level distance between the two in the research period.
d1ij=||X(0)(i)−X(0)(j)||2||X(0)(i)−X(0)(j)||2representstheEuclideannormofthematrixX(0)(i)−X(0)(j). | (7) |
Record d2ij as the "incremental" distance between research object i and research object j, which represents the difference between the two indexes over time. If the index changes in the same direction, it means that the two change trends are similar; otherwise, the greater the distance, the worse the similarity between the two.
d2ij=||5X(2)(i)−X(2)(j)||25X(2)(t)=(△xik(t)xik(t))N−Km,△xik(t)=xik(t)−xik(t−1),△xjk(t)=xjk(t)−xjk(t−1).||5X(2)(i)−X(2)(j)||2representtheEuclideannormofthematrix5X(2)(i)−X(2)(j). | (8) |
Record d3ij as the "variation" distance between the research object i and the research object j, which represents the degree of fluctuation of the two index values over time. If the risk degree of the two index fluctuations is similar, the two research objects are more similar.
d3ij=¯T∑t=1[¯xiteit−¯xjtejt]2 | (9) |
¯xit=1m∑mk=1xik(t), e2it=1m−1∑mk=1(xik(t)−¯xit)2, ¯xit and eit respectively represent the standardized variable mean value and standardized standard deviation of the m indexes of the i-th research object at period t.
Due to the different dimensions of different indexes, if the original data is used directly, it will have a greater impact on the modeling calculation and analysis, and its reliability cannot be better guaranteed. Based on this, the data is usually subjected to dimensionless processing. Commonly used methods include: initial value transformation, average value transformation, and interval value transformation.
Consider the system behavior matrix sequence:
X0={X0(1),X0(2),X0(3),···,X0(n)} | (10) |
X1={X1(1),X1(2),X1(3),···,X1(n)} | (11) |
X2={X2(1),X2(2),X2(3),···,X2(n)}⋯⋯ | (12) |
Xi={Xi(1),Xi(2),Xi(3),···,Xi(n)}⋯⋯ | (13) |
Xm={Xm(1),Xm(2),Xm(3),···,Xm(n)}. | (14) |
Xi(j) is a matrix, for ζ∈(0, 1), and let
γ(X0(k),Xi(k))=minimink|X0(k)−Xi(k)|+ζmaximaxk|X0(k)−Xi(k)||X0(k)−Xi(k)|+ζmaximaxk|X0(k)−Xi(k)| | (15) |
where |X0(k)−Xi(k)|=d10i+d20i+d30i. Then, the grey correlation degree of matrix sequence X0 and Xi is:
γ(X0,Xi)=1TT∑k=1γ(X0(k),Xi(k)). | (16) |
In the formula, γ(X0(k),Xi(k)) is the grey correlation coefficient of matrix X0(k) and Xi(k) at point k, which is expressed as the relative difference between the characteristic data sequence curves of the two behavior factors. ζ is the resolution coefficient, and generally ζ takes 0.5 to reduce the influence of extreme values on calculation.
The Affinity Propagation Clustering algorithm is an algorithm for clustering research objects based on the similarity of data nodes [36]. Different from traditional clustering algorithms, the AP clustering algorithm can efficiently cluster data with higher dimensions and more categories. It not only improves the clustering performance by a large margin, but it also greatly improves the efficiency. The final choice of the clustering center depends on the information transmission between nodes, and the two types of information are transmitted between the nodes and the nodes to achieve the clustering of the research objects. The result of clustering depends on the degree of similarity of the research objects and the "information dissemination" situation.
The AP algorithm takes the similarity matrix U of the research object as the input variable, and denote u(i,j) as the similarity between data point i and data point j, which can be interpreted as the suitability of data point j as the cluster center of data point i. Denote the attractiveness matrix as P, where p(i,j) represents the attractiveness of the research object xj to the research object xi, and the information dissemination direction is i to j. Denote the attribution matrix as K, where k(i,j) is called the attribution degree of the research object xj to the research object xi, and the information propagation direction is from j to i.
The greater the sum of the attractiveness and the attribution, the greater the degree of when the sample is the final cluster center. The specific operating rules are as follows.
1) Algorithm initialization. First, initialize the algorithms of the attraction matrix P and the attribution matrix K, and let p(i,j)=0,k(i,j)=0.
2) Update the attractiveness matrix P.
pt+1(i,k)={U(i,k)−maxj≠k{kt(i,k)+pt(i,k)},i≠kU(i,k)−maxj≠k{U(i,k)},i=k | (17) |
3) Update the attribute matrix K.
kt+1(i,k)={mini≠k{0,pt+1(i,k)+∑j≠i,kmax{pt+1(i,k)j≠k,0}∑j≠kmax{pt+1(i,k)j≠k,0},i=k | (18) |
4) Introduce a damping factor ε to attenuate the formula.
pt+1(i,k)=ε∗pt(i,k)+(1−ε)∗pt+1(i,k) | (19) |
kt+1(i,k)=ε∗kt(i,k)+(1−ε)∗kt+1(i,k) | (20) |
5) Measure the sum of the degrees of belonging and attractiveness of all nodes. When the cluster center is stable or reaches the maximum number of iterations, the optimal class representative point and the subordination relationship between the sample point and the class representative point are obtained.
Based on the grey correlation model, the grey correlation degree is used to measure the development gaps of technological innovation capabilities of the biomedical industry in various provinces. The degree of development gap is characterized by grey correlation degree. The bias parameter α represents the probability that a node becomes a representative point of a class, and its size will affect the number of clusters. Increasing the value of α can increase the number of classes. Therefore, the α value can be determined through multiple experiments, and all provinces can be divided into the three categories of excellent, qualified, and poor using the AP clustering algorithm. The specific steps are as follows.
1) Record γij as the grey correlation degree of the research object Xi and Xj, and calculate the grey matrix A.
A=(γ11γ12…γ1j…γ1mγ21γ22…γ2j…γ2m…γi1···γn1…γi2···γn2…······…γij···…······…γnj……γim···γnm) | (21) |
2) Record U as the development gap matrix between different provinces. The greater the distance and the development similarity between the two places, the smaller the distance, the higher the development similarity between the two places, and it is obtained through the transformation of the grey incidence matrix.
U=A−E | (22) |
3) Record each iteration result P′ of the initial p(i,j) and each iteration result K′ of k(i,j), and determine the number of iterations. Record Z=P′+K′, when Z > 0, and we can determine the cluster center object.
4) When the cluster center object does not change, the iteration is stopped when the iteration is the highest. At this time, the output is the final result of the cluster. Otherwise, the attribution and attraction matrix are re-determined, and a series of tasks are repeated.
This paper selects 30 provinces in mainland China as the research object and conducts a two-stage cluster analysis of these 30 provinces (for data reasons, Tibet, Macau, Hong Kong, and Taiwan are not included in the research object). From the China Statistical Yearbook, high-tech statistical yearbooks of various provinces, and other statistical data, we collected and sorted out the relevant data on the measurement indexes of the development level of the biomedical industry from 2013 to 2019 (the data is no longer listed). Based on the collected data, we separately measured the technological innovation capabilities of the biomedical industry in each province, analyzed the differences in the development of each region, and formulated policies and measures suitable for the development of the biomedical industry in different provinces and cities.
1) Measurement of technological innovation capability in the research and development stage of each province's biomedical industry
According to the previous research, the weights of the indexes in the technology research and development stage are as follows:w11=0.1,w12=0.12,w13=0.13,w14=0.09,w15=0.1,w16=0.11,w17=0.05,w18=0.03,w19=0.04,w110=0.03,w111=0.05,w112=0.04,w113=0.06,w114=0.05. At this time, the weights of spatiotemporal feature attributes are w11=0.7,w12=0.1,w13=0.2. According to the collected data, using the matrix grey correlation model, the grey correlation degree between the provinces and cities in the technology research and development stage can be calculated, as shown in Table S1. The 30 provinces and cities are clustered at the technology research and development stage, which can be grouped into three categories. The bias parameter α is 0.48. 1) Good: Fujian (FJ), Guangdong (GD), Zhejiang (ZJ), Chongqing (CQ), Hubei (HB), Jiangsu (JS), Shandong (SD), Shanghai (SH), Tianjin (TJ), Beijing (BJ). 2) Middle: Guangxi (GS), Hunan (HN), Sichuan (SC), Henan (HA), Yunnan (YN), Anhui (AH), Shaanxi (SX), Jilin (JL), Shanxi (SX), Hebei (HE); Heilongjiang (HL), Liaoning (LN), Gansu (GS), Hainan (HI), Jiangxi (JX). 3) Poor. Guizhou (GZ), Qinghai (QH), Ningxia (NX), Xinjiang (XJ), Inner Mongolia (NM).
From the grey correlation and clustering results, it can be seen that the pharmaceutical industry in the research and development stage is more in line with "the highest in the eastern region, and lower in the central and western regions". Eastern provinces and cities such as BJ, SH, JS, GD, FJ, SD, ZJ have strong technological innovation capabilities, followed by central and western provinces and cities such as HN, GX, SC, SN, HE, JX, JL, and HA. NM, NX, XJ, QH, GS, and other northwestern provinces and cities are the weakest. There are also provinces with strong technological innovation capabilities in the central and western regions, such as HB and CQ. Hubei Province (HB) is rich in medicinal material resources, strong in pharmaceutical scientific research, has a number of pharmaceutical enterprises with strong strength and popularity, and has formed its own advantages and characteristics in the fields of biological products, chemical raw materials and preparations. Chongqing (CQ) is very rich in medicinal plant resources. It is one of the important producing areas of Chinese herbal medicines in the country, and there are many units in CQ engaged in medical technology research and development, including many universities, research institutes and bases. The pharmaceutical industry, as an emerging pillar industry in CQ, has also received strong support from the government. Therefore, these factors and environments make these provinces and cities have strong technological innovation capabilities. Compared with other provinces and cities in the central and western regions, BJ, SH, JS, GD and other eastern provinces and cities have a large number of scientific and technological resources and talents, such as universities and research institutes. At the same time, local governments also attach great importance to scientific research investment. However, due to the relative shortage of funds and talents in the central and western provinces and cities, R&D investment is insufficient. Although TJ, SC, and SN have a large number of universities and research institutes, their level of technology research and development is relatively average due to relatively insufficient investment in research and development funds and related research personnel, or insufficient attention to research and development by local governments.
2) Measurement of the level of technological innovation capability in the transformation stage of the biomedical industry in each province
Correspondingly, the weights of various indexes and the weights of spatiotemporal feature attributes in the transformation stage of technological achievements are w21=0.3,w22=0.11,w23=0.2,w24=0.05,w25=0.03,w26=0.06,w27=0.03,w28=0.1,w29=0.05,w210=0.01,w211=0.06. The weights of spatiotemporal feature attributes are w21=0.8,w22=0.1,w23=0.1. According to the collected data, using the matrix grey correlation model, the grey correlation degree between provinces and cities in the technology transformation stage can be calculated, as shown in Table S2. The 30 provinces and cities are clustered at the technological transformation stage, which can be grouped into three categories, in which the bias parameter α is 0.51. 1) Strong: Fujian (FJ), Guangdong (GD), Guangxi (GX), Hunan (HN), Zhejiang (ZJ), Anhui (AH), Hubei (HB), Jiangsu (JS), Shandong (SD), Shaanxi (SN), Beijing (BJ), Hebei (HE), Shanghai (SH). 2) Medium: Jilin (JL), Sichuan (SC), Shanxi (SX), Yunnan (YN), Henan (HA), Tianjin (TJ), Chongqing (CQ). 3) Weak: Inner Mongolia (NM), Qinghai (QH), Ningxia (NX), Xinjiang (XJ), Guizhou (GZ), Heilongjiang (HL), Liaoning (LN), Gansu (GS), Hainan (HI), Jiangxi (JX). Based on the grey correlation and clustering results, the technology transformation ability of the pharmaceutical industry in eastern provinces such as BJ, SH, JS, and GD is significantly better than that of other provinces and cities; the overall technological transformation ability of the central and western provinces and cities is average. However, the technology transformation level of the pharmaceutical industry in northwestern provinces and cities lags far behind other provinces and cities. Compared with the research and development stage, it can be found that in the technology transformation stage, several provinces and cities in the central region have strong technological innovation capabilities.
3) Cluster analysis of technological innovation capability of China's biomedical industry
If the technology research and development level is taken as the x-axis, and the technology transformation capacity is used as the y-axis, the categories gathered at each stage of each province and city are all three categories, which can be combined to form nine categories (good level of technology research and development-strong ability to transform achievements, good level of technology research and development- mediate ability to transform achievements, good level of technology research and development- weak ability to transform achievements, medium level of technology research and development- strong ability to transform achievements, medium level of technology research and development- mediate ability to transform achievements, medium level of technology research and development- weak ability to transform achievements, poor level of technology research and development- strong ability to transform achievements, poor level of technology research and development- mediate ability to transform achievements, poor level of technology research and development- weak ability to transform achievements). According to the correlation and clustering results between the provinces and cities in the two stages, a two-dimensional cluster chart of the technological innovation capabilities of the pharmaceutical industry can be constructed, as shown in Figure 2.
It can be seen from the clustering results that the nine combined categories appear in six categories. However, the three categories of good level of technology research and development- weak ability to transform achievements, poor level of technology research and development- strong ability to transform achievements, poor level of technology research and development- mediate ability to transform achievements do not exist. This is also consistent with reality. Poor R&D levels are difficult to guarantee and explain the relatively good achievement transformation capabilities of the provinces and cities. Provinces and cities with better technological R&D levels generally actively implement the transformation of scientific and technological achievements to improve their technological innovation capabilities. For the six combination categories, the distribution of the provinces and cities is as follows. Category 1. (Good level of technology research and development-strong ability to transform achievements): ZJ, BJ, GD, JS, SD, HB, SH, FJ. These provinces and cities are mainly distributed in the eastern region, which indicates that the biomedical industry in the eastern region has stronger independent innovation and R&D capabilities, which is related to the gaps in the location, resource advantages, talent pool, technology and economic development level of the provinces and cities. Category 2 (Good level of technology research and development- mediate ability to transform achievements): CQ and TJ. These two provinces and cities have a good level of technological research and development, but their ability to transform scientific and technological achievements is average. Category 3 (Medium level of technology research and development-strong ability to transform results): SN, HE, HN, AH, GX. The level of technological research and development in these provinces and cities is average, but their ability to transform scientific and technological achievements in recent years is relatively strong. This is mainly due to the influence and effect of local government policy support, investment in scientific and technological services and other factors, which greatly improved their ability to transform scientific and technological achievements. Category 4 (Medium level of technology research and development level-medium achievement transformation ability): JL, SC, SX, YN, HA. The level of technological research and development and transformation of technological achievements in these provinces and cities are average. Although these provinces and cities have relatively rich scientific and educational resources, their due strength has not been fully utilized. This is related to the local government's insufficient attention to science and technology investment and transformation. Category 5 (Medium level of technology research and development-weak ability to transform results): HL, LN, GS, HI, JX. These provinces and cities have relatively weak technology research and development levels and the ability to transform scientific and technological achievements, which is related to the relative lack of regional resources in these provinces and cities and the relatively insufficient investment in science and education resources. Category 6 (Poor level of technology research and development-weak ability to transform results): NM, QH, NX, XJ, GZ. These provinces and cities have the weakest level of technological research and development and technological transformation ability, which is related to the relative lack of regional resources and the lack of attention from local governments.
According to the empirical analysis, the technological innovation capability of China' s biomedical industry is generally not strong. There are more provinces and cities with average and weak technology R&D level and technology transformation ability and fewer provinces and cities with good technology R&D level and transformation ability. The technological innovation capability of China's biomedical industry shows obvious regional development differences and imbalances. The eastern provinces and cities performed well in terms of technology R&D level and transformation capacity, followed by the central region, and the northwest region was the weakest. This shows that biomedical industry in the eastern region has stronger independent innovation and research and development capabilities, pays more attention to the transformation of technological achievements, and makes higher utilization of technological achievements.
This paper constructs an evaluation index system and grey cluster evaluation model for the technological innovation capability of the biomedical industry, and it uses it to analyze and evaluate the current status and existing problems of the technological innovation capability of the biomedical industry in China. The results show that China's pharmaceutical industry's overall technological innovation capability is not strong, showing obvious regional development differences and imbalances. There are more provinces and cities with average and weak technology R&D level and technology transformation capacity, and there are fewer provinces and cities with good technology R&D level and transformation capacity. The eastern provinces and cities performed well in terms of technology R&D level and transformation capacity, followed by the central region, and the northwest region was the weakest.
Given the current status and differences in technological innovation capabilities of the pharmaceutical industry in mainland China, the following differential development strategies can be given. For Type 1 provinces and cities, they have initially formed their characteristics and advantages, forming a relatively complete industrial chain. However, compared with advanced countries, there is still a huge gap. Taking innovative drugs as an example, there are currently 20,000 new drugs under research in the world: 50% in the United States, 40% in Europe, and only 3% in China. Therefore, the development and improvement of the industrialization level and benefits of the pharmaceutical industry in such provinces and cities require strengthening R&D investment, enhancing innovation capabilities, and aiming at the global frontier of biomedicine, establishing links between government and social resources, opening up links between scientific research and industry, and establishing an open "Biomedical Industry Technology Innovation Research Institute" to accelerate the transformation of achievements. For Type 2 provinces and cities, there is a good foundation in such provinces and cities. The only drawback is that the investment in technology transformation resources is not enough. This led to insufficient production and application transformation capabilities of technology and knowledge. The development of science and technology industries does not have an advantage in the market. The resources required by the industry cannot highly integrate. The development of infrastructure is relatively backward, the optimization of supporting industries is not enough, and the low efficiency of the transformation of technological achievements has become a weakness. For the development of such provinces, the best measure is to give full play to the advantages of its strong durability. The focus of work is on how to improve the region's ability to integrate and utilize innovative resources, do a good job in industrial innovation, closely combine scientific and technological research and development with market demand, strengthen key upstream and downstream technology research, and use scientific and technological innovation to promote the resilience of the industrial chain and product competitiveness. Promote industrial agglomeration, vigorously educate leading enterprises, promote the aggregate development of upstream and downstream enterprises and affiliated enterprises. To optimize the industrial ecology, departments at all levels must continuously improve industrial support policies, proactively provide enterprises with accurate and high-quality services, help enterprises grow and develop, and jointly promote the high-quality development of the biomedical industry. The industry is gradually moving forward on the road of systematic, large-scale, and high-end development. The goal is to gradually build a high-level innovative development chain among industries in various provinces and cities and implement it in the development process of the biomedical industry. For Type 3 provinces and cities, due to their average level of technological research and development and relatively strong technological transformation capabilities, local governments should guide them with key and important technological solutions, increase talent training and attractiveness, increase technological research and development funding, and policy support, improve its original technological innovation capabilities, increase the optimization of the pharmaceutical industry structure and improve the level of industrial upgrading. They also should focus on cultivating and expanding leading enterprises, promoting the leap-forward development of the medical device industry, vigorously creating five industrial clusters, accelerating the development of a number of high-growth small and medium-sized enterprises, and promoting the construction of a number of key biomedical projects, and they should accelerate the formation of a pharmaceutical industry cluster with field characteristics and professional levels. At the same time, optimizing the innovation environment, providing innovative conditions, tapping the innovation potential of the pharmaceutical industry, and expanding its development space is the only way to achieve the sustainable development of the pharmaceutical industry. For Type 4 provinces and cities, such provinces have average technology research and development levels, average technology transformation capabilities, low industrial levels, and low overall industrial quality. Based on resource endowment conditions, ecological environment capacity, and main functional positioning, to improve the soft and hard environment, they should expand domestic and foreign markets, turn the advantages of medicinal materials resources into economic advantages, support local enterprises to become bigger and stronger, introduce well-known enterprises and scientific research achievements to expand the increment, attract scientific research institutes from outside the province to develop in these provinces and cities, jointly cultivate talents, focus on R&D innovation, form a strong synergy to boost industrial development, and strive to build a strong pharmaceutical manufacturing province. For Type 5 provinces and cities, due to their relatively inadequate scientific and technological resources, local governments have not paid enough attention to them. The level of technological research and development is average, and the ability to transform scientific and technological achievements is weak. To develop such provinces and cities, we should strengthen the construction of technological innovation culture, increase investment in technological research and development and technological services, and improve the industry-university-research cooperative innovation system. We must continue to optimize the protection environment for technological innovation policies, and rely on the industry to gradually improve technological innovation capabilities and promote the development of the pharmaceutical industry. For Type 6 provinces and cities, due to the low level of technology research and development, poor technology transformation capabilities, the low starting point for the development of the pharmaceutical industry, and a small overall number, the technical contents of the biomedical industry are insufficient. The technical level of scientific and technical personnel still needs to be improved, and the overall industrial structure of provinces and cities is not yet perfect. In the long-term stage of the evolution of the industrial structure, it is still at a relatively low level. In this case, to promote regional progress and development, we should take the development of the region's unique advantageous industries and strategic emerging industries as carriers, and give play to the government's regulatory role. We need to increase our investment in technology research and development and scientific and technological services. Taking the central innovative city and the science and technology park as the main body of development, will promote the simultaneous development of the surrounding pharmaceutical industry enterprises. We also need to strengthen the horizontal inter-regional cooperation in nearby regions, promote regional cooperation, jointly solve major problems in regional development, and effectively resolve existing common problems. For the eastern and western regions, we should strengthen the vertical integration of industries.
At present, the development of technological innovation capabilities of the biomedical industry in various regions of China has made great progress, but there are still practical problems such as low level of industrial innovation and a widening technological gap. From the perspective of high-quality development, this research has the following policy implications.
1) Enhance the ability to transform technological achievements in the biomedical industry, and promote the integration of technology and the market. The technological innovation achievements of enterprises must eventually flow into the market in the form of products in order to truly realize their value. From the results of this paper, for my country's biomedical industry, the low efficiency of technological achievement transformation is an important shortcoming that restricts the improvement of its overall technological innovation capability. Therefore, efforts to improve the transformation ability of industrial technological achievements can not only bring more economic benefits to the industry but also effectively improve the overall innovation efficiency of the biomedical industry. To this end, the biomedical industry should establish a special talent team and formulate a scientific and technological achievement transformation evaluation index system to make the enterprise's technological achievement transformation process more standardized and efficient. At the same time, the biomedical industry should pay attention to the analysis of the biomedical industry and the market value of the technological achievements themselves, actively promote the combination of technology and the market, and accelerate the scientific and technological progress of my country's biomedical industry.
2) Optimize the allocation of scientific and technological resources in the biomedical industry to avoid waste of scientific and technological resources. From the research results of this paper, some provinces and cities have the problem of redundancy in R&D investment, which indicates that the innovation resources of my country's biomedical industry have not been reasonably allocated. At present, my country's biomedical industrial parks and medicine valleys are in full swing, but their technological innovation levels are uneven, and some of the so-called "biomedical" companies are just simple drug processing plants, which violates my country's original intention of developing the biomedical industry. This has a certain relationship with government officials' blind policy study, which not only creates the illusion of rapid industrial development, but also wastes precious resources, making it difficult to achieve the healthy development of the biomedical industry. To this end, local government officials should reasonably judge whether the local area has the foundation and advantages to develop the biomedical industry according to their own capabilities and actual development needs. The biomedical industry can improve its own innovation efficiency by optimizing the allocation of innovation resources and scientifically coordinating the ratio of various input elements, so as to maximize the output of innovation resources invested by regional industries into technological innovation achievements.
3) Attach importance to inter-regional cooperation and promote the flow of innovation resources between regions. Due to the disparity in the levels of economic and technological development, as well as the differences in regional financial and policy environments, there is a clear gap between the biomedical industry in the central and western regions and the biomedical industry in the eastern region in terms of knowledge stock and technical level. This makes the technological innovation abilities of enterprises in the two regions obviously differentiated. The results of this study show that the innovation capability level of the biomedical industry in the eastern region is significantly higher than that in the western region in the stage of technology research and development, and there is a problem of redundant investment in research and development funds. In this regard, through cross-regional cooperation, the redundant innovation investment in the eastern region can be transferred to the central and western regions; so as to make up for the shortage of innovation resources in the central and western regions, and at the same time, it can also improve the overall utilization rate of innovation resources. The eastern region should also give full play to its own advantages of knowledge spillover and numerous talents, and strive to promote the transfer and transfer of related industries and professionals to the central and western regions, so as to drive the overall improvement of the innovation capability of my country's biomedical industry.
The author declares no conflict of interest.
[1] | X. Wen, H. Huang, X. Wang, W. Gao, S. O. Management, Construction of biomedical industry innovation index system, Sci. Technol. Manage. Res., 36 (2016), 42–46+52. |
[2] |
H. Andreas, Value chain orchestration in action and the case of the global agrochemical industry, Long Range Plann., 35 (2002), 615–635. https://doi.org/10.1016/S0024-6301(02)00160-7 doi: 10.1016/S0024-6301(02)00160-7
![]() |
[3] | K. Xu, L. H. Sun, Development status and problems of biomedical industrial parks in China, Chin. J. New Drugs, 28 (2019), 2440–2446. |
[4] | Y. Y. Li, S. X. Gao, Biomedical industry's developing situation based on statistical data from 1995 to 2015, China Sci. Technol. Forum, 12 (2016), 42–47+97. |
[5] |
J. Y. Lee, C. L. Kim, G. Choi, Exploring data envelopment analysis for measuring collaborated innovation efficiency of small and medium-sized enterprises in Korea, Eur. J. Oper. Res., 278 (2019), 533–545. https://doi.org/10.1016/j.ejor.2018.08.044 doi: 10.1016/j.ejor.2018.08.044
![]() |
[6] |
C. H. Kim, W. S. Shin, Natural resource dependence and economic growth: A TOPSIS/DEA analysis of innovation efficiency, Asian J. Shipp. Logist., 35 (2019), 70–76. https://doi.org/10.1016/j.ajsl.2019.03.010 doi: 10.1016/j.ajsl.2019.03.010
![]() |
[7] | X. Q. Huang, The competitiveness of Singapore's biomedical industry: An analysis based on diamond model, Southeast Asia, 2 (2011), 31–36. |
[8] | Z. Yin, Analysis of various factors affecting China's biomedical innovation strategy, Sci. Res., 1 (2008), 13–18. |
[9] | Y. Cao, L. Zhu, N. Y. Mao, An empirical study on the innovation ability of biomedical industry cluster based on multi-level grey evaluation model, Bus. Econ., 31 (2012), 94–97. |
[10] | Y. M. Zhu, X. Y. Xi, S. Z. Chu, Factors impacting formation and development of bio-pharmaceutical cluster in China, Chin. J. New Drugs, 22 (2013), 900–904. |
[11] | X. M. Fu, S. Q. Wang, X. H. Lin, Y. Liu, Innovation efficiency and influencing factors of China's Marnie biomedical industry based on SFA method, Sci. Technol. Manage. Res., 40 (2020), 202–208. |
[12] | K. Xu, L. H. Sun, Empirical study on the dynamic efficiency of China's biomedical medicine industrial park based on Malmquist model, J. Shenyang Pharm. Univ., 36 (2019), 534–538. |
[13] | K. Xu, L. H. Sun, Efficiency evaluation of China's biomedical medicine industrial parks based on three-stage DEA, Chin. J. New Drugs, 28 (2019), 646–650. |
[14] | F. Zhang, J. Z. Chen, Design and calculation of evaluation index system of regional industrial development potential, Stat. Decis., 14 (2013), 31–34. |
[15] | L. Q. Zhang, Z. C. He, Y. Wu, Evaluation of strategic emerging industries based on grey relational analysis: Empirical analysis on biology and medicine industry, Econ. Math., 27 (2010), 79–84. |
[16] | Y. W. Chen, Y. Li, J. Xu, Empirical study by factor analysis in biomedical industry development in China, Chin. Pharm., 22 (2011), 3844–3846. |
[17] | J. Q. Ding, J. Y. Bai, X. F. Lin, Performance evaluation of Chinese bio-industry listed companies based on DEA model, Progress Biochem. Biophys., 1 (2020), 1–12. |
[18] | Z. C. He, M. Zhang, Y. Wu, Research on efficiency of service towards generic technology in biophrmaceutical industry, China Soft Sci., 2 (2014), 130–139. |
[19] |
T. E. Stuart, S. Z. Ozdemir, W. W. Ding, Vertical alliance networks: The case of university–biotechnology-pharmaceutical alliance chains, Res. Policy, 36 (2007), 477–498. https://doi.org/10.1016/j.respol.2007.02.016 doi: 10.1016/j.respol.2007.02.016
![]() |
[20] |
B. Achilladelis, N. Antonakis, The dynamics of technological innovation: The case of the pharmaceutical industry, Res. Policy, 30 (2001), 535–588. https://doi.org/10.1016/S0048-7333(00)00093-7 doi: 10.1016/S0048-7333(00)00093-7
![]() |
[21] |
C. J. Chen, C. C. Huang, A multiple criteria evaluation of high-tech industries for the science-based industrial park in Taiwan, Inf. Manage-Amster, 41 (2004), 839–851. https://doi.org/10.1016/j.im.2003.02.002 doi: 10.1016/j.im.2003.02.002
![]() |
[22] | Y. Yang, H. J. Xue, Study on regional diversity of industrial technology independent innovation capability, China's Ind. Econ., 11 (2011), 68–76. |
[23] | J. Q. Zhang, The comparative study on technology innovation ability of high technology industry under two phase angle—the empirical analysis from the industry data, Sci. Technol. Prog. Policy, 33 (2016), 72–77. |
[24] |
C. Dou, X. Xiong, G. H. Chen, Muti-dimension study on SME's innovation efficiency from the perspective of innovation value Chain—based on two-stage additive decomposition DEA Model, Sci. Technol. Prog. Policy., 36 (2019), 77–85. https://doi.org/10.6049/kjjbydc.2018040237 doi: 10.6049/kjjbydc.2018040237
![]() |
[25] | S. Y. Yin, L. T. Chen, Research on technological innovation efficiency of Chinese pharmaceutical enterprises based on two-stage SFA model, Soft Sci., 30 (2016), 54–58. |
[26] | Y. Zheng, Z. H. Dong, L. J. Dong, Research on technological innovation efficiency and influencing factors of listed pharmaceutical companies in my country, Mod. Comm. Ind., 40 (2019), 12–16. |
[27] | S. X. Zhou, S. X. Yu, Y. Z. Wang, Evaluation and suggestions on innovation efficiency in the stage of technology R&D and achievement transformation of China's pharmaceutical manufacturing industry, Jiangxi Soc. Sci., 42 (2022), 145–153. |
[28] | Z. J. Yu, S. Z. Chu, Analysis of the correlation between the competitiveness of my country's pharmaceutical manufacturing industry and the ability of innovative drug research and development, China J. New Drugs, 27 (2018), 279–284. |
[29] | P. Z. Liu, A. X. Ma, A comparative study on the technological innovation capability of the pharmaceutical industry and its influencing factors, China Drug Eval., 32 (2015), 59–64. |
[30] |
K. Y. Wan, B. X. Du, J. H. Wang, Z. Guo, D. Feng, X. Gao, et al., Deep learning-based intelligent management for sewage treatment plants, J. Cent. South Univ., 29 (2022), 1537–1552. https://doi.org/10.1007/s11771-022-5036-3 doi: 10.1007/s11771-022-5036-3
![]() |
[31] | Z. A. Gao, X. C. Wang, Research on the influencing factors of money laundering risk in my country's free trade zones based on fuzzy cognitive map, Oper. Res. Manage., 31 (2022), 177–182. |
[32] |
S. Liu, Y. Yang, Y. Cao, N. Xie, A summary on the research of GRA models, Grey Syst.: Theory Appl., 3 (2013), 7–15. https://doi.org/10.1108/20439371311293651 doi: 10.1108/20439371311293651
![]() |
[33] | Y. G. Dang, X. Y. Zhu, S. Ding, J. J. Wang, Panel data clustering method based on grey correlation degree and its application in air pollution analysis, Cont. Decis., 32 (2017), 2227–2232. |
[34] | X. M. Li, Y. G. Dang, J. J. Wang, Grey index correlation clustering model and application under panel data, Cont. Decis., 30 (2015), 1447–1452. |
[35] | T. B. Jia, S. F. Liu, Research on organizational power allocation model based on grey relational clustering, Pract. Understand. Math., 46 (2016), 31–37. |
[36] |
B. J. Frey, D. Dueck, Clustering by passing messages between data points, Science, 315 (2007), 972–976. https://doi.org/10.1126/science.1136800 doi: 10.1126/science.1136800
![]() |
1. | Jaeho Shin, Yeongjun Kim, Jiyoon Son, Changhee Kim, Is There a Difference in Innovation Performance Depending on the Investment in Each Stage of Development Process? Evidence From Medical Device Industry, 2023, 11, 2169-3536, 92092, 10.1109/ACCESS.2023.3308154 | |
2. | Adrian Domenteanu, Georgiana-Alina Crișan, Corina Frăsineanu, Camelia Delcea, Exploring Grey Systems in Uncertain Environments: A Bibliometric Analysis of Global Contributions and Research Themes, 2025, 17, 2071-1050, 2764, 10.3390/su17062764 |