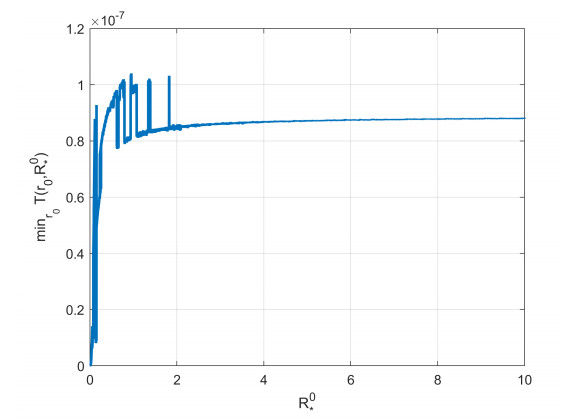
In the context of high-quality economic development in China, it is important to promote green innovation development by protecting intellectual property rights (IPR). Taking the pilot policy of the intellectual property courts in Beijing, Shanghai, and Guangzhou for example in a quasi-natural experiment, this article examines the effect of IPR protection on the development of corporate green innovation and its mechanisms by using a difference-in-differences model and a mediating effect model based on Chinese enterprise data from 2011 to 2019. The study found that first, IPR protection promotes enterprise green technological innovation; second, IPR protection affects green innovation through enterprise financing constraints and R&D investment; that is, increasing enterprise R&D investment and alleviating enterprise financing constraints are two important channels through which IPR protection promotes enterprise green technological innovation.
Citation: Yue Liu, Liming Chen, Han Luo, Yuzhao Liu, Yixian Wen. The impact of intellectual property rights protection on green innovation: A quasi-natural experiment based on the pilot policy of the Chinese intellectual property court[J]. Mathematical Biosciences and Engineering, 2024, 21(2): 2587-2607. doi: 10.3934/mbe.2024114
[1] | Shihe Xu, Junde Wu . Qualitative analysis of a time-delayed free boundary problem for tumor growth with angiogenesis and Gibbs-Thomson relation. Mathematical Biosciences and Engineering, 2019, 16(6): 7433-7446. doi: 10.3934/mbe.2019372 |
[2] | H. J. Alsakaji, F. A. Rihan, K. Udhayakumar, F. El Ktaibi . Stochastic tumor-immune interaction model with external treatments and time delays: An optimal control problem. Mathematical Biosciences and Engineering, 2023, 20(11): 19270-19299. doi: 10.3934/mbe.2023852 |
[3] | Yuting Ding, Gaoyang Liu, Yong An . Stability and bifurcation analysis of a tumor-immune system with two delays and diffusion. Mathematical Biosciences and Engineering, 2022, 19(2): 1154-1173. doi: 10.3934/mbe.2022053 |
[4] | Alessandro Bertuzzi, Antonio Fasano, Alberto Gandolfi, Carmela Sinisgalli . Interstitial Pressure And Fluid Motion In Tumor Cords. Mathematical Biosciences and Engineering, 2005, 2(3): 445-460. doi: 10.3934/mbe.2005.2.445 |
[5] | Jiaxin Nan, Wanbiao Ma . Stability and persistence analysis of a microorganism flocculation model with infinite delay. Mathematical Biosciences and Engineering, 2023, 20(6): 10815-10827. doi: 10.3934/mbe.2023480 |
[6] | Maria Vittoria Barbarossa, Christina Kuttler, Jonathan Zinsl . Delay equations modeling the effects of phase-specific drugs and immunotherapy on proliferating tumor cells. Mathematical Biosciences and Engineering, 2012, 9(2): 241-257. doi: 10.3934/mbe.2012.9.241 |
[7] | Marek Bodnar, Monika Joanna Piotrowska, Urszula Foryś . Gompertz model with delays and treatment: Mathematical analysis. Mathematical Biosciences and Engineering, 2013, 10(3): 551-563. doi: 10.3934/mbe.2013.10.551 |
[8] | Qiaoling Chen, Fengquan Li, Sanyi Tang, Feng Wang . Free boundary problem for a nonlocal time-periodic diffusive competition model. Mathematical Biosciences and Engineering, 2023, 20(9): 16471-16505. doi: 10.3934/mbe.2023735 |
[9] | Avner Friedman, Harsh Vardhan Jain . A partial differential equation model of metastasized prostatic cancer. Mathematical Biosciences and Engineering, 2013, 10(3): 591-608. doi: 10.3934/mbe.2013.10.591 |
[10] | Hui Cao, Dongxue Yan, Ao Li . Dynamic analysis of the recurrent epidemic model. Mathematical Biosciences and Engineering, 2019, 16(5): 5972-5990. doi: 10.3934/mbe.2019299 |
In the context of high-quality economic development in China, it is important to promote green innovation development by protecting intellectual property rights (IPR). Taking the pilot policy of the intellectual property courts in Beijing, Shanghai, and Guangzhou for example in a quasi-natural experiment, this article examines the effect of IPR protection on the development of corporate green innovation and its mechanisms by using a difference-in-differences model and a mediating effect model based on Chinese enterprise data from 2011 to 2019. The study found that first, IPR protection promotes enterprise green technological innovation; second, IPR protection affects green innovation through enterprise financing constraints and R&D investment; that is, increasing enterprise R&D investment and alleviating enterprise financing constraints are two important channels through which IPR protection promotes enterprise green technological innovation.
Over the past few decades, considerable attention has been paid to the rigorous analysis of mathematical models describing tumor growth and great progress has been achieved. Most work in this direction focuses on the sphere-shaped or nearly sphere-shaped tumor models; see [1,2,3,4,5,6,7,8,9] and the references therein. Observing that the work concerning models for tumors having different geometric configurations from spheroids is less frequent, in this paper we are interested in the situation of tumor cord–a kind of tumor that grows cylindrically around the central blood vessel and receives nutrient materials (such as glucose and oxygen) from the blood vessel [10]. The model only describes the evolution of the tumor cord section perpendicular to the length direction of the blood vessel due to the cord's uniformity in that direction. Assume that the radius of the blood vessel is r0 and denote by J and Γ(t) the section of the blood vessel wall and the section of the exterior surface of the tumor cord, respectively, then J={x∈R2;|x|=r0}. We also denote by Ω(t) the region of the section of the tumor cord, so that Ω(t) is an annular-like bounded domain in R2 and ∂Ω(t)=J∪Γ(t). The mathematical formulation of the tumor model under study is as follows:
cσt(x,t)−Δσ(x,t)+σ(x,t)=0,x∈Ω(t),t>0, | (1.1) |
−Δp(x,t)=μ[σ(ξ(t−τ;x,t),t−τ)−˜σ],x∈Ω(t),t>0, | (1.2) |
{dξds=−∇p(ξ,s),t−τ≤s≤t,ξ=x,s=t, | (1.3) |
σ(x,t)=ˉσ,∂→np(x,t)=0,x∈J,t>0, | (1.4) |
∂→νσ(x,t)=0,p(x,t)=γκ(x,t),x∈Γ(t),t>0, | (1.5) |
V(x,t)=−∂→νp(x,t),x∈Γ(t),t>0, | (1.6) |
Γ(t)=Γ0,−τ≤t≤0, | (1.7) |
σ(x,t)=σ0(x),x∈Ω0,−τ≤t≤0, | (1.8) |
p(x,t)=p0(x),x∈Ω0,−τ≤t≤0. | (1.9) |
Here, σ and p denote the nutrient concentration and pressure within the tumor, respectively, which are to be determined together with Ω(t), and c=Tdiffusion /Tgrowth is the ratio of the nutrient diffusion time scale to the tumor growth (e.g., tumor doubling) time scale; thus, it is very small and can sometimes be set to be 0 (quasi-steady state approximation). Assume that the time delay τ is reflected between the time at which a cell commences mitosis and the time at which the daughter cells are produced and ξ(s;x,t) represents the cell location at time s as cells are moving with the velocity field →V, then the function ξ(s;x,t) satisfies
{dξds=→V(ξ,s),t−τ≤s≤t,ξ|s=t=x. | (1.10) |
In other words, ξ tracks the path of the cell currently located at x. (1.3) is further derived from (1.10) under the assumption of a porous medium structure for the tumor, where Darcy's law →V=−∇p holds true. Because of the presence of time delay, the tumor grows at a rate that is related to the nutrient concentration when it starts mitosis and a combination of the conservation of mass and Darcy's law yields (1.2), in which μ represents the growth intensity of the tumor and ˜σ is the nutrient concentration threshold required for tumor cell growth. Additionally, ˉσ is the nutrient concentration in the blood vessel, ˉσ>˜σ, V, κ and →ν denote the normal velocity, the mean curvature and the unit outward normal field of the outer boundary Γ(t), respectively, →n denotes the unit outward normal field of the fixed inner boundary J, and γ is the outer surface tension coefficient. Thus, the boundary condition σ=ˉσ on J indicates that the tumor receives constant nutrient supply from the blood vessel, ∂→νσ=0 on Γ(t) implies that the nutrient cannot pass through Γ(t), ∂→np=0 on J means that tumor cells cannot pass through the blood vessel wall, p=γκ on Γ(t) is due to the cell-to-cell adhesiveness, and V=−∂→νp on Γ(t) is the well-known Stefan condition representing that the normal velocity of the tumor cord outer boundary Γ(t) is the same with that of tumor cells adjacent to Γ(t). Finally, σ0(x), p0(x), Γ0 are given initial data and Ω(t)=Ω0 for −τ≤t≤0.
Before going to our interest, we prefer to recall some relevant works. Models for the growth of the strictly cylindrical tumor cord were studied in [11,12,13]. For the model (1.1)–(1.9) without the time delay, if c=0, Zhou and Cui [14] showed that the unique radially symmetric stationary solution exists and is asymptotically stable for any sufficiently small perturbations. Meanwhile, if c>0, Wu et al. [15] proved that the stationary solution is locally asymptotically stable provided that c is small enough. On the other hand, Zhao and Hu [16] considered the multicell spheroids with time delays. For the case c=0, they analyzed the linear stability of the radially symmetric stationary solution as well as the impact of the time delay.
Motivated by the works [14,15,16], here we aim to discuss the linear stability of stationary solutions to the problem (1.1)–(1.9) with the quasi-steady-state assumption, i.e., c=0, and investigate the effect of time delay on tumor growth. Our first main result is given below.
Theorem 1.1. For small time delay τ, the problem (1.1)–(1.9) admits a unique radially symmetric stationary solution.
Next, in order to deal with the linear stability of the radially symmetric stationary solution, denoted by (σ∗,p∗,Ω∗), where Ω∗={x∈R2: r0<r=|x|<R∗}, we assume that the initial conditions are perturbed as follows:
Ω(t)={x∈R2:r0<r<R∗+ερ0(θ)},−τ≤t≤0,σ(r,θ,t)=σ∗(r)+εw0(r,θ),p(r,θ,t)=p∗(r)+εq0(r,θ),−τ≤t≤0. |
The linearized problem of (1.1)–(1.9) at (σ∗,p∗,Ω∗) is then obtained by substituting
Ω(t):r0<r<R∗+ερ(θ,t)+O(ε2), | (1.11) |
σ(r,θ,t)=σ∗(r)+εw(r,θ,t)+O(ε2), | (1.12) |
p(r,θ,t)=p∗(r)+εq(r,θ,t)+O(ε2) | (1.13) |
into (1.1)–(1.9) and collecting the ε-order terms. Now, we can state the second main result of this paper.
Theorem 1.2. For small time delay τ, the radially symmetric stationary solution (σ∗,p∗,Ω∗) of (1.1)–(1.9) with c=0 is linearly stable, i.e.,
max0≤θ≤2π|ρ(θ,t)|≤Ce−δt,t>0 | (1.14) |
for some positive constants C and δ.
Remark 1.1. Compared with results of the problem modeling the growth of tumor cord without time delays in [14], the introduction of the time delay does not affect the stability of the radially symmetric stationary solution even under non-radial perturbations. However, as we shall see in Subsection 3.3, the numerical result shows that adding time delay would result in a larger stationary tumor. Moreover, the stronger the growth intensity of the tumor is, the greater the influence of time delay on the size of the stationary tumor is.
Remark 1.2. Compared with results of the nearly sphere-shaped tumor model with time delays in [16], which state that the radially symmetric stationary solution is linearly stable for small μ in the sense that limt→∞max0≤θ≤2π|ρ(θ,t)−(a1cosθ+b1sinθ)|=0 for some constants a1 and b1, the radially symmetric stationary solution of tumor cord with the time delay is linearly stable for any μ>0 in the normal sense.
This paper is organized as follows. In Section 2, we give the proof of Theorem 1.1 by first transforming the free boundary problem into an equivalent problem with fixed boundary and then applying the contraction mapping principle combined with Lp estimates to this fixed boundary problem. In Section 3, we prove Theorem 1.2 and Remark 1.1 by first introducing the linearization of (1.1)–(1.9) at the radially symmetric stationary solution (σ∗,p∗,Ω∗), and then making a delicate analysis of the expansion in the time delay τ provided that τ is sufficiently small. A brief conclusion in Section 4 completes the paper.
In this section, we study radially symmetric stationary solutions (σ∗,p∗,Ω∗) to the system (1.1)–(1.9), which satisfy
−Δrσ∗(r)+σ∗(r)=0,σ∗(r0)=ˉσ,σ′∗(R∗)=0,r0<r<R∗, | (2.1) |
−Δrp∗(r)=μ[σ∗(ξ(−τ;r,0))−˜σ],p′∗(r0)=0,p∗(R∗)=γR∗,r0<r<R∗, | (2.2) |
{dξds(s;r,0)=−∂p∗∂r(ξ(s;r,0)),−τ≤s≤0,ξ(s;r,0)=r,s=0, | (2.3) |
∫R∗r0[σ∗(ξ(−τ;r,0))−˜σ]rdr=0, | (2.4) |
where Δr is the radial part of the Laplacian in R2.
Before proceeding further, let us recall that the modified Bessel functions Kn(r) and In(r), standard solutions of the equation
r2y′′+ry′−(r2+n2)y=0,r>0, | (2.5) |
have the following properties:
In+1(r)=In−1(r)−2nrIn(r),Kn+1(r)=Kn−1(r)+2nrKn(r),n≥1, | (2.6) |
I′n(r)=12[In−1(r)+In+1(r)],K′n(r)=−12[Kn−1(r)+Kn+1(r)],n≥1, | (2.7) |
I′n(r)=In−1(r)−nrIn(r), K′n(r)=−Kn−1(r)−nrKn(r),n≥1, | (2.8) |
I′n(r)=nrIn(r)+In+1(r), K′n(r)=nrKn(r)−Kn+1(r),n≥0, | (2.9) |
In(r)Kn+1(r)+In+1(r)Kn(r)=1r,n≥0 | (2.10) |
and
I′n(r)>0,K′n(r)<0. |
Proof of Theorem 1.1 In view of (2.5), the solution of (2.1) is clearly given by
σ∗(r)=ˉσI0(r)K1(R∗)+I1(R∗)K0(r)I0(r0)K1(R∗)+I1(R∗)K0(r0). | (2.11) |
Introducing the notations:
ˆr=r−r0R∗−r0,ˆσ(ˆr)=σ∗(r),ˆp(ˆr)=(R∗−r0)p∗(r),ˆξ(s,ˆr,0)=ξ(s,r,0)−r0R∗−r0, |
(2.1)–(2.4) reduces to the following system after dropping the "^" in the above variables:
∂2σ∂r2+R∗−r0r(R∗−r0)+r0∂σ∂r=(R∗−r0)2σ,σ(0)=ˉσ,σ′(1)=0, | (2.12) |
{∂2p∂r2+R∗−r0r(R∗−r0)+r0∂p∂r=−μ(R∗−r0)3[σ(r+1(R∗−r0)3∫0−τ∂p∂r((R∗−r0)ξ(s;r,0)+r0)ds)−˜σ],p′(0)=0,p(1)=γ(R∗−r0)R∗, | (2.13) |
{dξds(s;r,0)=−1(R∗−r0)3∂p∂r((R∗−r0)ξ(s;r,0)+r0),−τ≤s≤0,ξ(s;r,0)=r,s=0, | (2.14) |
∫10[r(R∗−r0)+r0][σ(r+1(R∗−r0)3∫0−τ∂p∂r((R∗−r0)ξ(s;r,0)+r0)ds)−˜σ]dr=0. | (2.15) |
It is clear that (2.12) can be solved explicitly. For convenience, we extend the solution of (2.12) outside [0, 1]:
σ(r;R∗)={ˉσI0(r(R∗−r0)+r0)K1(R∗)+I1(R∗)K0(r(R∗−r0)+r0)I0(r0)K1(R∗)+I1(R∗)K0(r0),0≤r≤1,ˉσI0(R∗)K1(R∗)+I1(R∗)K0(R∗)I0(r0)K1(R∗)+I1(R∗)K0(r0),1<r≤2. | (2.16) |
Assume that Rmin and Rmax are positive constants to be determined later and r0<Rmin<Rmax. For any R∗∈[Rmin,Rmax], we will prove that p is also uniquely determined by applying the contraction mapping principle.
Noticing that 0 is a lower solution of (2.14), but there is no assurance that ξ(s;r,0)≤1 for −τ≤s≤0, we suppose ξ(s;r,0)∈[0,2] and take
X={p∈W2,∞[0,2];‖p‖W2,∞[0,2]≤M}, |
where M>0 is to be determined. For each p∈X, we first solve for ξ from (2.14) and substitute it into (2.13), then the following system
{∂2ˉp∂r2+R∗−r0r(R∗−r0)+r0∂ˉp∂r=−μ(R∗−r0)3[σ(r+1(R∗−r0)3∫0−τ∂p∂r((R∗−r0)ξ(s;r,0)+r0)ds)−˜σ],ˉp(1)=γ(R∗−r0)R∗,∂ˉp∂r(0)=0 | (2.17) |
allows a unique solution ˉp∈W2,∞[0,1]. Applying the strong maximum principle combined with the Hopf lemma to (2.1) shows that σ(r;R∗)≤ˉσ. Thus, integrating (2.17), we obtain
‖1r(R∗−r0)+r0∂ˉp∂r‖L∞[0,1]≤μ2(Rmax−r0)2(ˉσ+˜σ), | (2.18) |
‖ˉp‖L∞[0,1]≤γ(Rmax−r0)Rmin+μ4R2max(Rmax−r0)(ˉσ+˜σ), | (2.19) |
‖∂2ˉp∂r2‖L∞[0,1]≤3μ2(Rmax−r0)3(ˉσ+˜σ). | (2.20) |
Define the mapping
Lp(r)={ˉp(r),0≤r≤1,ˉp(1)+ˉp′(1)(r−1),1<r≤2,p∈X, |
then ‖Lp‖∈W2,∞[0,2] and ‖Lp‖W2,∞[0,2]≤2‖ˉp‖W2,∞[0,1]. Combining (2.18)–(2.20), we find
‖Lp‖W2,∞[0,2]≤2{μ2(Rmax−r0)2(ˉσ+˜σ)+3μ2(Rmax−r0)3(ˉσ+˜σ)+γ(Rmax−r0)Rmin+μ4R2max(Rmax−r0)(ˉσ+˜σ)}≜ | (2.21) |
If we choose M \geq M_1 , then \mathcal{L}p \in X by (2.21) and \mathcal{L} maps X to itself.
We now show that \mathcal{L} is a contraction. Given p^{(1)}, p^{(2)} \in X , one can first get \xi^{(1)}, \xi^{(2)} from the following two systems:
\begin{align} \left\{\begin{array}{lc} \frac{\mathrm{d}\xi^{(1)}}{\mathrm{d}s}(s; r, 0) = -\frac{1}{(R_{*}-r_0)^3} \frac{\partial p^{(1)}}{\partial r}\left((R_{*}-r_0)\xi^{(1)}(s ; r, 0)+r_0\right), & -\tau \leq s \leq 0, \\ \xi^{(1)}(s; r, 0) = r, & s = 0, \end{array}\right. \end{align} | (2.22) |
\begin{align} \left\{\begin{array}{ll} \frac{\mathrm{d}\xi^{(2)}}{\mathrm{d}s}(s; r, 0) = -\frac{1}{(R_{*}-r_0)^3} \frac{\partial p^{(2)}}{\partial r}\left((R_{*}-r_0)\xi^{(2)}(s ; r, 0)+r_0\right), & -\tau \leq s \leq 0, \\ \xi^{(2)}(s; r, 0) = r, & s = 0. \end{array}\right. \end{align} | (2.23) |
Integrating (2.22) and (2.23) with regard to s over the interval [-\tau, 0] and making a subtraction yield
\begin{aligned} \left|\xi^{(1)}-\xi^{(2)}\right| \leq& \frac{\tau}{(R_{*}-r_0)^3}\max _{-\tau \leq s \leq 0 \atop 0 \leq r \leq 1}\bigg[\bigg|\frac{\partial p^{(1)}}{\partial r}((R_{*}-r_0)\xi^{(1)}+r_0)-\frac{\partial p^{(2)}}{\partial r}((R_{*}-r_0)\xi^{(1)}+r_0)\bigg| \\ &\; \; \; \; \; \; \; \; \; \; \; \; \; \; \; \; \; \; \; \; \; \; \; \; \; +\bigg|\frac{\partial p^{(2)}}{\partial r}((R_{*}-r_0)\xi^{(1)}+r_0)-\frac{\partial p^{(2)}}{\partial r}((R_{*}-r_0)\xi^{(2)}+r_0)\bigg|\bigg] \\ \leq& \frac{\tau}{(R_{*}-r_0)^3}\left\|p^{(1)}-p^{(2)}\right\|_{W^{2, \infty}[0,2]}+ \frac{\tau M}{(R_{*}-r_0)^2} \max _{-\tau \leq s \leq 0 \atop 0 \leq r \leq 1}\left|\xi^{(1)}-\xi^{(2)}\right| \end{aligned} |
for all -\tau \leq s \leq 0 and 0 \leq r \leq 1 . Consequently,
\begin{align} \max _{-\tau \leq s \leq 0 \atop 0 \leq r \leq 1}\left|\xi^{(1)}-\xi^{(2)}\right| \leq \frac{\tau}{(R_{*}-r_0)^3-\tau M(R_{*}-r_0)}\left\|p^{(1)}-p^{(2)}\right\|_{W^{2, \infty}[0,2]}. \end{align} | (2.24) |
Next, we substitute \xi^{(1)}, \xi^{(2)} into (2.17) and solve for \bar{p}^{(1)} and \bar{p}^{(2)} , respectively, then it follows from (2.17) that \left(\bar{p}^{(1)}-\bar{p}^{(2)}\right)(1) = 0 , \frac{\partial}{\partial r}\left(\bar{p}^{(1)}-\bar{p}^{(2)}\right)(0) = 0 and
\begin{align*} &-\frac{\partial^2 }{\partial r^2}(\bar{p}^{(1)}-\bar{p}^{(2)})-\frac{R_\ast-r_0}{r(R_\ast-r_0)+r_0} \frac{\partial }{\partial r}(\bar{p}^{(1)}-\bar{p}^{(2)})\nonumber \\ = &\mu (R_\ast-r_0)^3\bigg[\sigma\bigg(r+\frac{1}{(R_\ast-r_0)^3}\int^0_{-\tau}\frac{\partial p^{(1)}}{\partial r}((R_\ast-r_0)\xi^{(1)}(s;r,0)+r_0)ds\bigg) \\ &\; \; \; \; \; \; \; \; \; \; \; \; \; \; \; -\sigma\bigg(r+\frac{1}{(R_\ast-r_0)^3}\int^0_{-\tau}\frac{\partial p^{(2)}}{\partial r}((R_\ast-r_0)\xi^{(2)}(s;r,0)+r_0)ds\bigg)\bigg]. \end{align*} |
Using (2.24), we derive
\begin{align*} &\left\|\frac{1}{r(R_\ast-r_0)+r_0}\frac{\partial}{\partial r}\left(\bar{p}^{(1)}-\bar{p}^{(2)}\right)\right\|_{L^{\infty}[0,1]} \\ \leq& \frac{\mu}{2} (R_{*}-r_0)^2\bigg\|\sigma\bigg(r+\frac{1}{(R_\ast-r_0)^3}\int^0_{-\tau}\frac{\partial p^{(1)}}{\partial r}((R_\ast-r_0)\xi^{(1)}+r_0)ds\bigg) \\ &\; \; \; \; \; \; \; \; \; \; \; \; \; \; \; \; \; \; \; -\sigma\bigg(r+\frac{1}{(R_\ast-r_0)^3}\int^0_{-\tau}\frac{\partial p^{(2)}}{\partial r}((R_\ast-r_0)\xi^{(2)}+r_0)ds\bigg)\bigg\|_{L^{\infty}[0,1]} \\ \leq& \frac{\mu}{2(R_{*}-r_0)}\left\|\frac{\partial \sigma}{\partial r}\right\|_{L^{\infty}[0,2]} \int_{-\tau}^{0}\left(\frac{\partial p^{(1)}}{\partial r}((R_\ast-r_0)\xi^{(1)}+r_0)-\frac{\partial p^{(2)}}{\partial r}((R_\ast-r_0)\xi^{(2)}+r_0)\right)ds \\ \leq & \frac{\mu\tau}{2(R_\ast-r_0)}\left\|\frac{\partial \sigma}{\partial r}\right\|_{L^{\infty}[0,2]} \left(\left\|p^{(1)}-p^{(2)}\right\|_{W^{2, \infty}[0,2]}+(R_\ast-r_0)\left\|p^{(2)}\right\|_{W^{2, \infty}[0,2]} \max _{-\tau \leq s \leq 0 \atop 0 \leq r \leq 1}\left|\xi^{(1)}-\xi^{(2)}\right|\right) \\ \leq& M_2 \tau\left\|p^{(1)}-p^{(2)}\right\|_{W^{2, \infty}[0,2]} \end{align*} |
and similarly,
\begin{array}{c} \left\|\bar{p}^{(1)}-\bar{p}^{(2)}\right\|_{L^{\infty}[0,1]} \leq M_3 \tau\left\|p^{(1)}-p^{(2)}\right\|_{W^{2, \infty}[0,2]}, \\ \left\|\frac{\partial^{2}}{\partial r^{2}}\left(\bar{p}^{(1)}-\bar{p}^{(2)}\right)\right\|_{L^{\infty}[0,1]} \leq M_4 \tau\left\|p^{(1)}-p^{(2)}\right\|_{W^{2, \infty}[0,2]}, \end{array} |
where
\begin{align*} M_2& = \frac{\mu\bar{\sigma}R_{\max}}{2r_0}\frac{(R_{\max}-r_0)^3}{(R_{\min}-r_0)^2- M \tau}, \\ M_3& = \frac{\mu \bar{\sigma}R^3_{\max}}{4r_0}\frac{(R_{\max}-r_0)^2}{(R_{\min}-r_0)^2-M \tau},\\ M_4& = \frac{3\mu\bar{\sigma}R_{\max}}{2r_0}\frac{(R_{\max}-r_0)^4}{(R_{\min}-r_0)^2-M \tau}. \end{align*} |
Here, we employed the fact that
\begin{align*} &\left\|\frac{\partial \sigma}{\partial r}\right\|_{L^{\infty}[0,2]} = \left\|\frac{\partial \sigma}{\partial r}\right\|_{L^{\infty}[0,1]} \leq\frac{\bar{\sigma}R_{\max}}{r_0}(R_{\max}-r_0)^2 \end{align*} |
by (2.1) and \sigma\leq\bar{\sigma} . Let M_5 = M_2+M_3+M_4 , then M_5 is independent of \tau and
\begin{equation*} \left\|\bar{p}^{(1)}-\bar{p}^{(2)}\right\|_{W^{2, \infty}[0,1]} \leq M_5 \tau\left\|p^{(1)}-p^{(2)}\right\|_{W^{2, \infty}[0,2]}, \end{equation*} |
which together with \mathcal{L}p^{(1)}(1) = \mathcal{L}p^{(2)}(1) = \frac{\gamma(R_\ast-r_0)}{R_\ast} and (\mathcal{L}p^{(1)})^\prime(0) = (\mathcal{L}p^{(2)})^\prime(0) = 0 implies that
\begin{equation*} \left\|\mathcal{L}p^{(1)}-\mathcal{L}p^{(2)}\right\|_{W^{2, \infty}[0,2]}\leq 2 M_5 \tau\left\|p^{(1)}-p^{(2)}\right\|_{W^{2, \infty}[0,2]}. \end{equation*} |
Hence, if \tau is sufficiently small such that 2M_5 \tau < 1 , then we derive a contracting mapping \mathcal{L} . The existence and uniqueness of p are therefore obtained.
It suffices to prove that there exists a unique R_\ast \in[R_{\min}, R_{\max}] satisfying (2.15). Substituting (2.16) into (2.15), we find that it is equivalent to solving the following equation for R :
\begin{align*} G(R,\tau) = &\int^1_0\frac{r(R-r_0)+r_0}{R+r_0}\bigg[\sigma_\ast\bigg(r+\frac{1}{(R-r_0)^3} \\ &\; \; \; \; \; \; \; \; \; \; \; \; \; \; \; \; \; \; \; \; \; \; \; \; \; \; \; \; \; \; \; \; \; \; \; \; \; \int^0_{-\tau}\frac{\partial p_\ast}{\partial r}((R-r_0)\xi(s;r,0) +r_0)ds\bigg)-\tilde{\sigma}\bigg]dr = 0. \end{align*} |
Clearly,
\begin{align*} G(R,0)& = \int^1_0\bigg(\sigma_\ast(r;R)-\tilde{\sigma}\bigg)\frac{r(R-r_0)+r_0}{R+r_0}dr \\ & = \int^1_0\sigma_\ast(r;R)\frac{r(R-r_0)+r_0}{R+r_0}dr-\frac{\tilde{\sigma}}{2}. \end{align*} |
Using Lemma 3.1 and Theorem 3.2 in [14] and the condition \bar{\sigma} > \tilde{\sigma} , we know that
\begin{equation*} \lim\limits _{R \rightarrow r_{0}} G(R,0) = \frac{\bar{\sigma}-\tilde{\sigma}}{2} > 0, \quad \lim \limits_{R \rightarrow \infty} G(R,0) = -\frac{\tilde{\sigma}}{2} < 0, \quad \frac{\partial G(R,0)}{\partial R} < 0, \label{ZC-34} \end{equation*} |
which implies that the equation G(R, 0) = 0 has a unique solution, denoted by R_S , and
G\left(\frac{1}{2}(R_S+r_0), 0\right) > 0, \quad G\left(\frac{3}{2} R_S, 0\right) < 0. |
Since
\begin{equation*} \frac{\partial G(R, \tau)}{\partial R} = \frac{\partial G(R, 0)}{\partial R}+\frac{\partial^{2} G(R, \eta)}{\partial R \partial \tau} \tau+O(\tau^2), \qquad \qquad 0 \leq \eta \leq \tau \end{equation*} |
when \tau is sufficiently small, \frac{\partial G(R, \tau)}{\partial R} and \frac{\partial G(R, 0)}{\partial R} have the same sign. Thus, G(R, \tau) is monotone decreasing in R . Using the fact that G(R, \tau) is continuous in \tau , we further have
G\left(\frac{1}{2} (R_S+r_0), \tau\right) > 0, \quad G\left(\frac{3}{2} R_S, \tau\right) < 0. |
Hence, when \tau is sufficiently small, the equation G(R, \tau) = 0 has a unique solution R_\ast . Taking R_{\min } = \frac{1}{2} (R_{S}+r_0) and R_{\max } = \frac{3}{2} R_{S} , we complete the proof of the theorem.
This section is devoted to the linear stability of the radially symmetric stationary solution (\sigma_\ast, p_\ast, \Omega_\ast) of the problem (1.1)–(1.9) and the effect of time delay on the stability and the size of the stationary tumor. Let (\sigma, p, \Omega(t)) , given by (1.11)–(1.13), be solutions to (1.1)–(1.9), and denote by \vec{e}_r , \vec{e}_\theta the unit normal vectors in r , \theta directions, respectively. Written in the rectangular coordinates in \mathbb{R}^2 ,
\vec{e}_r = (\cos\theta,\sin\theta)^T,\quad \vec{e}_\theta = (-\sin\theta,\cos\theta)^T. |
Using the notation \xi_1(s; r, \theta, t) , \xi_2(s; r, \theta, t) for the polar radius and angle of \xi(s; r, \theta, t) , respectively, we have
\begin{equation*} \xi(s;r,\theta,t) = \xi_1(s; r, \theta, t)\vec{e}_r(\xi) = \xi_1(s; r, \theta, t)(\cos\xi_2(s;r,\theta,t),\sin\xi_2(s;r,\theta,t))^T. \end{equation*} |
Expand \xi_1 , \xi_2 in \varepsilon as
\begin{align} & \left\{ \begin{aligned} &\xi_1 = \xi_{10}+\varepsilon\xi_{11}+O(\varepsilon^2), \\ &\xi_2 = \xi_{20}+\varepsilon\xi_{21}+O(\varepsilon^2), \end{aligned} \right. \end{align} | (3.1) |
then we derive from (1.3) and (1.13) that
\begin{align} &\left\{\begin{array}{l} \frac{\mathrm{d}\xi_{10}}{\mathrm{d}s} = -\frac{\partial p_\ast}{\partial r}(\xi_{10}),\quad t-\tau\le s\le t,\\ \xi_{10}\big|_{s = t} = r; \end{array}\right. \end{align} | (3.2) |
\begin{align} &\left\{\begin{array}{l} \frac{\mathrm{d}\xi_{11}}{\mathrm{d}s} = -\frac{\partial^2 p_\ast}{\partial r^2}(\xi_{10})\xi_{11} -\frac{\partial q}{\partial r}(\xi_{10},\xi_{20},s),\quad t-\tau\le s\le t,\\ \xi_{11}\big|_{s = t} = 0; \end{array}\right. \end{align} | (3.3) |
\begin{align} &\left\{\begin{array}{l} \frac{\mathrm{d}\xi_{20}}{\mathrm{d}s} = 0,\quad t-\tau\le s\le t,\\ \xi_{20}\big|_{s = t} = \theta; \end{array}\right. \end{align} | (3.4) |
\begin{align} &\left\{\begin{array}{l} \frac{\mathrm{d}\xi_{21}}{\mathrm{d}s} = -\frac1{\xi_{10}^2}\frac{\partial q}{\partial\theta}(\xi_{10},\xi_{20},s),\quad t-\tau\le s\le t,\\ \xi_{21}\big|_{s = t} = 0. \end{array}\right. \end{align} | (3.5) |
It is evident that \xi_{20}\equiv\theta . Noticing that the equation for \xi_{10} is the same as that for \xi_\ast in the radially symmetric case, \xi_{10} is independent of \theta .
Substituting (1.11)–(1.13) and (3.1)–(3.5) into (1.1), (1.2), (1.4)–(1.6), using the mean-curvature formula in the 2-dimensional case for the curve r = \rho(\theta) :
\kappa = \frac{\rho^2+2\rho_\theta^2-\rho\cdot\rho_{\theta \theta}}{(\rho^2+(\rho_\theta)^2)^{3/2}} |
and collecting the \varepsilon -order terms, we obtain the linearized system in B_{R_\ast}\times\{t > 0\} :
\begin{align} &\Delta\omega(r,\theta,t) = \omega(r,\theta,t),\quad \omega(r_0, \theta, t) = 0,\quad\frac{\partial \omega}{\partial r}(R_\ast, \theta, t)+\sigma_\ast(R_\ast) \rho(\theta, t) = 0, \end{align} | (3.6) |
\begin{align} &\left\{\begin{array}{l} \Delta q(r,\theta,t) = -\mu\frac{\partial \sigma_\ast}{\partial r}(\xi_{10}(t-\tau;r,t))\xi_{11}(t-\tau;r,\theta,t)-\mu w(\xi_{10}(t-\tau;r,t),\theta,t-\tau),\\ \frac{\partial q}{\partial r}(r_0,\theta,t) = 0,\quad q(R_\ast, \theta, t)+\frac{\gamma}{R_\ast^2}\bigg(\rho(\theta, t)+\frac{\partial^2 \rho}{\partial \theta^2}(\theta, t)\bigg) = 0, \end{array}\right. \end{align} | (3.7) |
\begin{align} &\frac{\partial \rho(\theta, t)}{\partial t} = -\frac{\partial q}{\partial r}(R_\ast, \theta, t)-\frac{\partial^2 p_\ast}{\partial r^2}(R_\ast, \theta, t) \rho(\theta, t). \end{align} | (3.8) |
Due to the presence of the time delay, the linearization problem (3.6)–(3.8) cannot be solved explicitly. Assume that \omega, q , \rho and \xi_{11} have the following Fourier expansions:
\begin{align} \left\{\begin{array}{lc} \omega(r, \theta, t) = A_0(r, t)+\sum_{n = 1}^\infty[A_n(r, t) \cos n \theta+B_n(r, t) \sin n \theta], \\ q(r, \theta, t) = E_0(r, t)+\sum_{n = 1}^\infty[E_n(r, t) \cos n \theta+F_n(r, t) \sin n \theta], \\ \rho(\theta, t) = a_0(t)+\sum_{n = 1}^\infty[a_n(t) \cos n \theta+b_n(t) \sin n \theta],\\ \xi_{11}(s;r,\theta,t) = e_0(s;r,t)+\sum_{n = 1}^\infty[e_n(s;r,t) \cos n \theta+f_n(s;r,t) \sin n \theta]. \end{array}\right. \end{align} | (3.9) |
Substituting (3.9) into (3.6)–(3.8) yields the following system in B_{R_\ast}\times\{t > 0\} :
\begin{align} &\left\{\begin{array}{l} \frac{\partial^2 A_n}{\partial r^2}(r, t)+\frac{1}{r} \frac{\partial A_n}{\partial r}(r, t)-\frac{n^2}{r^2} A_n(r, t) = A_n(r, t), \\ A_n(r_0, t) = 0, \quad \frac{\partial A_n}{\partial r}(R_\ast, t)+\sigma_\ast(R_\ast) a_n(t) = 0, \end{array}\right. \end{align} | (3.10) |
\begin{align} &\left\{\begin{array}{l} \frac{\partial^2 B_n}{\partial r^2}(r, t)+\frac{1}{r} \frac{\partial B_n}{\partial r}(r, t)-\frac{n^2}{r^2} B_n(r, t) = B_n(r, t), \\ B_n(r_0, t) = 0, \quad \frac{\partial B_n}{\partial r}(R_\ast, t)+\sigma_\ast(R_\ast) b_n(t) = 0, \end{array}\right. \end{align} | (3.11) |
\begin{align} &\left\{\begin{array}{l} \frac{\partial^2 E_n}{\partial r^2}(r, t)+\frac{1}{r} \frac{\partial E_n}{\partial r}(r, t)-\frac{n^2}{r^2} E_n(r, t)\\ \qquad = -\mu\frac{\partial \sigma_\ast}{\partial r}(\xi_{10}(t-\tau;r,t))e_n(t-\tau;r,t)-\mu A_n(\xi_{10}(t-\tau;r,t),t-\tau), \\ \frac{\partial E_n}{\partial r}(r_0, t) = 0, \quad E_n(R_\ast, t)+\frac{\gamma(1-n^2)}{R_\ast^2} a_n(t) = 0, \end{array}\right. \end{align} | (3.12) |
\begin{align} &\left\{\begin{array}{l} \frac{\partial^2 F_n}{\partial r^2}(r, t)+\frac{1}{r} \frac{\partial F_n}{\partial r}(r, t)-\frac{n^2}{r^2} F_n(r, t)\\ \qquad = -\mu\frac{\partial \sigma_\ast}{\partial r}(\xi_{10}(t-\tau;r,t))f_n(t-\tau;r,t) -\mu B_n(\xi_{10}(t-\tau;r,t),t-\tau), \\ \frac{\partial F_n}{\partial r}(r_0, t) = 0, \quad F_n(R_\ast, t)+\frac{\gamma(1-n^2)}{R_\ast^2} b_n(t) = 0, \end{array}\right. \end{align} | (3.13) |
\begin{align} &\left\{\begin{array}{l} \frac{\partial e_n}{\partial s}(s;r,t) = -\frac{\partial^2 p_\ast}{\partial r^2}(\xi_{10})e_n(s;r,t)-\frac{\partial E_n}{\partial r}(\xi_{10},s), \quad t-\tau\leq s\leq t, \\ e_n\mid_{s = t} = 0, \end{array}\right. \end{align} | (3.14) |
\begin{align} &\left\{\begin{array}{l} \frac{\partial f_n}{\partial s}(s;r,t) = -\frac{\partial^2 p_\ast}{\partial r^2}(\xi_{10})f_n(s;r,t)-\frac{\partial F_n}{\partial r}(\xi_{10},s), \quad t-\tau\leq s\leq t, \\ f_n\mid_{s = t} = 0, \end{array}\right. \end{align} | (3.15) |
\begin{align} &\frac{\mathrm{d}a_n(t)}{\mathrm{d}t} = -\frac{\partial^2 p_\ast}{\partial r^2}(R_\ast)a_n(t)-\frac{\partial E_n}{\partial r}(R_\ast,t), \end{align} | (3.16) |
\begin{align} &\frac{\mathrm{d}b_n(t)}{\mathrm{d}t} = -\frac{\partial^2 p_\ast}{\partial r^2}(R_\ast)b_n(t)-\frac{\partial F_n}{\partial r}(R_\ast,t). \end{align} | (3.17) |
Since it is impossible to solve the systems (2.1)–(2.4) and (3.10)–(3.17) explicitly and the time delay \tau is actually very small, in what follows, we analyze the expansion in \tau for (2.1)–(2.4) and (3.10)–(3.17).
Let
\begin{align*} R_\ast& = R_\ast^0+\tau R_\ast^1+O(\tau^2), \\ \sigma_\ast& = \sigma_\ast^0+\tau \sigma_\ast^1+O(\tau^2), \\ p_\ast& = p_\ast^0+\tau p_\ast^1+O(\tau^2),\\ A_n& = A_n^0+\tau A_n^1+O(\tau^2), \\ B_n& = B_n^0+\tau B_n^1+O(\tau^2), \\ E_n& = E_n^0+\tau E_n^1+O(\tau^2), \\ F_n& = F_n^0+\tau F_n^1+O(\tau^2), \\ a_n& = a_n^0+\tau a_n^1+O(\tau^2), \\ b_n& = b_n^0+\tau b_n^1+O(\tau^2). \end{align*} |
Substitute these expansions into (2.1)–(2.4) and (3.10)–(3.17). Since a_n(t) and b_n(t) have the same asymptotic behavior at \infty , we will only make an analysis of a_n(t) . For this, we discuss the expansions of R_\ast , \sigma_\ast , p_\ast , A_n , E_n and a_n . Since the equations for the expansions of \sigma_\ast , p_\ast , A_n , E_n and a_n are the same as those in [16], here we only compute the expansions of the boundary conditions of \sigma_\ast , p_\ast , A_n and E_n .
● Expansions of the boundary conditions of \sigma_\ast :
It follows from (2.10) and (2.11) that
\begin{align*} \sigma_\ast(r) = &\bar{\sigma}\frac{K_1(R_\ast)I_0(r)+I_1(R_\ast)K_0(r)}{I_0(r_0)K_1(R_\ast)+I_1(R_\ast)K_0(r_0)} \\ = &\bar{\sigma}\frac{K_1(R^0_\ast)I_0(r)+I_1(R^0_\ast)K_0(r)}{I_0(r_0)K_1(R^0_\ast)+I_1(R^0_\ast)K_0(r_0)} +\tau\frac{\bar{\sigma}R^1_\ast}{R^0_\ast} \frac{I_0(r_0)K_0(r)-K_0(r_0)I_0(r)}{[I_0(r_0)K_1(R^0_\ast)+I_1(R^0_\ast)K_0(r_0)]^2}+O(\tau^2), \end{align*} |
which implies
\begin{align} &\sigma^0_\ast(r) = \bar{\sigma}\frac{K_1(R^0_\ast)I_0(r)+I_1(R^0_\ast)K_0(r)}{I_0(r_0)K_1(R^0_\ast)+I_1(R^0_\ast)K_0(r_0)}, \end{align} | (3.18) |
\begin{align} &\sigma^1_\ast(r) = \frac{\bar{\sigma}R^1_\ast}{R^0_\ast}\frac{I_0(r_0)K_0(r)-K_0(r_0)I_0(r)}{[I_0(r_0)K_1(R^0_\ast)+I_1(R^0_\ast)K_0(r_0)]^2}. \end{align} | (3.19) |
By the boundary conditions in (2.1) , we find
\begin{align*} &\sigma^0_\ast(r_0)+\tau\sigma^1_\ast(r_0)+O(\tau^2) = \bar{\sigma}, \\ &\frac{\partial \sigma^0_\ast}{\partial r}(R^0_\ast)+\tau\frac{\partial^2 \sigma^0_\ast}{\partial r^2}(R^0_\ast) R^1_\ast+\tau\frac{\partial \sigma^1_\ast}{\partial r}(R^0_\ast)+O(\tau^2) = 0. \end{align*} |
● Expansions of the boundary conditions of p_\ast :
One obtains from the boundary conditions in (2.2) that
\begin{align*} &\frac{\partial p^0_\ast}{\partial r}(r_0)+\tau\frac{\partial p^1_\ast}{\partial r}(r_0)+O(\tau^2) = 0,\\ &p_\ast^0(R_\ast^0)+\tau\frac{\partial p_\ast^0}{\partial r}(R_\ast^0)R_\ast^1+\tau p_\ast^1(R_\ast^0)+O(\tau^2) = \frac{\gamma}{R_\ast^0}-\tau \frac{\gamma R_\ast^1}{(R_\ast^0)^2}+O(\tau^2). \end{align*} |
● Expansion of (2.4):
In view of (4.31) in [16], there holds
\begin{align} 0& = \int_{r_0}^{R_\ast}[\sigma_\ast(\xi(-\tau; r, 0))-\widetilde{\sigma}]rdr \\ & = \int_{r_0}^{R_\ast}[\sigma_\ast^0(r)-\widetilde{\sigma}] rdr+\tau \int_{r_0}^{R_\ast^0}\left(\frac{\partial \sigma_\ast^0}{\partial r}(r)\frac{\partial p_\ast^0}{\partial r}(r)+\sigma_\ast^1(r)\right)rdr+O(\tau^2). \end{align} | (3.20) |
Using (3.18), we compute
\begin{align} \int_{r_0}^{R_\ast}[\sigma_\ast^0(r)-\widetilde{\sigma}] rdr = &\int_{r_0}^{R_\ast}\left(\bar{\sigma}\frac{K_1(R^0_\ast)I_0(r)+I_1(R^0_\ast)K_0(r)}{I_0(r_0)K_1(R^0_\ast)+I_1(R^0_\ast)K_0(r_0)}-\widetilde{\sigma}\right) rdr \\ = &\bar{\sigma}r_0\frac{I_1(R^0_\ast)K_1(r_0)-I_1(r_0)K_1(R^0_\ast)}{I_0(r_0)K_1(R^0_\ast)+I_1(R^0_\ast)K_0(r_0)} +\frac{\widetilde{\sigma}}{2}[r_0^2-(R^0_\ast)^2]\\ &+\tau R^1_\ast\bigg(\frac{\bar{\sigma}}{I_0(r_0)K_1(R^0_\ast)+I_1(R^0_\ast)K_0(r_0)}-\tilde{\sigma}R^0_\ast\bigg)+O(\tau^2). \end{align} | (3.21) |
A combination of (3.20) and (3.21) gives
\begin{align} &\tau\bigg[\frac{\bar{\sigma}R^1_\ast}{I_0(r_0)K_1(R^0_\ast)+I_1(R^0_\ast)K_0(r_0)}-\tilde{\sigma}R^0_\ast R^1_\ast+\int^{R^0_\ast}_{r_0}\left(\frac{\partial \sigma^0_\ast}{\partial r}(r)\frac{\partial p^0_\ast}{\partial r}(r)+\sigma^1_\ast(r)\right)rdr\bigg] \\ &\qquad+\bar{\sigma}r_0\frac{I_1(R^0_\ast)K_1(r_0)-I_1(r_0)K_1(R^0_\ast)}{I_0(r_0)K_1(R^0_\ast)+I_1(R^0_\ast)K_0(r_0)} +\frac{\widetilde{\sigma}}{2}[r_0^2-(R^0_\ast)^2]+O(\tau^2) = 0. \end{align} | (3.22) |
● Expansions of the boundary conditions of A_n :
We derive from the boundary conditions in (3.10) that
\begin{align*} &A^0_n(r_0,t)+\tau A^1_n(r_0,t)+O(\tau^2) = 0, \\ &0 = \frac{\partial A^0_n}{\partial r}(R^0_\ast+\tau R^1_\ast,t)+\tau\frac{\partial A^1_n}{\partial r}(R^0_\ast,t)+[\sigma^0_\ast(R^0_\ast+\tau R^1_\ast)+\tau\sigma^1_\ast(R^0_\ast)][a^0_n(t)+\tau a^1_n(t)]+O(\tau^2) \\ &\quad = \frac{\partial A^0_n}{\partial r}(R^0_\ast,t)+\sigma^0_\ast(R^0_\ast)a^0_n(t)+\tau\bigg(\frac{\partial^2 A^0_n}{\partial r^2}(R^0_\ast,t)R^1_\ast+\frac{\partial A^1_n}{\partial r}(R^0_\ast,t) +\frac{\partial \sigma^0_\ast}{\partial r}(R^0_\ast,t)R^1_\ast a^0_n(t) \\ &\; \; \; \; \; \; \; \; \; \; \; \; \; \; \; \; \; \; \; \; \; \; \; \; \; \; \; \; \; \; \; \; \; \; \; \; \; \; \; \; \; \; \; \qquad+\sigma^0_\ast(R^0_\ast)a^1_n(t)+\sigma^1_\ast(R^0_\ast)a^0_n(t)\bigg)+O(\tau^2). \end{align*} |
● Expansions of the boundary conditions of E_n :
Substituting the expansion of E_n into the boundary conditions in (3.12) yields
\begin{align*} &\frac{\partial E^0_n}{\partial r}(r_0,t)+\tau\frac{\partial E^1_n}{\partial r}(r_0,t)+O(\tau^2) = 0, \\ &0 = E^0_n(R_\ast^0+\tau R_\ast^1,t)+\tau E^1_n(R_\ast^0,t)+\gamma\frac{1-n^2}{(R_\ast^0+\tau R_\ast^1)^2}[a^0_n(t)+\tau a^1_n(t)]+O(\tau^2) \\ &\quad = E^0_n(R_\ast^0,t)+\gamma\frac{1-n^2}{(R_\ast^0)^2}a^0_n(t)+\tau\bigg(\frac{\partial E^0_n}{\partial r}(R_\ast^0,t)R_\ast^1+E^1_n(R_\ast^0,t) \\ &\; \; \; \; \; \; \; \; \; \; \; \; \; \; \; \; \; \; \; \; \; \; \; \; \; \; \; \; \qquad-2\gamma\frac{1-n^2}{(R_\ast^0)^3}R_\ast^1a^0_n(t)+ \gamma\frac{1-n^2}{(R_\ast^0)^2}a^1_n(t)\bigg)+O(\tau^2). \end{align*} |
Collecting all zeroth-order terms in \tau leads to the following system for r_0 < r < R^0_\ast :
\begin{align} &-\frac{\partial^2 \sigma^0_\ast}{\partial r^2}-\frac{1}{r}\frac{\partial \sigma^0_\ast}{\partial r} = -\sigma^0_\ast, \qquad \sigma^0_\ast(r_0) = \bar{\sigma}, \qquad \frac{\partial \sigma^0_\ast}{\partial r}(R_\ast^0) = 0, \end{align} | (3.23) |
\begin{align} &-\frac{\partial^2 p^0_\ast}{\partial r^2}-\frac{1}{r}\frac{\partial p^0_\ast}{\partial r} = \mu(\sigma^0_\ast-\tilde{\sigma}), \quad \frac{\partial p^0_\ast}{\partial r}(r_0) = 0, \quad p^0_\ast(R^0_\ast) = \frac{\gamma}{R^0_\ast}, \end{align} | (3.24) |
\begin{align} &\bar{\sigma}r_0\frac{I_1(R^0_\ast)K_1(r_0)-I_1(r_0)K_1(R^0_\ast)}{I_0(r_0)K_1(R^0_\ast)+I_1(R^0_\ast)K_0(r_0)} +\frac{\widetilde{\sigma}}{2}[r_0^2-(R^0_\ast)^2] = 0, \end{align} | (3.25) |
\begin{align} &\left\{\begin{array}{l} -\frac{\partial^2 A^0_n}{\partial r^2}-\frac{1}{r}\frac{\partial A^0_n}{\partial r}+\bigg(\frac{n^2}{r^2}+1\bigg)A^0_n = 0, \\ A^0_n(r_0,t) = 0,\quad\frac{\partial A^0_n}{\partial r}(R^0_\ast,t)+\sigma^0_\ast(R^0_\ast)a^0_n(t) = 0, \end{array}\right. \end{align} | (3.26) |
\begin{align} &\left\{\begin{array}{l} -\frac{\partial^2 B^0_n}{\partial r^2}-\frac{1}{r}\frac{\partial B^0_n}{\partial r}+\bigg(\frac{n^2}{r^2}+1\bigg)B^0_n = 0, \\ B^0_n(r_0,t) = 0,\quad\frac{\partial B^0_n}{\partial r}(R^0_\ast,t)+\sigma^0_\ast(R^0_\ast)b^0_n(t) = 0, \end{array}\right. \end{align} | (3.27) |
\begin{align} &\left\{\begin{array}{l} -\frac{\partial^2 E^0_n}{\partial r^2}-\frac{1}{r}\frac{\partial E^0_n}{\partial r}+\frac{n^2}{r^2}E^0_n = \mu A^0_n, \\ \frac{\partial E^0_n}{\partial r}(r_0,t) = 0, \quad E^0_n(R^0_\ast,t) = \gamma\frac{n^2-1}{(R_\ast^0)^2}a^0_n(t), \end{array}\right. \end{align} | (3.28) |
\begin{align} &\left\{\begin{array}{l} -\frac{\partial^2 F^0_n}{\partial r^2}-\frac{1}{r}\frac{\partial F^0_n}{\partial r}+\frac{n^2}{r^2}F^0_n = \mu B^0_n, \\ \frac{\partial F^0_n}{\partial r}(r_0,t) = 0, \quad F^0_n(R^0_\ast,t) = \gamma\frac{n^2-1}{(R_\ast^0)^2}b^0_n(t), \end{array}\right. \end{align} | (3.29) |
\begin{align} &\frac{\mathrm{d}a^0_n(t)}{\mathrm{d}t} = -\frac{\partial^2 p^0_\ast}{\partial r^2}(R^0_\ast)a^0_n(t)-\frac{\partial E^0_n}{\partial r}(R^0_\ast,t), \end{align} | (3.30) |
\begin{align} &\frac{\mathrm{d}b^0_n(t)}{\mathrm{d}t} = -\frac{\partial^2 p^0_\ast}{\partial r^2}(R^0_\ast)b^0_n(t)-\frac{\partial F^0_n}{\partial r}(R^0_\ast,t). \end{align} | (3.31) |
A direct calculation gives
\begin{align} &\frac{\partial p^0_\ast}{\partial r}(r) = \frac{\mu\bar{\sigma}}{I_0(r_0)K_1(R^0_\ast)+I_1(R^0_\ast)K_0(r_0)}\bigg\{K_1(R^0_\ast)\left(\frac{r_0}{r}I_1(r_0)-I_1(r)\right) \\ &\; \; \; \; \; \; \; \; \; \; \; \; \; \; \; \; \; \; \; \; \; \; \; \; \; \; \; \qquad\qquad+I_1(R^0_\ast)\left(K_1(r)-\frac{r_0}{r}K_1(r_0)\right)\bigg\}+\frac{\mu\tilde{\sigma}r}{2}-\frac{\mu\tilde{\sigma}r^2_0}{2r}, \end{align} | (3.32) |
\begin{align} &\frac{\partial^2 p^0_\ast}{\partial r^2}(R^0_\ast) = \frac{\mu\bar{\sigma}}{R^0_\ast[(R^0_\ast)^2-r^2_0]}\frac{2r_0R^0_\ast[I_1(R^0_\ast)K_1(r_0)-I_1(r_0)K_1(R^0_\ast)]-(R^0_\ast)^2+r^2_0}{I_0(r_0)K_1(R^0_\ast)+I_1(R^0_\ast)K_0(r_0)}, \end{align} | (3.33) |
\begin{align} &A^0_n(r,t) = \frac{\bar{\sigma}a^0_n(t)h_n(r_0,R^0_\ast)}{R^0_\ast }\frac{K_n(r_0)I_n(r)-I_n(r_0)K_n(r)}{I_0(r_0)K_1(R^0_\ast)+I_1(R^0_\ast)K_0(r_0)}, \end{align} | (3.34) |
\begin{align} &\frac{\partial A^0_n}{\partial r}(r,t) = \frac{-\bar{\sigma}a^0_n(t)h_n(r_0,R^0_\ast)}{R^0_\ast [I_0(r_0)K_1(R^0_\ast)+I_1(R^0_\ast)K_0(r_0)]}\frac1{h_n(r_0,r)}, \end{align} | (3.35) |
\begin{align} &\frac{\partial^2 A^0_n}{\partial r^2}(R^0_\ast,t) = \frac{-\bar{\sigma}a^0_n(t)h_n(r_0,R^0_\ast)g_n(r_0,R^0_\ast)}{R^0_\ast [I_0(r_0)K_1(R^0_\ast)+I_1(R^0_\ast)K_0(r_0)]}, \end{align} | (3.36) |
where
\begin{align} &h_n(r_0,x) = \frac1{I_n(r_0)\left(\frac{n}{x}K_n(x)-K_{n+1}(x)\right) -K_n(r_0)\left(\frac{n}{x}I_n(x)+I_{n+1}(x)\right)}, \\ &g_n(r_0,x) = I_n(r_0)\left[\left(1+\frac{n(n-1)}{x^2}\right)K_n(x)+\frac{1}{x}K_{n+1}(x)\right] \\ &\qquad\qquad\qquad\qquad-K_n(r_0)\bigg[\left(1+\frac{n(n-1)}{x^2}\right)I_n(x)-\frac{1}{x}I_{n+1}(x)\bigg]. \end{align} | (3.37) |
Let \eta^0_n = E^0_n+\mu A^0_n , then we find from (3.26) and (3.28) that \eta^0_n satisfies
\begin{align*} -\frac{\partial^2 \eta^0_n}{\partial r^2}-\frac{1}{r}\frac{\partial \eta^0_n}{\partial r}+\frac{n^2}{r^2}\eta^0_n = 0, \end{align*} |
whose solution is
\begin{align*} \eta^0_n(r,t) = C_1(t)r^n+C_2(t)r^{-n} \end{align*} |
and thus,
\begin{equation} E^0_n(r,t) = \eta^0_n(r,t)-\mu A^0_n(r,t) = C_1(t)r^n+C_2(t)r^{-n}-\mu A^0_n(r,t), \end{equation} | (3.38) |
where C_1(t) and C_2(t) are to be determined by the boundary conditions in (3.28). By (2.10), (3.34), (3.35) and (3.37), we get
\begin{align} C_1(t) = &\frac{\mu\bar{\sigma} a^0_n(t)h_n(r_0,R^0_\ast)}{nR^0_\ast[(R^0_\ast)^{2n}+r_0^{2n}]}\frac{n(R^0_\ast)^n[I_n(R^0_\ast)K_n(r_0)-I_n(r_0)K_n(R^0_\ast)]+r^n_0}{I_0(r_0)K_1(R^0_\ast)+I_1(R^0_\ast)K_0(r_0)} \\ &+\frac{(n^2-1)\gamma a^0_n(t)}{(R^0_\ast)^{2n}+r_0^{2n}}(R^0_\ast)^{n-2}, \end{align} | (3.39) |
\begin{align} C_2(t) = &\frac{\mu\bar{\sigma} a^0_n(t)h_n(r_0,R^0_\ast)r_0^n(R^0_\ast)^{n}}{nR^0_\ast[(R^0_\ast)^{2n}+r_0^{2n}]}\frac{nr_0^n[I_n(R^0_\ast)K_n(r_0)-I_n(r_0)K_n(R^0_\ast)]-(R^0_\ast)^n}{I_0(r_0)K_1(R^0_\ast)+I_1(R^0_\ast)K_0(r_0)} \\ &+\frac{(n^2-1)\gamma a^0_n(t)}{(R^0_\ast)^{2n}+r_0^{2n}}r_0^{2n}(R^0_\ast)^{n-2}. \end{align} | (3.40) |
Using (3.35) and (3.38)–(3.40), we further derive
\begin{align} \frac{\partial E^0_n}{\partial r}(r,t) = &\frac{\mu\bar{\sigma} a^0_n(t)h_n(r_0,R^0_\ast)}{R^0_\ast [I_0(r_0)K_1(R^0_\ast)+I_1(R^0_\ast)K_0(r_0)]}\bigg\{r_0^{n}\frac{r^{n-1}+(R^0_\ast)^{2n}r^{-n-1}}{(R^0_\ast)^{2n}+r_0^{2n}} \\ &\; \; \; \; \; \; \; \; \; \; +n(R^0_\ast)^n\frac{r^{n-1}-r_0^{2n}r^{-n-1}}{(R^0_\ast)^{2n}+r_0^{2n}}[I_n(R^0_\ast)K_n(r_0)-I_n(r_0)K_n(R^0_\ast)] \\ &\; \; \; \; \; \; \; \; \; \; +\frac1{h_n(r_0,r)}\bigg\}+n(n^2-1)\gamma a^0_n(t)(R^0_\ast)^{n-2}\frac{r^{n-1}-r_0^{2n}r^{-n-1}}{(R^0_\ast)^{2n}+r_0^{2n}}. \end{align} | (3.41) |
Substituting (3.33) and (3.41) into (3.30) yields
\begin{equation} \frac{\mathrm{d}a^0_n(t)}{\mathrm{d}t} = U_n(r_0,R^0_\ast)a^0_n(t), \end{equation} | (3.42) |
whose solution is explicitly given by
\begin{equation} a^0_n(t) = a^0_n(0)\exp\{U_n(r_0,R^0_\ast)t\}. \end{equation} | (3.43) |
Here,
\begin{align} U_n(r_0,R^0_\ast) = &\frac{\mu\bar{\sigma}h_n(r_0,R^0_\ast)} {I_0(r_0)K_1(R^0_\ast)+I_1(R^0_\ast)K_0(r_0)} \bigg[\frac{2r_0(I_1(r_0)K_1(R^0_\ast)-I_1(R^0_\ast)K_1(r_0))} {h_n(r_0,R^0_\ast)[(R^0_\ast)^2-r_0^2]} \\ &+\frac{n}{(R^0_\ast)^2}\frac{(R^0_\ast)^{2n}-r_0^{2n}}{(R^0_\ast)^{2n}+r_0^{2n}} (I_n(r_0)K_n(R^0_\ast)-I_n(R^0_\ast)K_n(r_0)) -\frac{2r_0^n(R^0_\ast)^{n-2}}{(R^0_\ast)^{2n}+r_0^{2n}}\bigg] \\ &-\frac{\gamma n(n^2-1) }{(R^0_\ast)^3}\frac{(R^0_\ast)^{2n}-r_0^{2n}}{(R^0_\ast)^{2n}+r_0^{2n}}. \end{align} | (3.44) |
It was proven in Lemma 4.4 of [14] that U_n(r_0, R^0_\ast) < 0 for any n\geq 0 . Thus, we have the following:
Lemma 3.1. For any n\geq 0 , there exists \delta > 0 such that |a_0^n(t)|\leq|a_0^n(0)|e^{-\delta t} for all t > 0 .
Lemma 3.1 shows that a_n^0(t) decays to 0 exponentially at +\infty ; hence, when \tau = 0 , the radially symmetric stationary solution is asymptotically stable for all \mu > 0 .
Recalling that R_\ast = R^0_\ast + \tau R^1_\ast + O(\tau^2) , in order to see the effect of the time delay \tau on the size of the stationary tumor, in this subsection we discuss the sign of R^1_\ast by a theoretical analysis combined with numerical simulations.
We obtain from (3.22) that
\begin{equation} \frac{\bar{\sigma}R^1_\ast}{I_0(r_0)K_1(R^0_\ast)+I_1(R^0_\ast)K_0(r_0)}-\tilde{\sigma}R^0_\ast R^1_\ast+\int^{R^0_\ast}_{r_0}\left(\frac{\partial \sigma^0_\ast}{\partial r}(r)\frac{\partial p^0_\ast}{\partial r}(r)+\sigma^1_\ast(r)\right)rdr = 0, \end{equation} | (3.45) |
then by using (2.6)–(2.10), (3.18), (3.19), (3.25) and (3.32), one can solve (3.45) to obtain
\begin{equation} R^1_\ast = -\frac{\mu\bar{\sigma}}{2R^0_\ast}\frac{T(r_0,R^0_\ast)}{S(r_0,R^0_\ast)}, \end{equation} | (3.46) |
where
\begin{align*} T(r_0,R^0_\ast)& = 1-r_0^2[I_0(r_0)K_1(R^0_\ast)+I_1(R^0_\ast)K_0(r_0)]^2 +r_0^2\frac{(R^0_\ast)^2-r_0^2-4}{(R^0_\ast)^2-r_0^2} [I_1(R^0_\ast)K_1(r_0)-I_1(r_0)K_1(R^0_\ast)]^2, \\ S(r_0,R^0_\ast)& = \frac{2r_0}{(R^0_\ast)^2-r_0^2}[I_1(r_0)K_1(R^0_\ast)-I_1(R^0_\ast)K_1(r_0)] [I_0(r_0)K_1(R^0_\ast) +I_1(R^0_\ast)K_0(r_0)]+\frac{1}{(R^0_\ast)^2}. \end{align*} |
Since
U_0(r_0, R^0_\ast) = \frac{\mu\bar{\sigma}}{[I_0(r_0)K_1(R^0_\ast)+I_1(R^0_\ast)K_0(r_0)]^2}S(r_0,R^0_\ast) |
by (3.44), we know S(r_0, R^0_\ast) < 0 . Additionally, we numerically compute the function T(r_0, R^0_\ast) and find it is positive (see Figure 1). Hence, it follows from (3.46) that R^1_\ast > 0 and R^1_\ast is monotone increasing in \mu .
Remark 3.1. The discussion above indicates that the presence of the time delay leads to a larger stationary tumor. Furthermore, the bigger the tumor aggressive parameter \mu is, the greater the effect of time delay on the size of the stationary tumor is.
Now, we tackle the system consisting of all the first-order terms in \tau for r_0 < r < R^0_\ast :
\begin{align} &-\frac{\partial^2 \sigma^1_\ast}{\partial r^2}-\frac{1}{r}\frac{\partial \sigma^1_\ast}{\partial r} = -\sigma^1_\ast, \quad \sigma^1_\ast(r_0) = 0, \quad \frac{\partial \sigma^1_\ast}{\partial r}(R^0_\ast)+\frac{\partial^2 \sigma^0_\ast}{\partial r^2}(R^0_\ast)R^1_\ast = 0, \end{align} | (3.47) |
\begin{align} &\left\{\begin{array}{l} -\frac{\partial^2 p^1_\ast}{\partial r^2}-\frac{1}{r}\frac{\partial p^1_\ast}{\partial r} = \mu\frac{\partial \sigma^0_\ast}{\partial r}\frac{\partial p^0_\ast}{\partial r}+\mu\sigma^1_\ast, \\ \frac{\partial p^1_\ast}{\partial r}(r_0) = 0,\quad p_\ast^1(R_\ast^0) = -\frac{\gamma R_\ast^1}{(R_\ast^0)^2}-\frac{\partial p_\ast^0}{\partial r}(R_\ast^0)R_\ast^1, \end{array}\right. \end{align} | (3.48) |
\begin{align} &\frac{\bar{\sigma}R^1_\ast}{I_0(r_0)K_1(R^0_\ast)+I_1(R^0_\ast)K_0(r_0)}-\tilde{\sigma}R^0_\ast R^1_\ast+\int^{R^0_\ast}_{r_0}\left(\frac{\partial \sigma^0_\ast}{\partial r}(r)\frac{\partial p^0_\ast}{\partial r}(r)+\sigma^1_\ast(r)\right)rdr = 0, \end{align} | (3.49) |
\begin{align} &\left\{\begin{array}{l} -\frac{\partial^2 A^1_n}{\partial r^2}-\frac{1}{r}\frac{\partial A^1_n}{\partial r}+\left(\frac{n^2}{r^2}+1\right)A^1_n = 0, \\ A^1_n(r_0,t) = 0, \quad\frac{\partial^2 A^0_n}{\partial r^2}(R^0_\ast,t)R^1_\ast+\frac{\partial A^1_n}{\partial r}(R^0_\ast,t)+\sigma^0_\ast(R^0_\ast)a^1_n(t)+\sigma^1_\ast(R^0_\ast)a^0_n(t) = 0, \end{array}\right. \end{align} | (3.50) |
\begin{align} &\left\{\begin{array}{l} -\frac{\partial^2 B^1_n}{\partial r^2}-\frac{1}{r}\frac{\partial B^1_n}{\partial r}+\left(\frac{n^2}{r^2}+1\right)B^1_n = 0, \\ B^1_n(r_0,t) = 0, \quad\frac{\partial^2 B^0_n}{\partial r^2}(R^0_\ast,t)R^1_\ast+\frac{\partial B^1_n}{\partial r}(R^0_\ast,t)+\sigma^0_\ast(R^0_\ast)b^1_n(t)+\sigma^1_\ast(R^0_\ast)b^0_n(t) = 0, \end{array}\right. \end{align} | (3.51) |
\begin{align} &\left\{\begin{array}{l} -\frac{\partial^2 E^1_n}{\partial r^2}-\frac{1}{r}\frac{\partial E^1_n}{\partial r}+\frac{n^2}{r^2}E^1_n = \mu\frac{\partial \sigma^0_\ast}{\partial r}\frac{\partial E^0_n}{\partial r}+\mu\frac{\partial A_n^0}{\partial r}\frac{\partial p^0_\ast}{\partial r}-\mu\frac{\partial A_n^0}{\partial t}+\mu A_n^1, \\ \frac{\partial E^1_n}{\partial r}(r_0,t) = 0,\; E^1_n(R_\ast^0,t) = \gamma\frac{n^2-1}{(R_\ast^0)^2}a^1_n(t)-\frac{\partial E^0_n}{\partial r}(R_\ast^0,t)R_\ast^1-2\gamma\frac{n^2-1}{(R_\ast^0)^3}R_\ast^1a^0_n(t), \end{array}\right. \end{align} | (3.52) |
\begin{align} &\left\{\begin{array}{l} -\frac{\partial^2 F^1_n}{\partial r^2}-\frac{1}{r}\frac{\partial F^1_n}{\partial r}+\frac{n^2}{r^2}F^1_n = \mu\frac{\partial \sigma^0_\ast}{\partial r}\frac{\partial F^0_n}{\partial r}+\mu\frac{\partial B_n^0}{\partial r}\frac{\partial p^0_\ast}{\partial r}-\mu\frac{\partial B_n^0}{\partial t}+\mu B_n^1, \\ \frac{\partial F^1_n}{\partial r}(r_0,t) = 0,\; F^1_n(R_\ast^0,t) = \gamma\frac{n^2-1}{(R_\ast^0)^2}b^1_n(t)-\frac{\partial F^0_n}{\partial r}(R_\ast^0,t)R_\ast^1-2\gamma\frac{n^2-1}{(R_\ast^0)^3}R_\ast^1b^0_n(t), \end{array}\right. \end{align} | (3.53) |
\begin{align} &\frac{\mathrm{d}a_n^1(t)}{\mathrm{d}t} = -\frac{\partial^2 p_\ast^0}{\partial r^2}(R_\ast^0) a_n^1(t)-\frac{\partial^3 p_\ast^0}{\partial r^3}(R_\ast^0)R_\ast^1a_n^0(t) \\ &\qquad\qquad-\frac{\partial^2 p_\ast^1}{\partial r^2}(R_\ast^0) a_n^0(t)-\frac{\partial^2 E_n^0}{\partial r^2}(R_\ast^0,t)R_\ast^1-\frac{\partial E_n^1}{\partial r}(R_\ast^0,t), \end{align} | (3.54) |
\begin{align} &\frac{\mathrm{d}b_n^1(t)}{\mathrm{d}t} = -\frac{\partial^2 p_\ast^0}{\partial r^2}(R_\ast^0) b_n^1(t)-\frac{\partial^3 p_\ast^0}{\partial r^3}(R_\ast^0)R_\ast^1b_n^0(t) \\ &\qquad\qquad-\frac{\partial^2 p_\ast^1}{\partial r^2}(R_\ast^0) b_n^0(t)-\frac{\partial^2 F_n^0}{\partial r^2}(R_\ast^0,t)R_\ast^1-\frac{\partial F_n^1}{\partial r}(R_\ast^0,t). \end{align} | (3.55) |
To obtain the asymptotic behavior of a_n^1(t) as \infty , by (3.54) and the boundedness of the modified Bessel functions I_n(r) and K_n(r) on [r_0, R^0_\ast] , it suffices to analyze \frac{\partial E^1_n}{\partial r}(R^0_\ast, t) . For this purpose, in view of (3.52), we first compute A^1_n(r, t) . Solving (3.50) yields
\begin{align} A_n^1(r,t) = \frac{\bar{\sigma}h_n(r_0,R^0_\ast)}{R^0_\ast} \frac{I_n(r_0)K_n(r)-K_n(r_0)I_n(r)}{I_0(r_0)K_1(R^0_\ast)+I_1(R^0_\ast)K_0(r_0)} V_n(r_0,R^0_\ast,R^1_\ast,a^0_n(t),a^1_n(t)) \end{align} | (3.56) |
with
\begin{align*} V_n(r_0,R^0_\ast,R^1_\ast,a^0_n(t),a^1_n(t)) = &a_n^0(t)R^1_\ast h_n(r_0,R^0_\ast)g_n(r_0,R^0_\ast)-a_n^1(t) \\ &+a_n^0(t)R^1_\ast\frac{I_0(R^0_\ast)K_0(r_0)-I_0(r_0)K_0(R^0_\ast)} {I_0(r_0)K_1(R^0_\ast)+I_1(R^0_\ast)K_0(r_0)}, \end{align*} |
where we have employed (2.8)–(2.10), (3.19), (3.36) and (3.37). Furthermore,
\begin{align} \frac{\partial A_n^1}{\partial r}(r,t) = \frac{\bar{\sigma}h_n(r_0,R^0_\ast)V_n(r_0,R^0_\ast,R^1_\ast,a^0_n(t),a^1_n(t))} {R^0_\ast[I_0(r_0)K_1(R^0_\ast)+I_1(R^0_\ast)K_0(r_0)]} \frac1{h_n(r_0,r)}. \end{align} | (3.57) |
Next, being similar to the computation of E^0_n , we set \eta^1_n = E^1_n+\mu A^1_n , then we derive from (3.50), (3.52) and (3.56) that
\begin{align} &\left\{\begin{array}{l} -\frac{\partial^2 \eta^1_n}{\partial r^2}-\frac{1}{r}\frac{\partial \eta^1_n}{\partial r}+\frac{n^2}{r^2}\eta^1_n = \mu\frac{\partial \sigma^0_\ast}{\partial r}\frac{\partial E^0_n}{\partial r}+\mu\frac{\partial A^0_n}{\partial r}\frac{\partial p^0_\ast}{\partial r}-\mu\frac{\partial A^0_n}{\partial t}, \\ \frac{\partial \eta^1_n}{\partial r}(r_0,t) = \mu\frac{\partial A^1_n}{\partial r}(r_0,t),\quad \eta^1_n(R^0_\ast,t) = E^1_n(R^0_\ast,t)+\mu A^1_n(R^0_\ast,t). \end{array}\right. \end{align} | (3.58) |
For brevity, we introduce the differential operator L_n = -\partial_{rr}-\frac1r\partial_r+\frac{n^2}{r^2} and write \eta^1_n = u^{(1)}_n+u^{(2)}_n+u^{(3)}_n+u^{(4)}_n , where u^{(1)}_n , u^{(2)}_n , u^{(3)}_n and u^{(4)}_n solve the following problems, respectively:
\begin{align} &\left\{\begin{array}{l} L_nu^{(1)}_n = \mu\frac{\partial\sigma^0_\ast}{\partial r}\frac{\partial E^0_n}{\partial r}, \\ \frac{\partial u^{(1)}_n}{\partial r}(r_0,t) = 0, \quad u^{(1)}_n(R^0_\ast,t) = 0; \end{array}\right. \end{align} | (3.59) |
\begin{align} &\left\{\begin{array}{l} L_nu^{(2)}_n = \mu\frac{\partial A^0_n}{\partial r}\frac{\partial p^0_\ast}{\partial r}, \\ \frac{\partial u^{(2)}_n}{\partial r}(r_0,t) = 0, \quad u^{(2)}_n(R^0_\ast,t) = 0; \end{array}\right. \end{align} | (3.60) |
\begin{align} &\left\{\begin{array}{l} L_nu^{(3)}_n = -\mu\frac{\partial A^0_n}{\partial t}, \\ \frac{\partial u^{(3)}_n}{\partial r}(r_0,t) = 0, \quad u^{(3)}_n(R^0_\ast,t) = 0; \end{array}\right. \end{align} | (3.61) |
\begin{align} &\left\{\begin{array}{l} L_nu^{(4)}_n = 0, \\ \frac{\partial u^{(4)}_n}{\partial r}(r_0,t) = \mu\frac{\partial A_n^1}{\partial r}(r_0,t), \quad u^{(4)}_n(R^0_\ast,t) = E^1_n(R^0_\ast,t)+\mu A^1_n(R^0_\ast,t). \end{array}\right. \end{align} | (3.62) |
Let us first estimate u^{(1)}_n . By (3.18), (3.41) and (3.59), we have
\begin{align} L_nu^{(1)}_n = &\mu\bar{\sigma}a_n^0(t)\frac{K_1(R^0_\ast)I_1(r)-I_1(R^0_\ast)K_1(r)}{I_0(r_0)K_1(R^0_\ast)+I_1(R^0_\ast)K_0(r_0)}\bigg\{n(n^2-1)\gamma(R^0_\ast)^{n-2}\frac{r^{n-1}-r_0^{2n}r^{-n-1}}{(R^0_\ast)^{2n}+r_0^{2n}} \\ &\; \; \; \; \; \; \; \; \; \; \; +\frac{\mu\bar{\sigma}h_n(r_0,R^0_\ast)}{R^0_\ast[I_0(r_0)K_1(R^0_\ast)+I_1(R^0_\ast)K_0(r_0)]} \bigg[\frac1{h_n(r_0,r)}+r_0^n\frac{r^{n-1}+(R^0_\ast)^{2n}r^{-n-1}}{(R^0_\ast)^{2n}+r_0^{2n}} \\ &\; \; \; \; \; \; \; \; \; \; \; +n(R^0_\ast)^n\frac{r^{n-1}-r_0^{2n}r^{-n-1}}{(R^0_\ast)^{2n}+r_0^{2n}}(I_n(R^0_\ast)K_n(r_0)-I_n(r_0)K_n(R^0_\ast))\bigg]\bigg\}. \end{align} | (3.63) |
Based on the properties of the modified Bessel functions I_n(r) and K_n(r) , the righthand side of (3.63) is less than Q(n)a^0_n(t) when r_0 \leq r < R^0_\ast . Here, Q(n) denotes a polynomial function of n . Similar estimates can be established for u^{(2)}_n and u^{(3)}_n by (3.60) and (3.61).
Lemma 3.2. Consider the elliptic problem
\begin{align} &-\Delta\omega(x,t)+\frac{n^2}{|x|^2}\omega(x,t) = b(x,t), \qquad x\in\Omega_R, \end{align} | (3.64) |
\begin{align} &\partial_{\vec n}\omega\big|_{|x| = r_0} = 0, \quad \omega\big|_{|x| = R} = 0, \end{align} | (3.65) |
where \Omega_R = \big\{x\in \mathbb{R}^2: r_0 < |x| < R\big\} . If b(x, t) = b(|x|, t) and b(\cdot, t)\in L^2(\Omega_R) , then the problem (3.64) and (3.65) admits a unique solution \omega in H^2(\Omega_R) with estimates
\begin{align} \|\omega(\cdot,t)\|_{H^2(\Omega_R)}\leq C\bigg(\int^R_{r_0}|b(r,t)|^2rdr\bigg)^{1/2}; \end{align} | (3.66) |
\begin{align} \big\|\partial_{\vec\nu}\omega(\cdot,t)\big\|_{L^\infty(\partial B_R)}\leq C\bigg(\int^R_{r_0}|b(r,t)|^2rdr\bigg)^{1/2}, \end{align} | (3.67) |
where the constant C in (3.66) and (3.67) is independent of n .
The lemma can be proven by combining the proofs of [16, Lemma 4.6] and [17, Lemma 3.2]. The details are omitted here.
Lemma 3.2 ensures the existence and uniqueness of u^{(k)}_n in H^2(\Omega{_\ast}) for k = 1, 2, 3 . Furthermore, there holds
\begin{equation} \bigg|\frac{\partial u^{(1)}_n}{\partial r}(R^0_\ast,t)\bigg|+\bigg|\frac{\partial u^{(2)}_n}{\partial r}(R^0_\ast,t)\bigg|+\bigg|\frac{\partial u^{(3)}_n}{\partial r}(R^0_\ast,t)\bigg|\leq Ce^{-\delta t}. \end{equation} | (3.68) |
Obviously, the solution u^{(4)}_n to the problem (3.62) has the form:
\begin{equation} u^{(4)}_n(r,t) = C_5(t)r^n+C_6(t)r^{-n}, \end{equation} | (3.69) |
where C_5(t) and C_6(t) are determined by the boundary conditions in (3.62). Using (2.10), (3.41), (3.56), (3.57) and the boundary conditions in (3.52), we get
\begin{align} C_5(t) = &\frac{a_n^1(t)}{(R^0_\ast)^{2n}+r_0^{2n}}\bigg\{\frac{\mu\bar{\sigma}h_n(r_0,R^0_\ast)}{R^0_\ast[I_0(r_0)K_1(R^0_\ast)+I_1(R^0_\ast)K_0(r_0)]}\bigg[\frac{r_0^n}{n}+(R^0_\ast)^n(K_n(r_0)I_n(R^0_\ast) \\ &\; \; \; \; \; \; \; \; \; \; \; \; \; \; \; \; \; \; -I_n(r_0)K_n(R^0_\ast))\bigg]+\gamma (n^2-1)(R^0_\ast)^{n-2}\bigg\}+H_1(r_0,R^0_\ast,R^1_\ast)a_n^0(t), \end{align} | (3.70) |
\begin{align} C_6(t) = &\frac{a_n^1(t)r_0^n(R^0_\ast)^n}{(R^0_\ast)^{2n}+r_0^{2n}}\bigg\{\frac{\mu\bar{\sigma}h_n(r_0,R^0_\ast)}{R^0_\ast[I_0(r_0)K_1(R^0_\ast)+I_1(R^0_\ast)K_0(r_0)]}\bigg[-\frac{(R^0_\ast)^n}{n}+r_0^n(K_n(r_0)I_n(R^0_\ast) \\ &\; \; \; \; \; \; \; \; \; \; \; \; \; \; \; \; \; \; -I_n(r_0)K_n(R^0_\ast))\bigg]+\frac{\gamma (n^2-1)r_0^n}{(R^0_\ast)^2}\bigg\}+H_2(r_0,R^0_\ast,R^1_\ast)a_n^0(t), \end{align} | (3.71) |
where H_1 , H_2 are functions of r_0 , R^0_\ast and R^1_\ast .
Now, since
\begin{align*} E^1_n(r,t) = \eta^1_n-\mu A^1_n = u^{(1)}_n+u^{(2)}_n+u^{(3)}_n+u^{(4)}_n-\mu A^1_n, \end{align*} |
we derive
\begin{align} &\frac{\partial E^1_n}{\partial r}(R^0_\ast,t) = \frac{\partial u^{(1)}_n}{\partial r}(R^0_\ast,t)+\frac{\partial u^{(2)}_n}{\partial r}(R^0_\ast,t)+\frac{\partial u^{(3)}_n}{\partial r}(R^0_\ast,t)+\frac{\partial u^{(4)}_n}{\partial r}(R^0_\ast,t)-\mu\frac{\partial A^1_n}{\partial r}(R^0_\ast,t). \end{align} | (3.72) |
By (3.33), (3.57) and (3.69)–(3.72), we obtain from (3.54) that
\begin{align*} \frac{\mathrm{d}a^1_n(t)}{\mathrm{d}t} = &-\frac{\partial^2 p^0_\ast}{\partial r^2}(R^0_\ast)a^1_n(t)-\frac{\partial^3 p^0_\ast}{\partial r^3}(R^0_\ast)R^1_\ast a^0_n(t)-\frac{\partial^2 p^1_\ast}{\partial r^2}(R^0_\ast)a^0_n(t)-\frac{\partial^2 E^0_n}{\partial r^2}(R^0_\ast,t)R^1_\ast-\frac{\partial E^1_n}{\partial r}(R^0_\ast,t) \\ = &a_n^1(t)\bigg\{\frac{\mu\bar{\sigma}h_n(r_0,R^0_\ast)} {I_0(r_0)K_1(R^0_\ast)+I_1(R^0_\ast)K_0(r_0)} \bigg[\frac{n}{(R^0_\ast)^2}\frac{(R^0_\ast)^{2n}-r_0^{2n}}{(R^0_\ast)^{2n} +r_0^{2n}}(I_n(r_0)K_n(R^0_\ast)-I_n(R^0_\ast)K_n(r_0)) \\ &\; \; \; \; \; \; \; \; \; \; \; +\frac{2 r_0(I_1(r_0)K_1(R^0_\ast)-I_1(R^0_\ast)K_1(r_0))} {(R^0_\ast)^2-r_0^2}\frac1{h_n(r_0,R^0_\ast)}-\frac{2r_0^n(R^0_\ast)^{n-2}}{(R^0_\ast)^{2n}+r_0^{2n}}\bigg] \\ &\; \; \; \; \; \; \; \; \; \; \; -\frac{\gamma n(n^2-1)}{(R^0_\ast)^3}\frac{(R^0_\ast)^{2n}-r_0^{2n}}{(R^0_\ast)^{2n}+r_0^{2n}}\bigg\}+\tilde{H}(n,r_0,R^0_\ast,R^1_\ast)a_n^0(t) \\ &-\frac{\partial u^{(1)}_n}{\partial r}(R^0_\ast,t)-\frac{\partial u^{(2)}_n}{\partial r}(R^0_\ast,t)-\frac{\partial u^{(3)}_n}{\partial r}(R^0_\ast,t) \\ = &a_n^1(t)U_n(r_0,R^0_\ast)-\frac{\partial u^{(1)}_n}{\partial r}(R^0_\ast,t)-\frac{\partial u^{(2)}_n}{\partial r}(R^0_\ast,t)-\frac{\partial u^{(3)}_n}{\partial r}(R^0_\ast,t) +\tilde{H}(n,r_0,R^0_\ast,R^1_\ast)a_n^0(t), \end{align*} |
where \tilde{H} is a known function of n , r_0 , R^0_\ast , R^1_\ast and satisfies
\begin{equation} |\tilde{H}(n,r_0,R^0_\ast,R^1_\ast)|\leq C. \end{equation} | (3.73) |
Thus, using Lemma 3.1, (3.68) and (3.73) gives
\begin{align} \bigg|&\frac{\mathrm{d}a_n^1(t)}{\mathrm{d}t}-a_n^1(t)U_n(r_0,R^0_\ast)\bigg| \\ \leq& |\tilde{H}(n,r_0,R^0_\ast,R^1_\ast)a_n^0(t)|+\bigg|\frac{\partial u^{(1)}_n}{\partial r}(R^0_\ast,t)\bigg|+\bigg|\frac{\partial u^{(2)}_n}{\partial r}(R^0_\ast,t)\bigg|+\bigg|\frac{\partial u^{(3)}_n}{\partial r}(R^0_\ast,t)\bigg| \\ \leq& Ce^{-\delta t}. \end{align} | (3.74) |
In addition, for n\geq0 , Lemma 3.1 implies
\begin{equation*} -U_n(r_0,R^0_\ast) > \delta > 0. \end{equation*} |
Therefore, applying [16, Lemma 4.7] to (3.74) yields
\begin{align} |a^1_n(t)|\leq Ce^{-\delta t}, \qquad \quad t > 0, \end{align} | (3.75) |
i.e., a^1_n(t) decays exponentially as t\to\infty . Noticing that b_n(t) and a_n(t) have the same asymptotic behavior, we also have
\begin{align} |b^0_n(t)|+|b^1_n(t)|\leq Ce^{-\delta t},\qquad t > 0. \end{align} | (3.76) |
Proof of Theorem 1.2 The desired result (1.14) follows from Lemma 3.1, (3.75) and (3.76). The proof is complete.
Remark 3.2. The results on tumor cord without time delays in [14] show that the radially symmetric stationary solution is asymptotically stable under nonradially symmetric perturbations. Here, our Theorem 1.2 says that such asymptotic stability does not be affected by small time delay.
In this paper, we have investigated the effects of a time delay in cell proliferation on the growth of tumor cords, where the domain is a bounded subset in \mathbb{R}^2 and its boundary consists of two disjoint closed curves, one fixed and the other moving and a priori unknown. The existence, uniqueness and linear stability of the radially symmetric stationary solution were studied.
Here are some interesting findings. 1) Adding the time delay would not change the stability of the radially symmetric stationary solution when compared with the same system without delay [14], but adding the time delay would result in a larger stationary tumor. The bigger the tumor growth intensity \mu is, the greater impact that time delay has on the size of the stationary tumor. 2) By the result of [16], we know that for tumor spheroids with the same time delay, there exists a threshold \mu_\ast > 0 for the tumor aggressiveness constant \mu such that only for \mu < \mu_\ast , the radially symmetric stationary solution is linearly stable under non-radial perturbations. For tumor cords, however, from Theorem 1.2 we saw that the radially symmetric stationary solution is always linearly stable, regardless of the value of \mu . It showed that there is an essential difference between tumor cords and tumor spheroids with the same time delay.
We think that the linear stability analysis for the full system without quasi-steady state simplification, i.e., c > 0 , may be very challenging, which we expect to solve in future work.
The authors declare they have not used Artificial Intelligence (AI) tools in the creation of this article.
The authors would like to thank the reviewers for their valuable suggestions. This work was partly supported by the National Natural Science Foundation of China (No. 12161045 and No. 12261047), Jiangxi Provincial Natural Science Foundation (No. 20224BCD41001 and No. 20232BAB201010), the Science and Technology Planning Project from Educational Commission of Jiangxi Province, China (No. GJJ2200319), the Scientific Research Project of Hunan Provincial Education Department, China (No. 22B0725, No. 23B0670 and No. 23C0234), and the Research Initiation Project of Hengyang Normal University, China (No. 2022QD01).
The authors declare there is no conflict of interest.
[1] | Y. Hao, Z. Gai, G. Yan, H. Wu, M. Irfan, The spatial spillover effect and nonlinear relationship analysis between environmental decentralization, government corruption and air pollution: Evidence from China, Sci. Total Environ., 763 (2021), 144183. https://doi.org/10.1016/j.scitotenv.2020.144183 |
[2] |
F. Allen, J. Qian, M. Qian, Law, finance, and economic growth in China, J. Financ. Econ., 77 (2004), 57–116. https://doi.org/10.1016/j.jfineco.2004.06.010 doi: 10.1016/j.jfineco.2004.06.010
![]() |
[3] |
X. B. Li, Behind the recent surge of Chinese patenting: An institutional view, Res. Policy, 41 (2011), 236–249. https://doi.org/10.1016/J.RESPOL.2011.07.003 doi: 10.1016/J.RESPOL.2011.07.003
![]() |
[4] |
L. Liu, M. Liu, How does the digital economy affect industrial eco-efficiency? Empirical evidence from China, Data Sci. Finance Econ., 2 (2022), 371–390. https://doi.org/10.3934/DSFE.2022019 doi: 10.3934/DSFE.2022019
![]() |
[5] |
Z. H. Li, J. H. Zhu, J. J. He, The effects of digital financial inclusion on innovation and entrepreneurship: A network perspective, Electron. Res. Arch., 30 (2022), 4697–4715. https://doi.org/10.3934/era.2022238 doi: 10.3934/era.2022238
![]() |
[6] |
A. Blackman, Z. Y. Li, A. A. Liu, Efficacy of command-and-control and market-based environmental regulation in developing countries, Annu. Rev. Resour. Econ., 10 (2018), 381–404. https://doi.org/10.1146/annurev-resource-100517-023144 doi: 10.1146/annurev-resource-100517-023144
![]() |
[7] |
M. Shugurov, International cooperation on climate research and green technologies in the face of sanctions: The case of Russia, Green Finance, 5 (2023), 102–153. https://doi.org/10.3934/GF.2023006 doi: 10.3934/GF.2023006
![]() |
[8] |
T. Lin, M. Y. Du, S. Y. Ren, How do green bonds affect green technology innovation? Firm evidence from China, Green Finance, 4 (2022), 492–511. https://doi.org/10.3934/GF.2022024 doi: 10.3934/GF.2022024
![]() |
[9] |
Y. Liu, P. Failler, Y. Ding, Enterprise financialization and technological innovation: Mechanism and heterogeneity, PLoS One, 17 (2022), e0275461. https://doi.org/10.1371/JOURNAL.PONE.0275461 doi: 10.1371/JOURNAL.PONE.0275461
![]() |
[10] |
X. Yang, J. Cao, Z. Liu, Y. Lai, Environmental policy uncertainty and green innovation: A TVP-VAR-SV model approach, Quant. Finance Econ., 6 (2022), 604–621. https://doi.org/10.3934/QFE.2022026 doi: 10.3934/QFE.2022026
![]() |
[11] |
Y. Liu, C. Ma, Z. H. Huang, Can the digital economy improve green total factor productivity? An empirical study based on Chinese urban data, Math. Biosci. Eng., 20 (2023), 6866–6893. https://doi.org/10.3934/mbe.2023296 doi: 10.3934/mbe.2023296
![]() |
[12] |
Z. Li, B. Mo, H. Nie, Time and frequency dynamic connectedness between cryptocurrencies and financial assets in China, Int. Rev. Econ. Finance, 86 (2023), 46–57. https://doi.org/10.1016/j.iref.2023.01.015 doi: 10.1016/j.iref.2023.01.015
![]() |
[13] |
P. Hsu, X. Tian, Y. Xu, Financial development and innovation: Cross-country evidence, J. Financ. Econ., 112 (2013), 116–135. https://doi.org/10.1016/j.jfineco.2013.12.002 doi: 10.1016/j.jfineco.2013.12.002
![]() |
[14] |
Y. M. Zhang, J. J. Zhang, Z. Cheng, Stock market liberalization and corporate green innovation: Evidence from China, Int. J. Environ. Res. Public Health, 18 (2021), 3412. https://doi.org/10.3390/ijerph18073412 doi: 10.3390/ijerph18073412
![]() |
[15] | Z. Li, C. Yang, Z. Huang, How does the fintech sector react to signals from central bank digital currencies? Finance Res. Lett., 50 (2022), 103308.https://doi.org/10.1016/j.frl.2022.103308 |
[16] |
Z. H. Li, G. K. Liao, Z. Z. Wang, Z. Huang, Green loan and subsidy for promoting clean production innovation, J Clean Prod., 187 (2018), 421–431. https://doi.org/10.1016/j.jclepro.2018.03.066 doi: 10.1016/j.jclepro.2018.03.066
![]() |
[17] |
Z. Li, Z. Huang, H. Dong, The influential factors on outward foreign direct investment: evidence from the "The Belt and Road", Emerging Mark. Finance Trade, 55 (2019), 3211–3226. https://doi.org/10.1080/1540496x.2019.1569512 doi: 10.1080/1540496x.2019.1569512
![]() |
[18] |
Y. Liu, Z. H. Li, M. R. Xu, The influential factors of financial cycle spillover: evidence from China, Emerging Mark. Finance Trade, 56 (2020), 1336–1350. https://doi.org/10.1080/1540496x.2019.1658076 doi: 10.1080/1540496x.2019.1658076
![]() |
[19] |
E. Dinopoulos, P. S. Segerstrom, Intellectual property rights, multinational firms and economic growth, J. Dev. Econ., 92 (2010), 13–27. https://doi.org/10.1016/j.jdeveco.2009.01.007 doi: 10.1016/j.jdeveco.2009.01.007
![]() |
[20] |
J. Hudson, A. Minea, Innovation, intellectual property rights, and economic development: A unified empirical investigation, World Dev., 46 (2013), 66–78, https://doi.org/10.1016/j.worlddev.2013.01.023 doi: 10.1016/j.worlddev.2013.01.023
![]() |
[21] |
C. M. Sweet, D. S. Maggio, Do stronger intellectual property rights increase innovation, World Dev., 66 (2015), 665–677. https://doi.org/10.1016/j.worlddev.2014.08.025 doi: 10.1016/j.worlddev.2014.08.025
![]() |
[22] |
Z. Li, J. Zhong, Impact of economic policy uncertainty shocks on China's financial conditions, Finance Res. Lett., 35 (2022), 101303. https://doi.org/10.1016/j.frl.2019.101303 doi: 10.1016/j.frl.2019.101303
![]() |
[23] |
Y. Liu, P. Failler, Z. Y. Liu, Impact of environmental regulations on energy efficiency: A case study of China's air pollution prevention and control action plan, Sustainability-Basel, 14 (2022), 3168. https://doi.org/10.3390/su14063168 doi: 10.3390/su14063168
![]() |
[24] |
N. Papageorgiadis, A. Sharma, Intellectual property rights and innovation: A panel analysis, Econ. Lett., 141 (2016), 70–72. https://doi.org/10.1016/J.ECONLET.2016.01.003 doi: 10.1016/J.ECONLET.2016.01.003
![]() |
[25] |
M. Grimaldi, M. Greco, L. Cricelli, A framework of intellectual property protection strategies and open innovation, J. Bus. Res., 123 (2021), 156–164. https://doi.org/10.1016/j.jbusres.2020.09.043 doi: 10.1016/j.jbusres.2020.09.043
![]() |
[26] | K. J. Arrow, Economic welfare and the allocation of resources for invention, in The Rate and Direction of Inventive Activity: Economic and Social Factors, Princeton University Press, (1962), 609–626. |
[27] |
M. Awada, R. Mestre, Revisiting the energy-growth nexus with debt channel. A wavelet time-frequency analysis for a panel of Eurozone-OECD countries, Data Sci. Finance Econ., 3 (2023), 133–151. https://doi.org/10.3934/DSFE.2023008 doi: 10.3934/DSFE.2023008
![]() |
[28] |
G. Garau, Total factor productivity and relative prices: the case of Italy, Natl. Account. Rev., 4 (2022), 16–37. https://doi.org/10.3934/NAR.2022002 doi: 10.3934/NAR.2022002
![]() |
[29] |
P. Klemperer, How broad should the scope of patent protection be, RAND J. Econ., 21 (1990), 113–130. https://doi.org/10.2307/2555498 doi: 10.2307/2555498
![]() |
[30] |
S. Scotchmer, Standing on the shoulders of giants: Cumulative research and the patent law, J. Econ. Perspect., 5 (1991), 29–41. https://doi.org/10.1257/JEP.5.1.29 doi: 10.1257/JEP.5.1.29
![]() |
[31] |
K. Rennings, Redefining innovation—eco-innovation research and the contribution from ecological economics, Ecol. Econ., 32 (2000), 319–332. https://doi.org/10.1016/S0921-8009(99)00112-3 doi: 10.1016/S0921-8009(99)00112-3
![]() |
[32] |
Y. X. Wen, Y. T. Xu, Statistical monitoring of economic growth momentum transformation: Empirical study of Chinese provinces, AIMS Math., 8 (2023), 24825–24847. https://doi.org/10.3934/math.20231266 doi: 10.3934/math.20231266
![]() |
[33] | J. Horbach, C. Rammer, K. Rennings, Determinants of eco-innovations by type of environmental impact: The role of regulatory push/pull, technology push and market pull, Ecol. Econ., 78 (2012), 112–122. https://doi.org/10.1016/j.ecolecon.2012.04.005 |
[34] |
M. C. Cuerva, A. Triguero-Cano, D. Córcoles, Drivers of green and non-green innovation: empirical evidence in Low-Tech SMEs, J. Clean Prod., 68 (2014), 104–113. https://doi.org/10.1016/J.JCLEPRO.2013.10.049 doi: 10.1016/J.JCLEPRO.2013.10.049
![]() |
[35] |
D. Krasnoselskaya, V. Timiryanova, Exploring the impact of ecological dimension on municipal investment: Empirical evidence from Russia, Natl. Account. Rev., 5 (2023), 227–244. https://doi.org/10.3934/NAR.2023014 doi: 10.3934/NAR.2023014
![]() |
[36] |
T. Roh, K. M. Lee, J. Y. Yang, How do intellectual property rights and government support drive a firm's green innovation? The mediating role of open innovation, J. Clean Prod., 317 (2021), 128422. https://doi.org/10.1016/J.JCLEPRO.2021.128422 doi: 10.1016/J.JCLEPRO.2021.128422
![]() |
[37] |
F. F. Adedoyin, A. A. Alola, F. V. Bekun, An assessment of environmental sustainability corridor: The role of economic expansion and research and development in EU countries, Sci. Total Environ., 713 (2020), 136726. https://doi.org/10.1016/j.scitotenv.2020.136726 doi: 10.1016/j.scitotenv.2020.136726
![]() |
[38] |
G. A. Crespi, P. Zúñiga, Innovation and productivity: Evidence from six Latin American countries, World Dev., 40 (2012), 273–290. https://doi.org/10.1016/j.worlddev.2011.07.010 doi: 10.1016/j.worlddev.2011.07.010
![]() |
[39] | M. Gürler, The effect of the researchers, research and development expenditure as innovation inputs on patent grants and high-tech exports as innovation outputs in OECD and emerging countries especially in BRIICS, Eur. J. Sci. Technol., 32 (2022). https://doi.org/10.31590/ejosat.1051899 |
[40] |
A. Idowu, O. M. Ohikhuare, M. A. Chowdhury, Does industrialization trigger carbon emissions through energy consumption? Evidence from OPEC countries and high industrialised countries, Quant. Finance Econ., 7 (2023), 165–186. https://doi.org/10.3934/QFE.2023009 doi: 10.3934/QFE.2023009
![]() |
[41] | H. C. Wang, T. Lv, Judicial protection of intellectual property and enterprise innovation—Based on the quasi-natural experiment of "three trials in one" of intellectual property cases in Guangdong Province, J. Manag. World, 10 (2016), 118–133. |
[42] | A. Guariglia, P. Liu, To what extent do financing constraints affect Chinese firms' innovation activities? Int. Rev. Financ. Anal., 36 (2014), 223–240. https://doi.org/10.1016/J.IRFA.2014.01.005 |
[43] | C. Lee, C. Lee, How does green finance affect green total factor productivity? Evidence from China, Energy Econ., 107 (2022). https://doi.org/10.1016/j.eneco.2022.105863 |
[44] |
C. H. Yu, X. Q. Wu, D. Y. Zhang, Demand for green finance: Resolving financing constraints on green innovation in China, Energy Policy, 153 (2021), 112255. https://doi.org/10.1016/J.ENPOL.2021.112255 doi: 10.1016/J.ENPOL.2021.112255
![]() |
[45] |
R. U. Khan, H. Arif, N. E. Sahar, A. Ali, M. A. Abbasi, The role of financial resources in SMEs' financial and environmental performance; the mediating role of green innovation, Green Finance, 4 (2022), 36–53. https://doi.org/10.3934/GF.2022002 doi: 10.3934/GF.2022002
![]() |
[46] | Z. Li, L. Chen, H. Dong, What are bitcoin market reactions to its-related events? Int. Rev. Econ. Finance, 73 (2021), 1–10. https://doi.org/10.1016/j.iref.2020.12.020 |
[47] | C. P. Wu, D. Tang, Intellectual property rights enforcement, corporate innovation and operating performance: Evidence from China's listed companies, Econ. Res. J., 51 (2016), 125–139. |
[48] |
P. Z. Liu, Y. M. Zhao, J. N. Zhu, C. Yang, Technological industry agglomeration, green innovation efficiency, and development quality of city cluster, Green Finance, 4 (2022), 411–435. https://doi.org/10.3934/GF.2022020 doi: 10.3934/GF.2022020
![]() |
[49] |
Y. X. Liu, Y. Liu, Z. B. Wei, Property rights protection, financial constraint, and capital structure choices: Evidence from a Chinese natural experiment, J. Corporate Finance, 73 (2022), 102167. https://doi.org/10.1016/j.jcorpfin.2022.102167 doi: 10.1016/j.jcorpfin.2022.102167
![]() |
[50] |
Z. Sun, W. Song, Investment's effect on innovation performance, J. Quant. Technol. Econ., 29 (2012), 49–63+122. https://doi.org/10.13653/j.cnki.jqte.2012.04.007 doi: 10.13653/j.cnki.jqte.2012.04.007
![]() |
[51] |
Q. H. Huang, Y. Z. Yu, S. L. Zhang, Internet development and productivity growth in manufacturing industry: Internal mechanism and China experiences, China Ind. Econ., 8 (2019), 5–23. https://doi.org/10.19581/j.cnki.ciejournal.2019.08.001 doi: 10.19581/j.cnki.ciejournal.2019.08.001
![]() |
[52] |
C. J. Hadlock, J. R. Pierce, New evidence on measuring financial constraints: Moving beyond the KZ index, Rev. Financ. Stud., 23 (2010), 1909–1940. https://doi.org/10.1093/RFS/HHQ009 doi: 10.1093/RFS/HHQ009
![]() |
[53] |
M. Song, P. Zhou, H. T. Si, Financial technology and enterprise total factor productivity—perspective of "enabling" and credit rationing, China Ind. Econ., 4 (2021), 138–155. https://doi.org/10.19581/j.cnki.ciejournal.2021.04.006 doi: 10.19581/j.cnki.ciejournal.2021.04.006
![]() |
[54] |
Z. Li, G. Liao, K. Albitar, Does corporate environmental responsibility engagement affect firm value? The mediating role of corporate innovation, Bus. Strategy Environ., 29 (2020), 1045–1055. https://doi.org/10.1002/bse.2416 doi: 10.1002/bse.2416
![]() |
[55] |
Z. Li, H. Chen, B. Mo, Can digital finance promote urban innovation? Evidence from China, Borsa Istanbul Rev., 23 (2023), 285–296. https://doi.org/10.1016/j.bir.2022.10.006 doi: 10.1016/j.bir.2022.10.006
![]() |
[56] |
Y. P. Deng, L. Wang, W. J. Zhou, Does environmental regulation promote green innovation capability?—Evidence from China, Stat. Res., 38 (2021), 76–86. https://doi.org/10.19343/j.cnki.11-1302/c.2021.07.006 doi: 10.19343/j.cnki.11-1302/c.2021.07.006
![]() |
[57] |
G. J. Prah, Innovation and economic performance: The role of financial development, Quant. Finance Econ., 6 (2022), 696–721. https://doi.org/10.3934/QFE.2022031 doi: 10.3934/QFE.2022031
![]() |
[58] | S. Z. Qi, S. Lin, J. B. Cui, Do environmental rights trading schemes induce green innovation? evidence from listed firms in China, Econ. Res. J., 53 (2018), 129–143. |
[59] |
M. Wang, L. Li, H. Lan, The measurement and analysis of technological innovation diffusion in China's manufacturing industry, Natl. Account. Rev., 3 (2021), 452–471. https://doi.org/10.3934/NAR.2021024 doi: 10.3934/NAR.2021024
![]() |
[60] |
Z. H. Li, Z. M. Huang, Y. Y. Su, New media environment, environmental regulation and corporate green technology innovation: Evidence from China, Energy Econ., 119 (2023), 106545. https://doi.org/10.1016/j.eneco.2023.106545 doi: 10.1016/j.eneco.2023.106545
![]() |