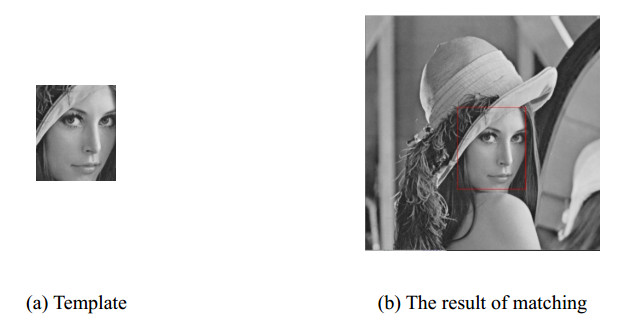
This paper proposes an anti-rotation template matching method based on a portion of the whole pixels. To solve the problem that the speed of the original template matching method based on NCC (Normalized cross correlation) is too slow for the rotated image, a template matching method based on Sub-NCC is proposed, which improves the anti-jamming ability of the algorithm. At the same time, in order to improve the matching speed, the rotation invariant edge points are selected from the rotation invariant pixels, and the selected points are used for rough matching to quickly screen out the unmatched areas. The theoretical analysis and experimental results show that the accuracy of this method is more than 95%. For the search map at any angle with the resolution at the level of 300,000 pixel, after selecting the appropriate pyramid series and threshold, the matching time can be controlled to within 0.1 s.
Citation: Yifan Zhang, Zhi Zhang, Shaohu Peng, Dongyuan Li, Hongxin Xiao, Chao Tang, Runqing Miao, Lingxi Peng. A rotation invariant template matching algorithm based on Sub-NCC[J]. Mathematical Biosciences and Engineering, 2022, 19(9): 9505-9519. doi: 10.3934/mbe.2022442
[1] | Zhenjun Tang, Yongzheng Yu, Hanyun Zhang, Mengzhu Yu, Chunqiang Yu, Xianquan Zhang . Robust image hashing via visual attention model and ring partition. Mathematical Biosciences and Engineering, 2019, 16(5): 6103-6120. doi: 10.3934/mbe.2019305 |
[2] | Jian Zhang, Yan Zhang, Cong Wang, Huilong Yu, Cui Qin . Binocular stereo matching algorithm based on MST cost aggregation. Mathematical Biosciences and Engineering, 2021, 18(4): 3215-3226. doi: 10.3934/mbe.2021160 |
[3] | Diguo Zhai, Xinqi Bao, Xi Long, Taotao Ru, Guofu Zhou . Precise detection and localization of R-peaks from ECG signals. Mathematical Biosciences and Engineering, 2023, 20(11): 19191-19208. doi: 10.3934/mbe.2023848 |
[4] | Jiali Tang, Yan Wang, Chenrong Huang, Huangxiaolie Liu, Najla Al-Nabhan . Image edge detection based on singular value feature vector and gradient operator. Mathematical Biosciences and Engineering, 2020, 17(4): 3721-3735. doi: 10.3934/mbe.2020209 |
[5] | Tinghuai Ma, Lei Guo, Xin Wang, Yurong Qian, Yuan Tian, Najla Al-Nabhan . Friend closeness based user matching cross social networks. Mathematical Biosciences and Engineering, 2021, 18(4): 4264-4292. doi: 10.3934/mbe.2021214 |
[6] | Haibo Li, Juncheng Tong . A novel clustering algorithm for time-series data based on precise correlation coefficient matching in the IoT. Mathematical Biosciences and Engineering, 2019, 16(6): 6654-6671. doi: 10.3934/mbe.2019331 |
[7] | Yan Liu, Bingxue Lv, Yuheng Wang, Wei Huang . An end-to-end stereo matching algorithm based on improved convolutional neural network. Mathematical Biosciences and Engineering, 2020, 17(6): 7787-7803. doi: 10.3934/mbe.2020396 |
[8] | Naigong Yu, Hongzheng Li, Qiao Xu . A full-flow inspection method based on machine vision to detect wafer surface defects. Mathematical Biosciences and Engineering, 2023, 20(7): 11821-11846. doi: 10.3934/mbe.2023526 |
[9] | Cheonshik Kim, Dongkyoo Shin, Ching-Nung Yang . High capacity data hiding with absolute moment block truncation coding image based on interpolation. Mathematical Biosciences and Engineering, 2020, 17(1): 160-178. doi: 10.3934/mbe.2020009 |
[10] | Qiuling Wu, Dandan Huang, Jiangchun Wei, Wenhui Chen . Adaptive and blind audio watermarking algorithm based on dither modulation and butterfly optimization algorithm. Mathematical Biosciences and Engineering, 2023, 20(6): 11482-11501. doi: 10.3934/mbe.2023509 |
This paper proposes an anti-rotation template matching method based on a portion of the whole pixels. To solve the problem that the speed of the original template matching method based on NCC (Normalized cross correlation) is too slow for the rotated image, a template matching method based on Sub-NCC is proposed, which improves the anti-jamming ability of the algorithm. At the same time, in order to improve the matching speed, the rotation invariant edge points are selected from the rotation invariant pixels, and the selected points are used for rough matching to quickly screen out the unmatched areas. The theoretical analysis and experimental results show that the accuracy of this method is more than 95%. For the search map at any angle with the resolution at the level of 300,000 pixel, after selecting the appropriate pyramid series and threshold, the matching time can be controlled to within 0.1 s.
As a basic technology, template matching is widely used in the fields of image processing and computer vision [1,2,3,4,5]. In many application scenarios, such as a robot arm grasping target and defect detection, the pose of the target and the height of the camera have been fixed, so the image to be matched often does not have scale change, occlusion or serious deformation. In such scenes, the matching task can be completed by using the well-known image features, and the matching method based on deep- learning is not cost-effective. In addition, some matching algorithms focus on solving the interference caused by image deformation, such as BBS [6] (Best-Buddies Similarity), DDIS [6] (Deformable Diversity Similarity), etc. Generally, the more robust the algorithm is to image deformation, the longer the matching time of the algorithm is. Feature-based methods also fail matching if not enough feature points are extracted, such as SIFT [7] (Scale-invariant Feature Transform), SURF [8] (Speeded Up Robust Features), etc. Gray-based method matching accuracy is not affected by the type of template map and is preferred for such scenarios.
The most intuitive feature in an image is the pixel value, which is most sensitive to noise. SSD and SAD [9] adopt different error measures, add up the differences of pixel values of each corresponding pixel of two images, and locate the template according to the difference values. The two methods are simple in principle, but there is still much room for improvement in matching speed, and the algorithm is sensitive to light changes. On this basis, various improved algorithms have been proposed [10,11] to increase the anti-jamming ability of the algorithm and improve the matching speed. NCC [12] uses the degree of image correlation as a measure of similarity and is robust to light variations due to normalization operations. The zero-mean normalized cross-correlation (ZNCC) [13] proposed on this basis has some resistance to additive and multiplicative noise. Compared with SAD and SSD, NCC traverses all pixels equally, and because of its more complex similarity measurement formula, it is difficult to guarantee real-time matching when the search map is large. To improve NCC matching speed, Tsai D-M et al. [14] proposed a summation table method to calculate the mean and square differences of images. For the gray sum operation of all pixel points in a rectangular area, the number of calculations can be reduced to three times by finding the table. Noticing that the molecular part in the NCC measurement formula is similar to signal convolution, Cao Liangcai et al. [15] used the Fast Fourier Transform (FFT) to convert the molecular part in the NCC formula to a frequency domain calculation and improve matching speed. Since most of the regions in the search graph are not optimal matching regions, Mattoccia et al. [16] proposed a greedy algorithm based on the Cauchy-Schwarz inequality. When calculating similarity using NCC, for the unmatched locations, if the inequality conditions are not met, the computation of similarity for the regions will be stopped directly, and the matching time will be reduced. Fouda [17] converted a two-dimensional image into one-dimensional vector information by summarizing the intensity values of all rows in the vertical direction, and improved computational speed by reducing the amount of data.
Although all of the above methods guarantee the matching accuracy, they have some limitations, such as not being suitable for rotated image matching, or matching speed is still slow. To further improve the matching speed while ensuring the accuracy of the algorithm, a Sub-NCC algorithm is proposed in this paper. The Sub-NCC algorithm adopts a hierarchical search strategy, selects rotation invariant points at the top level to ensure the accuracy of matching and combines the image shape with ZNCC to quickly screen the un-matched region. For non-top-level matching, a two-step search method is adopted to further improve the matching speed.
The remainder of this paper is organized as follows: In Section 2, the original ZNCC method is briefly described. In Section 3, the method we proposed is introduced in detail. Experiment is shown in Section 4, and the conclusion is summarized in Section 5.
The formula of ZNCC is given by Eq (1.1):
R(x,y)=1n∗∑(u,v)?Tt(u,v)−mt√st2∗f(r+u,c+v)−mf(r,c)√sf2(r,c) | (1.1) |
where mt and mf respectively represent the average values of the pixel values of the template image and the search area, and st2 and sf2 respectively represent the variances of the pixel values of the template image and the search area. The mean value of the image reflects the concentration trend of the overall gray value of the image. The molecular part in the ZNCC formula subtracts the mean value to eliminate the influence of additive noise. The variance of the image reflects the overall deviation degree, and the denominator part is to reduce the influence of multiplicative noise. Therefore, the similarity measure can solve the influence of illumination change. The mean m of the image is calculated according to Eq (1.2), and the variances is calculated according to Eq (1.3):
m=1h∗wh∑i=1w∑j=1Ix,y(i,j), | (1.2) |
s=1h∗wh∑i=1w∑j=1(Ix,y(i,j)−m)2 | (1.3) |
where h and w represent the height and width of the images respectively, and I(i, j) represents the pixel points located at (i, j) in the image. The original NCC algorithm is not sensitive to illumination changes. Figure 1 shows the matching results in the search graph with illumination changes.
Although the original method based on ZNCC has certain anti-interference ability, due to the complexity of its formula, the matching speed is far from meeting the industrial demand.
To improve the matching speed, a hierarchical matching strategy of image pyramids is used. The entire process includes preprocessing, top-level matching and non-top-level matching. Preprocessing includes the construction of the image pyramid, rotation of the template image and search of rotation invariant points and rotation invariant edge points, which can be completed offline. In top-level matching, use rotation invariant edge points to quickly filter unmatched areas, and in non-top-level matching, use two-step search to speed up.
The smaller the image size, the less time it takes to match. After building the same level pyramids for the template and search graph, matching only needs to be traversed at the top of the pyramid, and subsequent matching areas can be tracked by the top matching results. The matching process is shown in Figure 2.
Image pyramids are built from down-sampling operations. When constructing the pyramid, the down sampling methods include the nearest neighbor method, mean method and Gaussian sampling method. The results of down-sampling the image in three ways are shown in Figure 3.
When the nearest neighbor method is used for sampling, sawtooth appears at the edge of the graph. The down-sampling result of the Gaussian sampling method is fuzzy. Although it does not affect the matching result, compared with the other two sampling methods, this method takes the most time. Therefore, in practice, the 2 * 2 mean sampling method is used to down sample the image. The influence of noise pixels or pixel blocks in the original image decreases gradually with the increase of down sampling times.
When the image is rotated around the center point of the image, the pixel located at (x, y) is rotated to obtain (x', y') according to Eqs (2.1) and (2.2):
x′=(x−x0)∗cos(θ)+(y−y0)∗sin(θ)+x0 | (2.1) |
y′=−(x−x0)∗sin(θ)+(y−y0)∗cos(θ)+y0 | (2.2) |
where x0 and y0 represent the abscissa and ordinate of the center point of the template image, respectively, and θ represents the angle of rotation. The larger the values of x0 and y0 are, the larger the influence of θ on the coordinate values after rotation. Therefore, for images with different resolutions, different rotation steps are selected, and the closer to the top layer, the larger the selected step. In this paper, the top-level image step is set as 15°, and 24 template images with different angles are obtained after rotating within 360°. The rotation steps of the latter layers are 8°, 3° and 1°, respectively. After rotation, 45,120 and 360 images with different angles are obtained, respectively. A total of 549 images are obtained during the rotation process.
In the process of top-level traversal, when calculating the correlation coefficient with Eq (1.1), the final value R will be unreliable if all pixels in the rotated image participate in the calculation. Taking the search map in which the target area in the image is rotated by 45° as an example, when all pixels in the image participate in the calculation, put the calculated |R| into a matrix of Mat type, so as to observe the calculation results of correlation coefficients of each area in the search map, as shown in Figure 4.
Since the rotation step of the top-level template graph is 15° during preprocessing, the angle of the template graph is also 45 ° during the fourth traversal in the top-level matching process, and there is no angular deviation from the target area in the search graph. When traversing the search graph with the template graph of this angle, the correlation coefficient r of the best matching region should ideally be close to 1. When matching all pixels in the rotated template image, the best result is not ideal (where (14, 23) is the final best matching area in the upper left corner of the top-level image). When the score of the top-level best matching area is too low, the threshold condition may not be reached, which will cause the best matching area to be screened out during the top-level matching, making the matching fail. It is found that the reason for this result is that in the process of rotating the template image, the interpolation operation leads to the change of the pixel values of some pixels, which finally affects the score. In order to improve the score of the top-level best matching, the pixel points whose pixel values have not changed before and after the rotation of the template image are screened. When the rotation invariant points are used for matching, the matching results are shown in Figure 5.
When using rotation invariant pixel matching, the result is close to the ideal situation, and the maximum value of the calculated correlation coefficient r is close to 1. Using rotation invariant point matching not only solves the score problem of the top-level best matching area, but it also reduces the number of pixels involved in the calculation and improves the matching speed. In order to further reduce the matching time, the image shape is combined with ZNCC. At the same time, in order to ensure the accuracy of matching, it is necessary to further screen the rotation invariant edge points from the rotation invariant points.
Filter the rotation invariant points of the 549 template images obtained in the preprocessing according to Eq (2.3), and extract the edge pixels of the image. For the convenience of observation, set the pixel value of rotation invariant pixels to 255 (in order to see the intermediate results more intuitively, the pixel values of later filtered pixels are set to 255). The filtering results are shown in Figure 6.
I={0I′(x′,y′)≠I(x,y)continueotherwise | (2.3) |
where I'(x', y') is the pixel point in the rotated image, and its coordinates correspond to (x, y) in the coordinates of the original image. In the subsequent extraction of rotation invariant edge points, if the rotation invariant points and edge pixels are directly used to extract, the final number of pixels is not enough, which may lead to no discrimination in the final calculation result. In order to prevent too few rotation invariant edge points extracted later, eight pixels closest to the edge point are also extracted to form a feature pixel block. The results are shown in Figure 7.
On the basis of rotation invariant pixels, filter rotation invariant edge points according to Eq (2.4):
I={0I′(x′,y′)=0||Ib(x,y)=0continueotherwise | (2.4) |
where Ib (x, y) represents the image after filtering the feature pixel block. Compared with Figure 6, the number of pixels finally screened is further reduced, as shown in Figure 8.
When the rotation step of the top-level image is small, more template images with different angles are obtained by 0 to 360 degrees rotation, which will lead to a too slow overall matching speed. If the rotation step size is too large, the matching accuracy will decrease, and even wrong matching results may be obtained. Ideally, there is no angular deviation between the target in the search graph and the template graph. During top-level traversal, the region in the search graph gets the highest score. By selecting an appropriate threshold, the coordinates and angles of the best matching region can be transferred to the next level of matching. Assuming that the top-level rotation step is set to 8°, 45 template drawings with different angles can be obtained in the process of creating template drawings, i.e., 0°, 8°, 16°... 352°. For the search map with the target rotated by 4°, if the coordinates corresponding to the maximum value calculated from the template map rotated by 0 or 8° are close to the actual coordinates, the final accurate positioning can also be achieved. Select different top-level rotation steps. During top-level matching, the maximum coordinates and actual coordinates of the matching results with the template map with the smallest angle deviation from the search map are shown in Table 1.
Rotation step of the top- level | Rotation angle of the target | The minimum angle deviation | Matching coordinates | Actual coordinates |
8° | 4° | 4° | (26, 27) | (26, 27) |
9° | 4° | 4° | (26, 27) | (26, 27) |
10° | 5° | 5° | (26, 27) | (26, 27) |
12° | 6° | 6° | (27, 28) | (27, 28) |
15° | 7° | 7° | (28, 28) | (28, 28) |
18° | 9° | 9° | (0, 5) | (28, 30) |
20° | 10° | 10° | (0, 6) | (29, 30) |
22.5° | 11° | 11° | (29, 31) | (29, 31) |
When the minimum angle deviation between the template image and the target is larger than 7 °, the top-level matching will get wrong results. Table 2 shows the maximum score calculated from the template diagram of the minimum angle deviation and the number of other angle scores higher than the value in the top-level matching when selecting a smaller rotation step.
Rotation step of the top- level | The minimum angle deviation | The highest score | Number of other angles |
8° | 4° | 0.88 | 0 |
9° | 4° | 0.87 | 0 |
10° | 5° | 0.88 | 0 |
12° | 6° | 0.85 | 2 |
15° | 7° | 0.78 | 3 |
When the minimum angle deviation of the top layer is controlled to below 7°, the best matching area can have a higher score, and the maximum value obtained from other angle traversals is lower than this score, which is convenient for selection of the threshold. Therefore, the top-level rotation step can be set to 15 °. Subsequent rotation steps can be set to 8°, 3°, and 1°.
In top-level matching, before matching with rotation invariant points, the rotation invariant feature points are used for matching. The specific method is to set an appropriate threshold T (the correlation coefficient calculated by local pixels is often larger than that of the whole pixel, so the threshold can be set near the top-level threshold). If the conditions of threshold T are met, continue to match with rotation invariant points (at this time, the threshold used is the top-level threshold). On the contrary, this area is marked as a non-matching area, and the next area is matched directly. The top-level matching process is shown in Figure 9.
When matching at the top level, the rotation invariant edge points are used to match before matching with rotation invariant points. This is done by setting an appropriate threshold T and continuing to match using rotation invariants if the threshold T is met. Conversely, the region is marked as unmatched and matched directly to the next region. After the top-level matching, record the coordinates and angles that meet the threshold, which will be used for subsequent matching.
During down sampling, the length and width of the image are reduced by two times, and the next level coordinates tracked by the top-level matching results (i, j) are (2*i, 2*j). In order to accurately locate the target, the subsequent matching will be carried out in the neighborhood of 7 * 7 near the point. For each 7 * 7 area, the search needs to match 49 times. Because the score of the area near the best matching area is often very high, the two-step search method can be used to speed up the subsequent matching process, The two-step search method is shown in Figure 10.
In the first search, nine areas are involved in the calculation, and the point with the highest score among the nine points is selected as the central point of the second search. During the second search, the scores of eight points near the point are calculated and compared with the scores of its central point. At this time, the point with the highest score is the coordinate of the best matching area. When using the two-step search method for non-top-level matching, each 7 * 7 search area only needs to calculate the score of 9 + 8 = 17 areas, which can theoretically double the speed. Some matching results are shown in Figure 11.
In order to evaluate the accuracy and matching speed of the sub-NCC algorithm under the conditions of illumination, blur, noise and rotation, the original NCC, the improved circular projection method [18] and the improved SSDA algorithm [19] are compared with the proposed sub-NCC algorithm. The original NCC algorithm only adopts hierarchical search strategy to speed up.
The resolution of the search images tested is 640 * 480, including the classic test images of Halcon and the images randomly screened on the COCO dataset. The template image is captured in the search image, with resolutions of 208 * 123 and 200 * 200. Partial search images and templates are shown in Figure 12.
Add salt and pepper noise, Gaussian blur, rotation, illumination change and other interferences to the search map introduced in Section 4.1, and finally get 1500 test maps. The image data set is shown in Table 3.
Datasets | Type |
Dataset 1 | Source |
Dataset 2 | Salt and pepper noise, 10,000 |
Dataset 3 | Gaussian blur with Gaussian kernel size of 5 |
Dataset 4 | Rotate the image in the range of -50°–50° with 1° as the rotation step |
Dataset 5 | Global illumination variation |
The performance of the algorithm is evaluated from two aspects: the accuracy and the matching speed. The matching accuracy is obtained by dividing the number of accurately matched images by the number of all images. The matching time is the average of all image matching times. In addition to the failure to accurately match the target, if the matching time exceeds 0.2 s, the matching will also be considered as a failure. The programming language is C++ language running on a computer with the 64 bit operating system Windows 10. The machine is configured with an Intel Core i5-6300 (2.30 GHz) CPU and 8 GB of memory. The accuracy and time-consumption values of final matching are shown in Table 4.
Algorithm | Template resolution | Source | Illumination change | Gaussian blur | Salt and pepper noise | Rotation | Time(ms) |
Sub-NCC | 200*200 | 99.99% | 99.99% | 99.99% | 95.76% | 99.99% | 60 |
208*123 | 99.99% | 99.99% | 99.99% | 94.81% | 99.99% | 74 | |
Improved-CPJ | 200*200 | 99.99% | 99.99% | 91.47% | 84.31% | 99.99% | 46 |
208*123 | 99.99% | 99.99% | 86.77% | 78.46% | 99.99% | 55 | |
Improved-SSDA | 200*200 | 99.99% | 3.85% | 99.99% | 67.34% | 99.99% | 104 |
208*123 | 99.99% | 2.43% | 99.99% | 58.33% | 99.99% | 112 | |
Original NCC | 200*200 | 99.99% | 99.99% | 99.99% | 99.99% | 87.13% | 143 |
208*123 | 99.99% | 99.99% | 99.99% | 99.99% | 86.27% | 155 |
The original NCC algorithm has strong resistance to non-rotating interference, but the matching speed is too slow. The improved circular projection method has fast matching speed and is robust to illumination changes. When the image is disturbed by local noise, its matching accuracy is related to the size of the template image. The closer the length and width of the template image are, the more pixels are contained in each circular projection vector, so the higher the matching accuracy is. Based on the improved SSDA algorithm, in order to ensure the matching accuracy, the search image is processed more before matching, Especially when matching the rotated image, the top-level step size needs to be set smaller; otherwise, it will get wrong matching, resulting in a slow final matching speed. The proposed sub-NCC algorithm is stable under the interference of all kinds of noise, and the average matching speed is acceptable.
The Sub-NCC algorithm inherits the good anti-noise performance of the traditional NCC algorithm, and it solves the problem of inaccurate image matching after rotation. On the premise of ensuring the matching accuracy, the matching speed is further improved and can meet the needs of industry. This algorithm also has some shortcomings, such as not setting the adaptive threshold and the number of adaptive pyramid layers, not considering the problem of multi-scale and so on. There is more to be done for the matching speed.
This paper was supported by the University Scientific Research Project of Guangzhou Education Bureau with grant No. 202235165.
The authors declare there is no conflict of interest.
[1] |
Y. Lee, T. Hara, H. Fujita, S. Itoh, T. Ishigaki, Automated detection of pulmonary nodules in helical CT images based on an improved template-matching technique, IEEE T. Med. Imaging, 20 (2001), 595–604. https://doi.org/10.1109/42.932744 doi: 10.1109/42.932744
![]() |
[2] |
H. Li, X. Zhang, S. Yao, B. Zhu, S. Fatikow, An improved template-matching-based pose tracking method for planar nanopositioning stages using enhanced correlation coefficient, IEEE Sens. J., 12 (2020), 6378–6387. https://doi.org/10.1109/JSEN.2020.2977370 doi: 10.1109/JSEN.2020.2977370
![]() |
[3] |
D. Pandey, U. Rawat, N. K. Rathore, K. Pandey, P. K. Shukla, Distributed biomedical scheme for controlled recovery of medical encrypted images, IRBM, 43 (2022), 151–160. https://doi.org/10.1016/j.irbm.2020.07.003 doi: 10.1016/j.irbm.2020.07.003
![]() |
[4] | J. Gao, X. Li, J. Zhang, B. Lu, Image registration algorithm based on template matching, J. Xi'An Jiaotong Univ., 41 (2007), 307–311. |
[5] | Z. Y. Liu, Y. M. Jiang, Occlusion workpiece recognition method based on template matching, Appl. Res. Comput., 37 (2020), 392–394. |
[6] | T. Dekel, S. Oron, M. Rubinstein, S. Avidan, W. T. Freeman, Best-Buddies Similarity for robust template matching, in 2015 IEEE Conference on Computer Vision and Pattern Recognition (CVPR), (2015), 2021–2029. https://doi.org/10.1109/CVPR.2015.7298813 |
[7] | D. G. Lowe, Object recognition from local scale-invariant features, in Proceedings of the Seventh IEEE International Conference on Computer Vision, 2 (1999), 1150–1157. https://doi.org/10.1109/ICCV.1999.790410 |
[8] |
H. Bay, A. Ess, L. V. Gool, Speeded-Up Robust Features (SURF), Comput. Vis. Image Understand., 110 (2008), 346–359. https://doi.org/10.1016/j.cviu.2007.09.014 doi: 10.1016/j.cviu.2007.09.014
![]() |
[9] |
M. J. Atallah, Faster image template matching in the sum of the absolute value of differences measure, IEEE T. Image Process., 10 (2001), 659–659. https://doi.org/10.1109/83.913600 doi: 10.1109/83.913600
![]() |
[10] | K. Jia, S. R. Qu, Improved SSDA in image matching, Meas. Control, 10 (2012), 47–50. |
[11] | J. S. Li, Y. Y. Tan, Y. L. Zhang, Improved algorithm of SSDA, Elec. Opt. Control, 14 (2007), 66–68. |
[12] |
E. Elboher, M. Werman, Asymmetric correlation: A noise robust similarity measure for template matching, IEEE T. Image Process., 22 (2013), 3062–3073. https://doi.org/10.1109/TIP.2013.2257811 doi: 10.1109/TIP.2013.2257811
![]() |
[13] |
M. F. Tombari, ZNCC-based template matching using bounded partial correlation, Pattern Recogn. Lett., 26 (2005), 2129–2134. https://doi.org/10.1016/j.patrec.2005.03.022 doi: 10.1016/j.patrec.2005.03.022
![]() |
[14] |
D. M. Tsai, C. T. Lin, Fast normalized cross correlation for defect detection, Pattern Recogn. Lett., 24 (2003), 2625–2631. https://doi.org/10.1016/S0167-8655(03)00106-5 doi: 10.1016/S0167-8655(03)00106-5
![]() |
[15] |
S. F. Yin, Y. C. Wang, L. C. Cao, G. F. Jin, Y. S. Ling, Fast correlation matching based on fast Fourier transform and integral graph, Acta Phtonica. Sin., 39 (2010), 2246–2250. https://doi.org/10.3788/gzxb20103912.2246 doi: 10.3788/gzxb20103912.2246
![]() |
[16] | L. D. Stefano, S. Mattoccia, A sufficient condition based on the Cauchy-Schwarz inequality for efficient template matching, in Proceedings 2003 International Conference on Image Processing, 1 (2003), 269–272. |
[17] |
Y. M. Fouda, A. R. Khan, Normalize cross correlation algorithm in pattern matching based on 1-D information vector, Trends Appl. Sci. Res., 10 (2015), 195–206. https://doi.org/10.3923/tasr.2015.195.206 doi: 10.3923/tasr.2015.195.206
![]() |
[18] | T. Cao, B. Li, F. J. Ren, R. Dong, Fast circular projection image matching algorithm, J. Int. Syst., 15 (2020), 84–91. |
[19] | J. B. Zheng, L. X. Zheng, J. Q. Zhu, A fast template matching method based on gray scale, Mod. Comput., 626 (2018), 54–58. |
1. | Wuxin Li, Qian Chen, Guohua Gu, Xiubao Sui, Object matching of visible–infrared image based on attention mechanism and feature fusion, 2025, 158, 00313203, 110972, 10.1016/j.patcog.2024.110972 | |
2. | Hui Zhang, Zhen Guan, Joe Eastwood, Hongji Zhang, Xiaoyang Zhu, Effective Mean Square Differences: A Matching Algorithm for Highly Similar Sheet Metal Parts, 2023, 23, 1424-8220, 7300, 10.3390/s23167300 | |
3. | Zhijian Wen, J.M. Curran, G. Wevers, Shoeprint image retrieval and crime scene shoeprint image linking by using convolutional neural network and normalized cross correlation, 2023, 63, 13550306, 439, 10.1016/j.scijus.2023.04.014 | |
4. | Ao Guo, Huicheng Jing, Chao Ge, Zhongpan Qiu, Lijun Wu, 2024, Design and implementation of robot depalletizing system based on 3D vision, 9781510678682, 37, 10.1117/12.3029145 | |
5. | Bao Zhang, Zhipeng Yang, Tao Yang, 2024, Recognition Detection Method Based on Multi-Scale Feature Template Matching, 979-8-3315-2830-0, 27, 10.1109/ICFEEIE64494.2024.00012 | |
6. | Haoyu Wu, Jiwen Zhao, Ping Ge, Zhenbao Pan, Zixiang Yu, Linear Motor Mover Position Measurement Based on the Matching of Captured and Sample Images, 2025, 21, 1551-3203, 1980, 10.1109/TII.2024.3495790 |
Rotation step of the top- level | Rotation angle of the target | The minimum angle deviation | Matching coordinates | Actual coordinates |
8° | 4° | 4° | (26, 27) | (26, 27) |
9° | 4° | 4° | (26, 27) | (26, 27) |
10° | 5° | 5° | (26, 27) | (26, 27) |
12° | 6° | 6° | (27, 28) | (27, 28) |
15° | 7° | 7° | (28, 28) | (28, 28) |
18° | 9° | 9° | (0, 5) | (28, 30) |
20° | 10° | 10° | (0, 6) | (29, 30) |
22.5° | 11° | 11° | (29, 31) | (29, 31) |
Rotation step of the top- level | The minimum angle deviation | The highest score | Number of other angles |
8° | 4° | 0.88 | 0 |
9° | 4° | 0.87 | 0 |
10° | 5° | 0.88 | 0 |
12° | 6° | 0.85 | 2 |
15° | 7° | 0.78 | 3 |
Datasets | Type |
Dataset 1 | Source |
Dataset 2 | Salt and pepper noise, 10,000 |
Dataset 3 | Gaussian blur with Gaussian kernel size of 5 |
Dataset 4 | Rotate the image in the range of -50°–50° with 1° as the rotation step |
Dataset 5 | Global illumination variation |
Algorithm | Template resolution | Source | Illumination change | Gaussian blur | Salt and pepper noise | Rotation | Time(ms) |
Sub-NCC | 200*200 | 99.99% | 99.99% | 99.99% | 95.76% | 99.99% | 60 |
208*123 | 99.99% | 99.99% | 99.99% | 94.81% | 99.99% | 74 | |
Improved-CPJ | 200*200 | 99.99% | 99.99% | 91.47% | 84.31% | 99.99% | 46 |
208*123 | 99.99% | 99.99% | 86.77% | 78.46% | 99.99% | 55 | |
Improved-SSDA | 200*200 | 99.99% | 3.85% | 99.99% | 67.34% | 99.99% | 104 |
208*123 | 99.99% | 2.43% | 99.99% | 58.33% | 99.99% | 112 | |
Original NCC | 200*200 | 99.99% | 99.99% | 99.99% | 99.99% | 87.13% | 143 |
208*123 | 99.99% | 99.99% | 99.99% | 99.99% | 86.27% | 155 |
Rotation step of the top- level | Rotation angle of the target | The minimum angle deviation | Matching coordinates | Actual coordinates |
8° | 4° | 4° | (26, 27) | (26, 27) |
9° | 4° | 4° | (26, 27) | (26, 27) |
10° | 5° | 5° | (26, 27) | (26, 27) |
12° | 6° | 6° | (27, 28) | (27, 28) |
15° | 7° | 7° | (28, 28) | (28, 28) |
18° | 9° | 9° | (0, 5) | (28, 30) |
20° | 10° | 10° | (0, 6) | (29, 30) |
22.5° | 11° | 11° | (29, 31) | (29, 31) |
Rotation step of the top- level | The minimum angle deviation | The highest score | Number of other angles |
8° | 4° | 0.88 | 0 |
9° | 4° | 0.87 | 0 |
10° | 5° | 0.88 | 0 |
12° | 6° | 0.85 | 2 |
15° | 7° | 0.78 | 3 |
Datasets | Type |
Dataset 1 | Source |
Dataset 2 | Salt and pepper noise, 10,000 |
Dataset 3 | Gaussian blur with Gaussian kernel size of 5 |
Dataset 4 | Rotate the image in the range of -50°–50° with 1° as the rotation step |
Dataset 5 | Global illumination variation |
Algorithm | Template resolution | Source | Illumination change | Gaussian blur | Salt and pepper noise | Rotation | Time(ms) |
Sub-NCC | 200*200 | 99.99% | 99.99% | 99.99% | 95.76% | 99.99% | 60 |
208*123 | 99.99% | 99.99% | 99.99% | 94.81% | 99.99% | 74 | |
Improved-CPJ | 200*200 | 99.99% | 99.99% | 91.47% | 84.31% | 99.99% | 46 |
208*123 | 99.99% | 99.99% | 86.77% | 78.46% | 99.99% | 55 | |
Improved-SSDA | 200*200 | 99.99% | 3.85% | 99.99% | 67.34% | 99.99% | 104 |
208*123 | 99.99% | 2.43% | 99.99% | 58.33% | 99.99% | 112 | |
Original NCC | 200*200 | 99.99% | 99.99% | 99.99% | 99.99% | 87.13% | 143 |
208*123 | 99.99% | 99.99% | 99.99% | 99.99% | 86.27% | 155 |