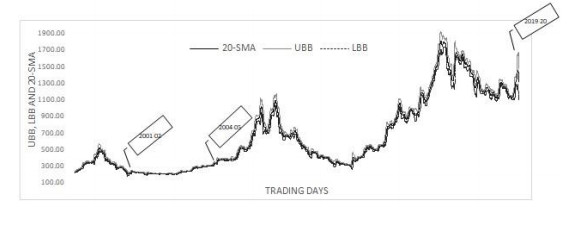
An investor uses the graphical presentation of Bollinger Bands to get signals of the ups and downs, as well the volatility of the market from the expansion and tightening of the UBB and LBB, reflecting higher and lower volatility. The percent (%) b helps determine the opportunities during extreme periods from the market, looking at the concentration of line graph at the value "0" or "1" reflecting the bearish and bullish trend, respectively. The Bandwidth Index was able to picture out the bullish trend with a squeeze at the upper band. The positive unimodality of Q for NEPSE daily return for the period of the fiscal year 1998–1999 to the fiscal year 2019–2020 indicated normality for the market return. Nevertheless, the results for the trading signals based on the Bollinger bands are seen as useful for an investor by giving a clear signal to "buy" or "sell". At the same time, relying only on Bollinger Bands with a specific period MA, i.e. the Bollinger Bands with a shorter moving average (MA) shows higher fluctuations and vice-versa, hence, could show false signals while choosing inappropriate MA, therefore, help of other technical analysis tools should be taken while going for an investment decision.
Citation: Rashesh Vaidya. NEPSE in Bollinger Bands[J]. National Accounting Review, 2021, 3(4): 439-451. doi: 10.3934/NAR.2021023
[1] | Jawad Saleemi . COVID-19 and liquidity risk, exploring the relationship dynamics between liquidity cost and stock market returns. National Accounting Review, 2021, 3(2): 218-236. doi: 10.3934/NAR.2021011 |
[2] | Mustafa Tevfik Kartal . Do activities of foreign investors affect main stock exchange indices? Evidence from Turkey before and in time of Covid-19 pandemic. National Accounting Review, 2020, 2(4): 384-401. doi: 10.3934/NAR.2020023 |
[3] | Changqing Luo, Zijing Li, Lan Liu . Does investor sentiment affect stock pricing? Evidence from seasoned equity offerings in China. National Accounting Review, 2021, 3(1): 115-136. doi: 10.3934/NAR.2021006 |
[4] | Mohammad Reza Abbaszadeh, Mehdi Jabbari Nooghabi, Mohammad Mahdi Rounaghi . Using Lyapunov's method for analysing of chaotic behaviour on financial time series data: a case study on Tehran stock exchange. National Accounting Review, 2020, 2(3): 297-308. doi: 10.3934/NAR.2020017 |
[5] | Dev Gupta, Akanksha Parikh, Tapan Kumar Datta . A multi-criteria decision-making approach to rank the sectoral stock indices of national stock exchange of India based on their performances. National Accounting Review, 2021, 3(3): 272-292. doi: 10.3934/NAR.2021014 |
[6] | Nikolaos T. Giannakopoulos, Damianos P. Sakas, Nikos Kanellos, Christos Christopoulos . Web analytics and supply chain transportation firms' financial performance. National Accounting Review, 2023, 5(4): 405-420. doi: 10.3934/NAR.2023023 |
[7] | Luccas Assis Attílio . Impact of Chinese financial shocks: A GVAR approach. National Accounting Review, 2024, 6(1): 27-49. doi: 10.3934/NAR.2024002 |
[8] | Jawad Saleemi . In COVID-19 outbreak, correlating the cost-based market liquidity risk to microblogging sentiment indicators. National Accounting Review, 2020, 2(3): 249-262. doi: 10.3934/NAR.2020014 |
[9] | Yanting Xu, Tinghui Li . Measuring digital economy in China. National Accounting Review, 2022, 4(3): 251-272. doi: 10.3934/NAR.2022015 |
[10] | Jiehua Ma, Zhenghui Li . Measuring China's urban digital economy. National Accounting Review, 2022, 4(4): 329-361. doi: 10.3934/NAR.2022019 |
An investor uses the graphical presentation of Bollinger Bands to get signals of the ups and downs, as well the volatility of the market from the expansion and tightening of the UBB and LBB, reflecting higher and lower volatility. The percent (%) b helps determine the opportunities during extreme periods from the market, looking at the concentration of line graph at the value "0" or "1" reflecting the bearish and bullish trend, respectively. The Bandwidth Index was able to picture out the bullish trend with a squeeze at the upper band. The positive unimodality of Q for NEPSE daily return for the period of the fiscal year 1998–1999 to the fiscal year 2019–2020 indicated normality for the market return. Nevertheless, the results for the trading signals based on the Bollinger bands are seen as useful for an investor by giving a clear signal to "buy" or "sell". At the same time, relying only on Bollinger Bands with a specific period MA, i.e. the Bollinger Bands with a shorter moving average (MA) shows higher fluctuations and vice-versa, hence, could show false signals while choosing inappropriate MA, therefore, help of other technical analysis tools should be taken while going for an investment decision.
Technical analysis is essentially the search for recurrent and predictable patterns in stock prices. The technique includes a variety of forecasting such as chart analysis, pattern recognition analysis, seasonality and cycle analysis, computerized technical systems. It is the study of past price movements to predict future price movements from the past. Ibrahim (2013) stated that chartists believe that everything that could influence the stock prices are already reflected in the historical stock prices, and are widely used by traders and financial professionals. Nevertheless, he concluded that the results have limited theoretical justifications and, their efficiency is questionable.
Technical analysts use different types of technical analysis tools to predict the market trend using historical data. A technical analyst has been developing tools to predict market trends. They believe that the stock price and market follows the repetitive pattern, which allows investors to use past data to predict the future trend of the market. Hence, a technical analyst has been developing tools to predict market trends.
John A. Bollinger, a financial analyst, in the early 1980s, first introduced the concept of Bollinger Bands. Bollinger (1992) discussed the envelope or trading bands that can provide the buying or selling signals to investors with the help of MA and deviation from the MA. The Bollinger Bands strategy does not chase momentum as by MA strategy, as it tries to recognize the point where either an upward trend or downward trend ends. At the same time, the length of time for MA also affects on providing trading signals with the help of the Bollinger Bands. If the shorter MA is taken, higher fluctuations are captured reflecting higher trading signals and vice versa. Traders and academicians were concern about the effectiveness of the result from the Bollinger Bands. Hence, Leung and Chong (2003) thought about the profitability result from two technical analysis devices to be specific; moving average envelopes and Bollinger Bands. They found that Bollinger Bands could capture sudden price fluctuations but could not out-perform the moving average.
The paper initially gave a concept of generating the upper band and lower band using a simple moving average and standard deviation. Essentially, the paper additionally gave an understanding of the estimation of %b and bandwidth index. The paper is focused mainly on the volatility of the price.
An investor is always interested to get a clear signal of bullish or, a bearish trend from the stock market, especially, those who follow technical analysis techniques to predict the future market trend. At the same time, an investor should note that the Bollinger Band gives an insight into the market trend for a short-term period. Nevertheless, the paper has gone further ahead to identify the market volatility and trend identification with the help of Bandwidth Indicator, BWI and measure the normality of the return from the stock market with the help of Unimodality Q. This paper tries to find out how the Bollinger Bands give the signal of market volatility, a signal of a bullish and bearish trends, and how it provides the buy signal, sell signal or no signals to the investor, in context to NEPSE.
Bollinger Bands is a technical analysis tool introduced by John Bollinger in 1983 and brought into practice on April 4, 1989, as well as, a term trademarked by him on February 7, 2011, under Status 702, Section 8 and 15 of the US Trademark Application. Bollinger Bands, along with indicators %b and bandwidth index (BWI), can be used to measure the "highness" or "lowness" of the price relative to previous trades. It evolved from the concept of trading bands. Similar to the Keltner channel, Bollinger Bands is also a volatility indicator. The Bollinger Band solely is not seen conclusive to bring out the best results while going for a better trading strategy. The empirical results have also shown that a combination of Bollinger Bands with other technical analysis tools could bring out better results.
Bollinger Bands consist of:
● an N-period moving average (MA)
● an upper band at K times an N-period standard deviation above the moving average (MA + Kσ)
● a lower band at K times an N-period standard deviation below the moving average (MA – Kσ)
Typical values for N and K are 20 and 2, respectively. The default choice for the average is a simple moving average, but other types of averages can be employed as needed. An exponential moving average is a common second choice. Usually, the same period is used for both the middle band and the calculation of standard deviation.
The purpose of Bollinger Bands (BB) is to provide a relative definition of high and low. By definition, prices are high at the upper band and low at the lower band. This definition can aid in rigorous pattern recognition and is useful in comparing price action to the action of indicators to arrive at systematic trading decisions. Similarly, the Upper Band could be used as a resistance level, and the Lower Band could be used as a support level.
In spring, 2011, John Bollinger introduced three new indicators based on Bollinger Bands. They are Bollinger Bands Impulse (BB Impulse), which measures price change as a function of the bands; percent bandwidth (%b), which normalizes the width of the bands over time; and bandwidth delta, which quantifies the changing width of the bands.
An application for %b incorporates framework building and recognition of the pattern. So also, the utilization for bandwidth incorporates identification of chances emerging from relative extremes in instability and pattern identification.
A utilization of Bollinger Bands varies generally among traders. A few traders purchase the stock when value contacts the lower Bollinger Band, and leave when value contacts the moving average in the middle point of the bands. Some traders purchase, when value breaks over the upper Bollinger Band or, sell when the value falls underneath the lower Bollinger Band. Also, the utilization of Bollinger Bands isn't limited to stock traders, options dealers, most outstandingly inferred unpredictability traders, regularly sell alternatives, when Bollinger Bands are generally far separated or, purchase choices. At the point when the Bollinger Bands are historically near one another, in the two examples, anticipating that unpredictability should return towards the average historical volatility level for the stock.
A period of low volatility is indicated when the bands lie close together. Conversely, an increase in price action/market volatility is indicated as the bands expand. The price will generally be found to oscillate between the bands as though in a channel when the bands have only a slight slope and track approximately parallel for an extended time.
Traders are often inclined to use Bollinger Bands with other indicators to confirm price action. In particular, the use of oscillator-like Bollinger Bands will often be coupled with a non-oscillator indicator-like chart pattern or a trend-line. If these indicators confirm the recommendation of the Bollinger Bands, the trader will have greater conviction that the bands are predicting correct price action about market volatility (Baiynd, 2011).
Bollinger Bands work best to indicate the beginning of trends when the upper and lower bounds are crossed. When a security's price reaches above the upper band, it is considered overbought and below the lower limit means the security is oversold (Leung & Chong, 2003). An overbought security should be sold or shorted and an oversold security should be purchased. When a price travels above the upper-line, the market tends to overcompensate and go too far in the other direction which can cause a rebound to quickly occur. This quick reaction allows for more trades to occur (Williams, 2013). As a result, they typically have more success in actively managed funds that can react to the overcompensation and they trade better in trending markets rather than horizontal. Additionally, Bollinger Bands may be better suited for commodities trading or exchange rate trading, where "over 90% of them… traders use technical analysis in their trading decisions" (Chen et al., 2014).
An indicator %b tells where the moving average is within the bands. Unlike stochastic that are bounded by 0 and 100 %b can assume negative values and, values above 100, when prices are outside of the bands. At 100, it is at the upper band, at 0 at the lower band, above 100, it is above the upper band and, below 0, and it is below the lower band (Bollinger, 1992).
Bandwidth, another indicator derived from Bollinger Bands, may also interest traders. It is the width of the bands expressed as a percent of the moving average. When the bands narrow drastically, a sharp expansion in volatility usually occurs in the very near future (Bollinger, 1992).
The trading rules for the results of Bollinger Bands are illustrated as:
Buy Rule: Closing Index (t) > BBLN(t)
Sell Rule: Closing Index (t) < BBUN(t)
A buy signal is generated when the closing index (price) crosses the lower band from below and then a sell signal will be generated when the closing index (price) penetrates the upper band from above (William, 2006).
One of the defining features is that they adjust in size which helps accounts for price volatility around the moving average. The bands tighten during times of higher stability and grow larger with higher volatility (Kirkpatrick and Dahlquist, 2015).
Other than the above strategies i.e. riding the bands, an investor can stay at the middle of the bands, i.e. at the point of 20-SMA and get out of the market. In other words, squeezing of the bands foreshadows a big move to an investor. In addition to Bollinger Band, an investor should adopt the candlestick chart to point out the double-top, double-bottom or, reversal trading strategy.
Leung and Chong (2003) compared the profitability of Moving Average Envelopes and Bollinger Bands. Although Bollinger Bands can capture quick market fluctuations that Moving Average Envelopes cannot, the study had shown that Bollinger Bands do not outperform Moving Average Envelopes.
Liu et al. (2006) found that the Black-Scholes stock price model has similar properties, that of the Bollinger Bands, i.e. widening when the market is volatile and vice versa. They came out with a new distribution of statistics, with the help of the concept of Bollinger Band.
Lento and Gradojevic (2007) tested five different technical analysis tools S & P/TSX 300 Index, DJIA, NASDAQ Composite Index, and Canada and US spot exchange rate, and found that the Bollinger Bands performed poorly and misleading the signals. They further found that even after adjusting the transaction cost, the Bollinger Bands was not seen as highly profitable. Lento et al. (2007) applied the concept of Bollinger Bands, with variations in the moving average, on the three leading stock markets and one foreign exchange trade, to see the informative effects in the market. They found that the Bollinger Bands are statistically inconsistent in producing better returns over the buy-hold strategy. Similarly, Balsara et al. (2007) examined the random walk model and technical trading rules in Chinese stock markets namely, Shanghai and Shenzhen stock markets. They found that the Bollinger Bands breakout rule can bring out better return for an individual stock.
Evans (2008) conducted the test on the S & P 500 data using Bollinger Bands basic calculation, %b oscillators and concluded that a rotational trading algorithm using relative %b rankings could select stock portfolios that beat the risk-adjusted return on the market.
Kabansinskas and Macys (2010) looked at trading Bollinger Bands in the Baltic market to establish the optimal parameters for the variables. As mentioned before, the value for N is 20, and K is 2. However, the study looked at the ideal parameters for short-term, as well as, long-term investing. They concluded that for indicating short-term trends, N should be set to 10 and K to 1.9. Setting the variables lower for short-term trading makes the metric more sensitive and, the security is more prone to exceeding or dropping below the bounds, sending more trading signals. Decreasing N decreases the days in the average so, each day carries more weight in the SMA, and therefore, the standard deviations. For long-term investing, they found the optimal parameters for N to be 50 and K to be 2.1. This is opposite to the short-term trading variables by increasing the standard deviations requirements for a signal and therefore helps eliminate false signals.
Kannan et al. (2010) adopted typical price, Chaikin money flow indicator, stochastic momentum index, relative strength index, moving average, and Bollinger Bands to discover the predicting capacity for the return from the stock market. They concluded that stochastic momentum, the best tool, followed by the Bollinger Bands with an accuracy of about 85 percent, gives a profitability signal from the historical data of the stock market.
Lee et al. (2013) constructed lower and upper bounds within which future values of the stochastic process run at a fixed probability level. During the short-selling of the stock, the bounds constructed were termed as price-bands or trading-bands. In the paper, they came out with a mathematical model for the construction of price bands using a stochastic programming formulation.
Chen et al. (2014) used the GARCH (1, 1) with Bollinger Bands to determine the distribution nature of the market return. The study found that the combination helped in producing a better performance for the results from the Bollinger Bands. Fang et al. (2014) found the results from the Bollinger Band as a part of the trading strategy in most of the international stock markets with the gradually declining popularity after the year 2001. Similarly, Lim et al. (2014) looked at the profitability of a trading rule based on Bollinger Bands in six different equity markets using large-cap indices, namely; CAC, DAX, FTSE, HSI, KOSPI, and NIKKEI. Besides, they also explored the performance of a trading strategy based on a combined signal approach with Bollinger Band signals; filtered using the ADX to avoid trending markets. They found evidence to support the use of Bollinger Bands for tactical trades over short time horizons, with a strong-positive skewed distribution for the returns. When comparing the performance of Bollinger bands with the strategy augmented by the ADX, they found the low-performance improvement when applied on a systematic basis as an initial filter. However, they suggested applying the ADX on a discretionary basis to limit losses.
In context to Indian listed companies, Shah and Patel (2015) found that the Bollinger Bands outperformed than Relative Strength Index (RSI) and SMA (Simple Moving Average) to find out the buy and sell signals as well as profitability from specific security. Similarly, Pushpa et al. (2017) found that Bollinger Bands help to give a strong buy signal and sell signal with other technical analysis tools like SMA, MACD, and RSI.
Similarly, Prasad et al. (2018) tested the volatility of the share prices of the six commercial banks of India using the Bollinger Bands. Based on the widening and tightness of the upper and lower bands, the paper found only two sample banks with less volatility among the sampled banks.
Chen et al. (2018) applied the concept of Bollinger Bands with wavelet analysis on stock index future CSI 300 of China to determine the trading strategy for an investor with a greater return, less risky and, higher applicability. They found that the combination of Bollinger Bands with wavelet analysis prevents a false break during transactions.
Khairi et al. (2019) found that technical analysis tools like stochastic oscillators, Moving Average Convergent Divergent (MACD), Bollinger Bands (BB), and Relative Strength Index (RSI) were seen significant in predicting the stock price trend for a shorter run only. Hence, to get an accurate prediction of the market trend, suitable fundamental analysis techniques, and machine learning techniques helped an investor.
Pramudya and Ichsani (2020) conducted a comparative study to determine the accuracy of buy and sell signals on the Indonesian stock market, IDX LQ45, provided by Moving Average Convergent Divergent (MACD), Bollinger Bands, and Relative Strength Index (RSI). They found that the Bollinger Bands and the MACD indicators captured sell signals better than the RSI. They also found that MACD was much slower to give a sell signal than the Bollinger Bands and, statistically significant RSI, thus an investor should be highly cautious in getting an accurate result.
Mkik and Menzhi (2020) revealed that the Bollinger Bands is not profitable. The various signals under Bollinger Bands are consistently underperforming the buyer's trading strategy and the hold. After adjusting for transit costs, the Bollinger Bands are only cost-effective for 2 out of 12 tests. Furthermore, no particular Bollinger Bands variant indicated robust and superior performance. Although the reason for the use of the BB oscillator (i.e., to sell when the market is overbought tainted when the market is oversold), when a contrary approach was used, the profitability of the BB has been improved impressive (i.e., buy on a sell signal and sell on a buy signal).
Ni et al. (2020) explored the Taiwan 50 whether an investor could beat the market by trading with the support of signals provided by the Bollinger Bands. The study revealed that the market could be beaten by taking a long position on stocks lying on the lower band, providing a significantly abnormal profit. The study also found that an investor should take a long position when the price reaches the upper band.
Chandima (2021) stated that the Bollinger Bands are used for the volatility evaluation of the stock market. The study found that the squeezing of the bands signals the start of a new move in the market. While testing the Bollinger Bands in Colombo Stock Exchange, the researcher found that the bands of Bollinger Bands could be viewed as support and resistance for the market. Similarly, Singh et al. (2021) found that the Bollinger Bands as a valuable tool for traders to measure volatility position and guide them into when to enter the market and leave a position.
The paper has followed the exploratory research design, as it tries to find out the new and unexplored side of the use of one of the technical analysis tools, namely, Bollinger Bands in Nepal Stock Exchange Limited (NEPSE). For the data analysis purpose, the paper has used the daily closing index recorded in the only secondary market of Nepal Stock Exchange Limited (NEPSE). The daily NEPSE Index is calculated based on the amount of market capitalization during a specific day. The daily indices have been considered of twenty-two (22) years from fiscal year (F/Y) 1998–1999 to the fiscal year (F/Y) 2019–2020. The fiscal year in Nepal starts in mid-July according to the Georgian calendar.
The paper initially calculated the 20-SMA for the daily NEPSE Index of the sample periods. Then, the Upper Bollinger Band (UBB) and Lower Bollinger Band (LBB) have been calculated as defined by Bollinger (1992). The calculated value was then plotted to get a glimpse of the market volatility. Similarly, the value of %b (percent b) and Bollinger BWI (Bandwidth Index) was calculated to determine the volatility signal from the NEPSE.
Writing upper band for the upper Bollinger Band, lower band for the lower Bollinger Band, and close for the closing (price) value:
%b = (Close − Lower Band) / (Upper Band − Lower Band)
Bandwidth tells how wide the Bollinger Bands are on a normalized basis. Writing the same symbols as before, and middle band for the moving average, or middle Bollinger Band:
Bandwidth = (Upper Band − Lower Band)/Middle Band
Using the default parameters of a 20-period simple moving average and, plus/minus two (2) standard deviations, bandwidth is equal to four times the 20-period coefficient of variation (Bollinger, 2001).
A popular indicator derived from the Bollinger Bands is the Bandwidth Indicator, BWI. It can be calculated as:
BWIt=UBδt−LBδtMAδt | (1) |
where, UBδt = Upper band at time "t" with a size of "δ"
LBδt = Lower band at time "t" with a size of "δ"
MAδt = Moving average for time "t" with a size of "δ"
Similarly, the estimated density of Q or unimodality of Q can be calculated as:
Q=∫10B2sds−(∫10B2sds)2 | (2) |
where, "Bs" is the Bandwidth indicator i.e. BWI.
For the normal distribution or a unimodal distribution, a positive value of "Q" states the strong unimodality and for the negative value, vice-versa (Ibrahim, 2013).
The concept of definite integral calculus has been followed for calculating the value of Q, where integral of "Bs" is from 1 to 0 and, the arbitrary constant "c" is absent. Here, B2s is called the integrand, 1 is called the upper limit, and 0 is called the lower limit of integration.
At the same time, the value of UBB and LBB were compared with the NEPSE Index (Closing) to determine the buy signal, sell signal, or no signal as defined by William (2006).
Figure 1 shows the movement of the Upper Bollinger Band (UBB) and Lower Bollinger Band (LBB) as well as Simple Moving Average (SMA). The figure will also help to state whether the market is at a bullish trend or in the bearish trend. As the SMA line passes between the UBB and LBB lines, the market can be stated as stable. But as the SMA line passes above the UBB, the trend market follows will be the bullish and vice-versa if passes below the LBB.
At the same time, when the bands, i.e. UBB and LBB lie close together, a period of low volatility is indicated. Conversely, as the bands expand, an increase in price action/market volatility is indicated. When the bands have only a slight slope and track approximately parallel for an extended time, the price will generally be found to oscillate between the bands as though in a channel.
The Figure 1 shows the band was tightly closed indicating low volatility (trading days of the year 2001–2002 to 2004–2005) and at the same time, the low parallel which indicates no visible market fluctuation. During this period only, the SMA is hardly moving between the UBB and LBB. When the LBB and UBB are seen parallel, the traders are interested to sell at the point of UBB and buy at the point of LBB.
The 20-SMA is seen closer to the UBB at the end of the year 2020 as the market closed with the bullish signal. Though, the market had shown the signal of high volatility, moving closer to lower band most of the days or, being tighter between the bands.
%b (pronounced "percent b") is derived from the formula for Stochastic and shows where price is in relation to the bands. %b equals "1" at the upper band and "0" at the lower band. The graphical presentation and the calculation value of %b is equal to the value 1 reflects the market is in bullish trend and when the calculation value of %b is equal to the value 0 reflects the market is in bearish trend. The result of the %b also helps to determine the market fluctuation is within the UBB and LBB of the Bollinger Band. Hence, it is used only for market pattern recognition. The figure below shows the plotted value of %b for NEPSE.
Under the Bollinger Band analysis, %b is one of the common indicators to see the where the market price is being positioned during a specific time period. When the %b graph is highly dense at the upper level, the market shows the bullish trend and vice-versa. In other words, if the graph moves closer to the value "1", the market is seen in the bullish trend and if the graph moves closer to the value "0", the market is seen in the bearish trend.
Bollinger Bandwidth Indicators (BWI) tells how wide the Bollinger Bands are on a normalized basis. It is the value of difference between the UBB and LBB divided by the MA for the specific time period. The main motto of plotting the value of BWI is to capture the opportunities from the extremes in market volatility and trend identifications.
The BWI shows how the market is taking its movement. This shows how the upper-band and lower-band are moving in a normalized basis i.e. ups and downs of the market. If the BWI is seen highly fluctuating, it can be stated that the market is ready for fluctuation. The market needs to show some fluctuation as it is a natural but abnormal fluctuation bring volatility to the market. If the BWI shows the regular squeezes at upper point, followed by a break, then the market tends to reflect the bullish trend and vice versa. A limited number of upper point extreme squeezes were seen during the study period showing few number bullish trends in NEPSE which ultimately brought volatility in the market.
For the normal distribution, a positive value of "Q" states the strong unimodality and for the negative value, vice-versa. Simply, unimodality of "Q" is the estimated density of the value "Q".
Instead of the finding of 95% of the data inside the bands, as would be the expectation with the default parameters if the data were normally distributed, studies have found that only about 88% of security prices (85%–90%) remain within the bands. Thus, the unimodality of Q is being calculated to see the exact distribution of the MA. As the values of unimodality of Q for the past twenty-two years are seen all positive, which state the strong unimodality for the NEPSE daily index.
Table 1 shows the number of buy signals and sell signals shown by Bollinger Bands for NEPSE.
Description | Number of Observations |
Total Trading Days | 4856 |
Buy Signals | 437 |
Sell Signals | 302 |
Source: Annex. |
More number of strong "buy signals" was seen provided from the Bollinger Bands analysis for the NEPSE than the "sell signals" during the observation period.
A buy signal is generated when the closing index (price) crosses the lower band from below and then a sell signal will be generated when the closing index (price) penetrates the upper band from above.
This shows that the daily closing NEPSE index were at higher level than the lower Bollinger Bands for more time periods during the period of F/Y 1998–1999 to F/Y 2019–2020. The buy and sell signals provided from the Bollinger Bands, also helps an investor to determine an entry or an exit strategy.
The graphical plotting of the Upper Bollinger Band (UBB) and Lower Bollinger Band (LBB) with the 20-SMA or, 20-EMA helps to visualize the stock market volatility. As the UBB and LBB tighten closer, the stock market reflects lesser volatility and vice versa. The graphical presentation of the NEPSE Index (Daily Closing) reflected a short period of market volatility during the study period of F/Y 1998–1999 to F/Y 2019–2020. The deviation in the Nepalese market from the upper and lower bands was so minimal, that the interpretation from the Bollinger Bands was not productive.
The result from the %b (percent b) reflected the market trend for NEPSE. When the plotted graph for the value of %b becomes denser at the value of "1", it reflected bullish, and when the graph for %b becomes denser at the value of "0", it reflected the market is a bearish trend.
The model developed for unimodality was put in question for its operational efficiency in the real market. The BWI, as well as, the unimodality indicator, is used to detect the change time at which the volatility changes its value, and the traders do not perfectly detect the change time. The BWI for daily NEPSE index clearly reflected a very low number of bull signals during the period of F/Y 1998–1999 to F/Y 2019–2020 with very few numbers of squeezes of BWI at the upper point, i.e., closer to the value of "1". Thus, only the detection strategies while investing in the Nepalese stock market could be applied to diffuse the volatility. Nevertheless, the trading signal calculation results based on the Bollinger Bands were seen as fruitful in context to the Nepalese stock market. Hence, the technique tested in the Nepalese stock market brought effective results that would be useful to an investor for finding out the level of stability of the market. Furthermore, support of one or more technical analysis tools result could be used jointly with the Bollinger Bands to reduce the number of false signals, minimize the risk, and enhance the better return from the market.
I would like to acknowledge University Grant Commission, Nepal for providing me financial support in due course of conducting this research work.
The author declares no conflicts of interest in this paper.
[1] | Baiynd AM (2011) The Trading Book: A Complete Solution to Mastering Technical Systems and Trading Psychology, New York: McGraw Hill. |
[2] | Balsara NJ, Chen G, Zheng L (2007) The Chinese stock market: An examination of the random walk model and technical trading rules. Qtly J Bus Eco 46: 43–63. |
[3] | Bollinger J (1992) Using Bollinge Bands. Techn Anal Stks Commit 10: 47–51. |
[4] | Bollinger J (2001) Bollinger on Bollinger Bands, New York: McGraw Hill Education. |
[5] | Chandima AVL (2021) Use stock market data to assist investors on getting more accurate transaction decision. Intl J Sci Res Pub 11: 762–770. |
[6] | Chen SL, Chen NJ, Chuang RJ (2014) An empirical study on technical analysis: GARCH (1, 1) model. J Appl Stat 41: 785–801. |
[7] | Chen S, Zhang B, Zhou G, et al. (2018) Bollinger Bands trading strategy based on wavelet analysis. Appl Econ Financ 5: 49–58. |
[8] | Evans HP (2008) An empirical study of rotational trading using the %b oscillator. J Techn Anal 65: 35–41. |
[9] | Fang J, Jacobsen B, Qin Y (2014) Popularity versus profitability: Evidence from Bollinger Bands. SSRN Electro J, 1–45. |
[10] | Ibrahim D (2013) Etude théorique d'indicateurs d'analyse technique [Theoretical Study of Technical Analysis Indicators].Université Nice Sophia Antipolis, Finance Quantitative Français: HAL archives-ouvertes. |
[11] | Kabasinskas A, Macys U (2010) Calibration of Bollinger Bands parameters for trading strategy development in the Baltic stock market. Inzinerine Ekonomika-Eng Econ 21: 244–254. |
[12] | Kannan KS, Sekar PS, Sathik MM, et al. (2010) Financial Stock Market Forecast using Data Mining Techniques. Proceedings of the Intl Multi Conf of Eng and Computer Scientists (IMECS), I, March 17–19, 1–5. |
[13] | Khairi TWA, Zaki RM, Mahmood WA (2019) Stock price prediction using technical, fundamental and news based approach. 2nd Sc. Conf of Computer Sci (SCCS), March 27–28,171–181. |
[14] | Kirkpatrick CD, Dahlquist JR (2015) Technical Analysis: The Complete Resource for Financial Market Technicians, New Jersy: Pearson Education, Inc. |
[15] | Lee J, Lee J, Prxekopa A (2013) Price-Bands: A Technical Tool for Stock Trading, New Jersy: Rutgers University. |
[16] | Lento C, Gradojevic N (2007) The profitability of trading rules: A combined signal approach. J Appl Bus Res 23: 13–28. |
[17] | Lento C, Gradojevic N, Wright CS (2007) Investment information content in Bollinger Bands? Appl Financ Econ Lets 3: 263–267. |
[18] | Leung JM, Chong TT (2003) An empirical comparison of moving average envelops and Bollinger Bands. Appl Econ Lets 10: 339–341. |
[19] | Lim S, Hisarli TT, Shi He N (2014) The profitability of a combined signal approach: Bollinger Bands and the ADX. Intl Fed Techn Anal J (IFTA Journal) 14: 30–36. |
[20] | Liu W, Huang X, Zheng W (2006) Black-Scholes' model and Bollinger Bands. Phys A 371: 565–571. |
[21] | Mkik M, Menzhi K (2020) The profitability of chartist analysis: Case of the Bollinge Bands indicator. IOSR J Econ Financ 11: 30–34. |
[22] | Ni Y, Day MY, Huang P, et al. (2020) The profitability of Bollinger Bands: Evidence from the constituent stocks of Taiwan. Phys A 551: 124144. |
[23] | Pramudya R, Ichsani S (2020) Efficiency of technical analysis for stock trading. Intl J Financ Bank Stud 9: 58–67. |
[24] | Prasad TD, Chaitanya C, Kumar AT (2018) A study on stock's volatility in banking sector using technical analysis. Intl Res J Eng Tech 05: 1016–1022. |
[25] | Pushpa BV, Sumithra CG, Hegde M (2017) Investment decision making using technical analysis: A study on select stocks in Indian stock market. IOSR J Econ Financ 19: 24–33. |
[26] | Rachev ST, Menn C, Fabozzi FJ (2005) Fat Tailed and Skewed Asset Return Distributions, Implications for Risk Management, Portfolio Selection, and Option Pricing, New York: John Wiley. |
[27] | Shah NP, Patel TM (2015) A comparative study on technical analysis by Bollinger Band and RSI. Intl J Mgmt Soci Sci 03: 234–251. |
[28] | Singh A, Gupta P, Thakur N (2021) An emprical research and comprehensive analysis of stock market prediction using machine learning and deep learning techniques. Intl Conf Comput Res and Data Analyt (ICCRDA 2020), IOP Conf Ser.: Mater. Sci. Eng., 10220(12098), October 24, 1–13. |
[29] | Williams B (2013) Volatility-Based Support Trading. Futures: News, Analysis & Strategies for Futures. Opts Derivs Traders 42: 20–22. |
[30] | Williams OD (2006) Emprical Optimization of Bollinger Bands for Profitability, Bumaby: Simon Fraser Univeristy. |
1. | Woojin Hong, Seong Cheon Choi, Seungwon Oh, Sibarama Panigrahi, Parametric seasonal-trend autoregressive neural network for long-term crop price forecasting, 2024, 19, 1932-6203, e0311199, 10.1371/journal.pone.0311199 | |
2. | Nhat M. Nguyen, Minh Tran, Simultaneous multi-objective optimization method for trading indicators, 2024, 26667207, 100501, 10.1016/j.rico.2024.100501 |
Description | Number of Observations |
Total Trading Days | 4856 |
Buy Signals | 437 |
Sell Signals | 302 |
Source: Annex. |