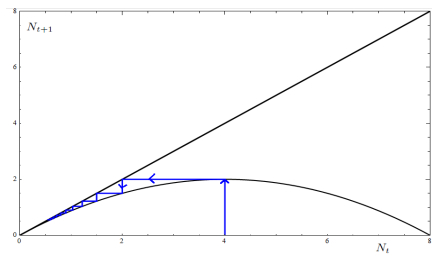
In this paper we analyze the behavior of the COVID-19 pandemic during a certain period of the year 2020 in the state of Mexico, Mexico. For this, we will use the discrete models obtained by the first, third and fourth authors of this work. The first is a one-dimensional model, and the second is two-dimensional, both non-linear. It is assumed that the population of the state of Mexico is constant and that the parameters used are the infection capacity, which we will initially assume to be constant, and the recovery and mortality parameters in that state. We will show that even when the statistical data obtained are disperse, and the process could be stabilized, this has been slow due to chaotic mitigation, creating situations of economic, social, health and political deterioration in that region of the country. We note that the observed results of the behavior of the epidemic during that period for the first variants of the virus have continued to be observed for the later variants, which has not allowed the eradication of the pandemic.
Citation: Erik A. Vázquez Jiménez, Jesús Martínez Martínez, Leonardo D. Herrera Zuniga, J. Guadalupe Reyes Victoria. Discrete models for analyzing the behavior of COVID-19 pandemic in the State of Mexico, Mexico[J]. Mathematical Biosciences and Engineering, 2023, 20(1): 296-317. doi: 10.3934/mbe.2023014
[1] | Rodolfo Acuňa-Soto, Luis Castaňeda-Davila, Gerardo Chowell . A perspective on the 2009 A/H1N1 influenza pandemic in Mexico. Mathematical Biosciences and Engineering, 2011, 8(1): 223-238. doi: 10.3934/mbe.2011.8.223 |
[2] | A Othman Almatroud, Noureddine Djenina, Adel Ouannas, Giuseppe Grassi, M Mossa Al-sawalha . A novel discrete-time COVID-19 epidemic model including the compartment of vaccinated individuals. Mathematical Biosciences and Engineering, 2022, 19(12): 12387-12404. doi: 10.3934/mbe.2022578 |
[3] | Roman Zúñiga Macías, Humberto Gutiérrez-Pulido, Edgar Alejandro Guerrero Arroyo, Abel Palafox González . Geographical network model for COVID-19 spread among dynamic epidemic regions. Mathematical Biosciences and Engineering, 2022, 19(4): 4237-4259. doi: 10.3934/mbe.2022196 |
[4] | A. Q. Khan, M. Tasneem, M. B. Almatrafi . Discrete-time COVID-19 epidemic model with bifurcation and control. Mathematical Biosciences and Engineering, 2022, 19(2): 1944-1969. doi: 10.3934/mbe.2022092 |
[5] | Pannathon Kreabkhontho, Watchara Teparos, Thitiya Theparod . Potential for eliminating COVID-19 in Thailand through third-dose vaccination: A modeling approach. Mathematical Biosciences and Engineering, 2024, 21(8): 6807-6828. doi: 10.3934/mbe.2024298 |
[6] | Jie Bai, Xiunan Wang, Jin Wang . An epidemic-economic model for COVID-19. Mathematical Biosciences and Engineering, 2022, 19(9): 9658-9696. doi: 10.3934/mbe.2022449 |
[7] | Rahat Zarin, Usa Wannasingha Humphries, Amir Khan, Aeshah A. Raezah . Computational modeling of fractional COVID-19 model by Haar wavelet collocation Methods with real data. Mathematical Biosciences and Engineering, 2023, 20(6): 11281-11312. doi: 10.3934/mbe.2023500 |
[8] | Avinash Shankaranarayanan, Hsiu-Chuan Wei . Mathematical modeling of SARS-nCoV-2 virus in Tamil Nadu, South India. Mathematical Biosciences and Engineering, 2022, 19(11): 11324-11344. doi: 10.3934/mbe.2022527 |
[9] | Ayako Suzuki, Hiroshi Nishiura . Transmission dynamics of varicella before, during and after the COVID-19 pandemic in Japan: a modelling study. Mathematical Biosciences and Engineering, 2022, 19(6): 5998-6012. doi: 10.3934/mbe.2022280 |
[10] | Ceyu Lei, Xiaoling Han, Weiming Wang . Bifurcation analysis and chaos control of a discrete-time prey-predator model with fear factor. Mathematical Biosciences and Engineering, 2022, 19(7): 6659-6679. doi: 10.3934/mbe.2022313 |
In this paper we analyze the behavior of the COVID-19 pandemic during a certain period of the year 2020 in the state of Mexico, Mexico. For this, we will use the discrete models obtained by the first, third and fourth authors of this work. The first is a one-dimensional model, and the second is two-dimensional, both non-linear. It is assumed that the population of the state of Mexico is constant and that the parameters used are the infection capacity, which we will initially assume to be constant, and the recovery and mortality parameters in that state. We will show that even when the statistical data obtained are disperse, and the process could be stabilized, this has been slow due to chaotic mitigation, creating situations of economic, social, health and political deterioration in that region of the country. We note that the observed results of the behavior of the epidemic during that period for the first variants of the virus have continued to be observed for the later variants, which has not allowed the eradication of the pandemic.
The COVID-19 pandemic that Mexico and the world in general is experiencing, since 2020, has led a large number of scientists to try solving the problem from different points of view and showing the scope it has within the economy, society, health and politics [1,2,3,4,5,6,7,8]. In particular, mathematicians around the world have responded by formulating models that have helped to partially understand the behavior of the infectious process at least in the first months since its appearance. Such models have followed the fundamental model called SIR by Kerman and Kendrick [9] by means of systems of differential equations. Many papers have been written modifying these primary systems throughout this period. However, the solutions obtained or approximated have not been able to give a satisfactory answer to the process, since in most cases their predictions are asymptotic and propose a stabilization and eradication of the pandemic, which has not happened to this day. The point is that the behavior of the solutions obtained is very simple in the configuration space, and it is not possible to observe at times the so-called outbreaks (as in Europe) or the chaotic behavior (as in America) of such process. On the other hand, several researchers in different parts of the world have proposed various models using discrete dynamics to study the behavior of the epidemic and have obtained partial, but altogether valuable results [10,11,12,13,14,15]. In our case, following the same line of research, we have preferred to use the analysis carried out by Reyes et al. in [16] using two discrete dynamic systems that allow one, from the first, one-dimensional, simple case, to predict chaotic behaviors of the process, because the homology of the solutions is richer than in the continuous case. The two-dimensional case proposed there shows a clearer approach to the process that continues to occur even to this day.
In this work we show the use of the model in [16] to study the behaviour of the epidemic process in the State of Mexico, Mexico, in 2020, using the proposed parameters and with statistical data obtained from reliable and relevant sources. We reiterate that this work is objectively theoretical by virtue of the fact that the data obtained in general by government agencies and institutes are not satisfactorily real, and for the problem of the epidemic it must necessarily be of this type to carry out a prevention project. We emphasize that in the period in which the model shown here was applied, there were still no vaccines, and the mitigation was carried out with isolations and contagion prevention methods in the region studied. However, the behavior of the epidemic for the following variants followed a similar route [1,2,3,4,17,19], sometimes weaker but still having repercussions on the economy, and social, health and political aspects.
The paper is organized as follows. We start in Section 2 by establishing the results in the simplest and most understandable way for a wide range of readers. In Section 3, we give the basic elements of the theory used for one-dimensional dynamical systems generated the classic quadratic family. In Section 4 we use the statistical data obtained for the pandemic in the state of Mexico, and we analyze it for the case when the used parameters are constant. In Section 5 we give the elements that define a chaotic one-dimensional dynamic system, and we use them in Section 6 to model a mitigation function for the basic contagion numbers with the data obtained for this Mexican state, which is chaotic in the first 12 months of contagion. Finally, in Section 7, using the mitigation function obtained, we define a two-dimensional dynamic system coupling it with the quadratic family, obtaining information on the eradication of a pandemic with weak chaos in this region of Mexico.
In this section we establish the main results of this work concerning the behavior of the pandemic in Result 1 for the case without mitigation and in Result 2 for the case in which preventive measures were taken in the analyzed period, leaving the proofs in Sections 4 and 6 respectively.
Result 1. The epidemic in the state of Mexico would have been stabilized (flattening the contagion curve) in the period of 2020–2021 in a natural way with the condition that both the basic contagion number, and the recovery and mortality parameters had remained constant.
Result 2. With the prevention policies applied for mitigation in the State of Mexico during the period from April to October 2020, the behavior of the pandemic in that region, although globally it was weakly chaotic, was eradicated slowly for almost all (in the topological and measurable senses) the initial conditions in the number of infected individuals and in the contagion capacity.
In this section we mention the necessary minimum number of elements [18] for the study of the first analysis, in the same way in which they were established in [16], with the aim of showing the possible current scenarios of the pandemic obtained with this model, assuming a constant contagion capacity. Also, we assume here, for simplicity, a closed population having a total number of (unknown) individuals N∞ [20].
In this work we denote the parameters of the contagion problem by
R0N∞=Probability of contagion of the population, α=Probability of recovery of the population, β=Rate of mortality of the population, |
subject to the conditions
0≤R0N∞,α,β≤1and0<α+β<1, | (3.1) |
where R0 is the number of individuals that can be infected by an already infected one, usually known as the basic reproductive number (at time t=0) in the epidemiological literature [21,22].
We will use here a modified logistic discrete model of May [23] and a fortnight as a unit of time, since in general it is the time in which the symptoms of COVID-19 appear after the contagion.
With these values in mind, if Nt denotes the number of total real infected individuals (not accumulated, including asymptomatic and suspected ones, because even when their values are unknown, together with the recovered ones that may recur, these are vectors for the next generation of infected ones) at time t∈N={0,1,2,…} after the epidemic begins, then, for constant R0,
Nt+1=R0N∞Nt(N∞−Nt)−αNt−βNt | (3.2) |
models the number of infected ones at time t+1, i.e., a unit of time after.
Here, the expression αNt+βNt acts, at first, as a control function to counterweight the contagion expression R0N∞Nt(N∞−Nt).
For the analysis of the dynamics of the unidimensional non-linear System (3.2), we use the properties of the function
f(Nt)=Nt+1=R0N∞Nt(N∞−Nt)−αNt−βNt. | (3.3) |
Rearranging this expression, the dynamical System (3.2) becomes
Nt+1=(R0−α−β)Nt−R0N∞N2t, | (3.4) |
defined in the domain D=[0,N∞(R0−α−β)/R0], with maximal number of infected individuals Nmax=N∞(R0−α−β)2/(4R0).
We have one well defined application f:D→D if and only if Nmax=N∞(R0−α−β)2/(4R0)≤N∞(R0−α−β)/R0, which follows, if and only if
α+β≤R0≤4+α+β. | (3.5) |
A straightforward computation shows that the fixed points for (3.2) are given by
Nt=0,NR0=N∞(R0−1−α−β)R0 | (3.6) |
However, if α+β≤R0≤1+α+β, then there only is one isolated fixed point NR0=0 in the domain D.
The fixed point NR0 (or Nt=0) of the map (3.3) is called hyperbolic if the absolute value |f′(NR0)|≠1 (or |f′(N0)|≠1, respectively).
For any initial condition N0∈D of the System (3.4), the sequence of points
Of(N0)={f(N0),f2(N0),f3(N0),f4(N0),⋯}={fk(N0)}∞k=1 |
is called the orbit of N0 under the the iteration map f. Here, fk indicates the composition of f with itself k times: fk=f∘f∘f⋯∘f.
We establish the following result on the stabilization of System (3.4).
Theorem 1. Let 0<N0<N∞(R0−α−β)R0 be an initial condition of infected individuals for System (3.2) at time t=0∈N. Then,
(a) If α+β≤R0≤1+α+β, then orbit Of(N0) is a monotonically decreasing sequence that converges to the disease-free hyperbolic fixed point NR0=0, i.e., fn→0, as n→∞.
(b) If 1+α+β<R0≤2+α+β, then orbit Of(N0) is a monotonically sequence that converges to the endemic hyperbolic fixed point NR0, i.e., fn(N0)→NR0, as n→∞.
(c) If 2+α+β<R0<3+α+β, then orbit Of(N0) is an oscillating (alternately increasing and decreasing) sequence that converges to the endemic hyperbolic fixed point NR0, i.e., fn(N0)→NR0, as n→∞.
Proof. If we compute the derivative of the generating function (3.3), we obtain
f′(Nt)=(R0−α−β)−2R0N∞Nt. | (3.7) |
(a) We evaluate the derivative in the unique fixed point NR0=0, and we obtain
f′(0)=R0−α−β, | (3.8) |
and it follows that |f′(0)|<1, if and only if, α+β≤R0≤1+α+β. This makes that NR0=0 be an attractor for the system.
By one graphical analysis we obtain that the subset
f([N∞(R0−α−β)2R0,N∞(R0−α−β)R0])⊂[0,N∞(R0−α−β)24R0] | (3.9) |
as we can see in the figure.
Moreover, using the same graphical analysis and condition (3.5), we have that for any initial condition
N0∈[0,N∞(R0−α−β)24R0], |
its orbit {fn(N0)} is decreasing and bounded. Therefore, it converges to some point, say M∈D. This is,
limn→∞fn(N0)=M. |
Applying again f and using the continuity, we have
f(M)=f(limn→∞fn(N0))=limn→∞f(fn(N0))=limn→∞fn+1(N0))=M, |
which implies that M is a fixed point of the system.
Therefore, M=0, since it is the unique fixed point inside the domain D, which proves the first item.
(b) If 1+α+β<R0, it is easy to see that there are the two aforementioned fixed points (3.6), and evaluating them in the derivative of f, we obtain
f′(0)=R0−α−β,f′(NR0)=−R0+α+β+2. | (3.10) |
In this way, we have that
f′(0)>1⇔1+α+β<R0,|f′(NR0)|≤1⇔1+α+β<R0≤3+α+β. | (3.11) |
In other words, the fixed point N0=0 is a repulsive point if 1+α+β<R0, and the fixed point NR0 is an attractor if 1+α+β<R0≤3+α+β.
For the case 1+α+β<R0≤2+α+β, we have, doing a graphical analysis that once again holds the contention of subsets (3.9).
In this way, from the concavity of the generating function f in the interval Ω=(0,N∞(R0−α−β)24R0) (see Figure 2), for any initial condition N0∈Ω, there exists one number 0<λ<1 such that
|f(N0)−NR0|=|f(N0)−f(NR0)|<λ|N0−NR0|. | (3.12) |
Applying again f, we have
|f2(N0)−NR0|=|f(f(N0))−NR0|<λ|f(N0)−NR0|<λ2|N0−NR0|, | (3.13) |
and inductively we obtain
|fn(N0)−NR0|<λn|N0−NR0|, | (3.14) |
which implies that orbit Of(N0) converges to the endemic fixed point NR0, i.e., fn(N0)→NR0, as n→∞. This proves the second item, and it can be seen in Figure 2.
(c) For the case 2+α+β<R0<3+α+β, let MR0 be the unique point in the interval (0,N∞(R0−α−β)2R0), such that f(MR0)=NR0.
We divide the interval
(0,N∞(R0−α−β)R0)=(0,MR0)∪[MR0,NR0]∪[NR0,N∞(R0−α−β)R0). |
Then, a simple graphical analysis shows that the image f([MR0,NR0]) is contained in the compact subset [MR0,fmax].
In a similar way, another graphical analysis shows that
f2([MR0,NR0])⊂[N∞(R0−α−β)2R0,NR0] |
as can be seen in the Figure 3.
Therefore, for any initial condition N0∈(MR0,NR0), it follows that f2(N0)∈[N∞(R0−α−β)2R0,NR0], and from this point on, the orbit Of(N0) is contained in that compact subset. This sequence is oscillating, which converges to the endemic hyperbolic fixed point NR0, as n→∞.
On the other hand, for any initial condition N0∈(0,MR0) there exists one positive integer k such that fk(N0) is contained in the subset [MR0,NR0], and therefore
limn→∞fn+k(N0)=NR0. |
Since
f([NR0,N∞(R0−α−β)R0])⊂(0,NR0), |
for any initial condition N0∈[NR0,N∞(R0−α−β)R0], its orbit Of(N0) converges to the endemic hyperbolic fixed point NR0, as shown in Figure 3.
This ends the proof.
In this subsection we will carry out an initial study of prevention for the pandemic in the state of Mexico, since its beginning in April of 2020, assuming that there was no mitigation action since then until now and that the parameters remain constant in all this period. We will continue considering the number of inhabitants of the state of Mexico as constant and equal to N∞, conforming to a closed population.
In order to understand the pandemic in the state of Mexico, we have obtained data from June of 2020 until September of 2021 from [24] and we have provided tables of contagion, mortality and rates of mortality, as shown in Appendix A.
We have obtained, by computing the mean from the early data, the following initial constant values for the parameters at the beginning of the pandemic in the state of Mexico, in the period of 2020–2021.
R0=2,α=0.20,β=0.19, |
and therefore, the generating function for dynamics becomes
f(Nt)=1.61Nt−2N∞N2t |
where the domain for the pandemic is
D=[0,N∞(2−0.2−0.19)2]=[0,0.80N∞], |
which indicates that, with this model, only 80 percent of the population N∞ of the state of Mexico faced the epidemic.
The maximum number of infected individuals should be
Nmax=N∞(2−0.2−0.19)24(2)=0.324N∞, |
that is, the maximum number of infected individuals should be 32.4 percent of the population N∞ of the state of Mexico.
The fixed points for the system obtained are
Nt=0(disease-free hyperbolic fixed point). |
and
NR0=N∞(2−1−0.2−0.19)2=0.30N∞(endemic hyperbolic fixed point) |
From Theorem 1, with R0=2, we are in the case
1+α+β<R0≤2+α+β, |
1.39<2≤2.39, |
and therefore, for any initial condition N0∈D, its orbit Of(N0) converges to the endemic fixed point NR0, i.e., fn(N0)→0.30N∞ as n→∞, which is shown in Figure 4.
This indicates that the epidemic stabilizes at a fixed point associated with the number of infected being 30 percent of the total population N∞ of the state of Mexico.
In other words, the epidemic would have been stabilized (called "flattening the contagion curve") in a natural way with these conditions if they did not change, both the basic contagion number and the recovery and mortality parameters. This ends the proof of Theorem 1.
However, this was not the case, and although the statistical data have shown for fortnightly periods that the basic reproductive number R0<1, the pandemic continues. We will discuss this in a later section.
To the aim of taking into account more values of R0, we note that System (3.2) makes sense for the problem in the case of a domain D when α+β≤R0≤4+α+β. Another direct calculation shows us that if
3+α+β<R0≤4+α+β, | (5.1) |
then f′(NR0)<−1, which yields the fixed point NR0 being repulsive for System (3.2), as well as Nt=0.
In this case, we have a possibly chaotic dynamics, which is due to compactness of the domain D and also to the fact that the orbit of any initial condition is repelled by both fixed points.
Let us introduce the following definitions [25].
Definition 1. A point NR0∈D is said to be periodic of (prime) period k≥1 for the System (3.3) if its orbit is finite of k-elements. That is, fk(NR0)=NR0 and fi(NR0)≠NR0 for i≤k−1 (i.e, the least positive is k).
In other words, a k-periodic point of System (3.3) is a fixed point of the iterate fk. In particular, a fixed point is 1-periodic, and so it is periodic of all the orders.
Definition 2. Let NR0∈D be a periodic point of prime period k. The point NR0 is called hyperbolic if |(fk)′(NR0)|≠1. The number (fk)′(NR0) is called the multiplier of the periodic point.
An important result toward finding simple conditions to characterize a chaotic system is given next [25].
Theorem 2. (Sarkovskii) Let f:D⊂R→D⊂R be a continuous function defined in a compact interval D, such that it has a periodic point of period three. Then, f has periodic points of all periods.
Let us introduce our last definition [25].
Definition 3. Let f:D→D be a continuous function associated with a discrete dynamical system
Nt+i=f(Nt). | (5.2) |
We say the following.
1. f is topologically transitive if for any open interval U⊂D its orbit {fn(U)} intersects any other open interval V⊂D.
2. f is sensibly dependent to initial conditions if for any pair of points closed to the same open set Nt,Ns∈U⊂D, the corresponding orbits {fn(Nt)},{fn(Ns)} depart from each other after some value n≥1.
Definition 4. Let f:D⊂R→D⊂R be a continuous function defined in a compact interval D. We say that the one-dimensional dynamical System (5.2) is chaotic if it satisfies the conditions from Definition 3, and the periodic points of f are dense in D.
The fundamental result which we will use in this work is due to Li and Yorke [26], and we state it as follows.
Theorem 3. (Li-Yorke) Let f:D⊂R→D⊂R be a continuous function defined in a compact interval D, such that it has a periodic point of period three. Then, the one-dimensional dynamical System (5.2) defined by f is chaotic.
In this way, showing that the Covid-19 pandemic might have chaotic behaviors, for certain values of the involved parameters, reduces to finding a periodic point of period three. This is shown in the next section.
In the time period studied, before vaccines and/or effective treatments were created to fight this disease, the only available control measures were lockdown, quarantine, etc. Indeed, in [22] it is said that applying mitigation strategies seems to decrease an initial R0 (at time t=0). Then, R (as a parameter) acts as one of the few available observable control measures in the curtailing of this disease.
Let us represent System (3.2) as the following affine control system:
Nt+1=f1(Nt)+f2(Nt)R=−(αNt+βNt)+Nt(1−NtN∞)R, | (6.1) |
where the parameter R is taken as one control input. Hereafter, we propose R=R(t)=Rt as a time-dependent control, the values of which are the estimated values of R, according to the adopted mitigation measures.
The aim of this section is to address how to eradicate the Covid-19 epidemic by regulating the contagion capacity, which will depend on discrete time units. In specific, we will provide conditions for the contagion capacity to decrease through a difference equation Rt+1=g(Rt), with g(Rt) a smooth enough map, defined in the interval [0,4+α+β], so that its solution decreases per unit of time and in turn leads to eventually eradicating the disease.
We shall call from now on the smooth function g:[0,4+α+β]→[0,4+α+β] the mitigation map.
Let us take the one dimensional discrete dynamical System (6.1) together with the difference equation Rt+1=g(Rt) as the following two-dimensional non-linear discrete dynamical system:
{Nt+1=(Rt−α−β)Nt−RtN∞N2t,Rt+1=g(Rt), | (6.2) |
defined in the rectangular set ˆD=[0,N∞(R0−α−β)/R0]×[0,4+α+β].
Let Rt=R(t) be a smooth function, obtained by integrating the discrete equation, Rt+1=g(Rt), for g a smooth mitigation map defined in the interval [0,4+α+β].
With data for the basic number of contagion Rt in the state of Mexico obtained from the AMMA model of CONACYT, Mexico [27], in the months of April, May, June, August, September and October of 2020, which are shown in Appendix B, we have in fortnights the quartic polynomial function
g(Rt)=1.8607R5t−17.355R4t+47.874R3t−51.806R2t+20.026Rt |
in the variable Rt by interpolating the points in the plane (Rt,Rt+1)
(1.7,1),(1.4,1.2),(0.7,1.2),(1.2,0.8),(1.0,0.6),(0,0). |
This polynomial fits (with a very good approximation) this process in the well observed interval of data for the capacity of contagion [0.6,1.6], which is a suitable interval for mitigation contained in the total interval of mitigation [0,4.39], as can be seen in Figure 5.
The fixed point in that interval is Rp=0.822, and since
g′(Rt)=9.3035R4t−69.42R3t+143.322R2t−103.612Rt+20.026, |
|g′(Rp)|=|g′(0.822)|=|−2.4|=2.4.
Therefore, in the global interval [0,4.39] there are two repulsive fixed points, at Rt=0 and Rp=0.822. Further, the derivative satisfies |g′(Rp)|≥1.
The dynamics of the system
Rt+1=1.8607R5t−17.355R4t+47.874R3t−51.806R2t+20.026Rt | (6.3) |
in the aforementioned interval [0.6,1.6] for the initial condition R0=0.7 can be seen in Figure 6.
On the other hand, a long but straight forward computation shows us the existence of the eight points of period three of g which we list above,
0.0,0.6601,0.6871,0.8242,0.8942,1.109,1.246,1.348. |
In fact, the point Rt=0.6871 is a periodic point of period 3 for the map g, as it can be obtained by the aforementioned computation, and its orbit is shown in the Figure 7.
By the Sarkovskii's Theorem [25], there is a dense set of periodic points of all periods for this mitigation function. Consequently, by the Yorke and Li Theorem [25], the map g is chaotic, as shown in Figure 6.
The System (6.2), with this mitigation function, becomes
{Nt+1=f(Nt,Rt)=(Rt−0.39)Nt−RtN∞N2t,Rt+1=g(Rt)=1.8607R5t−17.355R4t+47.874R3t−51.806R2t+20.026Rt, | (7.1) |
and it will be analyzed in the observable domain Ω=[0,0.8N∞]×[0.6,1.6].
The obtained dynamical relation is very difficult to integrate to obtain a time-dependent mitigation relationship R=R(t), and it will be qualitatively used to display results on the first coordinate of System (7.1).
As before, we assume still a closed population with a total number of individuals N∞.
In order to study the dynamics of (7.1), first, we obtain the fixed points of System (7.1) by solving the algebraic system of equations
{Nt=(Rt−α−β)Nt−RtN∞N2t,Rt=1.8607R5t−17.355R4t+47.874R3t−51.806R2t+20.026Rt, | (7.2) |
which gives us the points p1=(0,0) and p2=(0,Rp)=(0,0.822) in the whole set Λ=[0,0.8N∞]×[0,4.39].
We begin by defining the 2-real valued map
F(Nt,Rt)=((Rt−α−β)Nt−RtN2t/N∞, g(Rt)) | (7.3) |
in Λ, whose iterates determine the orbits of the System (7.1) as (Nt+1,Rt+1)=F(Nt,Rt).
In this case, the Jacobian of (7.3) is
DF(Nt,Rt)=(Rt−0.39−2RtNtN∞Nt−N2tN∞0g′(Rt)), | (7.4) |
and here (′) denotes the ordinary derivative with respect to Rt.
a. Therefore, the Jacobian matrix at the point p1=(0,0) is
DF(0,0)=(−0.3900g′(0)). | (7.5) |
A straightforward computation gives us the eigenvalues, μ1=−0.39, which in absolute value is less than 1, and μ2=20.02, which is great than 1.
It is easy to see that the coordinate axis Rt is an invariant under the System (7.1), which follows from the equality
F(0,Rt)=(0,g(Rt)), | (7.6) |
That invariance of the axis Rt, implies that in this direction p1 is a repulsive point for all the orbits close to this fixed point with initial conditions (0,R0). A similar analysis shows that the axis Nt (where Rt=0) is also invariant under System (6.2), which follows from the equality
F(Nt,0)=(−0.39Nt,0). | (7.7) |
Therefore, any orbit with initial conditions (N0,0) converges to the point p1=(0,0), along this axis.
We conclude that the fixed point p1=(0,0) is a saddle fixed point for the system.
b. For the fixed point p2=(0,Rp)=(0,0.822), we have the Jacobian matrix
DF(0,Rp)=(0.43200−2.4), | (7.8) |
whose eigenvalues are μ1=0.432, which in absolute value is less that 1, and μ2=−2.4, which in absolute value is great that 1. This implies that such point is also a saddle fixed point for the system.
We remark that the straight line Rt=Rp=0.822, (where g(Rp)=Rp) is also an invariant set under System (6.2), which follows from the equality
F(Nt,0.822)=(0.432Nt−N2t/N∞,0.822) | (7.9) |
which implies that any orbit with initial conditions (N0,0.822) in such line converges to the fixed point p2=(0,0.822).
It follows that the stable manifold for p2=(0,Rp) is the set Ws(Rp)=Λ∖{Rt=0} [25,28].
On the other hand, from the invariance of the axis Rt, any orbit with initial conditions (0,N0) remains in such axis, preserving the dynamics of System (6.3).
We observe that the aforementioned mitigation map turns the System (7.1) for this case into a weak chaotic one. That is, the whole system is not chaotic in the sense of Li and Yorke [25], but, as we have mentioned before, it is chaotic in the sense of Li and Yorke only in the vertical coordinate Rt, and this makes unpredictable the convergence of the system's orbits for any initial condition (N0,R0) which is not contained on the invariant coordinate axes or on the also invariant line Rt=Rp. We shall consider this type of system to be weak chaotic [16].
It is well known that, in general, a manifold, whether stable or unstable, is not a differentiable manifold in the sense of differential topology, but sometimes they are unions of pieces of differentiable manifolds. For the case of planar maps, sometimes one dimensional manifolds are unions of piecewise smooths curves [29].
The one dimensional unstable manifold Wu(p2) of System (7.1) at the point p2 is not the Rt-axis because by Sarkovskii's Theorem and in any neighborhood of p2 the subset of periodic point is dense and all those orbits returns to that neighbourhood. We note that the local stable manifold Wuloc(p2) is also not contained in the Rt-axis for the same reason. The one dimensional (curve) unstable manifold γ=Wu(p2) is found by iterating the local unstable manifold Wuloc(p2) using the technique of Yorke et al. in [30] (Figure 8).
Following the proof of Theorem 3 in [16], we can show that the one dimensional unstable and the stable global invariant manifolds Wu(p2) and Ws(p2) intersect transversally. Therefore, System (7.1) has transverse homoclinic points. Moreover, we can obtain a vertical "homoclinic tangle", and a high enough forward iterate of F in the obtained vertical "homoclinic tangle" will give us a vertical Smale horseshoe map [25,28,31] (Figure 8).
However, since the set intersection between the stable Ws(p2) and unstable Wu(p2) manifolds at point p2 has zero dimension and therefore zero Lebesgue measure, in topological and measurable terms, we can, summarizing the previous discussion, establish the following main result.
Theorem 4. The mitigation System (7.1) is vertically chaotic near the eradication point p2=(0,Rp)=(0,0.822). Therefore, although it is globally weak chaotic, the system eradicates slowly the pandemic for almost all (in the topological and measurable senses) initial conditions.
In fact, the generic behavior of the dynamical system is shown discretely in Figure 9.
The expected behavior of the actual infected function N(t)=Nt as a function of the time t measured in fortnights for the first 13 units since April 2020 is shown in Figure 10, and the eradication of the pandemic would have been expected during that period of time, according to the data obtained and mentioned above.
On the other hand, the expected actual capacity of the contagion function R(t)=Rt=, also as a function of the time t measured in fortnights for the first 15 fortnights since April 2020, is shown in Figure 11. This function has a chaotic behavior throughout the period, which causes the N(t) function to show a slow eradication trend. This ends the proof of Theorem 2.
The two-dimensional model defined by a discrete dynamic system and built with the statistical data obtained from the mentioned sources shows us that, for almost all initial conditions in the number of infected and in the basic number of infection, the eradication of the pandemic would have been possible, if we had been conserved, the conditions of contagion (variable), recovery and mortality (constant) even when the mitigation had a chaotic behavior by fortnightly units. However, the behavior of the epidemic for the following variants in 2021 and 2022 followed a similar way, but they are still having repercussions on the economy and social, health and political aspects. In a particular conclusion, the mitigation and prevention strategy in the State of Mexico followed only economic and health objectives, neglecting social aspects (mental health, divorces, femicides, etc.) and influencing political problems (through political parties, opposition to the current Mexican government, as well as the problem of sale and distribution of vaccines, social movements, etc.).
The authors would like to thank the Tecnologico de Estudios Superiores de Huixquilucan (TESH) and the Consejo Mexiquense de Ciencia y Tecnología (COMECYT) for the financial support to carry out this research. Also, the authors thank to mathematician Hiram Velásquez Alamilla from UAM-I, for his support in giving this paper its final Tex format.
The authors declare there is no conflict of interest.
We show here the obtained data from June of 2020 until September of 2021, from [24], providing the tables of contagion, mortality and the corresponding rates in the state of Mexico.
Fortnight | Mean of Contagious |
15-jun | 606.9 |
1-jul | 680.9 |
15-jul | 663.6 |
1-ago | 538.6 |
15-ago | 547.2 |
1-sep | 439.2 |
15-sep | 456.1 |
1-oct | 426.5 |
15-oct | 639.1 |
1-nov | 478.6 |
15-nov | 365.5 |
1-dic | 528.7 |
15-dic | 994.9 |
1-ene | 1210.1 |
15-ene | 1097.1 |
1-feb | 1788.2 |
15-feb | 999.6 |
1-mar | 782.7 |
15-mar | 624.6 |
1-abr | 463.4 |
15-abr | 413.8 |
1-may | 309.6 |
15-may | 197 |
1-jun | 186.53 |
15-jun | 315.57 |
1-jul | 291.06 |
15-jul | 601.07 |
1-ago | 1395.35 |
15-ago | 1670.71 |
1-sep | 1227.76 |
Fortnight | Mean of Mortality | M. Mortality/M. Contagious |
21-jun. | 68.1 | 0.11 |
07-jul | 140.8 | 0.21 |
21-jul | 66.7 | 0.10 |
07-ago | 63.5 | 0.12 |
21-ago | 54.8 | 0.10 |
07-sep | 43.1 | 0.10 |
21-sep | 48.8 | 0.11 |
07-oct | 60.9 | 0.14 |
21-oct | 25.8 | 0.04 |
07-nov | 34.5 | 0.07 |
21-nov | 297.9 | 0.82 |
07-dic | 516.1 | 0.98 |
21-dic | 102.2 | 0.10 |
07-ene | 126.5 | 0.10 |
21-ene | 169.9 | 0.15 |
07-feb | 209.2 | 0.12 |
21-feb | 155.1 | 0.16 |
07-mar | 123.1 | 0.16 |
21-mar | 80.9 | 0.13 |
07-abr | 75.6 | 0.16 |
21-abr | 114.2 | 0.28 |
07-may | 40.4 | 0.13 |
21-may | 32.2 | 0.16 |
07-jun | 144 | 0.77 |
21-jun | 13.4 | 0.04 |
07-jul | 17.3 | 0.06 |
21-jul | 20.4 | 0.03 |
07-ago | 54.8 | 0.04 |
21-ago | 87.4 | 0.05 |
The data for the basic numbers of contagion Rt in the state of Mexico obtained from the AMMA model of CONACYT, Mexico [27], in the months of April, May, June, August, September and October of 2020, give the following quantities in fortnights, as can be seen in the figures below.
– From Rt=1.7 on 28th April to Rt+1=1.0 on 27th May (see Figure A1).
– From Rt=1.4 on 28th may to Rt+1=1.2 on 26th June (see Figure A2).
– From Rt=0.7 on 27th August to Rt+1=1.2 on 24th September (see Figure A3).
– From Rt=1.2 on 09th September to Rt+1=0.8 on 08th October (see Figure A4).
– From Rt=1.0 on 23th September to Rt+1=0.6 on 22th October (see Figure A5).
[1] | M. Coccia, Pandemic prevention: Lessons from COVID-19 pandemic, Encyclopaedia, 1 (2021), 433–444. https://doi.org/103390/Encyclopaedia1020036 |
[2] |
M. Coccia, High health expenditures and low exposure of population to air pollution as critical factors that can reduce fatality rate in COVID-19 pandemic crisis: A global analysis, Environ. Res., 199 (2021), 111339. https://doi.org/10.1016/j.envrers.2021.111339 doi: 10.1016/j.envrers.2021.111339
![]() |
[3] |
M. Coccia, Preparedness of countries to face COVID-19 pandemic crisis: Strategic position and underlying structural factors to support strategies of prevention of pandemic threats, Environ. Res., 203 (2022), 111678. https://doi.org/10.1016/j.envrers.2021.111678 doi: 10.1016/j.envrers.2021.111678
![]() |
[4] |
M. Coccia, COVID-19 pandemic crisis over 2020 (with lock downs) and 2021 (with vaccinations): Similar effects for seasonality and environmental factors, Environ. Res., 208 (2022), 112711. https://doi.org/10.1016/j.envrers.2022.112711 doi: 10.1016/j.envrers.2022.112711
![]() |
[5] | X. Chen, B. Yu, First two months of the 2019 Corona virus Disease (COVID-19) epidemic in China: Realtime surveillance and evaluation with a second derivative model, Global Health Res. Policy, 5 (2020). https://doi.org/10.1186/s41256-020-00137-4 |
[6] |
F. Louchet, A brief theory of epidemic kinetics, Biology, 9 (2020), 134. https://doi.org/10.3390/biology9060134 doi: 10.3390/biology9060134
![]() |
[7] |
A. Nunez-Delgado, E. Bontemoi, M. Coccia, M. Kumar, K. Farkas, J. L. Domingo, SARS-COV-2 and other pathogenic micro-organisms in the environment, Environ. Res., 201 (2021), 111606. https://doi.org/10.1016/j.envrers.2021.111606 doi: 10.1016/j.envrers.2021.111606
![]() |
[8] | S. Sanche, Y. T. Lin, C. Xu, E. Romero-Severson, N. Hengartner, R. Ke, High contagiousness and rapid spread of severe acute respiratory syndrome coronavirus 2, Emerg. Infect. Dis., 26 (2020), https://doi.org/10.3201/eid2607.200282 |
[9] |
W. Kermack, A. McKendrick, A contribution to the mathematical theory of epidemics, Proc. Royal Soc. A., 115 (1927), 700–721. https://doi.org/10.3201/eid2607.200282 doi: 10.3201/eid2607.200282
![]() |
[10] | M. Canals, C. Cuadrado, A. Canals, COVID-19 in Chile: The usefulness of simple epidemic models in practice, Medwave, 8119 (2021). https://doi.org/10.5867/medwave.2021.01.8119 |
[11] |
T. S. Fernandes, Chaotic model for COVID-19 grow factor, Res. Biomed. Eng., 38 (2022), 299–303. https://doi.org/10.1007/s42600-020-00077-5 doi: 10.1007/s42600-020-00077-5
![]() |
[12] |
J. Guo, A. Wang, W. Zhou, Y. Gong, S. R. Smith, Discrete epidemic modelling of COVID-19 transmission in Shaanxi Providence with media reporting and imported caes, Math. Biosci. Eng., 19 (2022), 1388–1410. https://doi.org/10.3934/mbe.2022064 doi: 10.3934/mbe.2022064
![]() |
[13] |
M. T. Li, G. Q. Sun, J. Zhang, Y. Zhao, X. Pei, L. Li, et al., Analysis of COVID-19 transmission in Shanxi Province with discrete time imported cases, Math. Biosci. Eng., 17 (2020), 3710. https://doi.org/10.3934/mbe.2020208 doi: 10.3934/mbe.2020208
![]() |
[14] |
A. Mourad, F. Mroue, Z. Taha, Stochastic mathematical models for the spread of COVID-19: A model epidemiological approach, Math. Med. Biol., 39 (2022), 49–76. https://doi.org/10.1093/imammb/dqab019 doi: 10.1093/imammb/dqab019
![]() |
[15] |
T. Sitthiwirattham, A. Zeb, S. Chasreechai, M. Tilioua, S. Djilail, Analysis of a discrete mathematical COVID-19 model, Results Physicsy, 28, (2021), 104668. https://doi.org/10.1016/j.rinp.2021.104668 doi: 10.1016/j.rinp.2021.104668
![]() |
[16] | J. G. Reyes-Victoria, J. Solis-Daun, L. Herrera-Zuniga, E. Vázquez-Jiménez, Discrete model for prevention of Covid-19 Pandemic for closed populations: Stabilization, eradication and weak chaos, preprint, 2022. |
[17] |
E. Callaway, Fast spreading COVID-19 variant can elude immune responses, Nature, 589 (2021), 500–5001. https://doi.org/10.1038/d41586-021-00121-z doi: 10.1038/d41586-021-00121-z
![]() |
[18] |
E. B. Postnikov, Estimation of COVID-19 dynamics "on a back-of-envelop": Does the simplest SIR model provide quantitative parameters and predictions?, Chaos Solitons Fractals, 135 (2020), 109841. https://doi.org/10.1016/j.chaos.2020.109841 doi: 10.1016/j.chaos.2020.109841
![]() |
[19] |
C. Aschwanden, Five reasons why COVID-19 heard immunity is probably impossible, Nature, 591 (2021), 520–522. https://doi.org/10.1038/d41586-021-00728-2 doi: 10.1038/d41586-021-00728-2
![]() |
[20] | J. G. Reyes-Victoria, J. Solis-Daun, L. Herrera-Zuniga, Epidemic model as an aid to control decision-making in the Covid-19 Pandemic for closed populations: Qualitative analysis and Chaos, COVID-19 Res. Commun., 2020. |
[21] | Z. Cao, Q. Zhang, X. Lu, D. Pfeiffer, Z. Jia, H. Song, et al., Estimating the effective reproduction number of the 2019-nCoV in China, Biology, (2020). https://doi.org/10.1101/2020.01.27.20018952 |
[22] |
Y. Liu, A. Gayle, A. Wilder-Smith, J. Rocklöv, The reproductive number of COVID-19 is higher compared to SARS coronavirus, J. Travel Med., 27 (2020), 1–4. https://doi.org/10.1093/jtm/taaa021 doi: 10.1093/jtm/taaa021
![]() |
[23] |
R. May, Simple mathematical models with very complicated dynamics, Nature, 261 (1976), 459–467. https://doi.org/10.1038/261459a0 doi: 10.1038/261459a0
![]() |
[24] | John Hopkins University, Medicine, 2020. Available from: https://coronavirus.jhu.edu/map.html. |
[25] | R. Devaney, An Introduction to Chaotic Dynamical Systems, 2nd edition, Addison-Wesley, USA, 1989. |
[26] |
T. Y. Li, J. Yorke, Period three implies chaos, The Amer. Math. Monthly, 82 (1975), 985–992. https://doi.org/10.1007/978-0-387-21830-4_6 doi: 10.1007/978-0-387-21830-4_6
![]() |
[27] | A. Christen, M. Capistán, M. Daza-Torres, A. Capella Instituto de Matemáticas UNAM, U.C. DAVIS, CIMAT-CONACYT, CONACYT, Gobierno de México, (82: 10), (2020-2021). |
[28] | J. Guckenheimer, P. Holmes, Nonlinear Oscillations, Dynamical Systems, and Bifurcations of Vector Fields, 1st edition, Springer, New York, 1983. |
[29] |
R. McGehee, A stable manifold theorem for degenerate fixed points with applications to celestial mechanics, J. Differ. Equations, 14 (1973), 70–88. https://doi.org/10.1016/0022-0396(73)90077-6 doi: 10.1016/0022-0396(73)90077-6
![]() |
[30] | E. Zhipingyou, E. Kostelich, J. Kostelich, J. Yorke, Calculating stable and unstable manifolds, Int. J. Bifurcation Chaos, 1 (2012). https://doi.org/10.1142/S0218127491000440 |
[31] | J. Palis, W. De Melo, Geometric Theory of Dynamical Systems, 1st edition, Springer-Verlag, New York, 1982. |
Fortnight | Mean of Contagious |
15-jun | 606.9 |
1-jul | 680.9 |
15-jul | 663.6 |
1-ago | 538.6 |
15-ago | 547.2 |
1-sep | 439.2 |
15-sep | 456.1 |
1-oct | 426.5 |
15-oct | 639.1 |
1-nov | 478.6 |
15-nov | 365.5 |
1-dic | 528.7 |
15-dic | 994.9 |
1-ene | 1210.1 |
15-ene | 1097.1 |
1-feb | 1788.2 |
15-feb | 999.6 |
1-mar | 782.7 |
15-mar | 624.6 |
1-abr | 463.4 |
15-abr | 413.8 |
1-may | 309.6 |
15-may | 197 |
1-jun | 186.53 |
15-jun | 315.57 |
1-jul | 291.06 |
15-jul | 601.07 |
1-ago | 1395.35 |
15-ago | 1670.71 |
1-sep | 1227.76 |
Fortnight | Mean of Mortality | M. Mortality/M. Contagious |
21-jun. | 68.1 | 0.11 |
07-jul | 140.8 | 0.21 |
21-jul | 66.7 | 0.10 |
07-ago | 63.5 | 0.12 |
21-ago | 54.8 | 0.10 |
07-sep | 43.1 | 0.10 |
21-sep | 48.8 | 0.11 |
07-oct | 60.9 | 0.14 |
21-oct | 25.8 | 0.04 |
07-nov | 34.5 | 0.07 |
21-nov | 297.9 | 0.82 |
07-dic | 516.1 | 0.98 |
21-dic | 102.2 | 0.10 |
07-ene | 126.5 | 0.10 |
21-ene | 169.9 | 0.15 |
07-feb | 209.2 | 0.12 |
21-feb | 155.1 | 0.16 |
07-mar | 123.1 | 0.16 |
21-mar | 80.9 | 0.13 |
07-abr | 75.6 | 0.16 |
21-abr | 114.2 | 0.28 |
07-may | 40.4 | 0.13 |
21-may | 32.2 | 0.16 |
07-jun | 144 | 0.77 |
21-jun | 13.4 | 0.04 |
07-jul | 17.3 | 0.06 |
21-jul | 20.4 | 0.03 |
07-ago | 54.8 | 0.04 |
21-ago | 87.4 | 0.05 |
Fortnight | Mean of Contagious |
15-jun | 606.9 |
1-jul | 680.9 |
15-jul | 663.6 |
1-ago | 538.6 |
15-ago | 547.2 |
1-sep | 439.2 |
15-sep | 456.1 |
1-oct | 426.5 |
15-oct | 639.1 |
1-nov | 478.6 |
15-nov | 365.5 |
1-dic | 528.7 |
15-dic | 994.9 |
1-ene | 1210.1 |
15-ene | 1097.1 |
1-feb | 1788.2 |
15-feb | 999.6 |
1-mar | 782.7 |
15-mar | 624.6 |
1-abr | 463.4 |
15-abr | 413.8 |
1-may | 309.6 |
15-may | 197 |
1-jun | 186.53 |
15-jun | 315.57 |
1-jul | 291.06 |
15-jul | 601.07 |
1-ago | 1395.35 |
15-ago | 1670.71 |
1-sep | 1227.76 |
Fortnight | Mean of Mortality | M. Mortality/M. Contagious |
21-jun. | 68.1 | 0.11 |
07-jul | 140.8 | 0.21 |
21-jul | 66.7 | 0.10 |
07-ago | 63.5 | 0.12 |
21-ago | 54.8 | 0.10 |
07-sep | 43.1 | 0.10 |
21-sep | 48.8 | 0.11 |
07-oct | 60.9 | 0.14 |
21-oct | 25.8 | 0.04 |
07-nov | 34.5 | 0.07 |
21-nov | 297.9 | 0.82 |
07-dic | 516.1 | 0.98 |
21-dic | 102.2 | 0.10 |
07-ene | 126.5 | 0.10 |
21-ene | 169.9 | 0.15 |
07-feb | 209.2 | 0.12 |
21-feb | 155.1 | 0.16 |
07-mar | 123.1 | 0.16 |
21-mar | 80.9 | 0.13 |
07-abr | 75.6 | 0.16 |
21-abr | 114.2 | 0.28 |
07-may | 40.4 | 0.13 |
21-may | 32.2 | 0.16 |
07-jun | 144 | 0.77 |
21-jun | 13.4 | 0.04 |
07-jul | 17.3 | 0.06 |
21-jul | 20.4 | 0.03 |
07-ago | 54.8 | 0.04 |
21-ago | 87.4 | 0.05 |