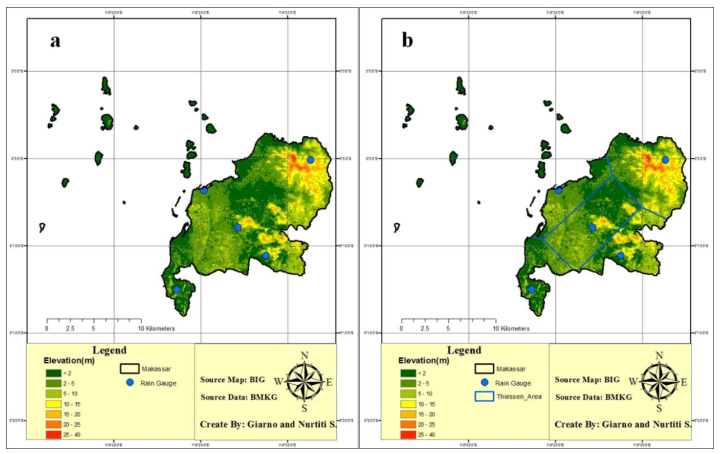
Improving the accuracy of rainfall forecasts is related to the number of rain gauges needed in an area, so determining the optimal number of rain gauges is very important. This study aimed to determine the best method for calculating the optimal number of rain gauges. Generally, the calculation of the optimal number of rain gauges using the coefficient of variation only takes into account the accumulation of rainfall at the station. The distance between the location and height of the rain gauge is not taken into account. The phenomenon of rain that occurs in the tropics is very dynamic, where one place compared to another tends to have different rain intensity and duration. In addition, the height and distance factors also greatly affect the measured rainfall. Therefore, it is very important to know the best method to calculate the optimal number of rain gauges needed in a particular area. This study implements 3 methods to determine the appropriate method to be used in determining the optimal rain gauge number for urban areas: namely, World Meteorological Organization (WMO) criteria, coefficient of variation, and Kagan-Rodda. In this study, rainfall data from 2010 to 2019 at 5 locations in Makassar were used in calculating the optimal number of rain gauges required. The results showed that the optimal number of rain gauges in Makassar as an urban area following the WMO recommendation was 9–18, where small islands around it are not considered. Another result obtained is that if the rainfall data for the Sudiang area, which is located at the coordinates (119.522° E, 5.085° S), is not included in the calculation, it will greatly reduce the accuracy in determining the optimal number of rain gauges in the Makassar area.
Citation: Nurtiti Sunusi, Giarno. Comparison of some schemes for determining the optimal number of rain gauges in a specific area: A case study in an urban area of South Sulawesi, Indonesia[J]. AIMS Environmental Science, 2022, 9(3): 260-276. doi: 10.3934/environsci.2022018
[1] | Nurtiti Sunusi, Giarno . Bias of automatic weather parameter measurement in monsoon area, a case study in Makassar Coast. AIMS Environmental Science, 2023, 10(1): 1-15. doi: 10.3934/environsci.2023001 |
[2] | RAHMOUN Ibrahim, BENMAMAR Saâdia, RABEHI Mohamed . Comparison between different Intensities of Rainfall to identify overflow points in a combined sewer system using Storm Water Management Model. AIMS Environmental Science, 2022, 9(5): 573-592. doi: 10.3934/environsci.2022034 |
[3] | Lei Wang, Huan Du, Jiajun Wu, Wei Gao, Linna Suo, Dan Wei, Liang Jin, Jianli Ding, Jianzhi Xie, Zhizhuang An . Characteristics of soil erosion in different land-use patterns under natural rainfall. AIMS Environmental Science, 2022, 9(3): 309-324. doi: 10.3934/environsci.2022021 |
[4] | Ronak P. Chaudhari, Shantanu R. Thorat, Darshan J. Mehta, Sahita I. Waikhom, Vipinkumar G. Yadav, Vijendra Kumar . Comparison of soft-computing techniques: Data-driven models for flood forecasting. AIMS Environmental Science, 2024, 11(5): 741-758. doi: 10.3934/environsci.2024037 |
[5] | Muhammad Rendana, Wan Mohd Razi Idris, Sahibin Abdul Rahim . Clustering analysis of PM2.5 concentrations in the South Sumatra Province, Indonesia, using the Merra-2 Satellite Application and Hierarchical Cluster Method. AIMS Environmental Science, 2022, 9(6): 754-770. doi: 10.3934/environsci.2022043 |
[6] | Swatantra R. Kethireddy, Grace A. Adegoye, Paul B. Tchounwou, Francis Tuluri, H. Anwar Ahmad, John H. Young, Lei Zhang . The status of geo-environmental health in Mississippi: Application of spatiotemporal statistics to improve health and air quality. AIMS Environmental Science, 2018, 5(4): 273-293. doi: 10.3934/environsci.2018.4.273 |
[7] | Dong Chen, Marcus Thatcher, Xiaoming Wang, Guy Barnett, Anthony Kachenko, Robert Prince . Summer cooling potential of urban vegetation—a modeling study for Melbourne, Australia. AIMS Environmental Science, 2015, 2(3): 648-667. doi: 10.3934/environsci.2015.3.648 |
[8] | Zinabu A. Alemu, Emmanuel C. Dioha, Michael O. Dioha . Hydro-meteorological drought in Addis Ababa: A characterization study. AIMS Environmental Science, 2021, 8(2): 148-168. doi: 10.3934/environsci.2021011 |
[9] | Robert Russell Monteith Paterson . Depletion of Indonesian oil palm plantations implied from modeling oil palm mortality and Ganoderma boninense rot under future climate. AIMS Environmental Science, 2020, 7(5): 366-379. doi: 10.3934/environsci.2020024 |
[10] | Meriatna, Zulmiardi, Lukman Hakim, Faisal, Suryati, Mizwa Widiarman . Adsorbent performance of nipa (nypafruticans) frond in methylene blue dye degradation: Response surface methodology optimization. AIMS Environmental Science, 2024, 11(1): 38-56. doi: 10.3934/environsci.2024003 |
Improving the accuracy of rainfall forecasts is related to the number of rain gauges needed in an area, so determining the optimal number of rain gauges is very important. This study aimed to determine the best method for calculating the optimal number of rain gauges. Generally, the calculation of the optimal number of rain gauges using the coefficient of variation only takes into account the accumulation of rainfall at the station. The distance between the location and height of the rain gauge is not taken into account. The phenomenon of rain that occurs in the tropics is very dynamic, where one place compared to another tends to have different rain intensity and duration. In addition, the height and distance factors also greatly affect the measured rainfall. Therefore, it is very important to know the best method to calculate the optimal number of rain gauges needed in a particular area. This study implements 3 methods to determine the appropriate method to be used in determining the optimal rain gauge number for urban areas: namely, World Meteorological Organization (WMO) criteria, coefficient of variation, and Kagan-Rodda. In this study, rainfall data from 2010 to 2019 at 5 locations in Makassar were used in calculating the optimal number of rain gauges required. The results showed that the optimal number of rain gauges in Makassar as an urban area following the WMO recommendation was 9–18, where small islands around it are not considered. Another result obtained is that if the rainfall data for the Sudiang area, which is located at the coordinates (119.522° E, 5.085° S), is not included in the calculation, it will greatly reduce the accuracy in determining the optimal number of rain gauges in the Makassar area.
A reliable number and network of weather stations can provide a high quality of rainfall data that is crucial for the effective design of water management systems. However, a rain gauge network was installed to make it easier for observers to collect data. The installation of this tool does not consider variations in the characterization of spatial and temporal variations of rainfall, which can reduce accuracy. A well-designed rain gauge network can illustrate the spatial and temporal rainfall variability in a catchment [1]. Generally, a denser rain gauge network will produce higher accuracy, but too much equipment will result in the redundancy of the rain gauges [2]. Rain gauge network design is the most important issue in water resources management. This has attracted the attention of researchers for many years [3]. The distribution of rain gauge stations greatly influences the quality of rainfall variables in a network. Furthermore, the lack of rain gauges in the hydrometeorological network causes a decrease in the quality of rain data. On the other hand, the existing network may consist of redundant stations that may make little or no contribution to data quality.
Indonesian territory has a complex topography. This archipelago country consists of more than 17 thousand islands with various topographies separated by seas and straits. The interaction of global and local weather circulation greatly affects the rainfall in a place, causing rainfall variations from one location to another. The calculation of the optimal number of rain gauges is based on topography, position, and population density [4]. This method is still very general because it does not take into account variations in rainfall but only calculates the amount of rain gauge without considering the rain gauge network. The WMO criteria are generally only used as a preliminary study in calculating the number of rain gauges [5,6].
The number of rain gauges in Java, especially in Central Java and East Java, has a high density, so it needs to be rationalized. On the other hand, in areas outside Java, the number of rain gauges is still lacking for the same level of accuracy [7]. Another problem is how to determine the rain gauges in an efficient rain gauge network so that the rain gauge location is not expected to be redundant. The Kagan-Rodda method is widely used in research to determine the rain network in Indonesia [8,9].
The number of rain gauges can be calculated based on a certain area in the topography and position of the area, as recommended by World Meteorological Organization (WMO). This method does not take into account different rainfall characteristics in each place, so research was developed to determine the optimal number of rain gauges based on a statistical approach. Rycroft (1949) determined the optimal rain gauge number by distance variance in the annual rainfall data at Jonker Shook catchment [10]. Furthermore, the coefficient of variation (CV) can be used to determine the location to be added or subtracted. Determination of the location still pays less attention to the spatial variance of rainfall [11,12]. Researchers in Indonesia mostly use the Kagan-Rodda method to determine the locations of the rain gauges based on maximum monthly rainfall data or annual rainfall data [13].
The determination of the locations of the rain gauge using Kagan-Rodda is based on a net of equilateral triangles whose side length is the optimal distance. Although elevation has a high correlation to rainfall [5,8,14], rainfall in Indonesia is known to vary with its position towards the coast, which has a low elevation [15]. Therefore, determining the optimal rain gauge number in a relatively flat area such as Makassar considers the variation in rainfall. This study aimed to calculate the number of optimal rain gauges and their placement by considering a variety of statistical rainfall data and rain gauge placement.
This paper is structured as follows: Section 2 describes the study area and the data used. Furthermore, the methodology is described to obtain the optimal number of rain gauges, the distribution, and the evaluation method used. Section 3 describes the results of this work and continues with the discussion in Section 4. The last section is the conclusion of this study.
The big cities that are densely populated are generally located on the edge of the sea, which has flat topography. A city is the center of economic, social, educational, cultural, and trade activities, so the management of water resources is vital. This research was conducted in Makassar city, which is the capital city of South Sulawesi province. The city has an area of 175.7 km2 with a population of more than 1,600,000 inhabitants. Furthermore, the highest elevation of Makassar city is 20 m, but in the east, there are high mountains. This region has a plain area with a slope of 0–5 degrees and is bordered by a shoreline.
Rainy and dry periods in this region are very strict, following the Asian monsoon. However, the intensity and duration of rain depend on the interactions of other global weather cycles, such as Madden–Julian oscillation (MJO), El Niño–Southern Oscillation (ENSO), Indian Ocean Dipole (IOD), cold surges, and local cycles of sea-land winds [16,17,18]. The MJO is the major fluctuation in tropical weather on weekly to monthly timescales, moving along the equator from the Indian Ocean to the Pacific. ENSO is a wind and sea surface temperature irregular periodic variation over the tropical eastern Pacific Ocean, and IOD induces climate and weather extremes in the Indian Ocean. The influence of the land-sea wind is very strong on the beach and decreases inland on Bawakaraeng Mountain. Although the rainfall pattern in this place is almost the same, sometimes there are differences during extreme conditions, such as a flood. The most extreme flood phenomena in January 2019 showed that rainfall measurements at the five stations are very different, especially between Barombong (346 mm) and Sudiang (1204 mm). This striking difference raises the question of how much rain gauge coverage there should be in Makassar City.
This research used rainfall data on 5 sites in Makassar, i.e., Paotere, Panaikang, Barombong, Biring Romang, and Sudiang, as depicted in Figure 1(a). The manual rain gauges had rainfall measurements taken simultaneously each 07.00 local time, and data was collected by the coordinating station for further analysis. Paotere marine station (119.420° E, 5.114° S) is located on the coast which is directly adjacent to the Makassar Strait, and its elevation is 2 meters. The second location close to the sea is Barombong (119.394° E, 5.208° S). Barombong is located south of Paotere, with an elevation of 3 meters. Meanwhile, the locations of Sudiang (119.522° E, 5.085° S) and Biring Romang (119.479° E, 5.176° S) are relatively far from the beach, with elevations of 14 meters and 8 meters. The last station is Panaikang (119.452° E, 5.149° S), which has an elevation of 5 meters. Meanwhile, Figure 1(b) shows the groupings of rainfall areas that are considered to have the same rainfall pattern according to the Thiessen polygon method.
This study uses monthly rainfall data from 2010 to 2019. Although the heights of the five rainfall measurement locations are relatively similar, the detailed characteristics of rainfall, namely the amount of rainfall in each place, show significant differences.
In Indonesia, the selection of a rain gauge location is generally not based on a thorough preliminary study. Rain gauges are installed in places owned by Indonesian Meteorology, Climatology, and Geophysical Agency (Badan Meteorologi, Klimatologi, dan Geofisika (BMKG)) or cooperating institutions. Therefore, sometimes they are found very close; on the other hand, some are located far away. Recalculation of the optimal number of rain gauges has been developed to correct the number of rain gauges and the network [7,9]. Determination of the number of rain gauges can use the WMO criteria, coefficient of variation, Kagan-Rodda, and kriging, with the following explanations.
A rain gauge is expected to be representative of a certain area. However, the surface of the earth is not homogeneous, so it is very difficult to generalize one criterion. Therefore, the World Meteorological Organization (WMO) determines the distances and density of rain gauges depending on the topography, location, and type of instrument. A rain gauge measurement can only be representative of or considered a certain area. Following the WMO recorded equipment standards for coastal, mountainous, interior plain silly, a small island, and urban areas, a rain gauge is representative of 9000 km2, 2500 km2, 5750 km2, 5750 km2, 250 km2, and 10–20 km2, respectively. Meanwhile, a non-recorded rain gauge can represent 900 km2, 250 km2, 575 km2, 575 km2, and 25 km2 for coastal, mountainous, interior plains, hilly and small island areas, respectively. Moreover, the non-recorded rain gauge for urban areas depends on the need, and the standard is not specified.
The Meteorology Climatology and Geophysics Agency, an Indonesian institution that organizes weather data, has 186 stations. Considering the complexity of Indonesian weather and topography, this number is not sufficient for the WMO standard. Makassar city, which covers 175 km2, is located in a coastal area, so if the deployment of rain gauges uses a coastal density standard, then only 1 rain gauge is needed. However, apart from being a coastal area, Makassar is also a densely populated urban area, so it requires a lot of rain gauges.
The variance is widely used to determine the optimal number of rain gauges [19,20,21]. If the coefficient of variation (CV) is obtained from standard deviation σ divided by the number of observations n, or CV=100∗σn, then the optimal rain gauge number (N0) based on the relation of coefficient of variation (CV) and ε, the level of desired error, is formulated by
N0=(CVϵ)2. | (1) |
The value of the error rate greatly affects the optimum rain gauge number N0. In the study of the need for rain gauges with various test error levels of 5%, 10%, 15%, 20%, and 25%, it turns out that the results show an error rate of 15% or more can produce several rain gauges that are optimal and do not fluctuate [13]. Considering that Makassar is an urban area and flood-prone area [20,22], in this study an ε of 5% was used in designing the rain station network.
Research to establish a rain station network is not only limited to the number of stations needed in an area but also to the location and distribution pattern. The number and location of rain gauges can be approached by the Kagan method as defined by the World Meteorological Organization [21]. This method uses statistical analysis that relates the density of the rain station network to interpolation errors and averaging errors.
A rain gauge represents a certain catchment of precipitation, and the Thiessen polygon method is a commonly used methodology for computing the mean areal precipitation for a catchment from rain gauge observations. The station network density calculation uses the Thiessen polygon method, where each rain station has an area of influence. In this study, R software was used to create polygons and calculate the area of influence of each rain station. The size of the area of influence is then checked with the World Meteorological Organization standards for urban areas.
Based on the calculation area using the Thiessen polygon method on the 5 rain gauges in Makassar city, as shown in Figure 1(b), it was found that the area of influence of the rain gauge in Barombong is 21.003 km2. Meanwhile, areas of influence were found for the rain gauges in Sudiang (52.310 km2), Birring Romang (30.912 km2), Panaikang (46.830 km2), and Paotere (31.827 km2). Based on the WMO guidelines for urban areas (a minimum of one rain gauge per 20 km2), all rain gauge areas are still inadequate. The steps for calculating the need for a rain gauge using Kagan-Rodda are as follows:
1) At each rain gauge location, the maximum monthly rainfall per year is selected.
2) Correlate between stations on the results of the rainfall in step 1) and then calculate the average correlation for each location.
3) Calculate the distances between rain stations at each rain gauge location, and then calculate the average distance for each location.
4) Graph the average correlation in step 2 with the mean distance in step 3.
5) Create the graph exponential equation in step 4.
Based on the exponential equation, the values of R0 and d(0) are calculated by connecting them to the equation.
Rd=ROe−d╱d(0) | (2) |
6) Calculate the smoothing error (Z1) and the interpolation error (Z2) of the formulation
Z1=CV√[1−r0+(0.23√A)d0√n]n. | (3) |
Z2=CV√1−r03+0.52r0d0√An | (4) |
7) Calculate the distance of the Kagan-Rodda net triangle using the equation
L=1.07√An | (5) |
with r(d) = correlation coefficient in distance d km
r(o) = correlation coefficient in distance 0 km
CV: variation coefficient
d: distance between locations (km)
A: area of region (km2)
n: number of locations
Z1: smoothing error (%)
Z2: interpolation error (%)
L: distance between rain gauges in the Kagan-Rodda triangle (km)
8) Build a Kagan-Rodda equilateral triangle net, and then place it on the Makassar map (Figure 1(b)) so that the number of vertices of the Kagan-Rodda equilateral triangle is the same as the optimum rain gauge location.
Although elevation has a high correlation with rainfall, rainfall in Indonesia is known to vary with its position towards the coast, which has a low elevation [5,7]. Therefore, determining the optimal rain gauge number in a relatively flat area such as Makassar considers the variations in rainfall. The optimal calculation of the rain gauge number using Kagan-Rodda in this research is calculated based on the variation of rainfall statistically, namely, the average and maximum rainfall values for each month, the maximum monthly rainfall in a year, and the monthly rainfall in 10 years.
Once the optimal number of rain gauges is determined, their accuracy will be tested using hold-out validation, taking into account the rainfall catchment in Makassar city. Because each rain gauge is considered to represent the catchment of water in a certain area and to measure how much water is accommodated in this city, the amount of rainfall recorded in the rain gauge is multiplied by the area represented by the rain gauge. The calculation of the watershed area represented by a rain gauge is obtained by applying the Thiessen polygon. The deviation from the rain gauge measurement results is calculated using correlation, root mean square prediction error, and mean absolute error, and it can be determined whether the estimated number of rain gauges is correct. Because the satellite estimation data has a very rough resolution, this study cannot use this type of data as a comparison. Because there is no weather radar data, the optimal number of rain gauges cannot be calculated for effectiveness if the number is more than the existing number of rain gauges.
Makassar city has an area of 175 km2 and is located on the coast. If the coastal density standard is used, this area only requires 1 rain gauge. However, the city of Makassar is an urban area with a high population density, so it is considered to use a standard urban area recording device, where the rainfall gauge represents an area of 10–20 km2. Therefore, for an area of 175 km2, 9–18 rain gauges must be installed in that location. When compared between unrecorded and recorded rain gauges, the unrecorded area coverage is 1:10 compared to recorded rain gauges, or 1–2 km2 for rain gauges. These requirements are difficult to fulfill, especially for developing countries like Indonesia. This work uses the maximum WMO standard for urban areas with a recorded rain gauge, and one rain gauge for 10 km2.
Maximum monthly rainfall is calculated at each location for each year. Based on the rainfall station data in Makassar city, the maximum monthly rainfall is obtained, as shown in Table 1. The maximum monthly rainfall varies between 346 mm and 1285 mm. The maximum rainfall intensity at the five stations varies, especially in Panaikang, Biringromang, and Sudiang, which reach more than 1000 mm/month. However, the accumulation of rainfall that reaches more than 1000 mm is not the same every year, except in 2015, when Panaikang and Biringromang stations have almost the same value. The smallest variation of maximum rainfall occurs in Paotere, and the lowest maximum rainfall compared to the other 4 locations is in Paotere. Another scheme is to calculate the average and maximum rainfall by month to analyze the variation of the optimal number of rain gauges against changes in the month. Finally, the scheme is calculated based on the monthly rainfall data according to the time sequence.
Year | Paotere | Panaikang | Biringromang | Barombong | Sudiang |
2010 | 891 | 822 | 1,058 | 444 | 492 |
2011 | 858 | 779 | 1,008 | 569 | 656 |
2012 | 639 | 564 | 1,115 | 396 | 552 |
2013 | 982 | 1,035 | 736 | 772 | 354 |
2014 | 836 | 878 | 617 | 391 | 380 |
2015 | 962 | 1,041 | 1,065 | 468 | 438 |
2016 | 727 | 714 | 934 | 370 | 620 |
2017 | 932 | 1,026 | 862 | 422 | 1,285 |
2018 | 858 | 787 | 598 | 457 | 1,187 |
2019 | 637 | 578 | 983 | 346 | 1,204 |
Furthermore, given the calculated correlation r between rainfall stations, the strength of the correlation is described as very weak (± 0.000 to ± 0.199), weak (±0.200 to ± 0.399), moderate (±0.400 to ± .599), strong (±0.600 to ± 0.799) or very strong (± 0.800 to ± 1.000) [23]. The strength of the relationship is represented by the magnitude of the value of the correlation calculated between the maximum monthly rainfall inter-station, The results of the calculation show that the correlation between stations in the monthly maximum rainfall varies each year. Paotere, and Panaikang have a very strong correlation, while negative correlations were also found, especially between Sudiang and the other 4 places, as shown in Table 2.
Correlation | Paotere | Panaikang | Biringromang | Barombong | Sudiang |
Paotere | 1.000 | 0.948 | -0.308 | 0.637 | -0.222 |
Panaikang | 0.948 | 1.000 | -0.322 | 0.535 | -0.210 |
Biringromang | -0.308 | -0.322 | 1.000 | -0.212 | -0.154 |
Barombong | 0.637 | 0.535 | -0.212 | 1.000 | -0.377 |
Sudiang | -0.222 | -0.210 | -0.154 | -0.377 | 1.000 |
It is different if the correlation is calculated using the average data every month, from the average January to December, which shows the correlation between stations is very high, almost close to 1. It is likewise if the correlation is calculated using the maximum data each month, from the most rainfall. The 10-year high from January to December also shows a very high correlation between stations. On the other hand, the correlation between time series rainfall data between stations is more varied, while the relationship is not too strong between rainfall in Biringromang and other places. The Paotere–Panaikang and Paotere–Barombong correlations are very strong, as shown in Table 3.
Correlation | Paotere | Panaikang | Biringromang | Barombong | Sudiang | |
Paotere | average | 1.000 | 0.997 | 0.985 | 0.985 | 0.984 |
max | 1.000 | 0.989 | 0.938 | 0.971 | 1.000 | |
series | 1.000 | 0.973 | 0.740 | 0.929 | 0.723 | |
Panaikang | average | 0.997 | 1.000 | 0.992 | 0.994 | 0.989 |
max | 0.989 | 1.000 | 0.953 | 0.978 | 0.989 | |
series | 0.973 | 1.000 | 0.719 | 0.927 | 0.718 | |
Biringromang | average | 0.985 | 0.992 | 1.000 | 0.992 | 0.996 |
max | 0.938 | 0.953 | 1.000 | 0.921 | 0.938 | |
series | 0.740 | 0.719 | 1.000 | 0.758 | 0.517 | |
Barombong | average | 0.985 | 0.994 | 0.992 | 1.000 | 0.991 |
max | 0.971 | 0.978 | 0.921 | 1.000 | 0.971 | |
series | 0.929 | 0.927 | 0.758 | 1.000 | 0.670 | |
Sudiang | ave | 0.984 | 0.989 | 0.996 | 0.991 | 1.000 |
max | 1.000 | 0.989 | 0.938 | 0.971 | 1.000 | |
series | 0.723 | 0.718 | 0.517 | 0.670 | 1.000 |
The next step is to obtain the distances between rain stations, available in previous work [24], and then the average distance between rain stations is calculated. The relationship between distance and correlation is calculated based on the average value as shown in Table 2, the maximum monthly rainfall in a year, and the order of the rainfall data in Table 3. The results showed that the maximum rainfall incidence every year in Biringromang and Sudiang has a very small and almost zero correlation with the rainfall in other places. Moreover, the distance between stations is not always directly proportional to the correlation. Panaikang, which is on average the closest distance to other locations, does not have the strongest. Meanwhile, Paotere, with an average distance of 7.510 km, has the highest average correlation, except for the average each month. Furthermore, Barombong, whose average distance is 10.042 km, has a high average correlation compared to Biringromang, whose average distance is only 7.022 km.
Network schemes between stations use the Kagan-Rodda technique. Relationships relate to distance and correlation in exponential equations. To illustrate this relationship, we used a graph and derived it in an exponential equation. On the exponential graph, all schematics and exponential equations are represented by the average distance between stations (x-axis) and the average correlation value of rain stations (y-axis) as shown in Figure 2. The four schemes are as follows: first, the maximum rainfall each year; second, average each month; third, maximum each month; fourth, time series monthly data.
The Kagan-Rodda network analysis uses Eq 6 as follows:
Rd=R0e−d╱d(0) | (6) |
The relationship between distance and regular correlation uses an exponential approach [25,26]. Based on the comparison with Eq 6 in Figure 2(a), the first scheme has 0.566 for R0 and 1.122 for d(0). The value of R0 is the initial value of the correlation, which will change with the distance d and the multiplier factor d(0). The last value is obtained from 1/d(0) = 0.821. Afterward, this coefficient will later be used in calculating the smoothing error (Z1) and interpolation error (Z2), which are formulated as follows.
Z1=Cv√[1−r0+(0.23√A)d0√n]n | (7) |
Z2=Cv√1−r03+0.52r0d0√An | (8) |
L=1.07√An | (9) |
The coefficient of variation using the maximum monthly rainfall method in Makassar is 0.229. This shows that the rainfall data at each rain station in Makassar has a small variation in rainfall. The values of Z1 and Z2 were calculated using the parameters that have been obtained, the results of which are tabulated in Table 4.
N | CV | R0 | A | d(0) | (A/N)1/2 | Z1 (%) | Z2 (%) | Density |
1 | 0.229 | 0.5657 | 182.883 | 1.218 | 13.523 | 39.584 | 42.293 | 182.883 |
2 | 0.229 | 0.5657 | 182.883 | 1.218 | 9.562 | 24.235 | 30.534 | 91.441 |
3 | 0.229 | 0.5657 | 182.883 | 1.218 | 7.808 | 18.266 | 25.433 | 60.961 |
4 | 0.229 | 0.5657 | 182.883 | 1.218 | 6.762 | 14.978 | 22.452 | 45.721 |
5 | 0.229 | 0.5657 | 182.883 | 1.218 | 6.048 | 12.858 | 20.457 | 36.577 |
6 | 0.229 | 0.5657 | 182.883 | 1.218 | 5.521 | 11.361 | 19.010 | 30.480 |
7 | 0.229 | 0.5657 | 182.883 | 1.218 | 5.111 | 10.239 | 17.905 | 26.126 |
8 | 0.229 | 0.5657 | 182.883 | 1.218 | 4.781 | 9.362 | 17.030 | 22.860 |
9 | 0.229 | 0.5657 | 182.883 | 1.218 | 4.508 | 8.655 | 16.316 | 20.320 |
10 | 0.229 | 0.5657 | 182.883 | 1.218 | 4.276 | 8.070 | 15.722 | 18.288 |
11 | 0.229 | 0.5657 | 182.883 | 1.218 | 4.077 | 7.577 | 15.219 | 16.626 |
12 | 0.229 | 0.5657 | 182.883 | 1.218 | 3.904 | 7.155 | 14.787 | 15.240 |
13 | 0.229 | 0.5657 | 182.883 | 1.218 | 3.751 | 6.789 | 14.411 | 14.068 |
14 | 0.229 | 0.5657 | 182.883 | 1.218 | 3.614 | 6.468 | 14.080 | 13.063 |
15 | 0.229 | 0.5657 | 182.883 | 1.218 | 3.492 | 6.183 | 13.788 | 12.192 |
16 | 0.229 | 0.5657 | 182.883 | 1.218 | 3.381 | 5.930 | 13.526 | 11.430 |
17 | 0.229 | 0.5657 | 182.883 | 1.218 | 3.280 | 5.701 | 13.292 | 10.758 |
18 | 0.229 | 0.5657 | 182.883 | 1.218 | 3.187 | 5.494 | 13.079 | 10.160 |
19 | 0.229 | 0.5657 | 182.883 | 1.218 | 3.102 | 5.306 | 12.887 | 9.625 |
20 | 0.229 | 0.5657 | 182.883 | 1.218 | 3.024 | 5.134 | 12.710 | 9.144 |
21 | 0.229 | 0.5657 | 182.883 | 1.218 | 2.951 | 4.976 | 12.549 | 8.709 |
22 | 0.229 | 0.5657 | 182.883 | 1.218 | 2.883 | 4.830 | 12.400 | 8.313 |
23 | 0.229 | 0.5657 | 182.883 | 1.218 | 2.820 | 4.695 | 12.263 | 7.951 |
24 | 0.229 | 0.5657 | 182.883 | 1.218 | 2.760 | 4.569 | 12.136 | 7.620 |
25 | 0.229 | 0.5657 | 182.883 | 1.218 | 2.705 | 4.452 | 12.018 | 7.315 |
Based on the results of the Kagan-Rodda calculations, the existing rain station has a smoothing error (Z1) of 12.858% and an interpolation error (Z2) of 20.547%. However, if using WMO's standard for an urban area of recording equipment, the smoothing error (Z1) of 5.494% and the interpolation error (Z2) of 13.079% are more suitable. Moreover, generally, Z1 is less than 10% [27], however since Makassar city is an urban area and flood-prone area [3,4], so the value of 10% cannot anticipate changes in rainfall that endanger the community. Furthermore, if you want the Z1 value to be less than 5%, 21 rain gauges must be installed. However, the interpolation error (Z2) is still greater than 12%. Furthermore, in the same way, the optimal numbers of rain gauges for scenarios 2 to 4 were calculated, the results of which are shown in Table 5.
RG | The second scheme | The third scheme | The fourth scheme | |||||||||
N | R0 | d(0) | Z1 (%) | Z2 (%) | R0 | d(0) | Z1 (%) | Z2 (%) | R0 | d(0) | Z1 (%) | Z2 (%) |
1 | 0.99 | 10,000 | 2.09 | 1.33 | 0.99 | 333 | 3.11 | 3.54 | 0.91 | 26 | 10.45 | 11.97 |
2 | 0.99 | 10,000 | 1.47 | 1.26 | 0.99 | 333 | 2.03 | 2.66 | 0.91 | 26 | 6.75 | 8.92 |
3 | 0.99 | 10,000 | 1.20 | 1.23 | 0.99 | 333 | 1.59 | 2.29 | 0.91 | 26 | 5.26 | 7.63 |
4 | 0.99 | 10,000 | 1.03 | 1.22 | 0.99 | 333 | 1.34 | 2.08 | 0.91 | 26 | 4.42 | 6.90 |
5 | 0.99 | 10,000 | 0.92 | 1.21 | 0.99 | 333 | 1.18 | 1.95 | 0.91 | 26 | 3.87 | 6.42 |
6 | 0.99 | 10,000 | 0.84 | 1.21 | 0.99 | 333 | 1.06 | 1.85 | 0.91 | 26 | 3.48 | 6.08 |
7 | 0.99 | 10,000 | 0.78 | 1.20 | 0.99 | 333 | 0.97 | 1.78 | 0.91 | 26 | 3.18 | 5.83 |
8 | 0.99 | 10,000 | 0.73 | 1.20 | 0.99 | 333 | 0.90 | 1.72 | 0.91 | 26 | 2.94 | 5.63 |
9 | 0.99 | 10,000 | 0.69 | 1.20 | 0.99 | 333 | 0.84 | 1.68 | 0.91 | 26 | 2.75 | 5.47 |
10 | 0.99 | 10,000 | 0.64 | 1.17 | 0.99 | 333 | 0.80 | 1.64 | 0.91 | 26 | 2.59 | 5.34 |
Average and maximum rainfall data every month between stations greatly affects the optimal amount of rain gauges. The Kagan-Rodda calculations show that the stations in Makassar, currently totaling 5 locations, have a smoothing error (Z1) and interpolation error (Z2) of less than 5%. This small error rate indicates that the number of existing stations is sufficient to capture variations in rainfall based on months and series of rainfall data. Meanwhile, based on the monthly series rainfall data, the values of Z1 and Z2 are 3.874% and 6.424%, respectively. This means that the existing 5 stations in Makassar are sufficient if you use a smoothing error (Z1).
Compared with the first scheme, the maximum rainfall is calculated every year, where the value of a smoothing error (Z1) reaches 12.858%, and the interpolation error (Z2) is 20.547% for the 5 existing rain gauges. This error is very large when compared to the average scheme, the maximum in the same month, where the error value is around 2%. Meanwhile, based on the monthly series rainfall data, only the Z1 value is less than 3.874%, while the Z2 value is still 6.424%.
An illustration of the rain gauge placement can be drawn on a map according to the distance calculated using Eq 5. Based on this distance, it is then used to make an equilateral triangle so that one of the existing rain gauge locations is at the point of the triangle as a reference [27]. An example of using the Kagan-Rodda method is the application of L, with values of 6.048 km and 4.976 km, respectively, representing the 5 existing stations in the scenarios of maximum monthly rainfall each year and 18 locations following WMO criteria. The results of the mapping using the Kagan-Rodda technique can be seen in Figure 3.
The Kagan-Rodda calculations can be used to describe the design for deploying rain gauges. Based on Figure 3, the number of network node points represents the optimal rain gauge number in the part of the city located on Sulawesi Island., while considering the small islands that surround Makassar city, there should be an additional rain gauge on the island. Moreover, according to WMO regulations, small island areas should have a rain gauge at a closer distance. Based on the Kagan-Rodda network, at least 5 rain gauges need to be added to represent the area of the islands.
Makassar city has an area of 175 km2 and is located on the coast. If the coastal density standard is used, this area only requires 1 rain gauge. However, the city of Makassar is an urban area with a high population density, so it is considered to use a standard urban area recording device, where the rainfall gauge represents an area of 10–20 km2. Therefore, for an area of 175 km2, 9–18 rain gauges must be installed in that location. When compared between unrecorded and recorded rain gauges, the unrecorded area coverage is 1:10 compared to recorded rain gauges, or 1–2 km2 for rain gauges. These requirements are difficult to fulfill, especially for developing countries like Indonesia. This work uses the maximum WMO standard.
The very high correlation values of these various schemes indicate that there is almost no difference between the 5th rain gauge rainfall catchment compared if the number is reduced. If there are only 4 rain gauges where accuracy is calculated every month, then not using a rain gauge located in Sudiang will cause the most severe error, with high RMSE and MAE values as shown in Table 6. As a result, the Sudiang station should not be omitted in the measurement of rainfall in Makassar city, because it will reduce its accuracy significantly. Moreover, based on the accuracy of using schemes 2, 3, and 4, it can be seen that the accuracy values of the three are almost the same, which means that the rain gauges in Biringromang, Panaikang, and Paotere can replace each other. Interestingly, there are differences in characteristics when comparing the accumulation of rainfall in this city based on the existing rain gauges. In the monthly data series, the absence of one rain gauge in Biringromang, Panaikang, and Paotere results in an MAE value of 6%–9%, but on the monthly average or maximum monthly data in a year, the effect is smaller, with an MAE value of 3%–4%. However, this variation changes when comparing it with the RMSE value, where the absence of the Biringromang station will cause a very large error in maximum rainfall. Therefore, Biringromang is very important in anticipating extreme rainfall. Finally, subtracting 2 rain gauges from rainfall measurements in Makassar will cause an increase in rainfall capture data errors and should be avoided.
Indicator | Scheme 1 | Scheme 2 | Scheme 3 | Scheme 4 | Scheme 5 | |
Series | Corel | 0.97502 | 0.99837 | 0.99901 | 0.99872 | 0.98961 |
RMSE | 1032 | 230 | 180 | 207 | 669 | |
MAE | 22% | 9% | 6% | 8% | 18% | |
Average | Corel | 0.99919 | 0.99982 | 0.99990 | 0.99965 | 0.99934 |
RMSE | 1646 | 214 | 222 | 431 | 1441 | |
MAE | 15% | 3% | 3% | 3% | 15% | |
Maximum | Corel | 0.99905 | 0.99872 | 0.99966 | 0.99832 | 0.99585 |
RMSE | 898 | 798 | 440 | 1032 | 2259 | |
MAE | 5% | 4% | 3% | 4% | 9% |
A well-designed rain gauge network is needed in planning analysis of water demand in a catchment [1], and although the denser network produces higher accuracy, too much equipment will be expensive and can result in the redundancy of the rain gauges [2]. On the other hand, the lack of a network causes a decrease in the quality of rain data. In Indonesia, initially, the airport installed a rain gauge to support aviation, which resulted in consideration of the need for water resources management and disasters not being a major consideration. However, along with the increasing need for adequate water availability and anticipation of disasters, the existing rain gauge network in Indonesia, especially in Makassar, needs to be reviewed [22].
The resulting calculation of the optimal number of rain gauges in Makassar following WMO coastal recommendations showed that in this area there should be installed 9–18 equipment instances without considering the small islands. This number will increase if you use an adjustment to the non-recorded criteria, which is 1/10 the number of recorded rain gauges. The results of calculations using the coefficient of variation technique in research in the same place show a variation in the optimal number of rain gauges, although it is said that the 5 existing rain gauges correspond to an error level of 10% [11]. This variation is influenced by a combination of the number of locations and the error level. However, considering that Makassar is an urban area, in this study ε values of 5% and 10% were used in designing the rain station network. Variations also occur if the number of rain gauges is calculated based on Kagan-Rodda, where the maximum monthly rainfall scheme every year requires that the Makassar area still lacks a rain gauge, at least the same as the WMO recommendation with coastal criteria. However, if you use the average or maximum in each month, the number of rain gauges that exist is sufficient, even excessive. The optimal number of rain gauges will increase if you use the data series scheme, but the current number of stations of 5 can reach an error level of less than 5%. This calculation does not take into account the need for a rain gauge on small islands to the west of Makassar city.
Based on all aspects of determining the optimal amount of rain gauges, the greatest number of rain gauges is from using the maximum rainfall in each year, then the data series, and using the average and maximum every month, from January to December. The numerous rain gauges required to reach an error level of 5% indicate that the variation between stations in this area is very large, whereas a small number means that the area is sufficient, or there is an excess of rainfall gauges. This means that the event of maximum or extreme rainfall in one location in Makassar will not necessarily occur in another, especially between the Barombong and Sudiang areas, where the average distance from other locations is the farthest, which is more than 10 km. However, based on the calculation of the accuracy of rainfall catchments in Makassar, it seems that certain places must be in place to maintain the accuracy of rainfall measurements, such as Sudiang, which is a very important place that should not be omitted.
Asynchrony of the optimal number of rain gauge inter stations in the event of high or extreme rainfall can also be detected when calculating the correlation. Based on the calculation of the relationship between distance and correlation, the maximum rainfall has a very small average correlation compared to other schemes. The problem of interstation correlation, which is not always proportional to distance, does not only occur in Makassar. In general, the correlation coefficients have been found to decrease exponentially with the distance in almost all the cases in Bangladesh [14]. In addition to rainfall duration, depth, maximum intensity, and the period of presence and absence of rain affect the correlation. Moreover, a fraction of intravenous non-existent periods and location orientation affect correlation. the correlation also depends on location orientation [28]. The exponential is also considered suitable for modeling correlation and distance relationships [29,30]. However, in this work, the exponential relation between distance and correlation does not completely work, especially when using maximum rainfall each year. Measurement of rainfall using a manual rain gauge requires resources that are difficult to realize. Therefore, the shortage of rain gauges for densely populated and flood-prone areas such as Makassar can be met using the integration of various sources, such as device microphones or cameras and telecommunications wave networks [31,32]. Although the location of the rain gauge generally only considers the climatological conditions of rainfall, for dense urban areas, it is necessary to consider the calculation of daily or hourly data in choosing the location of the rain gauge.
Four rainfall schemes and two methods for calculating the optimal number of rain gauges were introduced in this research. By using 5 locations to measure rainfall for ten years and comparing previous studies, it can be concluded that the existing rain gauges are quite representative of this city. However, the events of maximum or extreme rainfall in one location in Makassar will not necessarily occur in another, especially in areas between the Barombong and Sudiang areas, where the average distance from other locations is the farthest, which is more than 10 km. Moreover, this amount is the same as the calculation using the Kagan-Rodda technique on the maximum monthly rainfall scheme every year with an error rate of 5%, and this calculation does not yet take into account the need for a rain gauge on small islands to the west of Makassar city. Although the correlation coefficients generally decrease exponentially with the distance in this work, the exponential relation between distance and correlation does not completely work, especially when using maximum rainfall each year.
Furthermore, based on the calculation of the accuracy of the rain catch in Makassar, eliminating the rain gauge in Sudiang will cause a very large error in the measurement of rainfall in this city. Meanwhile, the presence of the Biring Romang station also needs to be considered, although the impact is not as big as the absence of rain gauges in Sudiang. In addition, the loss of one of the stations, namely Paotere or Panaikang, does not have much impact, because these two locations can replace each other. Future research potential needs to considered the expansion of the research area. Several factors that can affect hydrometeorological conditions include hills located east of the city, differences in elevation, temporal resolution, and global atmospheric phenomena that affect the weather in Makassar. It is also necessary to take advantage of advances in rain observation information technology such as satellites or radar.
The authors wish to thank the referees for their careful reading of the paper and useful comments.
All authors declare no conflicts of interest in this paper.
[1] |
S. K. Adhikary, A. G. Yilmaz, N. Muttil (2015) Optimal design of rain gauge network in the Middle Yarra River catchment, Australia. Hydrol Process 29: 2582–2599. https://doi.org/10.1002/hyp.10389 doi: 10.1002/hyp.10389
![]() |
[2] | P. R. Ahuja (1960) Planning of precipitation network for water resources development in India. WMO flood control series 15: 106–112. |
[3] | A. M. Al-Abadi, A. H. D. Al-Aboodi (2014) Optimum rain-gauges network design of some cities in Iraq. J Babylon Uni/Eng Sci 22: 946–958. |
[4] |
R. D'Arrigo, R. Wilson (2008) Short Communication: El Niño and Indian Ocean influences on Indonesian drought: implications for forecasting rainfall and crop productivity. Int J Climatology 28: 611–616. https://doi.org/10.1002/joc.1654 doi: 10.1002/joc.1654
![]() |
[5] | J. D. Evans (1996) Straight forward statistics for the behavioral sciences, Brooks/Cole Pub Co Pacific Grove. |
[6] |
Giarno, M. P. Hadi, S. Suprayogi, et al. (2018) Distribution of accuracy of TRMM daily rainfall in Makassar Strait. Forum Geografi 32: 38–52. https://doi.org/10.23917/forgeo.v32i1.5774 doi: 10.23917/forgeo.v32i1.5774
![]() |
[7] | Giarno, Muflihah, Mujahiddin (2001) Determination of optimal rain gauge on the coastal region use coefficient variation: Case study in Makassar. J Civil Eng Forum 7: 121–132. |
[8] | P. Goovaerts (1997) Geostatistics for Natural Resources Evaluation. Oxford Univ Press, New York. |
[9] |
M. Hanel, P. Máca (2014) Spatial variability and interdependence of rain event characteristics in the Czech Republic. Hydrol Process 28: 2929–2944. https://doi.org/10.1002/hyp.9845 doi: 10.1002/hyp.9845
![]() |
[10] |
D. Harisuseno, E. Suhartono, D. M. Cipta (2020) Rainfall streamflow relationship using stepwise method as a basis for rationalization of rain gauge network Density. Int J Recent Tech Eng 8: 2277–3878. https://doi.org/10.35940/ijrte.E6617.018520 doi: 10.35940/ijrte.E6617.018520
![]() |
[11] | R. L. Kagan (1972) Planning the spatial distribution of hydrometeorological stations to meet an error criterion. In: Casebook on Hydrological Network Design Practice, III-1, WMO Publication 324. |
[12] |
A. K. Mishra, P. Coulibaly (2009) Developments in hydrometric network design: A review. Rev Geoph 47: 1–24. https://doi.org/10.1029/2007RG000243 doi: 10.1029/2007RG000243
![]() |
[13] | S. Karmakar, A. Rahman, S. M. Q. Hassan (2012) Variability of monsoon rainfall and its interstation correlation in Bangladesh. The J Noami 29: 33–54. |
[14] | A. D. Patel, M. B. Dholakia, D. P. Patel, et al. (2016) Analysis of optimum number of rain Gauge in Shetrunji River Basin, Gujarat - India. Int J Sci Tech & Eng 2: 380–384. |
[15] |
B. U. Ngene, J. C. Agunwamba, B. A. Nwachukwu (2018) The Challenges to Nigerian raingauge network improvement. Res J Env Earth Sci 7: 68–74. https://doi.org/10.19026/rjees.7.2205 doi: 10.19026/rjees.7.2205
![]() |
[16] |
M. Martono, T. Wardoyo (2017) Impacts of El Niño 2015 and the Indian Ocean Dipole 2016 on rainfall in the Pameungpeuk and Cilacap regions. Forum Geografi 31: 184–195. https://doi.org/10.23917/forgeo.v31i2.4170 doi: 10.23917/forgeo.v31i2.4170
![]() |
[17] | F. Y. Pramono, S. Suripin, W. Sulistya (2019) Rationalization of rain stations in the Ciliwung Cisadane river basin. Int J Eng Res Tech 12: 2957–2963. |
[18] | Supari, F. Tangang, E. Salimun, et al. (2012) ENSO modulation of seasonal rainfall and extremes in Indonesia. Climate Dynamics 2012: 1–22. |
[19] | H. M. Raghunath (2006) Hydrology: Principles, Analysis, and Design. New Age International Publishers, Second Edition. |
[20] |
Z. Sen, Z. Habib (2001) Monthly spatial rainfall correlation functions and interpretations for Turkey. Hydrology Sciences Journal 46: 525–535. https://doi.org/10.1080/02626660109492848 doi: 10.1080/02626660109492848
![]() |
[21] | M. R. Shaghaghian, M. J. Abedini (2013) Rain gauge network design using coupled geostatistical and multivariate technique. Scientia Iranica 20: 259–269. |
[22] |
H. B. Rycroft (1949) Random sampling of rainfall. J South African Forestry Assoc 18: 71–81. https://doi.org/10.1080/03759873.1949.9630653 doi: 10.1080/03759873.1949.9630653
![]() |
[23] | Akoglu, H., 2018, User's guide to correlation coefficients, Turkish Journal of Emergency Medicine, 18, 91–93 |
[24] |
H. S. Lee (2015) General rainfall patterns in Indonesia and the potential impacts of local seas on rainfall intensity. Water 7: 1750-1768. https://doi.org/10.3390/w7041751 doi: 10.3390/w7041751
![]() |
[25] | WMO (1972) Casebook on hydrological network design practice. WMO, 324. |
[26] | WMO (1994) Guide to hydrological practices: Data acquisition and processing, analysis, forecasting, and other applications. WMO, 168. |
[27] |
F. A. Sneva, L. D. Calvin (1978) An improved Thiessen grid for eastern Oregon: An interstation correlation study determining the effect of the distance, bearing, and elevation between stations upon the precipitation correlation coefficient. Agricultural Meteorology 9: 471–483. https://doi.org/10.1016/0002-1571(78)90044-4 doi: 10.1016/0002-1571(78)90044-4
![]() |
[28] |
H. C. Yeh, Y. C. Chen, C. Wei, et al. (2011) Entropy and kriging approach to rainfall network design. Paddy Water Env 9: 343–355. https://doi.org/10.1007/s10333-010-0247-x doi: 10.1007/s10333-010-0247-x
![]() |
[29] |
N. Sunusi, E. T. Herdiani (2017) Modeling of extreme rainfall recurrence time by using point process models. J Env Sci Tech 10: 320–324. https://doi.org/10.3923/jest.2017.320.324 doi: 10.3923/jest.2017.320.324
![]() |
[30] |
V. Svoboda, P. Maca, M. Hanel, et al. (2015) Spatial correlation structure of monthly rainfall at a mesoscale region of north-eastern Bohemia. Theor Appl Climatol 121: 359–375. https://doi.org/10.1007/s00704-014-1241-9 doi: 10.1007/s00704-014-1241-9
![]() |
[31] |
R. Avanzato, F. Beritelli (2020) An innovative acoustic rain gauge based on convolutional neural networks. Information 11: 1–16. https://doi.org/10.3390/info11040183 doi: 10.3390/info11040183
![]() |
[32] |
R. Avanzato, F. Beritelli (2020) Hydrogeological risk management in smart cities: A new approach to rainfall classification based on LTE cell selection parameters. IEEE Access 8: 137161–137173. https://doi.org/10.1109/ACCESS.2020.3011375. doi: 10.1109/ACCESS.2020.3011375
![]() |
1. | Nurtiti Sunusi, , Bias of automatic weather parameter measurement in monsoon area, a case study in Makassar Coast, 2023, 10, 2372-0352, 1, 10.3934/environsci.2023001 | |
2. | Maulana Putra, Mohammad Syamsu Rosid, Djati Handoko, High-Resolution Rainfall Estimation Using Ensemble Learning Techniques and Multisensor Data Integration, 2024, 24, 1424-8220, 5030, 10.3390/s24155030 |
Year | Paotere | Panaikang | Biringromang | Barombong | Sudiang |
2010 | 891 | 822 | 1,058 | 444 | 492 |
2011 | 858 | 779 | 1,008 | 569 | 656 |
2012 | 639 | 564 | 1,115 | 396 | 552 |
2013 | 982 | 1,035 | 736 | 772 | 354 |
2014 | 836 | 878 | 617 | 391 | 380 |
2015 | 962 | 1,041 | 1,065 | 468 | 438 |
2016 | 727 | 714 | 934 | 370 | 620 |
2017 | 932 | 1,026 | 862 | 422 | 1,285 |
2018 | 858 | 787 | 598 | 457 | 1,187 |
2019 | 637 | 578 | 983 | 346 | 1,204 |
Correlation | Paotere | Panaikang | Biringromang | Barombong | Sudiang |
Paotere | 1.000 | 0.948 | -0.308 | 0.637 | -0.222 |
Panaikang | 0.948 | 1.000 | -0.322 | 0.535 | -0.210 |
Biringromang | -0.308 | -0.322 | 1.000 | -0.212 | -0.154 |
Barombong | 0.637 | 0.535 | -0.212 | 1.000 | -0.377 |
Sudiang | -0.222 | -0.210 | -0.154 | -0.377 | 1.000 |
Correlation | Paotere | Panaikang | Biringromang | Barombong | Sudiang | |
Paotere | average | 1.000 | 0.997 | 0.985 | 0.985 | 0.984 |
max | 1.000 | 0.989 | 0.938 | 0.971 | 1.000 | |
series | 1.000 | 0.973 | 0.740 | 0.929 | 0.723 | |
Panaikang | average | 0.997 | 1.000 | 0.992 | 0.994 | 0.989 |
max | 0.989 | 1.000 | 0.953 | 0.978 | 0.989 | |
series | 0.973 | 1.000 | 0.719 | 0.927 | 0.718 | |
Biringromang | average | 0.985 | 0.992 | 1.000 | 0.992 | 0.996 |
max | 0.938 | 0.953 | 1.000 | 0.921 | 0.938 | |
series | 0.740 | 0.719 | 1.000 | 0.758 | 0.517 | |
Barombong | average | 0.985 | 0.994 | 0.992 | 1.000 | 0.991 |
max | 0.971 | 0.978 | 0.921 | 1.000 | 0.971 | |
series | 0.929 | 0.927 | 0.758 | 1.000 | 0.670 | |
Sudiang | ave | 0.984 | 0.989 | 0.996 | 0.991 | 1.000 |
max | 1.000 | 0.989 | 0.938 | 0.971 | 1.000 | |
series | 0.723 | 0.718 | 0.517 | 0.670 | 1.000 |
N | CV | R0 | A | d(0) | (A/N)1/2 | Z1 (%) | Z2 (%) | Density |
1 | 0.229 | 0.5657 | 182.883 | 1.218 | 13.523 | 39.584 | 42.293 | 182.883 |
2 | 0.229 | 0.5657 | 182.883 | 1.218 | 9.562 | 24.235 | 30.534 | 91.441 |
3 | 0.229 | 0.5657 | 182.883 | 1.218 | 7.808 | 18.266 | 25.433 | 60.961 |
4 | 0.229 | 0.5657 | 182.883 | 1.218 | 6.762 | 14.978 | 22.452 | 45.721 |
5 | 0.229 | 0.5657 | 182.883 | 1.218 | 6.048 | 12.858 | 20.457 | 36.577 |
6 | 0.229 | 0.5657 | 182.883 | 1.218 | 5.521 | 11.361 | 19.010 | 30.480 |
7 | 0.229 | 0.5657 | 182.883 | 1.218 | 5.111 | 10.239 | 17.905 | 26.126 |
8 | 0.229 | 0.5657 | 182.883 | 1.218 | 4.781 | 9.362 | 17.030 | 22.860 |
9 | 0.229 | 0.5657 | 182.883 | 1.218 | 4.508 | 8.655 | 16.316 | 20.320 |
10 | 0.229 | 0.5657 | 182.883 | 1.218 | 4.276 | 8.070 | 15.722 | 18.288 |
11 | 0.229 | 0.5657 | 182.883 | 1.218 | 4.077 | 7.577 | 15.219 | 16.626 |
12 | 0.229 | 0.5657 | 182.883 | 1.218 | 3.904 | 7.155 | 14.787 | 15.240 |
13 | 0.229 | 0.5657 | 182.883 | 1.218 | 3.751 | 6.789 | 14.411 | 14.068 |
14 | 0.229 | 0.5657 | 182.883 | 1.218 | 3.614 | 6.468 | 14.080 | 13.063 |
15 | 0.229 | 0.5657 | 182.883 | 1.218 | 3.492 | 6.183 | 13.788 | 12.192 |
16 | 0.229 | 0.5657 | 182.883 | 1.218 | 3.381 | 5.930 | 13.526 | 11.430 |
17 | 0.229 | 0.5657 | 182.883 | 1.218 | 3.280 | 5.701 | 13.292 | 10.758 |
18 | 0.229 | 0.5657 | 182.883 | 1.218 | 3.187 | 5.494 | 13.079 | 10.160 |
19 | 0.229 | 0.5657 | 182.883 | 1.218 | 3.102 | 5.306 | 12.887 | 9.625 |
20 | 0.229 | 0.5657 | 182.883 | 1.218 | 3.024 | 5.134 | 12.710 | 9.144 |
21 | 0.229 | 0.5657 | 182.883 | 1.218 | 2.951 | 4.976 | 12.549 | 8.709 |
22 | 0.229 | 0.5657 | 182.883 | 1.218 | 2.883 | 4.830 | 12.400 | 8.313 |
23 | 0.229 | 0.5657 | 182.883 | 1.218 | 2.820 | 4.695 | 12.263 | 7.951 |
24 | 0.229 | 0.5657 | 182.883 | 1.218 | 2.760 | 4.569 | 12.136 | 7.620 |
25 | 0.229 | 0.5657 | 182.883 | 1.218 | 2.705 | 4.452 | 12.018 | 7.315 |
RG | The second scheme | The third scheme | The fourth scheme | |||||||||
N | R0 | d(0) | Z1 (%) | Z2 (%) | R0 | d(0) | Z1 (%) | Z2 (%) | R0 | d(0) | Z1 (%) | Z2 (%) |
1 | 0.99 | 10,000 | 2.09 | 1.33 | 0.99 | 333 | 3.11 | 3.54 | 0.91 | 26 | 10.45 | 11.97 |
2 | 0.99 | 10,000 | 1.47 | 1.26 | 0.99 | 333 | 2.03 | 2.66 | 0.91 | 26 | 6.75 | 8.92 |
3 | 0.99 | 10,000 | 1.20 | 1.23 | 0.99 | 333 | 1.59 | 2.29 | 0.91 | 26 | 5.26 | 7.63 |
4 | 0.99 | 10,000 | 1.03 | 1.22 | 0.99 | 333 | 1.34 | 2.08 | 0.91 | 26 | 4.42 | 6.90 |
5 | 0.99 | 10,000 | 0.92 | 1.21 | 0.99 | 333 | 1.18 | 1.95 | 0.91 | 26 | 3.87 | 6.42 |
6 | 0.99 | 10,000 | 0.84 | 1.21 | 0.99 | 333 | 1.06 | 1.85 | 0.91 | 26 | 3.48 | 6.08 |
7 | 0.99 | 10,000 | 0.78 | 1.20 | 0.99 | 333 | 0.97 | 1.78 | 0.91 | 26 | 3.18 | 5.83 |
8 | 0.99 | 10,000 | 0.73 | 1.20 | 0.99 | 333 | 0.90 | 1.72 | 0.91 | 26 | 2.94 | 5.63 |
9 | 0.99 | 10,000 | 0.69 | 1.20 | 0.99 | 333 | 0.84 | 1.68 | 0.91 | 26 | 2.75 | 5.47 |
10 | 0.99 | 10,000 | 0.64 | 1.17 | 0.99 | 333 | 0.80 | 1.64 | 0.91 | 26 | 2.59 | 5.34 |
Indicator | Scheme 1 | Scheme 2 | Scheme 3 | Scheme 4 | Scheme 5 | |
Series | Corel | 0.97502 | 0.99837 | 0.99901 | 0.99872 | 0.98961 |
RMSE | 1032 | 230 | 180 | 207 | 669 | |
MAE | 22% | 9% | 6% | 8% | 18% | |
Average | Corel | 0.99919 | 0.99982 | 0.99990 | 0.99965 | 0.99934 |
RMSE | 1646 | 214 | 222 | 431 | 1441 | |
MAE | 15% | 3% | 3% | 3% | 15% | |
Maximum | Corel | 0.99905 | 0.99872 | 0.99966 | 0.99832 | 0.99585 |
RMSE | 898 | 798 | 440 | 1032 | 2259 | |
MAE | 5% | 4% | 3% | 4% | 9% |
Year | Paotere | Panaikang | Biringromang | Barombong | Sudiang |
2010 | 891 | 822 | 1,058 | 444 | 492 |
2011 | 858 | 779 | 1,008 | 569 | 656 |
2012 | 639 | 564 | 1,115 | 396 | 552 |
2013 | 982 | 1,035 | 736 | 772 | 354 |
2014 | 836 | 878 | 617 | 391 | 380 |
2015 | 962 | 1,041 | 1,065 | 468 | 438 |
2016 | 727 | 714 | 934 | 370 | 620 |
2017 | 932 | 1,026 | 862 | 422 | 1,285 |
2018 | 858 | 787 | 598 | 457 | 1,187 |
2019 | 637 | 578 | 983 | 346 | 1,204 |
Correlation | Paotere | Panaikang | Biringromang | Barombong | Sudiang |
Paotere | 1.000 | 0.948 | -0.308 | 0.637 | -0.222 |
Panaikang | 0.948 | 1.000 | -0.322 | 0.535 | -0.210 |
Biringromang | -0.308 | -0.322 | 1.000 | -0.212 | -0.154 |
Barombong | 0.637 | 0.535 | -0.212 | 1.000 | -0.377 |
Sudiang | -0.222 | -0.210 | -0.154 | -0.377 | 1.000 |
Correlation | Paotere | Panaikang | Biringromang | Barombong | Sudiang | |
Paotere | average | 1.000 | 0.997 | 0.985 | 0.985 | 0.984 |
max | 1.000 | 0.989 | 0.938 | 0.971 | 1.000 | |
series | 1.000 | 0.973 | 0.740 | 0.929 | 0.723 | |
Panaikang | average | 0.997 | 1.000 | 0.992 | 0.994 | 0.989 |
max | 0.989 | 1.000 | 0.953 | 0.978 | 0.989 | |
series | 0.973 | 1.000 | 0.719 | 0.927 | 0.718 | |
Biringromang | average | 0.985 | 0.992 | 1.000 | 0.992 | 0.996 |
max | 0.938 | 0.953 | 1.000 | 0.921 | 0.938 | |
series | 0.740 | 0.719 | 1.000 | 0.758 | 0.517 | |
Barombong | average | 0.985 | 0.994 | 0.992 | 1.000 | 0.991 |
max | 0.971 | 0.978 | 0.921 | 1.000 | 0.971 | |
series | 0.929 | 0.927 | 0.758 | 1.000 | 0.670 | |
Sudiang | ave | 0.984 | 0.989 | 0.996 | 0.991 | 1.000 |
max | 1.000 | 0.989 | 0.938 | 0.971 | 1.000 | |
series | 0.723 | 0.718 | 0.517 | 0.670 | 1.000 |
N | CV | R0 | A | d(0) | (A/N)1/2 | Z1 (%) | Z2 (%) | Density |
1 | 0.229 | 0.5657 | 182.883 | 1.218 | 13.523 | 39.584 | 42.293 | 182.883 |
2 | 0.229 | 0.5657 | 182.883 | 1.218 | 9.562 | 24.235 | 30.534 | 91.441 |
3 | 0.229 | 0.5657 | 182.883 | 1.218 | 7.808 | 18.266 | 25.433 | 60.961 |
4 | 0.229 | 0.5657 | 182.883 | 1.218 | 6.762 | 14.978 | 22.452 | 45.721 |
5 | 0.229 | 0.5657 | 182.883 | 1.218 | 6.048 | 12.858 | 20.457 | 36.577 |
6 | 0.229 | 0.5657 | 182.883 | 1.218 | 5.521 | 11.361 | 19.010 | 30.480 |
7 | 0.229 | 0.5657 | 182.883 | 1.218 | 5.111 | 10.239 | 17.905 | 26.126 |
8 | 0.229 | 0.5657 | 182.883 | 1.218 | 4.781 | 9.362 | 17.030 | 22.860 |
9 | 0.229 | 0.5657 | 182.883 | 1.218 | 4.508 | 8.655 | 16.316 | 20.320 |
10 | 0.229 | 0.5657 | 182.883 | 1.218 | 4.276 | 8.070 | 15.722 | 18.288 |
11 | 0.229 | 0.5657 | 182.883 | 1.218 | 4.077 | 7.577 | 15.219 | 16.626 |
12 | 0.229 | 0.5657 | 182.883 | 1.218 | 3.904 | 7.155 | 14.787 | 15.240 |
13 | 0.229 | 0.5657 | 182.883 | 1.218 | 3.751 | 6.789 | 14.411 | 14.068 |
14 | 0.229 | 0.5657 | 182.883 | 1.218 | 3.614 | 6.468 | 14.080 | 13.063 |
15 | 0.229 | 0.5657 | 182.883 | 1.218 | 3.492 | 6.183 | 13.788 | 12.192 |
16 | 0.229 | 0.5657 | 182.883 | 1.218 | 3.381 | 5.930 | 13.526 | 11.430 |
17 | 0.229 | 0.5657 | 182.883 | 1.218 | 3.280 | 5.701 | 13.292 | 10.758 |
18 | 0.229 | 0.5657 | 182.883 | 1.218 | 3.187 | 5.494 | 13.079 | 10.160 |
19 | 0.229 | 0.5657 | 182.883 | 1.218 | 3.102 | 5.306 | 12.887 | 9.625 |
20 | 0.229 | 0.5657 | 182.883 | 1.218 | 3.024 | 5.134 | 12.710 | 9.144 |
21 | 0.229 | 0.5657 | 182.883 | 1.218 | 2.951 | 4.976 | 12.549 | 8.709 |
22 | 0.229 | 0.5657 | 182.883 | 1.218 | 2.883 | 4.830 | 12.400 | 8.313 |
23 | 0.229 | 0.5657 | 182.883 | 1.218 | 2.820 | 4.695 | 12.263 | 7.951 |
24 | 0.229 | 0.5657 | 182.883 | 1.218 | 2.760 | 4.569 | 12.136 | 7.620 |
25 | 0.229 | 0.5657 | 182.883 | 1.218 | 2.705 | 4.452 | 12.018 | 7.315 |
RG | The second scheme | The third scheme | The fourth scheme | |||||||||
N | R0 | d(0) | Z1 (%) | Z2 (%) | R0 | d(0) | Z1 (%) | Z2 (%) | R0 | d(0) | Z1 (%) | Z2 (%) |
1 | 0.99 | 10,000 | 2.09 | 1.33 | 0.99 | 333 | 3.11 | 3.54 | 0.91 | 26 | 10.45 | 11.97 |
2 | 0.99 | 10,000 | 1.47 | 1.26 | 0.99 | 333 | 2.03 | 2.66 | 0.91 | 26 | 6.75 | 8.92 |
3 | 0.99 | 10,000 | 1.20 | 1.23 | 0.99 | 333 | 1.59 | 2.29 | 0.91 | 26 | 5.26 | 7.63 |
4 | 0.99 | 10,000 | 1.03 | 1.22 | 0.99 | 333 | 1.34 | 2.08 | 0.91 | 26 | 4.42 | 6.90 |
5 | 0.99 | 10,000 | 0.92 | 1.21 | 0.99 | 333 | 1.18 | 1.95 | 0.91 | 26 | 3.87 | 6.42 |
6 | 0.99 | 10,000 | 0.84 | 1.21 | 0.99 | 333 | 1.06 | 1.85 | 0.91 | 26 | 3.48 | 6.08 |
7 | 0.99 | 10,000 | 0.78 | 1.20 | 0.99 | 333 | 0.97 | 1.78 | 0.91 | 26 | 3.18 | 5.83 |
8 | 0.99 | 10,000 | 0.73 | 1.20 | 0.99 | 333 | 0.90 | 1.72 | 0.91 | 26 | 2.94 | 5.63 |
9 | 0.99 | 10,000 | 0.69 | 1.20 | 0.99 | 333 | 0.84 | 1.68 | 0.91 | 26 | 2.75 | 5.47 |
10 | 0.99 | 10,000 | 0.64 | 1.17 | 0.99 | 333 | 0.80 | 1.64 | 0.91 | 26 | 2.59 | 5.34 |
Indicator | Scheme 1 | Scheme 2 | Scheme 3 | Scheme 4 | Scheme 5 | |
Series | Corel | 0.97502 | 0.99837 | 0.99901 | 0.99872 | 0.98961 |
RMSE | 1032 | 230 | 180 | 207 | 669 | |
MAE | 22% | 9% | 6% | 8% | 18% | |
Average | Corel | 0.99919 | 0.99982 | 0.99990 | 0.99965 | 0.99934 |
RMSE | 1646 | 214 | 222 | 431 | 1441 | |
MAE | 15% | 3% | 3% | 3% | 15% | |
Maximum | Corel | 0.99905 | 0.99872 | 0.99966 | 0.99832 | 0.99585 |
RMSE | 898 | 798 | 440 | 1032 | 2259 | |
MAE | 5% | 4% | 3% | 4% | 9% |