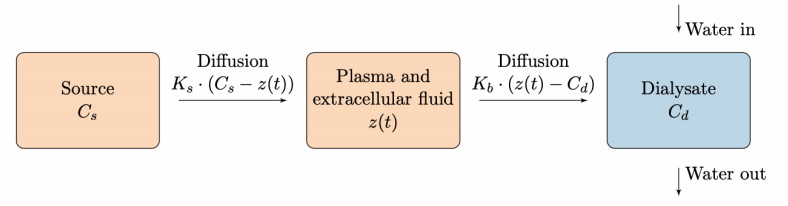
In this note, we approximate the von Neumann and Rényi entropies of high-dimensional graphs using the Euler-Maclaurin summation formula. The obtained estimations have a considerable degree of accuracy. The performed experiments suggest some entropy problems concerning graphs whose Laplacians are g-circulant matrices, i.e., circulant matrices with g-periodic diagonals, or quasi-Toeplitz matrices. Quasi means that in a Toeplitz matrix the first two elements in the main diagonal, and the last two, differ from the remaining diagonal entries by a perturbation.
Citation: Natália Bebiano, João da Providência, Wei-Ru Xu. Approximations for the von Neumann and Rényi entropies of graphs with circulant type Laplacians[J]. Electronic Research Archive, 2022, 30(5): 1864-1880. doi: 10.3934/era.2022094
[1] | Meixin Xiong, Liuhong Chen, Ju Ming, Jaemin Shin . Accelerating the Bayesian inference of inverse problems by using data-driven compressive sensing method based on proper orthogonal decomposition. Electronic Research Archive, 2021, 29(5): 3383-3403. doi: 10.3934/era.2021044 |
[2] | Yiyuan Qian, Haiming Song, Xiaoshen Wang, Kai Zhang . Primal-dual active-set method for solving the unilateral pricing problem of American better-of options on two assets. Electronic Research Archive, 2022, 30(1): 90-115. doi: 10.3934/era.2022005 |
[3] | Yu Xue, Zhenman Zhang, Ferrante Neri . Similarity surrogate-assisted evolutionary neural architecture search with dual encoding strategy. Electronic Research Archive, 2024, 32(2): 1017-1043. doi: 10.3934/era.2024050 |
[4] | Ziqing Yang, Ruiping Niu, Miaomiao Chen, Hongen Jia, Shengli Li . Adaptive fractional physical information neural network based on PQI scheme for solving time-fractional partial differential equations. Electronic Research Archive, 2024, 32(4): 2699-2727. doi: 10.3934/era.2024122 |
[5] | Showkat Ahmad Lone, Hanieh Panahi, Sadia Anwar, Sana Shahab . Estimations and optimal censoring schemes for the unified progressive hybrid gamma-mixed Rayleigh distribution. Electronic Research Archive, 2023, 31(8): 4729-4752. doi: 10.3934/era.2023242 |
[6] | Yao Sun, Lijuan He, Bo Chen . Application of neural networks to inverse elastic scattering problems with near-field measurements. Electronic Research Archive, 2023, 31(11): 7000-7020. doi: 10.3934/era.2023355 |
[7] | Zhiyuan Feng, Kai Qi, Bin Shi, Hao Mei, Qinghua Zheng, Hua Wei . Deep evidential learning in diffusion convolutional recurrent neural network. Electronic Research Archive, 2023, 31(4): 2252-2264. doi: 10.3934/era.2023115 |
[8] | Juan Li, Geng Sun . A rational resource allocation method for multimedia network teaching reform based on Bayesian partition data mining. Electronic Research Archive, 2023, 31(10): 5959-5975. doi: 10.3934/era.2023303 |
[9] | Ruyang Yin, Jiping Xing, Pengli Mo, Nan Zheng, Zhiyuan Liu . BO-B&B: A hybrid algorithm based on Bayesian optimization and branch-and-bound for discrete network design problems. Electronic Research Archive, 2022, 30(11): 3993-4014. doi: 10.3934/era.2022203 |
[10] | Bingjie Zhang, Junchao Yu, Zhe Kang, Tianyu Wei, Xiaoyu Liu, Suhua Wang . An adaptive preference retention collaborative filtering algorithm based on graph convolutional method. Electronic Research Archive, 2023, 31(2): 793-811. doi: 10.3934/era.2023040 |
In this note, we approximate the von Neumann and Rényi entropies of high-dimensional graphs using the Euler-Maclaurin summation formula. The obtained estimations have a considerable degree of accuracy. The performed experiments suggest some entropy problems concerning graphs whose Laplacians are g-circulant matrices, i.e., circulant matrices with g-periodic diagonals, or quasi-Toeplitz matrices. Quasi means that in a Toeplitz matrix the first two elements in the main diagonal, and the last two, differ from the remaining diagonal entries by a perturbation.
Phosphate enables the body to perform vital processes such as construction of nucleic acids, energy transport and bone tissue formation [1]. The level of phosphate is tightly controlled, and excess phosphate is excreted by the kidneys [2]. However, for patients with renal failure, the control of phosphate homeostasis is impaired. An abnormal level of phosphate is associated with increased vascular calcification and mortality [3,4].
About half of all dialysis patients suffer from hyperphosphataemia, and strategies to control phosphate levels include phosphate binders, low-phosphate diet and removal of phosphate by hemodialysis [5]. Hemodialysis (HD) is a conventional treatment for renal failure where a patient is coupled to a dialysis machine for four to eight hours. The blood plasma and dialysate fluid are passed through a filter that causes a diffusion process that removes toxic substances, e.g., phosphate, from the blood to the dialysate. The phosphate kinetics in HD is of particular interest because it differs the other removed toxins, e.g., urea, by the fact that hypophoshataemia is fatal for the patient [6]. Thus, the phosphate concentration should not be exhausted, but kept within the critical values.
The control of the phosphate concentration is a considerable clinical problem and has been studied extensively; The conventional hemodialysis treatment is the single-pass (SP) treatment. Agar et al. [7] and Debowska et al. [3] both study the SP treatment by considering a simple two-compartment ordinary differential equation (ODE) model for phosphate removal during HD. They present their results as an average of the measured patients to obtain confidence intervals for their parameters, however, these are not patient specific. Poleszczuk et al. [2] extend the model proposed by Debowska et al. [3] to include a time delay. The time delay is introduced to improve the fit at the later stage of the HD where a minor rebound is observed in some clinical experiments. Andersen et al. [8] analyze the same model analytically and estimate parameters using an optimization-driven approach. Here the parameters are estimated for each patient, but the uncertainty of the parameter estimates is not addressed. Laursen et al. [9,10] propose a two- and three-compartment model for phosphate clearance during SP and find that the three-compartment model produces the most satisfying fit but does not address the uncertainty associated with the parameter estimates. Spalding et al. [11] propose a complicated four-compartment model where the fourth pool is a control pool for avoiding dangerously low phosphate concentrations. They argue that a simple two-compartment model cannot fit the relapse phase sufficiently. The relapse phase refers to the period after ended treatment where the phosphate concentration starts to increase. However, both Andersen et al. [8] and Debowska et al. [3] demonstrate that the simple two-compartment model can produce adequate fits for the relapse phase as well. A novel HD treatment called multiple pass (MP) [12,13,14] provides an alternative to the conventional SP. This novel treatment reduces the amount of dialysis fluid needed for a single session of HD. Andersen et al. [8] and Heaf et al. [14] analyse and compare the MP treatment and SP treatment.
However, none of the above-listed models address patient specific uncertainties associated with the parameter estimates. Moreover, the reported uncertainty of the parameter estimates for the average of the measured patients is very large, e.g., Debowska et al. [3] report a phosphate clearance with a relative standard deviation of 79% and Agar et al. [7] report a relative standard deviation of 47%, indicating that parameters of the two-compartment model are poorly identified. Common for all models is that they assume that the phosphate concentration in the inner-source compartment is known exactly through measurements at time zero. However, measurements are noisy and can potentially bias the results.
The Bayesian approach for parameter estimation for ODE modeling has gained attention in later years [15,16] since it provides an elegant way of addressing the uncertainty associated with the estimated parameters and includes clinical knowledge. The Bayesian approach gives a complete image of the parameter estimation in terms of uncertainty quantification, i.e., posterior mean, credibility intervals and correlations. A Bayesian approach for patient-specific parameters for hemodialysis has been proposed by Bianchi et al. [17] but does not consider the phosphate kinetics.
We propose a Bayesian approach for estimating patient-specific parameters for phosphate dynamics during hemodialysis. Moreover, we include the phosphate concentration in the inner compartment as a parameter of the model. We use uncertainty quantification to assess the reliability of our parameter estimates and explore the full parameter space. We address the identifiability of the parameters for the SP, MP and the combination of the two, denoted combined-pass (CP). While CP has limited direct clinical impact it improves the parameter estimation slightly, since both SP and MP data are considered simultaneously. In addition, we also investigate how the parameter estimation can be improved by including relapse measurements and / or measure consecutive sessions.
Section 2 describes the phosphate kinetics during hemodialysis and introduces the single- and multiple-pass treatments. Section 3 introduces the Bayesian model and describes implementation and sampling diagnostics. In Section 4, we test and validate SP, MP and CP models on data sets and discuss findings from synthetic data which are found in the supplementary materials. Lastly, we conclude the paper in Section 5.
About 85% of the total phosphate in the human body is stored in the bones [18]. We assume that we have an inexhaustible source (bone) that excretes phosphate to the blood, including extracellular fluid. The phosphate transport from source to blood is driven by diffusion. The diffusion process is governed by the diffusion coefficient (permeability) and concentration gradient. The blood compartment is coupled to the dialysate compartment through a semipermeable membrane which generates a flow of phosphate to the dialysate fluid. The flow of phosphate from blood to dialysate is mainly governed by diffusion and to an insignificant degree by a convection process. [9] However, comprehensive investigations have shown that the convective flow has a negligible effect on the model and parameters during the normal range of dialysis treatment, i.e., up to eight hours [8]. Thus, we exclude the convection term from the models. In this paper, we consider three types of models for HD for phosphate clearance in dialysis patients, the conventional SP, MP and the combination CP.
The value of this analysis for clinicians is twofold. Firstly, accurate modeling permits the prediction of phosphate removal during different forms of dialysis, e.g., short and long dialysis or use of filters with standard or high phosphate clearances. Secondly, it is possible to get insight into the underlying physiological causes of phosphate dynamics.
The model parameters are individually calibrated, so the parameter values are patient specific signatures. Hence, reliable estimates for these parameters are clinically important in order to assess the phosphate kinetics.
For the SP treatment, the dialysate is constantly replenished by fresh dialysate such that the phosphate concentration in the outflowing dialysate remains low. Data shows this phosphate concentration to be approximately constant throughout the treatment. SP requires excessive amounts of dialysate for each session. A conceptual diagram of the SP treatment is depicted in Figure 1 that illustrates the removal of phosphate by diffusion.
Agar et al. [7] proposed a simple compartment model for SP consisting of a single linear autonomous ODE,
Vbdz(t)dt=CsKs−(Ks+Kb)z(t)+KbCd, | (2.1) |
where z(t) is the concentration of phosphate in the blood compartment at time t, Cs is the constant concentration in the source compartment and Cd is the phosphate concentration in the dialysate assumed to be constant and measurable. Ks and Kb are diffusion rates from source to blood and from blood to dialysate, respectively. Lastly, Vb denotes the blood volume taken as the blood plasma and extracellular volume. For the system to have a unique solution, we equip the ODE with the initial condition z(0)=z0. Notice that the system is not identifiable since Vb can be integrated in the remaining parameters and thus we assume that Vb is known through measurements for SP.
The assumption of a constant Cd is not crucial. If we allow the phosphate concentration to be a variable with initial value 0, then we can extend the model by an extra differential equation. This extension results in a fast transient in Cd toward the steady state value given by data shown in Table 1 with at doubling time of approximately 10-15 minutes (see supplementary, Figure E.14). Moreover, such extension does not affect the parameter estimates achieved. Hence we confine ourselves to consider Cd as a constant.
Estimate | Patient | |||||||||||
1 | 2 | 3 | 4 | 5 | 6 | 7 | 8 | 9 | 10 | |||
SP | Cd [mmol/L] | 0.16 | 0.12 | 0.11 | 0.16 | 0.18 | 0.21 | 0.14 | 0.09 | 0.14 | 0.09 | |
Vb [L] | 16.88 | 17.74 | 16.92 | 21.20 | 18.20 | 14.74 | 15.32 | 13.04 | 20.20 | 18.00 | ||
MP | Vb [L] | 16.99 | 17.80 | 17.43 | 21.20 | 18.39 | 15.15 | 15.48 | 13.76 | 20.21 | 18.25 | |
Vd [L] | 22.61 | 23.00 | 26.57 | 31.93 | 28.51 | 20.15 | 23.42 | 14.24 | 28.59 | 23.25 |
Contrary to SP where dialysate is constantly replenished, the dialysate for MP is recirculated, and consequently, the removed substances accumulate in the dialysate fluid over time. A conceptual diagram of the MP treatment is depicted in Figure 2.
MP is less effective than SP due to the accumulation of substances in dialysate. However, MP greatly reduces the amount of dialysate fluid needed for HD treatment, which makes a smaller clinical setting possible. Furthermore, it may ease HD treatment at home and treatment during travels, which can possibly greatly improve the quality of life for renal failure patients. [12,13,14]
The MP model can be described by the following system of linear autonomous ODEs,
Vbdx(t)dt=CsKs−(Ks+Kb)x(t)+Kby(t), | (2.2a) |
Vddy(t)dt=Kb(x(t)−y(t)), | (2.2b) |
where x(t) and y(t) are the time-varying phosphate concentrations for the blood compartment and in the dialysate at time t, respectively, and Vd is the volume of the dialysate. The remaining parameters, i.e., Vb,Cs,Ks and Kb, have the same interpretation as for the SP model in (2.1). The initial conditions are x(0)=x0 and y(0)=y0 corresponding to the phosphate concentration in blood and dialysate at time t=0, respectively. The phosphate concentration in the dialysate at time t=0 is zero, i.e., we assume y0=0 henceforth.
The MP model carries additional information compared to the SP model since the only new parameter, the dialysate volume Vd is accurately known from the dialysis equipment. Hence, we have an additional equation in the model but the same number of unknown parameters compared to SP. Thus, given sufficient data, the MP model allows for structural identifiability of the parameters due to the addition of (2.2b) since we cannot simply integrate Vb in the remaining parameters. However, since bioimpedance measurements of Vb are available, we will consider Vb to be known a priori, since it is not practically identifiable.
The parameters for a single patient are shared for the two treatments. Thus, if a patient completes both SP and MP, we can utilize all available information by considering the CP model,
Vbdz(t)dt=CsKs−(Ks+Kb)z(t)+KbCd, | (2.3a) |
Vbdx(t)dt=CsKs−(Ks+Kb)x(t)+Kby(t), | (2.3b) |
Vddy(t)dt=Kb(x(t)−y(t)), | (2.3c) |
with z(0)=z0, x(0)=x0 and y(0)=0, and the parameters as described for SP. The CP model, just as the MP model, allows for structural identifiability, and potentially even more precise estimation compared to the MP model due to the addition of the SP model.
We consider longitudinal data sets from 10 patients with renal failure that were measured during an SP session and an MP session. The measured phosphate concentrations for SP and MP (Z,X and Y) are depicted in Figure 3. Measurements were once every hour for a total of four and eight hours for SP and MP, respectively. No measurements were taken in the relapse phase, i.e., after ended treatment.
Considering the SP measurements (orange dotted line) in Figure 3, we see an exponential-like decay in the measured phosphate concentration after two hours as predicted by (2.1). Thereafter, phosphate concentration seems to stabilize around a reduced concentration level. For the MP measurements (green and blue dotted lines), we see similar exponential-like decay for the phosphate concentration in agreement with the bi-exponential solution to (2.2). However, this drop in phosphate concentration is a bit slower for some patients and after two hours it starts to slowly increase due to the accumulation of phosphate in the dialysate. The phosphate concentration in the dialysate increases rapidly in the beginning of the treatment but slows down and approaches an equilibrium with the phosphate concentration in the blood. This behavior is expected according to the model in (2.2) since the concentration gradient vanishes.
We solve the parameter estimation problem using a Bayesian approach, where we consider the parameters, measurement noise and initial conditions as random variables. In Bayesian inference, we are interested in the posterior probability of the parameters. The posterior probability consists of two components: a prior probability reflecting our knowledge or beliefs about likely parameter values, and a likelihood function that expresses how likely it is to observe the data for a set of parameters. Thus, the posterior allows us to formally include clinical prior knowledge in the model. Moreover, the inclusion of the prior may have a regularizing effect on the parameter estimation problem in the sense that the parameter estimates become less sensitive to measurement noise.
We use uncertainty quantification to assess the reliability of the parameter estimates and the concentrations in terms of posterior statistics, i.e., mean, correlation and 95% credibility intervals (CI). A strength of the uncertainty quantification is that the solution is based on all probable outcomes instead of being solely based on a point estimate [19]. Uncertainty quantification can also be used for model analysis and improvement, e.g., revealing strong correlation or identifying potential measurements that could improve identifiability of the model [20]. Hence, uncertainty quantification is a flexible method to assess how certain we are of the parameter values and parameter-dependent solutions
We describe the Bayesian model for the SP and MP treatments and presume data for the relevant state variables (phosphate concentrations) are measured. Notice that the Bayesian formulation is trivially extended to CP by combining the SP and MP models.
First, we consider the Bayesian formulation for SP. Let θ=[Cs,Ks,Kb] denote the vector of unknown parameters and zIC denote the initial condition for SP. We assume that Vb and Cd are known to a sufficient degree a priori and do not estimate them based on the model. A justification of this assumption is given in Section 4.
The state variable z(t,θ,zIC) is the solution to (2.1) and we wish to infer the model parameters θ and initial condition zIC defining the state variable. Henceforth, we shorten notation such that z(t)≡z(t,θ,zIC).
We assume that the measurement noise is normally distributed such that the state variable, z(t) is inferred through the Gaussian likelihood function,
Zi∼N(z(ti),σ2d),fori=1,2,...,m, | (3.1) |
where Z∈Rm is a vector with the measurement of the phosphate concentration in the blood at time t=ti. The parameter σ2d∈R+ is a hyperparameter describing the variance of the measurement noise. The hyperparameter σ2d is not known a priori. Thus, we infer σ2d as a parameter of the model and assign an inverse gamma prior [19]. We enforce non-negativity on the likelihood function by truncating it at 0 since the phosphate concentrations are non-negative.
We consider the initial condition zIC to have mean equal to the phosphate concentration at time t=0 and variance σ2d equal to the measurement error, i.e.,
zIC∼N(Z0,σ2d). | (3.2) |
This choice of prior for the initial condition can be interpreted as the initial measurement following the same measurement model as the measurements for time t>0, i.e., we do not assume that the first measurement is more accurately measured than the subsequent ones.
We model the prior of the unknown parameters θ by the Gaussian distribution,
θ∼N([μCsμKsμKb],[σ2Cs000σ2Ks000σ2Kb]), | (3.3) |
where μCs,μKs and μKb represent the prior clinical knowledge, i.e., our prior belief about most likely parameter values and σ2Cs, σ2Ks and σ2Kb are the variances for Cs, Ks and Kb, respectively. As with the likelihood function, we impose constraints such that we only consider the parameters in a physiologically meaningful range.
As commonly done, we assume that at the start of the dialysis, i.e., t=0, the patient's phosphate concentration is approximately in a steady state, i.e., we assume that Z0 is close to Cs and we choose μCs=Z0. The steady state assumption follows from (2.1) where Kb=0 when the patient is not receiving dialysis treatment.
In previous publications [3,7,8] Cs is fixed to the value of the initial phosphate measurement. However, the data from Agar et al. [7] show large uncertainty for the first measurement point. Our choice of prior allows Cs to deviate from the initial measurement of the phosphate concentration and thereby our model is not oblivious to measurement errors for the initial phosphate measurement.
We base our values for μKs and μKb on literature and we choose μKs=8.06 L/hour and μKb=7.56 L/hour [3].
We initially considered σ2Cs, σ2Ks and σ2Kb to be parameters of the model. However, preliminary results showed that it greatly decreased the stability of the results. Thus, we choose σ2Cs=0.2, σ2Ks=2.0 and σ2Kb=2.0 based on visual inspection of the prior to incorporate adequate uncertainty about the prior mean. Modest increase of the prior variances did not lead to change in conclusions, see subsection E.2 and Table E.4. Thus, the chosen prior variances are robust with respect to the results and not sensitive to the prior assumptions.
The main difference between the MP formulation and the SP formulation is the inclusion of an additional state variable through equation (2.2b). Hence, the likelihood function for MP is
[XiYi]∼N([x(ti)y(ti)],σ2dI),fori=1,2,...,n, | (3.4) |
where X∈Rn and Y∈Rn are vectors with the measurements of the phosphate concentration in the blood and dialysate at time t=ti, respectively, and I is the 2×2 identity matrix. The initial condition for the phosphate concentration in the dialysate is set to zero, i.e., yIC=0, and the initial condition for the phosphate concentration in the blood is assigned a prior with mean X0 and variance equal to the measurement variance, i.e.,
xIC∼N(X0,σ2d). | (3.5) |
Lastly, we choose the prior for the parameters θ to be (3.3) with the exception that μCs=X0.
We use sampling-based techniques to approximate the posterior [20]. Markov Chain Monte Carlo (MCMC) is a sampling technique that generates a Markov chain of samples that converges to the posterior distribution of the parameters [21]. Hence, we can compute posterior statistics, i.e., mean, 95% CI and correlation from the Markov chain.
The simple MCMC techniques such as random walk Metropolis Hastings and the Gibbs sampler are plagued by inefficient exploration of the parameter space via random walks and are highly sensitive to correlated parameters. Hamiltonian Monte Carlo (HMC) is an MCMC method that avoids random walk behavior by taking a series of first-order gradient informed steps in the simulation and explores the parameter space well even in the case of correlated parameters. The performance of the HMC sampler is highly sensitive to the choice of user-specified parameters. However, the No-U-Turn Sampler (NUTS) is an HMC method where the user-specified parameters are automatically estimated. [22] We use Runge-Kutta 45 (RK45) to solve the ODE system [23] and the PySTAN implementation of NUTS [24] with default choice for all associated parameters to compute the samples that approximate the posterior distribution.
For each simulation, we generate four sample chains from random initializations, and we consider the potential scale reduction statistic, the so-called ˆR value for sampling diagnostics [25]. The ˆR value measures the ratio of the average variance of samples within each chain to the variance of the pooled samples across chains, and if all chains are at equilibrium, then the ˆR value will be one.
In this section, we consider two data sets for dialysis patients during hemodialysis. For each patient, we generate 4000 samples and visualize the results in terms of posterior mean and 95% CIs for the estimated parameters and phosphate concentrations during and after hemodialysis. In addition, we also visualize the pairwise correlation for the parameters by scatter plots of the samples and compute the relative standard deviation. All presented results returned an ˆR value of one, indicating convergence of the sample chains. In addition, we visually inspected the sample chains, which appeared well mixed. Tables with estimated posterior means, 95% CIs and relative standard deviations are found in Appendix B, and RMSE is listed in Table 2. The assumptions of normal distributed measurement errors are investigated by the empirical error distributions and QQ-plots which are shown in Appendix D, Figure D.1 and Figure D.2. We may not reject the hypothesis about normal distributed measurement errors based on the investigations.
Patient | SP | MP | CP | |||
1 | 0.29 | 0.08 | 0.1 | |||
2 | 0.17 | 0.04 | 0.05 | |||
3 | 0.11 | 0.06 | 0.08 | |||
4 | 0.32 | 0.06 | 0.09 | |||
5 | 0.26 | 0.02 | 0.04 | |||
6 | 0.59 | 0.07 | 0.1 | |||
7 | 0.27 | 0.08 | 0.12 | |||
8 | 0.16 | 0.08 | 0.11 | |||
9 | 0.19 | 0.06 | 0.08 | |||
10 | 0.1 | 0.03 | 0.04 |
We have also investigated the models using synthetic data to confirm the findings of the results with real data. These synthetic experiments can be found in the supplementary. Here we present the results obtained by the Bayesian model described in Section 3 for the data depicted in Figure 3.
First, we consider the hemodialysis data for the ten patients shown in Figure 3. Beside phosphate concentrations in the blood and dialysate depicted, we have hourly measurements of the phosphate concentration in the dialysate (Cd) for SP, the volume of the blood compartment (Vb) for both SP and MP, and the dialysate volume (Vd) for MP. Cd was measured when exiting the dialysate compartment after initializing the dialysis process. We assume that the concentration of phosphate in the dialysis for SP is constant as suggested by data, and for each patient, we compute Cd as the spatial average of the concentration of phosphate from inlet to outlet of the dialysis machine. Table 1 lists Cd, Vd and Vb estimated directly from available data and Figure A.1 and Figure A.2 in Appendix A provide exploratory statistics of the corresponding data.
The estimated phosphate concentrations obtained for SP are depicted in Figure 4 along with the predicted relapse. The solid line represents the posterior mean, the full circles are data points and the transparent region indicates the 95% CI i.e., the region that contains 95% of the samples. Considering the estimated phosphate concentrations for SP, we see that the sampler has computed a decent fit in terms RMSE in Table 2 and posterior mean with a narrow 95% CI for the treatment phase. However, there is a large 95% CI for the relapse phase.
The corresponding parameter estimates with 95% CI for SP are visualized in Figure 5 and listed in Table B.1 where the average relative standard deviation is 10.3%, 18.4% and 18.6% for Cs, Ks and Kb, respectively. The full posterior density for the parameters for patient 2 is shown in Figure 6. We have chosen to only include a correlation plot for patient 2 in this section since it shows the general trend of the estimated parameters. The correlation plots for the remaining patients are found in Figure C.1-C.9 in Appendix C.
Figure 7 shows the estimated phosphate concentrations and predicted relapse phase for MP. Figure 7 and Table 2 show that the parameter estimation has found a satisfying fit both visually and in terms of RMSE for MP as for SP. However, the width of the 95% CIs is smaller for the relapse phase. The reduced uncertainty in the relapse can be explained by the reduced 95% CI for Cs in MP compared to SP which is shown in Figure 5 and Figure 6 and quantified by the decreased average standard deviation of 7.3% in Table B.2, i.e., a reduction of 3%.
Moreover, Figure 5 shows a great reduction in the uncertainty about Kb as expected from the addition of equation (2.2b) with a relative standard deviation of 9.6%, i.e., a reduction of 9% compared to SP. However, the uncertainty about Ks remains largely unaffected by the additional knowledge utilized by the MP model and the uncertainty actually increases on average with an average relative standard deviation of 24.8%.
Considering the CP results in Figure 8, we see that CP finds a unified set of parameters that describe the SP and MP sessions for each patient. Moreover, the CP estimates a satisfying fit both visually and in terms of RMSE in Table 2. The parameter estimates are very similar to the ones obtained by MP as seen in Figure 5 except for patient 6 and with only a slight reduction compared to MP in average relative standard deviation, 6.9%, 22.9% and 8.2% for Cs, Ks and Kb, respectively. A possible explanation for the difference in Ks for patient 6 is the large difference in initial measured phosphate concentration, indicating that steady state had not been reached before treatment onset.
The synthetic results in Figure E.2 and E.3 in the supplementary materials show that with fixed Cs and an uniform prior on Ks and Kb (mimicking the parameter estimation in [3,7,8]), we have a very limited identifiability of Ks and Kb for SP, whereas MP and CP recover values very close to the true parameters with significantly lower uncertainty. In addition, the parameter estimates for Ks and Kb were highly correlated and this correlation was significantly reduced by MP and CP. We also considered the full Bayesian model with priors on the synthetic data and the results are depicted in Figure E.5 and E.6 in the supplementary materials. The results showed that MP and CP in general came closer to the true parameters with smaller 95% CI and showed similar results in terms of relative standard deviation.
In summary, the uncertainty associated with the SP results is reduced significantly by using the Bayesian model with priors compared to the standard parameter estimation without the clinical knowledge incorporated. For the Bayesian models, we see that MP and CP are superior to SP in estimating patient-specific parameters Cs and Kb, but that the gain of considering CP compared to MP is limited. However, we see that the uncertainty about Ks is large even when using all available data with the CP model. These findings are further supported by the synthetic results in the supplementary materials, where the estimates obtained by MP and CP are closer to the true parameter value and with less uncertainty. Thus, based on the estimation results, it seems that the SP data without relapse data or consecutive sessions are not sufficient for estimating the parameters reliably.
Debowska et al. [3] present a data set consisting of 25 patients that were examined during three consecutive SP sessions of a one-week dialysis treatment cycle. They present the data as the average of the measurement for the 25 patients and we have read off the data from the figures. Measurements were obtained hourly for a total duration of four hours with the addition of a measurement 45 minutes after ended treatment, i.e., we have five SP measurements and a relapse measurement for each of the three consecutive SP sessions. We choose Vb=20 and Cd=0.
The aim of this subsection is to investigate the improvement of information obtained by including relapse measurement and / or consecutive sessions in the SP model. We investigate the four following scenarios, Scenario 1 (S1) where we consider the first SP treatment only, Scenario 2 (S2) with the first SP treatment with the addition of a measured relapse point, Scenario 3 (S3) where we consider the first SP treatment with relapse point and the first measured data point of the second SP, and Scenario 4 (S4) where we include the data from all three SP consecutive sessions.
The results for the four scenarios are depicted in Figure 9. The measurements included in each parameter estimation are marked with colored dots, whereas the measurements not included in the model estimation are marked with black open circles. The posterior statistics for the parameters are shown in Figure 10 and listed in Table B.4. Correlation of the parameters is shown in Figure 11.
Figure 9a shows estimation without relapse measurement for a single SP session, the phosphate concentration has a quite large 95% CI and undershoots the relapse. If we consider the uncertainty in the correlation plot for the parameters in Figure 11 and Figure 10, we see a large 95% CI for the parameter estimates and relative standard deviation in Table B.4 which is similar to the uncertainty associated with the estimate for the SP estimation in Section 4.1.
A model estimation including the measured relapse 45 minutes after ended treatment is depicted in Figure 9b. The 95% CIs for the phosphate concentration is slightly reduced, but the 95% CIs for the parameters have barely changed as seen in Figure 10, Figure 11 and Table B.4. Hence, including a measurement after 45 minutes relapse has limited effect on the uncertainty of the parameter estimates. This can also be seen by considering the correlation plot in Figure 11, where the width of the distribution is only slightly changed. It is noteworthy that the addition of the relapse point has such limited effect on the estimation. However, this limited effect is due to the very rapid dynamics in the initial relapse phase. The initial relapse is not very sensitive to small changes, whereas a relapse point measured later e.g., after two hours, will have a larger effect on the estimation process due to the slower change in the concentration.
Considering the full relapse in Figure 9c, we see the effect of having a relapse measurement several hours after ended treatment. The estimated steady state for the phosphate concentration has an increased posterior mean and narrower 95% CI compared to Figure 9a and 9b. This increase is explained by the increase for Cs which can be seen in Figure 10 and Figure 11. There is also a slight narrowing of the 95% CI for Ks whereas the effect on Kb is limited as the relative standard deviation actually increases from 16% to 18% compared to the partial relapse. Hence, including relapse measurements has limited effect on the identifiability of Kb, but reduces the uncertainty associated with the estimates for Cs and Ks. This observation is expected based on the model (2.1), since we have Kb=0 in the relapse phase.
Lastly, if we have three consecutive SP treatments for the same patient, we can reduce the uncertainty even further, as shown in Figure 9d. The three consecutive SP treatments carry significant information since the repetition makes the estimates less sensitive to fluctuations in the data, which can also be seen in Figure 10 and Figure 11. Considering the relative standard deviation for Ks in Table B.4, we find that it decreases from 20% to 5 % by considering the consecutive sessions compared to a single session. However, even in the case of a single session, our Bayesian approach has significantly smaller relative standard deviation compared to the estimates found by Debowska et al. [3] and Agar et al. [7], who report a relative standard deviation of 79% and 47%, respectively. Even for Kb, we see a significant narrowing of the 95% CI. Thus, measuring consecutive sessions greatly increases the identifiability of all three model parameters as the relative standard deviation decreases significantly for all three parameter estimates, as seen in Table B.4.
We also investigated the effect of including relapse measurements for the synthetic data for SP, MP and CP. The results including relapse measurements are shown in Figure E.8-E.11 and results for two consecutive sessions are shown in Figure E.12 and E.13. Here we found that the consecutive sessions were more effective than relapse measurements to reduce the uncertainty of the parameters which aligns with the findings in Figure 9. In general for the synthetic data, we found that MP compared to SP had less uncertainty and came closer to the true parameter values.
Phosphate clearance with hemodialysis is crucial for patients with renal failure since abnormal levels of phosphate are associated with increased vascular calcification and mortality. We propose a Bayesian approach to parameter estimation for patients undergoing hemodialysis treatments (SP, MP and CP). The Bayesian approach allows us to formally include clinical knowledge in the model and to use uncertainty quantification to assess how reliably we can estimate the three model parameters: phosphate concentration in the bones, phosphate clearance from bone to blood and from blood to dialysate.
We validated and tested our Bayesian model on two data sets for patients with renal failure. The results showed that the uncertainty for the parameter estimates is greatly reduced by considering MP and CP compared to SP while CP is not significantly better than MP. However, for the parameter governing the diffusion rate between bone phosphate and blood, the uncertainty remained unchanged. We also investigated the impact of including relapse data and consecutive treatments. The results showed that including an early relapse measurement (after 45 minutes) had little effect on the estimation process if not combined with a measurement in the later relapse phase. The relapse measurements taken more than 45 minutes after ended treatment had significant impact on the reliability of the model parameters. Moreover, the results showed that we can reduce the relative standard deviation for the phosphate clearance from blood to bone from 20% to 5% by including consecutive sessions in the estimation process compared to estimation based on a single session.
Numerical results on synthetic data confirmed the findings obtained from the real data, and showed that the parameters were poorly identified for SP if no prior information was included. The uncertainty of the estimates greatly decreased when using the Bayesian model incorporating clinical knowledge, and the MP model generally was closer to the true parameter values of the model. Compared to existing parameter estimates of the phosphate clearance from bone to blood, our Bayesian model can estimate a parameter associated with significantly lower uncertainty for both SP and MP. As the consecutive SP sessions may also be used to reduce the uncertainty of the estimated parameters significantly and such action comes without any costs, it seems very straight forward to implement clinically. Hence, pooling data, e.g., from three consecutive SP sessions to estimate patient specific parameters, is recommendable.
This work was supported by The Villum Foundation (grant no. 25893).
The authors declare there is no conflict of interest.
Patient | Cs | Ks | Kb | ||||||||||||
l | m | u | stdmean | l | m | u | stdmean | l | m | u | stdmean | ||||
1 | 1.41 | 1.71 | 2.06 | 9% | 6.18 | 9.33 | 12.1 | 16% | 10.14 | 13.16 | 15.31 | 10% | |||
2 | 0.88 | 1.12 | 1.44 | 13% | 5.03 | 8.94 | 12.96 | 22% | 6.48 | 10.03 | 13.63 | 17% | |||
3 | 0.98 | 1.23 | 1.51 | 11% | 6.06 | 9.56 | 13.23 | 19% | 5.38 | 8.57 | 12.25 | 21% | |||
4 | 1.61 | 1.90 | 2.23 | 8% | 7.50 | 10.66 | 13.13 | 13% | 8.75 | 11.39 | 13.46 | 11% | |||
5 | 1.33 | 1.63 | 1.98 | 10% | 4.79 | 8.36 | 11.94 | 22% | 6.42 | 9.54 | 12.63 | 17% | |||
6 | 2.22 | 2.60 | 2.99 | 8% | 4.10 | 4.97 | 6.10 | 10% | 9.27 | 10.25 | 11.20 | 5% | |||
7 | 1.45 | 1.78 | 2.08 | 9% | 5.42 | 8.86 | 12.33 | 20% | 2.30 | 4.97 | 8.24 | 30% | |||
8 | 0.85 | 1.08 | 1.39 | 13% | 5.45 | 8.43 | 11.52 | 18% | 6.43 | 9.35 | 12.18 | 16% | |||
9 | 1.10 | 1.4 | 1.71 | 11% | 4.07 | 8.06 | 11.93 | 25% | 1.90 | 4.69 | 8.14 | 34% | |||
10 | 0.97 | 1.23 | 1.51 | 11% | 5.93 | 9.27 | 12.73 | 19% | 3.43 | 6.60 | 9.91 | 25% |
Patient | Cs | Ks | Kb | ||||||||||||
l | m | u | stdmean | m | u | stdmean | l | m | u | stdmean | |||||
1 | 1.41 | 1.57 | 2.13 | 12% | 2.30 | 6.98 | 11.97 | 38% | 5.82 | 7.08 | 8.73 | 10% | |||
2 | 1.05 | 1.15 | 1.37 | 7% | 4.38 | 7.80 | 11.58 | 24% | 7.39 | 8.47 | 9.78 | 7% | |||
3 | 1.26 | 1.40 | 1.62 | 7% | 4.96 | 7.78 | 11.22 | 21% | 6.48 | 7.78 | 9.41 | 10% | |||
4 | 1.29 | 1.45 | 1.71 | 7% | 4.50 | 7.46 | 11.06 | 23% | 7.14 | 8.49 | 10.16 | 9% | |||
5 | 1.31 | 1.42 | 1.65 | 6% | 4.45 | 7.23 | 10.21 | 22% | 9.43 | 10.07 | 10.79 | 3% | |||
6 | 1.23 | 1.37 | 1.65 | 8% | 3.71 | 7.13 | 11.06 | 26% | 6.04 | 7.50 | 9.43 | 11% | |||
7 | 1.50 | 1.64 | 1.91 | 6% | 4.22 | 7.89 | 11.86 | 24% | 5.84 | 7.12 | 8.75 | 11% | |||
8 | 0.90 | 0.99 | 1.19 | 7% | 3.07 | 7.36 | 11.45 | 28% | 3.22 | 4.34 | 6.9 | 20% | |||
9 | 1.16 | 1.28 | 1.44 | 6% | 5.95 | 9.03 | 12.63 | 19% | 4.96 | 5.98 | 7.43 | 10% | |||
10 | 1.2 | 1.32 | 1.54 | 7% | 4.40 | 7.11 | 10.66 | 23% | 6.54 | 7.16 | 7.88 | 5% |
Patient | Cs | Ks | Kb | ||||||||||||
l | m | u | stdmean | l | m | u | stdmean | l | m | u | stdmean | ||||
1 | 1.37 | 1.51 | 1.91 | 8% | 3.19 | 7.26 | 11.34 | 28% | 7.07 | 8.27 | 9.78 | 8% | |||
2 | 1.03 | 1.11 | 1.27 | 5% | 5.44 | 8.45 | 11.72 | 19% | 8.2 | 9.30 | 10.51 | 6% | |||
3 | 1.24 | 1.38 | 1.60 | 7% | 5.11 | 7.70 | 11.14 | 19% | 7.23 | 8.38 | 9.74 | 8% | |||
4 | 1.34 | 1.51 | 1.79 | 8% | 4.30 | 7.25 | 11.14 | 24% | 6.57 | 7.59 | 8.79 | 7% | |||
5 | 1.26 | 1.34 | 1.50 | 5% | 6.72 | 10.48 | 13.93 | 18% | 8.20 | 9.09 | 10.05 | 5% | |||
6 | 1.37 | 1.66 | 2.24 | 14% | 2.02 | 4.21 | 8.41 | 39% | 5.67 | 6.70 | 7.86 | 8% | |||
7 | 1.55 | 1.67 | 1.86 | 5% | 5.89 | 9.20 | 12.74 | 19% | 4.82 | 5.81 | 7.08 | 10% | |||
8 | 0.86 | 0.96 | 1.13 | 7% | 3.39 | 7.09 | 11.05 | 27% | 4.27 | 5.64 | 7.59 | 16% | |||
9 | 1.22 | 1.33 | 1.52 | 6% | 5.42 | 8.54 | 12.28 | 20% | 4.59 | 5.36 | 6.27 | 9% | |||
10 | 1.17 | 1.24 | 1.36 | 4% | 6.70 | 9.62 | 12.62 | 16% | 6.28 | 6.97 | 7.68 | 5% |
Cs | Ks | Kb | |||||||||||||
l | m | u | stdmean | l | m | u | stdmean | l | m | u | stdmean | ||||
NR | 1.11 | 1.37 | 1.74 | 11% | 6.44 | 10.51 | 14.84 | 20% | 6.48 | 10.04 | 13.53 | 18% | |||
PR | 1.25 | 1.45 | 1.71 | 8% | 7.57 | 11.70 | 15.61 | 18% | 8.18 | 12.17 | 14.42 | 14% | |||
FR | 1.49 | 1.68 | 1.79 | 4% | 5.97 | 8.75 | 11.21 | 15% | 7.77 | 11.94 | 14.88 | 16% | |||
FTR | 1.64 | 1.68 | 1.71 | 1% | 8.64 | 9.66 | 10.57 | 5% | 13.01 | 14.4 | 15.51 | 4% |
Due the thin tails in the QQ-plots we supplement the visual inspections in Figure D.1 and Figure D.2 with an additional analytic test, the Anderson-Darling test [26]. We determine the p-values for the Anderson-Darling statistic [27]. The Anderson-Darling test gives p-values of p = 0.08, p = 0.04, and p = 0.07 for SP, MP, and CP, respectively. With a significant level of α=0.05, we see that all p-values are close to α but those for SP and CP are just above while that for MP is just below it. Thus, the conclusions are very sensitive to the choice of significant level, and one should be careful to make definitive conclusions until more data are collected. Altogether, we do not reject the hypothesis that the residuals are normal distributed but acknowledge that more data is needed to reach a robust conclusion.
We generate synthetic data for a patient with renal failure to investigate the identifiability of the hemodialysis model parameters. We base the true parameters on the estimates obtained for patient 2 and simulate both SP and MP treatments with relapse. We add Gaussian noise with mean equal to the "true" trajectory of the state variables and with variance σ2=0.05. Figure E.1 shows simulated data for a renal failure patient during hemodialysis with added Gaussian noise. The true parameters for the simulation are listed in Table E.1. Note that we have used the true values for the parameters estimated from data, i.e., Vb, Vd and Cd and thus the only uncertainty introduced arise from the Gaussian measurement noise.
Cs | Ks | Kb | Vb | Vd | Cd | Z0 | X0 |
1.23 | 8.39 | 9.23 | 17.77 | 23.0 | 0.12 | 1.23 | 1.23 |
We will use the simulated data to investigate the following four cases:
● Reduced Bayesian model with fixed Cs and uniform priors
● Full Bayesian model
● Full Bayesian model with relapse measurements
● Full Bayesian model with consecutive sessions
We fix the parameter Cs and reduce the priors to uniform priors. The uniform priors correspond to simply having bounds on the parameters but with equal probability for all outcomes in the specified interval. Hence this reduced Bayesian model mimics the simple model suggested by Debowska et al. [3] and Agar et al. [7]. The results for the reduced Bayesian model are shown in Figure E.2, the parameter estimates are visualized in Figure E.3 and listed in Table E.2. Correlation plot is shown in Figure E.4.
HD | Ks | Kb | ||||||||
l | m | u | stdmean | l | m | u | stdmean | |||
SP | 1.83 | 5.93 | 15.62 | 50% | 6.22 | 9.28 | 17.8 | 28% | ||
MP | 6.22 | 7.48 | 9.09 | 10% | 7.62 | 9.27 | 11.82 | 11% | ||
CP | 6.01 | 6.97 | 8.06 | 8% | 8.44 | 9.64 | 11.07 | 7% |
Remarkably, even though we have ignored the uncertainty in Cs, the SP model is very uncertain about Ks and Kb, as seen in Figure E.3 and in Table E.2. Moreover, we see a pronounced correlation between Ks and Kb in Figure E.4. Hence, the parameters of the SP model are poorly identified when no prior knowledge is included in the model. The parameters for MP and CP are, on the other hand, more certain about their estimates and closer to the true parameter values with lower relative standard deviation. However, this certainty comes at a cost in terms of validity of the available knowledge of Cs since the estimates for Ks and Kb may be biased if this estimate is not sufficiently close to the true parameter.
We consider Cs to be a parameter of the model such that we investigate the same estimation problem as presented in Section 4 for the SP and MP data. The results are shown in Figure E.5, the parameter estimates and corresponding 95% CI are visualized in Figure E.6 and listed in Table E.3. Correlation plot shown in Figure E.7.
HD | Cs | Ks | Kb | ||||||||||||
l | m | u | stdmean | l | m | u | stdmean | l | m | u | stdmean | ||||
SP | 0.86 | 1.13 | 1.48 | 14% | 3.94 | 6.85 | 10.52 | 24% | 5.76 | 8.32 | 11.19 | 17% | |||
MP | 1.14 | 1.27 | 1.5 | 7% | 4.39 | 7.23 | 10.94 | 23% | 7.51 | 8.99 | 10.78 | 9% | |||
CP | 1.16 | 1.31 | 1.55 | 8% | 3.97 | 6.36 | 9.7 | 22% | 8.31 | 9.47 | 10.79 | 7% |
Figure E.5 shows the same behavior as when using real data in Section 4. All three models find a satisfying fit to the data and all three models estimate posterior means of the parameters that are close to the true parameter values. Figure E.6 and E.4 show that the uncertainty in the parameter space is much larger for SP than for MP and CP for the parameters Cs and Kb, whereas the uncertainty is only slightly reduced for MP and CP compared to SP for the estimate of Ks. Hence, the identifiability of Cs and Kb is greatly increased by considering MP measurements since the mean is closer to the true value and the uncertainty associated with the estimate is greatly reduced compared to SP.
To ensure that the priors for Ks and Kb are not too strong, we redid the investigation with an 10% increase of the variance, i.e., σKs=2.2 and σKb=2.2. The results are shown in Table E.4 and show no significant change in the results.
HD | Cs | Ks | Kb | ||||||||||||
l | m | u | stdmean | l | m | u | stdmean | l | m | u | stdmean | ||||
SP | 0.86 | 1.15 | 1.5 | 14 % | 3.49 | 6.63 | 10.75 | 28% | 5.61 | 8.37 | 11.4 | 18% | |||
MP | 1.14 | 1.27 | 1.51 | 7 % | 4.24 | 7.21 | 11.09 | 24 % | 7.54 | 9.05 | 10.9 | 9 % | |||
CP | 1.16 | 1.31 | 1.56 | 8 % | 3.89 | 6.21 | 9.59 | 23 % | 8.32 | 9.47 | 10.75 | 6 % |
In the third simulated experiment, we investigate the effect of including relapse measurements 1, 2 and 4 hours after ended treatment for both SP and MP. The estimated phosphate concentrations are depicted in Figure E.8 and Figure E.9 for SP and MP, respectively. The corresponding parameter estimates are visualized in Figure E.11 and listed in Table E.5.
HD | Cs | Ks | Kb | |||||||||||||
l | m | u | stdmean | l | m | u | stdmean | l | m | u | stdmean | |||||
SP | None | 0.87 | 1.13 | 1.49 | 14% | 3.82 | 6.81 | 10.36 | 24% | 5.80 | 8.31 | 11.14 | 16% | |||
1 hour | 1.00 | 1.25 | 1.56 | 9% | 4.81 | 7.31 | 10.78 | 22% | 6.87 | 9.63 | 12.04 | 11% | ||||
2 hour | 1.00 | 1.18 | 1.46 | 10% | 4.55 | 7.46 | 10.96 | 22% | 6.88 | 8.99 | 11.07 | 12% | ||||
4 hour | 1.08 | 1.21 | 1.39 | 6% | 4.84 | 7.26 | 10.35 | 19% | 7.55 | 9.20 | 11.01 | 9% | ||||
MP | None | 1.14 | 1.27 | 1.50 | 7% | 4.34 | 7.27 | 10.97 | 23% | 7.54 | 9.02 | 10.76 | 9% | |||
1 hour | 1.14 | 1.25 | 1.44 | 6% | 4.68 | 7.72 | 11.33 | 21% | 7.5 | 8.97 | 10.81 | 9% | ||||
2 hour | 1.15 | 1.26 | 1.44 | 6% | 4.70 | 7.55 | 10.99 | 21% | 7.57 | 8.98 | 10.75 | 9% | ||||
4 hour | 1.15 | 1.22 | 1.33 | 4% | 5.72 | 8.34 | 11.24 | 17% | 7.57 | 8.99 | 10.71 | 9% | ||||
CP | None | 1.15 | 1.31 | 1.55 | 8% | 3.92 | 6.26 | 9.54 | 23% | 8.34 | 9.46 | 10.66 | 6% | |||
1 hour | 1.15 | 1.26 | 1.41 | 5% | 5.16 | 7.38 | 10.16 | 15% | 8.28 | 9.46 | 10.73 | 7% | ||||
2 hour | 1.17 | 1.27 | 1.41 | 5% | 5.04 | 6.96 | 9.39 | 16% | 8.35 | 9.47 | 10.76 | 7% | ||||
4 hour | 1.16 | 1.23 | 1.32 | 3% | 5.82 | 7.59 | 9.68 | 13% | 8.42 | 9.56 | 10.83 | 6% |
Figure E.11 shows that the uncertainty about Cs is reduced for all methods by including relapse measurements, especially by including a relapse measurement after 4 hours.
The uncertainty for Ks is only slightly reduced for SP and MP by including relapse measurements after 1, 2 and 4 hours. This observation is quantified in Table E.5 where the relative standard deviation is reduced by 5% and 6% by including relapse up to four hours for SP and MP, respectively. Considering the estimate for Ks for CP we obtain an reduction in relative standard deviation of 10% by including the relapse measurements.
For Kb, we see a limited effect of including relapse measurements for MP and CP, as expected, since Kb is not active in the equations during relapse since y(t) and Cd are zero. However, we do see a reduction in uncertainty for Kb for SP. These findings align very well with the data experiment including relapse presented in Section 4. Overall, it seems that SP has the greatest reduction in uncertainty by including relapse measurements, which might be explained by SP having the most uncertain starting point.
Lastly, we investigate the effect of measuring a patient for two consecutive sessions. We simulated that the patient is measured during two sessions with 18 hours between start of the first and second session. The phosphate concentration is in a steady state after 18 hours and thus, it does not affect the results if the time between the starts is larger, e.g., 48 hours. The results are shown in Figure E.12, Figure E.13 and Table E.6.
HD | Cs | Ks | Kb | ||||||||||||
l | m | u | stdmean | l | m | u | stdmean | l | m | u | stdmean | ||||
SP | 1.15 | 1.26 | 1.38 | 5% | 4.58 | 7.1 | 10.15 | 20% | 6.59 | 8.73 | 11.11 | 13% | |||
MP | 1.21 | 1.29 | 1.38 | 3% | 5.14 | 6.77 | 9.18 | 15% | 8.06 | 9.11 | 10.36 | 6% | |||
CP | 1.19 | 1.27 | 1.37 | 4% | 5.26 | 6.83 | 8.79 | 13% | 8.43 | 9.56 | 10.81 | 6% |
Figure E.12 shows that the uncertainty for the relapse phase, especially for SP, is greatly reduced compared to the results in Figure E.5. Similar for the parameter estimates, we see a great reduction in uncertainty for Cs and Kb for SP by comparing Figure E.6 and E.13 whereas there is only a slight reduction in the uncertainty for Ks. For MP and CP, we see a great reduction in uncertainty compared to SP for all three parameters, but that the mean estimate of Ks is slightly under estimated.
We assume that the phosphate concentration in the dialysate for SP is constant. However, the assumption of a constant Cd is not crucial. Allowing it to be a variable with initial value 0 extends the model by an extra differential equation. The result of the extended SP model is shown in Figure E.14. This extension results in a fast transient in Cd toward the steady state value given by data with at doubling time of approximately 10-15 minutes.
[1] |
N. Bebiano, S. Furtado, J. da Providência, W. R. Xu, J. P. da Providência, Approximations for the von Neumann and Renyi entropies of graphs using the Euler-Maclaurin formula, Electron. Trans. Numer. Anal., 48 (2018), 227–242. https://doi.org/10.1553/etna_vol48s227 doi: 10.1553/etna_vol48s227
![]() |
[2] |
M. Dairyko, L. Hogben, J. C. H. Lin, J. Lockhart, D. Roberson, S. Severini, et al., Note on von Neumann and Rényi entropies of a graph, Linear Algebra Appl., 521 (2017), 240–253. https://doi.org/10.1016/j.laa.2017.01.037 doi: 10.1016/j.laa.2017.01.037
![]() |
[3] | V. Giovannetti, S. Severini, The Kirchhoff's matrix tree theorem revisited: counting spanning trees with the quantum relative entropy, preprint, arXiv: 1102.2398. https://doi.org/10.48550/arXiv.1102.2398 |
[4] |
H. Lin, B. Zhou, On the von Neumann entropy of a graph, Discrete Appl. Math., 247 (2018), 448–455. https://doi.org/10.1016/j.dam.2018.04.004 doi: 10.1016/j.dam.2018.04.004
![]() |
[5] |
G. Minello, L. Rossi, A. Torsello, On the von Neumann entropy of graphs, J. Complex Networks, 7 (2019), 491–514. https://doi.org/10.1093/comnet/cny028 doi: 10.1093/comnet/cny028
![]() |
[6] |
D. E. Simmons, J. P. Coon, A. Datta, Symmetric Laplacians, quantum density matrices and their von Neumann entropy, Linear Algebra Appl., 521 (2017), 240–253. https://doi.org/10.1016/j.laa.2017.06.038 doi: 10.1016/j.laa.2017.06.038
![]() |
[7] |
D. E. Simmons, J. P. Coon, A. Datta, The von Neumann Theil index: characterizing graph centralization using the von Neumann index, J. Complex Networks, 6 (2018), 859–876. https://doi.org/10.1093/comnet/cnx061 doi: 10.1093/comnet/cnx061
![]() |
[8] |
C. Ye, R. C. Wilson, C. H. Comin, L. F. Costa, E. R. Hancock, Approximate von Neumann entropy for direct graphs, Phys. Rev. E, 89 (2014), 052804. https://doi.org/10.1103/PhysRevE.89.052804 doi: 10.1103/PhysRevE.89.052804
![]() |
[9] |
R. Grone, R. Merris, V. S. Sunder, The Laplacian spectrum of a graph, SIAM J. Matrix Anal. Appl., 11 (1990), 218–238. https://doi.org/10.1137/0611016 doi: 10.1137/0611016
![]() |
[10] | R. A. Horn, C. R. Johnson, Matrix Analysis, 2nd edition, Cambridge University Press, Cambridge, 2013. |
[11] | J. V. Neumann, Mathematical Foundations of Quantum Mechanics, Number 2, Princeton University Press, Princeton, N.J., 1955. |
[12] | L. D. Landau, E. M. Lifshitz, Statistical Physics, Pergamon Press, Oxford-Edinburgh-New York, 1969. |
[13] | A. Rényi, On measures of entropy and information, in Proceedings of the Fourth Berkeley Symposium on Mathematical Statistics and Probability, Volume 1: Contributions to the Theory of Statistics, 4 (1961), 547–562. |
[14] |
T. M. Apostol, An elementary view of Euler's summation formula, Amer. Math. Monthly, 106 (1999), 409–418. https://doi.org/10.1080/00029890.1999.12005063 doi: 10.1080/00029890.1999.12005063
![]() |
[15] |
V. Lampret, The Euler–Maclaurin and Taylor formulas: twin, elementary derivations, Math. Mag., 74 (2001), 109–122. https://doi.org/10.1080/0025570X.2001.11953046 doi: 10.1080/0025570X.2001.11953046
![]() |
[16] | V. Lampret, The Euler-Maclaurin Formula and Sums of Powers Revisited, Int. J. Contemp. Math. Sci., 5 (2010), 2401–2407. |
[17] |
M. Z. Spivey, The Euler-Maclaurin formula and sums of powers, Math. Mag., 79 (2006), 61–65. https://doi.org/10.1080/0025570X.2006.11953378 doi: 10.1080/0025570X.2006.11953378
![]() |
[18] |
A. Greenbaum, R. C. Li, M. L. Overton, First-order perturbation theory for eigenvalues and eigenvectors, SIAM Rev., 62 (2020), 463–482. https://doi.org/10.1137/19M124784X doi: 10.1137/19M124784X
![]() |
1. | M. Andersen, K. O. Bangsgaard, J. G. Heaf, J. T. Ottesen, Analytical solution of phosphate kinetics for hemodialysis, 2023, 87, 0303-6812, 10.1007/s00285-023-01942-4 | |
2. | Qin Gong, Rui Chen, Haiping Ren, Fan Zhang, Estimation of the Reliability Function of the Generalized Rayleigh Distribution under Progressive First-Failure Censoring Model, 2024, 13, 2075-1680, 580, 10.3390/axioms13090580 | |
3. | Mahmoud M. Ramadan, Rashad M. EL-Sagheer, Amel Abd-El-Monem, Estimating the Lifetime Parameters of the Odd-Generalized-Exponential–Inverse-Weibull Distribution Using Progressive First-Failure Censoring: A Methodology with an Application, 2024, 13, 2075-1680, 822, 10.3390/axioms13120822 | |
4. | Jacek Waniewski, Malgorzata Debowska, Alicja Wojcik-Zaluska, Wojciech Zaluska, Bengt Lindholm, Mathematical models for phosphate kinetics in patients on maintenance hemodialysis, 2025, 15, 2045-2322, 10.1038/s41598-025-93443-1 |
Estimate | Patient | |||||||||||
1 | 2 | 3 | 4 | 5 | 6 | 7 | 8 | 9 | 10 | |||
SP | Cd [mmol/L] | 0.16 | 0.12 | 0.11 | 0.16 | 0.18 | 0.21 | 0.14 | 0.09 | 0.14 | 0.09 | |
Vb [L] | 16.88 | 17.74 | 16.92 | 21.20 | 18.20 | 14.74 | 15.32 | 13.04 | 20.20 | 18.00 | ||
MP | Vb [L] | 16.99 | 17.80 | 17.43 | 21.20 | 18.39 | 15.15 | 15.48 | 13.76 | 20.21 | 18.25 | |
Vd [L] | 22.61 | 23.00 | 26.57 | 31.93 | 28.51 | 20.15 | 23.42 | 14.24 | 28.59 | 23.25 |
Patient | SP | MP | CP | |||
1 | 0.29 | 0.08 | 0.1 | |||
2 | 0.17 | 0.04 | 0.05 | |||
3 | 0.11 | 0.06 | 0.08 | |||
4 | 0.32 | 0.06 | 0.09 | |||
5 | 0.26 | 0.02 | 0.04 | |||
6 | 0.59 | 0.07 | 0.1 | |||
7 | 0.27 | 0.08 | 0.12 | |||
8 | 0.16 | 0.08 | 0.11 | |||
9 | 0.19 | 0.06 | 0.08 | |||
10 | 0.1 | 0.03 | 0.04 |
Patient | Cs | Ks | Kb | ||||||||||||
l | m | u | stdmean | l | m | u | stdmean | l | m | u | stdmean | ||||
1 | 1.41 | 1.71 | 2.06 | 9% | 6.18 | 9.33 | 12.1 | 16% | 10.14 | 13.16 | 15.31 | 10% | |||
2 | 0.88 | 1.12 | 1.44 | 13% | 5.03 | 8.94 | 12.96 | 22% | 6.48 | 10.03 | 13.63 | 17% | |||
3 | 0.98 | 1.23 | 1.51 | 11% | 6.06 | 9.56 | 13.23 | 19% | 5.38 | 8.57 | 12.25 | 21% | |||
4 | 1.61 | 1.90 | 2.23 | 8% | 7.50 | 10.66 | 13.13 | 13% | 8.75 | 11.39 | 13.46 | 11% | |||
5 | 1.33 | 1.63 | 1.98 | 10% | 4.79 | 8.36 | 11.94 | 22% | 6.42 | 9.54 | 12.63 | 17% | |||
6 | 2.22 | 2.60 | 2.99 | 8% | 4.10 | 4.97 | 6.10 | 10% | 9.27 | 10.25 | 11.20 | 5% | |||
7 | 1.45 | 1.78 | 2.08 | 9% | 5.42 | 8.86 | 12.33 | 20% | 2.30 | 4.97 | 8.24 | 30% | |||
8 | 0.85 | 1.08 | 1.39 | 13% | 5.45 | 8.43 | 11.52 | 18% | 6.43 | 9.35 | 12.18 | 16% | |||
9 | 1.10 | 1.4 | 1.71 | 11% | 4.07 | 8.06 | 11.93 | 25% | 1.90 | 4.69 | 8.14 | 34% | |||
10 | 0.97 | 1.23 | 1.51 | 11% | 5.93 | 9.27 | 12.73 | 19% | 3.43 | 6.60 | 9.91 | 25% |
Patient | Cs | Ks | Kb | ||||||||||||
l | m | u | stdmean | m | u | stdmean | l | m | u | stdmean | |||||
1 | 1.41 | 1.57 | 2.13 | 12% | 2.30 | 6.98 | 11.97 | 38% | 5.82 | 7.08 | 8.73 | 10% | |||
2 | 1.05 | 1.15 | 1.37 | 7% | 4.38 | 7.80 | 11.58 | 24% | 7.39 | 8.47 | 9.78 | 7% | |||
3 | 1.26 | 1.40 | 1.62 | 7% | 4.96 | 7.78 | 11.22 | 21% | 6.48 | 7.78 | 9.41 | 10% | |||
4 | 1.29 | 1.45 | 1.71 | 7% | 4.50 | 7.46 | 11.06 | 23% | 7.14 | 8.49 | 10.16 | 9% | |||
5 | 1.31 | 1.42 | 1.65 | 6% | 4.45 | 7.23 | 10.21 | 22% | 9.43 | 10.07 | 10.79 | 3% | |||
6 | 1.23 | 1.37 | 1.65 | 8% | 3.71 | 7.13 | 11.06 | 26% | 6.04 | 7.50 | 9.43 | 11% | |||
7 | 1.50 | 1.64 | 1.91 | 6% | 4.22 | 7.89 | 11.86 | 24% | 5.84 | 7.12 | 8.75 | 11% | |||
8 | 0.90 | 0.99 | 1.19 | 7% | 3.07 | 7.36 | 11.45 | 28% | 3.22 | 4.34 | 6.9 | 20% | |||
9 | 1.16 | 1.28 | 1.44 | 6% | 5.95 | 9.03 | 12.63 | 19% | 4.96 | 5.98 | 7.43 | 10% | |||
10 | 1.2 | 1.32 | 1.54 | 7% | 4.40 | 7.11 | 10.66 | 23% | 6.54 | 7.16 | 7.88 | 5% |
Patient | Cs | Ks | Kb | ||||||||||||
l | m | u | stdmean | l | m | u | stdmean | l | m | u | stdmean | ||||
1 | 1.37 | 1.51 | 1.91 | 8% | 3.19 | 7.26 | 11.34 | 28% | 7.07 | 8.27 | 9.78 | 8% | |||
2 | 1.03 | 1.11 | 1.27 | 5% | 5.44 | 8.45 | 11.72 | 19% | 8.2 | 9.30 | 10.51 | 6% | |||
3 | 1.24 | 1.38 | 1.60 | 7% | 5.11 | 7.70 | 11.14 | 19% | 7.23 | 8.38 | 9.74 | 8% | |||
4 | 1.34 | 1.51 | 1.79 | 8% | 4.30 | 7.25 | 11.14 | 24% | 6.57 | 7.59 | 8.79 | 7% | |||
5 | 1.26 | 1.34 | 1.50 | 5% | 6.72 | 10.48 | 13.93 | 18% | 8.20 | 9.09 | 10.05 | 5% | |||
6 | 1.37 | 1.66 | 2.24 | 14% | 2.02 | 4.21 | 8.41 | 39% | 5.67 | 6.70 | 7.86 | 8% | |||
7 | 1.55 | 1.67 | 1.86 | 5% | 5.89 | 9.20 | 12.74 | 19% | 4.82 | 5.81 | 7.08 | 10% | |||
8 | 0.86 | 0.96 | 1.13 | 7% | 3.39 | 7.09 | 11.05 | 27% | 4.27 | 5.64 | 7.59 | 16% | |||
9 | 1.22 | 1.33 | 1.52 | 6% | 5.42 | 8.54 | 12.28 | 20% | 4.59 | 5.36 | 6.27 | 9% | |||
10 | 1.17 | 1.24 | 1.36 | 4% | 6.70 | 9.62 | 12.62 | 16% | 6.28 | 6.97 | 7.68 | 5% |
Cs | Ks | Kb | |||||||||||||
l | m | u | stdmean | l | m | u | stdmean | l | m | u | stdmean | ||||
NR | 1.11 | 1.37 | 1.74 | 11% | 6.44 | 10.51 | 14.84 | 20% | 6.48 | 10.04 | 13.53 | 18% | |||
PR | 1.25 | 1.45 | 1.71 | 8% | 7.57 | 11.70 | 15.61 | 18% | 8.18 | 12.17 | 14.42 | 14% | |||
FR | 1.49 | 1.68 | 1.79 | 4% | 5.97 | 8.75 | 11.21 | 15% | 7.77 | 11.94 | 14.88 | 16% | |||
FTR | 1.64 | 1.68 | 1.71 | 1% | 8.64 | 9.66 | 10.57 | 5% | 13.01 | 14.4 | 15.51 | 4% |
Cs | Ks | Kb | Vb | Vd | Cd | Z0 | X0 |
1.23 | 8.39 | 9.23 | 17.77 | 23.0 | 0.12 | 1.23 | 1.23 |
HD | Ks | Kb | ||||||||
l | m | u | stdmean | l | m | u | stdmean | |||
SP | 1.83 | 5.93 | 15.62 | 50% | 6.22 | 9.28 | 17.8 | 28% | ||
MP | 6.22 | 7.48 | 9.09 | 10% | 7.62 | 9.27 | 11.82 | 11% | ||
CP | 6.01 | 6.97 | 8.06 | 8% | 8.44 | 9.64 | 11.07 | 7% |
HD | Cs | Ks | Kb | ||||||||||||
l | m | u | stdmean | l | m | u | stdmean | l | m | u | stdmean | ||||
SP | 0.86 | 1.13 | 1.48 | 14% | 3.94 | 6.85 | 10.52 | 24% | 5.76 | 8.32 | 11.19 | 17% | |||
MP | 1.14 | 1.27 | 1.5 | 7% | 4.39 | 7.23 | 10.94 | 23% | 7.51 | 8.99 | 10.78 | 9% | |||
CP | 1.16 | 1.31 | 1.55 | 8% | 3.97 | 6.36 | 9.7 | 22% | 8.31 | 9.47 | 10.79 | 7% |
HD | Cs | Ks | Kb | ||||||||||||
l | m | u | stdmean | l | m | u | stdmean | l | m | u | stdmean | ||||
SP | 0.86 | 1.15 | 1.5 | 14 % | 3.49 | 6.63 | 10.75 | 28% | 5.61 | 8.37 | 11.4 | 18% | |||
MP | 1.14 | 1.27 | 1.51 | 7 % | 4.24 | 7.21 | 11.09 | 24 % | 7.54 | 9.05 | 10.9 | 9 % | |||
CP | 1.16 | 1.31 | 1.56 | 8 % | 3.89 | 6.21 | 9.59 | 23 % | 8.32 | 9.47 | 10.75 | 6 % |
HD | Cs | Ks | Kb | |||||||||||||
l | m | u | stdmean | l | m | u | stdmean | l | m | u | stdmean | |||||
SP | None | 0.87 | 1.13 | 1.49 | 14% | 3.82 | 6.81 | 10.36 | 24% | 5.80 | 8.31 | 11.14 | 16% | |||
1 hour | 1.00 | 1.25 | 1.56 | 9% | 4.81 | 7.31 | 10.78 | 22% | 6.87 | 9.63 | 12.04 | 11% | ||||
2 hour | 1.00 | 1.18 | 1.46 | 10% | 4.55 | 7.46 | 10.96 | 22% | 6.88 | 8.99 | 11.07 | 12% | ||||
4 hour | 1.08 | 1.21 | 1.39 | 6% | 4.84 | 7.26 | 10.35 | 19% | 7.55 | 9.20 | 11.01 | 9% | ||||
MP | None | 1.14 | 1.27 | 1.50 | 7% | 4.34 | 7.27 | 10.97 | 23% | 7.54 | 9.02 | 10.76 | 9% | |||
1 hour | 1.14 | 1.25 | 1.44 | 6% | 4.68 | 7.72 | 11.33 | 21% | 7.5 | 8.97 | 10.81 | 9% | ||||
2 hour | 1.15 | 1.26 | 1.44 | 6% | 4.70 | 7.55 | 10.99 | 21% | 7.57 | 8.98 | 10.75 | 9% | ||||
4 hour | 1.15 | 1.22 | 1.33 | 4% | 5.72 | 8.34 | 11.24 | 17% | 7.57 | 8.99 | 10.71 | 9% | ||||
CP | None | 1.15 | 1.31 | 1.55 | 8% | 3.92 | 6.26 | 9.54 | 23% | 8.34 | 9.46 | 10.66 | 6% | |||
1 hour | 1.15 | 1.26 | 1.41 | 5% | 5.16 | 7.38 | 10.16 | 15% | 8.28 | 9.46 | 10.73 | 7% | ||||
2 hour | 1.17 | 1.27 | 1.41 | 5% | 5.04 | 6.96 | 9.39 | 16% | 8.35 | 9.47 | 10.76 | 7% | ||||
4 hour | 1.16 | 1.23 | 1.32 | 3% | 5.82 | 7.59 | 9.68 | 13% | 8.42 | 9.56 | 10.83 | 6% |
HD | Cs | Ks | Kb | ||||||||||||
l | m | u | stdmean | l | m | u | stdmean | l | m | u | stdmean | ||||
SP | 1.15 | 1.26 | 1.38 | 5% | 4.58 | 7.1 | 10.15 | 20% | 6.59 | 8.73 | 11.11 | 13% | |||
MP | 1.21 | 1.29 | 1.38 | 3% | 5.14 | 6.77 | 9.18 | 15% | 8.06 | 9.11 | 10.36 | 6% | |||
CP | 1.19 | 1.27 | 1.37 | 4% | 5.26 | 6.83 | 8.79 | 13% | 8.43 | 9.56 | 10.81 | 6% |
Estimate | Patient | |||||||||||
1 | 2 | 3 | 4 | 5 | 6 | 7 | 8 | 9 | 10 | |||
SP | Cd [mmol/L] | 0.16 | 0.12 | 0.11 | 0.16 | 0.18 | 0.21 | 0.14 | 0.09 | 0.14 | 0.09 | |
Vb [L] | 16.88 | 17.74 | 16.92 | 21.20 | 18.20 | 14.74 | 15.32 | 13.04 | 20.20 | 18.00 | ||
MP | Vb [L] | 16.99 | 17.80 | 17.43 | 21.20 | 18.39 | 15.15 | 15.48 | 13.76 | 20.21 | 18.25 | |
Vd [L] | 22.61 | 23.00 | 26.57 | 31.93 | 28.51 | 20.15 | 23.42 | 14.24 | 28.59 | 23.25 |
Patient | SP | MP | CP | |||
1 | 0.29 | 0.08 | 0.1 | |||
2 | 0.17 | 0.04 | 0.05 | |||
3 | 0.11 | 0.06 | 0.08 | |||
4 | 0.32 | 0.06 | 0.09 | |||
5 | 0.26 | 0.02 | 0.04 | |||
6 | 0.59 | 0.07 | 0.1 | |||
7 | 0.27 | 0.08 | 0.12 | |||
8 | 0.16 | 0.08 | 0.11 | |||
9 | 0.19 | 0.06 | 0.08 | |||
10 | 0.1 | 0.03 | 0.04 |
Patient | Cs | Ks | Kb | ||||||||||||
l | m | u | stdmean | l | m | u | stdmean | l | m | u | stdmean | ||||
1 | 1.41 | 1.71 | 2.06 | 9% | 6.18 | 9.33 | 12.1 | 16% | 10.14 | 13.16 | 15.31 | 10% | |||
2 | 0.88 | 1.12 | 1.44 | 13% | 5.03 | 8.94 | 12.96 | 22% | 6.48 | 10.03 | 13.63 | 17% | |||
3 | 0.98 | 1.23 | 1.51 | 11% | 6.06 | 9.56 | 13.23 | 19% | 5.38 | 8.57 | 12.25 | 21% | |||
4 | 1.61 | 1.90 | 2.23 | 8% | 7.50 | 10.66 | 13.13 | 13% | 8.75 | 11.39 | 13.46 | 11% | |||
5 | 1.33 | 1.63 | 1.98 | 10% | 4.79 | 8.36 | 11.94 | 22% | 6.42 | 9.54 | 12.63 | 17% | |||
6 | 2.22 | 2.60 | 2.99 | 8% | 4.10 | 4.97 | 6.10 | 10% | 9.27 | 10.25 | 11.20 | 5% | |||
7 | 1.45 | 1.78 | 2.08 | 9% | 5.42 | 8.86 | 12.33 | 20% | 2.30 | 4.97 | 8.24 | 30% | |||
8 | 0.85 | 1.08 | 1.39 | 13% | 5.45 | 8.43 | 11.52 | 18% | 6.43 | 9.35 | 12.18 | 16% | |||
9 | 1.10 | 1.4 | 1.71 | 11% | 4.07 | 8.06 | 11.93 | 25% | 1.90 | 4.69 | 8.14 | 34% | |||
10 | 0.97 | 1.23 | 1.51 | 11% | 5.93 | 9.27 | 12.73 | 19% | 3.43 | 6.60 | 9.91 | 25% |
Patient | Cs | Ks | Kb | ||||||||||||
l | m | u | stdmean | m | u | stdmean | l | m | u | stdmean | |||||
1 | 1.41 | 1.57 | 2.13 | 12% | 2.30 | 6.98 | 11.97 | 38% | 5.82 | 7.08 | 8.73 | 10% | |||
2 | 1.05 | 1.15 | 1.37 | 7% | 4.38 | 7.80 | 11.58 | 24% | 7.39 | 8.47 | 9.78 | 7% | |||
3 | 1.26 | 1.40 | 1.62 | 7% | 4.96 | 7.78 | 11.22 | 21% | 6.48 | 7.78 | 9.41 | 10% | |||
4 | 1.29 | 1.45 | 1.71 | 7% | 4.50 | 7.46 | 11.06 | 23% | 7.14 | 8.49 | 10.16 | 9% | |||
5 | 1.31 | 1.42 | 1.65 | 6% | 4.45 | 7.23 | 10.21 | 22% | 9.43 | 10.07 | 10.79 | 3% | |||
6 | 1.23 | 1.37 | 1.65 | 8% | 3.71 | 7.13 | 11.06 | 26% | 6.04 | 7.50 | 9.43 | 11% | |||
7 | 1.50 | 1.64 | 1.91 | 6% | 4.22 | 7.89 | 11.86 | 24% | 5.84 | 7.12 | 8.75 | 11% | |||
8 | 0.90 | 0.99 | 1.19 | 7% | 3.07 | 7.36 | 11.45 | 28% | 3.22 | 4.34 | 6.9 | 20% | |||
9 | 1.16 | 1.28 | 1.44 | 6% | 5.95 | 9.03 | 12.63 | 19% | 4.96 | 5.98 | 7.43 | 10% | |||
10 | 1.2 | 1.32 | 1.54 | 7% | 4.40 | 7.11 | 10.66 | 23% | 6.54 | 7.16 | 7.88 | 5% |
Patient | Cs | Ks | Kb | ||||||||||||
l | m | u | stdmean | l | m | u | stdmean | l | m | u | stdmean | ||||
1 | 1.37 | 1.51 | 1.91 | 8% | 3.19 | 7.26 | 11.34 | 28% | 7.07 | 8.27 | 9.78 | 8% | |||
2 | 1.03 | 1.11 | 1.27 | 5% | 5.44 | 8.45 | 11.72 | 19% | 8.2 | 9.30 | 10.51 | 6% | |||
3 | 1.24 | 1.38 | 1.60 | 7% | 5.11 | 7.70 | 11.14 | 19% | 7.23 | 8.38 | 9.74 | 8% | |||
4 | 1.34 | 1.51 | 1.79 | 8% | 4.30 | 7.25 | 11.14 | 24% | 6.57 | 7.59 | 8.79 | 7% | |||
5 | 1.26 | 1.34 | 1.50 | 5% | 6.72 | 10.48 | 13.93 | 18% | 8.20 | 9.09 | 10.05 | 5% | |||
6 | 1.37 | 1.66 | 2.24 | 14% | 2.02 | 4.21 | 8.41 | 39% | 5.67 | 6.70 | 7.86 | 8% | |||
7 | 1.55 | 1.67 | 1.86 | 5% | 5.89 | 9.20 | 12.74 | 19% | 4.82 | 5.81 | 7.08 | 10% | |||
8 | 0.86 | 0.96 | 1.13 | 7% | 3.39 | 7.09 | 11.05 | 27% | 4.27 | 5.64 | 7.59 | 16% | |||
9 | 1.22 | 1.33 | 1.52 | 6% | 5.42 | 8.54 | 12.28 | 20% | 4.59 | 5.36 | 6.27 | 9% | |||
10 | 1.17 | 1.24 | 1.36 | 4% | 6.70 | 9.62 | 12.62 | 16% | 6.28 | 6.97 | 7.68 | 5% |
Cs | Ks | Kb | |||||||||||||
l | m | u | stdmean | l | m | u | stdmean | l | m | u | stdmean | ||||
NR | 1.11 | 1.37 | 1.74 | 11% | 6.44 | 10.51 | 14.84 | 20% | 6.48 | 10.04 | 13.53 | 18% | |||
PR | 1.25 | 1.45 | 1.71 | 8% | 7.57 | 11.70 | 15.61 | 18% | 8.18 | 12.17 | 14.42 | 14% | |||
FR | 1.49 | 1.68 | 1.79 | 4% | 5.97 | 8.75 | 11.21 | 15% | 7.77 | 11.94 | 14.88 | 16% | |||
FTR | 1.64 | 1.68 | 1.71 | 1% | 8.64 | 9.66 | 10.57 | 5% | 13.01 | 14.4 | 15.51 | 4% |
Cs | Ks | Kb | Vb | Vd | Cd | Z0 | X0 |
1.23 | 8.39 | 9.23 | 17.77 | 23.0 | 0.12 | 1.23 | 1.23 |
HD | Ks | Kb | ||||||||
l | m | u | stdmean | l | m | u | stdmean | |||
SP | 1.83 | 5.93 | 15.62 | 50% | 6.22 | 9.28 | 17.8 | 28% | ||
MP | 6.22 | 7.48 | 9.09 | 10% | 7.62 | 9.27 | 11.82 | 11% | ||
CP | 6.01 | 6.97 | 8.06 | 8% | 8.44 | 9.64 | 11.07 | 7% |
HD | Cs | Ks | Kb | ||||||||||||
l | m | u | stdmean | l | m | u | stdmean | l | m | u | stdmean | ||||
SP | 0.86 | 1.13 | 1.48 | 14% | 3.94 | 6.85 | 10.52 | 24% | 5.76 | 8.32 | 11.19 | 17% | |||
MP | 1.14 | 1.27 | 1.5 | 7% | 4.39 | 7.23 | 10.94 | 23% | 7.51 | 8.99 | 10.78 | 9% | |||
CP | 1.16 | 1.31 | 1.55 | 8% | 3.97 | 6.36 | 9.7 | 22% | 8.31 | 9.47 | 10.79 | 7% |
HD | Cs | Ks | Kb | ||||||||||||
l | m | u | stdmean | l | m | u | stdmean | l | m | u | stdmean | ||||
SP | 0.86 | 1.15 | 1.5 | 14 % | 3.49 | 6.63 | 10.75 | 28% | 5.61 | 8.37 | 11.4 | 18% | |||
MP | 1.14 | 1.27 | 1.51 | 7 % | 4.24 | 7.21 | 11.09 | 24 % | 7.54 | 9.05 | 10.9 | 9 % | |||
CP | 1.16 | 1.31 | 1.56 | 8 % | 3.89 | 6.21 | 9.59 | 23 % | 8.32 | 9.47 | 10.75 | 6 % |
HD | Cs | Ks | Kb | |||||||||||||
l | m | u | stdmean | l | m | u | stdmean | l | m | u | stdmean | |||||
SP | None | 0.87 | 1.13 | 1.49 | 14% | 3.82 | 6.81 | 10.36 | 24% | 5.80 | 8.31 | 11.14 | 16% | |||
1 hour | 1.00 | 1.25 | 1.56 | 9% | 4.81 | 7.31 | 10.78 | 22% | 6.87 | 9.63 | 12.04 | 11% | ||||
2 hour | 1.00 | 1.18 | 1.46 | 10% | 4.55 | 7.46 | 10.96 | 22% | 6.88 | 8.99 | 11.07 | 12% | ||||
4 hour | 1.08 | 1.21 | 1.39 | 6% | 4.84 | 7.26 | 10.35 | 19% | 7.55 | 9.20 | 11.01 | 9% | ||||
MP | None | 1.14 | 1.27 | 1.50 | 7% | 4.34 | 7.27 | 10.97 | 23% | 7.54 | 9.02 | 10.76 | 9% | |||
1 hour | 1.14 | 1.25 | 1.44 | 6% | 4.68 | 7.72 | 11.33 | 21% | 7.5 | 8.97 | 10.81 | 9% | ||||
2 hour | 1.15 | 1.26 | 1.44 | 6% | 4.70 | 7.55 | 10.99 | 21% | 7.57 | 8.98 | 10.75 | 9% | ||||
4 hour | 1.15 | 1.22 | 1.33 | 4% | 5.72 | 8.34 | 11.24 | 17% | 7.57 | 8.99 | 10.71 | 9% | ||||
CP | None | 1.15 | 1.31 | 1.55 | 8% | 3.92 | 6.26 | 9.54 | 23% | 8.34 | 9.46 | 10.66 | 6% | |||
1 hour | 1.15 | 1.26 | 1.41 | 5% | 5.16 | 7.38 | 10.16 | 15% | 8.28 | 9.46 | 10.73 | 7% | ||||
2 hour | 1.17 | 1.27 | 1.41 | 5% | 5.04 | 6.96 | 9.39 | 16% | 8.35 | 9.47 | 10.76 | 7% | ||||
4 hour | 1.16 | 1.23 | 1.32 | 3% | 5.82 | 7.59 | 9.68 | 13% | 8.42 | 9.56 | 10.83 | 6% |
HD | Cs | Ks | Kb | ||||||||||||
l | m | u | stdmean | l | m | u | stdmean | l | m | u | stdmean | ||||
SP | 1.15 | 1.26 | 1.38 | 5% | 4.58 | 7.1 | 10.15 | 20% | 6.59 | 8.73 | 11.11 | 13% | |||
MP | 1.21 | 1.29 | 1.38 | 3% | 5.14 | 6.77 | 9.18 | 15% | 8.06 | 9.11 | 10.36 | 6% | |||
CP | 1.19 | 1.27 | 1.37 | 4% | 5.26 | 6.83 | 8.79 | 13% | 8.43 | 9.56 | 10.81 | 6% |