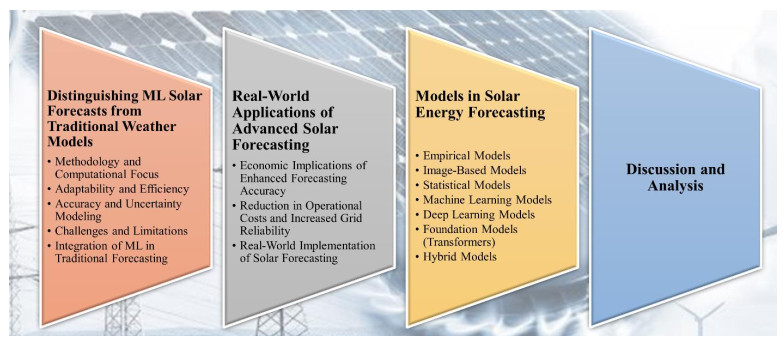
A useful family of integral operators and special functions plays a crucial role on the study of mathematical and applied sciences. The purpose of the present paper is to give sufficient conditions for the families of integral operators, which involve the normalized forms of the generalized Lommel functions of the first kind to be univalent in the open unit disk. Furthermore, we determine the order of the convexity of the families of integral operators. In order to prove main results, we use differential inequalities for the Lommel functions of the first kind together with some known properties in connection with the integral operators which we have considered in this paper. We also indicate the connections of the results presented here with those in several earlier works on the subject of our investigation. Moreover, some graphical illustrations are provided in support of the results proved in this paper.
Citation: Ji Hyang Park, Hari Mohan Srivastava, Nak Eun Cho. Univalence and convexity conditions for certain integral operators associated with the Lommel function of the first kind[J]. AIMS Mathematics, 2021, 6(10): 11380-11402. doi: 10.3934/math.2021660
[1] | Konstantinos X Soulis, Evangelos E Nikitakis, Aikaterini N Katsogiannou, Dionissios P Kalivas . Examination of empirical and Machine Learning methods for regression of missing or invalid solar radiation data using routine meteorological data as predictors. AIMS Geosciences, 2024, 10(4): 939-964. doi: 10.3934/geosci.2024044 |
[2] | Shuo Yang, Dong Wang, Zeguang Dong, Yingge Li, Dongxing Du . ANN prediction of the CO2 solubility in water and brine under reservoir conditions. AIMS Geosciences, 2025, 11(1): 201-227. doi: 10.3934/geosci.2025009 |
[3] | Adeeba Ayaz, Maddu Rajesh, Shailesh Kumar Singh, Shaik Rehana . Estimation of reference evapotranspiration using machine learning models with limited data. AIMS Geosciences, 2021, 7(3): 268-290. doi: 10.3934/geosci.2021016 |
[4] | Viviane Pierrard, David Bolsée, Alexandre Winant, Amer Al-Qaaod, Faton Krasniqi, Maximilien Péters de Bonhome, Edith Botek, Lionel Van Laeken, Danislav Sapundjiev, Roeland Van Malderen, Alexander Mangold, Iva Ambrozova, Marek Sommer, Jakub Slegl, Styliani A Geronikolou, Alexandros G Georgakilas, Alexander Dorn, Benjamin Rapp, Jaroslav Solc, Lukas Marek, Cristina Oancea, Lionel Doppler, Ronald Langer, Sarah Walsh, Marco Sabia, Marco Vuolo, Alex Papayannis, Carlos Granja . BIOSPHERE measurement campaign from January 2024 to March 2024 and in May 2024: Effects of the solar events on the radiation belts, UV radiation and ozone in the atmosphere. AIMS Geosciences, 2025, 11(1): 117-154. doi: 10.3934/geosci.2025007 |
[5] | Manogaran Madhiarasan . Long-term wind speed prediction using artificial neural network-based approaches. AIMS Geosciences, 2021, 7(4): 542-552. doi: 10.3934/geosci.2021031 |
[6] | Alexander S. Potapov, Tatyana N. Polyushkina . Response of IAR frequency scale to solar and geomagnetic activity in solar cycle 24. AIMS Geosciences, 2020, 6(4): 545-560. doi: 10.3934/geosci.2020031 |
[7] | Paolo Dell'Aversana . Reservoir prescriptive management combining electric resistivity tomography and machine learning. AIMS Geosciences, 2021, 7(2): 138-161. doi: 10.3934/geosci.2021009 |
[8] | Paolo Dell'Aversana . Reservoir geophysical monitoring supported by artificial general intelligence and Q-Learning for oil production optimization. AIMS Geosciences, 2024, 10(3): 641-661. doi: 10.3934/geosci.2024033 |
[9] | Joseph J. Kelly, Christopher I. McDermott . Numerical modelling of a deep closed-loop geothermal system: evaluating the Eavor-Loop. AIMS Geosciences, 2022, 8(2): 175-212. doi: 10.3934/geosci.2022011 |
[10] | Paolo Dell’Aversana, Gianluca Gabbriellini, Alfonso Iunio Marini, Alfonso Amendola . Application of Musical Information Retrieval (MIR) Techniques to Seismic Facies Classification. Examples in Hydrocarbon Exploration. AIMS Geosciences, 2016, 2(4): 413-425. doi: 10.3934/geosci.2016.4.413 |
A useful family of integral operators and special functions plays a crucial role on the study of mathematical and applied sciences. The purpose of the present paper is to give sufficient conditions for the families of integral operators, which involve the normalized forms of the generalized Lommel functions of the first kind to be univalent in the open unit disk. Furthermore, we determine the order of the convexity of the families of integral operators. In order to prove main results, we use differential inequalities for the Lommel functions of the first kind together with some known properties in connection with the integral operators which we have considered in this paper. We also indicate the connections of the results presented here with those in several earlier works on the subject of our investigation. Moreover, some graphical illustrations are provided in support of the results proved in this paper.
In recent years, the urgency of combating global warming has driven countries worldwide to prioritize the development of renewable energy sources. Among these, photovoltaic (PV) energy has gained significant attention due to its environmental benefits, inexhaustible nature, and cost-effectiveness [1]. As a result, PV energy is expanding rapidly and is expected to play a central role in future energy systems. However, the variable nature of PV power generation, caused by factors such as weather conditions, cloud cover, and diurnal cycles, and the associated forecasting uncertainties present challenges for maintaining the stability of power systems. Accurate forecasting of PV output is essential to ensure power system security and operational efficiency [2].
Despite significant advancements, traditional empirical and physical models often struggle with capturing the complex, nonlinear patterns inherent in solar irradiance (SI) data. These methods can be limited by data quality, model interpretability, and the need for extensive computational resources. Furthermore, the integration of cloud cover data, a major source of solar variability, is a significant gap in improving forecasting accuracy. There is a pressing need for models that can handle these complexities and provide reliable forecasts across different geographical regions and varying weather conditions.
Recent advancements in predictive modeling have shown significant improvements in various fields. Efficient parameter flexible fire prediction algorithms based on machine learning and reduced order modeling techniques, deep-learning-based digital twins, and novel data-model integration schemes have significantly enhanced wildfire forecasting accuracy [3,4,5]. These innovations highlight the transformative potential of machine learning and deep learning techniques in addressing complex and nonlinear patterns in data.
Energy storage systems, while capable of storing excess energy, are often prohibitively expensive for widespread use. Therefore, precise PV power forecasting is critical for optimizing industry applications and grid management. Forecasting methods can be broadly categorized into physical, statistical, and ML-based approaches. Physical methods rely on atmospheric parameters like temperature, pressure, and wind, utilizing Numerical Weather Prediction (NWP) models to generate forecasts. While ML methods have demonstrated significant improvements in forecasting accuracy, they remain limited when cloud cover observations, a major source of solar variability, are not included in the models. This limitation highlights the importance of integrating cloud cover data to enhance the performance of ML-based forecasting models [6]. Data preprocessing and post-processing are essential in these methods to extract relevant features and filter out noise, thereby enhancing forecasting performance. Techniques such as classification, regression, clustering, and dimension reduction are commonly employed in the preprocessing stage [7].
The evolution of PV power forecasting has seen significant advancements transitioning from traditional empirical models to advanced empirical models, including ML and DL models. Empirical models, which include methods based on historical time series and statistical relationships, laid the foundation for early forecasting techniques. These models typically used simple linear regressions and Autoregressive Integrated Moving Average (ARIMA) methods to predict SI based on past data. While effective for basic forecasting tasks, empirical models often struggled with capturing the complex, nonlinear patterns inherent in solar energy data [8,9].
The advent of ML models marked a significant shift in PV power forecasting. ML techniques, such as Artificial Neural Networks (ANN) [10], Support Vector Machines (SVM), Decision Trees, and Random Forests (RF), utilize historical data to learn patterns and make predictions [11]. These models offer improved accuracy over empirical methods by better handling the nonlinearities and interactions within the data. SVMs, for instance, are particularly effective in identifying the optimal hyperplane that separates different classes in the data, enhancing prediction accuracy [12].
DL models represent the latest advancement in PV power forecasting. These models, including Deep ANNs, Convolutional Neural Networks (CNN), and Recurrent Neural Networks (RNN), such as Long Short-term Memory (LSTM) networks, have revolutionized the field by leveraging their ability to learn from large datasets and capture complex patterns. ANNs, inspired by the human brain's neural architecture, consist of interconnected neurons that process data through multiple layers, enabling the extraction of high-level features from raw input [7]. CNNs are particularly adept at processing grid-like data, such as images or spatial data, making them suitable for applications that require detailed spatial analysis. RNNs, and LSTMs in particular, excel in modeling temporal dependencies, making them ideal for time-series forecasting tasks like predicting SI over time [13].
These DL models have demonstrated superior performance in PV power forecasting due to their ability to automatically learn relevant features from data, reducing the need for extensive manual feature engineering [14]. Furthermore, the adaptability and scalability of DL models allow them to handle large and complex datasets, providing more accurate and reliable forecasts [15].
As the field of solar forecasting evolves, integrating DL models with traditional forecasting approaches has become increasingly prominent. The adaptability of ML and DL models, combined with their ability to update predictions based on new data inputs, offers a significant advantage over traditional methods. This integration has led to improved forecasting accuracy, better handling of uncertainties, and more efficient grid management [16].
In this review, we aim to provide a comprehensive analysis of the latest advancements in solar energy forecasting, with a particular focus on ML and DL techniques. We systematically explore how these models have evolved from traditional empirical and physical approaches, demonstrating their ability to handle complex and nonlinear patterns in SI data. The novelty of this review lies in its in-depth examination of ML and DL models, highlighting their transformative impact on forecasting accuracy and reliability. Additionally, we highlight real-world applications and discuss the economic and policy implications of enhanced forecasting accuracy, emphasizing the critical role of precise solar energy predictions in optimizing energy production, reducing operational costs, and promoting sustainable energy practices.
The review is structured (as depicted in Figure 1) as follows: In Section 2, we distinguish ML-based solar forecasts from traditional weather models, highlighting the methodological differences and advancements. In Section 3, we focus on the real-world applications of advanced solar forecasting, emphasizing their economic benefits and policy implications. In Section 4, we provide a comprehensive overview of various models in solar energy forecasting, including empirical models, image-based models, statistical models, ML models, DL models, foundation models, and hybrid models. In Section 5, we present a detailed discussion and analysis of the findings, addressing the challenges and limitations of current forecasting techniques, particularly concerning data quality and model interpretability.
Integrating ML into solar forecasting significantly diverges from traditional weather forecasting methodologies due to their foundational principles, computational strategies, and applications. While traditional weather models rely on physical laws to predict weather conditions, ML-based solar forecasting models use historical data to learn and predict future SI or power generation. This section delves into a detailed comparison, emphasizing the methodologies, adaptability, accuracy, and challenges of both approaches, augmented with relevant research references.
Traditional weather forecasting models are grounded in the NWP approach, which solves the fundamental equations of atmospheric dynamics and thermodynamics (e.g., Navier-Stokes equations for fluid motion, thermodynamic energy equation, mass continuity equation) on a three-dimensional grid over time [14]. These models require substantial computational resources and sophisticated algorithms to approximate solutions for these equations, making them computationally intensive and time-consuming.
Conversely, ML-based solar forecasting models, including ANN, RF, Gradient Boosting (GB), and Transformers, leverage statistical learning approaches to identify patterns within historical data. These models predict solar output or irradiance by understanding the relationship between input features (e.g., temperature, humidity, time of day) and solar energy production without explicitly simulating atmospheric physics [15].
ML models are notably adaptable and capable of updating their predictions based on new data inputs. Domain adaptive learning models, for instance, dynamically adjust to new weather conditions, significantly improving prediction reliability without the need for constant retraining [16]. This adaptability is juxtaposed with the static nature of NWP models, which require manual updates to incorporate new data or changing atmospheric conditions.
The accuracy of ML models in solar forecasting is often higher for specific applications, such as solar power generation prediction. Techniques like diffusion models, which generate probabilistic forecasts, offer insights into prediction uncertainty, a critical aspect for grid integration of solar power [17]. However, traditional NWP models, despite their computational demand, provide comprehensive atmospheric forecasts that are essential for broad meteorological applications.
ML models are highly dependent on the availability and quality of training data, which can limit their accuracy and applicability in regions with insufficient historical data. Additionally, these models may overfit to specific patterns within the training dataset, potentially reducing their generalizability to new conditions. Another notable challenge is the interpretability of ML models, which can obscure the physical reasoning behind their predictions.
A promising approach to leveraging the strengths of both methodologies is the integration of ML models with traditional NWP outputs. For instance, ML models can be used to correct biases in SI estimates from NWP models or to enhance the spatial resolution of forecasts. This hybrid approach combines the physical rigor of NWP models with the computational efficiency and adaptability of ML techniques [18].
Machine learning (ML) models excel in adaptability due to their capacity to update predictions based on new data inputs, dynamically adjusting to varying conditions without the need for constant retraining. This characteristic is juxtaposed with the static nature of numerical weather prediction (NWP) models, which require manual updates to incorporate new data or changing atmospheric conditions. However, it is important to note that while ML models are highly adaptable, overfitting can potentially reduce their generalizability to new conditions. This happens when models learn the training data too well, capturing noise that does not generalize well to unseen data. Therefore, it is crucial to employ techniques such as cross-validation and regularization to enhance the generalizability of ML models across geographical regions and varying conditions.
Exploring the real-world applications of advanced solar forecasting, this part delves into the economic benefits of enhanced forecasting accuracy, the policy implications of accurate solar forecasts, and the integration of artificial intelligence in forecasting systems. It highlights how precise solar energy predictions can improve economic efficiency, influence energy policies, and leverage technological advancements in the sector. The development and utilization of hybrid models in solar forecasting have demonstrated significant improvements in the accuracy and reliability of solar energy predictions, which are crucial for the efficient management and utilization of solar resources. Enhanced prediction models, like the hybrid adaptive neuro-fuzzy inference systems, have shown a strong correlation between improved prediction accuracy and the efficiency of solar energy systems, allowing for better system design and optimization of energy output [17]. Similarly, accurate forecasts are essential for large-scale solar installations, such as data centers powered by solar energy, where they contribute to reducing operational costs and enhancing system reliability [18]. Technological advancements in forecasting models, particularly those incorporating ML techniques, have significantly improved the trade-off between accuracy and complexity, aiding in precise energy yield forecasts critical for energy-efficient building operations [19]. Furthermore, the economic and operational efficiency improvements noted from more accurate solar radiation prediction models underscore their value in enhancing the operational efficiency of solar PV systems and their integration into the power grid [20].
Advanced solar forecasting significantly impacts the economic aspects of solar energy by enhancing the predictability of solar output, which is crucial for both energy producers and consumers. Improved forecasting accuracy reduces the operational and capital costs associated with solar energy production. For instance, better forecasting can decrease the reliance on expensive peak-time energy reserves and reduce the charges related to imbalance penalties from unforeseen production variances. Economically, this translates into lower kWh costs, making solar energy more competitive against traditional energy sources.
Martinez-Anido et al. found that improvements in solar power forecasting could significantly reduce operational electricity generation costs by decreasing fuel and variable operation and maintenance costs, alongside reducing the start and shutdown costs of fossil-fueled generators by approximately 10–20% [21]. Furthermore, accurate forecasting has been shown to lower the need for reserve capacity by 15–30%, leading to substantial cost savings [21].
Statistically, integrating advanced forecasting tools can lead to a reduction in balance costs by up to 30%, depending on the region and grid requirements. For example, improved solar forecasting in the California Independent System Operator (CAISO) region has led to annual savings of approximately $20 million by reducing the need for ancillary services and reserve requirements [22]. Moreover, increased forecasting accuracy enhances the potential for solar to participate in electricity markets, potentially increasing revenue for solar producers by allowing more precise and competitive bidding in energy markets.
Kaur et al. quantified the benefits of solar forecasting in energy imbalance markets, showing that state-of-the-art forecasts can reduce flexibility reserves required and decrease the probability of imbalances, thus enhancing economic outcomes for solar energy stakeholders. They demonstrated that accurate solar forecasts could increase market revenues by up to 5% and reduce imbalance penalties by 10–15% [23].
These quantitative results underscore the significant economic benefits of advanced solar forecasting, highlighting its critical role in optimizing the financial performance of solar energy systems and enhancing their competitiveness in the energy market.
Accurate solar forecasts are crucial for shaping effective renewable energy policies. They enable governments to establish incentives for solar adoption, adjust tariffs to reflect solar energy's value, and create subsidies for expanding solar capacity. These forecasts aid in long-term energy planning and setting ambitious renewable energy targets. For instance, Shi et al. [24] highlight the importance of forecasting in planning power systems, noting that accurate forecasts reduce the unpredictability of solar energy and help maintain grid stability.
Moreover, policymakers use these forecasts to justify and plan the expansion of grid infrastructure, such as energy storage systems, essential for managing solar power's intermittency. Improved forecasting accuracy has led to significant investments in battery storage systems in regions like California, stabilizing the grid during peak demand. Furthermore, Mohanty et al. discuss how developing economies like India leverage solar forecasting to integrate solar energy more effectively into their national grids, thereby supporting economic development and energy security [25].
Enhanced solar forecasting aligns with global sustainability goals by facilitating larger renewable energy shares in the grid, thus reducing carbon emissions and fossil fuel dependency. Accurate forecasts improve solar resource utilization, minimizing waste and maximizing energy utilization per installed capacity. Enhanced accuracy in solar forecasts could help reduce CO2 emissions by up to 6% annually, supporting environmental goals while fostering economic resilience by diversifying energy sources and mitigating the impacts of volatile fossil fuel markets.
Researchers suggest that improving solar forecasting could significantly impact the efficiency of integrating solar energy into energy systems. For example, Sweeney et al. discuss the advances in forecasting that potentially enhance the economic and environmental benefits, underscoring the role of accurate solar forecasting in enabling the effective integration of renewables into the energy market and contributing to sustainability efforts [26].
Improving the accuracy of solar forecasting significantly impacts the operational costs and efficiency of energy production and grid management. Enhanced forecasting allows utility operators to better manage the necessary power inputs from renewable sources like solar. This reduces reliance on costly and less efficient fast-ramping or Peaker power plants, which are typically used to manage unexpected changes in energy supply and demand [27].
Quantitatively, each percentage point improvement in forecasting accuracy substantially diminishes the use of these costly backup systems. For example, improving solar forecast accuracy by 10% could potentially reduce the operational costs associated with maintaining and operating these Peaker plants by approximately 10–20%. This is primarily because less reserve power is needed to compensate for uncertainties in solar output, leading directly to reductions in fuel usage and maintenance costs for these facilities [27].
Furthermore, more accurate forecasting can decrease penalties associated with deviations from scheduled generation in electricity markets. These penalties occur when the actual power generation does not match the forecasts submitted to electricity market operators, leading to inefficiencies in grid management [22]. Enhanced accuracy in solar power forecasts helps minimize these discrepancies and the associated financial penalties.
Grid reliability, crucial for a consistent and stable energy supply, is significantly enhanced by accurate solar power forecasts. Solar energy is inherently intermittent, influenced by weather conditions such as cloud cover, humidity, and temperature. By improving the accuracy of these forecasts, grid operators can better anticipate fluctuations in solar output and adjust grid operations to maintain a stable energy supply [22].
Enhanced forecasting facilitates more precise scheduling of energy production from various sources, optimizing the use of renewable energy and reducing reliance on fossil fuel-based backup generation. It also supports integrating higher levels of solar power into the grid without compromising grid reliability or stability. For instance, better solar forecasting can lead to reduced reserve margins, which are buffers against unexpected changes in power supply, thus enhancing overall grid efficiency and stability [28].
Furthermore, accurate solar forecasts are vital for managing energy storage systems, which are key in balancing supply and demand. These systems store excess solar energy generated during peak sunlight hours and release it during periods of low solar output or high demand. Effective use of these storage systems, guided by accurate forecasts, can further stabilize the grid and reduce operational strain on conventional power plants [29].
Advanced numerical modeling methods, including ML and DL, are used to establish a more accurate mapping between input data, such as SI, and output predictions, like solar energy output. These "true mapping" techniques aim to capture the complex relationships and dynamics inherent in the physical processes governing SI, thus enhancing the reliability and accuracy of solar energy forecasts. DL, in particular, has shown promise in discovering inherent nonlinear features and high-level invariant structures in data, which are crucial for improving forecasting accuracy [30].
The accuracy of predictive models heavily depends on the quality and reliability of the input data. Uncertainties in the data set, such as measurement errors, incomplete data coverage, and temporal-spatial variations, significantly affect the performance of models. Addressing these uncertainties is crucial for improving the fidelity of predictions and making the forecasts more robust and dependable for practical use. ML models, including LSTM and Facebook Prophet, have been used to quantify and mitigate the impact of data uncertainty, showing improvements in forecast accuracy by effectively managing data uncertainties [31].
In the real-world application of solar forecasting, improvements in modeling have direct implications for grid management, economic planning, and policy formulation. Better predictive models enable more efficient integration of solar energy into power grids, reduce the need for costly backup power solutions, and support the creation of more informed and effective energy policies. Enhanced forecasting models, particularly those utilizing DL approaches, have been shown to significantly reduce the operational and economic challenges associated with integrating renewable energy sources into the grid [32].
The Solar Energy Technologies Office Fiscal Year 2020 (SETO 2020) funding program by the U.S. Department of Energy has significantly emphasized the integration of artificial intelligence (AI) into solar energy advancements. With a substantial allocation of $130 million distributed among 55–80 projects, the initiative underscores a serious commitment to enhancing the efficiency, reliability, and grid integration of solar technologies [33].
• Investment in AI for solar technologies
A specific portion of this funding, about $7.3 million, is dedicated to ten projects focusing on ML and other AI applications. These projects aim to leverage AI to advance early-stage PV, concentrating solar-thermal power (CSP), and systems integration technologies. This strategic funding is expected to spearhead innovations that could transform the operational aspects of solar energy systems.
• Enhancing predictive maintenance and reliability
Among the funded initiatives, one notable project at Arizona State University is pioneering the development of AI-driven algorithms for predictive maintenance in PV power plants. By employing real-time data analytics, these algorithms anticipate potential system failures and schedule maintenance proactively. This capability not only reduces downtime but also extends the lifespan of solar power installations, thus maintaining optimal financial performance.
• Improving grid situational awareness
Further, the program supports projects aimed at enhancing the accuracy of solar energy output predictions and grid situational awareness. Improved predictive capabilities are crucial for the efficient integration of solar power into the national grid, enhancing grid reliability, and reducing operational costs. These projects explore how AI can manage and synthesize data from diverse sources to provide accurate, real-time analyses of the grid state.
• Advancing solar forecasting capabilities
The integration of AI into solar forecasting involves developing sophisticated models that can accurately predict solar energy output. This forecasting is vital for grid operators, allowing for better resource planning and energy distribution. It ensures that solar integration does not compromise the stability of the grid and optimizes the use of renewable resources.
The SETO 2020 initiative illustrates the potential of AI to revolutionize solar energy forecasting and management. By harnessing the capabilities of AI, these projects aim to address some of the most pressing challenges in the solar industry, including variability in energy production, grid integration, and operational efficiency. The ongoing advancement in AI applications within the solar sector not only enhances the technical operations of solar systems but also supports broader energy policy objectives aimed at sustainable and reliable energy solutions.
In solar energy forecasting, historical solar irradiance data forms a fundamental component. This type of data is often collected in detailed time series formats, such as Excel spreadsheets, which record solar radiation levels over specific periods and locations. Time series data enables the analysis of trends and patterns, which is critical for the accurate prediction of solar energy output [34,35].
Images, especially those captured using infrared technology, are pivotal in solar forecasting. Infrared images provide thermal data that can predict solar irradiance based on heat patterns. Full-sky images capture comprehensive atmospheric conditions, offering insights into cloud cover and other factors influencing solar radiation. These images are crucial for deep learning and hybrid deep learning models [36,37].
The data required for ML models can be procured through various sources. Table 1. provides an overview of various data types and their sources with links. By integrating these diverse data types from multiple sources, solar forecasting models achieve high accuracy and reliability, ensuring efficient integration of solar energy into the power grid.
Data Type | Source | Description | References | Link |
Solar Irradiance | SoDa Service | Historical solar radiation data, crucial for model training and validation | Freitas et al., [34], Gaye et al., [36] |
https://www.soda-pro.com/ |
National Renewable Energy Laboratory (NREL) | Solar resource data and tools, including the National Solar Radiation Database (NSRDB) | Natei et al., [38] Li et al., [39], Long et al., [40] |
https://data.nrel.gov/ | |
European Commission's JRC-Photovoltaic Geographical Information System (PVGIS) | Solar radiation and photovoltaic performance data for Europe, Africa, and Asia | Freitas et al., [34], Gaye et al., [36] | https://joint-research-centre.ec.europa.eu/photovoltaic-geographical-information-system-pvgis_en | |
HelioClim Database | Satellite-derived solar radiation data | Jayalakshmi et al., [37], Jebli et al., [41] |
https://www.soda-pro.com/help/helioclim/helioclim-3-overview | |
Copernicus Atmosphere Monitoring Service (CAMS) | Solar radiation data derived from satellite observations and atmospheric models | Khandakar et al., [42], Jayalakshmi et al., [37] |
https://atmosphere.copernicus.eu/ | |
Geographical | PVGIS | Latitude, longitude, altitude, and slope data for specific locations | Jebli et al., [41], Kim et al., [43] | https://joint-research-centre.ec.europa.eu/photovoltaic-geographical-information-system-pvgis_en |
Calendar | NASA POWER | Time zone, hour of the day, month, and day of the year data capturing seasonal and diurnal variations | Jayalakshmi et al., [37], Natei et al., [38] |
https://power.larc.nasa.gov/ |
Astronomical | National Renewable Energy Laboratory (NREL) | Solar elevation angle, hour angle, and solar zenith angle for modeling sun's position | Li et al., [39], Long et al., [40] | https://data.nrel.gov/ |
Satellite-Based Data | MODIS | Provides consistent spatial coverage, crucial for global meteorological monitoring | Khandakar et al., [42], Jayalakshmi et al., [37] |
https://modis.gsfc.nasa.gov/data/ |
Meteorological | World Radiation Data Centre (WRDC) | Solar radiation data from ground-based observation stations around the world | Natei et al., [38]; Khandakar et al., [42] | http://wrdc.mgo.rssi.ru/ |
Meteonorm | Solar and meteorological data for various locations, using ground-based measurements and satellite data | Freitas et al., [34]; Jayalakshmi et al., [37] | https://www.pvsyst.com/help/meteo_source_meteonorm.htm | |
Korea Meteorological Administration (KMA) | Atmospheric parameters like temperature, humidity, and sunshine duration | Natei et al., [38], Gutiérrez et al., [44] | https://www.kma.go.kr/neng/index.do |
Solar irradiance data is typically sourced from the Solar Radiation Data website, renowned for its extensive and accurate datasets. This platform offers historical solar radiation data, which is essential for training and validating forecasting models.
Geographical parameters such as latitude, longitude, altitude, and slope are vital for estimating solar radiation at specific locations. Calendar parameters, including the time zone, hour of the day, month, and day of the year, capture seasonal and diurnal variations. Astronomical parameters, such as the solar elevation angle, hour angle, and solar zenith angle, are calculated based on geographical data and are crucial for modeling the sun's position [41.43].
Ground-based meteorological observation stations provide high-precision data necessary for solar irradiance prediction. These stations measure atmospheric parameters like temperature, humidity, and sunshine duration. For example, the Korea Meteorological Administration's station in Yuseong-gu, Daejeon, provides valuable data for research studies [38].
Moderate Resolution Imaging Spectroradiometer (MODIS) data serves as an alternative to ground-based measurements, especially in remote areas. While slightly less accurate, satellite data offers consistent spatial coverage, making it essential for global meteorological monitoring [42,44].
In solar forecasting, effectively pre-processing historical data is crucial due to common issues like outliers, noise, or incomplete data. This input data significantly impacts the forecasting results; thus, pre-processing is a vital step to enhance model performance. The major methods of data pre-processing include:
This process addresses abnormal data that could lead to deviations in prediction results. The goal is to fill in gaps or remove unnecessary data from the dataset. If the data has a high rate of missing information and low importance, it can be omitted. Techniques like the linear internal difference method or averaging are typically used to manage missing values, as referenced in [45].
This method involves scaling the original data to a range of [0, 1] without altering its distribution, as noted in [46]. The advantage of normalization in data processing is that it removes the limitations imposed by data units on the model, accelerates convergence, and shortens training time.
This standardization process transforms the data into a normal distribution with an average value of zero and a standard deviation of one, as detailed in [47]. It is beneficial in improving the convergence speed and forecasting accuracy. However, if the data distribution is not close to normal, the standard deviation can cause deviations in standardization. Z-score normalization also helps to reduce the influence of outliers when the extremes cannot be determined.
WT is a technique used to convert data into time domain and frequency domain features and is forwarded to the mother wavelet, as described in [48]. This method can identify existing frequencies with their corresponding occurrence. Although outliers have little effect on forecasting results using WT, the lack of adaptability is a drawback once the basis function is chosen. Zolfaghari et al. [49] demonstrated that WT could decompose input data into high-frequency and low-frequency sequences, improving prediction performance.
EMD, based on time-domain processing [50], differs from WT as it doesn't require selecting a mother wavelet and is not replaceable. EMD directly decomposes the original time series into intrinsic mode functions (IMFs) and a residual, with the IMFs representing frequency components ordered from high to low frequency. Despite its self-adaptive nature for decomposing non-linear and non-stationary signals, EMD faces challenges with mixed modes in IMF components. Wang et al. [51] utilized EMD to decompose wind speeds into IMFs of varying proportions for subsequent prediction modeling.
SSA deals with nonlinear time series and is applied in various fields, including climate and finance [52]. It embeds, decomposes, groups, and reconstructs time series data, identifying long-term trends, periodic signals, and noise. The process involves arranging time series into a trajectory matrix, decomposing them using singular value decomposition (SVD), and then reconstructing each component group into a new time series. Zhang et al. [53] used SSA to extract hidden features of wind power generation, showcasing its application in renewable energy forecasting.
Solar energy, increasingly recognized as a viable alternative to fossil fuels, introduces significant challenges in its integration into the power grid. The key to a successful power plant operation lies in balancing energy demand and supply. This balance is crucial, especially in markets where energy procurement is influenced by competitive bidding. Errors in forecasting can lead to increased costs, making precision in solar energy forecasting a vital aspect of grid management and economic efficiency [54,55]. This need for precision directly ties into the expanding role of solar photovoltaics, where the burgeoning capacity of solar energy necessitates refined forecasting methods to ensure optimal grid integration and reliability.
The inherently volatile and intermittent nature of solar energy poses a significant challenge to its market penetration. As a result, there is a pressing need for meticulous forecasting of solar energy availability, which is paramount for a range of applications from plant operation to energy trading [56]. Unfortunately, due to stringent data privacy policies, historical PV data critical for prediction are often unavailable. Instead, forecasts frequently rely on global horizontal irradiance (GHI), a key determinant of solar power generation. Although GHI data is a cornerstone for numerous solar energy operations, its acquisition is often hindered by the prohibitive costs of measurement equipment, leading to a scarcity of data, particularly in densely populated regions like China and India [57].
GHI forecasts are tailored to diverse applications, each requiring different forecasting horizons, ranging from immediate, very short-term predictions for system monitoring to long-term projections for site selection and plant installation [58]. The field has attracted considerable attention from both industry and academia, inspiring extensive research and the development of various forecasting models. These models span several categories, including traditional empirical models that leverage geographical and meteorological data, image-based models that utilize visual sky data, statistical models that analyze historical time series, and empirical ML models that employ advanced algorithms to detect complex patterns [59].
The advent of DL has marked a significant milestone in the forecasting landscape. These sophisticated models excel in feature extraction and pattern recognition, even from vast and complex datasets. DL's ability to autonomously learn from data and its robustness in generalization make it particularly suited for predicting SI with greater accuracy [60]. As we continue to harness the full potential of solar energy, DL stands as a beacon of innovation, guiding us toward more reliable and efficient forecasting methods that can adapt to the evolving dynamics of climate and weather patterns, ultimately supporting the sustainable advancement of solar energy within the global energy matrix. An overview of the solar forecasting method is presented in Figure 2, covering major steps like data collection/preparation, model coding/training/optimization, and model evaluation.
Empirical models, utilizing geographical and meteorological data, have been integral in modeling SI over the past few decades. These models, which range from temperature-based to hybrid meteorological-based, leveraging multiple weather parameters, have been extensively applied in estimating GHI [61]. The first empirical model for GHI estimation, based on sunshine duration, was developed by Angstrom et al. [62] in 1924 and has since been refined by various researchers, including Samuel et al. [63], Ögelman et al. [64], Badescu et al. [65], and Mecibah et al. [66]. Studies indicate that sunshine-based models are particularly effective due to the strong correlation between sunshine duration and data.
However, the availability of sunshine data is not consistent worldwide, leading researchers to explore temperature-based empirical models as an alternative. These models have seen widespread application due to the relative ease of obtaining temperature data. Notably, Hargreaves et al. [67] were among the first to propose a temperature-based model using minimum and maximum temperatures to estimate GHI. This was followed by an advanced model by Bristow et al. [68], which modeled SI as an exponential function of diurnal temperature changes. Despite the popularity of temperature-based models, they often yield less accurate results. To enhance accuracy, hybrid models incorporating additional meteorological parameters like rainfall, relative humidity, and pressure were developed. However, the complexity of these models due to the inclusion of multiple parameters sometimes limits their applicability.
Researchers have reviewed various empirical models and recommended that they are most suitable for long-term forecasting horizons, such as 6 to 72 hours ahead [69]. However, these models are not as effective for short-term horizon GHI prediction due to high computational demands and their inability to accurately capture real-time environmental changes, such as cloud movements. This limitation is particularly pronounced in environments with high variability and noise, restricting the preciseness of the GHI predictions [70]. As the solar energy sector continues to evolve, these challenges in forecasting highlight the need for ongoing innovation and development in the techniques used for SI estimation.
Image-based models, utilizing sky cameras or satellite imagery, represent a significant advancement in GHI prediction. This method has demonstrated promising results, especially in forecasting solar irradiance (SI) over large areas. One of the key strengths of this approach lies in its ability to capture cloud motion, thanks to the high temporal and spatial resolution of the images used. This feature is a notable improvement over empirical models, as image-based models can directly incorporate cloud information from the sky image dataset, leading to more accurate GHI forecasts [71].
Despite its effectiveness, the image-based method for GHI prediction faces several challenges. The availability of image datasets is one such hurdle, often compounded by the high costs associated with image-capturing instruments. Even if the cost is relatively high, it has been divided by a factor of approximately 10 over the past 10 years, and this cost needs to be put in perspective with the added value of more accurate solar forecasts based on sky images. Additionally, the process of image processing itself can be complex and resource-intensive. These factors contribute to making image-based techniques less favored in certain contexts of GHI prediction, despite their inherent advantages in accuracy and detail. A major challenge is the lack of large quantities of sky image data encompassing diverse sky conditions for model training. However, recent studies have identified 72 open-source sky image datasets globally that satisfy the needs of deep learning-based method development, including cloud segmentation, classification, and motion prediction. This extensive survey provides a database with information about various aspects of these datasets and a multi-criteria ranking system to evaluate each dataset based on eight dimensions that could impact data usage [72]. The SkyImageNet initiative, a significant project aimed at enhancing machine learning-based forecasting tools for solar power integration, supports the creation of a large-scale dataset of sky images for solar energy forecasting, cloud analysis, and modeling. By utilizing high-resolution sky images, SkyImageNet aims to improve the accuracy of solar irradiance predictions, aiding in better management and utilization of solar energy resources [73]. These advancements streamline the processes of identifying and selecting sky image datasets, potentially accelerating method development and benchmarking in solar forecasting and related fields, including energy meteorology and atmospheric science. These limitations highlight the need for further development and optimization in the realm of image-based SI forecasting, ensuring that the benefits of this advanced approach can be fully realized and more widely adopted [74].
A wide diversity of vision-based solar forecasting techniques based on deep learning have been developed in recent years. Recent examples and approaches, such as those highlighted by Paletta et al., illustrate significant advancements in anticipating cloud-induced solar variability. These methods employ sophisticated computer vision and deep learning algorithms to analyze sky images and predict solar irradiance with higher accuracy compared to standard statistical methods that rely solely on historical meteorological data [75].
Advancements in video analysis techniques have shown significant potential in improving solar forecasting accuracy. By analyzing time-lapse videos from ground-based cameras or satellite imagery, these methods can track cloud movement and development in real time. Techniques such as optical flow, CNNs, and RNNs are employed to predict the impact of cloud cover on solar irradiance. For example, studies have utilized CNNs to process video frames and extract features that indicate cloud patterns, while RNNs are used to model the temporal dependencies between consecutive frames. This combination allows for more accurate short-term predictions of solar variability due to cloud cover, surpassing the capabilities of standard statistical methods based solely on historical meteorological data.
In optical flow techniques, the motion of clouds is estimated between consecutive frames of video footage. This technique helps in understanding the speed and direction of cloud movement, which directly influences solar irradiance levels. Advanced implementations of optical flow can handle complex cloud dynamics, providing high-resolution predictions of solar irradiance fluctuations [71].
CNNs in video analysis serve to identify and classify cloud structures in each frame of the video. CNNs are adept at recognizing spatial patterns and textures within the cloud formations, which are critical for predicting the impact of these clouds on solar irradiance. By processing multiple frames, CNNs can build a comprehensive model of cloud behavior over time [60].
RNNs, including Long Short-Term Memory (LSTM) networks, are particularly effective in modeling temporal sequences, such as the progression of cloud cover over time. RNNs can remember important temporal features and provide a sequence of predictions that account for past cloud movements and predict future changes. Combining RNNs with CNNs, known as ConvLSTM, enhances the model's ability to predict solar irradiance by capturing both spatial and temporal dependencies [76].
Researchers have demonstrated the effectiveness of these video analysis techniques. For instance, Elsaraiti and Merabet compared the performance of LSTM networks with traditional models and found LSTM to be superior in forecasting accuracy [77]. Additionally, Ghimire et al. proposed a hybrid model integrating CNN and LSTM for half-hourly global solar radiation forecasting, which outperformed standalone models [76]. These advancements indicate a promising future for vision-based models in solar forecasting, combining high-resolution imagery with advanced deep learning techniques to achieve superior forecasting performance. By leveraging video analysis, researchers can anticipate rapid changes in solar irradiance with greater precision, enhancing grid management, and energy production planning.
Diverging from the methods previously discussed, statistical models offer a unique approach to SI forecasting by utilizing historical time series data. These models establish a mathematical relationship based on past records of SI, providing a basis for predicting future trends. Among the most prevalent statistical models used in this field are the ARIMA, Exponential Smoothing (ETS), and generalized autoregressive conditional heteroskedasticity (GARCH). These methods have been widely recognized and utilized in various studies for their efficacy in forecasting GHI over short time horizons ranging from 5 minutes to 6 hours [78,79,80].
Statistical methods capitalize on historical data to infer patterns and relationships between input factors and power production [81]. In the solar energy domain, these methods are prevalent and encompass various techniques, including Markov Chains [82], fuzzy logic [17], and auto-regressive models [83,84] such as NARX [85] and NARMAX [86]. Despite being generally less intricate than physical models, their dependence on historical data enables a more nuanced modeling of specific plant characteristics [87]. However, the requirement for extensive plant-specific data [83] can pose limitations in rapidly evolving or expanding energy environments.
Statistical models are generally effective in predicting the values of stationary GHI time series. However, a key challenge arises due to the non-stationary nature of SI data, often influenced by varying cloud cover and seasonal changes. This non-stationarity introduces complexities in the data that these models struggle to accurately capture, leading to reduced performance in prediction, especially in scenarios where non-linearity is prevalent [88]. This limitation underscores the need for continued innovation in statistical modeling techniques to enhance their predictive accuracy, particularly in the context of the dynamic and often unpredictable nature of SI.
The emergence of artificial intelligence has led to ML becoming the preferred method for SI forecasting. ML techniques focus on identifying patterns within data, shaping parameters, and creating predictive models. This approach allows for the extraction of complex and nonlinear features from the data. Various ML methods (refer to Figure 3), such as SVM, ANN, and RF, have been extensively applied to predict SI, demonstrating their effectiveness in this field [89].
Despite their strengths, ML methods are not without limitations. Challenges such as overfitting, high computational costs, and difficulties in managing complex, high-dimensional data are common [90]. To enhance model performance, there has been a growing interest in the ensemble approach, which combines multiple models to integrate their strengths, thereby improving prediction accuracy and stability [91].
ANN-based models are inspired by the learning mechanisms of biological neural systems [92,93], comprising interconnected units known as neurons [94]. These models are structured into three distinct layers: Input, hidden, and output layers [95,96]. Capable of addressing both linear and nonlinear challenges [97], ANNs outperform many conventional empirical models in their performance [92,98]. The functional representation of ANN models is given by the equation:
y(x)=F(∑Li=1ωi(t).xi(t)+b) | (1) |
where y(x) is the output; xi(t) is the input variables in discrete space t; F is transfer function for the hidden layer; L is the neurons; ωi(t) is the weight; and b is the bias. Depending on the connections among the input, hidden, and output layers, ANN models can be categorized into feedforward, recurrent, and symmetrical (or Hopfield) networks.
Feedforward Neural Networks (FF-NN) and Recursive Neural Network are illustrated in Figure 4. In FF-NNs, neurons are organized in sequential layers, with each neuron connected solely to those in the preceding layer, thus eliminating feedback between layers [99]. This architecture makes FF-NN one of the most prevalently applied ANNs. Conversely, Recursive Neural Networks (Figure 4), also referred to as recursive neural networks, retain the input from previous steps within the network to generate output alongside the current input, thereby offering the benefit of memory capability [100].
KNN is a widely applied non parametric statistical methods used for classification and regression related problems. It is based on the assumption that part of the past pattern series will reappear with similar patterns in the succeeding iterations [101]. For searching the most similar patterns, search range of nearest neighbor is defined by the user. This input is very critical as it affects the prediction results, which vary based on data size and search range [102]. The computation algorithm of KNN can be expressed as:
For a certain given search point xi, the search of K training points xj, where j = 1, 2, 3, ⋯, k, is computed using Euclidean Distance formula [103]. For more detailed description of KNN algorithm, works by Larose et al. [104], Sutton et al. [105], and references therein, give better understanding.
SVM models address regression challenges using various kernel functions, enabling the transformation of low-dimensional input data into a higher-dimensional feature space [106]. Studies have applied SVM models to predict SI (H) using data across different locations, demonstrating SVM's effectiveness in providing satisfactory estimations of H [107]. The SVM's objective function is given by:
f(x)=ωφ(x)+b | (2) |
Here, ϕ(x) represents the mapping to a higher-dimensional feature space, while ω and b denote the weight vector and bias, respectively. SVM employs several kernel functions, such as the Radial Basis Function (RBF), polynomial, linear, and sigmoid kernels [108]. Among these, the RBF kernel is favored for its efficiency, simplicity, robustness, and optimization convenience [109], described by the equation:
K(xi,xj)=exp(−γ‖xi−xj‖2 | (3) |
where γ = - 1/2σ2, with σ being the standard deviation of Gaussian noise, and xi and xj are the input feature vectors. Compared to traditional Artificial Neural Network (ANN) models, SVM typically offers superior accuracy and stability [110]. Research by Mohammadi et al. [111], Hassan et al. [112], Quej et al. [113], and Baser et al. [114] has shown that SVM can efficiently identify the optimal hyperplanes for support vectors during the training process and eliminate non-support vectors, resulting in faster training and reduced computational costs, thus achieving better overall performance.
RF, as identified by Breiman (2001) [115], stands out as one of the leading ML techniques. It operates as a collective of decision trees, each developed through a dual-randomization process. Initially, every tree is crafted from a bootstrap sample—randomly selected with replacement from the initial dataset, mirroring its size. This approach typically results in about 37% of the instances being duplicates. The second layer of randomness comes from attribute sampling: at every decision node, a randomly chosen subset of attributes is evaluated to determine the optimal split, with Breiman et al. [115] suggesting the formula ⌊log2(#features)+1⌋ for selecting the number of attributes.
For classification tasks, RF reaches its verdict through majority voting among its decision trees. The Strong Law of Large Numbers assures that, as the ensemble grows in tree count, its generalization error tends toward a limit, indicating the ensemble's size is not critical to its tuning. In essence, adding more trees generally doesn't compromise, but rather stabilizes, its predictive accuracy towards an asymptotic generalization error.
One of RF's key strengths is its minimal dependence on hyper-parameter adjustments; often, its default settings yield robust performance across various datasets (Fernández-Delgado et al. [116]). This characteristic positions RF among the top-performing methodologies in comparative studies, even when minimally tuned. However, this aspect can also be seen as a limitation, as there is scant scope to enhance performance through hyperparameter optimization.
Light GBM, introduced by Ke et al. [117], represents a comprehensive framework that enhances GB by introducing several innovative variants. Aimed at achieving computational efficiency, Light GBM adopts a feature histogram precomputation approach similar to that of XGBoost. The framework is versatile, supported by numerous hyper-parameters that ensure its adaptability across diverse scenarios. It is compatible with both GPU and CPU architectures and incorporates basic GB alongside various randomization techniques, such as column randomization and bootstrap subsampling.
Among its novel contributions [117], Light GBM introduces Gradient-based One-Side Sampling (GOSS) and Exclusive Feature Bundling (EFB). GOSS is a sampling technique designed to prioritize instances with higher classification uncertainty during the training of base trees. This is achieved by focusing on instances with larger gradients, thereby increasing their significance in the learning process. Specifically, the training sets for the base models consist of the top fraction of instances with the highest gradients and a randomly selected fraction from those with lower gradients. To maintain the integrity of the original data distribution, instances from the latter group are assigned increased weight during the calculation of information gain.
EFB, on the other hand, consolidates sparse features into a single feature without information loss, applicable when such features are mutually exclusive in their non-zero values. While EFB primarily serves as a preprocessing step to enhance training efficiency and can be applied broadly, we concentrate on the implications of GOSS within the LightGBM framework, given that the aspects of standard GB are already well-covered by existing GB implementations.
The XGBoost model is an extreme development of the RF under the umbrella of ML models. The RF-based models, being an ensemble algorithm containing quite long decision trees, has as a major drawback, namely its probity to overfit. To mitigate this problem, XGBoost reduces the high variance of RF using smaller random trees, together with parallel processing and better handling of missing values, managing to obtain a better learning algorithm from weaker trees [118]. Other markable aspects of XGBoost regard its scalability in several situations, meaning that the algorithm is capable of manipulating consistently an increasing dataset size while also being of easy use and having good generalization performance [118]. This model has been proven to reach state-of-the-art results, achieving the best results in many ML challenges. Furthermore, this model has been successfully applied in solar photovoltaic and radiation estimation, and also in astrophysics for pulsar candidates' classification, granting it a reference status among ML models [119].
In solar forecasting, DL models have marked a transformative advancement. Their ability to learn directly from datasets enables these models to detect intricate patterns in data, enhancing the overall training process. This proficiency addresses some key limitations of classical ML models, such as Autoregressive (AR) models, where manual feature engineering and parameter optimization are necessary.
Among the innovations in DL (refer to Figure 5) for PV power generation forecasting, the Gated Recurrent Unit (GRU) stands out. As part of the newer generation of RNNs, GRUs employ update and reset gates, effectively tackling the vanishing gradient problem that plagues traditional RNNs [120]. These mechanisms allow GRUs to retain critical information from past data while discarding irrelevant details, thus improving the accuracy of forecasts. Notably, GRUs have shown to outperform LSTM models in processing speed and parameter efficiency, making them a valuable tool for short-term PV power forecasting [121].
Deep Networks (DNN) [122] are used more selectively due to their broader approach to time-series data. RNN [123], including specialized versions like LSTM [124] and Gated Recurrent Unit (GRU) [125], focus on temporal data modeling, thereby enhancing prediction stability over multiple steps [126]. CNN [76] are particularly utilized for spatial analysis of power production data [127], enabling precise localized predictions [128].
Hybrid models blend both spatial and temporal considerations [129]. An example is the ConvLSTM [130], which integrates convolutional layers within LSTM units, affording translation invariance and a broader scope of analysis. The WaveNet [131] and Temporal Convolutional Network (TCN) [132] employ causal dilated convolutions to refine temporal data interpretation. The Transformer model [133] leverages an attention mechanism for focused prediction on specific time steps. Noteworthy implementations include the CNN-LSTM hybrid by Ghimire et al. [76] and Zang et al. [134], combining pattern recognition with time-series analysis to lower data dependencies. These models benefit from the incorporation of attention mechanisms, enhancing their ability to address both short-term and long-term temporal patterns [135]. However, each model type has its inherent challenges; RNNs, for instance, are prone to training difficulties due to vanishing gradients [136] and numerical instability [137], whereas CNNs require extensive training data and deep architectures to achieve broad receptive fields [128]. Despite these challenges, the continual evolution and integration of these models underscore the dynamic nature of solar forecasting, driving the field toward more precise and reliable prediction methods. The most prominent DL methods are discussed here.
CNNs, a subset of ANNs, are renowned for their proficiency in processing data that exhibits a grid-like topology, such as images or videos [138]. Drawing inspiration from the structure and function of the visual cortex in the human brain, CNNs leverage convolutional layers to extract localized features from input data [139]. A typical CNN architecture consists of convolutional layers, pooling layers, and fully connected layers.
The convolutional layers, foundational to CNNs (Figure 6), use filters or kernels to capture localized features from the input. These filters, essentially small matrices, traverse the input data, performing dot products to produce feature maps that highlight essential elements for the specific task [140]. The pooling layers, often succeeding the convolutional layers, reduce the spatial size of these feature maps, thereby enhancing the network's computational efficiency. Max pooling and average pooling are common methods used here, focusing respectively on the highest values and the average values within specific regions of the feature maps [141].
Finally, the fully connected layers integrate these learned features to make final predictions, similar to traditional neural networks. During the training phase, CNNs adjust their weights to minimize the error between predicted and actual outputs [142]. This optimization is facilitated by the back-propagation algorithm, which computes gradients of the loss function in relation to the weights and updates them accordingly [143]. This intricate interplay of layers and learning mechanisms positions CNNs as effective tools for tasks that require detailed analysis of grid-based data, including solar forecasting applications, where they can be adapted to interpret time-series data, typically handled by 1-dimensional CNNs [141].
The LSTM network, originally introduced by the pioneering work of Hochreiter et al. [136], represents a transformative advancement in the domain of RNNs. Its distinctive appellation, "Long-short term memory", aptly signifies its unique ability to adeptly address both long- and short-time lags, making it eminently suitable for a diverse range of complex tasks [144]. In stark contrast to traditional RNNs, LSTM offers two crucial advantages of paramount significance. First, it effectively mitigates the ubiquitous challenges of gradient vanishing and exploding, which often hinder the progress of conventional RNNs during training, thus ensuring the stability and efficacy of the learning process. Second, the ingenious architectural design of LSTM empowers it with remarkable proficiency in handling lengthy sequences, endowing it with a substantial leap in performance compared to its predecessors [145].
At the core of the LSTM unit lie three pivotal gates (refer to Figure 7), the forget gate, the input gate, and the output gate, each with a well-defined role. The forget gate diligently determines which fragments of information warrant retention and which should be discarded, enabling dynamic and adaptive memory mechanisms. Furthermore, the input gate skillfully integrates novel information into the cell state, while the output gate astutely governs the subsequent hidden state [146]. Moreover, LSTM ingeniously leverages an internal memory unit and gate mechanism to deftly overcome the adversarial challenges of gradient vanishing and exploding during the training process, thereby surmounting these obstacles with remarkable finesse and dexterity [147].
The calculation formulas related to the LSTM structure are as follows:
ft=σ(wf[ht−1,xt]+bf) | (4) |
ft is the output of forget gate and σ is sigmoid activation function.
uf=tanh(Wc[ht−1,xt]+bc) | (5) |
uf is indicating output value of update gate.
it=σ(Wi[ht−1,xt]+bi) | (6) |
it is the output value of input gate.
Ct=ft×Ct−1+it×ut | (7) |
Ct is indicating memory cell.
Ot=σ(W0[ht−1,xt]+b0) | (8) |
Ot is indicating of output value of output gate.
ht=Ot×tanh(Ct) | (9) |
ht is indicating the output vector result of the memory cell.
In the context of the LSTM model, the control gates, denoted by y ∈ (f, i, c, o), are associated with weight matrix Wy and bias by. These gates effectively integrate the weight matrices and biases with the previous output ht-1 and the current input xt during the input process. Additionally, the LSTM model maintains the previous cell state Ct-1 and the current cell state Ct, which are crucial components in its functioning.
The control gates employ the sigmoid activation function σ, responsible for determining the significance of input information, while the hyperbolic tangent activation function tanh governs the transformations inside the LSTM cell. These activation functions play a pivotal role in regulating the flow of information and the selective memory retention or forgetting mechanism, making the LSTM model a powerful tool for handling sequential data and maintaining long-term dependencies.
GRU is the latest generation of Recurrent Neural Networks, and it is quite similar to the LSTM in terms of functionality. They did away with the cell state and instead made use of the hidden state to transport information [148]. In addition, it only contains two gates: a reset gate and an update gate, which are both identical as shown in Figure 8.
Despite these advancements, challenges in renewable energy forecasting persist, necessitating ongoing research and development. Within DL modeling a new terminology referred as "foundation models" as coined by Stanford scholars [149] has emerged. As elucidated in Figure 9, a foundation model is capable of centralizing information from data across various modalities, creating a unified knowledge base. This singular model can afterward be adapted to a broad spectrum of downstream tasks, demonstrating its versatility and efficiency in handling diverse datasets [149]. Notable examples of such models include BERT [150], GPT-3 [151], and CLIP [152]. From a technical perception, the concept of foundation models is not an entirely novel one, as they are fundamentally built upon the principles of deep neural networks and self-supervised learning, concepts that have been a part of the AI modelling for several decades [151]. Furthermore, the transformer model, initially introduced by Ashish Vaswani. et.al. [133] in 2017, has been classified as a foundation model by Stanford researchers [149] as they see it driving a paradigm shift in AI.
The Transformer, consisting of encoder and decoder blocks with self-attention layers and feed-forward neural networks, effectively analyzes the relationship between forecasting values and encoded feature vectors in PV generation forecasting. To further refine self-attention-based models, several variants have been proposed, including the Sparse Transformer [153], LogSparse Transformer [153], Reformer [154], Longformer [155], Linformer [156], Compressive Transformer [157], and Transformer-XL [158]. These models have introduced modifications to enhance performance, particularly in handling long time-series data in forecasting tasks.
In the domain of long-sequence forecasting, the Informer [159] model stands out. It redesigns the conventional Transformer structure to accommodate long sequence inputs more efficiently. By replacing the standard self-attention block with a multi-head ProbSparse Self-attention mechanism and incorporating self-attention distilling, the Informer not only manages long sequences effectively but also improves computational complexity [160]. The decoder in the Informer, equipped to handle long inputs, forecasts output values directly through a fully connected layer after analyzing the feature map. This innovation showcases the evolving landscape of DL models in SI forecasting, where complexity and data volume present ongoing challenges [160].
Hybrid models in solar forecasting represent a cutting-edge approach that blends multiple predictive techniques to improve accuracy and reliability. These models often combine physical models, statistical methods, and ML algorithms, each bringing its unique strengths to enhance overall forecasting performance.
Hybrid physical and ML models integrate the deterministic elements of physical models with the adaptability of ML algorithms. This combination is particularly effective in solar forecasting, where the variability of weather conditions makes purely physical models less reliable. For instance, the integration of numerical weather prediction models with ML techniques like neural networks has been shown to improve the forecasting accuracy significantly by capturing both spatial and temporal features in the data [161].
Statistical methods have traditionally been used to model linear relationships and seasonal patterns in solar energy data. However, the integration of ML algorithms, such as SVM and DL networks, enhances the model's ability to handle non-linear relationships and high-dimensional data. This synergy is particularly useful in scenarios where solar radiation data exhibits complex behaviors that are difficult to model with traditional statistical approaches alone [162].
Ensemble methods that leverage multiple models to make a collective forecast have proven highly effective in reducing forecast error. These methods benefit from the diversity among individual models, which can capture different aspects of the data. For solar forecasting, ensembles often combine various ML models to predict SI, taking advantage of each model's strengths and mitigating their weaknesses. This approach not only improves accuracy but also enhances the robustness of the predictions against individual model biases [163].
The combination of ML models with data assimilation techniques, which incorporate real-time observational data into the forecasting process, offers a powerful tool for solar forecasting. This approach allows for continuous model updates based on the latest data, enhancing the model's responsiveness to changing weather conditions. For example, data-driven models that assimilate satellite imagery and ground-based sensor readings can dynamically adjust to sudden changes in cloud cover or atmospheric conditions, providing more accurate and timelier forecasts [148].
The CNN–LSTM architecture is an innovative combination designed to leverage the strengths of both CNNs and LSTM networks. This hybrid model is particularly adept at handling visual time series prediction problems and generating textual descriptions from sequences of images. In this architecture, CNN layers are employed for feature extraction from spatial inputs, and the extracted features are then fed into the LSTM component for sequence prediction. The result is an effective system for processing and interpreting complex data sequences, as depicted in Figure 10.
The CNN–LSTM model has been applied to a wide range of problems, showcasing its versatility. Its applications include rod pumping [164], particulate matter analysis [165], waterworks monitoring [166], and heart rate signal processing [167]. Xingjian et al. [130] employed this model for predicting future rainfall intensity over short periods, demonstrating its capability to capture spatiotemporal correlations effectively. Studies highlighted that the CNN–LSTM network consistently outperformed the fully connected LSTM (FC-LSTM) model in solar forecasting, showcasing the potential of this hybrid approach in a variety of practical applications [168,169].
Transfer learning has emerged as a powerful technique in machine learning, enabling models to leverage knowledge from related tasks to improve performance on the target task. In the context of solar forecasting, transfer learning involves using pre-trained models on large datasets and fine-tuning them for specific solar forecasting tasks.
Researchers have utilized pre-trained CNNs on large image datasets, such as ImageNet, and adapted them for solar forecasting by fine-tuning sky image datasets. This approach helps capture complex spatial patterns and improve the accuracy of solar irradiance predictions. For example, Covas employed transfer learning with spatial-temporal neural networks to forecast solar magnetic fields, demonstrating the effectiveness of pre-training on sunspot data to enhance model performance on magnetic field data [170].
Transfer learning techniques have also been applied to adapt models trained in one geographical region to another. This is particularly useful in solar forecasting, where local weather patterns can vary significantly. By transferring knowledge from regions with ample data to those with sparse data, models can achieve better performance with limited local training data. Sheng et al. proposed an online domain adaptive learning approach that dynamically adjusts to changing weather conditions, enhancing the model's adaptability and accuracy across different regions [171].
Combining transfer learning with other machine learning techniques, such as ensemble learning, has shown promising results. For instance, using transfer learning to initialize models in an ensemble framework can enhance robustness and accuracy. Ren et al. developed a hybrid approach that integrates transfer learning and meta-learning for few-shot solar power forecasting, significantly improving performance with limited training data [172].
Sequential models, like LSTM networks, pre-trained on historical weather data, have been fine-tuned for short-term solar forecasting tasks. This method leverages temporal dependencies captured in large-scale weather datasets to improve short-term prediction accuracy. Zhou et al. demonstrated the effectiveness of using pre-trained LSTM models for photovoltaic power forecasting, highlighting significant improvements in prediction accuracy by fine-tuning limited local data [173]. These studies underscore the potential of transfer learning in enhancing the performance of solar forecasting models, particularly in scenarios with limited data availability. By leveraging pre-trained models and adapting them to specific solar forecasting tasks, researchers can achieve higher accuracy and more reliable predictions. The integration of transfer learning in solar forecasting not only improves model performance but also provides a practical solution to the challenges of data scarcity and variability in weather patterns.
In this section, we provide a detailed comparative analysis of various ML, DL, and Hybrid models used for SI forecasting. The performance of these models is summarized in Table 2, which highlights key metrics, including Mean Absolute Error (MAE), Root Mean Squared Error (RMSE), R2 Score, prediction time horizon, and the predicted outcome.
Model Type | Model Name | MAE (W/m2) | RMSE (W/m2) | R2 Score | Time | Predicted Outcome | Reference |
Machine Learning Models | Random Forest (RF) | 15.2 | 20.1 | 0.95 | Short-term (day-ahead) | SI | (Soleymani & Mohammadzadeh, 2023) [174] |
XGBoost | 14.8 | 19.6 | 0.96 | SI | (Soleymani & Mohammadzadeh, 2023) [174] | ||
LightGBM | 15 | 19.8 | 0.95 | SI | (Soleymani & Mohammadzadeh, 2023) [174] | ||
CatBoost | 15.1 | 20 | 0.95 | SI | (Soleymani & Mohammadzadeh, 2023) [174] | ||
BiLSTM | 0.004 | 0.009 | N/A | Short-term (minute-ahead) | SI | (Sutarna et al., 2023) [175] | |
Random Forest (RF) | 36.52 | 82.22 | 0.95 | Short-term (hourly) | SI | (Bamisile et al., 2021) [176] | |
LSTM | N/A | 0.7 | N/A | Short-term (hourly) | SI | (Sahaya Lenin et al., 2023) [177] | |
BiLSTM | 0.0043 | 0.0092 | N/A | Short-term (minute-ahead) | SI | (Sutarna et al., 2023) [175] | |
SES | 7.13 | 9.38 | 0.94 | Short-term (monthly) | SI | (Syahab et al., 2023) [178] | |
PSO-BPNN | 0.7537 | 1.7078 | N/A | Short-term (5s, 1 min) | SI | (Aljanad et al., 2021) [179] | |
XGBoost | 1.081 | 1.6988 | 0.9977 | Short-term (daily) | SI | (Mbah et al., 2022) [180] | |
Deep Learning Models | LSTM | N/A | N/A | 0.95 | Short-term (hourly) | SI | (Cha et al., 2021) [181] |
FFNN | N/A | N/A | 0.99904 | Short-term (hourly) | SI | (Reddy & Ray, 2022) [182] | |
LSTM | N/A | 0.099–0.181 | N/A | Short-term (3/6/24 hrs) | SI | (Chandola et al., 2020) [183] | |
RSAM | 0.439–2.005 | 0.463–2.390 | 0.008– 0.059 | Short-term (hourly) | SI | (Yang et al., 2023) [184] |
Figure 11a compares four ML models—RF, XGBoost, LightGBM, and CatBoost—for short-term (day-ahead) forecasting. Among these, XGBoost stands out with the lowest MAE of 14.8 W/m2 and RMSE of 19.6 W/m2, along with the highest R2 score of 0.96. This indicates that XGBoost is the most accurate model for day-ahead predictions, outperforming the other models. In contrast, Random Forest, LightGBM, and CatBoost exhibit similar performance metrics, with MAE values around 15 W/m2 and RMSE values close to 20 W/m2, all maintaining an R2 score of 0.95. These findings suggest that while XGBoost is superior, the other models provide reliable predictions with slight variations in accuracy.
Figure 11b shifts focus to a broader range of ML and DL models, comparing their MAE values. The BiLSTM model, used for minute-ahead forecasting, demonstrates exceptional accuracy with MAE values of 0.004 W/m2, significantly lower than other models. This highlights its precision in very short-term predictions. Conversely, the SES model, used for monthly forecasts, shows a considerably higher MAE of 7.130 W/m2, indicating lower accuracy for longer-term predictions. The PSO-BPNN model, with an MAE of 0.754 W/m2 for very short-term predictions, and XGBoost, with an MAE of 1.081 W/m2 for daily forecasts, perform reasonably well, but not as impressively as BiLSTM. The RSAM model exhibits variability with MAE values ranging from 0.439 to 2.005 W/m2, depending on the prediction horizon.
Figure 11c provides an RMSE comparison across the same set of models. The BiLSTM model again shows superior performance with RMSE values of 0.009 W/m2 for minute-ahead forecasting, reinforcing its accuracy and reliability. The LSTM model, used for hourly forecasts, has a higher RMSE of 0.700 W/m2, indicating moderate accuracy. The SES model, with an RMSE of 9.380 W/m2 for monthly predictions, confirms its lower reliability for longer-term forecasting. PSO-BPNN and XGBoost models exhibit RMSE values of 1.708 W/m2 and 1.699 W/m2, respectively, showing good performance for their specific prediction periods. The RSAM model's RMSE ranges from 1.550 to 2.390 W/m2, reflecting its varying accuracy across different forecast intervals.
The analysis underscores the varying strengths of different models based on their prediction horizons. The BiLSTM model consistently excels in short-term (minute-ahead) forecasting, while XGBoost is most effective for short-term (day-ahead and daily) predictions among the ML models. Models like SES show less accuracy for longer-term forecasts, highlighting the importance of selecting models that align with specific forecasting needs. This comprehensive comparison illustrates the critical role of model choice in achieving accurate solar irradiance predictions.
The continuation of our comparative analysis now includes an evaluation of hybrid deep learning models for SI forecasting, as presented in Table 3. These models integrate various deep learning techniques, often combining CNN, LSTM networks, and other advanced architectures to enhance prediction accuracy and efficiency.
Model Type | Model Name | MAE (W/m2) | RMSE (W/m2) | R2 Score | Time | Predicted Outcome | Reference |
Hybrid Deep Learning | WTP-GAN | N/A | 0.0473–0.0946 | N/A | Short-term (1–6 pace) | SI | (Meng et al., 2021) [185] |
DL-NN | N/A | 12.1 | N/A | Short-term (hourly) | SI | (Kartini et al., 2022) [186] | |
RLMD BiLSTM | 16.34–35.07 | 1.81–28.46 | 0.977–0.995 | Short-term (1–3 steps) | SI | (Singla et al., 2022) [187] | |
CNN-LSTM | N/A | 36.24 | N/A | Short-term (5–30 min) | SI | (Marinho et al., 2022) [188] | |
LSTM-CNN | N/A | N/A | 0.37–0.45 | Short-term (hourly) | SI | (Kumari & Toshniwal, 2021) [189] | |
CNN-LSTM | N/A | 0.36 | 0.98 | Short-term (hourly) | SI | (Michael et al., 2022) [190] | |
Bi-LSTM-VMD-Grid Search | N/A | 5.456 | 0.924 | Short-term (hourly) | SI | (Srivastava & Gupta, 2023) [191] | |
ResTrans | 0.031 | 0.049 | 0.97 | Short-term (hourly) | SI | (Ziyabari et al., 2023) [192] |
For instance, the Wavelet Transform Package-Generative Adversarial Network (WTP-GAN) shows an RMSE range of 0.0473–0.0946 W/m2 for short-term (1–6 pace) forecasting, indicating high accuracy. The Deep Learning Neural Network (DL-NN) has an RMSE of 12.1 W/m2 for hourly predictions, demonstrating moderate performance. The Complete Ensemble Empirical Mode Decomposition with Adaptive Noise-BiLSTM (CEEMDAN-BiLSTM) shows a MAE range of 16.34–35.07 W/m2 and an RMSE range of 1.81–28.46 W/m2 for short-term (1–3 steps) forecasting, with an impressive R2 score range of 0.977–0.995, highlighting its strong predictive capability.
The CNN-LSTM model exhibits an RMSE of 36.24 W/m2 for short-term (5–30 minutes) forecasting, while the LSTM-CNN model's R2 score ranges from 0.37 to 0.45 for hourly predictions, showing variable performance. Another instance of the CNN-LSTM model demonstrates a low RMSE of 0.36 W/m2 and a high R2 score of 0.98 for hourly forecasting, indicating excellent accuracy. The Bi-LSTM-VMD-Grid Search model has an RMSE of 5.456 W/m2 and an R2 score of 0.924 for hourly predictions, showing robust performance. The ResTrans model, with an MAE of 0.031 W/m2, an RMSE of 0.049 W/m2, and an R2 score of 0.97 for hourly forecasts, stands out for its precision.
Figure 12 further illustrates the R2 score comparison among these hybrid deep learning models. The CNN-LSTM model shows the highest R2 score of 0.989, underscoring its superior accuracy in short-term predictions. The LSTM-CNN model follows with an R2 score of 0.42, indicating lower accuracy compared to other models. The Bi-LSTM-VMD-Grid Search model achieves an R2 score of 0.924, demonstrating strong predictive performance. The ResTrans model, with an R2 score of 0.97, also indicates high accuracy in hourly forecasts. These results highlight the effectiveness of combining different neural network architectures to improve the accuracy and reliability of solar irradiance forecasts.
In summary, the analysis of hybrid deep learning models reveals that the integration of various deep learning techniques significantly enhances the predictive capabilities of these models. The CNN-LSTM and ResTrans models, in particular, stand out for their high R2 scores, indicating superior performance in short-term forecasting. This comparative evaluation underscores the importance of selecting appropriate hybrid models to achieve precise and reliable solar irradiance predictions, tailored to specific forecasting needs.
The rapid development of ML and DL models has significantly advanced the field of solar energy forecasting. We highlight the transformative potential of these technologies in overcoming the limitations of traditional empirical and physical models. Each class of model—whether empirical, statistical, or ML/DL-based—offers unique strengths and faces specific challenges, emphasizing the need for a hybrid approach that combines the best attributes of each method.
ML and DL models have proven superior in handling the nonlinearities and complexities inherent in SI data. Models such as SVM, ANN, and more sophisticated architectures like CNN and LSTM networks demonstrate remarkable accuracy improvements over traditional models [7,12,13]. Their ability to learn from vast datasets and adapt to new data inputs offers a significant advantage, particularly in dynamic environments where weather patterns and solar output can vary widely.
The performance of these models is rooted in their capacity to learn intricate patterns within the data. For instance, SVMs are particularly effective in identifying the optimal hyperplane that separates different classes in the data, enhancing prediction accuracy [12]. Similarly, ANNs, inspired by the human brain's neural architecture, consist of interconnected neurons that process data through multiple layers, enabling the extraction of high-level features from raw input [7]. CNNs are adept at processing grid-like data, such as images or spatial data, making them suitable for applications requiring detailed spatial analysis. RNNs, and LSTMs in particular, excel in modeling temporal dependencies, making them ideal for time-series forecasting tasks like predicting SI over time [13].
The adaptability and scalability of ML and DL models are significant advantages over traditional methods. These models can continuously update predictions based on new data inputs, which is critical for real-time applications. Domain adaptive learning models, for instance, dynamically adjust to new weather conditions, significantly improving prediction reliability without the need for constant retraining [171]. This adaptability is juxtaposed with the static nature of NWP models, which require manual updates to incorporate new data or changing atmospheric conditions.
Real-world applications of advanced solar forecasting models extend beyond academic research into practical scenarios, contributing significantly to economic benefits and policy implications. Enhanced forecasting accuracy reduces operational costs by optimizing energy production and minimizing reliance on expensive backup power solutions, particularly in grid management, where precise forecasts can decrease imbalance penalties and improve efficiency [21,23,27] Accurate forecasts support more informed policy decisions, facilitating renewable energy integration into national grids and promoting sustainable energy practices [25,26]. For example, improvements in solar power forecasting can significantly reduce operational electricity generation costs by decreasing fuel and maintenance expenses, as well as start and shutdown costs for fossil-fueled generators [21]. Furthermore, these models enhance economic efficiency and grid reliability by decreasing the reliance on peak-time energy reserves, thus making solar energy more competitive against traditional sources [23].
Accurate solar forecasts are crucial for shaping effective renewable energy policies, enabling governments to establish incentives for solar adoption and create subsidies for expanding capacity. These forecasts aid in long-term energy planning, setting ambitious renewable energy targets, and justifying the expansion of grid infrastructure like energy storage systems essential for managing solar power's intermittency [25] Improved forecasting accuracy has led to significant investments in battery storage systems, stabilizing the grid during peak demand periods, and supporting economic development and energy security in developing economies like India [25]. Enhanced solar forecasting models align closely with global sustainability goals by optimizing the use of solar resources, thus contributing to a more sustainable and resilient energy infrastructure. These models support the development of energy storage systems, crucial for balancing supply and demand and ensuring grid stability [28,29,193]. Improved forecasts can also help reduce CO2 emissions by up to 6% annually, supporting environmental goals while fostering economic resilience by diversifying energy sources and mitigating the impacts of volatile fossil fuel markets [26].
Despite significant advancements in ML and DL models for solar forecasting, several challenges persist that hinder their broader applicability and adoption.
The effectiveness of ML and DL models is highly dependent on the availability of extensive, high-quality historical data. In regions with insufficient data, the models may perform suboptimally, limiting their usefulness. This dependency poses a significant challenge for implementing these advanced models in areas where historical solar irradiance data is sparse or unreliable [31,90].
One of the primary barriers to the widespread adoption of DL models is their interpretability. These models, particularly deep neural networks, often operate as "black boxes", making it difficult for stakeholders to understand how forecasts are generated. Stakeholders, including policymakers and energy practitioners, require clear and transparent explanations of model outputs to make informed decisions. The lack of interpretability can impede trust and acceptance of these advanced forecasting models [31,90].
Ongoing research is needed to enhance the quality and availability of historical solar data. Efforts should focus on employing techniques for data augmentation to generate synthetic data, which can help mitigate the scarcity of historical records. Additionally, utilizing remote sensing technologies and satellite data can fill gaps in ground-based measurements, providing more comprehensive datasets. Developing standardized protocols for data collection and preprocessing is also crucial to ensure consistency and reliability across different regions, further improving the robustness and applicability of solar forecasting models.
Interpreting the outputs of solar forecasting models is crucial for their practical application in energy management and decision-making. Enhancing the interpretability of these models can significantly improve their usability for a broader range of stakeholders. We review various methods to enhance the interpretability of solar forecasting models, drawing on recent research findings.
• Feature importance analysis
Feature importance analysis techniques, such as SHAP (SHapley Additive exPlanations) values and LIME (Local Interpretable Model-agnostic Explanations), help in understanding the contribution of each feature to the model's predictions. These methods provide insights into which variables most influence solar irradiance forecasts. For instance, a study by Chaibi et al. demonstrated the utility of SHAP in explaining the importance of extraterrestrial solar radiation and sunshine duration in global solar radiation estimation [194].
• Visualizations
Graphical representations, such as partial dependence plots and decision trees, can make complex models more understandable. These visual tools help stakeholders grasp how different factors interact to affect the model's output. Mason et al. introduced a tool that combines interactive visualization with empirical dynamic modeling to enhance the interpretability of solar forecasts [195].
• Model simplification
Simplifying complex models without significantly compromising accuracy can also improve interpretability. Ensemble methods, like Random Forests, can be pruned to reduce complexity while maintaining performance. Rafati et al. explored the effectiveness of data-driven heuristic methods to improve the accuracy of short-term solar power forecasting, showing that simpler models could perform effectively [196].
• Transparent modeling techniques
Utilizing inherently interpretable models, such as linear regression and decision trees, can sometimes be preferable. Even though these models might not always provide the highest accuracy, their transparency makes them easier to interpret and trust. Wang et al. proposed an explainable neural network that mathematically interprets the relationship between input features and solar irradiance predictions, offering a clear advantage in interpretability [197].
• Case-based reasoning
Providing case studies or examples of specific predictions and their corresponding inputs can help illustrate how the model arrives at its conclusions. This method can be particularly effective in demonstrating the practical application of the forecasting model. For example, Theocharides et al. validated their machine learning-based photovoltaic power production forecasting model in different climatic conditions, enhancing its practical relevance and interpretability [198].
By implementing these methods, we can enhance the transparency and usability of solar forecasting models, making them more accessible to a wider range of stakeholders, including non-experts. Improving interpretability not only aids in trust and acceptance of the models but also facilitates better decision-making in solar energy management.
Researchers should focus on enhancing the robustness of ML and DL models against data variability. This involves developing models that can adapt to new and diverse datasets without requiring extensive retraining, known as domain adaptation. Additionally, employing regularization methods to prevent overfitting can improve model generalization across different geographical regions, ensuring that models perform consistently under varying conditions.
To ensure the applicability of advanced forecasting models globally, efforts should be directed toward improving their generalizability. This includes training models on diverse datasets from multiple regions to enhance their performance in varied climatic conditions, known as cross-regional training. Leveraging transfer learning techniques allows knowledge gained from well-studied regions to be applied to areas with limited data, further extending the usability of these models.
Integrating ML and DL models with traditional forecasting methods can harness the strengths of both approaches, leading to more reliable and accurate forecasts. This hybrid approach can enhance model performance by combining statistical methods with advanced ML models, leveraging their complementary strengths. Additionally, it can facilitate adoption by providing a gradual transition for stakeholders familiar with traditional methods, easing the integration of advanced technologies into existing systems.
In conclusion, the evolution of solar forecasting from empirical models to advanced ML and DL techniques marks a significant milestone in renewable energy research. The integration of hybrid models combining physical, statistical, and ML approaches offers the most promising path forward, enhancing accuracy and reliability. Real-world applications of these models demonstrate substantial economic benefits and support policy-making for sustainable energy development. Researchers should focus on overcoming current challenges, particularly in data quality and model interpretability, to fully harness the potential of these advanced forecasting techniques.
In this review, we explored the transformative impact of ML and DL models on solar energy forecasting. We examined the evolution of forecasting methods from empirical and physical models to the advanced ML and DL approaches, highlighting the strengths, challenges, and potential of each. The integration of traditional methods with ML and DL models was emphasized to enhance forecasting accuracy and reliability. The crux of this review is as follows;
• ML and DL models have significantly improved the accuracy of SI forecasts by handling nonlinearities and complex patterns in the data [7,12,13]. These models offer superior adaptability and scalability, allowing continuous updates and adjustments based on new data inputs, which is crucial for real-time applications [171].
• Enhanced forecasting accuracy reduces operational costs by optimizing energy production and minimizing reliance on expensive backup power solutions, resulting in lower kWh costs and improved economic efficiency [21,23,27].
• Accurate solar forecasts support more informed policy decisions, facilitating the integration of renewable energy into national grids and promoting sustainable energy practices [25,26].
• Improved forecasts enhance grid reliability by allowing better anticipation of fluctuations in solar output and optimizing the scheduling of energy production from various sources [28,29,193].
• Enhanced forecasting models contribute to global sustainability goals by reducing CO2 emissions and promoting the efficient use of solar resources, supporting environmental and economic resilience [26].
• The dependency on high-quality, extensive historical data limits the applicability of ML models in regions with insufficient data. Future research should focus on improving data quality and availability [31,90].
• The complexity and interpretability of DL models pose challenges for widespread adoption. Developing more interpretable models is essential for broader acceptance among stakeholders [31,90].
• Enhancing the robustness and generalizability of ML and DL models across geographical regions is critical for their effective deployment in diverse environments.
In conclusion, we highlight the significant advancements in solar forecasting, transitioning from empirical models to sophisticated ML and DL techniques, emphasizing the novel integration of hybrid models that combine physical, statistical, and ML approaches. This evolution marks a crucial milestone in renewable energy research, offering enhanced accuracy and reliability. The real-world applications of these advanced models demonstrate substantial economic benefits and support effective policy-making for sustainable energy development. Addressing current challenges, particularly in data quality and model interpretability, is essential to fully harness the potential of these innovative forecasting techniques.
Conceptualization, A.N. and M.F.H.; methodology, A.N. and M.F.H.; software, M.F.H.; validation, M.S.N., M.T.H., and N.H.; formal analysis, A.N. and M.F.H.; investigation, A.N. and M.F.H.; resources, M.G.; data curation, M.S.N.; writing—original draft preparation, A.N. and M.F.H.; writing—review and editing, M.S.N., M.T.H., M.G., N.H., and J.M.; visualization, A.N. and M.F.H.; supervision, J.M.; project administration, J.M. All authors have read and agreed to the published version of the manuscript.
The authors declare they have not used Artificial Intelligence (AI) tools in the creation of this article.
The authors declare no conflict of interest.
[1] | L. V. Ahlfors, Sufficient conditions for quasiconformal extension, Ann. Math. Stud., 79 (1974), 23-29. |
[2] | H. A. Al-Kharsani, A. M. Al-Zahrani, S. S. Al-Hajri, T. K. Pogány, Univalence criteria for linear fractional differential operators associated with a generalized Bessel function, Math. Commun. 21 (2016), 171-188. |
[3] |
Á. Baricz, D. K. Dimitrov, H. Orhan, N. Yağmur, Radii of starlikeness of some special functions, Proc. Amer. Math. Soc., 144 (2016), 3355-3367. doi: 10.1090/proc/13120
![]() |
[4] |
Á. Baricz, B. A. Frasin, Univalence of integral operators involving Bessel functions, Appl. Math. Lett., 23 (2010), 371-376. doi: 10.1016/j.aml.2009.10.013
![]() |
[5] |
Á. Baricz, S. Koumandos, Turán type inequalities for some Lommel function of the first kind, Proc. Edinburgh Math. Soc., 59 (2016), 569-579. doi: 10.1017/S0013091515000413
![]() |
[6] |
Á. Baricz, R. Szász, Close-to-convexity of some special functions and their derivatives, Bull. Malays. Math. Sci. Soc., 39 (2016), 427-437. doi: 10.1007/s40840-015-0180-7
![]() |
[7] | J. Becker, Löwnersche Differentialgleichung und quasikonform fortsetzbare schlichte funktionen, J. Reine Angew. Math., 255 (1972), 23-43. |
[8] |
J. Becker, Löwnersche Differentialgleichung und Schlichtheitskriterien, Math. Ann., 202 (1973), 321-335. doi: 10.1007/BF01433462
![]() |
[9] | S. D. Bernardi, Convex and starlike univalent functions, Trans. Amer. Math. Soc., 35 (1969), 429-446. |
[10] | D. Breaz, N. Breaz, Univalence conditions for certain integral operators, Stud. Univ. Babeş-Bolyai Math., 47 (2002), 9-15. |
[11] | D. Breaz, N. Breaz, Univalence of an integral operator, Mathematica (Cluj), 47 (2005), 35-38. |
[12] |
D. Breaz, N. Breaz, H. M. Srivastava, An extension of the univalent condition for a family of integral operators, Appl. Math. Lett., 22 (2009), 41-44. doi: 10.1016/j.aml.2007.11.008
![]() |
[13] | D. Breaz, S. Owa, N. Breaz, A new integral univalent operator, Acta Univ. Apulensis Math. Inform., 16 (2008), 11-16. |
[14] | B. C. Carlson, D. B. Shaffer, Starlike and prestarlike hypergeometric functions, SIAM J. Math. Anal., 159 (1984), 737-745. |
[15] |
N. E. Cho, O. S. Kwon, H. M. Srivastava, Inclusion relationships and argument properties for certain subclasses of multivalent functions associated with a family of linear operators, J. Math. Anal. Appl., 292 (2004), 470-483. doi: 10.1016/j.jmaa.2003.12.026
![]() |
[16] |
J. H. Choi, M. Saigo, H. M. Srivastava, Some inclusion properties of a certain family of integral operators, J. Math. Anal. Appl., 276 (2002), 432-445. doi: 10.1016/S0022-247X(02)00500-0
![]() |
[17] | E. Deniz, H. Orhan, H. M. Srivastava, Some sufficient conditions for univalence of certain families of integral operators involving generalized Bessel functions, Taiwanese J. Math., 15 (2011), 883-917. |
[18] |
E. Deniz, Convexity of integral operators involving generalized Bessel functions, Integral Transforms Spec. Funct., 24 (2013), 201-216. doi: 10.1080/10652469.2012.685938
![]() |
[19] | A. W. Goodman, Univalent Functions, Mariner Publishing Company Incorporated, Tampa, FL, 1983. |
[20] | Y. J. Kim, E. P. Merkes, On an integral of powers of a spirallike function, Kyungpook Math. J., 12 (1972), 249-252. |
[21] |
R. J. Libera, Some classes of regular univalent functions, Proc. Amer. Math. Soc., 16 (1965), 755-758. doi: 10.1090/S0002-9939-1965-0178131-2
![]() |
[22] |
J. L. Liu, The Noor integral and strongly starlike functions, J. Math. Anal. Appl., 261 (2001), 441-447. doi: 10.1006/jmaa.2001.7489
![]() |
[23] |
E. Lommel, Über eine mit den Bessel'schen Functionen verwandte Function, Math. Ann., 9 (1875), 425-444. doi: 10.1007/BF01443342
![]() |
[24] | S. Moldoveanu, N. N. Pascu, Integral operators which preserve the univalence, Mathematica (Cluj), 32 (1990), 159-166. |
[25] | K. I. Noor, On new classes of integral operators, J. Natur. Geom., 16 (1999), 71-80. |
[26] |
K. I. Noor, H. A. Alkhorasani, Properties of close-to-convexity preserved by some integral operators, J. Math. Anal. Appl., 112 (1985), 509-516. doi: 10.1016/0022-247X(85)90260-4
![]() |
[27] |
S. Owa, H. M. Srivastava, Univalent and starlike generalized hypergeometric functions, Canad. J. Math., 39 (1987), 1057-1077. doi: 10.4153/CJM-1987-054-3
![]() |
[28] | J. H. Park, Coefficient Estimates and Univalancy of Certain Analytic Functions, Ph. D. Thesis, Pukyong National University, Busan, 2018. |
[29] | N. N. Pascu, An improvement of Becker's univalence criterion, In: Proceedings of the Commemorative Session: Simon Stoilow (Brasov, 1987), 43-48. |
[30] | V. Pescar, A new generalization of Ahlfors and Becker's critetion of univalence, Bull. Malays. Math. Sci. Soc., 19 (1996), 53-54. |
[31] | V. Pescar, Univalence of certain integral operators, Acta Univ. Apulensis Math. Inform., 12 (2006), 43-48. |
[32] | J. A. Pfaltzgraff, Univalence of the integral of (f′(z))λ, Bull. London Math. Soc., 7 (1975), 254-256. |
[33] | R. Raza, S. Noreen, S. N. Malik, Geometric properties of integral operators defined by Bessel functions, J. Inequal. Spec. Funct., 7 (2016), 34-48. |
[34] |
S. Ruscheweyh, New criteria for univalent functions, Proc. Amer. Math. Soc., 49 (1975), 109-115. doi: 10.1090/S0002-9939-1975-0367176-1
![]() |
[35] | N. Seenivasagan, D. Breaz, Certain sufficient conditions for univalence, Gen. Math., 15 (2007), 7-15. |
[36] |
H. M. Srivastava, Operators of basic (or q-) calculus and fractional q-calculus and their applications in geometric function theory of complex analysis, Iran. J. Sci. Technol. Trans. A: Sci., 44 (2020), 327-344. doi: 10.1007/s40995-019-00815-0
![]() |
[37] | H. M. Srivastava, E. Deniz, H. Orhan, Some general univalence criteria for a family of integral operators, Appl. Math. Comput., 215 (2010), 3696-3701. |
[38] |
H. M. Srivastava, B. A. Frasin, V. Pescar, Univalence of integral operators involving Mittag-Leffler functions, Appl. Math. Inform. Sci., 11 (2017), 635-641. doi: 10.18576/amis/110301
![]() |
[39] | H. M. Srivastava, R. Jan, A. Jan, W. Deebai, M. Shutaywi, Fractional-calculus analysis of the transmission dynamics of the dengue infection, Chaos, 31 (2021), Article ID 53130, 1-18. |
[40] |
H. M. Srivastava, A. R. S. Juma, H. M. Zayed, Univalence conditions for an integral operator defined by a generalization of the Srivastava-Attiya operator, Filomat, 32 (2018), 2101-2114. doi: 10.2298/FIL1806101S
![]() |
[41] |
H. M. Srivastava, S. Owa, Some characterization and distortion theorems involving fractional calculus, generalized hypergeometric functions, Hadamard products, linear operators, and certain subclasses of analytic functions, Nagoya Math. J., 106 (1987), 1-28. doi: 10.1017/S0027763000000854
![]() |
[42] | H. M. Srivastava, S. Owa, Univalent Functions, Fractional Calculus, and Their Applications, Halsted Press (Ellis Horwood Limited, Chichester), John Wiley and Sons, New York, Chichester, Brisbane, and Toronto, 1989. |
[43] |
H. M. Srivastava, J. K. Prajapat, G. I. Oros, R. Şendruţiu, Geometric properties of a certain family of integral operators, Filomat, 28 (2014), 745-754. doi: 10.2298/FIL1404745S
![]() |
[44] | L. F. Stanciu, D. Breaz, H. M. Srivastava, Some criteria for univalence of a certain integral operator, Novi Sad J. Math., 43 (2013), 51-57. |
[45] |
N. Yağmur, Hardy space of Lommel functions, Bull. Korean Math. Soc., 52 (2015), 1035-1046. doi: 10.4134/BKMS.2015.52.3.1035
![]() |
1. | M. S. Naveed, M. F. Hanif, M. Metwaly, I. Iqbal, E. Lodhi, X. Liu, J. Mi, Leveraging advanced AI algorithms with transformer-infused recurrent neural networks to optimize solar irradiance forecasting, 2024, 12, 2296-598X, 10.3389/fenrg.2024.1485690 | |
2. | Manvi Gupta, Archie Arya, Uday Varshney, Jishnu Mittal, Anuradha Tomar, A review of PV power forecasting using machine learning techniques, 2025, 29504252, 100058, 10.1016/j.pes.2025.100058 | |
3. | Girijapati Sharma, Subhash Chandra, Arvind Kumar Yadav, Rahul Gupta, Enhancing solar radiation forecasting accuracy with a hybrid SA-Bi-LSTM-Bi-GRU model, 2025, 18, 1865-0473, 10.1007/s12145-025-01791-3 | |
4. | Molaka Maruthi, Bubryur Kim, Song Sujeen, Jinwoo An, Zengshun Chen, Multi-model integration for dynamic forecasting (MIDF): a framework for wind speed and direction prediction, 2025, 58, 1573-7462, 10.1007/s10462-025-11140-x |
Data Type | Source | Description | References | Link |
Solar Irradiance | SoDa Service | Historical solar radiation data, crucial for model training and validation | Freitas et al., [34], Gaye et al., [36] |
https://www.soda-pro.com/ |
National Renewable Energy Laboratory (NREL) | Solar resource data and tools, including the National Solar Radiation Database (NSRDB) | Natei et al., [38] Li et al., [39], Long et al., [40] |
https://data.nrel.gov/ | |
European Commission's JRC-Photovoltaic Geographical Information System (PVGIS) | Solar radiation and photovoltaic performance data for Europe, Africa, and Asia | Freitas et al., [34], Gaye et al., [36] | https://joint-research-centre.ec.europa.eu/photovoltaic-geographical-information-system-pvgis_en | |
HelioClim Database | Satellite-derived solar radiation data | Jayalakshmi et al., [37], Jebli et al., [41] |
https://www.soda-pro.com/help/helioclim/helioclim-3-overview | |
Copernicus Atmosphere Monitoring Service (CAMS) | Solar radiation data derived from satellite observations and atmospheric models | Khandakar et al., [42], Jayalakshmi et al., [37] |
https://atmosphere.copernicus.eu/ | |
Geographical | PVGIS | Latitude, longitude, altitude, and slope data for specific locations | Jebli et al., [41], Kim et al., [43] | https://joint-research-centre.ec.europa.eu/photovoltaic-geographical-information-system-pvgis_en |
Calendar | NASA POWER | Time zone, hour of the day, month, and day of the year data capturing seasonal and diurnal variations | Jayalakshmi et al., [37], Natei et al., [38] |
https://power.larc.nasa.gov/ |
Astronomical | National Renewable Energy Laboratory (NREL) | Solar elevation angle, hour angle, and solar zenith angle for modeling sun's position | Li et al., [39], Long et al., [40] | https://data.nrel.gov/ |
Satellite-Based Data | MODIS | Provides consistent spatial coverage, crucial for global meteorological monitoring | Khandakar et al., [42], Jayalakshmi et al., [37] |
https://modis.gsfc.nasa.gov/data/ |
Meteorological | World Radiation Data Centre (WRDC) | Solar radiation data from ground-based observation stations around the world | Natei et al., [38]; Khandakar et al., [42] | http://wrdc.mgo.rssi.ru/ |
Meteonorm | Solar and meteorological data for various locations, using ground-based measurements and satellite data | Freitas et al., [34]; Jayalakshmi et al., [37] | https://www.pvsyst.com/help/meteo_source_meteonorm.htm | |
Korea Meteorological Administration (KMA) | Atmospheric parameters like temperature, humidity, and sunshine duration | Natei et al., [38], Gutiérrez et al., [44] | https://www.kma.go.kr/neng/index.do |
Model Type | Model Name | MAE (W/m2) | RMSE (W/m2) | R2 Score | Time | Predicted Outcome | Reference |
Machine Learning Models | Random Forest (RF) | 15.2 | 20.1 | 0.95 | Short-term (day-ahead) | SI | (Soleymani & Mohammadzadeh, 2023) [174] |
XGBoost | 14.8 | 19.6 | 0.96 | SI | (Soleymani & Mohammadzadeh, 2023) [174] | ||
LightGBM | 15 | 19.8 | 0.95 | SI | (Soleymani & Mohammadzadeh, 2023) [174] | ||
CatBoost | 15.1 | 20 | 0.95 | SI | (Soleymani & Mohammadzadeh, 2023) [174] | ||
BiLSTM | 0.004 | 0.009 | N/A | Short-term (minute-ahead) | SI | (Sutarna et al., 2023) [175] | |
Random Forest (RF) | 36.52 | 82.22 | 0.95 | Short-term (hourly) | SI | (Bamisile et al., 2021) [176] | |
LSTM | N/A | 0.7 | N/A | Short-term (hourly) | SI | (Sahaya Lenin et al., 2023) [177] | |
BiLSTM | 0.0043 | 0.0092 | N/A | Short-term (minute-ahead) | SI | (Sutarna et al., 2023) [175] | |
SES | 7.13 | 9.38 | 0.94 | Short-term (monthly) | SI | (Syahab et al., 2023) [178] | |
PSO-BPNN | 0.7537 | 1.7078 | N/A | Short-term (5s, 1 min) | SI | (Aljanad et al., 2021) [179] | |
XGBoost | 1.081 | 1.6988 | 0.9977 | Short-term (daily) | SI | (Mbah et al., 2022) [180] | |
Deep Learning Models | LSTM | N/A | N/A | 0.95 | Short-term (hourly) | SI | (Cha et al., 2021) [181] |
FFNN | N/A | N/A | 0.99904 | Short-term (hourly) | SI | (Reddy & Ray, 2022) [182] | |
LSTM | N/A | 0.099–0.181 | N/A | Short-term (3/6/24 hrs) | SI | (Chandola et al., 2020) [183] | |
RSAM | 0.439–2.005 | 0.463–2.390 | 0.008– 0.059 | Short-term (hourly) | SI | (Yang et al., 2023) [184] |
Model Type | Model Name | MAE (W/m2) | RMSE (W/m2) | R2 Score | Time | Predicted Outcome | Reference |
Hybrid Deep Learning | WTP-GAN | N/A | 0.0473–0.0946 | N/A | Short-term (1–6 pace) | SI | (Meng et al., 2021) [185] |
DL-NN | N/A | 12.1 | N/A | Short-term (hourly) | SI | (Kartini et al., 2022) [186] | |
RLMD BiLSTM | 16.34–35.07 | 1.81–28.46 | 0.977–0.995 | Short-term (1–3 steps) | SI | (Singla et al., 2022) [187] | |
CNN-LSTM | N/A | 36.24 | N/A | Short-term (5–30 min) | SI | (Marinho et al., 2022) [188] | |
LSTM-CNN | N/A | N/A | 0.37–0.45 | Short-term (hourly) | SI | (Kumari & Toshniwal, 2021) [189] | |
CNN-LSTM | N/A | 0.36 | 0.98 | Short-term (hourly) | SI | (Michael et al., 2022) [190] | |
Bi-LSTM-VMD-Grid Search | N/A | 5.456 | 0.924 | Short-term (hourly) | SI | (Srivastava & Gupta, 2023) [191] | |
ResTrans | 0.031 | 0.049 | 0.97 | Short-term (hourly) | SI | (Ziyabari et al., 2023) [192] |
Data Type | Source | Description | References | Link |
Solar Irradiance | SoDa Service | Historical solar radiation data, crucial for model training and validation | Freitas et al., [34], Gaye et al., [36] |
https://www.soda-pro.com/ |
National Renewable Energy Laboratory (NREL) | Solar resource data and tools, including the National Solar Radiation Database (NSRDB) | Natei et al., [38] Li et al., [39], Long et al., [40] |
https://data.nrel.gov/ | |
European Commission's JRC-Photovoltaic Geographical Information System (PVGIS) | Solar radiation and photovoltaic performance data for Europe, Africa, and Asia | Freitas et al., [34], Gaye et al., [36] | https://joint-research-centre.ec.europa.eu/photovoltaic-geographical-information-system-pvgis_en | |
HelioClim Database | Satellite-derived solar radiation data | Jayalakshmi et al., [37], Jebli et al., [41] |
https://www.soda-pro.com/help/helioclim/helioclim-3-overview | |
Copernicus Atmosphere Monitoring Service (CAMS) | Solar radiation data derived from satellite observations and atmospheric models | Khandakar et al., [42], Jayalakshmi et al., [37] |
https://atmosphere.copernicus.eu/ | |
Geographical | PVGIS | Latitude, longitude, altitude, and slope data for specific locations | Jebli et al., [41], Kim et al., [43] | https://joint-research-centre.ec.europa.eu/photovoltaic-geographical-information-system-pvgis_en |
Calendar | NASA POWER | Time zone, hour of the day, month, and day of the year data capturing seasonal and diurnal variations | Jayalakshmi et al., [37], Natei et al., [38] |
https://power.larc.nasa.gov/ |
Astronomical | National Renewable Energy Laboratory (NREL) | Solar elevation angle, hour angle, and solar zenith angle for modeling sun's position | Li et al., [39], Long et al., [40] | https://data.nrel.gov/ |
Satellite-Based Data | MODIS | Provides consistent spatial coverage, crucial for global meteorological monitoring | Khandakar et al., [42], Jayalakshmi et al., [37] |
https://modis.gsfc.nasa.gov/data/ |
Meteorological | World Radiation Data Centre (WRDC) | Solar radiation data from ground-based observation stations around the world | Natei et al., [38]; Khandakar et al., [42] | http://wrdc.mgo.rssi.ru/ |
Meteonorm | Solar and meteorological data for various locations, using ground-based measurements and satellite data | Freitas et al., [34]; Jayalakshmi et al., [37] | https://www.pvsyst.com/help/meteo_source_meteonorm.htm | |
Korea Meteorological Administration (KMA) | Atmospheric parameters like temperature, humidity, and sunshine duration | Natei et al., [38], Gutiérrez et al., [44] | https://www.kma.go.kr/neng/index.do |
Model Type | Model Name | MAE (W/m2) | RMSE (W/m2) | R2 Score | Time | Predicted Outcome | Reference |
Machine Learning Models | Random Forest (RF) | 15.2 | 20.1 | 0.95 | Short-term (day-ahead) | SI | (Soleymani & Mohammadzadeh, 2023) [174] |
XGBoost | 14.8 | 19.6 | 0.96 | SI | (Soleymani & Mohammadzadeh, 2023) [174] | ||
LightGBM | 15 | 19.8 | 0.95 | SI | (Soleymani & Mohammadzadeh, 2023) [174] | ||
CatBoost | 15.1 | 20 | 0.95 | SI | (Soleymani & Mohammadzadeh, 2023) [174] | ||
BiLSTM | 0.004 | 0.009 | N/A | Short-term (minute-ahead) | SI | (Sutarna et al., 2023) [175] | |
Random Forest (RF) | 36.52 | 82.22 | 0.95 | Short-term (hourly) | SI | (Bamisile et al., 2021) [176] | |
LSTM | N/A | 0.7 | N/A | Short-term (hourly) | SI | (Sahaya Lenin et al., 2023) [177] | |
BiLSTM | 0.0043 | 0.0092 | N/A | Short-term (minute-ahead) | SI | (Sutarna et al., 2023) [175] | |
SES | 7.13 | 9.38 | 0.94 | Short-term (monthly) | SI | (Syahab et al., 2023) [178] | |
PSO-BPNN | 0.7537 | 1.7078 | N/A | Short-term (5s, 1 min) | SI | (Aljanad et al., 2021) [179] | |
XGBoost | 1.081 | 1.6988 | 0.9977 | Short-term (daily) | SI | (Mbah et al., 2022) [180] | |
Deep Learning Models | LSTM | N/A | N/A | 0.95 | Short-term (hourly) | SI | (Cha et al., 2021) [181] |
FFNN | N/A | N/A | 0.99904 | Short-term (hourly) | SI | (Reddy & Ray, 2022) [182] | |
LSTM | N/A | 0.099–0.181 | N/A | Short-term (3/6/24 hrs) | SI | (Chandola et al., 2020) [183] | |
RSAM | 0.439–2.005 | 0.463–2.390 | 0.008– 0.059 | Short-term (hourly) | SI | (Yang et al., 2023) [184] |
Model Type | Model Name | MAE (W/m2) | RMSE (W/m2) | R2 Score | Time | Predicted Outcome | Reference |
Hybrid Deep Learning | WTP-GAN | N/A | 0.0473–0.0946 | N/A | Short-term (1–6 pace) | SI | (Meng et al., 2021) [185] |
DL-NN | N/A | 12.1 | N/A | Short-term (hourly) | SI | (Kartini et al., 2022) [186] | |
RLMD BiLSTM | 16.34–35.07 | 1.81–28.46 | 0.977–0.995 | Short-term (1–3 steps) | SI | (Singla et al., 2022) [187] | |
CNN-LSTM | N/A | 36.24 | N/A | Short-term (5–30 min) | SI | (Marinho et al., 2022) [188] | |
LSTM-CNN | N/A | N/A | 0.37–0.45 | Short-term (hourly) | SI | (Kumari & Toshniwal, 2021) [189] | |
CNN-LSTM | N/A | 0.36 | 0.98 | Short-term (hourly) | SI | (Michael et al., 2022) [190] | |
Bi-LSTM-VMD-Grid Search | N/A | 5.456 | 0.924 | Short-term (hourly) | SI | (Srivastava & Gupta, 2023) [191] | |
ResTrans | 0.031 | 0.049 | 0.97 | Short-term (hourly) | SI | (Ziyabari et al., 2023) [192] |