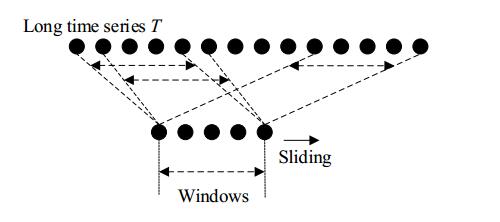
Citation: Haibo Li, Juncheng Tong. A novel clustering algorithm for time-series data based on precise correlation coefficient matching in the IoT[J]. Mathematical Biosciences and Engineering, 2019, 16(6): 6654-6671. doi: 10.3934/mbe.2019331
[1] | Yifan Zhang, Zhi Zhang, Shaohu Peng, Dongyuan Li, Hongxin Xiao, Chao Tang, Runqing Miao, Lingxi Peng . A rotation invariant template matching algorithm based on Sub-NCC. Mathematical Biosciences and Engineering, 2022, 19(9): 9505-9519. doi: 10.3934/mbe.2022442 |
[2] | Tian Ma, Boyang Meng, Jiayi Yang, Nana Gou, Weilu Shi . A half jaw panoramic stitching method of intraoral endoscopy images based on dental arch arrangement. Mathematical Biosciences and Engineering, 2024, 21(1): 494-522. doi: 10.3934/mbe.2024022 |
[3] | Jian Zhang, Yan Zhang, Cong Wang, Huilong Yu, Cui Qin . Binocular stereo matching algorithm based on MST cost aggregation. Mathematical Biosciences and Engineering, 2021, 18(4): 3215-3226. doi: 10.3934/mbe.2021160 |
[4] | Qun Song, Tengyue Li, Simon Fong, Feng Wu . An ECG data sampling method for home-use IoT ECG monitor system optimization based on brick-up metaheuristic algorithm. Mathematical Biosciences and Engineering, 2021, 18(6): 9076-9093. doi: 10.3934/mbe.2021447 |
[5] | J. Fernando Vera-Vera, J. Antonio Roldán-Nofuentes . A robust alternating least squares K-means clustering approach for times series using dynamic time warping dissimilarities. Mathematical Biosciences and Engineering, 2024, 21(3): 3631-3651. doi: 10.3934/mbe.2024160 |
[6] | Jun Gao, Fei Wu, Yakufu Yasen, Wanqing Song, Lijia Ren . Generalized Cauchy process based on heavy-tailed distribution and grey relational analysis for reliability predicting of distribution systems. Mathematical Biosciences and Engineering, 2022, 19(7): 6620-6637. doi: 10.3934/mbe.2022311 |
[7] | Hao Yuan, Qiang Chen, Hongbing Li, Die Zeng, Tianwen Wu, Yuning Wang, Wei Zhang . Improved beluga whale optimization algorithm based cluster routing in wireless sensor networks. Mathematical Biosciences and Engineering, 2024, 21(3): 4587-4625. doi: 10.3934/mbe.2024202 |
[8] | Jiushuang Wang, Ying Liu, Huifen Feng . IFACNN: efficient DDoS attack detection based on improved firefly algorithm to optimize convolutional neural networks. Mathematical Biosciences and Engineering, 2022, 19(2): 1280-1303. doi: 10.3934/mbe.2022059 |
[9] | Kenneth Li-minn Ang, Kah Phooi Seng . Biometrics-based Internet of Things and Big data design framework. Mathematical Biosciences and Engineering, 2021, 18(4): 4461-4476. doi: 10.3934/mbe.2021226 |
[10] | Wenzheng Wei, Ying Jin . A novel Internet of Things-supported intelligent education management system implemented via collaboration of knowledge and data. Mathematical Biosciences and Engineering, 2023, 20(7): 13457-13473. doi: 10.3934/mbe.2023600 |
In recent years, the development of smart environments promises a revolution for most kinds of human-related activities. The smart environment is a physical world that is richly and invisibly interwoven with sensors, actuators, displays, and computational elements, embedded seamlessly in the everyday objects of our lives, and connected through a continuous network [1]. Nowadays, the practical realization of smart environments has only become possible due to the fast-developing of the computer technology such as Internet of Things (IoT) [2,3] and Cloud Computing(CC) [4,5]. Particularly, benefits of the IoT and its relevant technologies can seamlessly integrate classical networks with networked instruments and devices [6]. The IoT is therefore the most fundamental enabler of smart environments, such as smart homes, smart buildings, smart cities and smart factories, among others [7].
The Internet of Things can be realized in three aspects: Internet-oriented (middleware), things-oriented (sensors) and semantic-oriented (knowledge) [8]. Among them, sensors are the basic equipment in the IoT, and they are used increasingly more. Data mining is the key technology for acquiring knowledge from the data that are collected by sensors, and it involves discovering novel, interesting, and potentially useful patterns and applying algorithms to the extraction of hidden information [9]. In the IoT, a large amount of data that are collected by the bottom sampling equipment is in the form of time series—which is a collection of values that are obtained from sequential measurements over time. Therefore, time series analysis is a hot research area in data mining in the IoT.
In the IoT, the correlation of time series can reflect the relationships of different objects, such as the collaborative relationship between manufacturing equipment, the similarity of geographic information between different environmental monitoring sites, and the correlation of electricity consumption between different buildings. In the IoT, studying the problem of time series correlation mining between different objects is of great significance for equipment anomaly detection, attribute value prediction, resource optimization, etc. For example, the correlation information of the daily electricity time series between different buildings from an area can provide a basis for optimizing the electricity price setting and power facility configuration [10]. The existing body of research on the correlation of time series suggests that correlation subsequences can be obtained based on similarity measurements. However, some methods fail to obtain clusters of related subsequences, such as the methods in [11,12]. In addition, the existing methods based on clustering algorithms may obtain inaccurate results and long computation times when mining the time series correlations from different objects, analyzing the correlation in the same time series, such as the method in [13]. Consequently, an effective clustering method is needed to analyze the correlation between different time series, which is more helpful for discovering the hidden behavioral relations between different objects in smart environments based on the IoT.
In this paper, a novel clustering algorithm named the CPCCM (clustering algorithm for time-series data based on precise correlation coefficient matching in the IoT) is presented. In this method, only the correlation between subsequences from different initial sequences should be computed in the density clustering process of subsequences while adjacent subsequences in the same cluster should be connected. Through the method that is proposed by this paper, clusters of high correlation subsequences from the time series of different objects in the IoT will be obtained, which will provide a basis for mining the hidden behavioral relations between objects.
The contributions of the proposed in this paper are as follows.
(1) To solve the problem of behavior analysis between different objects in smart environments, based on the IoT, a novel clustering algorithm for time-series data based on precise correlation coefficient matching is proposed. The proposed algorithm improves the cluster quality by matching the correlation coefficients between any pair of subsequence sets from two different time series.
(2) The proposed algorithm is independent of specific development tools and platforms, so it can be flexibly applied to similar scenarios.
The remainder of the article is organized as follows. In Section Ⅱ, a survey of the related work is presented, and the relative pros and cons of different related approaches are discussed. In Section Ⅲ, by analyzing the time series and its correlation coefficient, a mathematical description of the problem is presented. In Section Ⅳ, density clustering modeling based on precise correlation coefficient matching in the IoT is introduced. The evaluation criteria, the design of the experiments and the results are presented in Section Ⅴ. Finally, in Section Ⅵ, the work of this paper is summarized.
The fast-developing and expanding area known as the Internet of Things (IoT) involves expanding the Internet beyond the connection of various physical devices and objects [14]. The existing research topics are wide, including security and privacy issues in the IoT [15,16,17], the captured, stored, and managed big data in the IoT [18,19,20], the efficient digital media transmission [21,22], efficient energy and resource management [23,24,25], etc. To make the IoT smarter, lots of analysis technologies have been introduced into the IoT, and one of the most valuable technologies is data mining [9].
In the smart environments based on the IoT, the data that are collected by IoT devices are in the form of time series [26]. Therefore, time series analysis is an important task in IoT data mining. Time series analysis in the IoT has been applied to smart cities [22,27], environmental monitoring [11,12,13,28,29,30], industry and manufacturing [31,32,33,34], smart grids [35,36,37,38,39,40,41] and other research fields. In [27], to handle the large amount of heterogeneous data and sensory information in smart cities, a time series agammaegation method is proposed to agammaegate and represent the original data in an efficient and higher-granularity form. In environmental monitoring, the sea surface temperature (SST) time series [28] and air quality index time series [12,13] that are collected by IoT sensors are used for anomaly detection and prediction analysis. In [31,32,33], the methods based on the time series of resource services and their feature sequences are proposed to analyze the service selection and composition of cloud manufacturing in collaborative tasks. With respect to smart grids, in [36,37], time series of wind speeds and power outputs with respect to wind power generation are studied and analyzed. In addition, in [39,40,41], time series of power prices and loads are studied and analyzed.
Correlation analysis is an important part of time series analysis [42]. In recent years, increasingly more scholars have paid attention to this research direction. In [11], a method based on the traditional geophysical time series prediction model is proposed, which calculates the spatial correlation across several sites and the time correlation within each site to improve the accuracy of dynamic time series prediction. In [12,35], the proposed approaches studied the lag correlation between time series. In [37], mutual information was proposed to quantitatively evaluate the correlation and predictability of wind speed series and to improve the forecast accuracy. In [39], the proposed method developed a novel cross-correlation coefficient to explore the existence of asymmetric cross-correlation between two time series. In [43], a method based on the ARIMA model is proposed, which calculates the correlation of multivariate QoS time series to improve the quality of the QoS prediction in the service composition problem. In these methods, the time-series models can be improved by incorporating various correlation coefficients into similarity measurements. However, most of them focus only on resolving the correlation between two single sequences rather than two sets of sequences. Consequently, the correlation cannot be completely mined. To overcome the shortcoming, a method based on hierarchical clustering is proposed to analyze the time series that are formed by air quality monitoring, which uses the Pearson correlation coefficient and Euclidean distance as the distance metric for time series data [13]. In [41], a method based on the weighed Pearson distance is proposed to divide the huge load curve time series into several clusters since the load curves of the same cluster have similar power consumption patterns. In [44], a method based on the Copula clustering model is proposed, which allows one to extend the usual clustering methods for time series based on Pearson's correlation coefficient. All the clustering algorithms can find meaningful subsequence pattern. However, there are redundant scanning in their algorithms to reduce the performance of the algorithms.
As described above, the current works on time series correlation analysis are mostly based on time series similarity measures. However, some of these methods cannot obtain correlation clusters from different time series subsequences, and some pay attention to the redundant correlation information when using clustering algorithms.
A sequence is often the result of the observation of an underlying process in the IoT. In this process, according to a given sampling rate, data are collected by sensors at uniformly spaced time moments. Thus, a sequence can be defined as a set of contiguous time moments.
Definition 1 Initial sequence. An initial sequence T is an ordered sequence of n real-valued variables, which can be expressed as T = (t1, t2, …, tn), where ti∈R. The initial sequence can cover all the observation data that are obtained and can be quite long. Therefore, it is meaningful to consider the subsequences of a sequence.
Definition 2 Subsequence. The subsequence S of the initial sequence T = (t1, t2, …, tn) can be defined as S(T)k = (tk, tk+1, …, tk+m-1), where 1 ≤ k ≤ n -m +1.The set of all subsequences of length m from T can be denoted as SmT.
Definition 3 Adjacent subsequences. For subsequences S(T)i = (ti, ti+1, …, ti+m-1) and S(T)j = (tj, tj+1, …, tj+m-1) from the same sequence T = (t1, t2, …, tn), if j-I = 1, the subsequence S(T)j and S(T)i are adjacent subsequences, and S(T)j is S(T)i subsequent subsequence, which is denoted as S(T)i→S(T)j.
Definition 4 Correlation ρ-neighborhood. For the subsequence S(X)i from the sequence X, the correlation ρ-neighborhood is a subsequence set whose correlation coefficient with S(X)i is greater than ρ, and these subsequences do not from the sequence X. That is, Nρ(S(X)i)={Sj∈SD−∪1≤l≤|X|SlX|r(Si,Sj)≥ρ}, if a negative correlation is considered, then, N−ρ(S(X)i)={Sj∈SD−∪1≤l≤|X|SlX|r(Si,Sj)≤ρ}, where SD is a subsequence data set, r(Si, Sj) is a function for calculating the correlation between the subsequence Si and Sj, and |X| is the length of the sequence X.
Definition 5 Subsequence correlation clustering. For a given multiple initial sequence and correlation function r(Si, Sj), find the set of clusters C = {ci} where ci = { Sk | Sk∈SD } is a set of subsequences from different initial sequences within the same cluster that are more correlated than the subsequences of different clusters.
The problem that we want to solve in this paper is to obtain the correlation clusters of subsequences C. By our method, the quality of the clusters can be improved.
For the initial sequence data T = (t1, t2, …, tn), which are collected from the sensors of the sampling site, first, the sliding window is adopted. Using a given time window length l, one unit length is sequentially slid from the first recording point until all points of the initial sequence are traversed, as shown in Figure 1.
The subsequence of the initial sequence T that is split by the sliding window is S(T)i = (ti, ti+1, …, ti+l-1), where i = 1, 2, …, n - l + 1. There are n - l + 1 subsequences in total, and the set of these subsequences is SlT.
For two given initial sequences X and Y and a time window length l, after the subsequence sets SlX and SlY are obtained by dividing the subsequences using the sliding window described above, the correlation coefficient between subsequences S(X)i and S(Y)j is calculated by the correlation function r(S(X)i, S(Y)j), where 1 ≤ I ≤ n - l + 1 and 1 ≤ j ≤ n - l + 1. In this paper, we use Pearson's correlation coefficient as the measure of the correlation between subsequences. Therefore, r(S(X)i, S(Y)j) can be calculated by Eq 1.
r(S(X)i,S(Y)j)=Cov(S(X)i,S(Yj)σS(X)iσS(Y)j | (1) |
where Cov(S(X)i, S(Y)j) is the covariance of subsequences S(X)i and S(Y)j, and σ is their standard deviation. Pearson's correlation coefficient is a popular method to investigate the cross-correlation between sequences because it can be computed with just a linear scan and it is scale and offset invariant. Pearson's correlation coefficient reflects the trend of the sequence with respect to the similarity. Pearson's correlation coefficient's range is [-1, 1]. When the two subsequences are positively correlated, the Pearson correlation coefficient will be positive, and negatively correlated subsequences have a negative Pearson correlation coefficient. Furthermore, the larger that the absolute value of Pearson's correlation coefficient is, the greater the correlation between the subsequences.
For the subsequence sets that are obtained by the above method and the correlation coefficient calculation function given by Eq 1, clustering is performed using a method that precisely matches the correlation coefficients between subsequences from different initial sequences. And adjacent subsequences are combined during the clustering process to obtain longer subsequences.
When calculating the correlation coefficient of the merged subsequences, the length of the subsequences may be unequal. At this time, the shorter sequence can be used as a sliding window for correlation matching, and the correlation coefficient between them can be calculated by Eq 2.
r′(Su,Sv)=∑|Sv|−|Su|+1i=1r(Sv,Sui)|Sv|−|Su|+1 | (2) |
where Su and Sv are two subsequences from different initial sequence and their lengths are not equal. We assume that |Sv| > |Su|, that is, the length of the subsequence Sv is greater than Su. Then, the subsequence Sv is split into the subsequence Sv, where i = 1, 2, …, |Sv| - |Su| + 1, with a window length |Su|.
The strategy of precise matching is to match any pair of subsequences from different initial sequences completely with the giving threshold during the clustering process. The main idea of the CPCCM is that in each round of precise matching, the efficient clustering of all subsequences is accomplished using a cross-traversal strategy. For the subsequence S(X)i, first by traversing, the subsequences in the ε-neighborhood are clustered into positive correlation clusters and the subsequences in the-ε-neighborhood are clustered into negative correlation clusters. Then, by traversing, the intersections of the ε-neighborhoods of the subsequences in the positive correlation cluster are found, and we merge them into the positive correlation cluster. In addition, the intersections of the-ε-neighborhoods of the subsequences in the negative correlation cluster are found, and we merge them into the negative correlation cluster.
Take Figure 2 as an example. One cross-traversal round starting with S(X)1 is as follows. Find the subsequences in the ε-neighborhood of S(X)1, which are S(Y)3 and S(Y)6, and classify them into the positive correlation cluster of S(X)1. Then, find the subsequences in the-ε-neighborhood of S(X)1, which are S(Y)2, S(Y)4, and S(Y)5, and classify them into the negative correlation cluster of S(X)1. S(Y)4 and S(Y)5 are adjacent subsequences, and so they are combined. Then, find the intersection of the ε-neighborhoods of S(Y)3 and S(Y)6, which is S(X)6, and classify it into the positive correlation cluster of S(X)1. Next, find the intersections of the-ε-neighborhoods of S(Y)2 and S(Y)4, which are S(X)3 and S(X)5, and classify them into the negative correlation cluster of S(X)1. At this point, the positive correlation cluster in this round is { S(X)1, S(Y)3, S(Y)6, S(X)6}, and the negative correlation cluster is { S(X)1, S(Y)2, S(Y)4, S(X)3, S(X)5}.
For a given correlation threshold ε and subsequence sets SlX and SlY, the specific steps of the CPCCM algorithm's iterative execution are as follows.
Step 1: Initialize the final cluster sets C+=∅ and C−=∅.
Step 2: Extract a subsequence S(X)i from subsequence set SlX. By traversing SlY, we get the ε-neighborhood of S(X)i as Nε(S(X)i) and the -ε-neighborhood of S(X)i as N−ε(S(X)i) through Eq 1, Eq 2 and Definitions 4. Then, Nε(S(X)i) is clustered into positive correlation clusterc+k; N−ε(S(X)i) is clustered into the negative correlation cluster N−ε(S(X)i), and the adjacent subsequences are merged in the clustering process.
Step 3: Delete subsequence S(X)i from set SlX.
Step 4: By traversing SlX, for each subsequence of Nε(S(X)i), get their ε-neighborhoods using Eq 1, Eq 2 and Definitions 4, find their intersection, and add the intersection to the positive correlation cluster c+k. Next, for each subsequence of N−ε(S(X)i), get their -ε-neighborhoods using Eq 1, Eq 2 and Definitions 4, find their intersection, and add the intersection to the negative correlation cluster c−s.
Step 5: Positive correlation cluster c+k is merged into C+, and negative correlation cluster c−s is merged into C−.
Step 6: For each subsequence Sj in positive correlation cluster c+k or negative correlation cluster c−s, it is judged whether it is contained in both set C+ and set C−. If so, subsequence Sj is deleted from SlX or SlY.
Step 7: Go back to step 2 and repeat while SlX or SlY is null.
The pseudocode of CPCCM is shown in Algorithm 1.
Algorithm 1. |
Input: The two initial sequences and the correlation threshold ε(ε>0); |
Output: The positive correlation cluster set C+={c+1,…,c+m}, and the negative correlation cluster set C−={c−1,…,c−n} |
1: C+=∅,C−=∅ |
2: while (SlX≠∅) |
3: while (SlY≠∅)V // if the set SlY is not null, traversing SlY |
4: if (S(Y)j∈Nε(S(X)i)) then //if S(X)i is positively correlated with S(Y)j |
5: ck+←ck+∪{ S(X)i} |
6: ck+←ck+∪{S(Y)j} // subsequences are clustered into positive correlation cluster ck+ |
7: End if |
8: Else if (S(Y)j∈N-ε (S(X)i)) then //else if S(X)iis negatively correlated with S(Y)j |
9: cs-←cs-∪{ S(X)i} |
10: cs-←cs-∪{S(Y)j} // subsequences are clustered into negative correlation cluster cs- |
11: End else if |
12: if ({S(Y)j}⊆C+ and {S(Y)j}⊆C−) then //if the subsequence S(Y)j has been in positive correlation cluster and negative correlation cluster |
13: SlY←SlY−{S(Y)j} // S(Y)j is removed from the set SYl |
14: End if |
15: End while |
16: SlX←SlX−{S(X)i} //S(X)i is removed from the set SXl |
17: while (SlX≠∅) |
18: if (S(X)p∈Nε(c+k)) then // if S(X)p is positively correlated with any sequence of ck+ |
19: ck+←ck+∪{ xp} |
20: ck+←JoinOn(ck+) // Merge two adjacent subsequences which from the same initial sequence into a new long subsequence |
21: End if |
22: Else if (S(X)p∈N−ε(c+k)) then// if S(X)p is negatively correlated with any subsequence of cs- |
23: cs-←cs-∪{S(X)p}, |
24: cs-←JoinOn(Rs-) |
25: End else if |
26: if ({S(X)p}⊆C+ and {S(X)p}⊆C−) then SlX←SlX−{S(X)p} // if subsequence S(X)p is in positive correlation cluster and negative correlation cluster, S(X)p is removed from the set SXl |
27: End while |
28: C+←C+∪ck+, C-←C-∪cs-, k++, s++ |
29: End while |
30: return C+, C- |
If n is the number of records in the sequence and l is the given time window length, the time complexity of the algorithm is O(n-l) in the best case and the O((n-l)2) in the worst case. In the best case, the first subsequence from one initial sequence is positively or negatively correlated with all the adjacent subsequences from the other initial sequence. Due to merging all the adjacent subsequences after the first traversal, only n-l matches are needed. So the time complexity is O(n-l). In the worst case, adjacent subsequences cannot be merged during the clustering process, so that the time complexity is O((n-l)2).
The data set of the experiment is collected from a college in China. To achieve energy savings and emission reductions, in this college, more than 2, 000 sensors were installed on the electricity meters in more than 20 buildings. The data is collected at 30 min intervals from March 1, 2016 to June 27, 2016. From the entire electricity consumption dataset, we choose one sensor, and there are 5680 electricity consumption data points for this sensor. The data format is shown in Table 1.
time(Y-M-D H:M:S) | digits of electricity meter |
2016-03-01 11:10:07.0 | 157212.0 |
2016-03-01 11:40:08.0 | 157218.0 |
2016-03-01 12:10:09.0 | 157225.2 |
2016-03-01 12:40:10.0 | 157234.8 |
2016-03-01 13:10:11.0 | 157240.2 |
2016-03-01 13:40:12.0 | 157244.4 |
To analyze the correlation of the electricity consumption between different buildings, all the data should be preprocessed and converted to electricity consumption sequences. In Table 1, the times represent the ordered relationship among the digits of the electricity meter, and so the electricity consumption sequence can be represented by the difference in the sequence of the digits of the electricity meter. After preprocessing the data in Table 1, the electricity consumption sequence should be (6, 7.2, 9.6, 5.4, 4.2).
The simulation experiment has two steps: 1) splitting subsequence by sliding window; and 2) running CPCCM algorithm and verifying the validity of the clustering results.
Step 1) Splitting subsequence by sliding window.
In this paper, the time window length is set to 24. The electricity consumption sequence is split into 5557 subsequences by the sliding window method described in Sec 4.1. Only the first five subsequences are displayed, as shown in Table 2.
subsequence number | subsequences from initial sequence X |
1 | (6, 7.2, 9.6, 5.4, 4.2, 4.2, 4.8, 7.8, 7.8, 7.8, 9, 11.4, 11.4, 13.8, 14.4, 15.6, 16.2, 17.4, 13.2, 14.4, 14.4, 13.8, 12, 4.2) |
2 | (7.2, 9.6, 5.4, 4.2, 4.2, 4.8, 7.8, 7.8, 7.8, 9, 11.4, 11.4, 13.8, 14.4, 15.6, 16.2, 17.4, 13.2, 14.4, 14.4, 13.8, 12, 4.2, 2.4) |
3 | (9.6, 5.4, 4.2, 4.2, 4.8, 7.8, 7.8, 7.8, 9, 11.4, 11.4, 13.8, 14.4, 15.6, 16.2, 17.4, 13.2, 14.4, 14.4, 13.8, 12, 4.2, 2.4, 1.8) |
4 | (5.4, 4.2, 4.2, 4.8, 7.8, 7.8, 7.8, 9, 11.4, 11.4, 13.8, 14.4, 15.6, 16.2, 17.4, 13.2, 14.4, 14.4, 13.8, 12, 4.2, 2.4, 1.8, 1.2) |
5 | (4.2, 4.2, 4.8, 7.8, 7.8, 7.8, 9, 11.4, 11.4, 13.8, 14.4, 15.6, 16.2, 17.4, 13.2, 14.4, 14.4, 13.8, 12, 4.2, 2.4, 1.8, 1.2, 1.2) |
Step 2) Running CPCCM algorithm and verifying the validity of the clustering results.
Using the two subsequence sets from different buildings, the CPCCM-based experiment was done three times. We set the time window to 24, and set different correlation thresholds to 0.75, 0.8, and 0.9, respectively. To analyze the frequent correlation between subsequences, we use the positive correlation cluster and the negative correlation cluster in each clustering result to select the ten clusters with the largest number of elements in each cluster as the final result.
To verify the validity of the clustering results, the correlation coefficient of the intra-cluster subsequences (corrintra) and the correlation coefficient of the inter-cluster subsequences (corrinter) are calculated for each of the major clusters. corrintra is the average of the correlation coefficients between the subsequences in a cluster, and corrinter is the average of the correlation coefficients between the cluster subsequence and other cluster subsequences. For cluster c, its corrintra and corrinter can be calculated by equations 3 and 4, respectively.
corrintra(c)=∑ncXi=1∑ncYj=1r(s(X)cis(Ycj)ncX×ncY | (3) |
where ncX is the number of subsequences from sequence X in cluster c, and S(X)ci is the i-th subsequence from sequence X in cluster c.
corrinter(c)=1m−1∑c′∈C−c∑ncXi=1∑n′cYj=1r(s(X)ci,S(Y)c′j)+∑nc′Xi=1∑ncYj=1r(s(X)c′i′,S(Y)cj)ncX×nc′Y+nc′X×ncY | (4) |
where c′ is a cluster that is different from cluster c in the final clustering result set, and m is the number of clusters in the final clustering result.
The positive correlation cluster and the negative correlation cluster are calculated separately, and the results(to 4 decimal places) are shown in Tables 3 and 4.
Top 10 largest clusters | positive correlation with ε = 0.75 | positive correlation with ε = 0.8 | positive correlation with ε = 0.9 | |||
corrintra | corrinter | corrintra | corrinter | corrintra | corrinter | |
c1 | 0.8017 | 0.1850 | 0.8347 | 0.2362 | 0.9123 | 0.2895 |
c2 | 0.7886 | 0.1568 | 0.8217 | 0.1995 | 0.9095 | 0.2505 |
c3 | 0.7924 | 0.1876 | 0.8254 | 0.2324 | 0.9102 | 0.3088 |
c4 | 0.7760 | 0.1390 | 0.8315 | 0.2608 | 0.9134 | 0.2628 |
c5 | 0.7853 | 0.1585 | 0.8207 | 0.1977 | 0.9090 | 0.2294 |
c6 | 0.7729 | 0.1820 | 0.8259 | 0.1483 | 0.9123 | 0.3049 |
c7 | 0.7825 | 0.1697 | 0.8218 | 0.2168 | 0.9163 | 0.3127 |
c8 | 0.7737 | 0.1117 | 0.8325 | 0.1885 | 0.9150 | 0.1978 |
c9 | 0.7796 | 0.1665 | 0.8472 | 0.1400 | 0.9138 | 0.3006 |
c10 | 0.7749 | 0.1614 | 0.8159 | 0.1393 | 0.9161 | 0.1764 |
Top 10 largest clusters | negative correlation with ε = 0.75 | negative correlation with ε = 0.8 | negative correlation with ε = 0.9 | |||
corrintra | corrinter | corrintra | corrinter | corrintra | corrinter | |
c1 | -0.7913 | -0.2474 | -0.8227 | -0.2161 | -0.9130 | -0.3112 |
c2 | -0.7846 | -0.1923 | -0.8334 | -0.3034 | -0.9108 | -0.3785 |
c3 | -0.7840 | -0.2498 | -0.8224 | -0.2446 | -0.9150 | -0.2679 |
c4 | -0.7941 | -0.2165 | -0.8289 | -0.2778 | -0.9088 | -0.4079 |
c5 | -0.7746 | -0.1162 | -0.8197 | -0.2664 | -0.9141 | -0.2806 |
c6 | -0.7754 | -0.2317 | -0.8240 | -0.1630 | -0.9151 | -0.2674 |
c7 | -0.7772 | -0.2362 | -0.8164 | -0.2917 | -0.9184 | -0.2492 |
c8 | -0.7815 | -0.2217 | -0.8208 | -0.2214 | -0.9115 | -0.3874 |
c9 | -0.7806 | -0.1533 | -0.8289 | -0.1634 | -0.9144 | -0.3806 |
c10 | -0.7672 | -0.2442 | -0.8314 | -0.2099 | -0.9092 | -0.2487 |
As shown in Tables 3 and 4, for each run's result, the number of elements is ranked in the top ten clusters, and each cluster has | corrintra | > |ε| and | corrinter | < |ε|. This indicates that the clustering results have high correlation between the subsequences within the cluster, and the correlation between the subsequences of different clusters is low, thus indicating that the clustering algorithm is effective. In addition, the electricity consumption-time sequence diagrams of the clusters with the most subsequences under each threshold operation result in Tables 3 and 4 are drawn, as shown in Figure 3. 3a, 3b and 3c are the positive correlation clusters, and 3d, 3e, and 3f are the negative correlation clusters. 3a and 3d are the results with the threshold of ε = 0.75, 3b and 3e are the results with the threshold of ε = 0.8, and 3c and 3f are the results with the threshold of ε = 0.9.
From Figure 3, we can find that the CPCCM algorithm can classify subsequences with similar electricity consumption into one class, and the trend of the correlation relationship that is presented in the clustering results is more practical-even if some subsequences do not overlap on the coordinate axes but they have similar (e.g., 3a-c) or opposite (e.g., 3d-f) trends. Moreover, in Figure 3, the lower the threshold is, the greater the number of subsequences, and the longer the length of the merged subsequences. Conversely, the higher the threshold is, the less the number of subsequences, and the trend between the subsequences from the different initial sequences is more similar (such as 3c) or the opposite (such as 3f), which means that the positive or negative correlation between subsequences is more obvious.
The agglomerative hierarchical clustering (AHC) algorithm is a popular solution for subsequence correlation clustering. For example, in [13], the temporal sequence correlation of air quality testing sites using AHC algorithm is analyzed to evaluate the similarity of these testing sites. And in [41], several user consumption models is found for load curve time series data using the AHC algorithm based on Pearson similarity.
AHC is a bottom-up clustering method. The main steps can be demonstrated as follows: 1) assign N subsequences to N clusters where each cluster only contains one subsequence; 2) find the closest pair of clusters and merge them into a cluster; 3) compute distances between the clusters; and 4) repeat step 2) and step 3) until all items are clustered into a cluster or reach the specified termination condition.
The comparison is designed as follows. First, using the same data set, the AHC-based experiment was done three times. We set the time window as 24, and set different correlation thresholds of 0.75, 0.8, and 0.9, respectively. The electricity consumption-time diagrams of the clusters with the most elements under each threshold based on the subsequences are shown in Figure 4. 4a, 4b and 4c are the positive correlation clusters, and 4d, 4e, and 4f are the negative correlation clusters. 4a and 4d are the results with the threshold of ε = 0.75, 4b and 4e are the results with the threshold of ε = 0.8, and 4c and 4f are the results with the threshold of ε = 0.9.
Comparing the running results of the hierarchical clustering algorithm in Figure 4 and the CPCCM running results in Figure 3, we can intuitively find that under the same threshold, the CPCCM method mines more long subsequences than the hierarchical clustering algorithm. Furthermore, in the high correlation threshold mining, the CPCCM method mines more subsequence pairs than the hierarchical clustering algorithm, as shown by 3c, 4c, 3f, and 4f.
To quantitatively verify our algorithm, we consider the MHΓ-Modified Hubert's Γ Statistic [45] and the DVI-Dunn's Validity Index [46] to assess the quality of the resulting clusters. Both of these assessment methods are classical methods for judging clustering quality by comparing the resulting cluster structure with other clustering results for the same data set. The modified Hubert's Γ index is given by the following:
MHΓ=1M∑ni∑mj=i+1P(S(X)i,S(Y)j)⋅Q(S(X)i,S(Y)j) | (5) |
where M = n × m; n and m respectively represent the number of subsequences from the initial sequence X and Y in the clustering result, assuming that n ≤ m; P is the proximity matrix; and Q is a n × m matrix, where each Q(S(X)i, S(Y)j) is the distance between the clusters to which S(X)i and S(Y)j belong. The distance between two clusters c1 and c2 can be calculated by Eq 6.
dist(c1,c2)=∑nc1Xi=1∑nc2Yj=11−|r(S(X)c1i,S(Y)c2j)|+∑nc2Xi=1∑nc1Yj=11−|r(S(X)c2i,S(Y)c1j)|nc1X×nc2Y+nc2X×nc1Y | (6) |
where nc1X is the number of subsequences from sequence X in cluster c1,nc2Y is the number of subsequences from sequence Y in cluster c2,S(X)c1i is the i-th subsequence from sequence X in cluster c1,1≤i≤nc1X, r(S(X)i , S(Y)j) is the Pearson correlation coefficient between S(X)c1i and S(Y)c2j that can be calculated through Eq 1 or 2.
High values of the MHΓ index represent compact and well-separated clustering result, That is, there are more correlated between the subsequences within same cluster than the subsequences from different clusters. It is especially well suited for detecting compact and well-separated clusters, but it has the drawback of being dependent on the number of clusters. The DVI criterion does not depend on the number of clusters but it is more unstable than the MHΓ since it is based on single linkage distances. Therefore, we choose the average linkage distances to calculate the distance between two clusters, as shown in Eq 6. Dunn's Validity Index is given as follows:
DVI=mini,j{dist(ci,cj)maxk{diam(ck)}} | (6) |
where diam(ck) is the diameter of cluster ck, i.e., the maximum distance of the subsequences in cluster ck. High values of this index also represent compact and well-separated clusters.
Using Eq 5 and 7, the CPCCM algorithm and the AHC algorithm are used to calculate the MHΓ and DVI indicators in the positive correlation and the negative correlation top ten clusters, respectively, under the respective thresholds, and then the index values of the positive correlation clusters under the same operation of the same algorithm are obtained. Next, we add the index values that are obtained by the negative correlation cluster, and the results that are obtained are shown in Figures 5 and 6.
It can be seen from Figures 5 and 6 that the MHΓ and DVI index values of the CPCCM algorithm under the various thresholds are greater than or similar to the AHC algorithm results. Moreover, it can be found that under a high correlation threshold (such as 0.9), the difference between the evaluation index values of the CPCCM algorithm and the AHC algorithm is the largest, which indicates that the clustering quality of the CPCCM algorithm is more advantageous than the AHC algorithm when assessing the high correlation subsequence cluster set. Therefore, through the comparison experiments, it can be found that the CPCCM algorithm is efficient and accurate for solving the problem of time series subsequence correlation clustering.
To analyze the time series correlation of different objects in smart environments based on the Internet of Things, a time series clustering algorithm based on precise correlation coefficient matching (CPCCM) is proposed. After splitting the initial sequence that is acquired from the sensor using a sliding window, the algorithm performs precise correlation coefficient matching on the subsequences from different objects, clusters the subsequences that meet some preset threshold for the Pearson correlation coefficients, and merges the adjacent subsequences in the clustering process to obtain longer subsequences. Finally, the effectiveness of the method is verified using practical smart grid use cases and comparative experiments.
The merits of the proposed approach are as follows: 1) by using the strategy of precise matching and cross-traversal, the proposed algorithm improves the clustering quality, by merging adjacent subsequences, the complexity of the algorithm is reduced; and 2) the proposed algorithm is independent of specific development tools and platforms, so it can be flexibly applied to similar scenarios. However, the complexity of the algorithm will be increased in a higher dimension time series than in 1-dimension. In addition, in our method, time series should be kept continuous. If some segments are missing, they must be filled through some methods. The future work will focus on algorithm for high-dimensional time series data and reducing its complexity. We will apply the algorithm for high-dimensional time series data to intelligent manufacturing as sequence of resource service has varying number of dimensions.
This work was supported in part by a grant from JZ160409 of the Natural Foundation Key Program for Young Scholars in the Universities of Fujian Province, 2018C110R of the Quanzhou City Science & Technology Plan of China, and 2019H01010129, 2019H0017 of the Fujian Province Science and Technology Plan.
The authors declare that there is no conflict of interests regarding the publication of this article.
[1] | M. Weiser, R. Gold and J. S. Brown, The origins of ubiquitous computing research at PARC in the late 1980s, IBM Syst. J., 38 (1999), 693–696. |
[2] | V. A. Memos, K. E. Psannis, Y. Ishibashi, et al., An efficient algorithm for media-based surveillance system (EAMSuS) in IoT smart city framework, Future Gener. Comp. Syst., 83 (2018), 619–628. |
[3] | S. Tang, D. R. Shelden, C. M. Eastman, et al., A review of building information modeling (BIM) and the internet of things (IoT) devices integration: Present status and future trends, Automat. Const., 101 (2019), 127–139. |
[4] | T. Baker, A. Taleb-Bendiab, M. Randles, et al., Understanding elasticity of cloud services compositions, In 2012 IEEE Fifth International Conference on Utility and Cloud Computing, Chicago(USA), IEEE, (2012), 231–232. |
[5] | A. Jula, E. Sundararajan and Z. Othman, Cloud computing service composition: A systematic literature review, Expert Syst. Appl., 41 (2014), 3809–3824. |
[6] | Q. Wu, G. Ding, Y. Xu, et al., Cognitive internet of things: a new paradigm beyond connection, IEEE Int. Things J., 1 (2014), 129–143. |
[7] | C. Gomez, S. Chessa, A. Fleury, et al., Internet of Things for enabling smart environments: A technology-centric perspective, J. Ambient Int. Smart Environ., 11 (2019), 23–43. |
[8] | L. Atzori, A. Iera and G. Morabito, The internet of things: A survey, Comput. Netw., 54 (2010), 2787–2805. |
[9] | F. Chen, P. Deng, J. F. Wan, et al., Data Mining for the Internet of Things. Literature Review and Challenges, Int. J. Distrib. Sensor Netw., 11 (2015), P431047. |
[10] | H. Li, Z. Zhang, X. Wang, et al., Electricity consumption behaviour analysis based on time sequence clustering, In 2018 International Conference on Computer Information Engineering and Bioinformatics, Guangzhou(China), IOP Publishing, (2018), 032011. |
[11] | S. Pravilovic, M. Bilancia, A. Appice, et al., Using multiple time series analysis for geosensor data forecasting, Inf. Sci., 380 (2017), 31–52. |
[12] | J. Liu, W. Li, J. Wu, et al., Visualizing the intercity correlation of PM2. 5 time series in the Beijing-Tianjin-Hebei region using ground-based air quality monitoring data, PloS One, 13 (2018), e0192614. |
[13] | J. Soares, P. A. Makar, Y. Aklilu, et al., The use of hierarchical clustering for the design of optimized monitoring networks, Atmos. Chem. Phys., 18 (2018), 6543–6566. |
[14] | A. Zaslavsky, C. Perera and D. Georgakopoulos, Sensing as a service and big data, In International Conference on Advances in Cloud Computing (ACC-2012), Bangalore(India), Eprint Arxiv, (2012), 21–29. |
[15] | C. Chang and C. Li, Algebraic secret sharing using privacy homomorphisms for IoT-based healthcare systems, Math. Biosci. Eng., 16 (2019), 3367–3381. |
[16] | Y. Ren, Y. Leng, Y Cheng, et al., Secure data storage based on blockchain and coding in edge computing, Math. Biosci. Eng., 16 (2019), 1874–1892. |
[17] | C. Li and B. Palanisamy, Privacy in internet of things: From principles to technologies, IEEE Int. Things J., 6 (2019), 488–505. |
[18] | A. P. Plageras, K. E. Psannis, C. Stergiou, et al., Efficient IoT-based sensor BIG Data collection–processing and analysis in smart buildings, Future Gener. Comp. Syst., 82 (2018), 349–357. |
[19] | K. P. Kibiwott, Y. Zhao, J. Kogo, et al., Verifiable fully outsourced attribute-based signcryption system for IoT eHealth big data in cloud computing, Math. Biosci. Eng., 16 (2019), 3561–3594. |
[20] | S. K. Jensen, T. B. Pedersen and C. Thomsen, Time series management systems: A survey, IEEE T. Knowledge Data Eng., 29 (2017), 2581–2600. |
[21] | C. Stergiou, K. E. Psannis, A. P. Plageras, et al., Algorithms for efficient digital media transmission over IoT and cloud networking, J. Multimedia Inf. Syst., 5 (2018), 27–34. |
[22] | K. E. Psannis, C. Stergiou and B. B. Gupta, Advanced media-based smart big data on intelligent cloud systems, IEEE T. Sustain. Comput., 4 (2018), 77–87. |
[23] | W. Ejaz, M. Naeem, A. Shahid, et al., Efficient energy management for the internet of things in smart cities, IEEE Commun. Mag., 55 (2017), 84–91. |
[24] | A. F. Mohammad and V. Korosh, Energy management-as-a-service over fog computing platform, IEEE Int. Things J., 3 (2015), 161–169. |
[25] | F. Adenugba, S. Misra, R. Maskeliūnas, et al., Smart irrigation system for environmental sustainability in Africa: An Internet of Everything (IoE) approach, Math. Biosci. Eng., 16 (2019), 5490–5503. |
[26] | M. Izal, D. Morató, E. Magaña, et al., Computation of traffic time series for large populations of IoT devices, Sensors, 19 (2019), 78. |
[27] | Ş. Kolozali, D. Puschmann, M. Bermudez-Edo, et al., On the effect of adaptive and nonadaptive analysis of time-series sensory data, IEEE Int. Things J., 3 (2016), 1084–1098. |
[28] | R. Salles, P. Mattos, A. M. D. Iorgulescu, et al., Evaluating temporal aggregation for predicting the sea surface temperature of the Atlantic Ocean. Ecol. Inform., 36 (2016), 94–105. |
[29] | J. Roberts, M. Curran, S. Poynter, et al., Correlation confidence limits for unevenly sampled data, Comput. Geosci., 104 (2017), 120–124. |
[30] | I. Ozken, D. Eroglu, S. F. Breitenbach, et al., Recurrence plot analysis of irregularly sampled data, Phys. Rev. E., 98 (2018), 052215. |
[31] | H. Li, K. C. C. Chan, M. Liang, et al., Composition of resource-service chain for cloud manufacturing, IEEE T. Ind. Informat., 12 (2016), 211–219. |
[32] | H. Li, M. Liang and T. Liang, Optimizing the composition of a resource service chain with inter-organizational collaboration, IEEE T. Ind. Informat., 13 (2017), 1152–1161. |
[33] | H. Li and T. He, Selecting key feature sequence of resource services in industrial internet of things, IEEE Access, 6 (2018), 72152–72162. |
[34] | L. Wen, L. Gao, Y. Dong, et al., A negative correlation ensemble transfer learning method for fault diagnosis based on convolutional neural network, Math. Biosci. Eng., 16 (2019), 3311–3330. |
[35] | Z. Zhang, L. Liu, S. Zhang, et al., A service-based method for multiple sensor streams aggregation in fog computing, Wireless Commun. Mobile Comput., 1 (2018), 1–11. |
[36] | M. Mehdizadeh, R. Ghazi and M. Ghayeni, Power system security assessment with high wind penetration using the farms models based on their correlation, IET Renew. Power Gener., 12 (2018), 893–900. |
[37] | Z. Chen, Z. Xue, L. Zhang, et al., Analyzing the correlation and predictability of wind speed series based on mutual information, IEEE T. Electr. Electr. Eng., 13 (2018), 1829–1830. |
[38] | J. Olauson and M. Bergkvist, Correlation between wind power generation in the European countries, Energy, 114 (2016), 663–670. |
[39] | F. Wang, A novel coefficient for detecting and quantifying asymmetry of California electricity market based on asymmetric detrended cross-correlation analysis, Chaos Interdiscipl. J. Nonlinear Sci., 26 (2016), 063109. |
[40] | T. Cui, F. Caravelli and C. Ududec, Correlations and clustering in wholesale electricity markets, Physica A., 492 (2018), 1507–1522. |
[41] | R. Lin, B Wu and Y Su, An adaptive weighted pearson similarity measurement method for load curve clustering, Energies, 11 (2018), 1–17. |
[42] | A. Mueen, H. Hamooni and T. Estrada, Time series join on subsequence correlation, In 2014 IEEE International Conference on Data Mining, Shenzhen(China), IEEE Computer Society Press, (2014), 450–459. |
[43] | Z. Ye, S. Mistry, A. Bouguettaya, et al., Long-term QoS-aware cloud service composition using multivariate time series analysis, IEEE T. Services Comput., 9 (2014), 382–393. |
[44] | M. Disegna, P. D'Urso and F. Durante, Copula-based fuzzy clustering of spatial time series, Spat. Stat., 21 (2017), 209–225. |
[45] | J. C. Dunn, Well-separated clusters and optimal fuzzy partitions, J. Cybernetics, 4 (1974), 95–104. |
[46] | M. Halkidi, Y. Batistakis and M Vazirgiannis, On clustering validation techniques, J. Intell. Inf. Syst., 17 (2001), 107–145. |
1. | Haibo Li, Shaoyuan Weng, Juncheng Tong, Ting He, Wenyun Chen, Mengmeng Sun, Yingtong Shen, Composition of Resource-Service Chain Based on Evolutionary Algorithm in Distributed Cloud Manufacturing Systems, 2020, 8, 2169-3536, 19911, 10.1109/ACCESS.2020.2969234 | |
2. | Chengshuang Miao, Clustering of different dimensional variables based on distance correlation coefficient, 2021, 1868-5137, 10.1007/s12652-020-02817-y | |
3. | Yawen Zhang, Application of distributed data system and fuzzy feature recognition in school enterprise cooperative talent training mode, 2021, 1868-5137, 10.1007/s12652-021-03206-9 | |
4. | Dashe Li, Xueying Wang, Jiajun Sun, Huanhai Yang, AI-HydSu: An advanced hybrid approach using support vector regression and particle swarm optimization for dissolved oxygen forecasting, 2021, 18, 1551-0018, 3646, 10.3934/mbe.2021182 | |
5. | Haibo Li, Yongbo Yu, Xiang Chen, Hao Xie, Gengqian Wei, Sina Xu, 2022, Chapter 12, 978-3-030-81006-1, 96, 10.1007/978-3-030-81007-8_12 | |
6. | Haibo Li, Cheng Wang, Gengqian Wei, Sina Xu, Mining the Coopetition Relationship of Urban Public Traffic Lines Based on Time Series Correlation, 2021, 2138, 1742-6588, 012005, 10.1088/1742-6596/2138/1/012005 | |
7. | Haibo Li, Yongbo Yu, Detecting a multigranularity event in an unequal interval time series based on self-adaptive segmenting, 2021, 25, 1088467X, 1407, 10.3233/IDA-205480 | |
8. | J. Fernando Vera-Vera, J. Antonio Roldán-Nofuentes, A robust alternating least squares K-means clustering approach for times series using dynamic time warping dissimilarities, 2024, 21, 1551-0018, 3631, 10.3934/mbe.2024160 | |
9. | Keruo Jiang, Zhen Huang, Xinyan Zhou, Chudong Tong, Minjie Zhu, Heshan Wang, Deep belief improved bidirectional LSTM for multivariate time series forecasting, 2023, 20, 1551-0018, 16596, 10.3934/mbe.2023739 |
time(Y-M-D H:M:S) | digits of electricity meter |
2016-03-01 11:10:07.0 | 157212.0 |
2016-03-01 11:40:08.0 | 157218.0 |
2016-03-01 12:10:09.0 | 157225.2 |
2016-03-01 12:40:10.0 | 157234.8 |
2016-03-01 13:10:11.0 | 157240.2 |
2016-03-01 13:40:12.0 | 157244.4 |
subsequence number | subsequences from initial sequence X |
1 | (6, 7.2, 9.6, 5.4, 4.2, 4.2, 4.8, 7.8, 7.8, 7.8, 9, 11.4, 11.4, 13.8, 14.4, 15.6, 16.2, 17.4, 13.2, 14.4, 14.4, 13.8, 12, 4.2) |
2 | (7.2, 9.6, 5.4, 4.2, 4.2, 4.8, 7.8, 7.8, 7.8, 9, 11.4, 11.4, 13.8, 14.4, 15.6, 16.2, 17.4, 13.2, 14.4, 14.4, 13.8, 12, 4.2, 2.4) |
3 | (9.6, 5.4, 4.2, 4.2, 4.8, 7.8, 7.8, 7.8, 9, 11.4, 11.4, 13.8, 14.4, 15.6, 16.2, 17.4, 13.2, 14.4, 14.4, 13.8, 12, 4.2, 2.4, 1.8) |
4 | (5.4, 4.2, 4.2, 4.8, 7.8, 7.8, 7.8, 9, 11.4, 11.4, 13.8, 14.4, 15.6, 16.2, 17.4, 13.2, 14.4, 14.4, 13.8, 12, 4.2, 2.4, 1.8, 1.2) |
5 | (4.2, 4.2, 4.8, 7.8, 7.8, 7.8, 9, 11.4, 11.4, 13.8, 14.4, 15.6, 16.2, 17.4, 13.2, 14.4, 14.4, 13.8, 12, 4.2, 2.4, 1.8, 1.2, 1.2) |
Top 10 largest clusters | positive correlation with ε = 0.75 | positive correlation with ε = 0.8 | positive correlation with ε = 0.9 | |||
corrintra | corrinter | corrintra | corrinter | corrintra | corrinter | |
c1 | 0.8017 | 0.1850 | 0.8347 | 0.2362 | 0.9123 | 0.2895 |
c2 | 0.7886 | 0.1568 | 0.8217 | 0.1995 | 0.9095 | 0.2505 |
c3 | 0.7924 | 0.1876 | 0.8254 | 0.2324 | 0.9102 | 0.3088 |
c4 | 0.7760 | 0.1390 | 0.8315 | 0.2608 | 0.9134 | 0.2628 |
c5 | 0.7853 | 0.1585 | 0.8207 | 0.1977 | 0.9090 | 0.2294 |
c6 | 0.7729 | 0.1820 | 0.8259 | 0.1483 | 0.9123 | 0.3049 |
c7 | 0.7825 | 0.1697 | 0.8218 | 0.2168 | 0.9163 | 0.3127 |
c8 | 0.7737 | 0.1117 | 0.8325 | 0.1885 | 0.9150 | 0.1978 |
c9 | 0.7796 | 0.1665 | 0.8472 | 0.1400 | 0.9138 | 0.3006 |
c10 | 0.7749 | 0.1614 | 0.8159 | 0.1393 | 0.9161 | 0.1764 |
Top 10 largest clusters | negative correlation with ε = 0.75 | negative correlation with ε = 0.8 | negative correlation with ε = 0.9 | |||
corrintra | corrinter | corrintra | corrinter | corrintra | corrinter | |
c1 | -0.7913 | -0.2474 | -0.8227 | -0.2161 | -0.9130 | -0.3112 |
c2 | -0.7846 | -0.1923 | -0.8334 | -0.3034 | -0.9108 | -0.3785 |
c3 | -0.7840 | -0.2498 | -0.8224 | -0.2446 | -0.9150 | -0.2679 |
c4 | -0.7941 | -0.2165 | -0.8289 | -0.2778 | -0.9088 | -0.4079 |
c5 | -0.7746 | -0.1162 | -0.8197 | -0.2664 | -0.9141 | -0.2806 |
c6 | -0.7754 | -0.2317 | -0.8240 | -0.1630 | -0.9151 | -0.2674 |
c7 | -0.7772 | -0.2362 | -0.8164 | -0.2917 | -0.9184 | -0.2492 |
c8 | -0.7815 | -0.2217 | -0.8208 | -0.2214 | -0.9115 | -0.3874 |
c9 | -0.7806 | -0.1533 | -0.8289 | -0.1634 | -0.9144 | -0.3806 |
c10 | -0.7672 | -0.2442 | -0.8314 | -0.2099 | -0.9092 | -0.2487 |
time(Y-M-D H:M:S) | digits of electricity meter |
2016-03-01 11:10:07.0 | 157212.0 |
2016-03-01 11:40:08.0 | 157218.0 |
2016-03-01 12:10:09.0 | 157225.2 |
2016-03-01 12:40:10.0 | 157234.8 |
2016-03-01 13:10:11.0 | 157240.2 |
2016-03-01 13:40:12.0 | 157244.4 |
subsequence number | subsequences from initial sequence X |
1 | (6, 7.2, 9.6, 5.4, 4.2, 4.2, 4.8, 7.8, 7.8, 7.8, 9, 11.4, 11.4, 13.8, 14.4, 15.6, 16.2, 17.4, 13.2, 14.4, 14.4, 13.8, 12, 4.2) |
2 | (7.2, 9.6, 5.4, 4.2, 4.2, 4.8, 7.8, 7.8, 7.8, 9, 11.4, 11.4, 13.8, 14.4, 15.6, 16.2, 17.4, 13.2, 14.4, 14.4, 13.8, 12, 4.2, 2.4) |
3 | (9.6, 5.4, 4.2, 4.2, 4.8, 7.8, 7.8, 7.8, 9, 11.4, 11.4, 13.8, 14.4, 15.6, 16.2, 17.4, 13.2, 14.4, 14.4, 13.8, 12, 4.2, 2.4, 1.8) |
4 | (5.4, 4.2, 4.2, 4.8, 7.8, 7.8, 7.8, 9, 11.4, 11.4, 13.8, 14.4, 15.6, 16.2, 17.4, 13.2, 14.4, 14.4, 13.8, 12, 4.2, 2.4, 1.8, 1.2) |
5 | (4.2, 4.2, 4.8, 7.8, 7.8, 7.8, 9, 11.4, 11.4, 13.8, 14.4, 15.6, 16.2, 17.4, 13.2, 14.4, 14.4, 13.8, 12, 4.2, 2.4, 1.8, 1.2, 1.2) |
Top 10 largest clusters | positive correlation with ε = 0.75 | positive correlation with ε = 0.8 | positive correlation with ε = 0.9 | |||
corrintra | corrinter | corrintra | corrinter | corrintra | corrinter | |
c1 | 0.8017 | 0.1850 | 0.8347 | 0.2362 | 0.9123 | 0.2895 |
c2 | 0.7886 | 0.1568 | 0.8217 | 0.1995 | 0.9095 | 0.2505 |
c3 | 0.7924 | 0.1876 | 0.8254 | 0.2324 | 0.9102 | 0.3088 |
c4 | 0.7760 | 0.1390 | 0.8315 | 0.2608 | 0.9134 | 0.2628 |
c5 | 0.7853 | 0.1585 | 0.8207 | 0.1977 | 0.9090 | 0.2294 |
c6 | 0.7729 | 0.1820 | 0.8259 | 0.1483 | 0.9123 | 0.3049 |
c7 | 0.7825 | 0.1697 | 0.8218 | 0.2168 | 0.9163 | 0.3127 |
c8 | 0.7737 | 0.1117 | 0.8325 | 0.1885 | 0.9150 | 0.1978 |
c9 | 0.7796 | 0.1665 | 0.8472 | 0.1400 | 0.9138 | 0.3006 |
c10 | 0.7749 | 0.1614 | 0.8159 | 0.1393 | 0.9161 | 0.1764 |
Top 10 largest clusters | negative correlation with ε = 0.75 | negative correlation with ε = 0.8 | negative correlation with ε = 0.9 | |||
corrintra | corrinter | corrintra | corrinter | corrintra | corrinter | |
c1 | -0.7913 | -0.2474 | -0.8227 | -0.2161 | -0.9130 | -0.3112 |
c2 | -0.7846 | -0.1923 | -0.8334 | -0.3034 | -0.9108 | -0.3785 |
c3 | -0.7840 | -0.2498 | -0.8224 | -0.2446 | -0.9150 | -0.2679 |
c4 | -0.7941 | -0.2165 | -0.8289 | -0.2778 | -0.9088 | -0.4079 |
c5 | -0.7746 | -0.1162 | -0.8197 | -0.2664 | -0.9141 | -0.2806 |
c6 | -0.7754 | -0.2317 | -0.8240 | -0.1630 | -0.9151 | -0.2674 |
c7 | -0.7772 | -0.2362 | -0.8164 | -0.2917 | -0.9184 | -0.2492 |
c8 | -0.7815 | -0.2217 | -0.8208 | -0.2214 | -0.9115 | -0.3874 |
c9 | -0.7806 | -0.1533 | -0.8289 | -0.1634 | -0.9144 | -0.3806 |
c10 | -0.7672 | -0.2442 | -0.8314 | -0.2099 | -0.9092 | -0.2487 |